- Faculty of Education, Brock University, St. Catharines, ON, Canada
Introduction: Robotics is viewed as a viable pedagogical strategy for STEM learning because it is characterized by many practices common to the STEM disciplines such as engineering design. With many national curricular calling for STEM integration in K-12 formal educational settings, there is a need for empirical evidence about the effectiveness of different pedagogical approaches to teach with robotics-based activities to promote curriculum learning outcomes and teaching practice. This exploratory study investigated the effectiveness of a scaffolded robotics intervention and a self-guided robotics intervention on pre-service teacher knowledge (PST) of science concepts related to gears and on PST self-efficacy to teach with the robotics-based activities.
Methods: A quasi-experimental, pre-post intervention study was implemented with two non-equivalent groups of elementary preservice teachers (PSTs) in a Bachelor of Education program. PSTs in the self-guided group (n = 11) worked with robotics kits in the library at their own pace. PSTs in the scaffolded intervention group (n = 16) were guided through the activity by the author with instructional scaffolds. IBM SPSS Statistics 27 was used to analyze the data.
Results: The relationship between intervention type and gains in science knowledge was not statistically significant for the self-guided group but was statistically significant for the scaffolded group suggesting that scaffolding supported PST’s learning of the science concepts. With respect to PST self-efficacy to teach with the robotics-based activity, both intervention types revealed statistically significant gains from pre to post tests, however effect sizes indicated that the scaffolded intervention resulted in greater gains in PST self-efficacy to teach with the robotics-based activities.
Discussion: The results provide exploratory evidence that the scaffolded robotics approach, modelled for and experienced by the pre-service teachers in this study, was effective for their learning of science curricular concepts related to gears and for developing their self-efficacy for teaching the robotics-based activities. It should be noted that findings may not be generalizable due to the small sample sizes, especially of the self-guided group. Nevertheless, the findings do provide insights for teacher educators incorporating robotics-based activities into curricular courses such as science methods as it provides specific examples of scaffolds that were effective for science learning and for developing PST self-efficacy. The study also contributes to the literature on instructional strategies that promote robotics adoption in K-12 schools to support development of STEM knowledge and skills.
1. Introduction
With many economic sectors requiring workers to have knowledge and skills in the STEM (science, technology, engineering and mathematics) fields, STEM in education is viewed as a strategy to promote sustainable economic development, job growth and competitiveness in the global economy (Carnevale et al., 2011; National Research Council, 2014; Warde and Sah, 2019). The teaching of STEM content is traditionally organized in most elementary and secondary school curricular as discrete disciplines focused on canonical content and practices such as science and mathematics, respectively. Many countries are therefore revisiting school curricula to include engineering and technology practices into these existing STEM disciplines. For example, in 2012, the revised K-12 Science Education Standards in the United States was published with the goal that by the end of the 12th grade,
all students have some appreciation of the beauty and wonder of science; possess sufficient knowledge of science and engineering to engage in public discussions on related issues; are careful consumers of scientific and technological information related to their everyday lives; are able to continue to learn about science outside school; and have the skills to enter careers of their choice, including (but not limited to) careers in science, engineering, and technology (National Research Council, 2012, p. 1).
Interestingly, in Canada, engineering and technology practices were proposed as an integral part of the science curriculum in the late 1990s. The Pan Canadian Science framework (CMEC (Council of Ministers of Education, Canada), 1997), developed to guide provincial development of K-12 science curricula in Canada, outlined a vision for scientific literacy that included engineering and technology practices such as engineering design, problem solving and decision making in relation to science-technology-society-environment (STSE) content and canonical science content. Canadian provinces adopted some version of the Pan Canadian Science Framework and incorporated engineering design and technology applications in the elementary and middle school science curriculum. The incorporation of engineering design into European school science curricula, is also occurring as evidenced by large scale international projects such as ENGINEER, that promoted integration of engineering design practices based on the “Engineering is Elementary” (EiE) program, created by the Boston Museum of Natural Science (BMOS) in the US (Anyfandi et al., 2013). K-12 science education in many countries therefore include the learning of content and practices related to engineering and technology; mathematics however is usually taught as a discrete subject in most K-12 public school systems. One of the reasons for the latter could be the challenges experienced with enhancing math achievement when math is taught through integration in other STEM disciplines such as engineering (Tran and Nathan, 2010). Some research has shown that when math is integrated with other STEM subjects, there was limited development of foundational math thinking and concepts as assessed by curriculum standards (Tran and Nathan, 2010; Tytler, 2020) and the smallest gains occurred in mathematics achievement (Becker and Park, 2011). If math is to be integrated into other STEM disciplines in formal contexts, additional supports may need to be provided for students to gain knowledge of specific math concepts and for math teachers to design effective interdisciplinary activities (National Research Council, 2014; Williams et al., 2016).
The impetus to include STEM outcomes in science curricular has gained momentum. For example, in Ontario, Canada, where this study occurred, the revised grades 1–8, Science and Technology Curriculum (Ontario Curriculum and Resources, 2022) calls for an understanding of the cross-curricular and cross-disciplinary nature of problem-solving within the STEM disciplines to develop STEM skills such as computational thinking, coding, and innovation. There are many problem-solving approaches that promote STEM learning in classrooms such as engineering design, scientific inquiry and problem-based learning. These approaches are characterized by experiential learning and student centeredness with real-world problems or case-based scenarios acting as both the focus of and stimulus for learning (National Research Council, 2014). Robotics is a learning tool that lends itself to experiential and student-centered approaches because it is a concrete manipulative that children interact with and explore while solving real-world problems and constructing knowledge (Eguchi, 2021; Glezou, 2021). Moreover, with technology and engineering often underrepresented in schools (Bybee, 2010), robotics is viewed as a strategy to engage K-12 students in the components of STEM and develop their 21 century competencies such as problem solving and creativity (Taylor, 2016). While studies show that robotics supports students learning STEM knowledge and gaining 21st century skills such as critical thinking (Benitti, 2012; Anwar et al., 2019), robotics programs are not widely implemented in schools for a variety of reasons such as lack of resources and lack of pedagogical knowledge about how to integrate robotics as an instructional strategy in teaching and learning (Vollstedt et al., 2007; Park, 2015). One of the ways to address the lack of pedagogical knowledge about teaching with robotics is in preservice teacher education courses.
In most Teacher Education programs, methods courses parallel how subjects are taught at schools, for example separate science and mathematics curricula. So, Science Education courses in Ontario, Canada emphasize the S, T, and E in STEM. Preservice teachers have a separate math education course that teaches math content and skills including coding. Besides robotics being introduced to PSTs to learn how to build and program it, PSTs need to learn how to create learning experiences to meet STEM-related curriculum outcomes. Moreover, it is important for science educators to model instructional approaches for teaching with robotics because teachers’ self-efficacy beliefs have been found to play a role in teachers’ implementation of new technology in teaching (Estes, 2019). Hands-on engagement with robotics tools in learning and teaching contexts is a strategy that can enhance PSTs self efficacy to integrate robotics in K-12 classroom teaching (Jaipal-Jamani and Angeli, 2017). Subsequently, when PSTs and teachers expose their students to hands-on, fun and exciting learning environments in STEM, these experiences can lead to positive student attitudes and interest in STEM fields which has been shown to influence career choices and career goals (Vedder-Weiss and Fortus, 2012; Adedokun et al., 2013; Wang, 2013). What pedagogical/instructional approaches with robotics-based activities support the learning of science and support development of teacher self-efficacy to teach with robotics? There are few studies with preservice teachers comparing the effect of different instructional approaches with robotics on learning of science and pre-service teacher self efficacy. For example, the study by Sullivan and Moriarty (2009) explored the effectiveness of the discovery method to learn robotics content but participants were practicing middle and high school teachers. While Ortiz et al. (2015) implemented a 2-h, scaffolded integrated, robotics experience, as part of a teacher preparation course, the disciplinary content focus of this qualitative study was on mathematical connections. Alimisis (2012) did explore how robotics-based activities supported development of physics concepts, but their study was with secondary preservice teachers and data were reported from a qualitative case study of two preservice teachers.
This paper reports on an exploratory quantitative study with elementary preservice teachers, certified to teach from kindergarten to grade 6, who participated in a robotics-based activity in a science education teaching methods course at a Canadian university. The main purpose of this study was to examine how a scaffolded and a self-guided (similar to discovery) robotics-based activity supported science learning and developed preservice teachers’ self-efficacy to teach using robotics-based activities. These two different instructional approaches were used with two different classes of pre-service teachers (PSTs) for learning about gear concepts.
The research questions were:
1. How does a self-guided instructional approach with robotics-based activities affect elementary preservice teachers’ learning of science concepts?
2. How does a scaffolded instructional approach with robotic-based activities affect elementary preservice teachers’ learning of science concepts?
3. How does a self-guided instructional approach with robotics-based activities affect elementary preservice teachers’ self-efficacy to teach with robotics?
4. How does a scaffolded instructional approach with robotics-based activities affect elementary preservice teachers’ self-efficacy to teach with robotics?
This study is timely considering the global focus to promote the teaching of STEM concepts and skills in K-12 schools through technology such as educational robotics (e.g., National Research Council, 2014; Ontario Curriculum and Resources, 2022). The findings provide empirical evidence that will add to the literature reporting on the effect of instructional approaches with robotics-based activities on preservice teachers’ science learning and self-efficacy to teach with robotics. Such insights can be used to inform the development of science education courses, specifically the design of instructional strategies for robotics-based activities to enhance PST self-efficacy to teach science and integrated STEM with robotics. It can also inform the design of professional development for teachers in the field to support teaching with robotics-based activities in teaching practice.
2. Literature review
2.1. Theoretical framework
The study draws on the theory of constructionism which emphasizes knowledge being constructed during physical interactions with objects such as building of concrete models (Papert and Harel, 1991). As learners engage physically with concrete objects, the objects may become, as Papert stated, objects-to-think-with and facilitate thinking about ideas that learners may not otherwise be able to grasp (Sullivan, 2017). It is through the act of building a meaningful or tangible product that knowledge is constructed and reinforced (Martinez and Stager, 2013). Learners are regarded as creators, are self-directed and learn by themselves through building/construction (Holbert et al., 2020). Educational Robotics (ER) therefore lends itself to constructionism when learners engage with concrete materials and are active creators of knowledge through self-guided learning and problem solving. The most common conceptualization of self-guided learning in the literature is discovery learning. Discovery learning as articulated by Bruner (1961) involves constructing one’s own knowledge by discovering it rather than being instructed on it or being told about it by the teacher. In learning environments based on discovery learning, students are supposed to independently explore and solve problems with little to no guidance (Mayer, 2004). The learner is not provided with the target information or knowledge and must learn it independently from the resources given (Alfieri et al., 2011). The teacher’s role in this type of instructional approach is to therefore provide students with the relevant resources they need to obtain information from and allow them to explore and discover the information or relationships between concepts In this paper, the term self-guided will be used to refer to this type of discovery approach.
