- 1Department of Endocrinology and Metabolism, Renji Hospital, School of Medicine, Shanghai Jiao Tong University, Shanghai, China
- 2Department of Pharmacy, Renji Hospital, School of Medicine, Shanghai Jiao Tong University, Shanghai, China
Background: The gut microbiota is recognized as a major modulator of metabolic disorders such as type 2 diabetes. Dapagliflozin, sodium glucose cotransporter 2 inhibitors (SGLT2i), enhances renal glucose excretion, and lowers blood glucose levels. The study aimed to determine the effects of dapagliflozin on fecal microbiota in a type 2 diabetic rat model.
Methods: Four-week-old male Sprague Dawley rats (n = 24) were fed a high-fat diet (HFD) for 8 weeks and then given a single dose of STZ injection (30 mg/kg, i.p). They were randomly divided into three groups (n = 8). Each group received intragastric infusion of normal saline (2 ml, 0.9%) or metformin (215.15 mg/kg/day) or dapagliflozin (1 mg/kg/day) for 4 weeks. Blood glucose levels and plasma insulin levels were detected during intragastric glucose tolerance. Fecal samples were collected to access microbiome by 16S ribosomal RNA gene sequencing.
Results: Dapagliflozin significantly decreased fasting and postprandial blood glucose levels as metformin in type 2 diabetic rats (P < 0.001). Enterotype was composed of Ruminococcaceae after treatment of dapagliflozin, whereas Ruminococcaceae and Muribaculaceae were the main enterotypes following metformin treatment. Dapagliflozin did not increase the abundance of beneficial bacteria including Lactobacillaceae and Bifidobacteriaceae. However, these were increased in the metformin group. It is surprising to find that Proteobacteria (especially Desulfovibrionaceae) were enriched in the dapagliflozin group.
Conclusion: Dapagliflozin and metformin exerted complementary effects on the main beneficial bacteria. A combination of these two drugs might be beneficial to improve the structure of fecal microbiota in the treatment of type 2 diabetes.
Introduction
Type 2 diabetes (T2D) affects nearly 373.5 million individuals worldwide. An increasing number of studies have connected the gut microbiome with metabolic diseases such as diabetes and obesity (1). It has been reported that there was a decreased amount of beneficial bacteria in patients with type 2 diabetes (2). Fecal microbiota transplantation holds potential as a promising option in the treatment of diabetes (3).
The composition and richness of intestinal flora are unique and highly variable. It can be modulated by diet, host health, age, ethnicity, genetics, and antidiabetic medication (4). Metformin is a first-line medication in the treatment of type 2 diabetes, as it increases the abundance of beneficial bacteria (4, 5), and is associated with higher levels of short-chain fatty acids (SCFAs: acetate, propionate, and butyrate) (6). SCFAs conferred beneficial metabolic effects, including improving body weight and glycemic management (7). Treatment of metformin could restore sodium glucose cotransporter 1 (SGLT1) expression and glucose sensing to alter the upper small intestinal microbiota (8).
Sodium glucose cotransporter 2 (SGLT2) inhibitors are used to achieve the glucose-lowering effect by increasing urinary glucose excretion (9). SGLT2 is mainly expressed in the proximal tubule of the kidney, while SGLT1 is highly expressed in the gut (9). SGLT2 inhibitors could act on SGLT1 to reduce intestinal glucose uptake (10). SGLT2 inhibitors decreased the glucose excursion and increased glucagon-like peptide-1 (GLP-1) and peptide YY secretion following carbohydrates (CHO) ingestion (11). The latter may augment glucose exposure of the colonic microbiota and formation of metabolites (11). It has been shown that canagliflozin altered the intestinal microbiota composition in mice with chronic kidney disease (12). The effects of dapagliflozin on fecal microbiota remain controversial. A recent study demonstrated that dapagliflozin altered the fecal microbiota in diabetic mice (13). However, another human study reported that dapagliflozin treatment had no effects on fecal microbiota. It should be noted that it was a combinative effect of metformin and dapagliflozin. Furthermore, there was no placebo in the study (14). Therefore, we aimed to explore the effects of dapagliflozin on fecal microbiota in a type 2 diabetic rat model.
Methods
Animals and Ethics Statement
Four-week-old male Sprague Dawley (SD) rats weighing 200–250 g were purchased from Shanghai SLAC Laboratory Animal (Shanghai, China, SCXK2017-0005, SYXK2017-0008). All the SD rats were housed in a controlled environment with a temperature of (20–25°C) and humidity of (40–60%) and allowed free access to water and food. Animal handling and experimental procedures were consistent with the regulations of the Shanghai Government and the Principles of Laboratory Animal Care, published by the US National Institutes of Health (publication no.85-23, revised 1996).
The detailed animal ethics including the animal tests and its handing procedures were approved by the institutional animal care and use committee of SLAC.
Experimental Protocol
Rats were fed with a high-fat diet for 8 weeks, followed by a single dose of injection of STZ (30 mg/kg, ip). The high-fat diet (HFD) contained 20% protein, 20% carbohydrate, and 60% fat. The blood glucose was detected about 3–7 days after STZ administration. Rats were considered the model of type 2 diabetes mellitus when they met the following criteria (the highest random blood glucose concentrations were higher than 16.7 mmol/L, and the 2 h postprandial glucose concentrations were higher than 11.1 mmol/L). They were randomly divided into three groups (n = 8): control, metformin, and dapagliflozin. All the rats received daily gastric gavage with 2 ml saline (0.9%), or metformin (215.15 mg/kg/day, Sino-American Shanghai Squibb Pharmaceuticals, H20023370) or dapagliflozin (1 mg/kg/day, AstraZeneca, H20170117) for 4 weeks. The formula used for the dosage of dapagliflozin and metformin was: drat=dhuman*0.71/0.11 (15).
Intragastric Glucose Tolerance Test (IGGTT)
After 4 weeks of dosage, overnight-fasted rats were given 50% glucose at a dose of 2 g/kg by intragastric administration. Blood samples were collected from the tip of the rats' tails to test glucose levels at 0, 30, 60, 120, and 180 min by using a glucose meter (Accu-Chek, Roche, Swiss). The samples were centrifuged for 10 min at 3,000 rpm (4°C) and plasma was used for insulin measurement.
DNA Extraction and 16S rRNA Gene Sequencing
We performed 16S rRNA sequencing including fecal sample collection, DNA extraction, and PCR amplification; Illumina MiSeq sequencing and processing of data (16). Bacterial genomic DNA was performed by using the EZNA soil DNA Kit (Omega Bio-tek, Norcross, GA, USA). The 16S rRNA V3-V4 region was amplified via PCR and then sequenced by the Illumina MiSeq platform (Illumina, San Diego, USA).
