- 1Nanjing Maternity and Child Health Care Institute, Women's Hospital of Nanjing Medical University (Nanjing Maternity and Child Health Care Hospital), Nanjing, China
- 2Department of Clinical Laboratory, Women's Hospital of Nanjing Medical University (Nanjing Maternity and Child Health Care Hospital), Nanjing, China
- 3Affiliated Maternity and Child Health Care Hospital of Nantong University, NanTong, China
- 4Department of Endocrinology, Children's Hospital of Nanjing Medical University, Nanjing, China
As an important secretory organ, skeletal muscle has drawn attention as a potential target tissue for type 2 diabetic mellitus (T2DM). Recent peptidomics approaches have been applied to identify secreted peptides with potential bioactive. However, comprehensive analysis of the secreted peptides from skeletal muscle tissues of db/db mice and elucidation of their possible roles in insulin resistance remains poorly characterized. Here, we adopted a label-free discovery using liquid chromatography tandem mass spectrometry (LC-MS/MS) technology and identified 63 peptides (42 up-regulated peptides and 21 down-regulated peptides) differentially secreted from cultured skeletal muscle tissues of db/db mice. Analysis of relative molecular mass (Mr), isoelectric point (pI) and distribution of Mr vs pI of differentially secreted peptides presented the general feature. Furthermore, Gene ontology (GO) and pathway analyses for the parent proteins made a comprehensive functional assessment of these differential peptides, indicating the enrichment in glycolysis/gluconeogenesis and striated muscle contraction processes. Intercellular location analysis pointed out most precursor proteins of peptides were cytoplasmic or cytoskeletal. Additionally, cleavage site analysis revealed that Lysine (N-terminal)-Alanine (C-terminal) and Lysine (N-terminal)-Leucine (C-terminal) represents the preferred cleavage sites for identified peptides and proceeding peptides respectively. Mapped to the precursors' sequences, most identified peptides were observed cleaved from creatine kinase m-type (KCRM) and fructose-bisphosphate aldolase A (Aldo A). Based on UniProt and Pfam database for specific domain structure or motif, 44 peptides out of total were positioned in the functional motif or domain from their parent proteins. Using C2C12 myotubes as cell model in vitro, we found several candidate peptides displayed promotive or inhibitory effects on insulin and mitochondrial-related pathways by an autocrine manner. Taken together, this study will encourage us to investigate the biologic functions and the potential regulatory mechanism of these secreted peptides from skeletal muscle tissues, thus representing a promising strategy to treat insulin resistance as well as the associated metabolic disorders.
Introduction
Skeletal muscle is considered as the primary tissue for insulin-stimulated glucose uptake, accounting for up to 80% of the insulin-dependent glucose disposal in whole body glucose homeostasis (1). Accordingly, the dysregulation of skeletal muscle metabolism also arise a number of metabolic disorders such as hyperinsulinaemia, excessive hepatic gluconeogenesis (2), abnormal lipid accumulation (3), impaired glucose uptake and metabolic inflexibility (4). Furthermore, disorders in skeletal muscle play a central role in the development of type 2 diabetic mellitus (T2DM), obesity and lead to other related complications (5). Thus, it is of great interest to deeply characterize the pathogenesis of dysregulation on skeletal muscle glucose/lipid homeostasis to whole-body endocrine and metabolic functions.
As an important secretory organ, skeletal muscle has drawn attention to be a potential target tissue for treating metabolic disorders (6). Thus, analysis of skeletal muscle secretome opens up a novel route for comprehending the communication of this tissue with other tissues such as adipose tissue (7), bone (8), liver (9) and pancreas (10, 11). In light of recently reported experimental evidence, a variety of proteins generated by muscles fibers and released into the circulation are classified as myokines (12), most of which have autocrine, paracrine, and endocrine effects not only in muscle fiber growth (13) but also systemic metabolism (14). The first identified myokine IL-6 presented a vital locally muscular effects such as skeletal muscle growth and glucose/lipid metabolism (15, 16). Furthermore, IL-6 also could be released from contracting muscle, exerting endocrine effects on peripherally insulin sensitive tissues (17–19). Another known contraction-induced myokines including IL-15, IL-8, Irisin, and Myonectin showed potential metabolic function for preventing and treating T2DM (20). These accumulating evidence of myokine from skeletal muscle secretome are central to our understanding of the cross talk between skeletal muscle and other organs during exercise. However, knowledge of the skeletal muscle secretome is scarcely reported under pathophysiology of metabolic diseases such as T2DM and obesity. Identification of more types of muscle-secreted factors and exploration of the potential regulatory mechanisms by which they act remain to be established.
Peptides in length of 3–50 amino acids residues, which are widely characterized in mouse and human, are termed as a sort of compounds produced or secreted by endocrine gland tissues as well as certain types of cells (21, 22). And these endogenous peptides have important physiological action, including neuroregulation (23), cell differentiation (24) and energy metabolism (25) and dysregulation of peptide hormone signaling have been implicated in a wide range of diseases (26, 27). In view of that, further insight into identification of novel peptides is of major importance. Benefited from the progresses in peptide extraction method and application of modern analytical methods, (U)HPLC, nano-LC and CE, hyphenated with tandem mass spectrometry (MS/MS) technology (28, 29), various techniques used for quantitative peptidomics have been applied to address the challenging question of identifying peptides with potential bioactive under the physiological or disease condition (30). More importantly, the peptidomics is widely used to identify biological markers (31), discover new drug (32) and therapeutic targets (33). Recently, quantative peptidomics has also been conducted in endocrine studies (22, 34, 35), however, the secreted peptidomics from skeletal muscle under the insulin-resistant condition was not fully characterized.
Herein, we performed liquid chromatography tandem mass spectrometry (LC-MS/MS) technology to help characterize the secretome from cultured skeletal muscle tissues of db/db mice at peptides level and identify putative bioactive peptides. A global secreted peptides were established and bioinformatics analysis of precursor proteins provided a possible relationship of differential peptides with T2DM or insulin resistance. Additionally, the biological effects of these secreted peptides on C2C12 myotubes elucidated a possible regulatory role in insulin signaling- and mitochondrial-related genes expression. Taken together, these observations will encourage us to investigate function of these secreted peptides from cultured skeletal muscle tissues with other tissues under the diabetic state, thus representing a promising strategy for prevention and treatment of insulin resistance as well as the associated metabolic disorders.
Materials and Methods
Ethics Statement
All the studies involving mice acquired approval from the Ethical Committee of Nanjing Medical University. All procedure involving mice were carried out in accordance with the guidelines of the Institutional Animal Care and Use Committee of Nanjing Medical University (Approval Number: IACUC-1812053).
Animal Experiments and Sample Preparation
Twelve-week-old male C57BLKS/J db/db mice (n = 8, db/db group) and age-matched WT controls (n = 8, NC group) were purchased from the Model Animal Research Center of Nanjing Medical University. After adaptive raising for one week, mice were sacrificed by cervical dislocation and skeletal muscle tissues were isolated from the left hind leg (each mice of 100 ~ 150 mg). Subsequent operations were carried out under a laminar airflow hood to decrease contamination. The visible blood vessels and connective tissue were removed from the tissue. After rinsed with PBS, the skeletal muscle tissues were cut into small pieces (3–4 mm3) with scissors as described by an established protocol (36, 37). Tissue cutting will lead to release of damaged cells slowly into the medium. Additionally, a small amount of serum proteins in the tissue pieces will diffuse out during culture period. Therefore, necessary washing procedures during culture were adopted to obtain medium samples (referred to as secretome) containing mainly skeletal muscle tissue-derived secreted components as previously reported (38, 39). Tissue fragments were placed in a 10 cm plate (200~300 mg from two mice as one sample) containing 10 mL serum/phenol red free DMEM/F12 medium (Gibco, Grand Island, CA, USA). After incubation in a humidified incubator at 37°C under 95% O2 and 5% CO2 for 48 h, the medium was immediately supplemented with protease inhibitors and centrifuged (845 g, 10 min, 4°C) to wipe off cell debris and dead cell. Supernatant samples from each group (NC or db/db group) were harvested from four individual tissue culture dishes independently (n = 4 per group). Lactate dehydrogenase (LDH) (36, 40, 41) and IL-6 (42) expression levels were detected to evaluate skeletal muscle vitality and capability during the in vitro culture. Then the supernatant samples obtained were stored at −80°C until further processing.
Peptide Extraction and Desalting
First, both supernatant samples were concentrated to 1–2 mL by centrifuges for speed vacuum (LaboGene, Allerød, Denmark). Then equal volume of U2 solution containing 8 M urea and 100 mM tetraethyl-ammonium bromide in pH 8.0 was added to the concentrated supernatant for denaturation. Followed by centrifugation for 30 min (13,000 g, 30°C), the medium supernatant was transferred to a new centrifuge tube. Subsequently, proteins were reduced by 10 mM dithiothreitol and alkylated with 55 mM iodoacetamide successively. The protein concentrations of supernatant from cultured skeletal muscle samples were detected by Bradford method (43) and integrity of these samples were evaluated by sodium dodecyl sulfate (SDS)-polyacrylamide gel electrophoresis (PAGE) combined with silver staining. Afterwards the peptides were separated from samples by treatment with Amicon® Ultra Centrifugal Filters in 10-kDa (Merck Millipore, Billerica, MA, USA) according to the manufacturer's instruction as previously described (37). The “peptidome” present in the filtrates was desalted using a Strata X C18 column (Phenomenex, Torrance, CA, USA) and the desalted peptide solution was vacuum-dried with centrifuges for speed vacuum (SCAN SPEED 40, LaboGene) as previously described (44) and immediately frozen at −80°C until the following processing.
Liquid Chromatography Tandem Mass Spectrometry (LC-MS/MS)
The LC-MS/MS analysis was conducted similarly to the previous protocols (45). The peptide samples were redissolved in 2% (v/v) acetonitrile/0.1% formic acid (FA) (v/v) and 5 μL solution was injected into an A Triple TOFTM 5600 mass spectrometer (AB Sciex, Redwood City, CA, USA) coupled to a ekspert™ nanoLC400 liquid chromatography (AB Sciex) via a nanosource electrospray interface equipped with distal coated SilicaTip emitters (New Objective, Woburn, MA, USA). First, load peptide onto a C18 trap column (5 μm, 100 μm ×20 mm, AB Sciex) and elute at 300 nL/min onto a C18 analytical column (3 μm, 75 μm ×150 mm, Welch Materials, Shanghai, China) in the gradient as long as 120 min. These two mobile phases included buffer A containing 2% acetonitrile/ 0.1% FA/ 98% H2O (v/v) and buffer B containing 98% acetonitrile/0.1% FA/2% H2O (v/v). Then peptide mixture was eluted at a flow rate of 0.3 μL/min in a gradient generated with Solvent A containing 98% water and 2% acetonitrile containing 0.1% FA (v/v) and Solvent B containing 2% water and 98% acetonitrile containing 0.1% FA (v/v) according to the previously described reports (45, 46). The mass spectrometer was operated in positive mode with a spray voltage of 2500 V, 206.84kPa for the curtain gas, 41.37kPa for the nebulizer gas and 150°C as temperature. A data dependent acquisition (DDA) method was applied and a full scan MS spectrum (300–1500 m/z) with accumulation time of 0.25 s was adopted. Top 30 precursor ions for fragmentation based on the highest intensity were selected. The collections of MS1 spectra were in the range 350–1500 m/z, and MS2 spectra were in the range of 100–1500 m/z. A total of 47,709 MS/MS were collected from all LC-MS/MS analyses.
