- 1Laboratoire EMC Etude des Mécanismes Cognitifs - MSH LSE (USR CNRS 2005) - Université Lyon 2, LabEx CORTEX ANR-11-LABX-0042, Université de Lyon, Lyon, France
- 2Association Agir Pour l’Ecole - Paris, Paris, France
- 3Centre de Recherches sur la Cognition et l’Apprentissage (CeRCA), Université de Poitiers, Université de Tours, CNRS, Poitiers, France
- 4Laboratoire Paragraphe, Université de Cergy-Pontoise, Cergy-Pontoise, France
- 5Institut National d’études démographiques (Ined)/Unité mixte Ined/Inserm/EFS Elfe, Paris, France
As part of the French national cohort ELFE, a two-year longitudinal study was conducted and children’s literacy skills were assessed twice (N = 2,767), namely at the end of preschool (t1) and at the end of Grade 1 (t2). At t1, letter-name knowledge (LNK), phonological skills (PhoSk) and vocabulary were assessed and at t2, phonemic segmentation, word reading, listening and reading comprehension. Latent profile analyses conducted at t2 resulted in five profiles, ranging from good readers (48.8%), to average readers above the mean (16.2%), readers with very low phonological awareness skill (8.2%), poor readers with low reading comprehension skill (19.4%), and poor readers with general difficulties (7.4%). Two early literacy skills (LNK and PhoSk) and two environmental variables (parents’ educational level and household income) constituted good predictors of membership of a profile. However, home literacy practices also predicted membership of the good reader/poor reader profiles, albeit at a lower level.
Introduction
Some large-scale studies have examined predictors of reading in the earlier school grades but very few have done so in French-speaking children. This research, aimed to describe the heterogeneity of literacy skills observed at the end of Grade 1 through profiles of learner readers and then to highlight the early literacy skills and the environmental predictors that best contribute to these profiles.
This research, which was conducted within the framework of the French national cohort ELFE1 (Charles et al., 2020), aimed to examine the expected heterogeneity in literacy skills at the end of Grade 1 by defining profiles of learner readers. The second aim of this large-scale study was to identify the best predictors of literacy skills in preschool and examine three environmental factors (home literacy practices, parents’ education and household income) that could better explain interindividual differences. To this end, an integrative model of reading will first be presented as a theoretical framework before we go on to review the main variables used in this study.
The componential model of reading
Instead of using the well-known model of reading, i.e., the Simple View of Reading (SVR; Gough and Tunmer, 1986), which states that reading difficulties (and consequently inter-individual differences) can be explained by two cognitive components, namely decoding and comprehension, we call on a broader model. In fact all reading difficulties could have origins other than cognitive causes and researchers should examine other variables. Working from this perspective, Aaron et al. (2008) present an integrative model with three domains: The cognitive domain subsumes the SVR, the psychological domain includes components such as motivation and interest, learning styles, teacher expectation, and gender differences, and the ecological domain includes the components of home environment and culture, parental involvement, classroom environment, dialect, and home language other than that used at school. For the authors, “the CMR envisages that a child can fail to acquire satisfactory levels of literacy skills because of deficits in any component in any one of these three domains” (p. 69).
In the present study, we will examine how some components in both the cognitive and ecological domains could explain the individual differences in literacy skills and reading level. For the cognitive domain, we will attempt to identify the extent to which early literacy skills (code-related and meaning-related) are predictive of reading ability. For the ecological domain, two components, namely home literacy practices and parental socio-economic status, will be taken into account in order to explain reading ability.
Early literacy skills as predictors of reading skills
Numerous studies have shown that primary reading skills are predicted by the literacy skills of preschool children2 (e.g. Lonigan et al., 2000; Storch and Whitehurst, 2002; Kim and Petscher, 2011; Munger and Blachman, 2013; Ozernov-Palchik et al., 2017). This finding suggests that the foundations of reading skills are established in the early childhood years (Whitehurst and Lonigan, 2001; Lonigan, 2004; Scarborough, 2009). Reading requires the interaction of multiple skills, including word decoding, word meaning (i.e., vocabulary) and listening comprehension skills (Tunmer and Chapman, 2012). Storch and Whitehurst (2002) evaluated the linguistic and cognitive skills predictive of the future reading achievement of Head Start children from preschool to Grade 4. The structural equation model shows that emergent literacy skills can be separated into two distinct and interrelated domains: oral language and code-related skills. Moreover, the strength of the relationships between the two domains and reading components (word decoding or reading comprehension) may change during the course of development. Concerning reading comprehension, studies have revealed that the key predictors are word decoding and oral language, including vocabulary in Grades 2 (Kendeou et al., 2009; Papadimitriou and Vlachos, 2014) and 3 and 4 (Storch and Whitehurst, 2002). Kindergarten measures of vocabulary knowledge predicted Grade 1 listening comprehension, reading fluency and reading comprehension (Hulme et al., 2012; Torppa et al., 2016).
In a review of 61 studies, Scarborough (2001) indicates that code-related skills such as PA and letter-name knowledge (LNK) in kindergarten are the skills most highly associated with subsequent word reading accuracy in Grade 2. LNK and PA in kindergarten explain more than 50% of the variance in word reading in Grade 1 (Lonigan et al., 2000) and Grade 2 (Piquard-Kipffer and Sprenger-Charolles, 2013). Papadimitriou and Vlachos (2014) evaluated PA, RAN, phonological short-term memory, auditory processing, motor skills and oral language in kindergarten and reading in Grades 1 and 2. Results showed that PA, and to a lesser extent phonological short-term memory, contribute to explaining the variation in reading accuracy performance. Moreover, in pre-kindergarten (Furnes and Samuelsson, 2009) and kindergarten (Morris et al., 2003; Foulin, 2005), LNK is a good predictor of success in word recognition in first grade. Hulme et al. (2012) and Torppa et al. (2016) found that PA, LNK, and RAN measured in kindergarten predicted reading fluency, but not listening comprehension, in first grade. In a longitudinal study (Powell and Atkinson, 2021), a sample of very young English children (3 years 11 months) were initially assessed on RAN and PA. They were then reassessed on RAN, PA, and word-level reading 18 months later (Time 2) and then once again after another year (Time 3). Analyses showed that both RAN and PA predicted the development of word reading between Time1 and Time2. At Time 3, RAN still predicted reading accuracy and fluency, while PA only predicted reading accuracy.
Most studies have been conducted in English. However, alphabetic orthographies vary in the degree to which they are regular in their representation of sound. We distinguish between transparent or shallow orthographies, in which sound-symbol matches are very consistent, and opaque or deep orthographies which are less consistent because each letter or group of letters can represent different sounds in different words. Ziegler et al. (2010) investigated the role of phonological awareness, memory, vocabulary, rapid naming, and nonverbal intelligence in reading performance across five languages located at differing positions along a transparency continuum (Finnish, Hungarian, Dutch, Portuguese, and French). They showed that the predictors of reading performance in alphabetic languages are relatively universal, although their precise weight varies systematically as a function of orthographic transparency. Similarly, Caravolas et al. (2019) examined the longitudinal influences of the precursors of word reading ability (phoneme awareness, letter knowledge, rapid naming) and of oral language skills on individual differences in decoding and reading comprehension in children learning to read in English, Spanish, Czech, and Slovak. They showed that in all four languages, early variations in phoneme awareness, letter knowledge, RAN and emerging decoding skills predicted variations in decoding skills at the end of Grade 1. For the three consistent orthographies (Spanish, Czech, and Slovak), kindergarten language skills were a significant predictor of reading comprehension in Grade 2. This last result confirm that early oral language skills directly influence both emergent decoding skills and reading comprehension. In French, very few studies have been conducted in children starting from before formal reading instruction. Piquard-Kipffer and Sprenger-Charolles (2013) performed a follow-up study of French-speaking children from the beginning of kindergarten to the end of second grade and showed that a high proportion of the variance in reading at age 8 (52.8%) was predicted by pre-reading level, letter-name knowledge, phonemic segmentation and phonological short-term memory assessed at age 5. In summary, numerous studies have shown the importance of specific emergent literacy skills for later successful reading development. Among these early literacy skills, three variables have been considered important in identifying children at risk of reading delay: PA, LNK and vocabulary. Our study will also explore how the environmental variables of home literacy activities and the socio-economic status could impact reading skills.
Environmental predictors of reading skills
Effects of home literacy practices
Home literacy environment (HLE) includes general aspects of family literacy such as parental reading habits, the number of books in a household, parental involvement in their children’s literacy activities, the parental attitudes toward shared reading, parents’ literacy-related attitudes. Socioeconomic status-related factors of the family environment such as parental years of school education, also called parental education level, family possessions and parent-to-child speech are also a part of HLE. Thus, HLE is a complex, multifaceted concept and its multidimensional nature has led to inconsistent results regarding the relationship between early language and HLE (Dodici et al., 2003; Fish and Pinkerman, 2003; Umek et al., 2005; Deckner et al., 2006). Moreover, the relative contributions of different HLE factors (parents’ literacy-related attitudes, parental education level, parental literacy involvement, and home literacy resources) to reading acquisition across grades remain unclear (for example, see the meta-analysis of Dong et al., 2020). In all recent studies (for example, Lehrl et al., 2020), the separation of HLE process indicators (i.e., reading books) and socio-demographic (i.e., parental education level) indicators takes into account findings from previous studies which suggest that the activities parents perform with their children are more important than their socio-economic status. Recently, Dong et al. (2020) showed that parental involvement in their children’s literacy activities has a strong relationship to children’s reading. In the same way, many studies have shown that home literacy practices (HLP) are likely to improve the development of oral language, reading and writing (Frijters et al., 2000; Burgess et al., 2002; Roberts et al., 2005; Bracken and Fischel, 2008; Sénéchal and LeFevre, 2014; Sénéchal et al., 2017; Tamis-LeMonda et al., 2019). HLP is defined as the various literacy activities that take place at home and includes reading practices (for example, parent–child joint reading, parent teaching of letter names), writing practices (parent–child joint writing activities, parent teaching in writing names or other words) and more general aspects of family literacy such as the frequency of library visits.
The assessment of HLP has evolved over the years. Different inventories are used to evaluate all the aspects of HLP (see Burgess et al., 2002; Umek et al., 2005; Schmitt et al., 2011). Generally, HLP is measured by means of self-report surveys. A current review indicates that children often exposed to shared book reading have more vocabulary and better language skills than children with less frequent exposure to shared book reading (Zauche et al., 2016).
In their home literacy model (Sénéchal et al., 1998), the authors distinguish between two types of HLP, one that focuses on phonological and orthographic knowledges (termed “formal” or “code focused”) and another, pleasure-oriented approach that focuses on sharing the meaning of oral or written language (called “informal” or “meaning-oriented”). Evidence for this model has been found in different orthographies, such as English (Sénéchal and LeFevre, 2014), French (Sénéchal, 2006), Greek (Manolitsis et al., 2011, 2013), German (Rose et al., 2018), and Finnish (Silinskas et al., 2012).
