- 1Department of Nutrition, Food Sciences and Physiology, University of Navarra, Pamplona, Spain
- 2Centre for Nutrition Research, University of Navarra, Pamplona, Spain
- 3Centro de Investigación Biomédica en Red Fisiopatología de la Obesidad y Nutrición (CIBERobn), Instituto de Salud Carlos III, Madrid, Spain
- 4Navarra Institute for Health Research (IdiSNa), Pamplona, Spain
- 5Department of Nutrition, Diabetes and Metabolism, School of Medicine, Pontificia Universidad Católica de Chile, Santiago, Chile
- 6Department of Biochemistry and Genetics, University of Navarra, Pamplona, Spain
- 7Precision Nutrition and Cardiometabolic Health Program, Madrid Institute for Advanced Studies (IMDEA), IMDEA Food, Madrid, Spain
The distribution of adipose tissue is influenced by gender and by age, shifting from subcutaneous to visceral depots with longevity, increasing the development of several aging-related diseases and manifestations such as obesity, metabolic syndrome, and insulin resistance. Epigenetics might have an important role in aging processes. The aim of this research was to investigate the interactions between aging and epigenetic processes and the role of visceral adipose tissue, insulin resistance, and dyslipidaemia. Two different study samples of 366 and 269 adult participants were analyzed. Anthropometric, biochemical (including the triglycerides-glucose (TyG) index), and blood pressure measurements were assessed following standardized methods. Body composition measurements by Dual-energy X-ray absorptiometry (DXA) were also performed for the second sample. Methylation data were assessed by Infinium Human Methylation BeadChip (Illumina) in peripheral white blood cells. Epigenetic age acceleration was calculated using the methods DNAmAge (AgeAcc) and GrimAge (AgeAccGrim). Age acceleration (AgeAccGrim) showed better correlations than AgeAcc with most of the measured variables (waist circumference, glucose, HOMA-IR, HDL-cholesterol, triglycerides, and TyG index) for the first sample. In the second sample, all the previous correlations were confirmed, except for HOMA-IR. In addition, many of the anthropometrical measurements assessed by DXA and C-reactive protein (CRP) were also statistically associated with AgeAccGrim. Associations separated by sex showed statistically significant correlations between AgeAccGrim and HDL-cholesterol or CRP in women, whereas, in men, the association was with visceral adipose tissue mass DXA, triglycerides and TyG index. Linear regression models (model 1 included visceral adipose tissue mass DXA and TyG index and model 2 included HDL-cholesterol and CRP) showed a significant association for men concerning visceral adipose tissue mass DXA and TyG index, while HDL-cholesterol and CRP were associated in women. Moreover, structural equation modeling showed that the TyG index was mediating the majority of the visceral adipose tissue mass action on age acceleration. Collectively, these findings showed that there are different mechanisms affecting epigenetic age acceleration depending on sex. The identified relationships between epigenetic age acceleration and disease markers will contribute to the understanding of the development of age-related diseases.
Introduction
Adipocytes are not only cells responsible for fuel storage triggered by a chronic imbalance between energy intake and energy expenditure, but they also participate in hormonal regulatory functions as well as in the synthesis of several endocrine/autocrine factors implicated in body homeostasis (1).
Excessive adipose tissue mass, particularly in the visceral compartment, has been related to hyperglycaemia, hypertension, hypercholesterolemia, and inflammatory processes (2). Indeed, obesity is associated with insulin resistance (IR), which, in turn, is also related to increased circulating levels of non-esterified fatty acids (3). This excess of lipids not only accumulates in different tissues, but it is also transported as very low-density lipoprotein triglycerides and secreted by the liver, leading to dyslipidaemia, which is a common feature of obesity and diabetes (4). These metabolic dysregulations are usually accompanied by changes in the endocrine balance (5) and in the secretory pattern of the adipose tissue (6, 7). Most of these metabolic processes are also related to aging, since nutrient-regulated pathways and energy balance may have a role in accelerating or delaying senescence (8).
On the other hand, the distribution of adipose tissue changes during the course of a lifetime, shifting from subcutaneous depots to intra-abdominal and ectopic fat deposition (9), and it is also influenced by sex (10). This fat/fuel redistribution has also been associated with the development of several aging-related diseases and manifestations, including obesity, metabolic syndrome and IR (11). Inadequate dietary habits and sedentarism, which are intimately related to increases in visceral fat, also contribute to the aging process, partly mediated by epigenetic mechanisms (12, 13).
Epigenetics involves a number of processes that modulate gene expression without altering the DNA sequence, such as DNA methylation, histone modifications and miRNAs (14). Several reports have related epigenetic signatures with longevity and aging. For example, Salas-Pérez et al., identified several differentially methylated sites that could be implicated in longevity and the development of metabolic disturbances (15). Furthermore, Horvath et al. have published several investigations relating DNA methylation with aging, and subsequently, developing different epigenetic clocks to measure “biological age” (16–18). Additionally, there is a general loss of histones in aging cells, and several modifications have been related to lifespan, such as histone methylation or histone acetylation (19). Moreover, miRNAs have also been involved in aging processes; for example, miR-34a has been described as an aging marker in several tissues and systems since it is upregulated in the aging heart, and its inhibition reduces cell death and fibrosis after an acute myocardial infarction (20).
The aim of this research was to investigate the interactions between aging and epigenetic processes, as well as the role of visceral adipose tissue, IR and dyslipidaemia on epigenetic age acceleration, together with putative mediating effects.
Subjects and Methods
Participants
This research was performed in two different study samples. The first study involved 366 adult participants from studies and cohorts belonging to the Methyl Epigenome Network Association (MENA) project such as DiOGenes-UNAV with n = 52 (21), OBEPALIP with n = 29 (22), Food4Me-UNAV with n = 39 (23), GEDYMET with n = 57 (24), ICTUS with n = 7 (25), NUGENOB-UNAV with n = 22 (26), PREDIMED-UNAV with n = 116 (27), and RESMENA with n = 44 (28), whose providers are gratefully acknowledged.