Peptide Identification and Quantitative Analysis
Protein Pilot Software (https://sciex.com.cn/products/software/proteinpilot-software, version4.5,AB SCIEX) was adopted to analyze the original MS/MS file data (47). For peptides identification, the Paragon algorithm was employed against the Mus_musculus SwissProt sequence database (a total of 85210 items, updated in January 2019). The following parameters were installed: The parameters were set as follows: 1) cysteine modified with iodoacetamide; 2) biological modifications were selected as ID focus. The strategy of the automatic decoy database search was employed to estimate false discovery rate (FDR) calculation using the Proteomics System Performance Evaluation Pipeline (PSPEP) Software integrated in protein pilot-software. Only unique peptides (global FDR values < 1%) were considered for further analysis. Skyline v4.2 software was employed for MS1 filtering and ion chromatogram extractions for peptides label-free quantification (48, 49). And the parameters setting for skyline MS1 filtering were according to previous studies (48). Using the results of the Skyline quantification, the mean value of the ratio of each group was used to calculate the fold change.
Bioinformatics and Annotations
The relative molecular mass and isoelectric point of each peptide were calculated by the online tool (http://web.expasy.org/compute.pi/). All the precursors protein of differentially expressed peptides as one group were imported into for GO (http://www.geneontology.org/) (50) and pathway analysis (https://www.kegg.jp/) for predicting potential functions. The online tools UniProt Database (http://www.uniprot.org/) and Pfam (http://pfam.xfam.org/) were adopted to explore if the peptides' sequences were positioned in the conserved structural domains or regions of their precursors. The Open Targets Platform database (www.targetvalidation.org/) was adopted to investigate precursors associated with diabetes and obesity as previously reported (24). For visualization, clustergram and volcano plot graphs in this study were drawn with R language (http://www.r-project.org/). For determination of differentially expressed peptides, fold change was computed as the average values of biological duplication (n = 4).
Synthetic Peptides
All the peptides used in this study were custom-synthesized and HPLC-purified by Science Peptide Biological Technology Co., Ltd. (Shanghai, China) through the solid-phase method as described reported (51). The purity in 95% for each peptide was confirmed by HPLC-MS method. All the used peptides were stored in lyophilization at−20°C until dissolved with sterile water immediately for treatment with cells in vitro.
Cell Culture and Peptide Treatment
C2C12 cells, purchased from Cell Bank of the Chinese Academy of Sciences (Shanghai, China), were maintained in DMEM (Gibco, Carlsbad, CA, USA) supplemented with 10% Fetal Bovine Serum (Gibco) and 1% penicillin–streptomycin (Keygen Biotech, Nanjing, China) at 37°C with 5% CO2. On the fourth day of cell differentiation, the C2C12 cells were pre-treated with synthesized peptides or solvent for 48 h at the same concentration of 50 μM, and then starved for 24 h with serum-free L-DMEM (Gibco). Subsequently, myotubes were incubated in L-DMEM in the presence or absence of 100 nM of insulin for 30 min. At the indicated time, the cells were collected for the following analysis.
Western Blot Analysis and Antibodies
At the indicated time, C2C12 myotubes were lysed in Radio Immunoprecipitation Assay (RIPA) lysis buffer containing protease and phosphatase inhibitors (Roche, Mannheim, Germany). Protein concentrations were measured with the BCA Protein Assay Kit (Thermo Fisher Scientific, Rockford, USA). Proteins were loaded and separated by 8%-10% (v/v) SDS-PAGE, transferred to polyvinylidene fluoride (PVDF) membrane and blocked with 5% milk. The membrane was incubated with the determined primary antibodies, respectively overnight at 4 °C as follows: Insulin receptor substrate 1 (Irs-1) (1:1000 in dilution, Cat No: 2382; Cell Signaling Technology, Danvers, MA, USA), Phospho-Irs-1(ser307) (1:1000 in dilution, Cat No: 2381; CST), RAC-alpha serine/threonine-protein kinase (Akt)(1:1000 in dilution, Cat No: 4685; CST), Phospho-Akt (Ser473)(1:1000 in dilution, Cat No: 4060; CST), Pgc1α(1:1000 in dilution, Cat No: ab54481; Abcam, Cambridge, UK), Tublin (1:1000 in dilution, Cat No: 10094-1-AP; Proteintech, Rosemont, USA). Then the membrane was incubated with goat anti-rabbit HRP secondary antibody (1:5000 in dilution, CAT: BL003A; Biosharp, Hefei, China). Proteins bands were visualized using a chemiluminescence kit and analyzed using Image J software.
Reverse Transcription and Real-Time Quantitative PCR
Total RNA was isolated using trizol reagent (Life Technologies, Carlsbad, CA, USA). And the cDNA was synthesized by RevertAid First Strand cDNA Synthesis Kit (Thermo Fisher Scientific, Waltham, MA, USA). Gene expression was determined by real-time quantitative PCR (ABI ViiA7, Applied Biosystems, Foster City, California, USA) using the SYBR Green array. The relative gene expression was analyzed based on the 2−ΔΔCT method with normalization of the data to PPIA. The primers used for the real-time quantitative PCR were listed in Table S1.
Statistical Analysis
Data were analyzed with GraphPad Prism 7 (San Diego, CA, USA), and the results were shown as the mean ± standard deviation (SD). Peptides with a fold change larger than 1.5 or <0.67 with a Student's t-test p-value <0.05 were selected as differently expressed peptides.
Results
Identification of Secreted Peptides From Cultured db/db Skeletal Muscle Tissues
Considering skeletal muscle tissues as an important secretory organ, which communicate with other organs though the secreted proteins, miRNAs, metabolites and others, we were interested in identifying peptides secreted from skeletal muscle tissues under the pathophysiology of metabolic diseases such as diabetes and obesity. LDH and IL-6 release were evaluated in the supernatant from skeletal muscle explants isolated from the control mice, which partly reflected the signs of tissue damage along the incubation period. LDH content of culture medium was assessed as an indicator of cell lysis at 0 h~24 h, 24 h~48 h and 48 h~72 h; no significant increased in LDH occurred from 48 h to 72 h (not shown in the manuscript). Secretion of IL-6 remained stable from 24 h to 48 h in culture by ELISA (not shown in the manuscript). Therefore, we chose the 24 h~48 h culture as an optimal time point. The protein composition and integrity of the secreted samples were evaluated by SDS-PAGE with silver staining in Figure S1. After validation of skeletal muscle culture system and samples assessment, peptides from four different culture dishes per group (control and db/db mice) were individually extracted from supernatants and analyzed via label-free mass spectrometry based quantification. To gain more insights into biological differences between control and db/db mice skeletal muscle, we employed LC-MS/MS technology and identified 3384 peptides, of which 2664 peptides showed valid quantitative values in the detection. A total of 63 peptides were identified differentially secreted, of which 42 peptides were up-regulated (fold change > 1.5, P < 0.05) and 21 peptides were down-regulated (fold change < 0.67, P < 0.05) in medium supernatants from insulin resistant-skeletal muscle tissues. Visualization methods such as hierarchical clustering and volcano plot showed distinct peptides secretion profiles as shown in Figures 1A,B. The differentially secreted peptides were summarized in Table 1.
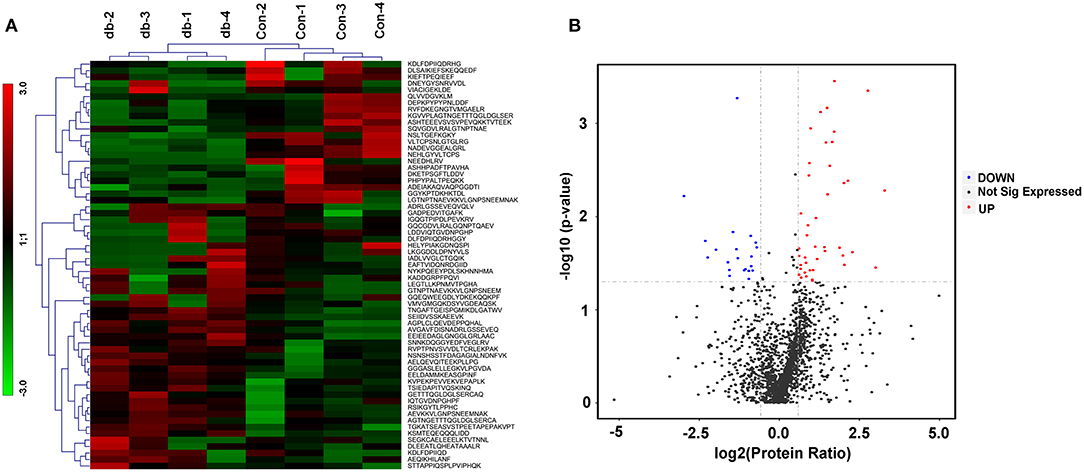
Figure 1. Differentially secreted peptides from the cultured skeletal muscle tissues from db/db and Control mice. (A) Heatmaps highlight the peptides intensity patterns differentially secreted from the medium supernatants of cultured skeletal muscle tissues from control (con) vs. db/db (db) groups, n = 4 per group. (B) Volcano plot after two-sample t test for peptides detected in medium supernatants derived from the cultured skeletal muscle from control and db/db mice. Red color indicates peptides we defined as up-regulated peptides and blue indicates down-regulated peptides.