Bioinformatic Analysis and 16S rRNA Gene Sequencing
We used bioinformatic analysis and 16S rRNA sequencing mainly including the clustering of microbial community types and metabolic function prediction (16). We analyzed microbial community types by investigating operational taxonomic units (OTU) level microbial alpha (Sobs, Shannon, Ace, and Chao1) and beta diversity, principal coordinates analysis (PCoA), and analysis metabolic function prediction by using Phylogenetic Investigation of Communities by Reconstruction of Unobserved States (PICRUSt).
Statistical Analysis
SPSS Statistics V 23.0 software (IBM, Chicago, IL, USA) and the online platform Majorbio I-Sanger Cloud Platform were used to analyze the results. Blood glucose was calculated by repeated measures analysis of variance (ANOVA) and expressed as the mean ± SEM. The area under the curve (AUC) was calculated by the trapezoidal method (17). HOMA-IR was calculated by the following formula: HOMA-IR= Fasting blood glucose (FBG, mmol/L) × Fasting insulin (FINS, ng/ml)/22.5.
Indexes of alpha diversity (Sobs, Shannon, Ace, and Chao1) were analyzed by the Kruskal-Wallis test. Beta diversity was calculated by the pMANOVA analysis based on Bray Curtis distances. Kruskal-Wallis sum rank test was used to detect the differences of the relative abundance of bacteria between the groups, including the LEfSe algorithm. Only functional categories reaching a log LDA significant threshold value of >2.0 were shown. Spearman's correlations were calculated between the abundance of bacterial families and blood glucose levels during IGGTT. P < 0.05 was considered statistically significant.
Results
Blood Glucose Levels During IGGTT
Fasting blood glucose levels were significantly decreased after metformin and dapagliflozin treatments compared with the control (P < 0.001, respectively), with no statistical differences between metformin and dapagliflozin treatments. Postload blood glucose levels in the metformin and dapagliflozin group were markedly decreased at 30, 60, 120, and 180 min (Supplementary Figures 1A,B) compared to the control. There were no significant differences in postload blood glucose levels between metformin and dapagliflozin. Dapagliflozin decreased the levels of HOMA-IR when compared with metformin and the control (Supplementary Figure 1C). There was no difference between metformin treatment and the placebo.
Fecal Microbiota Diversity
The shannon index of dapagliflozin treatment was higher than that of metformin (P = 0.039) (Figure 1B). No statistical difference was found in the indexes indicating richness, including Sobs, Ace, and Chao1 (Figures 1A,C,D). The pMANOVA analysis, which was based on Bray Curtis distances, revealed significant alterations of the microbiota communities between metformin, dapagliflozin, and control rats (P = 0.001) (Figure 2A).
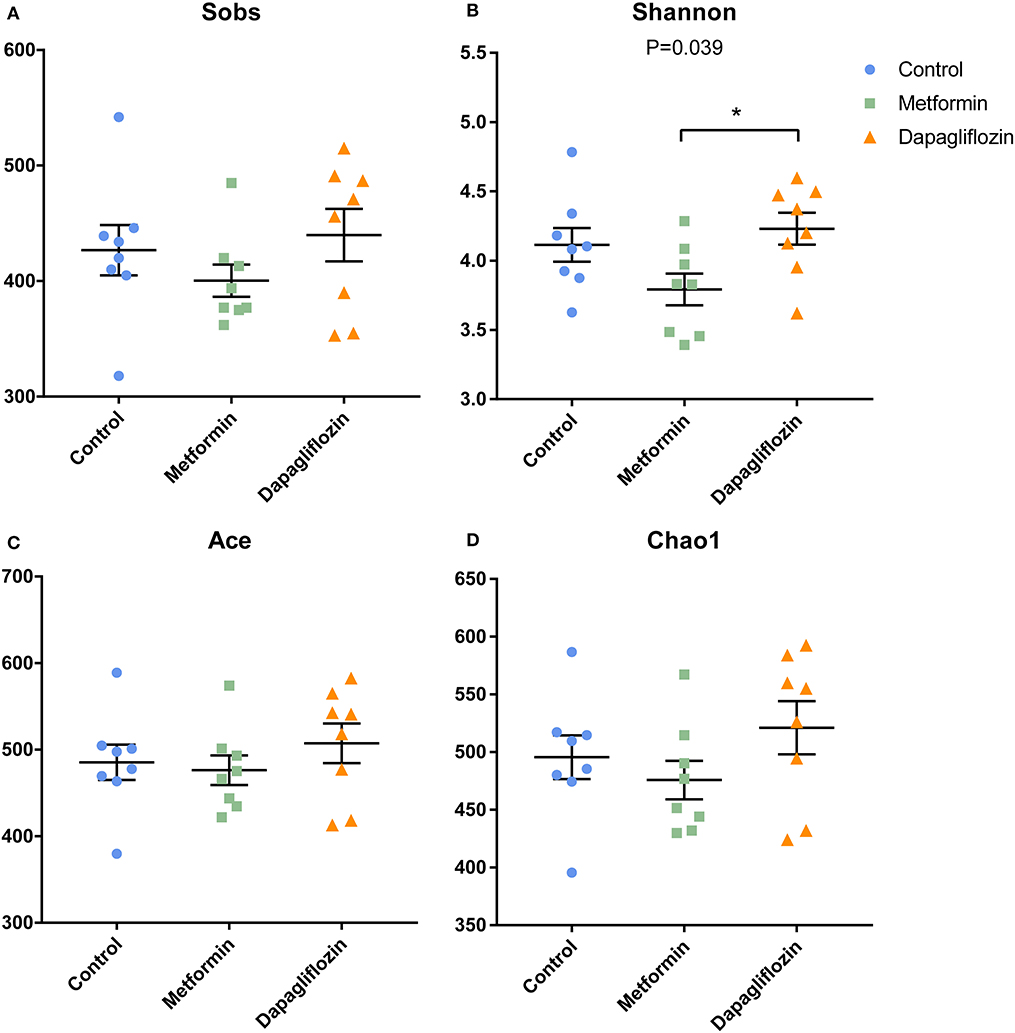
Figure 1. Indexes of alpha diversity in three groups. (A) Sobs (B) Shannon (C) Ace (D) Chao1. Data were expressed as mean ± SEM. *P < 0.05.