The second study involved 269 adult participants from the OBEKIT study with n = 203 (29) and the NormoP study with n = 66 (30). Study design, characteristics, inclusion and exclusion criteria of each of these studies have been previously described. This research was carried out in accordance with the recommendations of the Research Ethics Committee of the University of Navarra (CEI-UN, Pamplona, Spain). All subjects gave written informed consent in accordance with the Declaration of Helsinki. The protocol was approved by the Research Ethics Committee of the University of Navarra (CEI-UN, Pamplona, Spain), except for GEDYMET, which was approved by the ethics committee of the School of Medicine, Pontificia Universidad Católica de Chile (Santiago, Chile).
Study Variables
Anthropometric (waist circumference), blood pressure and biochemical measurements [glucose, HOMA-IR, HDL-cholesterol, triglycerides, TyG index, C-reactive protein (CRP)] were retrieved from databases of the aforementioned studies following validated protocols. The HOMA-IR index was calculated as fasting insulin (μUI/mL) × fasting glucose (mg/dL)/405. The triglycerides-glucose (TyG) index was calculated as Ln(triglycerides [mg/dL] x glucose [mg/dL]/2) as an indicator of IR (31). Body composition in the second study was assessed by Dual energy X-ray absorptiometry (DXA) according to the manufacturer′s instructions (Lunar iDXA, enCORE 14.5, GE Healthcare, Madison, WI, USA). Visceral adipose tissue mass was assessed with the CoreScan application for the software enCORE (GE Healthcare, Madison, WI, USA).
DNA Extraction and DNA Methylation Analysis
Venous blood samples were collected in EDTA tubes. Genomic DNA was extracted from peripheral white blood cells using the MasterPure™ DNA Purification kit (Epicenter, Madison, WI) and quantified with Pico Green dsDNA Quantitation Reagent (Invitrogen, Carlsbad, CA). In order to convert cytosine into uracil, high-quality DNA samples (500 ng) were treated with bisulfite using the EZ-96 DNA Methylation Kit (Zymo Research Corporation, Irvine, CA) according to the manufacturer's protocol. Infinium Human Methylation 450K BeadChip technology (Illumina, San Diego, CA, USA) was employed to measure DNA methylation levels in all the studies, except for OBEKIT and NormoP studies, which were performed with Infinium MethylationEPIC BeadChip (Illumina). The analyses were conducted in the Unidad de Genotipado y Diagnóstico Genético from Fundación Investigación Clínico de Valencia, as detailed elsewhere (32).
Treatment of Methylation Raw Data
Beta-values have been used to assess methylation levels in order to estimate the methylation degree using the ratio of the methylation probe intensity and the overall intensity, corresponding to the percentage of methylation on a specific site (33). Intensity data were obtained using the ChAMP package for R v.1.11.0 (34) as described elsewhere (35). Normalization of methylation samples from both studies was performed with the function preprocessNoob of Minfi package from Bioconductor. This function allows background correction with dye-bias normalization (36).
Estimation of Epigenetic Age Acceleration
Epigenetic age accelerations were defined as the residual (difference between the observed and the predicted value) from regressing the epigenetic age on chronological age (AgeAcc for DNAmAge method and AgeAccGrim for GrimAge method) as described by (37). After pre-processing, epigenetic age accelerations were calculated using two different methods available on the website DNA methylation Age Calculator (https://dnamage.genetics.ucla.edu/home) (16). The first method, denominated DNAmAge and developed by Horvath (16), was designed to calculate the epigenetic age (DNAm age) from human samples profiled with the Illumina Infinium 450K platform. This procedure was based on the DNA methylation levels of 353 CpGs. The second method, denominated GrimAge, was recently created by Horvath and collaborators (18). Briefly, this epigenetic clock was a method of epigenetic age prediction based on a linear combination of chronological age, sex, and DNAm-based surrogate biomarkers for seven plasma proteins and smoking pack-years. As indicated by Horvath on the DNA methylation Age Calculator website, samples with a correlation coefficient <0.8 with the gold standard (defined by averaging the beta values across the samples from the largest blood data set) were excluded (data provided by the website application).
Statistical Analysis
Variables with skewness >1 were log2-transformed [glucose, HOMA-IR, triglycerides and CRP (adding +1 to avoid the logarithm of zero)] as previously described (38). Age accelerations (AgeAcc and AgeAccGrim) from the first study were correlated (Pearson's r) with different variables including waist circumference, glucose (log2), HOMA-IR (log2), HDL-cholesterol, triglycerides (log2), systolic and diastolic blood pressure and the TyG index. Differences between women and men in the second study (OBEKIT + NormoP) were calculated using two-independent samples Student t-test. AgeAccGrim data from the second study were correlated (Pearson's r) with different anthropometric measurements determined by DXA (fat mass, lean mass, trunk fat mass, android fat mass, gynoid fat mass, visceral adipose tissue mass), the metabolic syndrome variables as measured in the MENA study, and the CRP (log2) as a marker of inflammation. Multiple linear regression models between AgeAccGrim and some variables from the second study (model 1: visceral adipose tissue mass and TyG index, model 2: HDL-cholesterol and CRP) were fitted, separating by sex. Mediation by TyG index in the relationship between AgeAccGrim and visceral adipose tissue mass DXA in men was assessed using structural equation modeling following the Zhao et al. approach (39).
p-values were considered statistically significant if p <0.05. Statistical calculations were performed with Stata version 12.1 (StataCorp 2011, College Station, TX, USA).
Results
Correlations in the First Study Sample
Anthropometric, biochemical and blood pressure measurements of the first study are reported (Table 1).