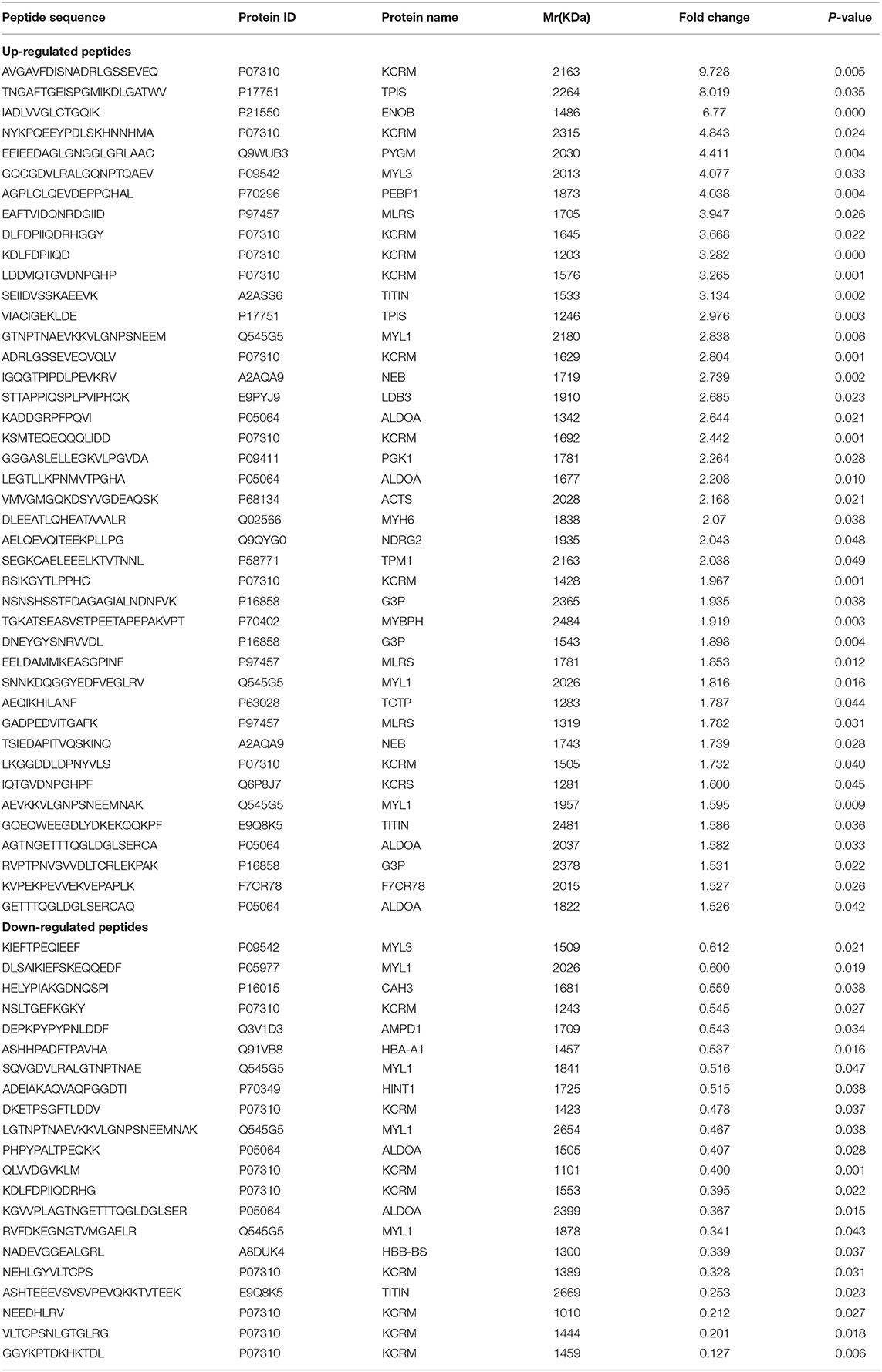
Table 1. Differentially expressed peptides secreted from the medium supernatants of cultured skeletal muscle tissues from db/db and control mice.
Charactrization of the Basic Feature of Differentially Secreted Peptides
To characterize the general feature of differentially secreted peptides, we further analyzed the relative molecular mass (Mr), isoelectric point (pI) and distribution of Mr vs. pI. The results indicated that the Mr varied from 1011.1 Da to 2670.9 Da with 70% between 1,300 and 2,100 Da (Figure 2A), and pI varied from 3.7 to 9.7, with 51% between 4 and 7 (Figure 2B). Additionally, distribution of pI vs. Mr could divide these peptides into three groups around pI4, pI6 and pI10 (Figure 2C).
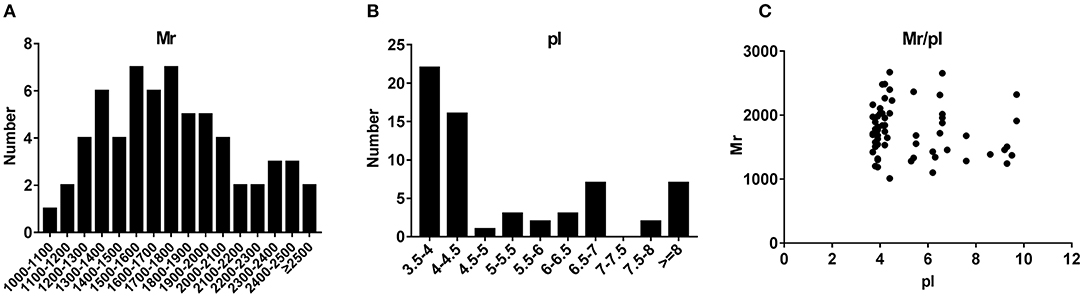
Figure 2. Charactrization of the basic feature of differentially secreted peptides. (A) Histogram displaying the Mr distribution of differentially secreted peptides from skeletal muscle peptidomics. (B) Histogram displaying the pI distribution of differentially secreted peptides from skeletal muscle peptidomics. (C) Scatter plot of Mr vs. pI.
Comprehensive Functional Assessment and Intercellular Location of Precursor Proteins of These Differential Peptides
To make comprehensive functional assessment of these differential peptides, we consider all the precursor proteins as one group and performed GO and Pathways analysis of them. First of all, the precursor proteins of these differential peptides identified from the cultured control and db/db skeletal muscle were classified using Gene Ontology categories, which revealed the majority of proteins were associated with striated muscle contraction and glucose metabolic process (Figure 3A). The top 20 GO terms were listed in Figure 3A. The precursor proteins annotated involved in these GO terms were presented in Table S2. Subsequent pathway analysis in the KEGG database revealed a significant enrichment in metabolic pathway and glycolysis/gluconeogenesis process (Figure 3B). The top 14 pathway terms were listed in Figure 3B. The precursor proteins annotated involved in these pathway terms were presented in Table S3. We further discriminated the up- and down-regulated peptides and performed GO and Pathways analysis of these two groups of precursor proteins. This analysis may bring overlapping terms such as phosphorylation, phosphagen metabolic process and phosphorus metabolic process in up- or down-regulated peptides, and a smaller number of terms in Pathway analysis (deriving up-regulated peptides). These analysis were presented in Figure S2. Additionally, the major intracellular locations of the precursor proteins were considered from literature sources, illustrating that 64% proteins were cytoplasmic and 15% proteins were cytoskeletal seen in Figure 3C. Based on above analysis, we found that a vast of precursor proteins were assigned to glucose metabolic process include ALDO A, triosephosphate isomerase (TPIS), beta-enolase (ENOB), glycogen phosphorylase, muscle form (PYGM), glyceraldehyde-3-phosphate dehydrogenase (GAPDH) and phosphoglycerate kinase 1(PGK1), specifically assigned to glycolysis/gluconeogenesis process. Another kind of proteins enriched in regulation of striated muscle contraction terms (GO) and regulation of actin cytoskeleton (Pathway) were myosin regulatory light chain 2, skeletal muscle isoform(MLRS), myosin heavy chain 6 (MYH6), myosin heavy chain 7 (MYH7), myosin-binding protein H (MYBPH) and myosin light chain 3 (MYL3),both belonging to sarcomeric proteins. Subsequently, we used search tool for the retrieval of interacting genes/proteins (STRING) to construct a graphical network for describing the interaction between these precursor proteins corresponding to the identified peptides in Figure 3D. Based on protein-protein interaction and co-occurrence in KEGG pathways and literature mining, this network was constructed from 25 proteins that matched to the STRING database and the matching results were listed in Table S4. As shown from this network, 22 nodes representing precursor protein constituted the interaction diagram, which could form 93 reliable a-to-b interaction relationship by combined score (more than 0.4) as shown in Table S5.
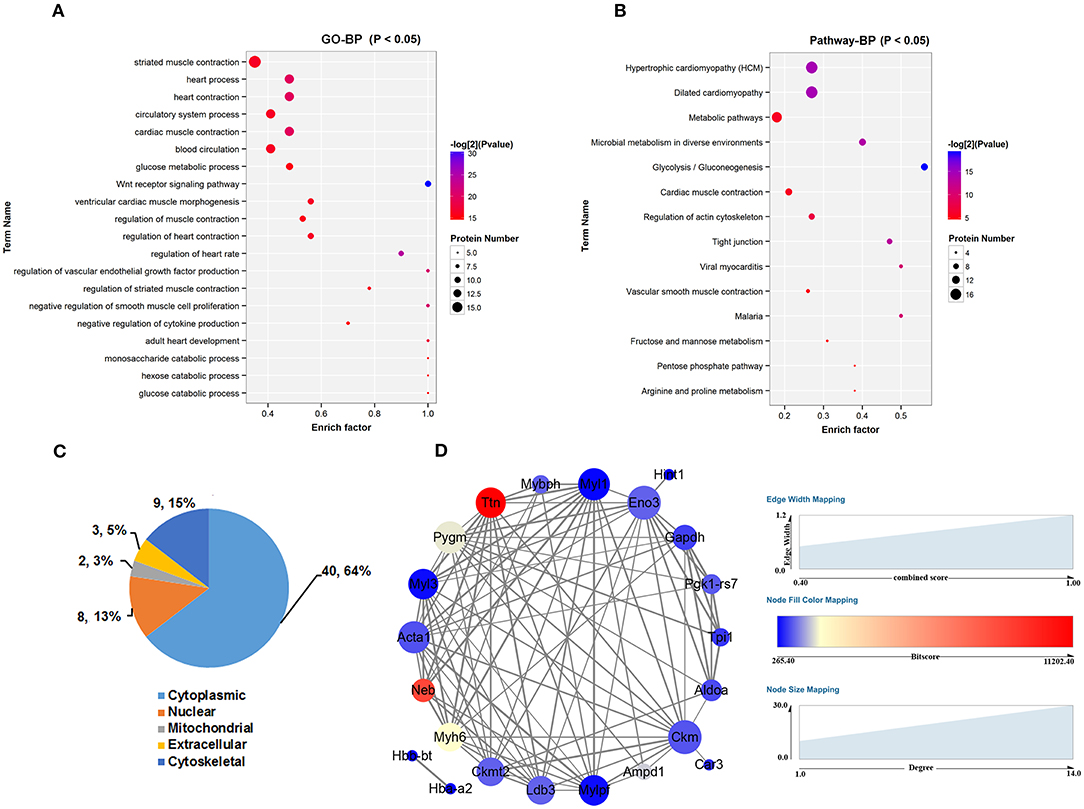
Figure 3. Comprehensive location and functional assessment of precursor proteins of these differential peptides. (A) GO analysis of precursor proteins and TOP 20 GO terms in the biological process categories. (B) Canonical pathways analysis of precursor proteins and TOP 14 pathways terms in the biological process categories. (C) Distribution of intercellular location of precursor proteins. (D) Search tool for the retrieval of interacting genes/proteins (STRING) was used to construct a graphical network for describing the interaction between these precursor proteins corresponding to the identified peptides. The size of node represents the number of interacting proteins and the color of node represents the bitscore of matching results from STRING database. The thicker the line (edge), the higher the reliability (evaluated by combined score >0.4).
Cleavage Pattern of Differentially Secreted Peptides
It is widely postulated that most peptides can be attributed mainly to the proteolytic enzymes as well as their type and level (cleavage specificity and activity), which can differ during disease (52–54). Briefly, the N-terminal pre-cleavage site, N terminus, C terminus, and C-terminal post-cleavage site of the identified peptides were commonly used to investigate the nature of proteolytic enzymes within serum (55) or tissues (56). Thus, we analyzed the distribution of peptide cleavage site and found that Lysine (K) was the most frequent cleavage site of N-terminal amino acid of identified peptides and Alanine (A) was the most frequent cleavage site of C-terminal amino acid of identified peptides. Lysine (K) was the most frequent cleavage site of N-terminal amino acid of preceding peptides, while Leucine (L) was the commonest C-terminal amino acid of preceding peptides (Figure 4A). These data indicate that the pattern of cleavage sites may represent the specificity of cleavage and activity of proteolytic enzymes under the diabetic condition. Additionally, we also tried to align peptide sequences on the same precursor sequence to construct a “peptide alignment map”. Searched from our samples, the largest number of identified peptides (n = 1 7) (Figure 4B) originated from KCRM and the second largest number of identified peptides (n = 6) came from ALDO A (Figure 4B), elucidating that these peptides were easily cleaved by certain kind of endogenous enzymes.