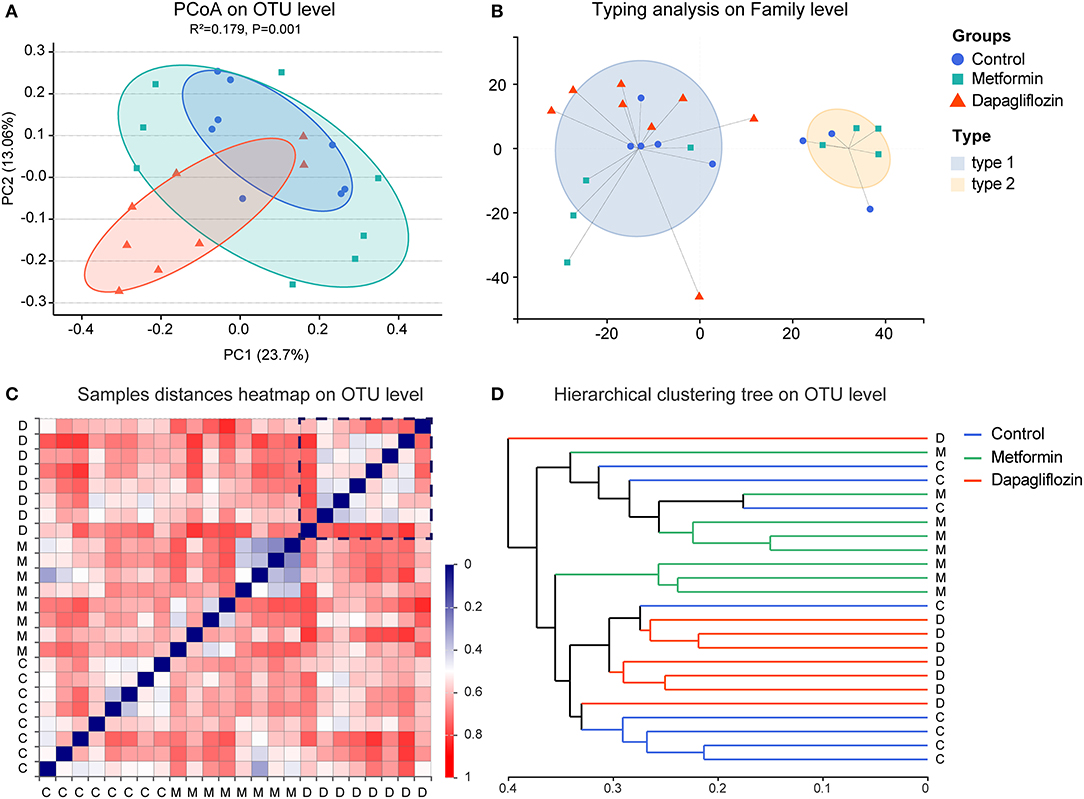
Figure 2. Beta diversity and enterotypes analysis in three groups. (A) Principal coordinates analysis (PCoA). The two principal coordinates (PC1-PC2) explain 23.7 and 13.06%, respectively, and the analysis of permutational multivariate analysis of variance (pANOVA) was p = 0.001. (B) Clustering of all 24 samples into two enterotypes on the family level. Type1 was presented by Ruminococcaceae and type 2 was Muribaculaceae. (C) Samples distance heatmap on operational taxonomic units (OTU) level. Clustering of samples based on Bray-Curtis distances. Each row or column represents one sample. (D) Hierarchical clustering tree on OTU level. One straight line represents on sample. C, control group; M, metformin group; D, dapagliflozin group.
The composition of fecal microbiota was distinguished into two enterotypes (Figure 2B). Ruminococcaceae was the dominant enterotype in the dapagliflozin group, while the metformin group displayed enterotypes mainly composed by Ruminococcaceae and Muribaculaceae. The clustering analysis showed that there was more consistency in the dapagliflozin group (Figures 2C,D).
The Composition of Fecal Microbiota
The six major phyla dominated in microbiota were Firmicutes, Bacteroidetes, Proteobacteria, Actinobacteria, Verrucomicrobia, and Spirochates (Figures 3A,B). The abundance of Firmicutes and Bacteroidetes did not change significantly among the three groups (Figure 3B) and the ratio of Firmicutes to Bacteroidetes also exhibited no significant differences (Figure 3C). Type 2 diabetic rats treated with dapagliflozin showed a higher abundance of Proteobacteria (P < 0.05, Figure 3B), mainly Proteobacteria (Delta) but not Proteobacteria (Gramma and Alpha) (Figure 3E). The ratio of Bacteroidetes/Proteobacteria was decreased in the dapagliflozin group as compared with metformin (Figure 3D). Meanwhile, dapagliflozin lowered the abundance of Actinobacteria and Spriochaetes compared with metformin treatment (Figure 3B).