Another relevant construct in this study is learning. This study is grounded in Vygotsky’s (1978) sociocultural theory where higher mental processes or knowledge construction is mediated by psychological tools (e.g., language). In his work, Vygotsky emphasized two types of mediational means, signs and sign systems and interpersonal relations. Accordingly, the learning of concepts is mediated by psychological tools such as language, diagrams, pneumonic techniques, text, and symbols during social interactions with more capable peers and adults. Learners first use external mediational means to make sense of the activities and concepts at the social or interpersonal level; then they construct their own, individual meanings by interpreting in the context of existing or prior knowledge and experiences, Hence, learning concepts is also mediated by prior knowledge and experiences of the learner (Vygotsky, 1987) where prior learning in one situation acts as the foundation for learning in another situation (Dewey, 1938). The study also draws on theories of scaffolding during the learning process to explain how scaffolds act as mediational means. According to Wood et al. (1976, p. 90), scaffolding enables “a child or novice to solve a problem, carry out a task, or achieve a goal which would be beyond his unassisted effort” and during this interaction, the adult controls “those elements of the task that are initially beyond the learner’s capacity, thus permitting him to concentrate upon and complete only those elements that are within his range of competence.” Wood et al. identified six scaffolding functions that the instructor could apply in learning situations.
• Recruitment—which is to gain the learner’s interest in the task and focus them on the steps required to complete the task.
• Reduction in degrees of freedom—involves simplifying the task by reducing how many steps are required to match the learners’ level of knowledge/competence.
• Direction maintenance—is to motivate learners to stay focused on the task and to take risks to progress to the next steps.
• Marking critical features—is to provide feedback on learner’s progress by highlighting relevant features of the task so learner modifies and changes any incorrect steps.
• Frustration control—involves minimizing frustration in learner in ways that do not create emotional dependency on tutor.
• Demonstration—where tasks that learners can achieve are demonstrated or partial solutions to tasks are modeled so learner can imitate and use as stepping-stone to complete task.
According to Shvarts and Bakker (2019), contemporary views on scaffolding envision scaffolding as a temporary adaptive support. Scaffolding is described by them as “an adult’s activity of “bridging” the current state of the child’s abilities with the future, required state, which is known by the adult” (p. 15). As the activity progresses, external support should fade so the learner develops competence to complete the task unaided (Pea, 2004; Puntambekar and Hubscher, 2005). In this way, students begin to learn how to self-regulate and self scaffold and can perform tasks independently (Shvarts and Bakker, 2019). Hence, an important outcome of scaffolding is self-directed learning which is promoted by gradual fading of supports.
2.2. Educational robotics and science learning
Educational robotics (ER) involves the creation of learning environments where robotics kits, programming software, and computers are used as hands-on learning tools to foster problem-solving, critical thinking, collaboration and learning of abstract concepts and ideas (Eguchi, 2016, 2021).
Robotics-based activities are particularly suited for the development of STEM skills, especially the practices that characterize scientific inquiry and engineering as outlined in the Framework for K-12 Science Education (National Research Council, 2012). According to the National Research Council (NRC) framework, certain practices are closely aligned with each discipline with some practices common to both disciplines. For example, scientific inquiry is characterized by practices such as posing questions about puzzling phenomena and constructing explanations whereas engineering is characterized by defining problems and constructing prototypes of products. Both disciplines involve practices such as stating a hypothesis, designing investigations, repeating experiments, analyzing and interpreting data to confirm or reject the hypothesis and evaluating and communicating results or information. Robotics specifically promotes engineering design practices by engaging students in practices such as defining the engineering problem (e.g., how does the robot work to solve the problem), proposing the solution to the problem (e.g., how to build the robot), and optimization (e.g., how to improve the efficiency of the robot to complete the task; Ziaeefard et al., 2017). Some research on robotics in K-12 education (Benitti, 2012; Anwar et al., 2019) shows that ER supports student learning of concepts in the STEM areas in both formal and after-school or extracurricular contexts. With respect to gains in science learning, studies with middle school students show, for example, that gains were made in conceptual knowledge of evolution concepts after participation in a robotics teaching unit (Whittier and Robinson, 2007) and in physics content knowledge after participation in a summer robotics camp (Williams et al., 2007). A recent review by Kyriazopoulos et al. (2022) expands prior reviews by focusing on research in primary education with students 6–12 years. Although their review findings show that ER promotes cognitive and affective outcomes in STEM, there were mixed findings about robotics impacts on science attitudes and learning. For example, Ching et al. (2019) investigated how 18, grade 4–6 students participated in a STEM, PBL robotics curriculum conducted as an after-school program. Students worked with EV3 robots and results showed no statistically significant improvement in attitudes towards science, engineering and technology; however, there was significant improvement in mathematics attitudes. Results reported by Sáez-López et al. (2019), who investigated 93, grade 6, middle school students’ integration of mBot and Scratch programming, were statistically significant for improved comprehension of math and programming concepts but were not significant for science concepts. Kyriazopoulos et al. (2022) also identified challenges experienced during robotics activities. For example, building robots was found to be the most challenging aspect of the activity for the grade 4–6 students in the Ching et al. (2019) study due to minimal prior experience, complicated designs, and missing robot parts. Similarly, in another study of a school-based STEM implementation with grade 5 students, Kopcha et al. (2017) reported that students experienced challenges building robots; teachers and students found the visuals and guides difficult to follow to construct robots. It was also found that challenges arising during robotics and programming implementation in schools could be linked to teacher training (Sáez-López et al., 2019), specifically teachers’ lack of knowledge and experience with coding and programming (Kopcha et al., 2017). Other challenges reported by teachers during implementation were that science standards were not emphasized as much as math and engineering in the robotics activities, with teachers calling for stronger connections to be made to science curriculum standards (Kopcha et al., 2017).
Besides the complex nature of robotics kits and materials, lack of teacher training, and inadequate connections to science standards identified as factors affecting science learning outcomes during robotics-based activities with elementary students, the instructional approach used by the teacher/instructor also impacts student learning (Sullivan and Moriarty, 2009; Fanchamps et al., 2021). ER kits are designed to enable students to learn with minimal guidance how to design, build, and program robots and support discovery learning (Sullivan and Moriarty, 2009). Ormrod (1995) explains discovery learning as an instructional approach where students explore and manipulate objects, seek answers to questions and solve problems, or perform experiments. It is a minimally guided approach – minimum guidance is given during the activity or information about the task is available for learners if they choose to refer to it (Kirschner et al., 2006). Learners are expected to “discover” for themselves knowledge about the concepts by being active inquirers and the teacher’s role is to support in the form of relevant materials and socratic questions with minimal direct instruction (Hammer, 1997; Sullivan and Moriarty, 2009). The literature on the effect of discovery approaches on learning in the STEM subjects reports mixed results. Some studies found discovery approaches with teachers learning with robotics as supporting the development of robotics and programming knowledge (Chambers and Carbonaro, 2003; Sullivan and Moriarty, 2009). However, majority of teachers in the Sullivan and Moriarty study expressed criticism of discovery as a teaching method, calling it frustrating and slow, based on their own experiences as learners during the robotics activities. The teachers felt the time spent on figuring out one small feature increased their frustration which could have been alleviated by more guidance or some form of direct instruction. The latter sentiments expressed by teachers seems to support results of investigations of discovery approaches with students in classrooms. For example, Klahr and Nigam (2004) reported that the discovery approach with elementary students was less effective in promoting science learning when compared to direct/scaffolded instruction (examples and explanations provided by teacher). However, Roll et al. (2018) reported no significant difference in science knowledge gains by university students between discovery and the guided inquiry approach (written guidance on how to complete task is provided). These mixed results in different instructional contexts suggests that further investigation into learning science with robotics using different instructional approaches is needed.
2.3. Scaffolded instruction in classrooms
While Wood et al. (1976) theorized about scaffolding from a study involving a one-to-one tutor relationship, real classrooms in many countries face many constraints such as 30 students taught by one teacher and lack of resources. Scaffolding in classroom contexts is much more complex and involves the teacher establishing conditions or providing support by structuring a learning activity to help a diverse group of students bridge the gap between current abilities and the target task or knowledge outcomes. Features of scaffolding and support materials suggested for classroom learning are instructor demonstration or modelling the task, peer collaboration, targeted questions, repetition, and visual aids (Taber, 2018a). Another form of scaffolding supporting learning is process worksheets that give information on the steps or phases to solve the task and hints for successful completion of the task (Van Merriënboer, 1997). Scaffolding is important to help students focus on elements of a task that is within their capabilities (Simons and Klein, 2007). Scaffolding becomes even more important in problem-centered approaches such as design based and problem-based learning as these approaches are often characterized by ill-structured problems with no clear directions to the goal and require self-directed learning (Jonassen, 2011). Studies have shown that students with little prior knowledge significantly benefit from guidance and structure (Mayer, 2004; Kirschner et al., 2006; Oksa et al., 2010; Kalyuga, 2013). Hence in the current study, conceptual scaffolds in the form of questions on a worksheet supported students construct meanings about gear concepts and strategic scaffolds such as process worksheets that modelled how to program, guided learning of phases such as sequencing and debugging.
Computer programs (e.g., robotics programs, mathematics problem solving applications, science simulations) also incorporate different forms of scaffolding to support problem-solving. Belland et al. (2017) explain that computer-based scaffolding employs similar strategies as proposed by Wood et al. (1976) but the way computers enact the scaffolding is different because of the preprogramming that needs to be done. They explain that scaffolding logic assesses where learners are at and what type of scaffolds to provide and that scaffolds can be fixed—scaffolds are added or faded during the program and performance adapted; and self-selected—choice of scaffolds is provided or enabled during the activity. There are many types of computer scaffolding intervention types based on the work of Hannafin et al. (1999): conceptual scaffolds—e.g., knowledge question prompts, links or hints to experts, and concept mapping and visualizations tools; metacognitive scaffolds—e.g., prompts to self-reflect on learning and evaluate choice of strategies; strategic scaffolds—e.g., shows and guides how to solve unique problems such as in algebra; and motivation scaffolds—enhances self-efficacy, interest and perceptions of autonomy (Belland et al., 2017). A meta-analysis of 144 experimental studies investigating the effectiveness of computer-based scaffolding on problem-centered approaches in STEM education such as design based and problem-based learning showed that students who received the scaffolds did better on cognitive tests than students who did not receive scaffolds and all scaffolding intervention types except for motivation scaffolds improved cognitive outcomes (Belland et al., 2017).
The positive impact of computer-based scaffolding for supporting cognitive outcomes in problem-based learning in STEM was also shown through Bayesian meta-analysis (Kim et al., 2018). The latter results point to the notion that scaffolding is important to support learning of STEM knowledge through computer-based activities such as robotics in formal classroom settings, especially for novice learners.