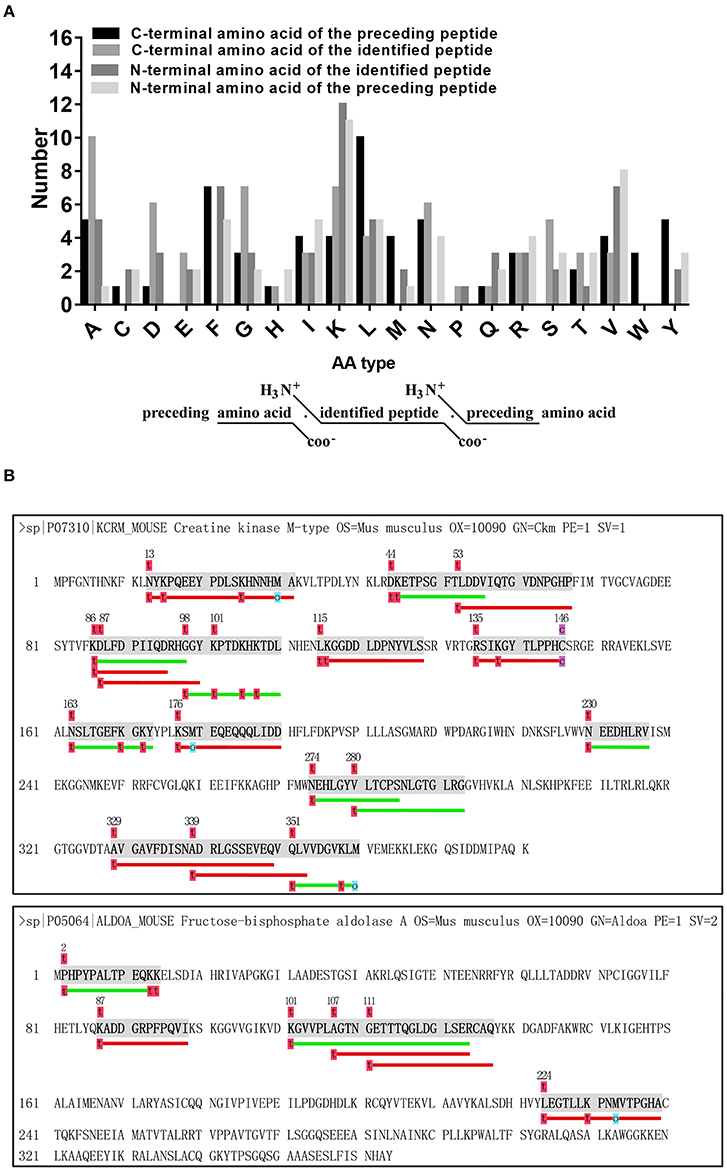
Figure 4. Analysis of cleavage pattern of differentially secreted peptides. (A) Distribution of the four cleavage sites in the identified differentially secreted peptides. (B) Peptides derived from the same precursor protein KCRM or ALDOA.
Putative Bioactive Peptides Associated With Diabetes and Obesity
To check for specific domain structures or patterns in the identified peptides comparison with its precursor proteins, we retrieved domain information from the UniProt and Pfam database. In order to validate that if the peptides exerted important roles in the related metabolic diseases, we adopted the Open Targets Platform database to investigate protein precursors. In our searching results, most peptides (n = 44) from the identified pepetides (n = 63) were located in the functional domains (Table 2). Additionally, 27 out of 44 peptides located in the functional domain were predicted closely related with both obesity and diabetes (Table 3). These observations will encourage us to further investigate properties of the putative secreted peptides in skeletal muscle under the diabetic state.
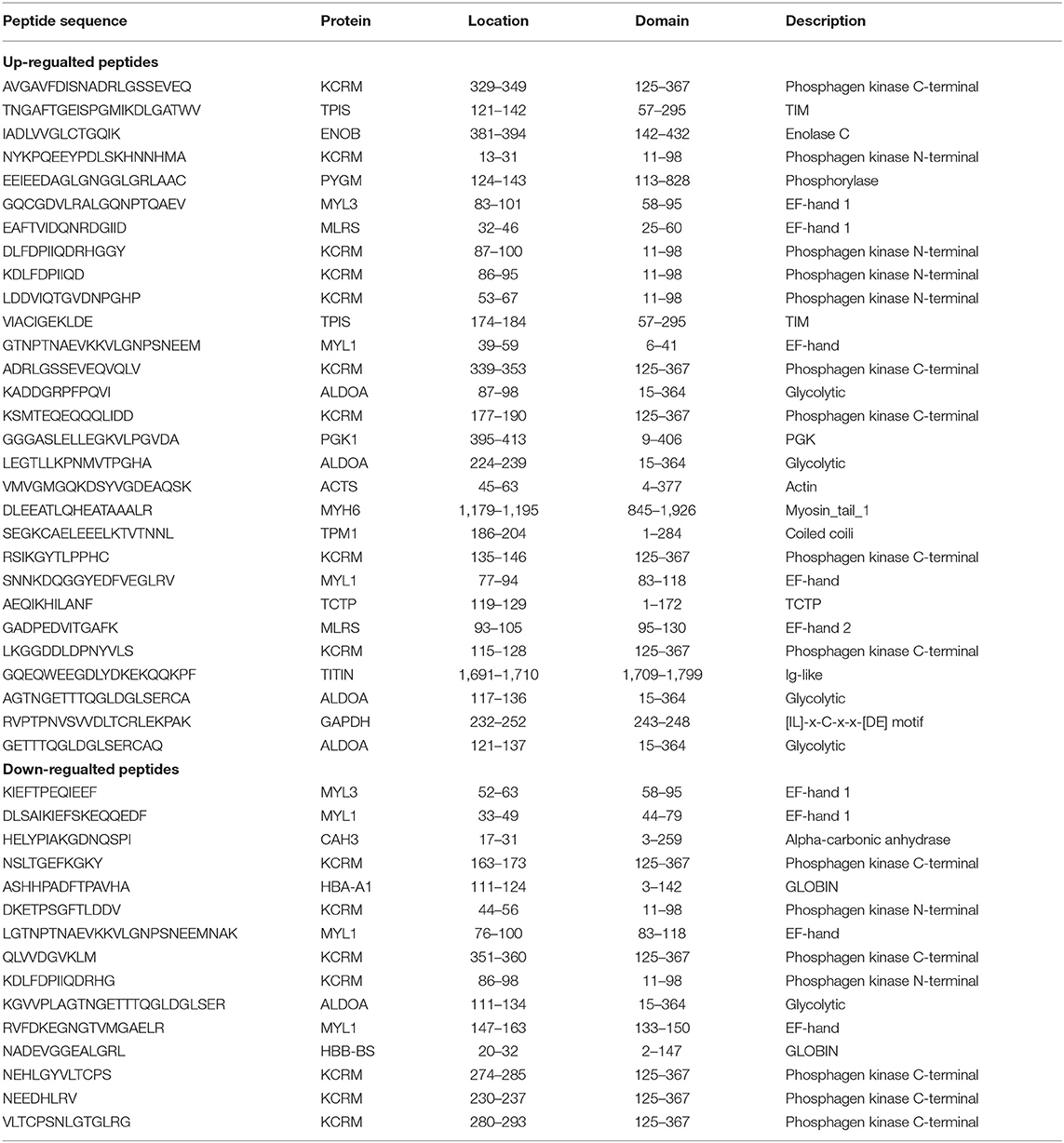
Table 2. Differentially secreted peptides located in functional domain or region based on Uniprot or Pfam database.
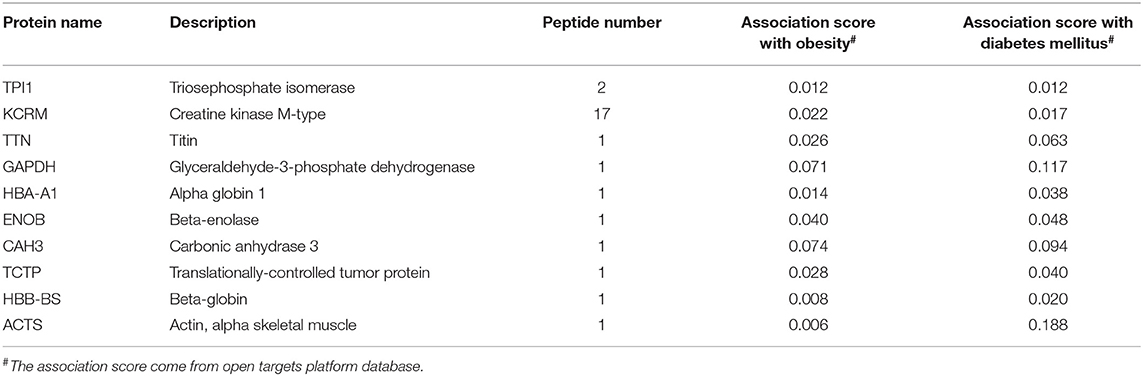
Table 3. Protein precursors of peptides which are both associated with obesity and diabtetes (Score >0).
The Effect of Candidated Peptides (P1-P7) on Insulin and Mitochondrial-Related Pathways in Skeletal Muscle Cells
To explicit the putative function of differentially secreted peptides, 7 differentially secreted peptides, which had already been annotated derived from authentic protein from Uniprot database, located in the functional motifs and showed relatively high abundance from MS detection, were chosen for further analysis. The up-regulated peptides were AVGAVFDISNADRLGSSEVEQ (KCRM/P07310), ADRLGSSEVEQVQLV (KCRM/P07310), GADPEDVITGAFK (MLRS/P97457), RVPTPNVSVVDLTCRLEKPAK (GAPDH/P16858), whereas the down-regulated peptides were DKETPSGFTLDDV (KCRM/P07310), NEHLGYVLTCPS (KCRM/P07310), VLTCPSNLGTGLRG (KCRM/P07310) as listed in Table S6. Sequentially, we termed these candidated peptides P1-P7 in order. To use C2C12 myotubes as cell models in vitro, the results of changes in inuslin signaling revealed that up-regulated peptides P2-P4 addition could both significantly decrease the phosphorylation of Irs1 (Ser-307) and Akt (Ser-473) under insulin stimulation (100 nM, 30 min) as shown in Figures 5A,B, in spite of bringing a modestly up-regulation of p-Irs1 (Ser-307) at the basal status. Moreover, mitochondrial-related marker protein Pgc1α was significantly attenuated in the insulin-stimulated C2C12 cells by up-regulated peptides P1-P4 as shown in Figures 5E,F. Among these down-regulated peptides, only peptide 7 presented a promotion on p-Akt (Ser-473) expression level both under the basal and insulin stimulation status as shown in Figures 5C,D. And these up-regulated peptide 1-4 could decrease Pgc1α protein level and down-regulated peptide 6 and 7 administration enhanced Pgc1α protein level by the stimulation of insulin presented in Figures 5E,F. We further evaluated Gut 4 (a key gene for glucose transport) and Pgc1α mRNA levels by peptides treatment upon insulin stimulation. The results also indicated that Peptide 3 and 4 modestly decreased Pgc1αexpression at mRNA levels seen in Figure 5G, while Peptide 6 and 7 significantly up-regulated both Glut4 and Pgc1α expression at mRNA levels seen in Figure 5H. These observations revealed that these candidated peptides (P1-P7) may affect the expressions of insulin signaling or mitochondrial-related genes in skeletal muscle cells.