Correlations between age accelerations measured by Horvath (AgeAcc) or by Lu et al. (AgeAccGrim) and the anthropometric, biochemical and blood pressure variables were performed for participants from the first study (Table 2). Results showed statistically significant associations between both age accelerations and all the anthropometric and biochemical measurements, except for glucose and triglycerides, which were only significant for AgeAccGrim. In addition, AgeAccGrim showed higher correlation coefficients and statistical significance with most of the variables, while no statistical associations were found with blood pressure.
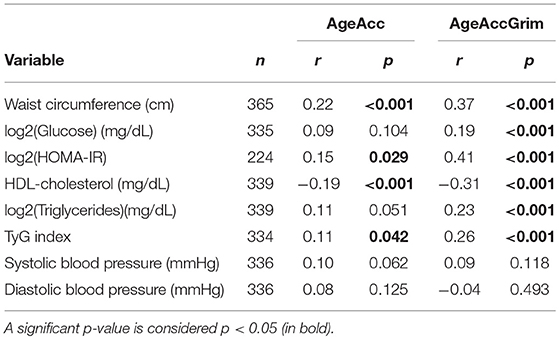
Table 2. Correlations in the first study sample between epigenetic age accelerations calculated by two different methods and anthropometric, biochemical, and blood pressure variables.
Correlations in the Second Study Sample
Anthropometric, biochemical, and blood pressure measurements of the second study are reported (Table 3). Since several variables showed differences between women and men (Table 3), further analyses were performed separating by sex.
Correlations in the second study for both sexes and separated by sex were calculated with AgeAccGrim (Table 4) since it was the epigenetic age acceleration that showed better correlation coefficients and significance with many variables analyzed in the first study (Table 2). Correlations in the second study were statistically significant for the same variables as those obtained from the first study, except for HOMA-IR, which was not significant in the OBEKIT+NormoP study. Moreover, both CRP and many of the anthropometrical measurements assessed by DXA were also significantly associated with AgeAccGrim. When analyzing by sex, women showed statistically significant correlations between AgeAccGrim and HDL-cholesterol or CRP, whereas in men the identified associations were significant with visceral adipose tissue mass measured by DXA, triglycerides and TyG index. In order to analyse whether these significant correlations were different between both sexes, comparison of both correlation coefficients was performed. Significant differences were only found for triglycerides and TyG index (Table S1).
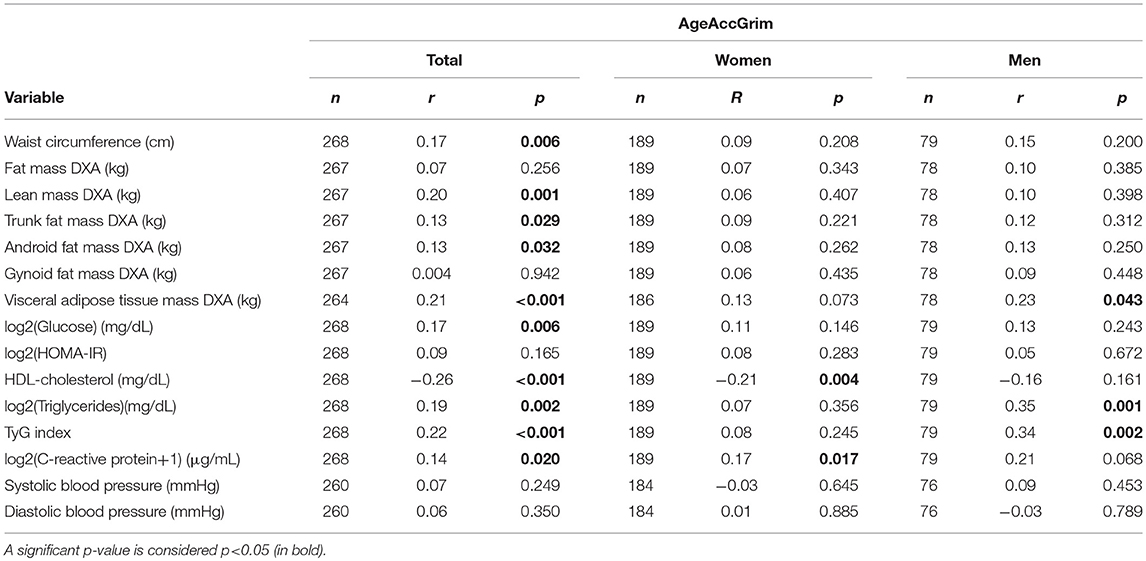
Table 4. Correlations in the second study between epigenetic age acceleration estimated by AgeAccGrim and anthropometric, body composition, biochemical and blood pressure variables.
Linear Regression Models in the Second Study
Linear regression models were then fitted for the second study separated by sex (Table 5). Two different models were applied: model 1 included visceral adipose tissue mass measured by DXA and TyG index, whereas model 2 included HDL-cholesterol and CRP. Model 1 was statistically significant for men, but not for women; on the contrary, model 2 was statistically significant for women, but not for men.
Mediation Model in the Second Study
Since visceral adiposity has been suggested as the key point for the onset of IR, a possible mediation by TyG index in the relationship between visceral adipose tissue mass DXA and AgeAccGrim was assessed in men. Results showed that TyG index was mediating the majority of the effects of visceral adipose tissue mass accumulation on age acceleration (Figure 1).