2.4. Self efficacy and teaching
According to Bandura (1994), self-efficacy refers to a person’s belief in his or her capability to carry out actions or complete a task to produce specific outcomes and it includes a judgment regarding how well he or she can perform the task or action, and confidence in having the skills to do the task or action. As such Bandura (1977) distinguished between two types of expectations related to self-efficacy: outcomes expectations and efficacy expectations. He defined outcomes expectations as a person’s guess that a given behavior will lead to certain outcomes and efficacy expectations as conviction that one can successfully perform the behavior to produce the favorable outcome. Self-efficacy beliefs therefore play a significant role in how people are motivated, make choices and behave in specific settings such as teachers in classrooms. Bandura (1994) suggested four ways to develop a person’s self-efficacy: mastery experiences which involve direct experience with and successful completion of the action or task; vicarious experiences through observing social role models successfully complete a task; social persuasion through positive verbal feedback; and emotional and physiological states that are managed to reduce stress reactions. These four strategies have been applied in many educational contexts for developing self-efficacy among teachers with varying results on the strategy’s relative importance (Morris et al., 2017). For example, while vicarious experiences such as modeling experiences (e.g., observing another person teach) and enactive mastery experiences (that is perceived successes in prior teaching) were found to enhance self-efficacy among elementary science teachers, it was cognitive mastery of pedagogical content knowledge and verbal persuasion through in situ feedback that were more effective for developing self-efficacy among teachers in the study by Palmer (2011). On the other hand, Tschannen-Moran and Woolfolk Hoy (2007) found that it was enactive mastery experiences that mostly explained the increases in self-efficacy among novice and experienced teachers. Likewise, Velthuis et al. (2014) reported that it was the experiences of preservice teachers when they began to apply content to the practice of teaching science to students in the classroom that most impacted their self efficacy beliefs about teaching science. Findings on the role played by subject-matter knowledge on teacher self-efficacy in general suggest that there was a relationship between subject matter knowledge and self-efficacy (Yilmaz-Tuzun, 2008; Rohaan et al., 2012). The latter results are reinforced in the review on teaching efficacy by Morris et al. (2017) who found that studies show overall that knowledge of subject matter led to an improved sense of efficacy among teachers.
With respect to technology and teachers’ self-efficacy, studies (e.g., Lemon and Garvis, 2016; Valtonen et al., 2018) show that many preservice teachers do not feel confident about integrating technology into teaching practice. Many studies have also investigated in-service teacher self efficacy and knowledge to teach with robotics (e.g., Hamner et al., 2016; Hodges et al., 2016; Sinay et al., 2016; You et al., 2021; Fridberg et al., 2022). However, there are a limited number of studies reporting on preservice teachers’ self-efficacy and robotics. For example, Schina et al. (2021) designed a 3 session ER training program at a university in Spain where preschool PSTs engaged in mastery experiences in the form of structured, hands-on experimentation to program a Blue Bot robotic toy, followed by experimentation with Blue Bot classroom application projects, with a final group project being to create a Blue Bot project to teach a topic to preschool students. The findings showed that self efficacy towards educational robotics such as PSTs skills and knowledge about how to use robotics for classroom instruction and for teaching science improved after participation in the ER training module. In another study, Martin et al. (2020) explored ECE and Primary Education PSTs self-efficacy beliefs to use appropriate technology including robotics in teaching in a technology course at an Australian university. A sample of 92 participants completed a pre-post self efficacy survey. Results indicated that PSTs confidence post course was strongest for the technical use and pedagogical applications of technology such as robotics. The authors suggest this strong increase in confidence may be due to PSTs being exposed to structured, hands-on learning experiences grounded in curriculum-related contexts. In another study conducted with 26 PSTs in a Master of Teaching Informatics program at a Portuguese university, Piedade et al. (2020) investigated the self-confidence of PSTs to use educational robotics to teach computational thinking and programming. In the course, PSTs created a learning scenario (real or artificial situation) about how robotics could be used to teach computer science content to K-12 students. The results of the self-report scale indicated PSTs exhibited high interest and self-efficacy to teach with robotics as well as gained greater knowledge about robotics and problem-solving suggesting that collaborative, problem solving activities such as planning, designing and implementing scenarios with robots also supports the development of PSTs confidence to teach with robotics.
The few studies reported above show the positive impact that structured, hands-on programs can have on PST self efficacy to teach with robotics. However, two of the studies above are in ECE/primary contexts and the studies occurred in European and Australian universities. There is therefore a need for more studies in different contexts to examine the process of developing elementary preservice teacher self-efficacy to teach with robotics during the Teacher Education program years, so novice teachers go into the field with knowledge and some degree of self-efficacy to teach STEM with robotics. The current study extends the literature by investigating elementary, PSTs confidence to use robotics-based activities in teaching at a Canadian university.
3. Methodology
The intent of this study was to explore which type of instructional intervention with robotics-based activities – scaffolded learning approach/intervention or self-guided learning approach/intervention (with minimal scaffolding) – supported preservice teachers (PSTs) learn science content knowledge and develop their self-efficacy to teach with robotics. As such, a quasi-experimental, pretest-posttest non-equivalent groups intervention study (Price et al., 2015) was implemented with two pre-existing classes of elementary preservice teachers (PSTs) in a Bachelor of Education (B.Ed.) program at a Canadian university. One group (n = 11) engaged in self-guided learning, following the visual computer instructions on the robotics application, while the second group (n = 16) received scaffolding in the form of instructor facilitated pre-activity discussion and content worksheets that supplemented computer instructions. A description of and the differences between the interventions is provided in the section below.
3.1. Participants
After Ethics approval was obtained from the university Research Ethics Office, PSTs in the two sections of the elementary science methods course were invited to participate in the study by a research assistant. All PSTs participated in the robotics activity as part of requirements in the course curriculum; however, participation in the research study was voluntary and non-participation in the study did not affect PSTs course grades. PSTs were either in the fifth year of a 6-year, concurrent undergraduate degree/B.Ed. degree program or in year one of a two-year B.Ed. degree (students have completed a 4-year undergraduate degree). For most B.Ed. courses, PSTs from both university entry pathways take the same courses to obtain their primary-junior (PJ) teaching certification (teaching grades K-6). PSTs in the PJ program have completed a variety of undergraduate degrees and have majors in subjects such as history, English, math, early childhood education and environmental science and are expected to be generalists and teach all subjects in grades K-6. Hence, majority of the participants in the two groups did not have science majors or had not taken any high school specialist STEM subjects such as physics and computer science.
3.2. Curriculum context and instructional interventions
The PJ science methods course was designed to prepare PSTs to teach science outcomes from grades 1–6 as outlined in the Ontario Science and Technology Curriculum, Grades 1–8 (2007). The focus was on how to teach curriculum content through scientific inquiry and engineering design to solve practical problems. In line with the widespread call for STEM integration over the past 10 years, many Ontario school boards such as the Toronto District School Board (TDSB) began implementing STEM professional learning with robotics for elementary teachers (Sinay et al., 2016). It was in the context of this provincial professional learning initiative for teachers that the robotics-based activity was introduced in this B.Ed. course. The goal of the robotics-based activity was to learn science content through building and programming with robotic kits and associated computer applications – emphasizing the S, T and E in STEM. Since the curriculum expectations for the activity were about learning gear types and their functions from the grade 4 curriculum, the robotics kits used were LEGO® Education WeDo v.1.2, designed for elementary students with easy-to-follow visual computer instructions to build and program models that are not very complicated.
3.3. Internal validity threats
The instructional interventions with the two classes of PSTs were implemented by the author on the same day minimizing diffusion of treatment effects between groups. While classes were at different times, PSTs had fixed timetables with three-hour classes scheduled back-to-back which minimized interactions between students during classes. The two classes were taught by two different instructors during the semester. During the three-hour intervention, course instructors observed the author facilitate the activity because they were not familiar with the robotics kit and coding. They assisted with setting up kits and administration of the pre and posttests. PSTs worked in pairs in both interventions, and in a group of 3 if an odd number of students was present. Since all PSTs did not have any courses on robotics and computer programming in the B.Ed. program, the author began both group interventions with a brief PowerPoint introduction about robotics and its applications, some information on coding such as algorithms and debugging, and an overview of the WeDo program. A copy of the WeDo programming key guide was also provided to PSTs in both groups.
3.4. Self-guided robotics intervention
In the self-guided robotics intervention (n = 11), PSTs worked with robotics kits set up at tables in a private, but open area in the Education resource center/library. A task card at each table provided information on the curriculum outcomes for the activity related to which gear types and functions to explore, and what robot model to build and program on the WeDo computer application.
The task card also listed the general STEM process goals:
• Think creatively to make a working model.
• Develop vocabulary and communication skills to explain how the model works.
• Establish links between cause and effect.
• Follow 2D drawings to build a 3D model.
• Think logically and create a code to produce a specific behavior.
Participants were left to work at their own pace to follow the computer instructions to build gear models and robot models and program them. For example, the instruction on the computer screen to build a gear model illustrating gearing down was a visual diagram (Figure 1) and the instruction to follow to program a model called the Dancing Birds, that incorporated gears, was another visual as shown in Figure 2. No other type of information was provided on the computer or by the instructor/author. The author responded when PSTs asked for assistance about how to use the program. No other pedagogical scaffolds were incorporated during the activity by the author.
3.5. Scaffolded robotics intervention
In the scaffolded robotics intervention class (n = 16), PSTs received additional scaffolding through oral discussion and demonstration provided by the instructor and then through a worksheet. The instructor scaffolding was in line with constructivist learning; the instructor probed for prior knowledge of gears and gear functions though oral questioning and provided a brief recap of gear types with the aid of visuals on a PowerPoint. PSTs then followed instructions on the exploring gear worksheet (see Figure 3) that is an instructor support with the WeDo application. Questions 1 to 4 on this worksheet directed PSTs to the model-building visual on the computer and supplemented the computer visuals with verbal directions for building the gear model, Questions a to f in Figure 3 were discussion questions that acted as conceptual scaffolds to engage PSTs in developing knowledge about gears and their functions.
PSTs were guided to build four different models of gears (i.e., exploring two gears, idler gear, gearing down and gearing up). As PSTs followed the worksheet directions and constructed the different gear models, they were then asked to program, test their gear models and record their observations on the worksheet. During this process, they were introduced to gear terminology such as driver and follower gears on the worksheet. The worksheet also included math calculations to figure out gear ratios for gearing up and gearing down. After completing this activity to learn about gears, PSTs then followed instructions on the WeDo computer program to construct the same robot models as participants were asked to explore in the self-guided robotics intervention. The first model built by PSTs in the scaffolded group was the Dancing Bird and a preconstructed model was available to aid students who had difficulty visualizing from 2D to 3D. PSTs were provided with a process worksheet (See sample worksheet in Appendix 1) to scaffold the development of skills for programming/coding. PSTs followed the sequence of programming steps on the programming worksheet that modeled how to code, provided partial codes for debugging, and then asked students to produce their own codes.