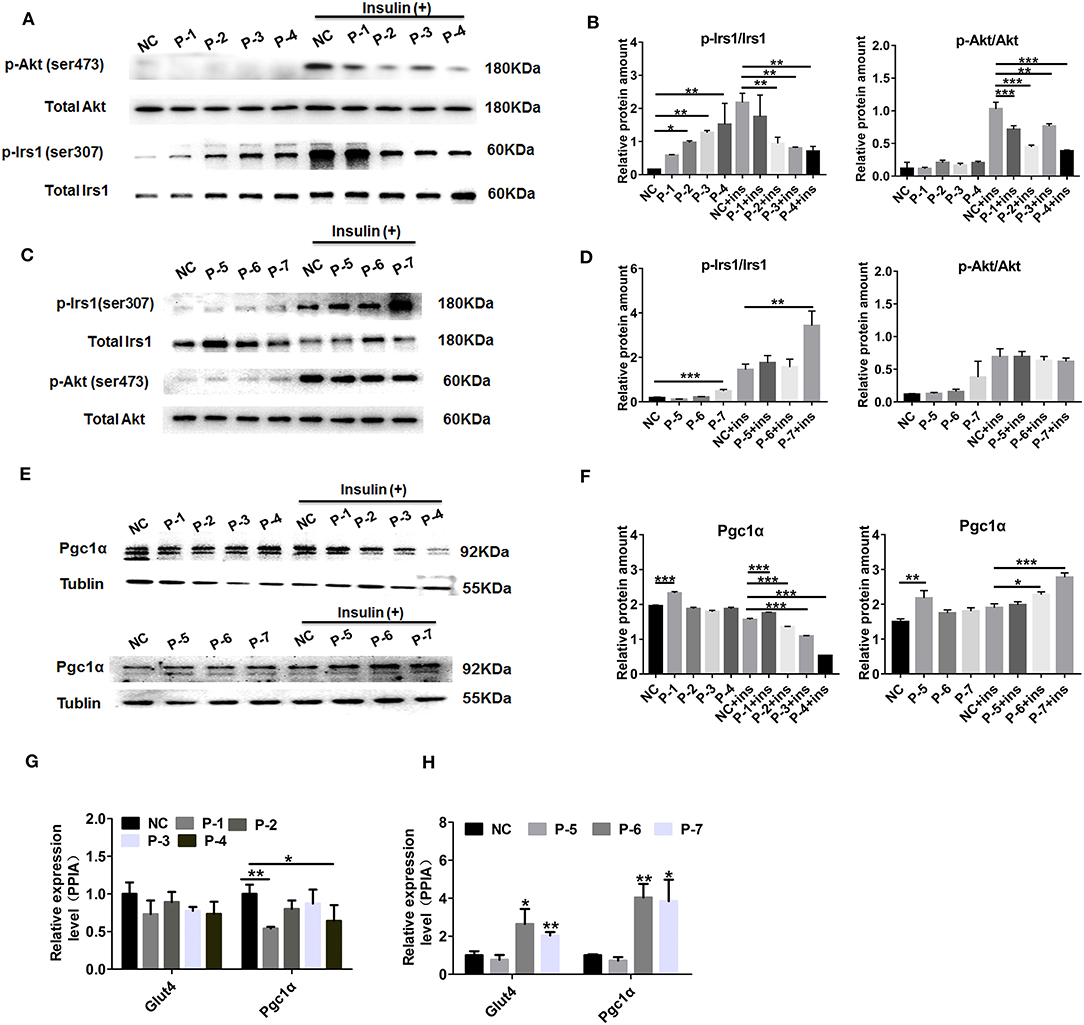
Figure 5. Effect of the candidate peptides on insulin and mitochondrial-related pathways. The differentiated C2C12 motes were treated with the candidate peptides (50 uM) named P1–P7 groups or solvent termed untreated groups. After incubation with or without 100 nM insulin for 30 min, cell lysates were analyzed by Western blot. (A,C) Total protein and phosphorylation of Irs1 at ser307 (p-Irs1) and Akt at ser473 (p-Akt). (B,D) Quantification of the protein levels of p-Irs1 (ser307) and p-Akt (ser473) relative to total protein, respectively. (E) Mitochondrial-related protein Pgc1α was detected and Tublin was used as internal control. (F) Quantification of the protein levels of Pgc1α relative to Tublin. Values are the means ± SD of three separate experiments. (G,H) Real-time quantitative PCR detection of the mRNA levels of Glut4 and Pgc1 relative to internal control PPIA, respectively. Values are the means ± SD of three separate experiments. *P < 0.05; **P < 0.01; ***P < 0.001.
Discussion
Emerging as a widely known secrete organ, skeletal muscle tissues have been extensively discussed using a wide range of comparative and quantitative proteomic methods (57). Proteomic scannings of the medium supernatant from skeletal muscle cells have already afford approaches to identify a large number of secreted proteins (58–63). Particularly, one such research reported the alteration of insulin effect on the secretome profile of skeletal muscle cells, revealing the changes of protein levels secreted from skeletal muscle during activation of insulin signaling pathway (58). A recent study also generated a comprehensive secretome analysis of skeletal muscle cells under palmitic acid-induced insulin resistance (61). In addition to these proteins, many other muscle-secreted compounds have been also come to light, including exosome (64, 65), metabolites (66), and miRNAs (67). These studies have greatly expanded our knowledge of skeletal muscle secretome and might afford possibilities for exploring novel molecular targets in the maintenance of skeletal muscle physiology and even whole-body metabolism. However, to date few studies has focused on the peptides present in either skeletal muscle tissues or cells. Therefore, we attempted to comprehensively profile peptides that may play roles in regulating insulin sensitivity and offer enormous promise for exploring molecular mechanisms underlying insulin resistance.
In present study, a total of 63 peptides were differentially secreted in medium supernatants from cultured skeletal muscle tissues of db/db mice, of which 42 peptides were up-regulated (fold change > 1.5, P < 0.05) and 21 were down-regulated (fold change < 0.67, P < 0.05) shown in Figures 1A,B. The differences of dysregulation peptides may indeed reflect the changes between control and insulin-resistant mice in some extent. However, db/db mouse is a well-established leptin receptor-deficient animal model (68, 69). Despite no studies revealed the association between leptin receptor deficiency and peptide dysregulation/protein degradation so far, the possible effect by this mutation deserves to be taken into account and other non-genetic mice models could be adopted in the future studies. Based on our previous study (70), 10-kDa filters method was used to extract peptides in order to intercept proteins secreted by skeletal muscle to the conditional media. Characterization of the basic feature (Mr and pI) of these identified peptides (Figures 2A,B) only reflected the differences of distribution in the diabetic skeletal muscle tissues, but also proved the reliability of the used peptide extraction method.
Subsequently, GO and Pathway analysis revealed that the precursors protein of these peptides were mostly involved in muscle contraction and metabolism processes (Figures 3A,B). An interesting finding from our study was that most of the differentially identified peptides derived from cytosolic, cytoskeletal and mitochondrial proteins (Figures 3A,B), which differed from the secretory pathway peptides (71). This observation was also demonstrated by other peptidomic studies. From Steven W. Taylor group' results, several identified peptides in the human islet cultures were derived from intracellular and cytoskeletal proteins such as microtubule-associated protein 4 and ubiquitin, which may result from a greater level of cellular stress (34). Similarly, another peptidomic anaylsis of brain slices cultures and media also pointed that vast majority of secreted peptides arose from intracellular proteins (72). There does also exist some evidence that these identified N- or C-terminus protein yielding peptides, rather than internal fragments, raised the possibility that they are produced by selective processing rather than protein degradation (73). Taken together with previous researches, the current results show us meaningful hints that these intracellular peptides may be secreted via non-classical mechanisms. Actually, another important origin of peptides is proteolytic degradation processes in body fluid under the physiological or pathologically processes. Most regulatory peptides were efficiently degraded by plasma enzymes once secreted into the bloodstream, which exerted anti-diabetic therapeutic function (74, 75) or were identified as disease markers (76). Recently, Federico Aletti et al. (77) used protease activity detection and specific enzymes analysis to explain a large presence of circulating peptides under hemorrhagic shock, which gave us a possible way to evaluate peptides origin. Thus, a fundamental validation is whether the proteins-derived peptides are actually secreted from skeletal muscle cells per se or proteolytic degradation and are of biologically active.
Among the differentially secreted peptides from cultured skeletal muscle tissues of db/db mice, we found that three peptides derived from GAPDH, four peptides derived from ALDO A and one peptides derived from PYGM were up-regulated in the conditional medium from db/db groups as shown in Table 1. As described by Dustin S. Hittel et.al, a global proteomic survey of skeletal muscle revealed a statistically significant up-regulation in glycolytic enzymes GAPDH and ALDO A protein levels in obese/overweight patients (78). Another quantitative protein profile also identified a more abundant levels of GAPDH and PYGM in skeletal muscle from T2DM groups compared with the control groups (79). In addition to pointing the importance of the mitochondrial numbers and impairments under the insulin-resistant states (80–82), several studies noted that glycolytic capacity is higher in skeletal muscle of patients with T2DM or obesity (83). Notably, stronger changes of peptides derived from sarcomeric proteins such as myosin light chain 1 (MYL1) and MYL3 were also observed in the conditional medium from diabetic muscles as shown in Table 1. MYL1 and MYL3 are representive markers of fast-muscle and slow-muscle respectively, which were both regulated by insulin stimulation (79). And previous studies observed that property of T2DM individuals muscle was shifted to a fast-twitch glycolytic phenotype (84). In fact, deficiency in sarcomeric proteins in skeletal muscle also suggested their importance in skeletal muscle physiologic and pathological processes. On the whole, our results of increased glycolytic enzyme- or sarcomeric proteins- derived peptides secreted from insulin resistant skeletal muscle may support the hypothesis that altered glycolytic capacities or fiber types under the diabetic status contribute to this difference.
Till now, the putative function of these peptides are not clear. Therefore, we evaluated whether these peptides originated from functional domains of the corresponding precursor protein using the UniProt and Pfam database. In our searching results, most peptides (n = 44) from the identified peptides (n = 63) were located in the functional domains as listed in Table 2. Specifically, most peptides derived from functional enzymes were located in the enzymatic activity region, including TPI-derived peptides (121-TNGAFTGEISPGMIKDLGATWV-142, 174-VIACIGEKLDE-184), PGK-derived peptide (395-GGGASLELLEGKVLPGVDA-413), ALDOA-derived peptides (87-KADDGRPFPQVI-98, 224-LEGTLLKPNMVTPGHA-239, 117-AGTNGETTTQGLDGLSERCA-136, 121-GETTTQGLDGLSERCAQ-137), TCTP-derived peptide (119-AEQIKHILANF-129) and CAH3-derived peptide (17- HELYPIAKGDNQSPI-31). We also found another kind of peptides (n = 9) were located in the EF-hand domain from sarcomeric proteins (MYL1, MYL3 and MLRS). Generally, all EF-hand proteins display regulatory effect in two ways (85), calcium sensors for translating the signal to various responses and calcium buffers for controlling free Ca2+ ions level in the cytoplasm. On the other side, Ca2+ binding could induce a change of structural dynamics in the EF-hand motif, resulting in the activation or inactivation of target proteins (86, 87). Importantly, Ca2+ signal participates a variety of physiological processes in skeletal muscle, especially acting as second messengers for GLUT4 translocation mediated by contraction (88) and insulin treatment (89). Additionally, GAPDH-derived peptide (231- RVPTPNVSVVDLTCRLEKPAK−252) contained [IL]-x-C-x-x-[DE] motif (243-248), which has been reported as S-Nitrosylation modifying sites for affecting GAPDH enzymatic activity (90). Therefore, future efforts need to be established for investigating the potential role of peptides on insulin sensitive cells in vitro or whole-body metabolism in vivo.