Sénéchal and Lefevre (2014) have shown that parents adapt the frequency with which they teach letters and words to their children according to their child’s progress, thus improving support where progress is slower than expected. Additionally, when young children have begun to acquire the alphabetic principle, shared storybook reading can facilitate the acquisition of emerging code-based literacy skills (Mol and Bus, 2011). Such findings could explain contradictions in the literature, which sometimes reports positive effects between parental education and children’s literacy skills (Evans et al., 2000; Sénéchal and LeFevre, 2002; Hood et al., 2008) and sometimes negative effects (Silinskas et al., 2012; Sénéchal and LeFevre, 2014). Moreover, cultural differences in home literacy practices and literacy habits increase the diversity of developmental trajectories in children’s language and literacy skills (i.e., Phillips and Lonigan, 2009; Evans et al., 2010).
In our study, we will distinguish between HLP, on the one hand, and what constitutes socioeconomic status, on the other. We will then calculate an overall score including different activities such as shared book reading, shared drawing activities, shared singing activities, copying letters, thus providing an indicator of HLP.
Effects of parental socioeconomic status: household income level and parental education level
Parental socioeconomic status (SES), generally indicated by household income and parental education, impacts children’s literacy skills (Huttenlocher et al., 2010; Fernald et al., 2013; Gonzalez et al., 2017; Pace et al., 2017). SES is not only linked to children’s language skills but also to the type of parental speech addressed to a child at home. Several studies show that parents with a higher SES use more words, a richer vocabulary and some grammatically more complex utterances when interacting with their children than parents with a low SES (Bernstein, 1973; Rowe, 2008; Rowe et al., 2012). Moreover, studies have shown that children from families with low SES tend to have fewer books and read less frequently than those from higher SES families (Burgess et al., 2002; Crosnoe et al., 2010; Niklas et al., 2020); this difference results in higher performance in vocabulary, oral language and emergent literacy skills in children from higher SES families (Mol and Bus, 2011; Farver et al., 2013; Froiland et al., 2014). Several authors have shown that parents who have a high education level provide a family environment enriched in literacy, have more positive attitudes toward shared reading and therefore have a positive influence on the development of their children’s language and literacy skills (Lonigan, 2004; Umek et al., 2005).
In summary, in many studies, the family’s SES is considered to comprise both the educational level of the parents and the family income. It affects children’s language development and the acquisition of literacy skills. Few studies have made direct comparisons of the unique roles of parents’ education and household income. However, some authors (i.e., Davis-Keane, 2005; Zheng and Libertus, 2018) suggest that parents’ education and household income play different roles in determining parents’ beliefs about the importance of the school and parenting behavior at home in teaching math or reading/writing to their child. Using Finnish register data, Erola et al. (2016) compare the contribution of parents’ education, occupational class and income observed at different ages during childhood and youth to children’s adult socioeconomic status. Parental status indicators were observed during five periods of their children’s development 0–4, 5–9, 10–14, 15–19, 20–24 and then again in adulthood at 25–29 for 29,282 children. The results indicate that parental education level explains children’s occupation most and parental income least. The authors point out that the most unexpected result concerns the importance of income: contrary to what is often expected, the independent effect of income during early childhood was non-existent.
We will distinguish these two variables and will examine on the one hand, the role of the level of household income and, on the other, the role of the level of parental education.
Examining heterogeneity in literacy skills
Some studies have highlighted the different level of performance in reading and reading-related skills from preschool to the early primary grades. Using K-means cluster analysis, Cabell et al. (2011) distinguished five profiles in preschoolers (N = 492) based on various data collected for receptive and expressive grammar, receptive and expressive vocabulary, and code-related skills. Boscardin et al. (2008) followed 411 children from kindergarten to Grade 2 assessed four times per year for PA and word recognition (WR). Growth mixture modeling analyses revealed five profiles (Pr) in kindergarten, going from children in Pr1 with the lowest PA scores (with no growth during the year) to those with the highest phonological awareness scores (Pr5), with the scores increasing from Pr2 to Pr5 and during the year for each profile. Once again, in Grades 1 and 2, five profiles were observed, going from the profile with the poorest word reading scores (Pr1 with no growth during 2 years) to the four profiles which all exhibited progress during these 2 years, with scores increasing from Pr2 to Pr5.
In subsequent studies, latent profiles analyses (LPA) were run to discover what lies behind this high level of heterogeneity. LPA aims to determine a number of subgroups/profiles in a population and estimate the probability of membership of each profile by analyzing different configurations of many variables (see Spurk et al., 2020). In the first part of their large-scale study concerning data from kindergarten to Grade 2 (N = 2,295), Foorman et al. (2017) highlighted six profiles in Kg and G2 and five profiles in G1 obtained from data from computer-based assessments, mainly, phonological awareness (PA), letter-sound knowledge (LSK), sentence comprehension, word reading, spelling. Two other studies investigated whether low performance profiles are related to reading difficulties. In a study involving only first graders (N = 290), Grimm et al. (2018) highlighted two at-risk groups with low scores in reading tasks and three profiles for higher performances (without risk) differentiated on PA and decoding. At the same time, Ozernov-Palchik et al. (2017) succeeded in predicting reading difficulties in Grade 1 on the basis of data obtained at the beginning of kindergarten (N = 1,215). Six profiles were revealed in the light of the scores in PA, RAN, LSK, and verbal short-term memory measures. The authors also observed a higher frequency of PA and RAN deficits in children from lower SES backgrounds.
While these previous studies were carried out with English-speaking students, the two described next concern three languages, German, Finnish and Dutch. In the Dutch study, designed to identify children at risk, Verwimp et al. (2020) examined the variability of pre-reading skills in kindergartners (N = 1,091). After a latent profile analysis on scores in PA, RAN, LNK, and IQ, they obtained four profiles, with the two profiles with the lowest scores being distinguished by IQ, having either average (25.6%) or below-average IQ (17.7%). In a longitudinal study (Holopainen et al., 2020), 445 German-speaking children and 324 Finnish children performed several reading tasks in two sessions, one in G1 and the other in G2. Classical tasks were used to investigate PA, LSK, WR, fluency and reading comprehension (at sentence level). Four profiles were revealed in the two languages and RAN distinguished well between all profiles in Finnish, while LSK did so only in German.
The present study conducted in French should help complete the cross-linguistic investigation of the heterogeneity of reading performance observed at the end of G1 and of the predictors (early literacy skills and environmental variables) which might be associated with this.
Research questions
Beyond the very great heterogeneity generally observed in all studies on reading performance, the aim here is to highlight groups that have similar performance levels in literacy skills (that is, reading and associated reading skills) at the end of Grade 1. Two sets of predictors will then be examined to explain the difference between groups, literacy variables observed 2 years previously (in preschool) and environmental variables concerning home literacy practices, parents’ educational level and household income. To summarize, two mains research questions will be addressed:
RQ1 - What are the profiles of learner readers at the end of G1? We expected the distribution of literacy skills in G1 to be heterogeneous. In other words, low literacy skills did not necessarily imply weakness in other skills.
RQ2 - For each profile, what are their predictors observed earlier in different surveys and literacy skills assessments? More specifically what are the main predictors related to the observed profiles? Because what a child learns at home is important and associated with the parents’ education level and their expectations about the academic achievement of their child, we expected that this environmental variable would be one of the most powerful predictors.
Method
Participants
A set of data was extracted from the French national cohort of children ELFE (for a presentation of this cohort,3 see Charles et al., 2020). In this study, data concerning different variables were collected via interviews with parents at 1 year (when their child was 1 year-old) and at 3.5 years, and at school from the children. The children performed various (literacy and numeracy) tasks in booklets in response to the instructions given by their teachers in preschool (t1) and then 2 years later in first grade (t2); (then descriptive data are presented in Table 1 and correlations matrix in Table 2). Children with a disability status established by a psychologist or recognized by the teacher were not included in this sample. Finally, this longitudinal study is based on data collected from parent interviews and from 2,767 children (1,182 boys, 1,207 girls; 378 not specified; mean age (t1) = 57.3 months; sd = 2.88) with no missing literacy skills data for either session. However, not all the environmental data are available for each child. To ensure that the 129 children for whom we had no socio-economic data were comparable to the other 2,638 children in the analysis of the influence of environmental variables, we conducted means comparisons tests on cognitive variables at age 4, and literacy variables at age 6. The results show that the 129 children differed significantly only on listening comprehension (LC) at age 6 (see Appendix B). We examined the distribution of these 129 children and the 5 SES categories across the 5 profiles (see Appendix C) and found that these children are similarly distributed across the profiles. This suggests that the data for 129 children and the 2,638 children are comparable.
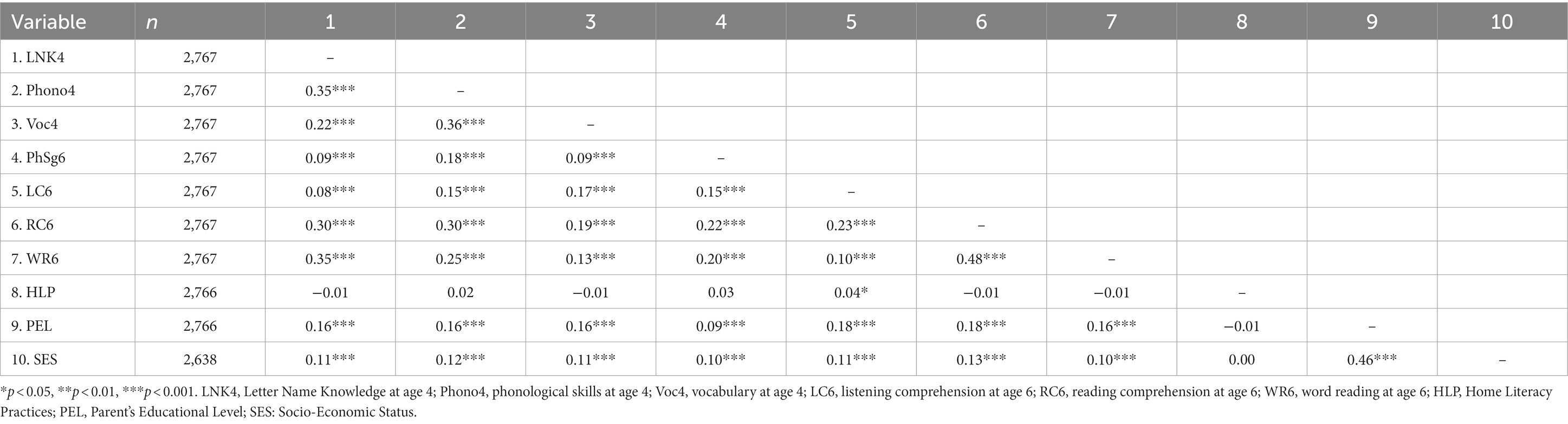
Table 1. Matrix of Spearman’s correlations between raw scores of literacy skills and environmental variables.