3.6. Instruments
A knowledge test on how gears work and a self-efficacy for teaching robotics questionnaire were filled in by participants at the beginning of the intervention and the same measures were filled in by participants 1 week after the intervention, to minimize testing effects. Both measures were used in previous studies with PSTs (Jaipal-Jamani and Angeli, 2017). The knowledge test consisted of 5 questions which included what a gear is and its use, examples of objects that use gears, how a gear worked, how to make an object move faster using gears, and how to make an object move slower using gears. Each question was scored out of 3 from a precise scoring rubric. For example, for the question, how do gears work, the scoring was based on key words or ideas as follows: gears mesh and one gear (driver) drives/turns another gear (follower) (1 mark); driver gear is turned by an outside effort [power, input force, energy] (1 mark); output force/motion experienced by follower gear (1mark). The total test score was 15. The author scored items and then this scoring was validated by another university science educator who reviewed the scores of all PSTs. Any discrepancies in scoring were discussed to come to a consensus score. The interrater test scoring reliability was 94%.
3.7. Instrument validity and reliability
A Pearson Correlation analysis was conducted between the total scores and the five test items from pretest scores. The significance value for all five items in the scaffolded group was less than 0.05 indicating all items were valid for this group. The significance value for four out of five items (questions 2, 3, 4, and 5) in the self-guided group was less than 0.05 indicating validity of four items. All five items were retained for the calculations based on the reliability analysis outcomes. To measure reliability of the knowledge test, Cronbach’ s alpha was calculated to show the degree of internal consistency or how closely related the set of items were as a group for knowledge items. The Cronbach’s alpha obtained for the 5 items on the knowledge tests for the different groups were: self-guided group: post = 0.680; pre = 0.663; scaffolded group: post = 0.863 and pre = 0.809. Taber (2018b) points out that in Science Education studies, no clear consensus exists about what the most appropriate acceptability value for alpha is; however, the consensus value of 0.7 and over was considered acceptable. Nevertheless, Taber demonstrates and argues that Cronbach’s alpha values cannot be assumed to be fixed in a knowledge scale or instrument because it applies to a particular sample responding at a particular time so scales lower than 0.7 can by justified. The two groups in the current study came with different prior levels of knowledge which may have affected their responses in terms of random guesses or no responses at all resulting in low pretest alpha scores but with the intervention, reliability of items increased. Inconsistent and low values have been reported for other science knowledge tests (Berger and Hänze, 2015; Nehring et al., 2015) and Berger and Hänze (2015) explained low reliability values in their study in terms of the limited number of test items and the range of knowledge tested. This may be another possible factor that explains the lower reliability values in the current study as the test has 5 items only which may not be representative of a unidimensional construct as has been pointed out by Taber (2018b). Taber explains that items on a science test because it focuses on knowledge and understanding of sometimes complex science topics, may not be conceptualized well as a single, coherent construct. Lower reliability values for the self-guided group could be related to their prior knowledge and also could be due to the small sample size.
The self-efficacy for teaching measure consisted of 4 items that rated confidence on a scale from 0 to 100 (from not confident to completely confident). Items were (A) I feel confident that I have the skills necessary to use robotics for classroom instruction; (B) I feel confident that I can engage my students to participate in robotics-based projects; (C) I feel confident that I can help students when they have difficulty with robotics; and (D) I feel confident about teaching students’ science using WeDo. Pearsons correlation between the summed score and the 4 items from the pre-efficacy questionnaire revealed p < 0.05 for all four items in both PST groups. Cronbachs alpha values obtained for the four items were for self-guided group: posttest = 0.967; pretest = 0.970 and scaffolded group: posttest = 0.980; pretest = 0.936; indicating that the instrument reliability was good.
3.8. Data analysis
IBM SPSS Statistics version 27 was used to analyze the data. Normality tests were run using Shapiro Wilks test because of the small sample sizes (Ghasemi and Zahediasl, 2012). All statistical tests were conducted at the 95% confidence level.
3.8.1. Knowledge test
The scores of the 5 items on the knowledge test were summed to provide a knowledge pretest and posttest score. While the knowledge posttest scores in both groups were normal with p > 0.05 (self-guided group: p = 0.838; scaffolded group: p = 0.519), the knowledge pretest scores in both intervention groups were not normally distributed with Shapiro–Wilk significance value less than 0.05. Hence, the non-parametric Wilcoxon Signed test was used to compare knowledge gains within groups. For non-parametric test calculations, the effect size was calculated as recommended by the literature with the formular: r = z/√2n with values for r = 0.10 (small effect), 0.30 (moderate effect) and 0.5 (large effect; Fritz et al., 2012).
3.8.2. Self-efficacy questionnaire
To investigate PSTs self-efficacy gains within groups, scores of the four confidence items for each group were summed to provide a pre and post self-efficacy score. The distribution of the pre and post self-efficacy scores were normal for both groups with Shapiro–Wilk test significance showing p > 0.05 as follows:
• scaffolded group – pre score p = 0.383, post score p = 0.083.
• self-guided group – pre score p = 0.109, post score p = 0.088.
Therefore, a paired T-test was used to compare pre and post changes in self-efficacy and effect size was calculated using Cohen’s d where d = 0.2 (small effect), d = 0.5 (medium effect), and d = 0.8 (large effect) as suggested by Cohen (1988).
4. Results
4.1. Knowledge of gears: Within group comparison
4.1.1. Self -guided robotics intervention
Null Hypothesis: The median difference between knowledge pretest and posttest scores is symmetric around zero.
Alternate Hypothesis: The median difference between knowledge pretest and posttest scores is not symmetric around zero.
Results from the Wilcoxon Signed Test showed that the posttest knowledge of gears (mean rank = 6.07) was not significantly different compared to pretest knowledge of gears (mean rank = 4.17) with z = − 1.551, p = 0.148. The null hypothesis is not rejected, and the findings suggest there is no statistically significant gain in science knowledge by PSTs due to the self-guided intervention at α = 0.05 to show that the median difference between pre and post knowledge is not symmetric around zero. The median of the score differences was 1 (Table 1). Three PSTs had negative median differences meaning that they scored lower in the posttest than in the pretest (Table 2). The findings therefore indicate that there was no significant gain in knowledge by PSTs due to the self-guided intervention.
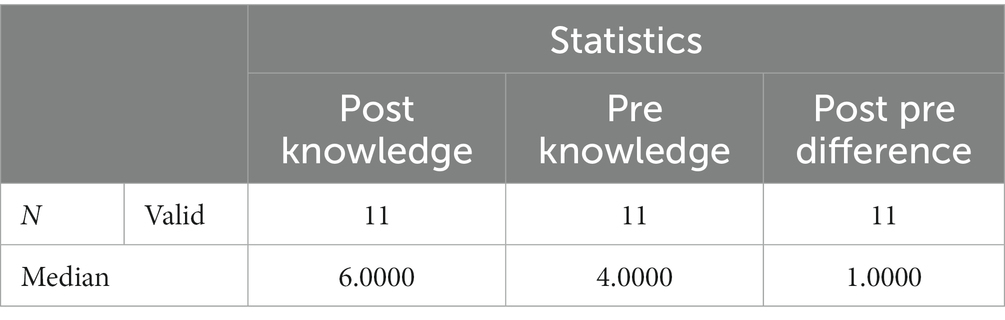
Table 1. Median values for posttest, pretest, and differences in post-pretest knowledge scores for self-guided group.
4.1.2. Scaffolded robotics intervention
Null Hypothesis: The median difference between knowledge pretest and posttest is symmetric around zero.
Alternate Hypothesis: The median difference between knowledge pretest and posttest is not symmetric around zero.
A Wilcoxon signed-rank test showed a significant median difference between posttest knowledge scores (mean rank = 8) when compared to pretest knowledge scores (mean rank = 0.00) with z = −3.428, p < 0.001. Since p is statistically significant, the null hypothesis is rejected indicating there were significant differences between pretest and posttest knowledge scores. Table 3 shows that the median of the differences in scores was 3 and as seen in Table 4, 15 PSTs out of 16 had positive ranks with median difference in pre-post knowledge scores greater than zero. The findings suggest that there was a significant gain in knowledge by PSTs due to the scaffolded intervention. The effect size was r = z/√2n; r = 3.428/√32 = 0.607 which indicates a large effect size suggesting that the scaffolded intervention did have practical significance for PSTs learning of science content.
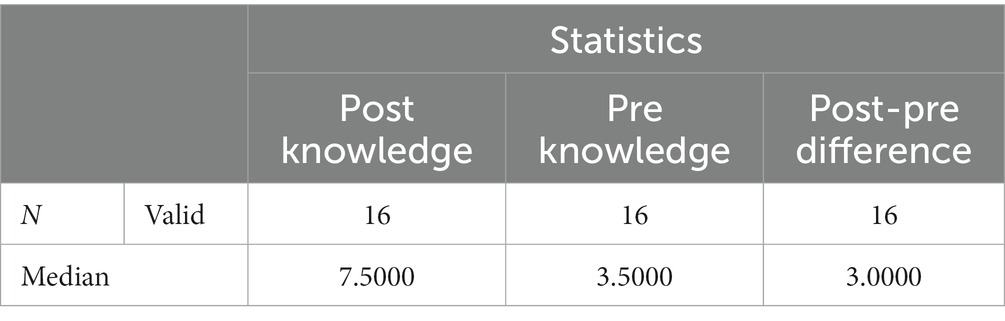
Table 3. Median values for posttest, pretest, and differences in post-pretest knowledge scores for scaffolded Group.
4.2. Self-efficacy to teach science using robotics: Within group comparison
4.2.1. Self-guided robotics intervention
Null Hypothesis: Ho: A self-guided robotics intervention has no effect on preservice teachers’ self-efficacy to teach with robotics-based activities.
Alternate Hypothesis: A self-guided robotics intervention has a positive effect on preservice teachers’ self-efficacy to teach with robotics-based activities.
A paired samples T-Test at the 95% confidence level showed there was a significant increase in PST self efficacy to teach with robotics a week after the self-guided intervention (M = 27.9, SD = 11.3) compared to before the self-guided intervention (M = 18.7, SD = 7.8), t (10) = 4.635, p < 0.001. The null hypothesis is rejected, and results indicate that the self-guided intervention had a positive effect on PST self-efficacy. Mean change was from 18.7 to 27.9 with the difference being 9.2 (Table 5). Cohen’s d was 1.398 indicating a large effect size that was illustrated in practice. As shown in Table 6, positive ranks were 11 indicating that all participants reported their self-efficacy as higher after the self-guided intervention. The results suggest that the self-guided intervention increased PST self-efficacy to teach using robotics-based activities.