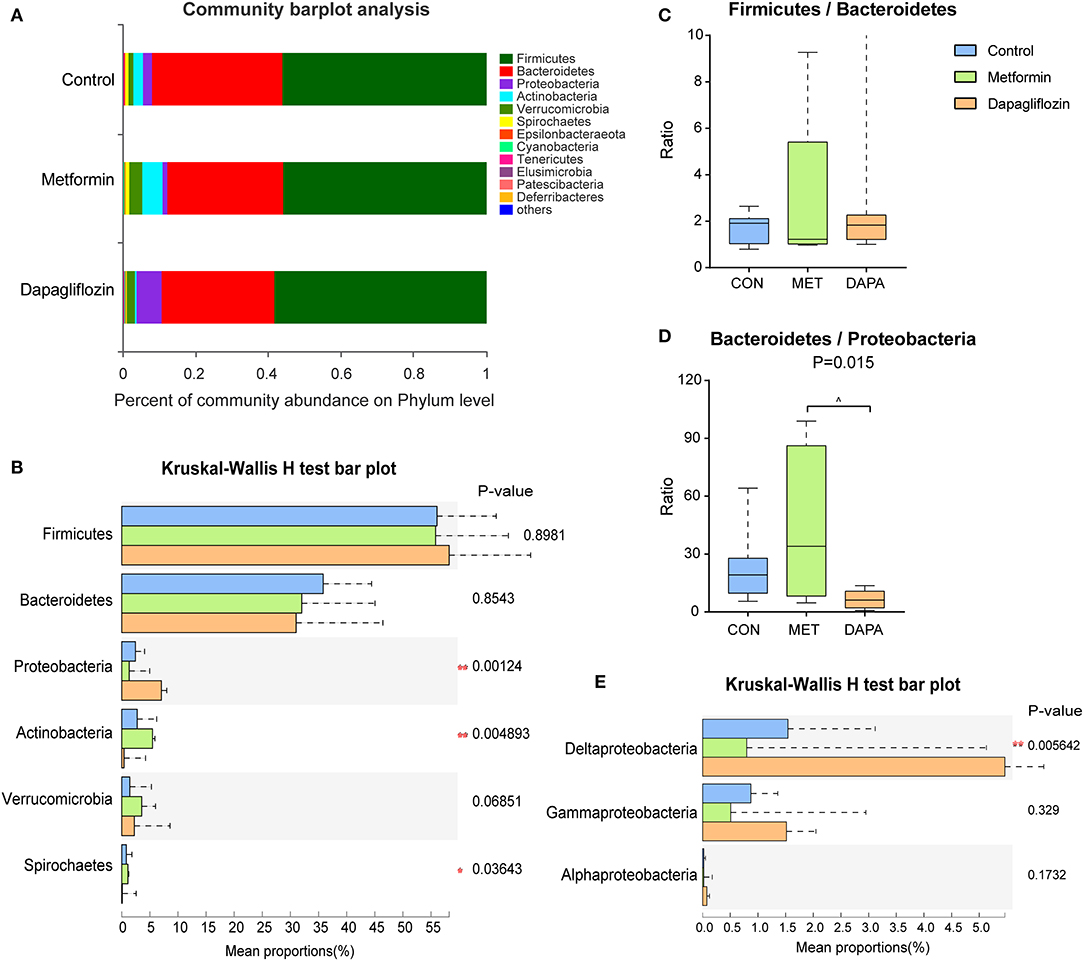
Figure 3. Characterization of core microbial communities. (A) Community barplot analysis showing the relative abundance on the phylum level. (B) Firmicutes to Bacteroidetes ratio. (C) Relative abundance of the domain phyla Firmicutes, Bacteroidetes, Proteobacteria, Actinobacteria, Verrucomicrobia, and Spirochaetes. (D) Ratio of Bacteroidetes to Proteobacteria. Kruskal-Wallis rank-sum test was used to assess the ratio of Firmicutes to Bacteroidetes and the ratio of Bacteroidetes to Proteobacteria. (E) Relative abundance of Proteobacteria (delta, gamma, and alpha). *P < 0.05, **P ≤ 0.01, ^metformin versus dapagliflozin P < 0.05.
Overall, community diversity was different among the three groups on the family level (Figure 4, Supplementary Figure 2). The analysis of community relative abundance demonstrated that Lactobacillaceae was increased in the metformin group, but decreased in the dapagliflozin group (Figure 4C). Metformin also increased the relative abundance of Bifidobacteriaceae and decreased that of Veillonellaceae compared with dapagliflozin. Desulfovibrionaceae were increased in the dapagliflozin group as compared with the metformin and control group (Figure 4C). Spearman's correlations between the relative abundance of bacterial families and blood glucose were performed. A negative correlation between Desulfovibrionaceae and blood glucose was indicated, especially in the fasting blood glucose and 30 min postload (P < 0.01) (Figure 5).
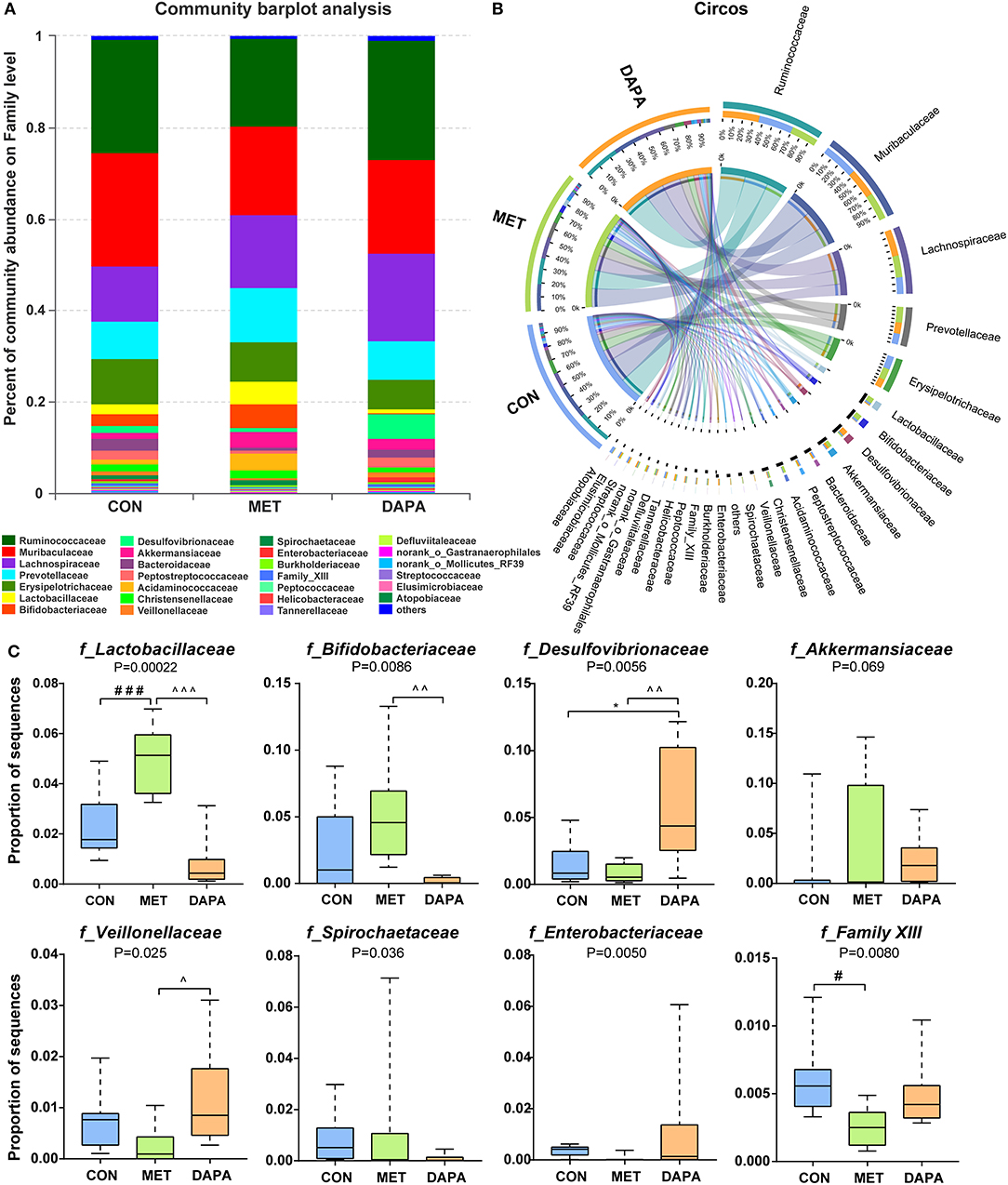
Figure 4. Baterial community abundance on the family level of each group. (A) Community barplot demonstrating the relative abundance on the family level. (B) Circos plot displaying the relationship between samples and bacteria. (C) Relative abundance of core microbial families. * control versus dapagliflozin; # control versus metformin; ^metformin versus dapagliflozin. One special character meant P < 0.05, two meant P ≤ 0.01 and three meant P ≤ 0.001.