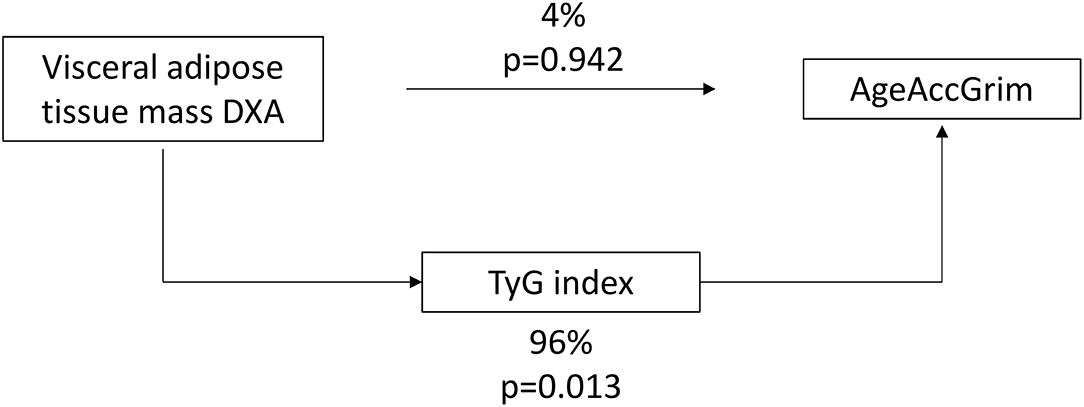
Figure 1. TyG index-mediated relationship between visceral adipose tissue mass measured by DXA and age acceleration GrimAge in men.
Discussion
Aging is an irreversible and progressive process that occurs from birth (40). Many theories have been proposed about aging (41), including wear-and-tear theories, the free radical hypothesis of aging, the cross-linking theory, and the neuroendocrine theory, among others (40). Interestingly, newer studies have suggested a role of epigenetic processes in lifespan regulation that should be further investigated (42). In this context, aging-related DNA methylation has been hypothesized as a biomarker that predicts both cellular age and chronological age (42).
Fat accumulation, and specifically, excessive visceral fat deposition, is common in elderly individuals. Excessive visceral fat deposition usually increases with age, as it is related to obesity, diabetes, cardiovascular diseases, cancer and fatty liver disease (11, 43). Visceral fat has an important role in the onset of IR (44). Subjects with accumulation of abdominal fat presented enhanced lipolysis and alteration of the flux of free fatty acids (44). The rise of circulating free fatty acids in turn increases hepatic gluconeogenesis and glycogenolysis, as well as increasing insulin secretion by the pancreas, resulting in hyperglycaemia (45). In addition, in the liver, there is a higher production of triglycerides and less insulin breakdown, leading to hyperinsulinemia (45). Hyperglycaemia and hyperinsulinemia promote IR (46). Interestingly, the TyG index has been determined as a useful marker of IR (44). Indeed, it has been demonstrated as a better marker for the diagnosis of metabolic syndrome than HOMA-IR, since it involves measures of both triglycerides and glucose (47).
Aging is an important contributor to metabolism and health impairments, increasing the risk for several diseases (48). Numerous mechanisms related to longevity and age-related metabolic dysfunctions occur in adipose tissue (48). Therefore, the investigation of the relationships between adipose tissue, IR and aging and the role of epigenetics in this process is a challenging task that could provide new understanding about the putative interactions among them.
The assessment of aging has received attention because chronological age is sometimes different from biological and metabolic age (49). Therefore, different methods based on epigenetic measurements have been designed for that purpose: an estimator developed by Hannum et al. (50) based on 71 CpGs in leukocytes; DNAmAge clock created by Horvath (16) based on 353 CpGs in different tissues; PhenoAge designed by Levine et al. (17) for predicting lifespan based on regressing a phenotypic measure of mortality risk on CpGs; and the newest one, GrimAge, developed by Lu et al. (18) using DNAm-based biomarkers for seven plasma proteins and smoking. GrimAge has been reported as a better predictor of lifespan than currently available DNAm-based predictors (18). Studies with these epigenetic clocks have demonstrated epigenetic age acceleration in obesity (37, 51), diabetes (51, 52), non-alcoholic fatty liver disease (53) and different metabolic and inflammatory biomarkers (54). In this sense, epigenetic age acceleration could be defined as the aging of epigenetic DNA regulation, which may lead to longevity functions impairments earlier than expected.
A number of factors, such as sex, could mediate the interactions between epigenetic aging and adiposity biomarkers, since it is known that visceral fat accumulation is more common in men than in women (10). Furthermore, sex differences elicited by sexual hormones affect this adiposity dimorphism. In this context, estrogens may direct the expansion of fat through the increase of adiposity progenitor cells (hyperplasia) as well as regulate the vascular supply into adipose tissues (55) with impact on epigenetic mechanisms (56).
Indeed, our investigation demonstrated that sex is important in the analysis of the relationship between epigenetic age acceleration and adiposity biomarkers. Men showed a tighter association of epigenetic age acceleration with visceral adipose tissue mass and the TyG index, whereas HDL-cholesterol and CRP were associated with epigenetic age acceleration in women. However, we cannot conclude that the correlations observed were statistically different between sex groups, except for TyG index. These results are also in agreement with the fact that aging is usually accompanied by a deterioration of metabolic markers (57, 58). Furthermore, we found that the relationship between visceral adipose tissue and epigenetic age acceleration in men is mainly mediated by the TyG index. Thus, the accumulation of abdominal fat, through TyG index mediation, suggest an older biological age in men than the expected. This result is in accordance with the fact that visceral adipose tissue contributes to the development of IR (44) and that both are influencing aging processes (37, 59).
Other possible factors that might influence epigenetic aging are HDL-cholesterol or CRP, as described in our research. HDL-cholesterol relationship with epigenetic age acceleration was negative, thereby suggesting a deceleration of biological age with higher levels of this lipoprotein. Thus, HDL-cholesterol might modulate epigenetic aging processes due to its antiatherogenic effects such as the removal of lipid deposits, which is accompanied by the reduction of cytotoxic effects (60). Furthermore, HDL reduces the oxidative stress in plasma and cellular compartments, and the signaling pathways which it participates in are interconnected with stress response and survival pathways (60). On the other hand, higher CRP levels were related to accelerated biological age, suggesting a negative influence of inflammation on aging-related epigenetic marks. Indeed, CRP is a molecule involved in inflammatory and immune processes (61), which has been associated with aging-related diseases such as diabetes, cardiovascular diseases and hypertension (62). Additionally, previous research showed that after adjusting for age, sex, body mass index, lipid levels and smoking status, increased levels of CRP were associated with cognitive and poor physical performances and reduced survival (63).