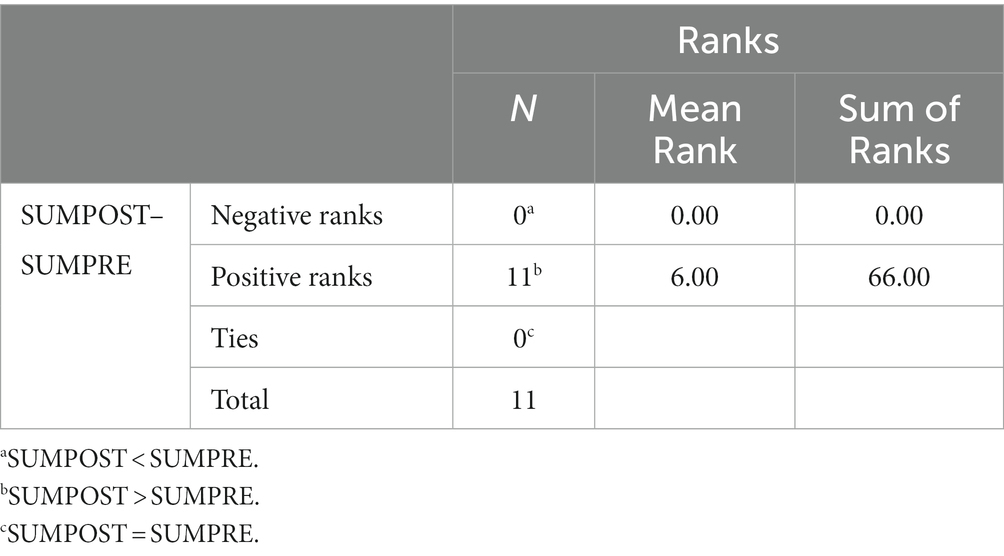
Table 6. Positive, negative, and tied paired differences in PST self-efficacy scores for self-guided group.
4.2.2. Scaffolded robotics intervention
Null Hypothesis: A scaffolded robotics intervention has no effect on preservice teachers’ self-efficacy to teach with robotics-based activities.
Alternate Hypothesis: A scaffolded robotics intervention has a positive effect on preservice teachers’ self-efficacy to teach with robotics-based activities.
A paired samples T-Test at the 95% confidence level showed there was a significant increase in PST self-efficacy to teach with robotics after the scaffolded intervention (M = 27.4, SD = 8.8) compared to before the scaffolded intervention (M = 11.7, SD = 10.3), t (15) = 6.598, p < 0.001. The null hypothesis is rejected, and results indicate that the scaffolded intervention had a positive effect on PST self-efficacy. Mean change was from 11.7 to 27.4 with the difference being 15.7 (Table 7). Cohen’s d was 1.649 indicating a large effect size. Table 8 also shows that 15 PSTs had positive differences in the ranked score differences meaning that they reported higher efficacy scores after the intervention suggesting that the scaffolded intervention had a large practical effect resulting in an increase of PST self-efficacy to teach using robotics-based activities.
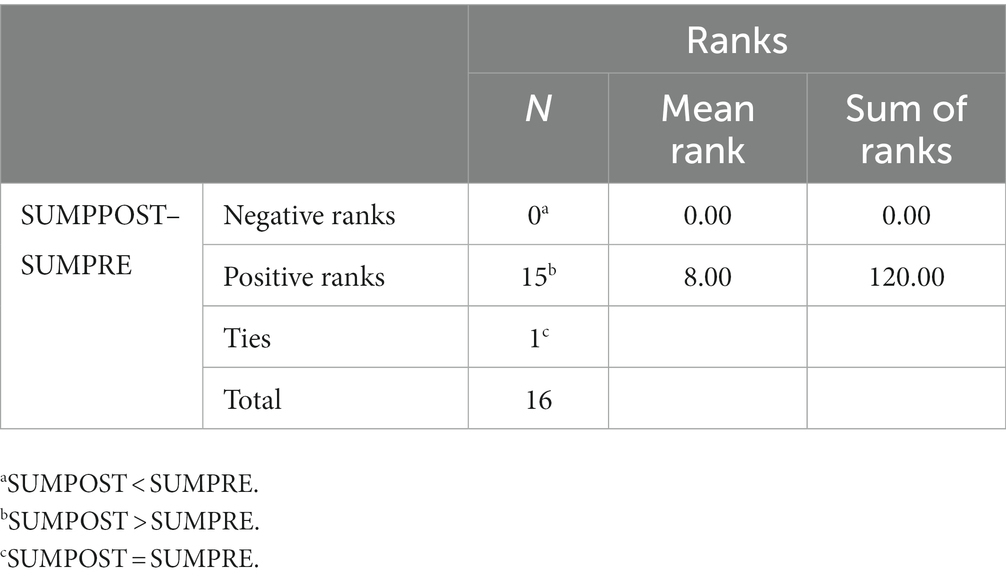
Table 8. Positive, negative, and tied paired differences of PST self-efficacy scores for scaffolded group.
5. Discussion
This pre-post intervention with two different groups of elementary preservice teachers in the same Bachelor of Education program investigated the effectiveness of a self-guided instructional approach and a scaffolded instructional approach to learn science knowledge through a robotics-based activity. The study also investigated the effect of these two instructional approaches to develop elementary PSTs self-efficacy to teach with robotics-based activities.
The findings in relation to knowledge gains were statistically significant for the effect of the scaffolded robotics approach on gains in science knowledge for this sample of PSTs with a high effect size of 0.607 suggesting high practical significance of the scaffolded robotics intervention to support content learning of science. In comparison, the findings showed that the self-guided robotics intervention did not result in statistically significant gains in knowledge of gears among this sample of elementary PSTs. While PSTs were not assigned to the two intervention groups randomly because of pre-established student course timetables, the PSTs in both groups in this study exhibited similar prior knowledge of gear concepts as evidenced by the pretest medians (self-guided = 4 and scaffolded = 3.5). So, it is reasonable to conclude that the main difference between the two groups was the scaffolding provided to one group and not the other. In the scaffolded PST intervention, the author/instructor facilitated pre-discussion of gears and a gear worksheet engaged PSTs during the activity in thinking about how gears worked. These scaffolds were not provided in the self-guided intervention group. The self-guided PST group only received the visual scaffolding on the computer in relation to gear functions and there were no built-in prompts or questions in the computer program to engage PSTs to reflect on gear concepts as they constructed and programmed the gear models. The study results therefore suggest that the scaffolding provided during the robotics activity played a role in enhancing PSTs knowledge of science concepts. Notably, the questions on the worksheet supported development of conceptual knowledge in a series of steps and acted as conceptual scaffolds for learning about gears (Belland et al., 2017). The worksheet, coupled with the hands-on experience of constructing, programming, and testing the different gear models also enabled the preservice teachers in the scaffolded group to learn new scientific terminology such as gearing up and gearing down. Although the visual instructions on the computer program introduced scientific terminology such as gearing down to both groups as illustrated in Figure 1, none of the PSTs in the self-guided group included these terms in their posttest response for knowledge questions 4 and 5 (which asked about gears moving slower and faster respectively). However, in the posttest responses of the scaffolded group, 8 out of 16 PSTs used the terms gearing down and gearing up in their responses to these two questions. This qualitative observation suggests that the process worksheet as a scaffold reinforced the connection between the scientific term associated with the scientific phenomenon for at least 50% of PSTs in the scaffolded group and strengthens the practical significance of the scaffolded intervention for PSTs learning of science content.
The study result, that the PSTs in the scaffolded robotics group showed statistically significant gains in knowledge compared to PSTs in the self-guided robotics group, supports other findings in the literature about the effectiveness of scaffolding for students with little prior knowledge (Kirschner et al., 2006; Oksa et al., 2010; Kalyuga, 2013). As mentioned above, most PSTs in both intervention groups had little prior knowledge of how gears worked, except for a few PSTs who came with some science background. In these cases, students with prior knowledge of science and/prior experiences with robotics supported peers during construction and collaborative discussions and mediated construction of knowledge. With respect to how the different instructional approaches affected the learning of science concepts and skills particularly, the findings support results obtained by Klahr and Nigam (2004). Klahr and Nigam (2004) found that oral scaffolding by the teacher resulted in more gains in elementary students’ science learning than a discovery/self-guided intervention. Although Klar and Nigam labelled the instructional approach with teacher scaffolding, direct instruction, the teacher actions—of providing different examples of the phenomenon and explanations for the differences—can be argued to be an example of the demonstration scaffolding function by Wood et al. (1976).
Demonstration includes examples or partial solutions being modelled through teacher oral explanation and findings suggest scaffolding contributed to greater gains in student learning of science compared to the self-guided approach. It should be noted that in practice, teachers may use a mix of the self-guided approach and the scaffolded approach in teaching to promote the learning of different competencies in students. For example, if the intention is to have students learn about gearing up and gearing down, then the scaffolded approach with targeted questions is likely to result in students gaining that specific knowledge as shown in this study. A self-guided approach may be effective to gain a different set of competencies such as problem-solving skills (Mayer, 2004) or practical knowledge when exploring a new topic such as which gears make robots work best. In these latter instances, the knowledge test would be designed to test these problem-solving competencies or knowledge derived from the practical activities. In the current study, the author’s intention was to explore the effectiveness of the self-guided and scaffolded approach to develop knowledge of gear concepts through a robotics program, so the same knowledge test was administered to both groups. This study results provide insights about the effectiveness of the two instructional approaches for learning specific gear concepts and may assist PSTs and novice teachers during instructional design related to this topic.
The finding of the current study that the self-guided robotics intervention did not result in statistically significant gains in knowledge also emphasizes the significance of built-in computer scaffolds for self-guided learning. While the WeDo visual scaffolds were easy to follow to build and program simple models, the WeDo program itself was not effective at scaffolding learning of the science concepts in this study. This limitation in the robotics program becomes important when an experienced teacher or tutor is not available to provide supplementary scaffolding which may be the case for learners who for example, are homeschooled or engage in distance learning. Studies have shown that computer programs that incorporate different types of scaffolds, e.g., conceptual scaffolds such as links or hints to experts and metacognitive scaffolds such as prompts to self-reflect on learning resulted in improved cognitive outcomes in STEM (Belland et al., 2017; Kim et al., 2018). It is therefore important for science educators and practicing teachers to explore the robotics computer programs they incorporate to teach disciplinary STEM content to PSTs and K-12 students, respectively. Identifying the types of scaffolding that are built-in to computer applications associated with robotics kits will enable educators to provide the additional conceptual, metacognitive and strategic scaffolds necessary to support learning of STEM content knowledge and skills.