Literacy skills
All the tasks were administered collectively by the teachers in small groups (maximum four children) with the cohort of ELFE children.4 These literacy skills were assessed twice, in preschool (April–May 2016; t1) and in Grade 1 (April–May 2018; t2). All these tasks had already been used in previous studies (Ecalle et al., 2015, 2019).
Letter-name knowledge (t1). The children were asked to circle the letter named by the teacher out of a choice of seven (max = 10).
Phonological skills (t1). Two tasks were administered. In the oddity task, the teacher named three pictures and the children had to circle the one which did not share a common unit, i.e., a syllable or phoneme (max = 9) e.g., with a common syllable: toupie–balai–bateau (spinner top–broom–boat). In the syllable deletion task, the teacher named all the pictures, i.e., a first word [e. g., bijou (jewel) and then the four test words joue (cheek), genou (knee), couronne (crown), cœur (heart)]. The children had to retrieve a new pictured word (joue) after having deleted the first syllable of the first word (max = 6). A global score was measured (max = 15).
Vocabulary (t1). In a classic receptive vocabulary task, the teacher named a word and the children were then asked to circle the picture corresponding to the named word out of a choice of four (max = 10).
Phonemic awareness (t2). In a phonemic segmentation task, the children had to draw as many circles as the number of phonemes heard in a pseudoword spoken by the teacher (max = 10).
Word reading (t2). The teacher named the pictured word (e. g., mouton; sheep) and the children were asked to circle the written word that corresponded to the picture out of a choice of five test items consisting of words (mouton; mouture) and pseudowords (mauton; moutton; mtonou) (max = 14).
Listening comprehension (t2). A short narrative text (136 words) was read twice. The teacher then asked 10 questions in succession. The children had to circle the picture which corresponded to their answers out of a choice of four test items (max = 9).
Reading comprehension (t2). The children read a narrative text (111 words) silently and on their own and then had to answer the written questions by circling their response out of a choice of three items. They were told that if they did not know the response they could circle “I do not know” (max = 12).
Environmental variables
These variables were collected during a phone interview with mothers and fathers at 1 year (1y) and then at 3.5 years with the referent parent only (3y).
Home literacy practices (HLP; 1y; 3y). This variable was constructed from answers to the questions proposed during the phone interview about the parent–child activities which could contribute to the quality of HLP. At 1 year, three questions were asked separately to the mother and father about shared book reading, shared drawing activities and shared singing activities: often (indicated by 1), from time to time (2) and rarely or never (3). The responses for each parent were taken into account. Later, during the phone interview at 3 years, four questions were asked to the referent parent only: Do you tell stories to your child? Do you sing or listen to music with him/her? Do you re-read books to him/her? Do you copy letters or words with him/her? Yes was indicated by 1, no by 2. We calculated a global score with both the 1y-total and 3y-total scores. If HLP data were obtained only at 3y and not at 1y, we retained them; but if HLP data were provided only at 1y but not at 3y, we discarded them. Finally, in order to estimate the quality of HLP, we calculated an inverse score from the raw scores so that the low scores corresponded to poor HLP and the high scores to rich HLP (m = 6.19; sd = 1.4; range: 4–14.3). Pairwise correlations indicate significant coefficients between the mother’s and father’s HLP scores at 1 year (r = 0.26), between the 1y-mother scores and 3y-referent scores (0.24) and between the 1y-father scores and 3y-referent scores (0.14).
Parents’ educational level (PEL; 1y). The International Standard Classification of Education (ISCED; UNESCO, 2012) was used to classify the educational levels of the mother and father separately. We considered the highest educational level out of that of the mother and father. We distinguished five levels, from lower secondary general education (A corresponding to ISCED 2; n = 13), to upper secondary general education (B; ISCED 3; n = 116), to bachelor’s degree (C; ISCED 6; n = 318), then 2 years after bachelor’s degree (D; ISCED: 7; n = 639) and finally more than 2 years after bachelor’s degree (E; ISCED: 8; n = 1,680).
Household income (HInc; 3y). Based on the global income provided by the referent parent and the number of members living in the household dwelling (and their ages), it was possible to calculate a more specific level of HInc, with income being weighted by the number of consumption units for each household that included an ELFE child. In the light of this income, each child was assigned to a household income, from the lowest (A) to the highest (E), based on a quintile distribution calculated from all families in the ELFE cohort (see Appendix D).
Results
Data analyses
After the presentation of the descriptive data, analyses were conducted with Mplus Version 8.3 (Muthén and Muthén, 1998/2017). We used the automatic three-step approach (R3STEP command in Mplus; Vermunt, 2010; Asparouhov and Muthén, 2014). The first and second steps made it possible to obtain the number of profiles and the most likely class membership, while the third step allowed us to examine the antecedents of the profiles using multinomial logistic regression analyses.
To answer the first research question, we analyzed the children’s reading profiles at the end of Grade 1 based on their literacy skills: phonemic awareness (PA), word reading (WR), listening comprehension (LC), and reading comprehension (RC). We tested Latent Profile Analysis (LPA) models with different numbers of classes using the Mixture Modeling Method. We used robust maximum likelihood estimation (MLR) because the data did not follow a normal distribution. Means were allowed to be different across classes and residual variance was allowed to be equal but freely estimated. We then compared the fits of these models. The selection of the optimal number of profiles was based on a consideration of the theoretical meaning of the profiles, and the need to obtain a statistically satisfactory solution (see Spurk et al., 2020). Different criteria were applied to evaluate the fit of the LPA model and to select the best reading profile models:
1. Information criteria: Akaike Information Criteria (AIC), Bayesian Information Criteria (BIC), and Sample-sized Adjusted Bayesian Information Criteria (SSBIC). The model with the smallest values was considered the best choice.
2. Entropy: values close to one indicate good classification accuracy.
3. Statistical tests: Parametric bootstrap likelihood ratio difference (BLR), Vuong-Lo–Mendell–Rubin (VLMR) and Adjusted Lo–Mendell–Rubin (A-LMR). With regard to the relative fit of the model, the significance (p-values) < 0.05 indicates that a model with an additional profile can be tested.
4. The percentage of participants in the profiles (> or = 5%).
To answer the second research question, we used the R3STEP command in Mplus to perform a series of multinomial logistic regressions to assess whether an increase in antecedent would result in a higher probability of belonging to one class compared to another. Our antecedents had some missing data. In the R3STEP procedure, the analyses use listwise deletion. As a result, tests of our antecedents were performed with the 2,638 participants for whom we had all four environmental variables and with the 2,767 participants for the literacy skills variables (see Appendix E). We conducted two separate analyses per antecedent variable: one for early literacy skills variables, i.e., letter-name knowledge (LNK), phonological skills (PHO), and vocabulary (VOC), and one for environmental variables, i.e., home literacy practices (HLP), parental education level (PEL), and household income (HInc).
Descriptive data and correlations matrix
The first are presented in Table 2 and the second in Table 1.
Learner reader profiles
Table 3 provides the fit statistics for possible latent profile structures. From the 6-profile structure, one of the classes represents a proportion of less than 0.006 of the sample, which is not enough to form a group (about 10 participants). We therefore stopped at a 5-profile structure. Other arguments led us to choose the 5-profile solution because it has lower LL, AIC, BIC, and SSA-BIC than the 4-profile solution and a higher entropy index. The non-significant LL, A-LMR and BLRT values compared to the 4-profile values indicate that the 5-profile structure does not differ from the 4-profile structure.
Figure 1 illustrates the selected 5-profile structure. Table 4 indicates the number and percentage of children for each learner-reader profile, as well as the percentage probability of children belonging to one or more profiles.
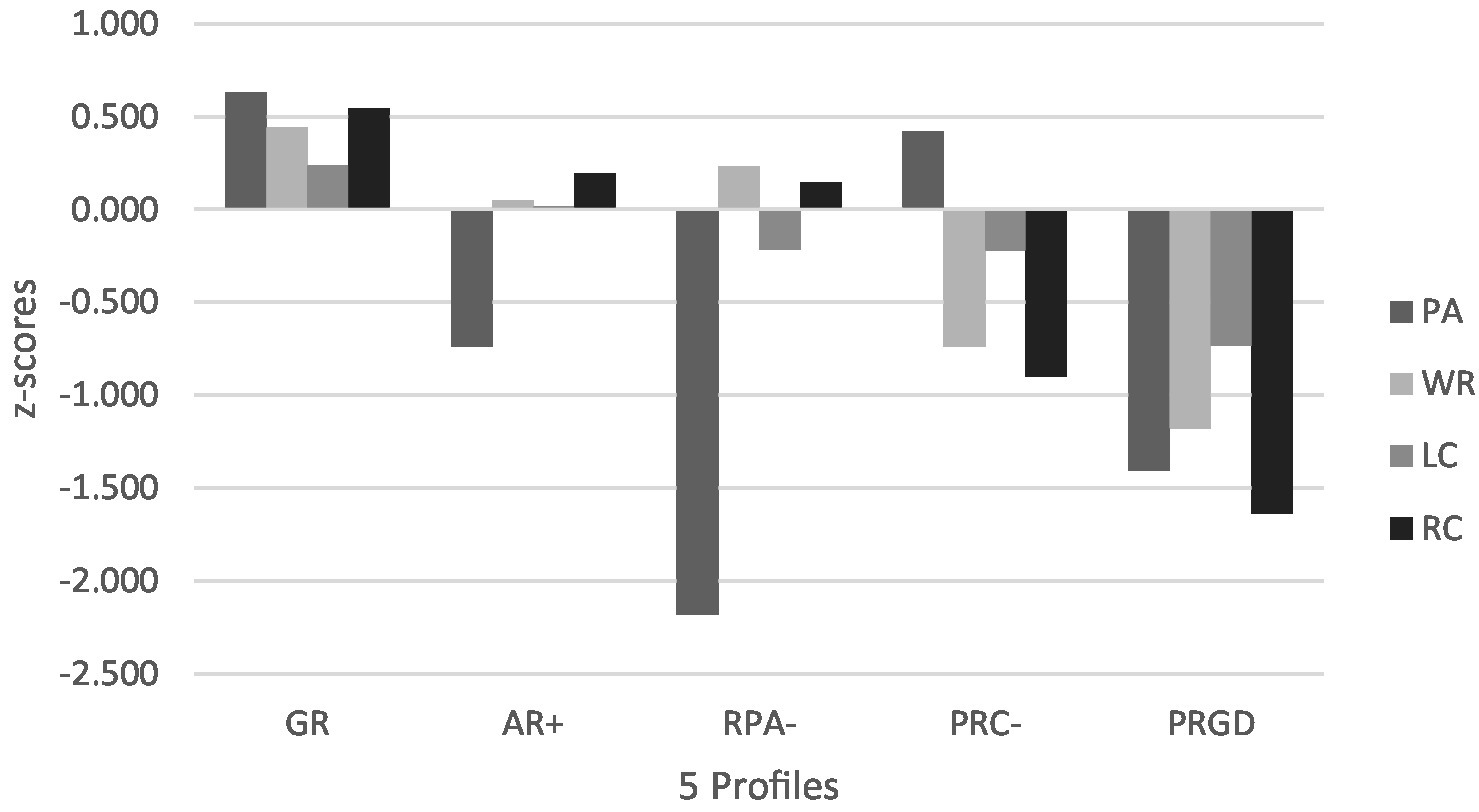
Figure 1. Mean standardized scores for phonemic awareness (PA), word reading (WR), listening comprehension (LC), and reading comprehension (RC) according to the 5 profiles. GR, Good Readers; AR+, Average Readers above the mean; RPA-, Readers with a low phonological skill; PRC-, Poor Readers with a low reading Comprehension skill; PRGD, Poor Reader with General Difficulties.