This research did have some limitations. The ideal tissue assessed in this study would have been adipose tissue since the relationship was established between visceral adipose tissue mass and age acceleration. However, several studies have demonstrated that peripheral blood is a valid non-invasive alternative tissue that reflects metabolic and inflammatory pathways and serves as a surrogate for assessing methylation (64–67). Furthermore, DNAm age performs well in blood, (16) and GrimAge has been developed with methylation data from blood samples (18). Another possible limitation is that methylation samples in the MENA and in the OBEKIT+NormoP studies were assessed with different Illumina arrays (Infinium 450K BeadChip and EPIC, respectively). Nevertheless, McEwen et al. revealed that both methods were equivalent in the determination of epigenetic age (68). Moreover, it must be noted that the percentage of contribution of our variables to epigenetic age acceleration is at maximum of 10%. Thus, there must be other factors influencing the progression of aging.
In summary, our research has revealed that the mechanisms that affect epigenetic age acceleration are different in women and men. Whereas, in men the accumulation of visceral adipose tissue accelerates the epigenetic age through mechanisms mostly mediated by IR, mechanisms in women seemed to be more related to HDL-cholesterol and CRP. These findings support the hypothesis that obesity and metabolic syndrome features, intimately related to adiposity and dyslipidaemia, are associated with accelerated aging effects (43), although in a different manner for each sex. Further research about the relationship between epigenetic age acceleration and disease markers will allow for a better understanding of the molecular mechanisms involved in the development of age-related diseases. This knowledge is needed to create new useful clinical and public health tools based on DNA methylation biomarkers and epigenetic age acceleration. Furthermore, sex differences concerning the factors influencing epigenetic age acceleration should be taken into account in future development of precision strategies and management of healthy aging.
Data Availability
The data of the first study have been deposited in NCBI's Gene Expression Omnibus (69) and are accessible through GEO Series accession number GSE115278 (https://www.ncbi.nlm.nih.gov/geo/query/acc.cgi?acc=GSE115278). The remaining data will be made available by the authors, without undue reservation, to any qualified researcher.
Ethics Statement
This study was carried out in accordance with the recommendations of the Research Ethics Committee of the University of Navarra (CEI-UN, Pamplona, Spain). All subjects gave written informed consent in accordance with the Declaration of Helsinki. The protocol was approved by the Research Ethics Committee of the University of Navarra (CEI-UN, Pamplona, Spain), except for GEDYMET, which was approved by the Ethics committee of the School of Medicine, Pontificia Universidad Católica de Chile (Santiago, Chile).
Author Contributions
AA performed data analysis and wrote the first version of the paper. JS helped with data interpretation. MG-G helped with the statistical analysis. J-IR-B, FM, and JM supervised data analysis and helped with interpretation and with manuscript elaboration, as well as provided the conceptual design and financial support. All authors read and approved the final manuscript.
Funding
This work was supported by grants from the Government of Navarra (PT024), CIBERobn (CB12/03/30002) and Ministerio de Economía y Competitividad (AGL2013-45554-R). AA was supported by a Formación de Profesorado Universitario predoctoral fellowship from Ministerio de Educación, Cultura y Deporte (FPU15/02790).
Conflict of Interest Statement
The authors declare that the research was conducted in the absence of any commercial or financial relationships that could be construed as a potential conflict of interest.
Acknowledgments
Other members of the MENA project are: Abete I, Crujeiras AB, Cuervo M, Goni L, Marti A, Martinez-Gonzalez MA, Moreno-Aliaga MJ, Navas-Carretero S, San-Cristobal R, and Zulet MA. Also, leaders of specific cohorts such as Astrup A, Saris WHM, Mathers JC, Gibney M, Lopez de Munain A, Sorensen TI, Arner P, Langin D, Salas-Salvadó J, and Estruch R, are gratefully acknowledged. The authors wish to thank all the volunteers who took part in the study, the personnel of the primary care centres and other investigators of the different research groups for their participation in the recruitment process and the acquisition of the data. The authors would also like to thank Nora Goodwin (from Dublin Institute of Technology, Dublin, Ireland) and Sanjana Srinivasan (from Chicago) for reviewing the manuscript.