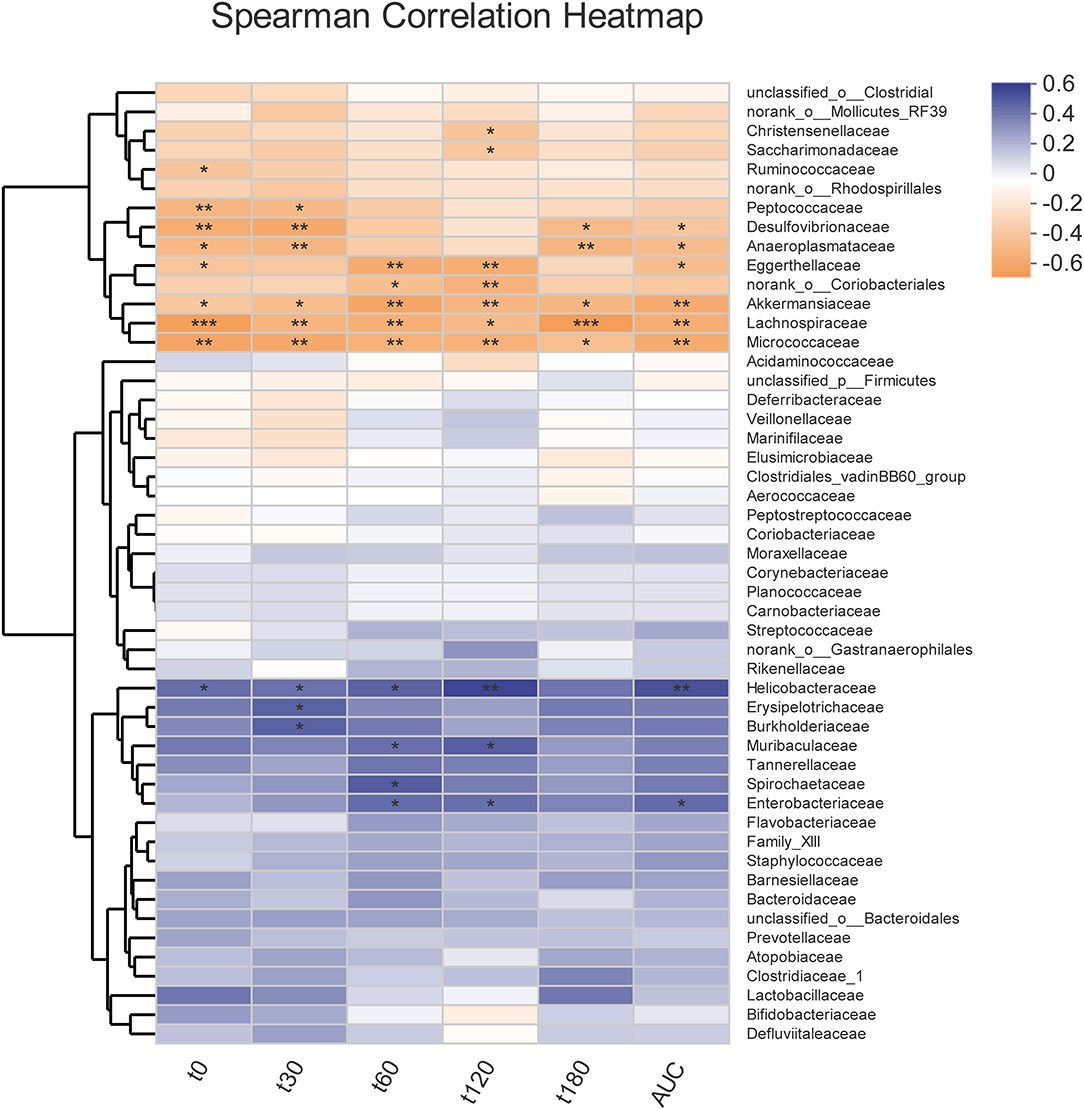
Figure 5. Correlation heatmap of the fecal microbiota with blood glucose indexes. Spearman correlation analysis between the top 50 most abundant bacterial families and blood glucose levels during intragastric glucose tolerance test. *P < 0.05, **P ≤ 0.01, ***P ≤ 0.001.
The linear discriminant analysis (LDA) effect size (LEfSe) revealed that genus, including Bifidobacterium, Lactobacillus, and Treponema 2 were predominant in rats treated with metformin (Figure 6). Lachnoapiraceae, Desulfovibrionaceae, Oscillibacter, Ruminiclostridium 9 were enriched in the dapagliflozin group (Figure 6).