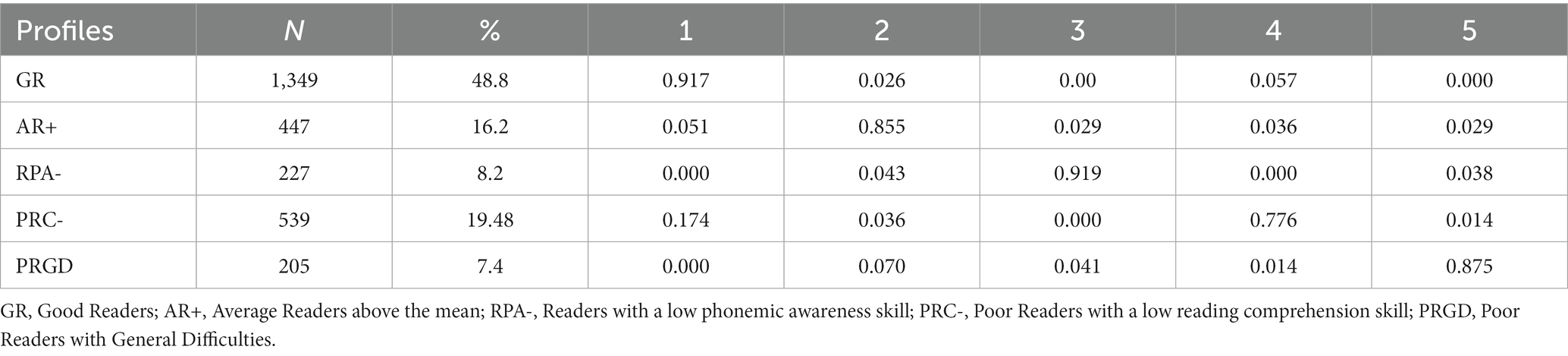
Table 4. Number (N) and percentage of children (%) in each profile, and probability of profile membership.
Profile 1: Good Readers (GR, proportion: 48.8%). This profile includes the most children. They have the highest scores for all the literacy variables. It therefore corresponds to children with strong reading and listening comprehension and strong phonemic awareness and word reading skills.
Profile 2: Average Readers above the mean (AR+, proportion: 16.2%). This profile seems to correspond to children with listening, reading comprehension and word reading skills around the mean, but with a lower phonemic awareness (just below the mean).
Profile 3: Readers with a very low Phonological Awareness skill (RPA-, proportion: 8.2%). The phonological awareness score is the lowest in this profile. The reading, listening comprehension and word reading scores did not differ significantly from those of the AR+ profile. It therefore appears to correspond to children with low phonemic awareness, but with average skills in listening, reading comprehension and word reading skills.
Profile 4: Poor Readers with low reading Comprehension skill (PRC-; proportion: 19.48%). The scores for phonemic awareness are unexpectedly high in comparison to the low scores in word reading, listening comprehension, and more specifically in reading comprehension, which represents the lowest score for this profile.
Profile 5: Poor Readers with General Difficulties (PRGD; proportion: 7.4%). This profile has the lowest scores in listening, reading comprehension and word reading skills. It has a lower phonemic awareness score than the profiles 1,2, 4 and therefore corresponds to the children with the poorest overall skills.
Wald’s tests of two-by-two mean comparisons were performed to specify the differences between each profile on the different literacy variables at 6 y-o. Only a few differences were not significant. First, listening comprehension scores did not differ between the AR+ and the RPA- profiles (Wald χ2 = 3.299, df = 1, p = 0.072), between the RPA- and the PRGD profiles (Wald χ2 = 3.71, df = 1, p = 0.054), between the RPA- and the PRC- (Wald χ2 = 0.001, df = 1, p = 0.973). Second, reading comprehension scores did not differ significantly between the AR+ and the RPA- profiles (Wald χ2 = 0.085, df = 1, p = 0.77). Finally, word recognition scores were not significantly different between the AR+ and the RPA- profiles (Wald χ2 = 1.77, df = 1, p = 0.183), or between the AR+ and the GR profiles (Wald χ2 = 3.18, df = 1, p = 0.074).
Relationships between early literacy skills and children’s learner-reader profiles
Figure 2 illustrates the mean standardized literacy skills scores at age 4 (i.e., LNK, PHO, VOC) according to the five learner-reader profiles. The results of multinomial logistic regressions conducted to assess whether an increase in one antecedent variable would result in a higher probability of belonging to one profile compared to the others (see Table 5).
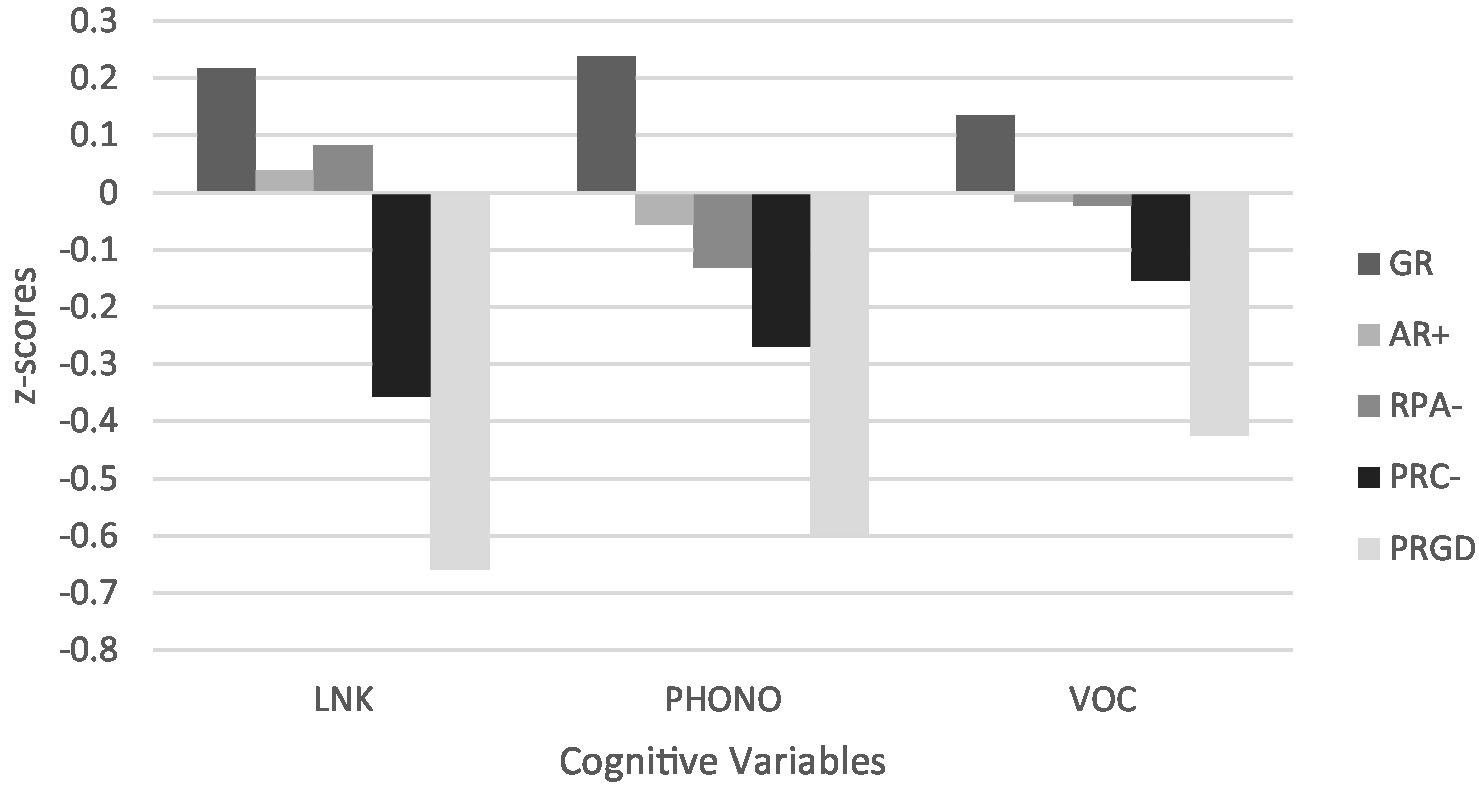
Figure 2. Mean standardized scores on literacy skills at age 4 according to the learner-reader profiles. LNK, letter name knowledge; PHONO, phonological skills; VOC, vocabulary; GR, Good Readers; AR+, Average Readers above the mean; RPA-, Readers with a low phonological awareness skill; PRC, Poor Readers with a low reading Comprehension skill; PRGD, Poor Reader with General Difficulties.