The second research question investigated how the self-guided instructional approach and the scaffolded instructional approach with robotics-based activities affected elementary preservice teachers’ self-efficacy to teach with robotics. The results indicated that both interventions were statistically significant for gains in self efficacy with p < 0.001 but the effect size was higher in the scaffolded group (d = 1.649) compared to the self-guided group (d = 1.398) suggesting the scaffolded intervention was more effective at developing PST self-efficacy to teach with robotics-based activities. To explain the practical significance of the results, it is important to look at some of the characteristics of PSTs in the two groups. Some PSTs explained during the activity that they had some prior exposure or experiences with robotics activities in high school or by facilitating robotics-based activities at summer camps. It was observed that during the activity, more knowledgeable peers with ER assisted peers who had minimal or no experiences with robotics in both the self-guided and scaffolded interventions. In the self-guided group, 6 out of 11 PSTs (55%) rated their pre-efficacy teaching with robotics as high (high was arbitrarily selected as having 50% or greater summed efficacy score) and 4/16 PSTs (25%) in the scaffolded group rated their pre-efficacy as high. The pretest self-efficacy mean was 18.7 for the self-guided group and was 11.7 for the scaffolded group. It is not unreasonable to assume that having prior hands-on experiences working with robotics kits may have led to some PSTs rating their perceptions of their self-efficacy to use robotics in classroom instruction prior to the interventions higher. However, PSTs confidence to teach with robotics post intervention for both groups were similar, with means around 27 (the maximum being 40) suggesting that the self-guided intervention gains were smaller. This observation supports the results that the scaffolded intervention had a greater effect on PSTs self-efficacy to teach with robotics in this study. When scaffolds such as conceptual worksheets are used in robotics activities, they also model how learning is scaffolded in K-12 classroom settings. This study finding is consistent with the findings of Blackley et al. (2017) who found that when PSTs were explicitly taught about pedagogical scaffolding, it increased their confidence to facilitate robotics-based activities with elementary school students. The lack of explicit pedagogical strategies to make connections to targeted curricular concepts was also not explicit in the self-guided intervention and may have contributed to PSTs smaller gains in confidence to use robotics in their future teaching. It has been shown that when PSTs are exposed to structured, hands-on learning experiences with robotics that have strong connections to the K-12 curriculum, PST confidence to teach with robotics increased (Martin et al., 2020; Schina et al., 2021).
It is also important to analyse the nature of the scaffolding during the robotics activity to understand the role played by different scaffolding types to support learning. The scaffolded intervention in this study incorporated the scaffolding functions as identified by Wood et al. (1976). The scaffolding function, recruitment was done by using the robotics kits and visual programming blocks on screen to gain PSTs interest in the task; direction maintenance was facilitated by asking PSTs to focus on completing the assigned tasks on the computer and challenges on the worksheet; demonstration was done by showing a preconstructed model of the Dancing Birds and by modeling partial solutions on the programming worksheet; reducing degrees of freedom was reflected in the incremental nature of the task from simple to complex as shown on the gears worksheet and part 1of the programming worksheet; marking critical features occurred when learners wrote down a code, tested the program and received instant feedback on screen to correct their written code if necessary; and frustration control was built in as worksheet steps built on each other incrementally to minimize frustration and the instructor was there to help when asked. As illustrated above, the scaffolded robotics intervention incorporated Woods et al.’s scaffolding strategies which has been shown to contribute to gains in knowledge and self-efficacy. Some implications for teaching practice are that it may be beneficial to incorporate different types of scaffolding to support learning during a robotics-based activity. The scaffolded robotics intervention also modelled temporary adaptive support (Shvarts and Bakker, 2019) as the programming worksheet was designed to decrease or fade modelling and support so that the PSTs could complete the coding task independently as they progressed (Pea, 2004; Puntambekar and Hubscher, 2005). Through completing this worksheet task, PSTs also learned how to self-regulate and perform tasks independently (Shvarts and Bakker, 2019). Most importantly, the various types of scaffolding were being experienced by these elementary PSTs as they were learning through first-hand experience, how to teach with robotics-based activities to scaffold elementary students’ science learning. Palmer (2011) also demonstrated that it was through a combination of strategies that included explicit demonstration of how to teach the science activity, observation of others teaching the activity and then practicing it themselves that enhanced novice elementary teachers’ self-efficacy to teach science.
6. Limitations of the study
The study results are exploratory as they are based on a quasi-experimental design since it was not possible to create two random groups. Students were in pre-established, self-selected classes and the robotics activity was part of the course curriculum. Since the sample sizes were based on the pre-established class sizes, a limitation of the study is that the sample sizes are small and study findings are not generalizable. Results are interpreted and discussed in relation to this study sample and context. In terms of gender representation, the samples were from the same population of PSTs in an elementary Teacher Education program with similar approximation of gender within each sample: 3/16 males (19%) in the scaffolded group and 2/11 (18%) males in the self-guided group. Self-efficacy measures were also self-reports from preservice teachers so PSTs could have over-estimated or underestimated their confidence during the pretest. Confounding variables such as PSTs prior content knowledge and PSTs prior experiences with robotics can contribute to measurement errors decreasing the robustness of results (Jennings and Cribbie, 2016). To minimize the measurement errors that arose due to pre-existing group differences, the t-test (and its non-parametric counterpart) was used to compare pre-post changes (Jamieson, 2004). It has been shown that in a two-phase design with a single dependent variable, a difference test, when compared to an ANCOVA, is more effective as it is less affected by baseline group differences, has less bias and demonstrates good statistical power even when reliability is low (Thomas and Zumbo, 2012; Jennings and Cribbie, 2016). All knowledge test items were valid in both groups except for question one in the self-guided group. This suggests that question 1 was not a useful item for the self-guided group and this may have been due to the differences in characteristics of this group compared to the scaffolded group – all PSTs in the self-guided group were able to answer question 1 but there were some PSTs in the scaffolded group who did not know the answer to question 1. The knowledge test would therefore need to be modified for future group comparison studies. Question 1 was not removed as five items yielded the best validity and reliability results across both groups. Another limitation of the study was that the posttest was done 1 week later, and the same measure may elicit different responses from PSTs after a longer time interval. It is also the case that participants were elementary pre-service teachers, therefore results may not be applicable to secondary preservice teachers at this university as the factors affecting self-efficacy for the different groups may be different (Morris et al., 2017). Another limitation is that three of the four self-efficacy questions were phrased to elicit responses about robotics in general when PSTs were exposed to one type of robotics kit for use in gear-related building and programming activities. The scale was on a continuum from 0 to 100 and provided PSTs with opportunities to express their feelings about being able to use a robotics kit in the classroom setting before and after the intervention. Since the study investigated how PSTs feelings changed after participation in the different gear-related activities, the difference in pre and post confidence results is interpreted in the context of PSTs being confident to use the robotics kit for similar types of building and programming and is not interpreted as being applicable to teaching robotics in general. The wording of self-efficacy items will be modified to refer to the specific robotics activity in future studies. A final critique that could be made of this study is that all areas of STEM were not investigated even though robotics-based activities support STEM learning. In practice, robotics is generally integrated into disciplinary curricular, and the way teachers in the field tend to approach instructional design is to use the robotics-based activities as the context to meet disciplinary curriculum learning outcomes (Ntemngwa and Oliver, 2018). Due to the practicalities of how subjects are taught at schools, the science methods course was also focused on teaching PSTs how to teach science and general STEM skills. The nature of the course therefore limited the scope of the study investigation.
7. Recommendations for future research
This research study was limited to investigating robotics-based activities for enhancing science content outcomes, specifically gears, and technology and engineering skills as part of a PST course in elementary science education. Future studies are needed to demonstrate how longer robotics-based activity interventions affect PST content knowledge and self-efficacy gains and with different topics. PSTs self-efficacy can also be measured at different times to assess the impact of the intervention. It would also be beneficial to observe how PSTs implement robotics in teaching practice to investigate the factors that affect implementation and how this may affect self-efficacy to teach with robotics. Such studies would also enhance the findings and provide illuminating insights about how K-12 students learn with robotics in similar scaffolded interventions. Field observations were not possible in this study as PSTs were on summer break after the science methods course and commenced the next university semester in the fall. It is also the case that all schools do not have robotics kits to support teacher integration of robotics-based activities in classes. Studies are also needed to explore how scaffolded robotics-based activities can be used in different disciplinary areas to enhance knowledge of disciplinary and integrated STEM knowledge. It may be that investigating all aspects of STEM is best done in an integrated STEM course that is not focused on core disciplinary knowledge. Such investigations are possible in schools where STEM is taught as a distinct course or STEM is taught in an integrated manner. Such findings may promote the implementation of integrated STEM courses in more educational contexts, especially if research shows that students are gaining STEM knowledge and skills that enable them to participate in emerging technology fields such as biotechnology and automation. Robotics-based activities have potential to also foster innovation and creativity among learners. An interesting observation made in the self-guided group was that some PSTs chose to build their own models and create their own programs. This creative building was not observed in the scaffolded group. An interesting area for future research would be to investigate conditions for enhancing development of innovation and creativity during robotics activities as these are essential skills for future economies and societies (Vincent-Lancrin et al., 2019).
8. Conclusion
Overall, the study results show that elementary PSTs experiences with robotics-based activities through a scaffolded instructional approach improved both their learning of science content knowledge and their reported confidence to teach with robotics-based activities in future practice. PSTs in the self-guided robotics-based intervention experienced gains in self-efficacy to teach with robotics but gains were smaller compared to PST self-efficacy gains in the scaffolded group. Enhanced teacher knowledge and confidence contributes to robotics adoption in K-12 schools and to the development of STEM skills in K-12 students (Sinay et al., 2016). Experiencing how content and programming knowledge is scaffolded for student learning in a robotics-based activity serves as a model for pedagogy in the K-6 classroom and could be a factor that may have contributed to greater gains in PST confidence to teach with robotics in the scaffolded group compared to PSTs in the self-guided group. These results have implications for science teacher educator’s instructional practices and practicing teachers’ professional development. The findings emphasize the importance of modeling scaffolding strategies for teaching robotics so preservice and in-service teachers experience them and can reflect on its use to teach STEM content and skills in elementary classrooms. Teacher educators that want to integrate robotics-based activities into their courses should demonstrate or model to PSTs how to make connections between curriculum knowledge and the robotics activity so PSTs can make those same connections for their students in the classroom.
Scaffolding strategies should therefore be demonstrated or modelled with authentic examples from the elementary school curriculum relevant to the context. While in-service teachers are already familiar with the curriculum and many can make links to how robotics can be used to support curriculum outcomes (Chambers and Carbonaro, 2003), in-service teachers may not be familiar with the different types of scaffolds that are associated with computer applications and programming. The current study can inform the design of robotics professional development workshops or courses to highlight the different types of scaffolds that can be used to enhance student learning during robotics-based activities. The more experiences PSTs and practicing teachers, especially non-specialist STEM teachers, have with scaffolded robotics-based activities in STEM disciplinary contexts, the greater the potential for them to teach with and integrate robotics-based activities in future teaching practice.
Data availability statement
The datasets presented in this article are not readily available because as per university ethics approval, data sets are not shared. Requests to access the datasets should be directed to a2phaXBhbEBicm9ja3UuY2E=.
Ethics statement
The studies involving human participants were reviewed and approved by Brock University Research Ethics Office. The patients/participants provided their written informed consent to participate in this study.
Author contributions
The author confirms being the sole contributor of this work and has approved it for publication.
Funding
The study was funded by the Deans office in the Faculty of Education, Brock University and by the Brock Library Open Access Publishing Fund.
Conflict of interest
The author declares that the research was conducted in the absence of any commercial or financial relationships that could be construed as a potential conflict of interest.
Publisher’s note
All claims expressed in this article are solely those of the authors and do not necessarily represent those of their affiliated organizations, or those of the publisher, the editors and the reviewers. Any product that may be evaluated in this article, or claim that may be made by its manufacturer, is not guaranteed or endorsed by the publisher.