Supplementary Material
The Supplementary Material for this article can be found online at: https://www.frontiersin.org/articles/10.3389/fendo.2019.00496/full#supplementary-material
References
1. Kershaw EE, Flier JS. Adipose tissue as an endocrine organ. J Clin Endocrinol Metab. (2004) 89:2548–56. doi: 10.1210/jc.2004-0395
2. Grundy SM, Brewer HB Jr, Cleeman JI, Smith SC Jr, Lenfant C National Heart Lung and Blood Institute et al. Definition of metabolic syndrome: Report of the National Heart, Lung, and Blood Institute/American Heart Association conference on scientific issues related to definition. Circulation. (2004) 109:433–8. doi: 10.1161/01.ATV.0000111245.75752.C6
3. Yazici D, Sezer H. Insulin resistance, obesity and lipotoxicity. Adv Exp Med Biol. (2017) 960:277–304. doi: 10.1007/978-3-319-48382-5_12
4. Smith U, Kahn BB. Adipose tissue regulates insulin sensitivity: role of adipogenesis, de novo lipogenesis and novel lipids. J Intern Med. (2016) 280:465–75. doi: 10.1111/joim.12540
5. Vegiopoulos A, Rohm M, Herzig S. Adipose tissue: between the extremes. EMBO J. (2017) 36:1999–2017. doi: 10.15252/embj.201696206
6. Trayhurn P, Beattie JH. Physiological role of adipose tissue: white adipose tissue as an endocrine and secretory organ. Proc Nutr Soc. (2001) 60:329–39. doi: 10.1079/PNS200194
7. McGown C, Birerdinc A, Younossi ZM. Adipose tissue as an endocrine organ. Clin Liver Dis. (2014) 18:41–58. doi: 10.1016/j.cld.2013.09.012
8. Majidinia M, Reiter RJ, Shakouri SK, Yousefi B. The role of melatonin, a multitasking molecule, in retarding the processes of ageing. Ageing Res Rev. (2018) 47:198–213. doi: 10.1016/j.arr.2018.07.010
9. Mau T, Yung R. Adipose tissue inflammation in aging. Exp Gerontol. (2018) 105:27–31. doi: 10.1016/j.exger.2017.10.014
10. Link JC, Reue K. Genetic basis for sex differences in obesity and lipid metabolism. Annu Rev Nutr. (2017) 37:225–45. doi: 10.1146/annurev-nutr-071816-064827
11. Palmer AK, Kirkland JL. Aging and adipose tissue: potential interventions for diabetes and regenerative medicine. Exp Gerontol. (2016) 86:97–105. doi: 10.1016/j.exger.2016.02.013
12. Daniel M, Tollefsbol TO. Epigenetic linkage of aging, cancer and nutrition. J Exp Biol. (2015) 218:59–70. doi: 10.1242/jeb.107110
13. Niculescu MD, Lupu DS. Nutritional influence on epigenetics and effects on longevity. Curr Opin Clin Nutr Metab Care. (2011) 14:35–40. doi: 10.1097/MCO.0b013e328340ff7c
14. Egger G, Liang G, Aparicio A, Jones PA. Epigenetics in human disease and prospects for epigenetic therapy. Nature. (2004) 429:457–63. doi: 10.1038/nature02625
15. Salas-Perez F, Ramos-Lopez O, Mansego ML, Milagro FI, Santos JL, Riezu-Boj JI, et al. DNA methylation in genes of longevity-regulating pathways: association with obesity and metabolic complications. Aging (Albany NY). (2019) 11:1874–99. doi: 10.18632/aging.101882
16. Horvath S. DNA methylation age of human tissues and cell types. Genome Biol. (2013) 14:R115. doi: 10.1186/gb-2013-14-10-r115
17. Levine ME, Lu AT, Quach A, Chen BH, Assimes TL, Bandinelli S, et al. An epigenetic biomarker of aging for lifespan and healthspan. Aging (Albany NY). (2018) 10:573–91. doi: 10.18632/aging.101414
18. Lu AT, Quach A, Wilson JG, Reiner AP, Aviv A, Raj K, et al. DNA methylation GrimAge strongly predicts lifespan and healthspan. Aging (Albany NY). (2019) 11:303–27. doi: 10.18632/aging.101684
19. Sen P, Shah PP, Nativio R, Berger SL. Epigenetic mechanisms of longevity and aging. Cell. (2016) 166:822–39. doi: 10.1016/j.cell.2016.07.050
20. Boon RA, Iekushi K, Lechner S, Seeger T, Fischer A, Heydt S, et al. MicroRNA-34a regulates cardiac ageing and function. Nature. (2013) 495:107–10. doi: 10.1038/nature11919
21. Larsen TM, Dalskov S, van Baak M, Jebb S, Kafatos A, Pfeiffer A, et al. The diet, obesity and genes (Diogenes) dietary study in eight european countries - a comprehensive design for long-term intervention. Obes Rev. (2010) 11:76–91. doi: 10.1111/j.1467-789X.2009.00603.x
22. Huerta AE, Navas-Carretero S, Prieto-Hontoria PL, Martinez JA, Moreno-Aliaga MJ. Effects of alpha-lipoic acid and eicosapentaenoic acid in overweight and obese women during weight loss. Obesity (Silver Spring). (2015) 23:313–21. doi: 10.1002/oby.20966
23. Celis-Morales C, Livingstone KM, Marsaux CF, Forster H, O'Donovan CB, Woolhead C, et al. Design and baseline characteristics of the Food4Me study: a web-based randomised controlled trial of personalised nutrition in seven European countries. Genes Nutr. (2015) 10:450. doi: 10.1017/S0029665115001330
24. Santos JL, Yevenes I, Cataldo LR, Morales M, Galgani J, Arancibia C, et al. Development and assessment of the disposition index based on the oral glucose tolerance test in subjects with different glycaemic status. J Physiol Biochem. (2016) 72:121–31. doi: 10.1007/s13105-015-0458-0
25. Abete I, Gomez-Uriz AM, Mansego ML, De Arce A, Goyenechea E, Blazquez V, et al. Epigenetic changes in the methylation patterns of KCNQ1 and WT1 after a weight loss intervention program in obese stroke patients. Curr Neurovasc Res. (2015) 12:321–33. doi: 10.2174/1567202612666150731110247