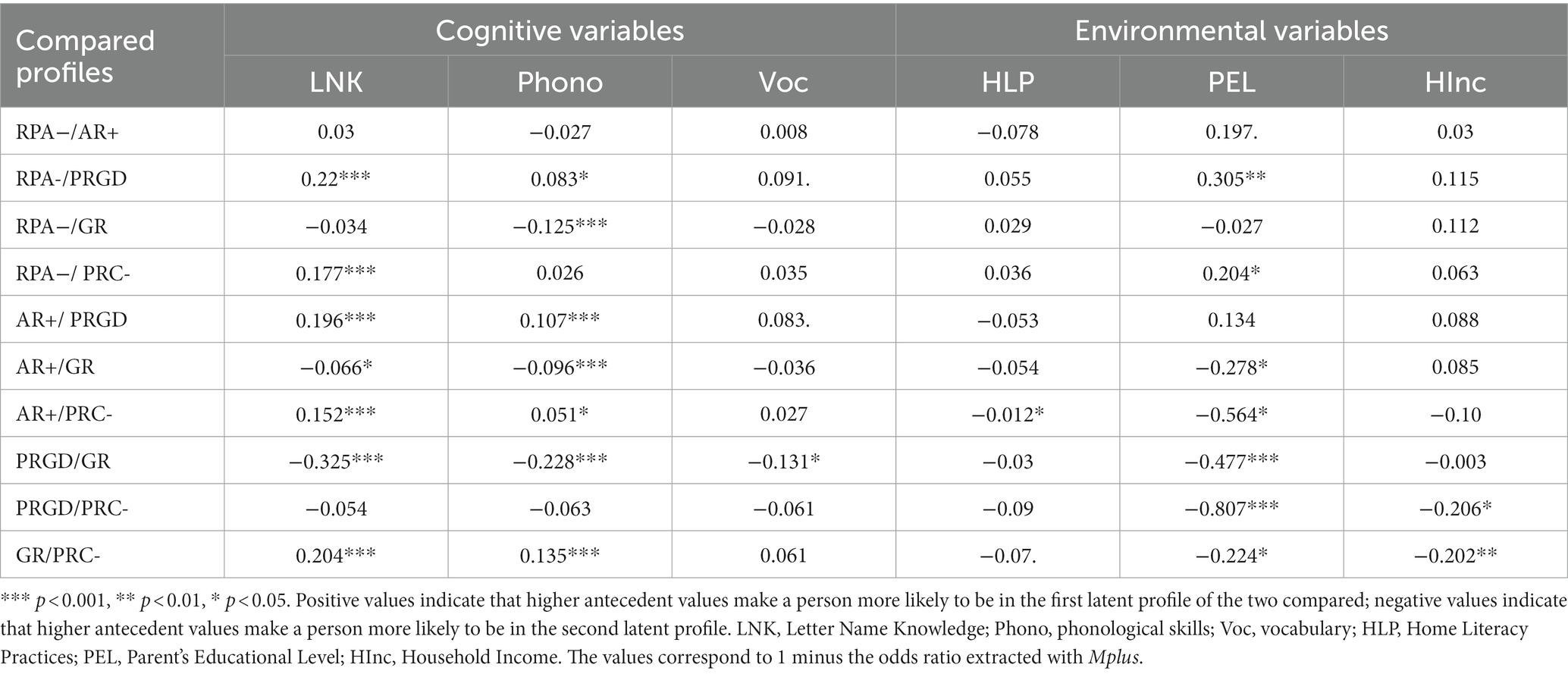
Table 5. Results from the multinomial logistic regression using the three-steps approach for cognitive and environmental variables.
The results of the pairwise profile comparisons suggest that when a literacy variable has a significant effect on the probability of belonging to one of the profiles, a higher score on the letter knowledge, phonological skills, and vocabulary tasks makes a child likely to belong to the profile of learner-readers with the highest skills. For instance (Table 5), a higher phonological skill score at age 4 makes a child 12.5% more likely to belong to the GR profile than to the RPA- profile (p < 0.001). Letter name knowledge and phonological skills were the variables that influenced profile membership the most, except for 3 profile comparisons (i.e., RPA−/AR+, RPA−/GR, PRGD/PRC-; see Table 5). Only one significant difference was found in the form of vocabulary: a higher vocabulary score makes children 13.1% more likely to belong to the GR than the PRGD profile.
To go further, pairwise comparisons of z-score means were performed on cognitive variables at age 4 and environmental variables for each of the 5 profiles of learner readers at age 6 (see Appendix A). The results show, for example, that vocabulary is a variable in literacy that differentiates well between the PRGD profile (the one with the most difficulties) and the other profiles at age 4.
Relationships between environmental variables and children’s learner-reader profiles
The multinomial logistic regression analyses assessed whether an increase in one antecedent environmental variable would result in a higher probability of belonging to one profile over another. Figure 3 illustrates the mean standardized scores on the environmental variables (HLP, PEL, HInc) according to the five learner-reader profiles. The results of the pairwise profile comparisons suggest that when an environmental variable has a significant effect on the probability of belonging to one of the profiles, a higher score on home literacy practices (HLP), parent’s educational level (PEL), and household income (HInc) makes a child more likely to belong to the profile of learner-reader with the highest skills. For example (Table 5), a higher PEL makes a child 30.5% more likely to belong to the RPA- than the PRGD profile (p < 0.001). PEL and HInc are the variables that best allow us to determine profile membership. HInc has a greater influence on membership of the GR profile. Finally, a higher HLP score makes a child 12% more likely to belong to the PRC- than the AR+ profile (p < 0.01).
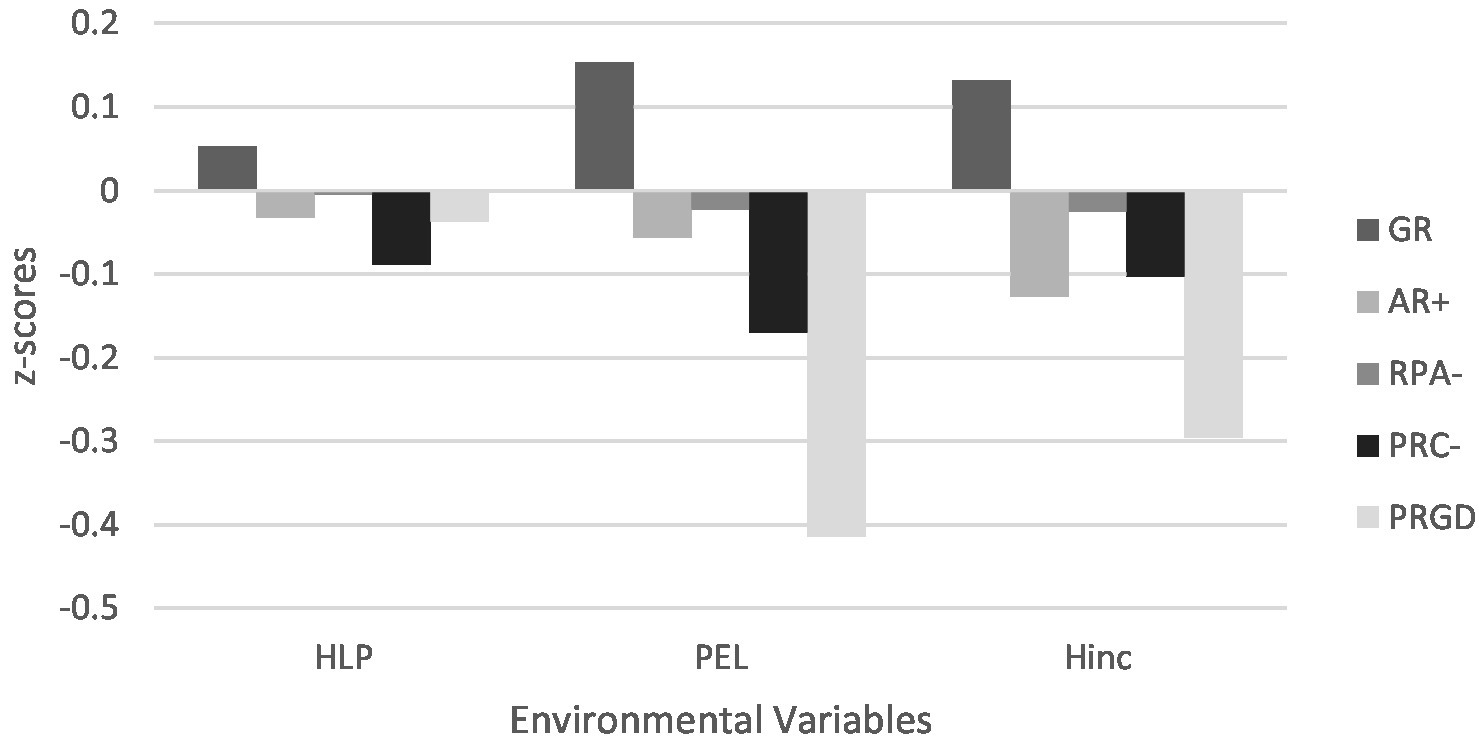
Figure 3. Mean standardized scores on environmental variables according to the learner-reader profiles. HLP, Home Literacy Practices; PEL, Parent’s Educational Level; HInc, Household Income; GR, Good Readers; AR+, Average Readers above the mean; RPA-, Readers with a low phonological awareness skill; PRC-, Poor Readers with a low reading Comprehension skill; PRGD, Poor Reader with General Difficulties.
To go further (see Appendix A), PEL is the variable that differentiates better each profile from other profiles at a significant level, whereas HInc is the variable that differentiates only GR from other profiles.
Discussion
Reading ability and its predictors in the light of componential model of reading
The integrative model CMR emphasizes three domains explaining reading difficulties and, consequently, interindividual differences which have very often been documented in the literature. Two domains were examined in the present study; cognitive and ecological (renamed here environmental). We sought to determine the nature of the learner reader profiles at the end of G1 and, on the other, to examine the impact of early literacy skills and environmental variables on these profiles. The main result can be summarized as follow. First, we extracted five profiles of learner readers at the end of first grade, differing according to their scores in phonemic awareness, word reading, listening comprehension, and reading comprehension. Second, we identified a number of early literacy skills and environmental variables that appear to determine the probability of membership of one profile rather than another. We observed that higher scores in letter-name knowledge and phonological skills at age 4 increase the probability of membership of a profile with higher literacy skills at age 6. Furthermore, a higher vocabulary score increases the probability of belonging to a GR rather than a PRGD profile. Moreover, parents’ educational level (PEL) is the variable that best predicts membership of a profile, followed by the household income (HInc). Higher PEL and HInc scores increase the probability of belonging to a profile with higher literacy skills at age 6. Finally, higher home literacy practices (HLP) scores only predicted the membership to PRC- compared to AR+. The negative value is unexpectedly in favor of PRC-.
For the literacy skills, we therefore confirmed what has been found many times before (Foulin, 2005; Furnes and Samuelsson, 2009), namely that letter-name knowledge and phonological skills as code-related skills appear to be the two main early predictors of reading ability. However, we expected that vocabulary, as a meaning-related skill, would be a better predictor of different reader profiles than was actually observed. Indeed, vocabulary has been found to be associated with word recognition and reading comprehension (Verhoeven et al., 2011; Suggate et al., 2018). Perfetti and Stafura (2014) emphasize the core role of vocabulary as an element involved in the cognitive components of reading.