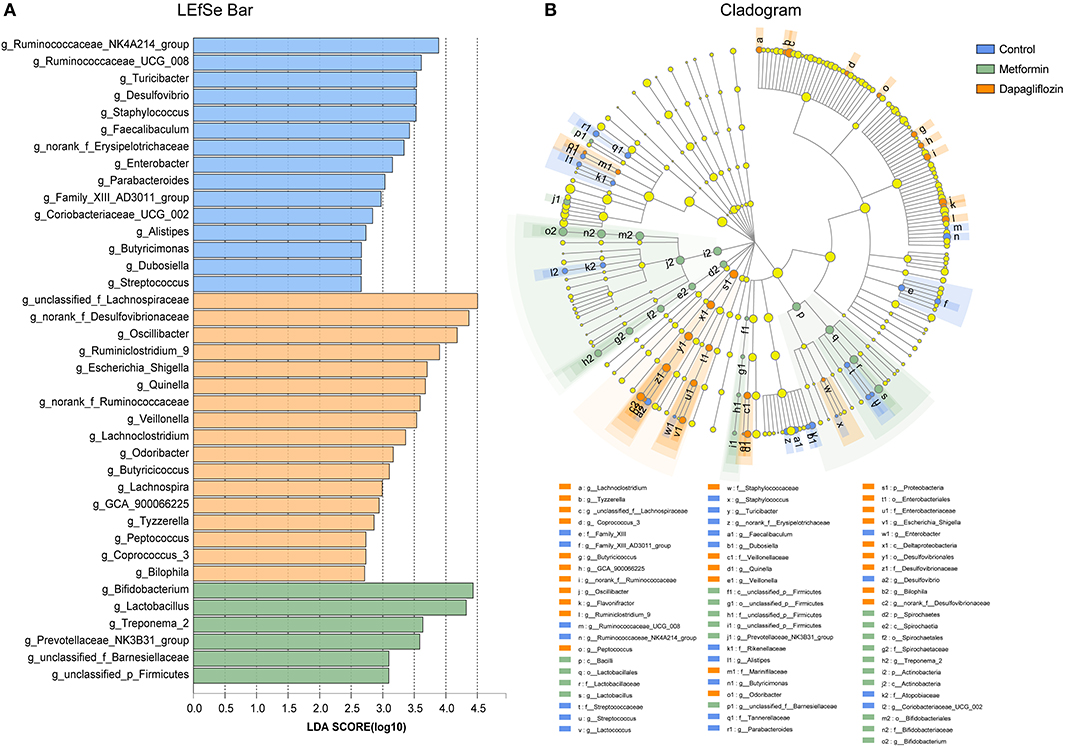
Figure 6. Linear discriminant analysis (LDA) effect size (LEfSe) analysis on genus level among control, metformin, and dapagliflozin group. (A) LEfSe barplot on the bacterial genus level. (B) Cladogram demonstrating the taxonomic levels with phyla in the innermost and genera in the outermost ring. Only LDA score > 2 are shown. All against all as the multiple comparisons. The prefixes “p” represented as phylum, “c” as class, “o” as order, “f” as family, and “g” as genus.
16S rRNA Functional Prediction
By using the PICRUSt and KEGG database on KEGG level 3, glycerolipid metabolism and ion-coupled transporters were enriched in the dapagliflozin group. The Metformin group had more reads which involved purine metabolism and mismatch repair (Supplementary Figure 3A). Concerning the KEGG pathway, glycerolipid metabolism (KO00561) and cytochrome P450 (KO00980) were enriched in dapagliflozin group and three functional categories, purine metabolism (KO00230), nucleotide excision repair (KO03420), and glutathione metabolism (KO00480) (Supplementary Figure 3B), were enriched in the metformin group.
Discussion
Our study demonstrated that dapagliflozin and metformin monotherapy lowered blood glucose levels to a similar extent. They differentially affected the diversities, and composition of fecal microbiota in type 2 diabetic rats. Of interest, is the result that dapagliflozin had no effects on beneficial bacteria (the abundance of Lactobacillaceae, Bifidobacteriaceae, and Akkermansiaceae when compared with control) and that it increased the relative abundance of Proteobacteria (especially Desulfovibrionaceae).
In our study, dapagliflozin did not increase diversity and richness compared with control via alpha diversity analysis, although it has been indicated that dapagliflozin could reduce richness and diversity in mice (13). This result was in line with a recent human study (14). The reason might be the length and dosage difference of the drug intervention (4 weeks vs. 8 weeks; 1 mg/kg/day vs. 60 mg dapagliflozin /kg diet). Beta-diversity analysis and enterotypes were used to further analyze core microbial composition after different treatments.
The concept of “enterotypes” was driven to get an overview of the robust clustering of samples species variation (18). Dapagliflozin and metformin had differential effects on the composition of fecal microbiota enterotypes on the family level. The Dapagliflozin group mainly displayed with Ruminococcaceae and the metformin group presented Ruminococcaceae and Muribaculaceae (18). Ruminococcaceae was regarded as certain SCFA-producing bacteria (19). A Muribaculaceae-history named the S24-7 belongs to the phylum Bacteroidates (20) and it has been suggested that Muribaculaceae abundance is an important predictor of SCFAs levels (21).
Firmicutes and Bacteroidetes are the predominant phyla in gut microbiota, and account for around 90% of the known phylogenetic categories (22). The ratio of Firmicutes to Bacteroidetes was positively related to fecal SCFAs (23). A Danish study found that the ratio of Firmicutes to Bacteroidetes in patients with type 2 diabetes was lower than those in non-diabetic subjects (24, 25). It has been verified that the ratio of Firmicutes to Bacteroidetes was also negatively correlated to fasting blood glucose levels and inflammation status (26). T2DM, induced by HFD and STZ, presented higher contents of pro-inflammatory cytokines and insulin resistance, accompanied by a larger proportion of Firmicutes and a relatively lower Bacteroidetes in the fecal microbiota (27). We did not show any differences in the ratio of Firmicutes to Bacteroidetes, although Lee et al. (13) reported that the ratio of Firmicutes to Bacteroidetes was decreased in diabetic mice after dosage with dapagliflozin. Our findings were consistent with a short time intervention study, which showed that canagliflozin, did not influence the abundance of the Firmicutes to Bacteroidetes ratio (12).
Proteobacteria are enriched in the fecal microbiota of patients with obesity and T2DM (28). Desulfovibrionaceae belongs to the phylum Proteobacteria (Delta) (29). We demonstrated that dapagliflozin increased the relative abundance of Proteobacteria (especially Delta) and Desulfovibrionaceae compared with the metformin and control group. This study firstly presented that dapagliflozin increased Desulfovibrionaceae, but revealed that, on the contrary, metformin decreased Desulfovibrionaceae which was reported in metabolically dysfunctional mice (30).
Actinobacteria is a group of Gram-positive bacteria (31). Bifidobacteriaceae belong to Actinobacteria, and this was considered to improve metabolic endotoxemia and glucose intolerance (32). The abundance of Bifidobacteriaceae was lower in patients with diabetes (32). It has been reported that canagliflozin increased the abundance of Bifidobacterium in mice with chronic kidney disease (12). Dapagliflozin is a much weaker SGLT1 inhibitor as compared with canagliflozin (33), which suppresses SGLT1 by 40–60% (34). Notably, we showed that dapagliflozin and metformin exerted a complementary effect in the relative abundance of Actinobacteria and Bifidobacteriaceae. They were decreased in dapagliflozin while increased in the metformin group. This implied that the combination therapy of dapagliflozin and metformin might be of clinical significance.
Recently, it was revealed that dapagliflozin sustained beta cell mass (35). Our study found that dapagliflozin lowered similar blood glucose levels to metformin and further improved insulin resistance. However, the relationship between beta cell function and fecal microbiota remains uncertain. Whether fecal microbiota improves pancreatic beta cell growth by generating SCFAs needs to be further clarified (36).