The above analyses provided a possibility to evaluate the biological effects of these differentially secreted peptides. As widely reported, insulin resistance in skeletal muscle is tightly connected with the deficit in insulin signaling (91). Consequently, the role of phosphorylation of Irs1 and Akt in signaling pathways is very crucial in anti-hyperglycemia and insulin sensitivity (92). In this result, we found these up-regulated peptides 1–4 both exerted a significantly attenuated insulin action in C2C12 cells, evaluated by a decreased level of p-Irs1 (Ser-307) and p-Akt (Ser-473) seen in Figures 5A,B, whereas only one down-regulated peptide P7 could remarkably promote insulin signaling only via Irs1 signaling pathway seen in Figures 5C,D. Peroxisome proliferator-activated receptor γ coactiva-tor-1(Pgc-1), which displays a dominant role through tight modulation of mitochondrial biogenesis and respiration, has also been demonstrated to participate in skeletal muscle insulin signaling and metabolic homeostasis (93). The up-regulated peptides 1–4 also brought out a decreased protein level of Pgc-1α in C2C12 cells as shown in Figures 5E,F, and down-regulated peptide 6 and 7 administration also gave rise to the increased Pgc1α mRNA and protein level in Figures 5E,F,H. Taken together, these selected peptides secreted from db/db mice skeletal muscle presented a promotive or inhibitory effect on insulin and mitochondrial-related pathways in skeletal muscle cells by an autocrine manner. Notably, peptide 4 (231- RVPTPNVSVVDLTCRLEKPAK−252) derived from GAPDH displayed a most significant inhibitory effect toward these candidate peptides. However, the relationship between GAPDH-derived peptide and its precursor protein is to be determined. On the other hand, more methods need to be employed for the wider cell signaling screen and further research is required to explore the biologic function of skeletal muscle-secreted peptides on adipocytes or liver cells.
To our knowledge, no large-scale quantitative peptidomic analysis has been performed on skeletal muscle to elucidate secreted peptides profiles under the diabetic status. The present study identified and quantified changes with a label-free discovery using LC-MS/MS technology to construct a global secreted peptides picture. Further bioinformatics analysis of precursors comprehensively provided an atlas of peptides that may exist roles in regulating insulin sensitivity. This represented a new perspective toward exploring insulin resistance pathogenesis. Additionally, the detailed biological effects of these secreted peptides on skeletal muscle insulin resistance or cross-talk with other tissues remained to be elucidated in the future study.
Data Availability Statement
The raw data supporting the conclusions of this manuscript will be made available by the authors, without undue reservation, to any qualified researcher.
Ethics Statement
The protocol has been approved by the Institutional Animal Care and Use Committee of Nanjing medical university (Approval Number: IACUC-1812053).
Author Contributions
YWu and MH performed experiments and interpreted results of experiments. YWa and YG prepared the figures. XC and PX analyzed the data. CJ and TZ participated discussion. YZ helped write the manuscript with providing assistance. LY conceived and designed experiments, provided funding to regents, and approved final version of manuscript. All authors read and approved the final manuscript.
Funding
This study was supported by National Natural Science Foundation of China (Grant No. 81600687, 81700738, 81770866, 81770837, 81870546), the Jiangsu Provincial Key Research and Development Program (BE2016619), Jiangsu Provincial Medical Innovation Team Program, 333 high level talents training project of Jiangsu Province, Jiangsu Provincial Medical Youth Talent, Jiangsu Province Natural Science Foundation (BK20170153), Nanjing Medical Science and Technique Development Foundation (Grant No. QRX17160, YKK17177), Science and Technology Development Foundation Item of Nanjing Medical University (Grant No. 2017NJMUZD067), and Postgraduate Research & Practice Innovation Program of Jiangsu Province (Grant No. JX22013535).
Conflict of Interest
The authors declare that the research was conducted in the absence of any commercial or financial relationships that could be construed as a potential conflict of interest.
Supplementary Material
The Supplementary Material for this article can be found online at: https://www.frontiersin.org/articles/10.3389/fendo.2019.00741/full#supplementary-material
Figure S1. SDS-PAGE associated with silver staining of the medium supernatants from cultured skeletal muscle tissues (Con vs. db groups).
Figure S2. Functional assessment of precursor proteins deriving up- and down-regulated peptides, respectively. (A,B) GO analysis of precursor proteins deriving up- and down-regulated peptides, respectively. (C,D) Canonical pathways analysis of precursor proteins deriving up- and down-regulated peptides, respectively.
Table S1. Primers used in this study.
Table S2. GO terms corresponded to the precursor proteins relative to these differentially secreted peptides in skeletal muscle from db/db mice.
Table S3. Pathway terms corresponded to the precursor proteins relative to these differentially secreted peptides in skeletal muscle from db/db mice.
Table S4. The matching results of the precursor proteins from STRING database.
Table S5. Precursor proteins-to-precursor proteins interaction from STRING database.
Table S6. The information of candidate peptides for functional evaluation.
Abbreviations
T2DM, type 2 diabetic mellitus; GO, gene ontology; KCRM, creatine kinase m-type; Aldo A, fructose-bisphosphate aldolase A; Mr, relative molecular mass; pI, isoelectric point; LC-MS/MS, Liquid chromatography tandem mass spectrometry; FA, formic acid; DDA, data dependent acquisition; FDR, false discovery rate; PSPEP, Proteomics System Performance Evaluation Pipeline; RIPA, Radio Immunoprecipitation Assay; PVDF, polyvinylidene fluoride; SDS, sodium dodecyl sulfate; PAGE, polyacrylamide gel electrophoresis; SD, standard deviation; TPIS, triosephosphate isomerase; ENOB, beta-enolase; PYGM, glycogen phosphorylase, muscle form; GAPDH, glyceraldehyde-3-phosphate dehydrogenase; PGK1, phosphoglycerate kinase 1; MLRS, myosin regulatory light chain 2, skeletal muscle isoform; MYH6, myosin heavy chain 6; MYH7, myosin heavy chain 7; MYBPH, myosin-binding protein H; MYL3, myosin light chain 3; MYL1; LDH, myosin light chain 1; lactate dehydrogenase.
References
1. Thiebaud D, Jacot E, DeFronzo RA, Maeder E, Jequier E, Felber JP. The effect of graded doses of insulin on total glucose uptake, glucose oxidation, and glucose storage in man. Diabetes. (1982) 31:957–63. doi: 10.2337/diacare.31.11.957
2. Li LO, Grevengoed TJ, Paul DS, Ilkayeva O, Koves TR, Pascual F, et al. Compartmentalized acyl-CoA metabolism in skeletal muscle regulates systemic glucose homeostasis. Diabetes. (2015) 64:23–35. doi: 10.2337/db13-1070
3. Wu W, Xu Z, Zhang L, Liu J, Feng J, Wang X, et al. Muscle-specific deletion of Prkaa1 enhances skeletal muscle lipid accumulation in mice fed a high-fat diet. J Physiol Biochem. (2018) 74:195–205. doi: 10.1007/s13105-017-0604-y
4. Consitt LA, Saxena G, Saneda A, Houmard JA. Age-related impairments in skeletal muscle PDH phosphorylation and plasma lactate are indicative of metabolic inflexibility and the effects of exercise training. Am J Physiol Endocrinol Metab. (2016) 311:E145–56. doi: 10.1152/ajpendo.00452.2015
5. Petersen KF, Dufour S, Savage DB, Bilz S, Solomon G, Yonemitsu S, et al. The role of skeletal muscle insulin resistance in the pathogenesis of the metabolic syndrome. Proc Natl Acad Sci USA. (2007) 104:12587–94. doi: 10.1073/pnas.0705408104
6. Giudice J, Taylor JM. Muscle as a paracrine and endocrine organ. Curr Opin Pharmacol. (2017) 34:49–55. doi: 10.1016/j.coph.2017.05.005
7. Boström P, Wu J, Jedrychowski MP, Korde A, Ye L, Lo JC, et al. A PGC1-alpha-dependent myokine that drives brown-fat-like development of white fat and thermogenesis. Nature. (2012) 481:463–8. doi: 10.1038/nature10777
8. Tagliaferri C, Wittrant Y, Davicco MJ, Walrand S, Coxam V. Muscle and bone, two interconnected tissues. Ageing Res Rev. (2015) 21:55–70. doi: 10.1016/j.arr.2015.03.002
9. Montgomery MK, Mokhtar R, Bayliss J, Parkington HC, Suturin VM, Bruce CR, et al. Perilipin 5 deletion unmasks an endoplasmic reticulum stress-fibroblast growth factor 21 axis in skeletal muscle. Diabetes. (2018) 67:594–606. doi: 10.2337/db17-0923
10. Christensen CS, Christensen DP, Lundh M, Dahllöf MS, Haase TN, Velasquez JM, et al. Skeletal muscle to pancreatic β-Cell cross-talk: the effect of humoral mediators liberated by muscle contraction and acute exercise on β-cell apoptosis. J Clin Endocrinol Metab. (2015) 100:E1289–98. doi: 10.1210/jc.2014-4506
11. Kozinski K, Jazurek M, Dobrzyn P, Janikiewicz J, Kolczynska K, Gajda A, et al. Adipose- and muscle-derived Wnts trigger pancreatic beta-cell adaptation to systemic insulin resistance. Sci Rep. (2016) 6:31553. doi: 10.1038/srep31553
12. Pedersen L, Hojman P. Muscle-to-organ cross talk mediated by myokines. Adipocyte. (2012) 1:164–7. doi: 10.4161/adip.20344
13. Lightfoot AP, Cooper RG. The role of myokines in muscle health and disease. Curr Opin Rheumatol. (2016) 28:661–6. doi: 10.1097/BOR.0000000000000337
14. Eckardt K, Görgens SW, Raschke S, Eckel J. Myokines in insulin resistance and type 2 diabetes. Diabetologia. (2014) 57:1087–99. doi: 10.1007/s00125-014-3224-x