Beyond the well-known heterogeneity observed in literacy skills at the end of Grade 1, we were able to highlight five distinct subgroups using latent profile analysis. These results are compatible with those already obtained in other research. In fact, the majority of studies with English-speaking children have identified five profiles in preschoolers (Cabell et al., 2011), in children from kindergarten to Grade 2 (Boscardin et al., 2008) and in Grade 1 (Foorman et al., 2017; Grimm et al., 2018). However, only four profiles have been identified in other languages, namely in Dutch with children in kindergarten (Verwimp et al., 2020) and in German and Finnish in Grade 1 and Grade 2 children (Holopainen et al., 2020). We have no hypothesis about this small difference (4 or 5 profiles) and how the type of language might influence the number of profiles. It will be necessary to collect further similar data to explain these differences and possibly examine how the analytical techniques used might also impact the results.
Finally, the literacy skills in first grade cannot be reduced to high literacy level and low literacy level and a latent profile analysis is a good way to examine the considerable heterogeneity observed in literacy skills at the end of Grade 1 which, in France, is 1 year after children have been taught to read.
Literacy skills in primary schools are dependent on early literacy skills and on varying environmental experiences and we could consider that interactions between these intrinsic and extrinsic variables contribute to literacy development. Empirical research suggests that when the home literacy environment is richer, children may have stronger literacy abilities. In fact, it is well known that shared book reading and shared writing activities are directly associated with the development of emergent literacy. However, like other authors (Einarsdottir et al., 2009; Puranik et al., 2018), we consider that shared singing and drawing activities might also contribute to creating a home learning environment in which the child’s language and, more generally, his/her literacy skills are stimulated. In this study, we have only considered the frequency of these shared activities that could impact early literacy skills, i.e., we have studied only the quantity but not the quality of interactions.
Moreover, beyond the low impact of the HLP, the level of parental education determines membership of each subgroup. Parents’ educational level seems to have the greatest influence on children’s education. Some studies show that more educated parents start teaching their children earlier (Hemmerechts et al., 2017) and provide literacy practice of higher quality (Vasilyeva et al., 2018), which translates into greater gains in their children’s emergent literacy skills and word reading (Myrberg and Rosén, 2009; Hartas, 2011; Crampton and Hall, 2017).
The environmental variables of socio-economic status and home literacy environment are often invoked to explain interindividual differences in reading. We decided to analyze the impact of three socio-familial variables separately, indicating what they specifically represent in the child’s family environment. We found that three environmental variables are associated with profile membership. In decreasing order of importance, these are: parents’ education level, household income and, to a lesser extent, home literacy practices. Instead of using a global socio-economic variable, we distinguished two aspects of SES, namely parents’ education level and household income. It is one of the strengths of this study with French children. As shown in other studies (Davis-Keane, 2005; Erola et al., 2016), these two environmental variables clearly have different impacts on the development of literacy skills, reading skills and more general academic achievement. We might expect the verbal interactions between parents and their children to be more dependent on PEL than on an income variable. However, the parents’ educational expectations (see Davis-Keane, 2005) and their confidence in the school could be two mediators between PEL and literacy and reading skills that future research might wish to consider.
Limitations
The conduct of this study was subject to significant constraints in that many different research teams (medical, sociological and psychological) participated in this national cohort, meaning that the evaluation time was reduced for each team. This is one of the limitations of this study. In fact, a short screening tool (Ecalle et al., 2020) was required for the researchers in cognitive psychology, meaning that there were only a small number of items in three domains in preschool (phonological awareness, letter knowledge and vocabulary) and in four domains in Grade 1 (phonemic segmentation, word reading, and listening and reading comprehension). Other literacy skills data would have been useful, in particular listening comprehension in preschool and reading fluency, rapid automatized naming and short-term verbal memory in Grade 1. For vocabulary, only receptive vocabulary was tested using an insufficient number of items and only in preschool. Another constraint was that the literacy skills of the ELFE children had to be tested in groups in their classrooms. Finally, two other limitations concern HLP, for which there was a reduced number of questions related to home literacy practices, and the form of the answers proposed (for example, a Likert scale would have been more appropriate). Moreover, it would have been more appropriate to collect HLP data during the same year as the one in which literacy skills were assessed, i.e., at 4 years of age. Last but not least, one of the three domains of CMR (Aaron et al., 2008) was absent in the present study: no “psychological” component, such as motivation or interest, was examined. These conative dimensions are the subject of many studies and our longitudinal study did not investigate this aspect during learning to read (McTigue et al., 2019).
Beyond these limitations, the number of participants (more than 2,700 children whose literacy skills were observed for a period of 2 years), the focus on French children and the differential approach to the analyses constitute some strengths of this longitudinal study. Moreover, by analyzing two types of variables, namely cognitive and environmental, this study is the first in France to contribute to the international research in this domain.
Implications
This type of profiling approach argues in favor of two types of interventions through the identification of low-performing subgroups that may be at risk of difficulties during learning to read. Knowledge of the literacy predictors reminds us that early targeted interventions in phonological skills and letter knowledge contribute to reducing difficulties in decoding and word reading (see, for example, in French, Bianco et al., 2012; Ecalle et al., 2019; in English, Hulme et al., 2012; and the recent meta-analysis by Verhoeven et al., 2020). Furthermore, knowledge of the importance of parents’ educational level for literacy skills argues in favor of the implementation of parental guidance programs in low PEL families in order to stimulate home literacy practices (Sénéchal et al., 2017), which are known to be related to the development of children’s literacy skills.
Data availability statement
The datasets presented in this article are not readily available. Requests to access the datasets should be directed to https://www.elfe-france.fr/fr/cote-recherche/acces-aux-donnees-et-questionnaires.
Ethics statement
The studies involving human participants were reviewed and approved by Elfe cohort. Written informed consent to participate in this study was provided by the participants' legal guardian/next of kin.
Author contributions
JE, HL, XT, and AM contributed to conception and design of the study. JE organized the database. ED performed the statistical analysis. JE, HL, and AM wrote the first draft of the manuscript. JE, ED, HL, XT, and AM wrote sections of the manuscript. All authors contributed to manuscript revision, read, and approved the submitted version.
Funding
The Elfe survey is a joint project between the French Institute for Demographic Studies (INED) and the National Institute of Health and Medical Research (INSERM), in partnership with the French blood transfusion service (Etablissement français du sang, EFS), Santé publique France, the National Institute for Statistics and Economic Studies (INSEE), the Direction générale de la santé (DGS, part of the Ministry of Health and Social Affairs), the Direction générale de la prévention des risques (DGPR, Ministry for the Environment), the Direction de la recherche, des études, de l’évaluation et des statistiques (DREES, Ministry of Health and Social Affairs), the Département des études, de la prospective et des statistiques (DEPS, Ministry of Culture), and the Caisse nationale des allocations familiales (CNAF), with the support of the Ministry of Higher Education and Research and the Institut national de la jeunesse et de l’éducation populaire (INJEP). Via the RECONAI platform, it receives a government grant managed by the National Research Agency under the “Investissements d’avenir” programme (ANR-11-EQPX-0038 and ANR-19-COHO-0001).
Aknowledgements
We thank Laure Gravier (Ined) for creating the task administration workbooks, Elodie Lordmi, for creating the data selection, and Marie-Aline Charles and Bertrand Geay, Director and Deputy Director of the Elfe Unit, respectively. We want also to thank Léandre Alexis Chénard-Poirier (Ph. D.), post-doctoral researcher in the Substantive Methodological Synergy Research Laboratory, Concordia University for his invaluable help and support in our statistical analysis.
Conflict of interest
The authors declare that the research was conducted in the absence of any commercial or financial relationships that could be construed as a potential conflict of interest.
Publisher’s note
All claims expressed in this article are solely those of the authors and do not necessarily represent those of their affiliated organizations, or those of the publisher, the editors and the reviewers. Any product that may be evaluated in this article, or claim that may be made by its manufacturer, is not guaranteed or endorsed by the publisher.
Supplementary material
The Supplementary material for this article can be found online at: https://www.frontiersin.org/articles/10.3389/feduc.2023.1189046/full#supplementary-material
Footnotes
1. ^Etude Longitudinale Française depuis l’Enfance (French Longitudinal study of Children) www.elfe.fr/en
2. ^Literacy skills in preschool refers to basic oral competencies related to later reading ability (see below).
3. ^Recruitment to the ELFE cohort (Etude Longitudinale Française depuis l’Enfance/French Longitudinal Study of Children) is representative of live births in France in 2011. “The general objective of the cohort is to study determinants of child development, health and socialization from birth to adulthood” (ibid, p. 369).
4. ^One constraint was that the teachers could not evaluate the cohort ELFE children alone in their classrooms, but in a group with 1 to 3 children of the same class and of approximately the same age.