This study has several limitations. Firstly, 16S rRNR gene sequencing rather than metagenomics sequencing was used, which limits species level and function analysis on data interpretation. Secondly, we employed HFD and STZ induced diabetic model, which might be a lack of genetic influence. It might be different in the distribution of the fecal microbiota from other diabetic models such as Zucker diabetic fatty rats in our previous study (16). Thirdly, the model of HFD and low-dose STZ to induce a diabetic model might have some deficiencies, as STZ can destroy the beta cells thereby increasing hyperglycemia. However, this is not just the same case in human T2D since the insulin secretion is unchanged or increased. As diabetes progressed, the function of beta cells in some diabetic patients also declined, but there are differences between animal studies and patients with type 2 diabetes. Therefore, the results of our study may need to be verified by diabetic patients. Finally, this study was only a preliminary observation, and the mechanism needs to be further explored. In future studies, we would like to detect the roles of the Desulfovibrionaceae on the pathophysiology and mechanism of diabetes and obesity.
In conclusion, our study demonstrated preliminary results that dapagliflozin had no effects on the abundance of beneficial bacteria. Of interest, is the observation that dapagliflozin enriched Proteobacteria (especially Desulfovibrionaceae), which were associated with obesity and diabetes. Combination dapagliflozin with metformin might have complementary effects on fecal microbiota in the treatment of diabetes.
Data Availability Statement
The datasets presented in this study can be found in online repositories. The names of the repository/repositories and accession number(s) can be found below: NCBI BioProject [accession: PRJNA649663].
Ethics Statement
The animal study was reviewed and approved by Institutional Animal Care and Use Committee of SLAC.
Author Contributions
MY conducted the animal experiments. F-HS wrote the manuscript, modifications, and submission. WenL wrote the methods and results. M-CZ analyzed the data and prepared the figures. R-LF and CQ performed the fecal DNA extraction. WeiL contributed to the study design and review the manuscript. JM was the guarantor of this study who has full access to all the data to guarantee the accuracy and integrity of the data. All authors contributed to the article and approved the submitted version.
Funding
This work was supported by the National Natural Science Foundation of China (grant number NSFC81670728), the Shanghai Municipal Education Commission–Gaofeng Clinical Medicine Grant Support (grant number 20181807), the Shanghai Pujiang Program (2019PJD027), the Shanghai Yangfan Program (grant number 19YF1428500), the Clinical Pharmacy Innovation Research Institute of Shanghai Jiao Tong University School of Medicine (2019) (CXYJY2019QN004), and the Research Funds of Shanghai Health and Family Planning Commission (20204Y0011).
Conflict of Interest
The authors declare that the research was conducted in the absence of any commercial or financial relationships that could be construed as a potential conflict of interest.
Supplementary Material
The Supplementary Material for this article can be found online at: https://www.frontiersin.org/articles/10.3389/fendo.2020.00635/full#supplementary-material
References
1. Singer-Englar T, Barlow G, Mathur R. Obesity, diabetes, and the gut microbiome: an updated review. Expert Rev Gastroenterol Hepatol. (2019) 13:3–15. doi: 10.1080/17474124.2019.1543023
2. Gurung M, Li Z, You H, Rodrigues R, Jump DB, Morgun A, et al. Role of gut microbiota in type 2 diabetes pathophysiology. EBioMedicine. (2020) 51:102590. doi: 10.1016/j.ebiom.2019.11.051
3. Aron-Wisnewsky J, Clément K, Nieuwdorp M. Fecal microbiota transplantation: a future therapeutic option for obesity/diabetes? Curr Diabetes Rep. (2019) 19:51. doi: 10.1007/s11892-019-1180-z
4. Montandon SA, Jornayvaz FR. Effects of antidiabetic drugs on gut microbiota composition. Genes. (2017) 8:250. doi: 10.3390/genes8100250
5. Wu H, Esteve E, Tremaroli V, Khan MT, Caesar R, Manneras-Holm L, et al. Metformin alters the gut microbiome of individuals with treatment-naive type 2 diabetes, contributing to the therapeutic effects of the drug. Nat Med. (2017) 23:850–8. doi: 10.1038/nm.4345
6. de la Cuesta-Zuluaga J, Mueller NT, Corrales-Agudelo V, Velasquez-Mejia EP, Carmona JA, Abad JM, et al. Metformin is associated with higher relative abundance of mucin-degrading akkermansia muciniphila and several short-chain fatty acid-producing microbiota in the gut. Diabetes Care. (2017) 40:54–62. doi: 10.2337/dc16-1324
7. Hernandez MAG, Canfora EE, Jocken JWE, Blaak EE. The short-chain fatty acid acetate in body weight control and insulin sensitivity. Nutrients. (2019) 11:1943. doi: 10.3390/nu11081943
8. Bauer PV, Duca FA, Waise TMZ, Rasmussen BA, Abraham MA, Dranse HJ, et al. Metformin alters upper small intestinal microbiota that impact a glucose-sglt1-sensing glucoregulatory pathway. Cell Metab. (2018) 27:101–17.e105. doi: 10.1016/j.cmet.2017.09.019
9. Tahrani AA, Barnett AH, Bailey CJ. SGLT inhibitors in management of diabetes. Lancet Diabetes Endocrinol. (2013) 1:140–51. doi: 10.1016/s2213-8587(13)70050-0
10. van Bommel EJ, Muskiet MH, Tonneijck L, Kramer MH, Nieuwdorp M, van Raalte DH. SGLT2 inhibition in the diabetic kidney-from mechanisms to clinical outcome. Clin J Am Soc Nephrol. (2017) 12:700–10. doi: 10.2215/cjn.06080616
11. Lehmann A, Hornby PJ. Intestinal SGLT1 in metabolic health and disease. Am J Physiol Gastrointest Liver Physiol. (2016) 310:G887–98. doi: 10.1152/ajpgi.00068.2016