15. Nieto-Vazquez I, Fernández-Veledo S, de Alvaro C, Lorenzo M. Dual role of interleukin-6 in regulating insulin sensitivity in murine skeletal muscle. Diabetes. (2008) 57:3211–21. doi: 10.2337/db07-1062
16. Al-Khalili L, Bouzakri K, Glund S, Lönnqvist F, Koistinen HA, Krook A. Signaling specificity of interleukin-6 action on glucose and lipid metabolism in skeletal muscle. Mol Endocrinol. (2006) 20:3364–75. doi: 10.1210/me.2005-0490
17. van Hall G, Steensberg A, Sacchetti M, Fischer C, Keller C, Schjerling P, et al. Interleukin-6 stimulates lipolysis and fat oxidation in humans. J Clin Endocrinol Metab. (2003) 88:3005–10. doi: 10.1210/jc.2002-021687
18. Kim HJ, Higashimori T, Park SY, Choi H, Dong J, Kim YJ, et al. Differential effects of interleukin-6 and−10 on skeletal muscle and liver insulin action in vivo. Diabetes. (2004) 53:1060–7. doi: 10.2337/diabetes.53.4.1060
19. Barlow JP, Solomon TP. Do skeletal muscle-secreted factors influence the function of pancreatic beta-cells? Am J Physiol Endocrinol Metab. (2018) 314:E297–307. doi: 10.1152/ajpendo.00353.2017
20. Carson BP. The potential role of contraction-induced myokines in the regulation of metabolic function for the prevention and treatment of type 2 diabetes. Front Endocrinol. (2017) 8:97. doi: 10.3389/fendo.2017.00097
21. Sasaki K, Takahashi N, Satoh M, Yamasaki M, Minamino N. A peptidomics strategy for discovering endogenous bioactive peptides. J Proteome Res. (2010) 9:5047–52. doi: 10.1021/pr1003455
22. Roberts GP, Larraufie P, Richards P, Kay RG, Galvin SG, Miedzybrodzka EL, et al. Comparison of human and murine enteroendocrine cells by transcriptomic and peptidomic profiling. Diabetes. (2019) 68:1062–72. doi: 10.2337/db18-0883
23. Liu YC, Patel HJ, Khawaja AM, Belvisi MG, Rogers DF. Neuroregulation by vasoactive intestinal peptide (VIP) of mucus secretion in ferret trachea: activation of BK(Ca) channels and inhibition of neurotransmitter release. Br J Pharmacol. (1999) 126:147–58. doi: 10.1038/sj.bjp.0702288
24. Wang X, Xu S, Chen L, Shen D, Cao Y, Tang R, et al. Profiling analysis reveals the potential contribution of peptides to human adipocyte differentiation. Proteomics Clin Appl. (2018) 12:e1700172. doi: 10.1002/prca.201700172
25. Bartolomucci A, La Corte G, Possenti R, Locatelli V, Rigamonti AE, Torsello A, et al. TLQP-21, a VGF-derived peptide, increases energy expenditure and prevents the early phase of diet-induced obesity. Proc Natl Acad Sci USA. (2006) 103:14584–9. doi: 10.1073/pnas.0606102103
26. Zois NE, Bartels ED, Hunter I, Kousholt BS, Olsen LH, Goetze JP. Natriuretic peptides in cardiometabolic regulation and disease. Nat Rev Cardiol. (2014) 11:403–12. doi: 10.1038/nrcardio.2014.64
27. DaSilva JO, Amorino GP, Casarez EV, Pemberton B, Parsons SJ. Neuroendocrine-derived peptides promote prostate cancer cell survival through activation of IGF-1R signaling. Prostate. (2013) 73:801–12. doi: 10.1002/pros.22624
28. Schrader M. Origins, technological development, and applications of peptidomics. Methods Mol Biol. (2018) 1719:3–39. doi: 10.1007/978-1-4939-7537-2_1
29. Štěpánová S, Kašička V. Recent developments and applications of capillary and microchip electrophoresis in proteomics and peptidomics (2015-mid 2018). J Sep Sci. (2019) 42:398–414. doi: 10.1002/jssc.201801090
30. Fricker L. Quantitative peptidomics: general considerations. Methods Mol Biol. (2018) 1719:121–40. doi: 10.1007/978-1-4939-7537-2_8
31. Klein J, Ramirez-Torres A, Ericsson A, Huang Y, Breuil B, Siwy J, et al. Urinary peptidomics provides a noninvasive humanized readout of diabetic nephropathy in mice. Kidney Int. (2016) 90:1045–55. doi: 10.1016/j.kint.2016.06.023
32. Krochmal M, Schanstra JP, Mischak H. Urinary peptidomics in kidney disease and drug research. Expert Opin Drug Discov. (2018) 13:259–68. doi: 10.1080/17460441.2018.1418320
33. Wang S, Blois A, El Rayes T, Liu JF, Hirsch MS, Gravdal K, et al. Development of a prosaposin-derived therapeutic cyclic peptide that targets ovarian cancer via the tumor microenvironment. Sci Transl Med. (2016) 8:329r−34r. doi: 10.1126/scitranslmed.aad5653
34. Taylor SW, Nikoulina SE, Andon NL, Lowe C. Peptidomic profiling of secreted products from pancreatic islet culture results in a higher yield of full-length peptide hormones than found using cell lysis procedures. J Proteome Res. (2013) 12:3610–9. doi: 10.1021/pr400115q
35. Ye H, Wang J, Tian Z, Ma F, Dowell J, Bremer Q, et al. Quantitative mass spectrometry reveals food intake-induced neuropeptide level changes in rat brain: functional assessment of selected neuropeptides as feeding regulators. Mol Cell Proteomics. (2017) 16:1922–37. doi: 10.1074/mcp.RA117.000057
36. Zhu L, Hou M, Sun B, Burén J, Zhang L, Yi J, et al. Testosterone stimulates adipose tissue 11beta-hydroxysteroid dehydrogenase type 1 expression in a depot-specific manner in children. J Clin Endocrinol Metab. (2010) 95:3300–8. doi: 10.1210/jc.2009-2708
37. Jia G, Tao H, Xue Y, Xu S, Xue K, Zhu Q, et al. Analysis of secreted peptidome from omental adipose tissue in polycystic ovarian syndrome patients. J Cell Physiol. (2018) 233:5885–94. doi: 10.1002/jcp.26393
38. Alvarez-Llamas G, Szalowska E, de Vries MP, Weening D, Landman K, Hoek A, et al. Characterization of the human visceral adipose tissue secretome. Mol Cell Proteomics. (2007) 6:589–600. doi: 10.1074/mcp.M600265-MCP200
39. Roca-Rivada A, Alonso J, Al-Massadi O, Castelao C, Peinado JR, Seoane LM, et al. Secretome analysis of rat adipose tissues shows location-specific roles for each depot type. J Proteomics. (2011) 74:1068–79. doi: 10.1016/j.jprot.2011.03.010
40. Casabiell X, Pineiro V, Peino R, Lage M, Camiña J, Gallego R, et al. Gender differences in both spontaneous and stimulated leptin secretion by human omental adipose tissue in vitro: dexamethasone and estradiol stimulate leptin release in women, but not in men. J Clin Endocrinol Metab. (1998) 83:2149–55. doi: 10.1210/jcem.83.6.4849
41. Phillips SA, Ciaraldi TP, Oh DK, Savu MK, Henry RR. Adiponectin secretion and response to pioglitazone is depot dependent in cultured human adipose tissue. Am J Physiol Endocrinol Metab. (2008) 295:E842–50. doi: 10.1152/ajpendo.90359.2008
42. Roca-Rivada A, Al-Massadi O, Castelao C, Senín LL, Alonso J, Seoane LM, et al. Muscle tissue as an endocrine organ: comparative secretome profiling of slow-oxidative and fast-glycolytic rat muscle explants and its variation with exercise. J Proteomics. (2012) 75:5414–25. doi: 10.1016/j.jprot.2012.06.037
43. Mæhre HK, Dalheim L, Edvinsen GK, Elvevoll EO, Jensen IJ. Protein determination-method matters. Foods. (2018) 7:5. doi: 10.3390/foods7010005
44. Robinson MM, Dasari S, Karakelides H, Bergen HR, Nair KS. Release of skeletal muscle peptide fragments identifies individual proteins degraded during insulin deprivation in type 1 diabetic humans and mice. Am J Physiol Endocrinol Metab. (2016) 311:E628–37. doi: 10.1152/ajpendo.00175.2016
45. Zhang X, Liu Q, Zhou W, Li P, Alolga RN, Qi LW, et al. A comparative proteomic characterization and nutritional assessment of naturally- and artificially-cultivated Cordyceps sinensis. J Proteomics. (2018) 181:24–35. doi: 10.1016/j.jprot.2018.03.029
46. Yin P, Knolhoff AM, Rosenberg HJ, Millet LJ, Gillette MU, Sweedler JV. Peptidomic analyses of mouse astrocytic cell lines and rat primary cultured astrocytes. J Proteome Res. (2012) 11:3965–73. doi: 10.1021/pr201066t
47. Shilov IV, Seymour SL, Patel AA, Loboda A, Tang WH, Keating SP, et al. The Paragon Algorithm, a next generation search engine that uses sequence temperature values and feature probabilities to identify peptides from tandem mass spectra. Mol Cell Proteomics. (2007) 6:1638–55. doi: 10.1074/mcp.T600050-MCP200
48. Schilling B, Rardin MJ, MacLean BX, Zawadzka AM, Frewen BE, Cusack MP, et al. Platform-independent and label-free quantitation of proteomic data using MS1 extracted ion chromatograms in skyline: application to protein acetylation and phosphorylation. Mol Cell Proteomics. (2012) 11:202–14. doi: 10.1074/mcp.M112.017707
49. Rardin MJ, Newman JC, Held JM, Cusack MP, Sorensen DJ, Li B, et al. Label-free quantitative proteomics of the lysine acetylome in mitochondria identifies substrates of SIRT3 in metabolic pathways. Proc Natl Acad Sci USA. (2013) 110:6601–6. doi: 10.1073/pnas.1302961110
50. Ashburner M, Ball CA, Blake JA, Botstein D, Butler H, Cherry JM, et al. Gene ontology: tool for the unification of biology. The Gene Ontology Consortium. Nat Genet. (2000) 25:25–9. doi: 10.1038/75556
51. Shen D, Li Y, Wang X, Wang F, Huang F, Cao Y, et al. A novel peptide suppresses adipogenic differentiation through activation of the AMPK pathway. Biochem Biophys Res Commun. (2019) 510:395–402. doi: 10.1016/j.bbrc.2019.01.112
52. Villanueva J, Philip J, Chaparro CA, Li Y, Toledo-Crow R, DeNoyer L, et al. Correcting common errors in identifying cancer-specific serum peptide signatures. J Proteome Res. (2005) 4:1060–72. doi: 10.1021/pr050034b
53. Koomen JM, Li D, Xiao LC, Liu TC, Coombes KR, Abbruzzese J, et al. Direct tandem mass spectrometry reveals limitations in protein profiling experiments for plasma biomarker discovery. J Proteome Res. (2005) 4:972–81. doi: 10.1021/pr050046x
54. Villanueva J, Shaffer DR, Philip J, Chaparro CA, Erdjument-Bromage H, Olshen AB, et al. Differential exoprotease activities confer tumor-specific serum peptidome patterns. J Clin Invest. (2006) 116:271–84. doi: 10.1172/JCI26022
55. Wang F, Zhu J, Hu L, Qin H, Ye M, Zou H. Comprehensive analysis of the N and C terminus of endogenous serum peptides reveals a highly conserved cleavage site pattern derived from proteolytic enzymes. Protein Cell. (2012) 3:669–74. doi: 10.1007/s13238-012-2934-4