References
Adedokun, O. A., Bessenbacher, A. B., Parker, L. C., Kirkham, L. L., and Burgess, W. D. (2013). Research skills and STEM undergraduate research students' aspirations for research careers: mediating effects of research self-efficacy. J. Res. Sci. Teach. 50, 940–951. doi: 10.1002/tea.21102
Alfieri, L., Brooks, P. J., Aldrich, N. J., and Tenenbaum, H. R. (2011). Does discovery-based instruction enhance learning? J. Educ. Psychol. 103, 1–18. doi: 10.1037/a0021017
Alimisis, D. (2012). “Robotics in education & education in robotics: shifting focus from technology to pedagogy” in Proceedings of the 3rd international conference on robotics in education. ed. D. Obdrzálek (Prague, Czech Republic: Charles University in Prague), 7–14.
Anwar, S., Bascou, N. A., Menekse, M., and Kardgar, A. (2019). A systematic review of studies on educational robotics. J. Pre-Coll. Eng. Educ. Res. 9:2. doi: 10.7771/2157-9288.1223
Anyfandi, G., Filippoupoliti, A., and Alexopoulos, I. (2013). Engineer project: breaking new ground in the science education realm. Strand 9 Environ. Health Outdoor Sci. Educ. 145–157.
Bandura, A. (1977). Self-efficacy: toward a unifying theory of behavioral change. Psychol. Rev. 84, 191–215. doi: 10.1037/0033-295X.84.2.191
Bandura, A. (1994). “Self-efficacy” in Encyclopedia of human behavior. ed. V. S. Ramachaudran, vol. 4 (New York: Academic Press), 71–81.
Becker, K. H., and Park, K. (2011). Integrative approaches among science, technology, engineering, and mathematics (STEM) subjects on students’ learning: a meta-analysis. J. STEM Educ. Innovat. Res. 12, 23–37.
Belland, B. R., Walker, A. E., Kim, N. J., and Lefler, M. (2017). Synthesizing results from empirical research on computer-based scaffolding in STEM education: a meta-analysis. Rev. Educ. Res. 87, 309–344. doi: 10.3102/0034654316670999
Benitti, F. B. V. (2012). Exploring the educational potential of robotics in schools: a systematic review. Comput. Educ. 58, 978–988. doi: 10.1016/j.compedu.2011.10.006
Berger, R., and Hänze, M. (2015). Impact of expert teaching quality on novice academic performance in the jigsaw cooperative learning method. Int. J. Sci. Educ. 37, 294–320. doi: 10.1080/09500693.2014.985757
Blackley, S., Sheffield, R., Maynard, N., Koul, R., and Walker, R. (2017). Makerspace and reflective practice: advancing pre-service teachers in STEM education. Australian J. Teach. Educ. 42, 22–37. doi: 10.14221/ajte.2017v42n3.2
Bybee, R. (2010). Advancing STEM education: a 2020 vision. Technol. Eng. Teach. 70, 30–35. doi: 10.1126/science.1194998
Carnevale, A. P., Smith, N., and Melton, M. (2011). STEM: Science technology engineering mathematics. Washington, DC: Georgetown University Center on Education and the Workforce.
Chambers, J. M., and Carbonaro, M. (2003). Designing, developing, and implementing a course on LEGO robotics for technology teacher education. J. Technol. Teach. Educ. 11, 209–241.
Ching, Y. H., Yang, D., Wang, S., Baek, Y., Swanson, S., and Chittoori, B. (2019). Elementary school student development of STEM attitudes and perceived learning in a STEM integrated robotics curriculum. TechTrends 63, 590–601. doi: 10.1007/s11528-019-00388-0
CMEC (Council of Ministers of Education, Canada) (1997). Common framework of science learning outcomes, K to 12: Pan-Canadian protocol for collaboration on school curriculum for use by curriculum developers. Toronto, ON: CMEC
Cohen, J. (1988). The analysis of variance and covariance. Statistical power analysis for the behavioural sciences. Mahwah, NJ: Lawrence Erlbaum Associates.
Eguchi, A. (2016). “Educational robotics as a learning tool for promoting rich environments for active learning (REALs)” in Human-computer interaction: concepts, methodologies, tools, and applications. ed. M. Khosrow-Pour (Hershey, PA: IGI Global), 740–767.
Eguchi, A. (2021). “Theories and practices behind educational robotics for all” in Handbook of research on using educational robotics to facilitate student learning. eds. S. Papadakis and M. Kalogiannakis (Hershey, PA: IGI Global), 68–106.
Estes, J. S. (2019). “A call for teacher preparation programs to model technology integration into the instructional process” in Pre-service and in-service teacher education: Concepts, methodologies, tools, and applications. ed. M. Khosrow-Pour (Hershey, PA: IGI Global), 1442–1458.
Fanchamps, N. L., Slangen, L., Hennissen, P., and Specht, M. (2021). The influence of SRA programming on algorithmic thinking and self-efficacy using Lego robotics in two types of instruction. Int. J. Technol. Des. Educ. 31, 203–222. doi: 10.1007/s10798-019-09559-9
Fridberg, M., Redfors, A., Greca, I. M., and Terceño, E. M. G. (2022). Spanish and Swedish teachers’ perspective of teaching STEM and robotics in preschool–results from the botSTEM project. Int. J. Technol. Des. Educ. 33, 1–21. doi: 10.1007/s10798-021-09717-y
Fritz, C. O., Morris, P. E., and Richler, J. J. (2012). Effect size estimates: current use, calculations, and interpretation. J. Exp. Psychol: General 141, 2–18. doi: 10.1037/a0024338
Ghasemi, A., and Zahediasl, S. (2012). Normality tests for statistical analysis: a guide for non-statisticians. Int. J. Endocrinol. Metab. 10, 486–489. doi: 10.5812/ijem.3505
Glezou, K. V. (2021). “Robotics as a powerful vehicle toward learning and computational thinking in secondary education of 21st century” in Handbook of research on using educational robotics to facilitate student learning. eds. S. Papadakis and M. Kalogiannakis (Hershey, PA: IGI Global), 1–40.
Hammer, D. (1997). Discovery learning and discovery teaching. Cogn. Instr. 15, 485–529. doi: 10.1207/s1532690xci1504_2
Hamner, E., Cross, J., Zito, L., Bernstein, D., and Mutch-Jones, K. (2016). “Training teachers to integrate engineering into non-technical middle school curriculum” in 2016 IEEE Frontiers in education conference (FIE). (IEEE), 1–9.
Hannafin, M., Land, S., and Oliver, K. (1999). Open learning environments: foundations, methods, and models. Instructional-design theories and models: a new paradigm of instructional theory, Vol. 2. New York: Taylor and Francis, 115–140.
Hodges, C., Gale, J., and Meng, A. (2016). Teacher self-efficacy during the implementation of a problem-based science curriculum. Contemp. Issues Technol. Teach. Educ. 16, 434–451.
Holbert, N., Berland, M., and Kafai, Y. B. (Eds.). (2020). Designing constructionist futures: the art, theory, and practice of learning designs. Cambridge, MA: MIT Press.
Jaipal-Jamani, K., and Angeli, C. (2017). Effect of robotics on elementary preservice teachers’ self-efficacy, science learning, and computational thinking. J. Sci. Educ. Technol. 26, 175–192. doi: 10.1007/s10956-016-9663-zQ
Jamieson, J. (2004). Analysis of covariance (ANCOVA) with difference scores. International J. Psychophysiol. 52, 277–283.
Jennings, M. A., and Cribbie, R. A. (2016). Comparing pre-post change across groups: guidelines for choosing between difference scores, ANCOVA, and residual change scores. J. Data Sci. 14, 205–230. doi: 10.6339/JDS.201604_14(2).0002
Jonassen, D. H. (2011). Design problems for secondary students. Logan, Utah: National Center for Engineering and Technology Education.
Kalyuga, S. (2013). Effects of learner prior knowledge and working memory limitations on multimedia learning. Procedia Soc. Behav. Sci. 83, 25–29. doi: 10.1016/j.sbspro.2013.06.005
Kim, N. J., Belland, B. R., and Walker, A. E. (2018). Effectiveness of computer-based scaffolding in the context of problem-based learning for STEM education: Bayesian meta-analysis. Educ. Psychol. Rev. 30, 397–429. doi: 10.1007/s10648-017-9419-1
Kirschner, P., Sweller, J., and Clark, R. E. (2006). Why unguided learning does not work: an analysis of the failure of discovery learning, problem-based learning, experiential learning and inquiry-based learning. Educ. Psychol. 41, 75–86. doi: 10.1207/s15326985ep4102_1
Klahr, D., and Nigam, M. (2004). The equivalence of learning paths in early science instruction: effects of direct instruction and discovery learning. Psychol. Sci. 15, 661–667. doi: 10.1111/j.0956-7976.2004.00737.x
Kopcha, T. J., McGregor, J., Shin, S., Qian, Y., Choi, J., Hill, R., et al. (2017). Developing an integrative STEM curriculum for robotics education through educational design research. J. Formative Design Learn. 1, 31–44. doi: 10.1007/s41686-017-0005-1
Kyriazopoulos, I., Koutromanos, G., Voudouri, A., and Galani, A. (2022). Educational robotics in primary education: a systematic literature review. Research anthology on computational thinking, programming, and robotics in the classroom. Editor-in-Chief Mehdi Khosrow-Pour, 782–806.
Lemon, N., and Garvis, S. (2016). Pre-service teacher self-efficacy in digital technology. Teach. Teach. 22, 387–408. doi: 10.1080/13540602.2015.1058594
Martin, D. A., McMaster, N., and Carey, M. D. (2020). Course design features influencing preservice teachers’ self-efficacy beliefs in their ability to support students’ use of ICT. J. Digital Learn. Teach. Educ. 36, 221–236. doi: 10.1080/21532974.2020.1781000
Martinez, S. L., and Stager, G. (2013). “Invent to learn” in Making, tinkering, and engineering in the classroom (Torrance, ON: Constructing Modern Knowledge)
Mayer, R. E. (2004). Should there be a three-strikes rule against pure discovery learning? Am. Psychol. 59, 14–19. doi: 10.1037/0003-066X.59.1.14
Morris, D. B., Usher, E. L., and Chen, J. A. (2017). Reconceptualizing the sources of teaching self-efficacy: a critical review of emerging literature. Educ. Psychol. Rev. 29, 795–833. doi: 10.1007/s10648-016-9378-y
National Research Council. (2012). A framework for K-12 science education: practices, crosscutting concepts, and core ideas. Washington, DC: The National Academies Press. 602.
National Research Council (2014). STEM integration in K-12 education: status, prospects, and an agenda for research. Washington, DC: The National Academies Press. 605.