References
Aaron, P. J., Joshi, R. M., Gooden, R., and Bentum, K. E. (2008). Diagnosis and treatment of reading disabilities based on the component model of reading. J. Learn. Disabil. 41, 67–84. doi: 10.1177/0022219407310838
Asparouhov, T., and Muthén, B. (2014). Auxiliary variables in mixture modeling: three-step approaches using M plus. Struct. Equ. Model. Multidiscip. J. 21, 329–341. doi: 10.1080/10705511.2014.915181
Bianco, M., Pellenq, C., Lambert, E., Bressoux, P., Lima, L., and Doyen, A.-L. (2012). Impact of early-code skill and oral comprehension training on reading achievement in first grade. J. Res. Read. 4, 427–455. doi: 10.1111/j.1467-9817.2010.01479.x
Boscardin, C. K., Muthén, B., Francis, D. J., and Baker, E. L. (2008). Early identification of reading difficulties using heterogeneous developmental trajectories. J. Educ. Psychol. 100, 192–208. doi: 10.1037/0022-0663.100.1.192
Bracken, S. S., and Fischel, J. E. (2008). Family reading behavior and early literacy skills in preschool children from low- income backgrounds. Early Educ. Dev. 19, 45–67. doi: 10.1080/10409280701838835
Burgess, S. R., Hecht, S. A., and Lonigan, C. J. (2002). Relations of the home literacy environment to the development of reading-related abilities: a one-year longitudinal study. Read. Res. Q. 37, 408–426. doi: 10.1598/RRQ.37.4.4
Cabell, S. Q., Justice, L. M., Konold, T. R., and McGinty, A. S. (2011). Profiles of emergent literacy skills among preschool children who are at risk for academic difficulties. Early Child. Res. Q. 26, 1–14. doi: 10.1016/j.ecresq.2010.05.003
Caravolas, M., Lervag, A., Mikulajova, M., Defior, S., Seidlova-Malkova, G., and Hulme, C. (2019). A Cross-Linguistic, Longitudinal Study of the Foundations of Decoding and Reading Comprehension Ability. Sci. Stud. Read. 23, 386–402. doi: 10.1080/10888438.2019.1580284
Charles, M.-A., Thierry, X., Lanoë, J.-L., Bois, C., Dufourg, M.-N., Popa, R., et al. (2020). Cohort profile: the French national cohort of children ELFE: birth to 5 years. Int. J. Epidemiol. 49, 368–369. doi: 10.1093/ije/dyz227
Crampton, A., and Hall, J. (2017). Unpacking socioeconomic risks for reading and academic self-concept in primary school: differential effects and the role of the preschool home learning environment. Br. J. Educ. Psychol. 87, 365–382. doi: 10.1111/bjep.12154
Crosnoe, R., Leventhal, T., Wirth, R. J., Pierce, K. M., and Pianta, R. C. (2010). Family socioeconomic status and consistent environmental stimulation in early childhood. Child Dev. 81, 972–987. doi: 10.1111/j.1467-8624.2010.01446.x
Davis-Keane, P. E. (2005). The influence of parent education and family income on child achievement: the indirect role of parental expectations and the home environment. J. Fam. Psychol. 19, 294–304. doi: 10.1037/0893-3200.19.2.294
Deckner, D. F., Adamson, L. B., and Bakeman, R. (2006). Child and maternal contributions to shared reading: effects on language and literacy development. Appl. Dev. Psychol. 27, 31–41. doi: 10.1016/j.appdev.2005.12.001
Dodici, B. J., Draper, D. C., and Peterson, C. A. (2003). Early parent-child interactions and early literacy development. Top. Early Child. Spec. Educ. 23, 124–136. doi: 10.1177/02711214030230030
Dong, Y., Wu, S. X.-Y., Dong, W.-Y., and Tang, Y. (2020). The effects of home literacy environment on children’s reading comprehension development: a meta-analysis. Educ. Sci. 20, 63–82. doi: 10.12738/jestp.2020.2.005
Ecalle, J., Gomes, C., Auphan, P., Cros, L., and Magnan, A. (2019). Effects of policy and educational interventions intended to reduce difficulties in literacy skills in Grade 1. Stud. Educ. Eval. 61, 12–20. doi: 10.1016/j.stueduc.2019.02.001
Ecalle, J., Labat, H., Le Cam, M., Rocher, T., Cros, L., and Magnan, A. (2015). Evidence-based practices to stimulate emergent literacy skills in kindergarten in France: A large-scale study. Teach. Teacher Educ. 50, 102–113. doi: 10.1016/j.tate.2015.05.002
Ecalle, J., Thierry, X., and Magnan, A. (2020). A brief screening tool for literacy skills in preschool children: An item response theory analysis. J. Psychoeduc. Assess. 38, 995–1013. doi: 10.1177/0734282920922079
Einarsdottir, J., Dockett, S., and Perry, B. (2009). Making meaning: children’s perspectives expressed through drawings. Early Child Dev. Care 179, 217–232. doi: 10.1080/03004430802666999
Erola, J., Jalonen, S., and Lehti, H. (2016). Parental education, class and income over early life course and children’s achievement. Res. Soc. Stratif. Mobil. 44, 33–43. doi: 10.1016/j.rssm.2016.01.003
Evans, M. D. R., Kelley, J., Sikora, J., and Treiman, D. J. (2010). Family scholarly culture and educational success: books and schooling in 27 nations. Res. Soc. Stratif. Mobil. 28, 171–197. doi: 10.1016/j.rssm.2010.01.002
Evans, M. A., Shaw, D., and Bell, M. (2000). Home literacy activities and their influence on early literacy skills. Can. J. Exp. Psychol. 54, 65–75. doi: 10.1037/h0087330
Farver, J. A. M., Xu, Y., Lonigan, C. J., and Eppe, S. (2013). The home literacy environment and Latino head start children's emergent literacy skills. Dev. Psychol. 49, 775–791. doi: 10.1037/a0028766
Fernald, A., Marchman, V. A., and Weisleder, A. (2013). SES differences in language processing skill and vocabulary are evident at 18 months. Dev. Sci. 16, 234–248. doi: 10.1111/desc.12019
Fish, M., and Pinkerman, B. (2003). Language skills in low-SES rural Appalachian children: normative development and individual differences, infancy to preschool. J. Appl. Dev. Psychol. 23, 539–565. doi: 10.1016/S0193-3973(02)00141-7
Foorman, B. R., Petscher, Y., Stanley, C., and Truckenmiller, A. (2017). Latent profiles of Reading and language and their association with standardized Reading outcomes in kindergarten through tenth grade. J. Res. Educ. Effect. 10, 619–645. doi: 10.1080/19345747.2016.1237597
Foulin, J. N. (2005). Why is letter-name knowledge such a good predictor of learning to read? Read. Writ. 18, 129–155. doi: 10.1007/s11145-004-5892-2
Frijters, J., Barron, R., and Brunello, M. (2000). Direct and mediated influences of home literacy and literacy interest on prereaders’ oral vocabulary and early written language skill. J. Educ. Psychol. 92, 466–477. doi: 10.1037/0022-0663.92.3.466
Froiland, J. M., Powell, D. R., and Diamond, K. E. (2014). Relations among neighborhood social networks, home literacy environments, and children’s expressive vocabulary in suburban at-risk families. Sch. Psychol. Int. 35, 429–444. doi: 10.1177/0143034313500415
Furnes, B., and Samuelsson, S. (2009). Preschool cognitive and language skills predicting kindergarten and grade 1 reading and spelling: a cross-linguistic comparison. J. Res. Read. 32, 275–292. doi: 10.1111/j.1467-9817.2009.01393.x
Gonzalez, J. E., Acosta, S., Davis, H., Pollard-Durodola, S., Saenz, L., Soares, D., et al. (2017). Latino maternal literacy beliefs and practices mediating socioeconomic status and maternal education effects in predicting child receptive vocabulary. Early Educ. Dev. 28, 78–95. doi: 10.1080/10409289.2016.1185885
Gough, P. B., and Tunmer, W. E. (1986). Decoding, reading, and reading disability. Remedial Spec. Educ. 7, 6–10. doi: 10.1177/074193258600700
Grimm, R. P., Solari, E. J., McIntyre, N. S., and Denton, C. A. (2018). Early reading skill profiles in typically developing and at-risk first grade readers to inform targeted early reading instruction. J. Sch. Psychol. 69, 111–126. doi: 10.1016/j.jsp.2018.05.009
Hartas, D. (2011). Families’ social backgrounds matter: socio-economic factors, home learning and young children's language, literacy and social outcomes. Br. Educ. Res. J. 37, 893–914. doi: 10.1080/01411926.2010.506945
Hemmerechts, K., Agirdag, O., and Kavadias, D. (2017). The relationship between parental literacy involvement, socio-economic status, and reading literacy. Educ. Rev. 69, 85–101. doi: 10.1080/00131911.2016.1164667
Holopainen, L., Hoang, N., Koch, A., and Kofler, D. (2020). Latent profile analysis of students’ reading development and the relation of cognitive variables to reading profiles. Ann. Dyslexia 70, 94–114. doi: 10.1007/s11881-020-00196-9
Hood, M., Conlon, E., and Andrews, G. (2008). Preschool home literacy practices and children’s literacy development: a longitudinal analysis. J. Educ. Psychol. 100, 252–271. doi: 10.1037/0022-0663.100.2.252
Hulme, C., Bowyer-Crane, C., Carroll, J. M., Duff, F. J., and Snowling, M. J. (2012). The causal role of phoneme awareness and letter-sound knowledge in learning to read: combining intervention studies with mediation analyses. Psychol. Sci. 23, 572–577. doi: 10.1177/0956797611435921
Huttenlocher, J., Waterfall, H., Vasilyeva, M., Vevea, J., and Hedges, L. V. (2010). Sources of variability in children’s language growth. Cogn. Psychol. 61, 343–365. doi: 10.1016/j.cogpsych.2010.08.002
Kendeou, P., Van den Broek, P., White, M. J., and Lynch, J. S. (2009). Predicting reading comprehension in early elementary school: The independent contributions of oral language and decoding skills. J. Educ. Psychol. 101, 765–778.
Kim, Y. S., and Petscher, Y. (2011). Relations of emergent literacy skill development with conventional literacy skill development in Korean. Read. Writ. Interdiscip. J. 24, 635–656. doi: 10.1007/s11145-010-9240-4
Lehrl, S., Evangelou, M., and Sammons, P. (2020). The home learning environment and its role in shaping children’s educational development. Sch. Eff. Sch. Improv. 31, 1–6. doi: 10.1080/09243453.2020.1693487
Lonigan, C. J. (2004). “Emergent literacy skills and family literacy” in Handbook of family literacy. ed. B. H. Wasik (Mahwah, NJ, USA: Lawrence Erlbaum), 57–81.
Lonigan, C. J., Burgess, S. R., and Anthony, J. L. (2000). Development of emergent literacy and early reading skills in preschool children: evidence from a latent-variable longitudinal study. Dev. Psychol. 36, 596–613. doi: 10.1037//0012-1649.36.5.596
Manolitsis, G., Georgiou, G., and Parrila, R. (2011). Revisiting the home literacy model of reading development in an orthographically consistent language. Learn. Instr. 21, 496–505. doi: 10.1016/j.learninstruc.2010.06.005
Manolitsis, G., Georgiou, G. K., and Tziraki, N. (2013). Examining the effects of home literacy and numeracy environment on early reading and math acquisition. Early Child. Res. Q. 28, 692–703. doi: 10.1016/j.ecresq.2013.05.004
McTigue, E. M., Solheim, O. J., Walgermo, B., Frijters, J., and Foldnes, N. (2019). How can we determine students’ motivation for reading before formal instruction? Results from a self-beliefs and interest scale validation. Early Child. Res. Q. 48, 122–133. doi: 10.1016/j.ecresq.2018.12.013
Mol, S. E., and Bus, A. G. (2011). To read or not to read: a meta-analysis of print exposure from infancy to early adulthood. Psychol. Bull. 137, 267–296. doi: 10.1037/a0021890
Morris, D., Bloodgood, J., and Perney, J. (2003). Kindergarten predictors of first-and second-grade reading achievement. Elem. Sch. J. 104, 93–109. doi: 10.1086/499744
Munger, K. A., and Blachman, B. A. (2013). Taking a “simple view” of the dynamic indicators of basic early literacy skills as a predictor of multiple measures of third-grade reading comprehension. Psychol. Sch. 50, 722–737. doi: 10.1002/pits.21699
Muthén, L. K., and Muthén, B. O.. (1998/2017). Mplus User’s Guide. 8th Edn. Los Angeles, CA: Muthén & Muthén.