56. Zhang J, Liang D, Cheng Q, Cao L, Wu Y, Wang Y, et al. Peptidomic analysis of fetal heart tissue for identification of endogenous peptides involved in tetralogy of fallot. DNA Cell Biol. (2017) 36:451–61. doi: 10.1089/dna.2017.3647
57. Deshmukh AS. Proteomics of skeletal muscle: focus on insulin resistance and exercise biology. Proteomes. (2016) 4:6. doi: 10.3390/proteomes4010006
58. Yoon JH, Yea K, Kim J, Choi YS, Park S, Lee H, et al. Comparative proteomic analysis of the insulin-induced L6 myotube secretome. Proteomics. (2009) 9:51–60. doi: 10.1002/pmic.200800187
59. Norheim F, Raastad T, Thiede B, Rustan AC, Drevon CA, Haugen F. Proteomic identification of secreted proteins from human skeletal muscle cells and expression in response to strength training. Am J Physiol Endocrinol Metab. (2011) 301:E1013–21. doi: 10.1152/ajpendo.00326.2011
60. Raschke S, Eckardt K, Bjørklund Holven K, Jensen J, Eckel J. Identification and validation of novel contraction-regulated myokines released from primary human skeletal muscle cells. PLoS ONE. (2013) 8:e62008. doi: 10.1371/journal.pone.0062008
61. Deshmukh AS, Cox J, Jensen LJ, Meissner F, Mann M. Secretome analysis of lipid-induced insulin resistance in skeletal muscle cells by a combined experimental and bioinformatics workflow. J Proteome Res. (2015) 14:4885–95. doi: 10.1021/acs.jproteome.5b00720
62. Yoon JH, Kim D, Jang JH, Ghim J, Park S, Song P, et al. Proteomic analysis of the palmitate-induced myotube secretome reveals involvement of the annexin A1-formyl peptide receptor 2 (FPR2) pathway in insulin resistance. Mol Cell Proteomics. (2015) 14:882–92. doi: 10.1074/mcp.M114.039651
63. Furuichi Y, Manabe Y, Takagi M, Aoki M, Fujii NL. Evidence for acute contraction-induced myokine secretion by C2C12 myotubes. PLoS ONE. (2018) 13:e206146. doi: 10.1371/journal.pone.0206146
64. Forterre A, Jalabert A, Chikh K, Pesenti S, Euthine V, Granjon A, et al. Myotube-derived exosomal miRNAs downregulate Sirtuin1 in myoblasts during muscle cell differentiation. Cell Cycle. (2014) 13:78–89. doi: 10.4161/cc.26808
65. Jalabert A, Vial G, Guay C, Wiklander OP, Nordin JZ, Aswad H, et al. Exosome-like vesicles released from lipid-induced insulin-resistant muscles modulate gene expression and proliferation of beta recipient cells in mice. Diabetologia. (2016) 59:1049–58. doi: 10.1007/s00125-016-3882-y
66. Ibrahim A, Neinast M, Arany ZP. Myobolites: muscle-derived metabolites with paracrine and systemic effects. Curr Opin Pharmacol. (2017) 34:15–20. doi: 10.1016/j.coph.2017.03.007
67. Nielsen S, Scheele C, Yfanti C, Akerström T, Nielsen AR, Pedersen BK, et al. Muscle specific microRNAs are regulated by endurance exercise in human skeletal muscle. J Physiol. (2010) 588:4029–37. doi: 10.1113/jphysiol.2010.189860
68. Coleman DL. Obese and diabetes: two mutant genes causing diabetes-obesity syndromes in mice. Diabetologia. (1978) 14:141–8. doi: 10.1007/BF00429772
69. Rees DA, Alcolado JC. Animal models of diabetes mellitus. Diabet Med. (2005) 22:359–70. doi: 10.1111/j.1464-5491.2005.01499.x
70. Gao Y, Wang X, Huang F, Cui X, Li Y, Wang X, et al. Identification and characterization of metformin on peptidomic profiling in human visceral adipocytes. J Cell Biochem. (2018) 119:1866–78. doi: 10.1002/jcb.26347
71. Sasaki K, Satomi Y, Takao T, Minamino N. Snapshot peptidomics of the regulated secretory pathway. Mol Cell Proteomics. (2009) 8:1638–47. doi: 10.1074/mcp.M900044-MCP200
72. Gelman JS, Dasgupta S, Berezniuk I, Fricker LD. Analysis of peptides secreted from cultured mouse brain tissue. Biochim Biophys Acta. (2013) 1834:2408–17. doi: 10.1016/j.bbapap.2013.01.043
73. Fricker LD. Analysis of mouse brain peptides using mass spectrometry-based peptidomics: implications for novel functions ranging from non-classical neuropeptides to microproteins. Mol Biosyst. (2010) 6:1355–65. doi: 10.1039/c003317k
74. Silvestre RA, Rodríguez-Gallardo J, Egido EM, Hernández R, Marco J. Stimulatory effect of xenin-8 on insulin and glucagon secretion in the perfused rat pancreas. Regul Pept. (2003) 115:25–9. doi: 10.1016/S0167-0115(03)00147-2
75. Martin CM, Parthsarathy V, Pathak V, Gault VA, Flatt PR, Irwin N. Characterisation of the biological activity of xenin-25 degradation fragment peptides. J Endocrinol. (2014) 221:193–200. doi: 10.1530/JOE-13-0617
76. Wiita AP, Hsu GW, Lu CM, Esensten JH, Wells JA. Circulating proteolytic signatures of chemotherapy-induced cell death in humans discovered by N-terminal labeling. Proc Natl Acad Sci USA. (2014) 111:7594–9. doi: 10.1073/pnas.1405987111
77. Aletti F, Maffioli E, Negri A, Santamaria MH, DeLano FA, Kistler EB, et al. Peptidomic analysis of rat plasma: proteolysis in hemorrhagic shock. Shock. (2016) 45:540–54. doi: 10.1097/SHK.0000000000000532
78. Hittel DS, Hathout Y, Hoffman EP, Houmard JA. Proteome analysis of skeletal muscle from obese and morbidly obese women. Diabetes. (2005) 54:1283–8. doi: 10.2337/diabetes.54.5.1283
79. Giebelstein J, Poschmann G, Højlund K, Schechinger W, Dietrich JW, Levin K, et al. The proteomic signature of insulin-resistant human skeletal muscle reveals increased glycolytic and decreased mitochondrial enzymes. Diabetologia. (2012) 55:1114–27. doi: 10.1007/s00125-012-2456-x
80. Mogensen M, Sahlin K, Fernström M, Glintborg D, Vind BF, Beck-Nielsen H, et al. Mitochondrial respiration is decreased in skeletal muscle of patients with type 2 diabetes. Diabetes. (2007) 56:1592–9. doi: 10.2337/db06-0981
81. Cheng Z, Tseng Y, White MF. Insulin signaling meets mitochondria in metabolism. Trends Endocrinol Metab. (2010) 21:589–98. doi: 10.1016/j.tem.2010.06.005
82. Ritov VB, Menshikova EV, Azuma K, Wood R, Toledo FG, Goodpaster BH, et al. Deficiency of electron transport chain in human skeletal muscle mitochondria in type 2 diabetes mellitus and obesity. Am J Physiol Endocrinol Metab. (2010) 298:E49–58. doi: 10.1152/ajpendo.00317.2009
83. Thingholm TE, Bak S, Beck-Nielsen H, Jensen ON, Gaster M. Characterization of human myotubes from type 2 diabetic and nondiabetic subjects using complementary quantitative mass spectrometric methods. Mol Cell Proteomics. (2011) 10:M110.006650. doi: 10.1074/mcp.M110.006650
84. Oberbach A, Bossenz Y, Lehmann S, Niebauer J, Adams V, Paschke R, et al. Altered fiber distribution and fiber-specific glycolytic and oxidative enzyme activity in skeletal muscle of patients with type 2 diabetes. Diabetes Care. (2006) 29:895–900. doi: 10.2337/diacare.29.04.06.dc05-1854
85. Denessiouk K, Permyakov S, Denesyuk A, Permyakov E, Johnson MS. Two structural motifs within canonical EF-hand calcium-binding domains identify five different classes of calcium buffers and sensors. PLoS ONE. (2014) 9:e109287. doi: 10.1371/journal.pone.0109287
86. Lakowski TM, Lee GM, Lelj-Garolla B, Okon M, Reid RE, McIntosh LP. Peptide binding by a fragment of calmodulin composed of EF-hands 2 and 3. Biochemistry-us. (2007) 46:8525–36. doi: 10.1021/bi700265j
87. Kawasaki H, Kretsinger RH. Calcium-binding proteins 1: EF-hands. Protein Profile. (1995) 2:297–490.
88. Wright DC, Hucker KA, Holloszy JO, Han DH. Ca2+ and AMPK both mediate stimulation of glucose transport by muscle contractions. Diabetes. (2004) 53:330–5. doi: 10.2337/diabetes.53.2.330
89. Park DR, Park KH, Kim BJ, Yoon CS, Kim UH. Exercise ameliorates insulin resistance via Ca2+ signals distinct from those of insulin for GLUT4 translocation in skeletal muscles. Diabetes. (2015) 64:1224–34. doi: 10.2337/db14-0939
90. Jia J, Arif A, Terenzi F, Willard B, Plow EF, Hazen SL, et al. Target-selective protein S-nitrosylation by sequence motif recognition. Cell. (2014) 159:623–34. doi: 10.1016/j.cell.2014.09.032
91. Guo S. Insulin signaling, resistance, and the metabolic syndrome: insights from mouse models into disease mechanisms. J Endocrinol. (2014) 220:T1–23. doi: 10.1530/JOE-13-0327
92. Wang ZQ, Zhang XH, Yu Y, Poulev A, Ribnicky D, Floyd ZE, et al. Bioactives from bitter melon enhance insulin signaling and modulate acyl carnitine content in skeletal muscle in high-fat diet-fed mice. J Nutr Biochem. (2011) 22:1064–73. doi: 10.1016/j.jnutbio.2010.09.004
Keywords: mass spectrometry, secreted peptide, skeletal muscle, insulin resistance, Irs1-Akt signaling pathway, Pgc1α
Citation: Wu Y, Han M, Wang Y, Gao Y, Cui X, Xu P, Ji C, Zhong T, You L and Zeng Y (2019) A Comparative Peptidomic Characterization of Cultured Skeletal Muscle Tissues Derived From db/db Mice. Front. Endocrinol. 10:741. doi: 10.3389/fendo.2019.00741
Received: 22 July 2019; Accepted: 14 October 2019;
Published: 29 October 2019.
Edited by:
Honoo Satake, Suntory Foundation for Life Sciences, JapanReviewed by:
Vaclav Kasicka, Institute of Organic Chemistry and Biochemistry (ASCR), CzechiaMartin Hubalek, Institute of Organic Chemistry and Biochemistry (ASCR), Czechia
Copyright © 2019 Wu, Han, Wang, Gao, Cui, Xu, Ji, Zhong, You and Zeng. This is an open-access article distributed under the terms of the Creative Commons Attribution License (CC BY). The use, distribution or reproduction in other forums is permitted, provided the original author(s) and the copyright owner(s) are credited and that the original publication in this journal is cited, in accordance with accepted academic practice. No use, distribution or reproduction is permitted which does not comply with these terms.
*Correspondence: Lianghui You, eV9saWFuZ2h1aUAxMjYuY29t; Yu Zeng, emVuZ3l1QG5qbXUuZWR1LmNu
†These authors have contributed equally to this work as joint first authors