26. Petersen M, Taylor MA, Saris WH, Verdich C, Toubro S, Macdonald I, et al. Randomized, multi-center trial of two hypo-energetic diets in obese subjects: high- versus low-fat content. Int J Obes (Lond). (2006) 30:552–60. doi: 10.1038/sj.ijo.0803186
27. Estruch R, Ros E, Salas-Salvado J, Covas MI, Corella D, Aros F, et al. Primary prevention of cardiovascular disease with a Mediterranean diet. N Engl J Med. (2013) 368:1279–90. doi: 10.1056/NEJMoa1200303
28. Zulet MA, Bondia-Pons I, Abete I, de la Iglesia R, Lopez-Legarrea P, Forga L, et al. The reduction of the metabolyc syndrome in Navarra-Spain (RESMENA-S) study: a multidisciplinary strategy based on chrononutrition and nutritional education, together with dietetic and psychological control. Nutr Hosp. (2011) 26:16–26. doi: 10.3305/nh.2011.26.1.5050
29. Ramos-Lopez O, Riezu-Boj JI, Milagro FI, Alfredo Martinez J, Project M. Association of methylation signatures at hepatocellular carcinoma pathway genes with adiposity and insulin resistance phenotypes. Nutr Cancer. (2019) 71:840–51. doi: 10.1080/01635581.2018.1531136
30. Arpon A, Milagro FI, Ramos-Lopez O, Mansego ML, Santos JL, Riezu-Boj JI, et al. Epigenome-wide association study in peripheral white blood cells involving insulin resistance. Sci Rep. (2019) 9:2445. doi: 10.1038/s41598-019-38980-2
31. Unger G, Benozzi SF, Perruzza F, Pennacchiotti GL. Triglycerides and glucose index: a useful indicator of insulin resistance. Endocrinol Nutr. (2014) 61:533–40. doi: 10.1016/j.endoen.2014.11.006
32. Arpón A, Riezu-Boj JI, Milagro FI, Razquin C, Martinez-Gonzalez MA, Corella D, et al. Adherence to Mediterranean diet is associated with methylation changes in inflammation-related genes in peripheral blood cells. J Physiol Biochem. (2017) 73:445–55. doi: 10.1007/s13105-017-0552-6
33. Du P, Kibbe WA, Lin SM. lumi: a pipeline for processing Illumina microarray. Bioinformatics. (2008) 24:1547–8. doi: 10.1093/bioinformatics/btn224
35. Morris TJ, Butcher LM, Feber A, Teschendorff AE, Chakravarthy AR, Wojdacz TK, et al. ChAMP: 450k chip analysis methylation pipeline. Bioinformatics. (2014) 30:428–30. doi: 10.1093/bioinformatics/btt684
36. Triche TJ Jr, Weisenberger DJ, Van Den Berg D, Laird PW, Siegmund KD. Low-level processing of Illumina Infinium DNA Methylation BeadArrays. Nucleic Acids Res. (2013) 41:e90. doi: 10.1093/nar/gkt090
37. Horvath S, Erhart W, Brosch M, Ammerpohl O, von Schonfels W, Ahrens M, et al. Obesity accelerates epigenetic aging of human liver. Proc Natl Acad Sci USA. (2014) 111:15538–43. doi: 10.1073/pnas.1412759111
38. Quach A, Levine ME, Tanaka T, Lu AT, Chen BH, Ferrucci L, et al. Epigenetic clock analysis of diet, exercise, education, and lifestyle factors. Aging (Albany NY). (2017) 9:419–46. doi: 10.18632/aging.101168
39. Zhao X, Lynch JG, Chen Q. Reconsidering baron and kenny: myths and truths about mediation analysis. J Consumer Res. (2010) 37:197–206. doi: 10.1086/651257
40. Vina J, Borras C, Miquel J. Theories of ageing. IUBMB Life. (2007) 59:249–54. doi: 10.1080/15216540601178067
41. Medvedev ZA. An attempt at a rational classification of theories of ageing. Biol Rev Camb Philos Soc. (1990) 65:375–98. doi: 10.1111/j.1469-185X.1990.tb01428.x
42. Park JH, Yoo Y, Park YJ. Epigenetics: linking nutrition to molecular mechanisms in aging. Prev Nutr Food Sci. (2017) 22:81–9. doi: 10.3746/pnf.2017.22.2.81
44. Navarro-Gonzalez D, Sanchez-Inigo L, Fernandez-Montero A, Pastrana-Delgado J, Martinez JA. TyG index change is more determinant for forecasting type 2 diabetes onset than weight gain. Medicine (Baltimore). (2016) 95:e3646. doi: 10.1097/MD.0000000000003646
45. Ibrahim MM. Subcutaneous and visceral adipose tissue: structural and functional differences. Obes Rev. (2010) 11:11–8. doi: 10.1111/j.1467-789X.2009.00623.x
46. Lee Y, Fluckey JD, Chakraborty S, Muthuchamy M. Hyperglycemia- and hyperinsulinemia-induced insulin resistance causes alterations in cellular bioenergetics and activation of inflammatory signaling in lymphatic muscle. FASEB J. (2017) 31:2744–59. doi: 10.1096/fj.201600887R
47. Khan SH, Sobia F, Niazi NK, Manzoor SM, Fazal N, Ahmad F. Metabolic clustering of risk factors: evaluation of Triglyceride-glucose index (TyG index) for evaluation of insulin resistance. Diabetol Metab Syndr. (2018) 10:74. doi: 10.1186/s13098-018-0376-8
48. Jura M, Kozak LP. Obesity and related consequences to ageing. Age (Dordr). (2016) 38:23. doi: 10.1007/s11357-016-9884-3
49. Jia L, Zhang W, Chen X. Common methods of biological age estimation. Clin Interv Aging. (2017) 12:759–72. doi: 10.2147/CIA.S134921
50. Hannum G, Guinney J, Zhao L, Zhang L, Hughes G, Sadda S, et al. Genome-wide methylation profiles reveal quantitative views of human aging rates. Mol Cell. (2013) 49:359–67. doi: 10.1016/j.molcel.2012.10.016
51. Dugue PA, Bassett JK, Joo JE, Baglietto L, Jung CH, Wong EM, et al. Association of DNA methylation-based biological age with health risk factors and overall and cause-specific mortality. Am J Epidemiol. (2018) 187:529–38. doi: 10.1093/aje/kwx291