Myrberg, E., and Rosén, M. (2009). Direct and indirect effects of parents' education on reading achievement among third graders in Sweden. Br. J. Educ. Psychol. 79, 695–711. doi: 10.1348/000709909X453031
Niklas, F., Wirth, A., Guffler, S., Drescher, N., and Ehmig, S. C. (2020). The home literacy environment as a mediator between parental attitudes toward shared Reading and Children's linguistic competencies. Front. Psychol. 11:1628. doi: 10.3389/fpsyg.2020.01628
Ozernov-Palchik, O., Norton, E. S., Sideridis, G., Beach, S. B., Wolf, M., Gabrieli, J. D. E., et al. (2017). Longitudinal stability of pre-reading skill profiles of kindergarte children: implications for early screening and theories of reading. Dev. Sci. 20:e12471. doi: 10.1111/desc.12471
Pace, A., Luo, R., Hirsh-Pasek, K., and Golinkoff, R. M. (2017). Identifying pathways between socioeconomic status and language development. Ann. Rev. Ling. 3, 285–308.
Papadimitriou, A. M., and Vlachos, F. M. (2014). Which specific skills developing during preschool years predict the reading performance in the first and second grade of primary school? Early Child Dev. Care 184, 1706–1722. doi: 10.1080/03004430.2013.875542
Perfetti, C., and Stafura, J. (2014). Word knowledge in a theory of comprehension. Sci. Stud. Read. 18, 22–37. doi: 10.1080/10888438.2013.827687
Phillips, B. M., and Lonigan, C. J. (2009). Variations in the home literacy environment of preschool children: a cluster analytic approach. Sci. Stud. Read. 13, 146–174. doi: 10.1080/10888430902769533
Piquard-Kipffer, A., and Sprenger-Charolles, L. (2013). Early predictors of future reading skills: a follow-up of French-speaking children from the beginning of kindergarten to the end of the second grade (age 5 to 8). Annee Psychol. 113, 491–521.
Powell, D., and Atkinson, L. (2021). Unraveling the links between rapid automatized naming (RAN), phonological awareness, and reading. J. Educ. Psychol. 113, 706–718. doi: 10.1037/edu0000625
Puranik, C. S., Phillips, B. M., Lonigan, C. J., and Gibson, E. (2018). Home literacy practices and preschool children’s emergent writing skills: an initial investigation. Early Child. Res. Q. 42, 228–238. doi: 10.1016/j.ecresq.2017.10.004
Roberts, J., Jurgens, J., and Burchinal, M. (2005). The role of home literacy practices in preschool children’s language and emergent literacy skills. J. Speech Lang. Hear. Res. 48, 345–359. doi: 10.1044/1092-4388(2005/024)
Rose, E., Lehrl, S., Ebert, S., and Weinert, S. (2018). Long-term relations between children’s language, the home literacy environment, and socioemotional development from ages 3 to 8. Early Educ. Dev. 29, 342–356. doi: 10.1080/10409289.2017.1409096
Rowe, M. L. (2008). Child-directed speech: relation to socioeconomic status, knowledge of child development and child vocabulary skill. J. Child Lang. 35, 185–205. doi: 10.1017/s0305000907008343
Rowe, M. L., Raudenbush, S., and Goldin-Meadow, S. (2012). The pace of vocabulary growth helps predict later vocabulary skill. Child Dev. 83, 508–525. doi: 10.1111/j.1467-8624.2011.01710.x
Scarborough, H. S. (2001). “Connecting early language and literacy to later reading (dis)abilities: evidence, theory, and practice” in Handbook of early literacy research. eds. S. B. Neuman and D. K. Dickinson (New York: Guilford Press), 97–110.
Scarborough, H. S. (2009). “Connecting early language and literacy to later reading (dis) abilities: evidence, theory, and practice” in Approaching difficulties in literacy development: Assessment, pedagogy, and programmes. eds. F. F. Campbell, J. Soler, and G. Reid (London, England: Sage), 23–39.
Schmitt, S. A., Simpson, A. M., and Friend, M. (2011). A longitudinal assessment of the home literacy environment and early language. Infant Child Dev. 20, 409–431. doi: 10.1002/icd.733
Sénéchal, M. (2006). Testing the home literacy model: parent involvement in kindergarten is differentially related to grade 4 reading comprehension, fluency, spelling, and reading for pleasure. Sci. Stud. Read. 10, 59–87. doi: 10.1207/s1532799xssr10014
Sénéchal, M., and LeFevre, J. A. (2002). Parental involvement in the development of children’s reading skill: a five-year longitudinal study. Child Dev. 73, 445–460. doi: 10.1111/1467-8624.00417
Sénéchal, M., and LeFevre, J. A. (2014). Continuity and change in the home literacy environment as predictors of growth in vocabulary and reading. Child Dev. 85, 1552–1568. doi: 10.1111/cdev.12222
Sénéchal, M., LeFevre, J.-A., Thomas, E. M., and Daley, K. E. (1998). Differential effects of home literacy experiences on the development of oral and written language. Read. Res. Q. 33, 96–116. doi: 10.1598/RRQ.33.1.5
Sénéchal, M., Whissell, J., and Bildfell, A. (2017). “Starting from home: home literacy practices that make a difference” in Theories of Reading development. eds. K. Cain, D. L. Compton, and R. K. Parrila (Amsterdam, Netherlands: John Benjamins Publishing Company), 383–407.
Silinskas, G., Lerkkanen, M. K., Tolvanen, A., Niemi, P., Poikkeus, A. M., and Nurmi, J. E. (2012). The frequency of parents’ reading-related activities at home and children’s reading skills during kindergarten and grade 1. J. Appl. Dev. Psychol. 33, 302–310. doi: 10.1016/j.appdev.2012.07.004
Spurk, D., Hirschi, A., Wang, M., Valero, D., and Kauffeld, S. (2020). Latent profile analysis: a review and “how to” guide of its application within vocational behavior research. J. Vocat. Behav. 120:103445. doi: 10.1016/j.jvb.2020.103445
Storch, S. A., and Whitehurst, G. J. (2002). Oral language and code-related precursors to Reading: evidence from a longitudinal structural model. Dev. Psychol. 38, 934–947. doi: 10.1037//0012-1649.38.6.934
Suggate, S., Schaughency, E., McAnally, H., and Reese, E. (2018). From infancy to adolescence: the longitudinal links between vocabulary, early literacy skills, oral narrative, and reading comprehension. Cogn. Dev. 47, 82–95. doi: 10.1016/j.cogdev.2018.04.005
Tamis-LeMonda, C. S., Luo, R., McFadden, K. E., Bandel, E. T., and Vallotton, C. (2019). Early home learning environment predicts children’s 5th grade academic skills. Appl. Dev. Sci. 23, 153–169. doi: 10.1080/10888691.2017.1345634
Torppa, M., Georgiou, G. K., Lerkkanen, M.-K., Pekka, N., Poikkeus, A.-M., and Nurmi, J.-E. (2016). Examining the simple view of Reading in a transparent orthography: a longitudinal study from kindergarten to grade 3. Merrill-Palmer Q. 62, 179–206. doi: 10.13110/merrpalmquar1982.62.2.0179
Tunmer, W. E., and Chapman, J. W. (2012). The simple view of reading redux: vocabulary knowledge and the independent components hypothesis. J. Learn. Disabil. 45, 453–466. doi: 10.1177/0022219411432685
Umek, L. M., Podlesek, A., and Fekonja, U. (2005). Assessing the home literacy environment: relationships to child language comprehension and expression. Eur. J. Psychol. Assess. 21, 271–281. doi: 10.1027/1015-5759.21.4.271
UNESCO. (2012). International Standard Classification of Education ISCED 2011. Available at http://uis.unesco.org/sites/default/files/documents/international-standard-classification-of-education-isced-2011-en.pdf.
Vasilyeva, M., Dearing, E., Ivanova, A., Shen, C., and Kardanova, E. (2018). Testing the family investment model in Russia: estimating indirect effects of SES and parental beliefs on the literacy skills of first graders. Early Child. Res. Q. 42, 11–20. doi: 10.1016/j.ecresq.2017.08.003
Verhoeven, L., Voetena, M., van Settena, E., and Segers, E. (2020). Computer-supported early literacy intervention effects in preschool and kindergarten: a meta-analysis. Educ. Res. Rev. 30. doi: 10.1016/j.edurev.2020.100325
Verhoeven, L., van Leeuwe, J., and Vermeer, A. (2011). Vocabulary growth and reading development across the elementary school years. Sci. Stud. Read. 15, 8–25. doi: 10.1080/10888438.2011.536125
Vermunt, J. K. (2010). Latent class modeling with covariates: two improved three-step approaches. Polit. Anal. 18, 450–469. doi: 10.1093/pan/mpq025
Verwimp, C., Van den Bempt, F., Kellens, S., Economou, M., Vandermosten, M., Wouters, J., et al. (2020). Pre-literacy heterogeneity in Dutch-speaking kindergartners: latent profile analysis. Ann. Dyslexia 70, 275–294. doi: 10.1007/s11881-020-00207-9
Whitehurst, G. J., and Lonigan, C. J. (2001). “Emergent literacy: development from prereaders to readers” in Handbook of early literacy research. eds. S. B. Neuman and D. K. Dickensen (New York: Guilford Press), 11–29.
Zauche, L. H., Thul, T. A., Mahoney, A. E. D., and Stapel-Wax, J. L. (2016). Influence of language nutrition on children’s language and cognitive development: an integrated review. Early Child. Res. Q. 36, 318–333. doi: 10.1016/j.ecresq.2016.01.015
Zheng, P., and Libertus, M. (2018). The role of parental education, household income, and race on parents’ academic beliefs and the provision of home learning opportunities for 4- to 8-year-old children. J. Educ. Dev. Psychol. 8, 118–132. doi: 10.5539/jedp.v8n1p118
Keywords: reading development, literacy abilities, parents’ education level, household income, latent profile analysis
Citation: Ecalle J, Dujardin E, Labat H, Thierry X and Magnan A (2023) Profiles of learner readers and their early literacy skills and environmental predictors: a large-scale longitudinal study from preschool to grade 1. Front. Educ. 8:1189046. doi: 10.3389/feduc.2023.1189046
Edited by:
Luana Sorrenti, University of Messina, ItalyReviewed by:
Nenagh Meredith Kemp, University of Tasmania, AustraliaJeffrey M. DeVries, Technical University Dortmund, Germany
Copyright © 2023 Ecalle, Dujardin, Labat , Thierry and Magnan. This is an open-access article distributed under the terms of the Creative Commons Attribution License (CC BY). The use, distribution or reproduction in other forums is permitted, provided the original author(s) and the copyright owner(s) are credited and that the original publication in this journal is cited, in accordance with accepted academic practice. No use, distribution or reproduction is permitted which does not comply with these terms.
*Correspondence: Jean Ecalle, ZWNhbGxlLmplYW5Ad2FuYWRvby5mcg==