Nehring, A., Nowak, K. H., zu Belzen, A. U., and Tiemann, R. (2015). Predicting students’ skills in the context of scientific inquiry with cognitive, motivational, and sociodemographic variables. Int. J. Sci. Educ. 37, 1343–1363. doi: 10.1080/09500693.2015.1035358
Ntemngwa, C., and Oliver, J. S. (2018). The implementation of integrated science technology, engineering and mathematics (STEM) instruction using robotics in the middle school science classroom. Int. J. Educ. Math. Sci. Technol. 6, 12–40. doi: 10.18404/ijemst.380617
Oksa, A., Kalyuga, S., and Chandler, P. (2010). Expertise reversal effect in using explanatory notes for readers of Shakespearean text. Instr. Sci. 38, 217–236. doi: 10.1007/s11251-009-9109-6
Ontario Curriculum and Resources (2022). Grades 1–8: science and technology. Available at: https://www.dcp.edu.gov.on.ca/en/curriculum/science-technology.
Ormrod, J. (1995). Educational psychology: principles and applications. Englewood Cliffs, NJ: Prentice-Hall.
Ortiz, A. M., Bos, B., and Smith, S. (2015). The power of educational robotics as an integrated STEM learning experience in teacher preparation programs. J. Coll. Sci. Teach. 44, 42–47. doi: 10.2505/4/jcst15_044_05_42
Palmer, D. H. (2011). Sources of efficacy information in an in-service program for elementary teachers. Sci. Educ. 95, 577–600. doi: 10.1002/sce.20434
Papert, S., and Harel, I. (1991). “Situating constructionism. constructionism” in Constructionist learning. ed. Harel (Cambridge, MA: MIT Media Laboratory), 1–11.
Park, J. (2015). Effect of robotics enhanced inquiry-based learning in elementary science education in South Korea. J. Comput. Math. Sci. Teach. 34, 71–95.
Pea, R. D. (2004). The social and technological dimensions of scaffolding and related theoretical concepts for learning, education, and human activity. J. Learn. Sci., 13, 423–614 451. doi: 10.1207/s15327809jls1303_6
Piedade, J., Dorotea, N., Pedro, A., and Matos, J. F. (2020). On teaching programming fundamentals and computational thinking with educational robotics: a didactic experience with pre-service teachers. Educ. Sci. 10:214. doi: 10.3390/educsci10090214
Price, P. C., Jhangiani, R., and Chiang, I. C. A. (2015). Research methods in psychology (2nd Canadian ed.). Victoria: BC Campus.
Puntambekar, S., and Hubscher, R. (2005). Tools for scaffolding students in a complex learning environment: what have we gained and what have we missed? Educ. Psychol. 40, 1–12. doi: 10.1207/s15326985ep4001_1
Rohaan, E. J., Taconis, R., and Jochems, W. M. (2012). Analysing teacher knowledge for technology education in primary schools. Int. J. Technol. Des. Educ. 22, 271–280. doi: 10.1007/s10798-010-9147-z
Roll, I., Butler, D., Yee, N., Welsh, A., Perez, S., Briseno, A., et al. (2018). Understanding the impact of guiding inquiry: the relationship between directive support, student attributes, and transfer of knowledge, attitudes, and behaviours in inquiry learning. Instr. Sci. 46, 77–104. doi: 10.1007/s11251-017-9437-x
Sáez-López, J. M., Sevillano-García, M. L., and Vazquez-Cano, E. (2019). The effect of programming on primary school students’ mathematical and scientific understanding: educational use of mBot. Educ. Technol. Res. Dev. 67, 1405–1425. doi: 10.1007/s11423-019-09648-5
Schina, D., Valls-Bautista, C., Borrull-Riera, A., Usart, M., and Esteve-González, V. (2021). An associational study: preschool teachers’ acceptance and self-efficacy towards educational robotics in a pre-service teacher training program. Int. J. Educ. Technol. High. Educ. 18, 1–20. doi: 10.1186/s41239-021-00264-z
Shvarts, A., and Bakker, A. (2019). The early history of the scaffolding metaphor: Bernstein, Luria, Vygotsky, and before. Mind Cult. Activity 26, 4–23. doi: 10.1080/10749039.2019.1574306
Simons, K. D., and Klein, J. D. (2007). The impact of scaffolding and student achievement levels in a problem-based learning environment. Instr. Sci. 35, 41–72. doi: 10.1007/s11251-006-9002-5
Sinay, E., Jaipal-Jamani, K., Nahornick, A., and Douglin, M. (2016). STEM teaching and learning in the Toronto District School Board: Towards a strong theoretical foundation and scaling up from initial implementation of the K-12 STEM strategy. Research Series I. (Report No. 15/16-16). Toronto, Ontario, Canada: Toronto District School Board.
Sullivan, F. R. (2017). Creativity, technology, and learning: theory for classroom practice. London: Routledge.
Sullivan, F. R., and Moriarty, M. A. (2009). Robotics and discovery learning: pedagogical beliefs, teacher practice, and technology integration. J. Technol. Teach. Educ. 17, 109–142.
Taber, K. S. (2018a). “Scaffolding learning: principles for effective teaching and the design of classroom resources” in Effective teaching and learning: perspectives, strategies and implementation. ed. M. Abend (New York: Nova Science Publishers), 1–43.
Taber, K. S. (2018b). The use of Cronbach’s alpha when developing and reporting research instruments in science education. Res. Sci. Educ. 48, 1273–1296. doi: 10.1007/s11165-016-9602-2
Taylor, B. (2016). Evaluating the benefit of the maker movement in K-12 STEM education. Electron. Int. J. Educ. Arts Sci. 2, 1–22.
Thomas, D. R., and Zumbo, B. D. (2012). Difference scores from the point of view of reliability and repeated-measures ANOVA: in defense of difference scores for data analysis. Educ. Psychol. Meas. 72, 37–43. doi: 10.1177/0013164411409929
Tran, N. A., and Nathan, M. J. (2010). An investigation of the relationship between precollege engineering studies and student achievement in science and mathematics. J. Eng. Educ. 99, 143–157. doi: 10.1002/j.2168-9830.2010.tb01051.x
Tschannen-Moran, M., and Woolfolk Hoy, A. (2007). The differential antecedents of self-efficacy beliefs of novice and experienced teachers. Teach. Teach. Educ. 23, 944–956. doi: 10.1016/j.tate.2006.05.003
Tytler, R. (2020). “STEM education for the twenty-first century” in Integrated approaches to STEM education. Advances in STEM education (Cham: Springer), 21–43.
Valtonen, T., Kukkonen, J., Kontkanen, S., M€akitalo-Siegl, K., and Sointu, E. (2018). Differences in pre-service teachers’ knowledge and readiness to use ICT in education. J. Comput. Assist. Learn. 34, 174–182. doi: 10.1111/jcal.12225
Van Merriënboer, J. J. (1997). Training complex cognitive skills: a four-component instructional design model for technical training. Englewood Cliffs, NJ: Educational Technology Publication.
Vedder-Weiss, D., and Fortus, D. (2012). Adolescents' declining motivation to learn science: a follow-up study. J. Res. Sci. Teach. 49, 1057–1095. doi: 10.1002/tea.21049
Velthuis, C., Fisser, P., and Pieters, J. (2014). Teacher training and pre-service primary teachers’ self-efficacy for science teaching. J. Teach. Educ. 25, 445–464. doi: 10.1007/s10972-013-9363-y
Vincent-Lancrin, S., González-Sancho, C., Bouckaert, M., de Luca, F., Fernández-Barrerra, M., Jacotin, G., et al. (2019). Fostering students' creativity and critical thinking: what it means in school, educational research and innovation. Paris: OECD Publishing.
Vollstedt, A. M., Robinson, M., and Wang, E. (2007). “Using robotics to enhance science, technology, engineering, and mathematics curricula” in Proceedings of American Society for Engineering 635Education Pacific southwest annual conference, Hawaii, Honolulu
Vygotsky, L. S. (1978). Mind in society: the development of higher psychological processes. Cambridge, MA: Harvard University Press.
Vygotsky, L. S. (1987). “Thinking and speech” in The collected works of L.S. Vygotsky, volume 1: Problems of general psychology. eds. R. W. Rieber and A. S. Carton (New York: Plenum Press), 39–285.
Wang, X. (2013). Why students choose STEM majors: motivation, high school learning, and postsecondary context of support. Am. Educ. Res. J. 50, 1081–1121. doi: 10.3102/0002831213488622
Warde, C., and Sah, D. (2019). Creating a STEM-based economic pillar for the Caribbean: a blueprint. J. Educ. Dev. Caribb. 18, 234–270. doi: 10.46425/j091801a838
Whittier, L. E., and Robinson, M. (2007). Teaching evolution to non-English proficient students by using Lego robotics. Am. Second. Educ. 35, 19–28.
Williams, D. C., Ma, Y., Prejean, L., Ford, M. J., and Lai, G. (2007). Acquisition of physics content knowledge and scientific inquiry skills in a robotics summer camp. J. Res. Technol. Educ. 40, 201–216. doi: 10.1080/15391523.2007.10782505
Williams, J., Roth, W. M., Swanson, D., Doig, B., Groves, S., Omuvwie, M., et al. (2016). Interdisciplinary mathematics education. Switzerland: Springer Nature.
Wood, D., Bruner, J. S., and Ross, G. (1976). The role of tutoring in problem solving, vol. 17 Child Psychology & Psychiatry & Allied Disciplines, 89–100.
Yilmaz-Tuzun, O. (2008). Preservice elementary teachers’ beliefs about science teaching. J. Sci. Teach. Educ. 19, 183–204. doi: 10.1007/s10972-007-9084-1
You, H. S., Chacko, S. M., and Kapila, V. (2021). Examining the effectiveness of a professional development program: integration of educational robotics into science and mathematics curricula. J. Sci. Educ. Technol. 30, 567–581. doi: 10.1007/s10956-021-09903-6
Ziaeefard, S., Miller, M. H., Rastgaar, M., and Mahmoudian, N. (2017). Co-robotics hands-on activities: a gateway to engineering design and STEM learning. Robot. Auton. Syst. 97, 40–50. doi: 10.1016/j.robot.2017.07.013
Appendix 1
Sample programming worksheet (Jaipal-Jamani and Angeli, 2017).
Keywords: scaffolded instruction, self-guided learning, preservice teachers, robotics, pre-post intervention, science learning, self-efficacy
Citation: Jaipal-Jamani K (2023) Preservice teachers’ science learning and self-efficacy to teach with robotics-based activities: Investigating a scaffolded and a self-guided approach. Front. Educ. 8:979709. doi: 10.3389/feduc.2023.979709
Edited by:
Lucio Negrini, University of Applied Sciences and Arts of Southern Switzerland (SUPSI), SwitzerlandReviewed by:
Dorit Assaf, University of Applied Sciences and Arts Northwestern Switzerland, SwitzerlandBenadette Spieler, Zurich University of Teacher Education, Switzerland
Copyright © 2023 Jaipal-Jamani. This is an open-access article distributed under the terms of the Creative Commons Attribution License (CC BY). The use, distribution or reproduction in other forums is permitted, provided the original author(s) and the copyright owner(s) are credited and that the original publication in this journal is cited, in accordance with accepted academic practice. No use, distribution or reproduction is permitted which does not comply with these terms.
*Correspondence: Kamini Jaipal-Jamani, a2phaXBhbEBicm9ja3UuY2E=