52. Grant CD, Jafari N, Hou L, Li Y, Stewart JD, Zhang G, et al. A longitudinal study of DNA methylation as a potential mediator of age-related diabetes risk. Geroscience. (2017) 39:475–89. doi: 10.1007/s11357-017-0001-z
53. Loomba R, Gindin Y, Jiang Z, Lawitz E, Caldwell S, Djedjos CS, et al. DNA methylation signatures reflect aging in patients with nonalcoholic steatohepatitis. JCI Insight. (2018) 3: 96685. doi: 10.1172/jci.insight.96685
54. Irvin MR, Aslibekyan S, Do A, Zhi D, Hidalgo B, Claas SA, et al. Metabolic and inflammatory biomarkers are associated with epigenetic aging acceleration estimates in the GOLDN study. Clin Epigenetics. (2018) 10:56. doi: 10.1186/s13148-018-0481-4
55. Palmer BF, Clegg DJ. The sexual dimorphism of obesity. Mol Cell Endocrinol. (2015) 402:113–9. doi: 10.1016/j.mce.2014.11.029
56. Dahlman I, Sinha I, Gao H, Brodin D, Thorell A, Ryden M, et al. The fat cell epigenetic signature in post-obese women is characterized by global hypomethylation and differential DNA methylation of adipogenesis genes. Int J Obes (Lond). (2015) 39:910–9. doi: 10.1038/ijo.2015.31
57. Lawton KA, Berger A, Mitchell M, Milgram KE, Evans AM, Guo L, et al. Analysis of the adult human plasma metabolome. Pharmacogenomics. (2008) 9:383–97. doi: 10.2217/14622416.9.4.383
58. Fontana L, Partridge L, Longo VD. Extending healthy life span–from yeast to humans. Science. (2010) 328:321–6. doi: 10.1126/science.1172539
59. Gilbert ER, Liu D. Epigenetics: the missing link to understanding beta-cell dysfunction in the pathogenesis of type 2 diabetes. Epigenetics. (2012) 7:841–52. doi: 10.4161/epi.21238
60. Walter M. Interrelationships among HDL metabolism, aging, and atherosclerosis. Arterioscler Thromb Vasc Biol. (2009) 29:1244–50. doi: 10.1161/ATVBAHA.108.181438
61. Mortensen RF. C-reactive protein, inflammation, and innate immunity. Immunol Res. (2001) 24:163–76. doi: 10.1385/IR:24:2:163
62. Tang Y, Fung E, Xu A, Lan HY. C-reactive protein and ageing. Clin Exp Pharmacol Physiol. (2017) 44 (Suppl. 1):9–14. doi: 10.1111/1440-1681.12758
63. Puzianowska-Kuznicka M, Owczarz M, Wieczorowska-Tobis K, Nadrowski P, Chudek J, Slusarczyk P, et al. Interleukin-6 and C-reactive protein, successful aging, and mortality: the PolSenior study. Immun Ageing. (2016) 13:21. doi: 10.1186/s12979-016-0076-x
64. Muka T, Nano J, Voortman T, Braun VE, Ligthart S, Stranges S, et al. The role of global and regional DNA methylation and histone modifications in glycemic traits and type 2 diabetes: a systematic review. Nutr Metab Cardiovasc Dis. (2016) 26:553–66. doi: 10.1016/j.numecd.2016.04.002
65. Bacos K, Gillberg L, Volkov P, Olsson AH, Hansen T, Pedersen O, et al. Blood-based biomarkers of age-associated epigenetic changes in human islets associate with insulin secretion and diabetes. Nat Commun. (2016) 7:11089. doi: 10.1038/ncomms11089
66. Crujeiras AB, Diaz-Lagares A, Sandoval J, Milagro FI, Navas-Carretero S, Carreira MC, et al. DNA methylation map in circulating leukocytes mirrors subcutaneous adipose tissue methylation pattern: a genome-wide analysis from non-obese and obese patients. Sci Rep. (2017) 7:41903. doi: 10.1038/srep41903
67. San-Cristobal R, Navas-Carretero S, Milagro FI, Riezu-Boj JI, Guruceaga E, Celis-Morales C, et al. Gene methylation parallelisms between peripheral blood cells and oral mucosa samples in relation to overweight. J Physiol Biochem. (2016) 73:465–74. doi: 10.1007/s13105-017-0560-6
68. McEwen LM, Jones MJ, Lin TS, Edgar RD, Husquin LT, MacIsaac JL, et al. Systematic evaluation of DNA methylation age estimation with common preprocessing methods and the Infinium MethylationEPIC BeadChip array. Clin Epigenetics. (2018) 10:123. doi: 10.1186/s13148-018-0556-2
Keywords: DNA methylation, visceral adipose tissue, C-reactive protein, HDL-cholesterol, TyG index
Citation: Arpón A, Milagro FI, Santos JL, García-Granero M, Riezu-Boj JI and Martínez JA (2019) Interaction Among Sex, Aging, and Epigenetic Processes Concerning Visceral Fat, Insulin Resistance, and Dyslipidaemia. Front. Endocrinol. 10:496. doi: 10.3389/fendo.2019.00496
Received: 17 April 2019; Accepted: 08 July 2019;
Published: 17 July 2019.
Edited by:
Bruno Ramos-Molina, Biomedical Research Institute of Malaga, University of Málaga, SpainReviewed by:
Marie-Claude Vohl, Laval University, CanadaVibha Singhal, Massachusetts General Hospital, Harvard Medical School, United States
Neil Youngson, University of New South Wales, Australia
Copyright © 2019 Arpón, Milagro, Santos, García-Granero, Riezu-Boj and Martínez. This is an open-access article distributed under the terms of the Creative Commons Attribution License (CC BY). The use, distribution or reproduction in other forums is permitted, provided the original author(s) and the copyright owner(s) are credited and that the original publication in this journal is cited, in accordance with accepted academic practice. No use, distribution or reproduction is permitted which does not comply with these terms.
*Correspondence: José-Ignacio Riezu-Boj, amlyaWV6dUB1bmF2LmVz