12. Mishima E, Fukuda S, Kanemitsu Y, Saigusa D, Mukawa C, Asaji K, et al. Canagliflozin reduces plasma uremic toxins and alters the intestinal microbiota composition in a chronic kidney disease mouse model. Am J Physiol Renal Physiol. (2018) 315:F824–33. doi: 10.1152/ajprenal.00314.2017
13. Lee DM, Battson ML, Jarrell DK, Hou S, Ecton KE, Weir TL, et al. SGLT2 inhibition via dapagliflozin improves generalized vascular dysfunction and alters the gut microbiota in type 2 diabetic mice. Cardiovasc Diabetol. (2018) 17:62. doi: 10.1186/s12933-018-0708-x
14. van Bommel EJM, Herrema H, Davids M, Kramer MHH, Nieuwdorp M, van Raalte DH. Effects of 12-week treatment with dapagliflozin and gliclazide on faecal microbiome: Results of a double-blind randomized trial in patients with type 2 diabetes. Diabetes Metab. (2019) 46:164–8. doi: 10.1016/j.diabet.2019.11.005
15. Zhang Q, Xiao X, Li M, Li W, Yu M, Zhang H, et al. Acarbose reduces blood glucose by activating miR-10a-5p and miR-664 in diabetic rats. PLoS ONE. (2013) 8:e79697. doi: 10.1371/journal.pone.0079697
16. Zhang M, Feng R, Yang M, Qian C, Wang Z, Liu W, et al. Effects of metformin, acarbose, and sitagliptin monotherapy on gut microbiota in Zucker diabetic fatty rats. BMJ Open Diabetes Res Care. (2019) 7:e000717. doi: 10.1136/bmjdrc-2019-000717
17. Kayano Y, Horiuchi I, Mori YI, Ishida K, Saito T, Taguchi M, et al. A simulation study to evaluate limited sampling strategies to estimate area under the curve of drug concentration versus time following repetitive oral dosing: limited sampling model versus naive trapezoidal method. Biol Pharm Bull. (2009) 32:1486–90. doi: 10.1248/bpb.32.1486
18. Arumugam M, Raes J, Pelletier E, Le Paslier D, Yamada T, Mende DR, et al. Enterotypes of the human gut microbiome. Nature. (2011) 473:174–80. doi: 10.1038/nature09944
19. Zhao L, Lou H, Peng Y, Chen S, Zhang Y, Li X. Comprehensive relationships between gut microbiome and faecal metabolome in individuals with type 2 diabetes and its complications. Endocrine. (2019) 66:526–37. doi: 10.1007/s12020-019-02103-8
20. Lagkouvardos I, Lesker TR, Hitch TCA, Galvez EJC, Smit N, Neuhaus K, et al. Sequence and cultivation study of Muribaculaceae reveals novel species, host preference, and functional potential of this yet undescribed family. Microbiome. (2019) 7:28. doi: 10.1186/s40168-019-0637-2
21. Smith BJ, Miller RA, Ericsson AC, Harrison DC, Strong R, Schmidt TM. Changes in the gut microbiome and fermentation products concurrent with enhanced longevity in acarbose-treated mice. BMC Microbiol. (2019) 19:130. doi: 10.1186/s12866-019-1494-7
22. Qin J, Li R, Raes J, Arumugam M, Burgdorf KS, Manichanh C, et al. A human gut microbial gene catalogue established by metagenomic sequencing. Nature. (2010) 464:59–65. doi: 10.1038/nature08821
23. Fernandes J, Su W, Rahat-Rozenbloom S, Wolever TM, Comelli EM. Adiposity, gut microbiota and faecal short chain fatty acids are linked in adult humans. Nutr Diabetes. (2014) 4:e121. doi: 10.1038/nutd.2014.23
24. Larsen N, Vogensen FK, van den Berg FW, Nielsen DS, Andreasen AS, Pedersen BK, et al. Gut microbiota in human adults with type 2 diabetes differs from non-diabetic adults. PLoS ONE. (2010) 5:e9085. doi: 10.1371/journal.pone.0009085
25. Demirci M, Bahar Tokman H, Taner Z, Keskin FE, Çagatay P, Ozturk Bakar Y, et al. Bacteroidetes and firmicutes levels in gut microbiota and effects of hosts TLR2/TLR4 gene expression levels in adult type 1 diabetes patients in Istanbul, Turkey. J Diabetes Compl. (2020) 34:107449. doi: 10.1016/j.jdiacomp.2019.107449
26. Yan H, Lu J, Wang Y, Gu W, Yang X, Yu J. Intake of total saponins and polysaccharides from Polygonatum kingianum affects the gut microbiota in diabetic rats. Phytomedicine. (2017) 26:45–54. doi: 10.1016/j.phymed.2017.01.007
27. Han L, Li T, Du M, Chang R, Zhan B, Mao X. Beneficial effects of potentilla discolor bunge water extract on inflammatory cytokines release and gut microbiota in high-fat diet and streptozotocin-induced type 2 diabetic mice. Nutrients. (2019) 11. doi: 10.3390/nu11030670
28. Zhang Q, Yu H, Xiao X, Hu L, Xin F, Yu X. Inulin-type fructan improves diabetic phenotype and gut microbiota profiles in rats. PeerJ. (2018) 6:e4446. doi: 10.7717/peerj.4446
29. Schoenborn L, Abdollahi H, Tee W, Dyall-Smith M, Janssen PH. A member of the delta subgroup of proteobacteria from a pyogenic liver abscess is a typical sulfate reducer of the genus Desulfovibrio. J Clin Microbiol. (2001) 39:787–90. doi: 10.1128/jcm.39.2.787-790.2001
30. Just S, Mondot S, Ecker J, Wegner K, Rath E, Gau L, et al. The gut microbiota drives the impact of bile acids and fat source in diet on mouse metabolism. Microbiome. (2018) 6:134. doi: 10.1186/s40168-018-0510-8
31. Kothe E. Special focus: actinobacteria. J Basic Microbiol. (2018) 58:719. doi: 10.1002/jobm.201870028
32. Gonai M, Shigehisa A, Kigawa I, Kurasaki K, Chonan O, Matsuki T, et al. Galacto-oligosaccharides ameliorate dysbiotic Bifidobacteriaceae decline in Japanese patients with type 2 diabetes. Benef Microbes. (2017) 8:705–16. doi: 10.3920/bm2016.0230
33. Papakitsou I, Vougiouklakis G, Elisaf MS, Filippatos TD. Differential pharmacology and clinical utility of dapagliflozin in type 2 diabetes. Clin Pharmacol. (2019) 11:133–43. doi: 10.2147/cpaa.s172353
34. Mori K, Saito R, Nakamaru Y, Shimizu M, Yamazaki H. Physiologically based pharmacokinetic-pharmacodynamic modeling to predict concentrations and actions of sodium-dependent glucose transporter 2 inhibitor canagliflozin in human intestines and renal tubules. Biopharm Drug Dispos. (2016) 37:491–506. doi: 10.1002/bdd.2040
35. Omori K, Nakamura A, Miyoshi H, Takahashi K, Kitao N, Nomoto H, et al. Effects of dapagliflozin and/or insulin glargine on beta cell mass and hepatic steatosis in db/db mice. Metabolism. (2019) 98:27–36. doi: 10.1016/j.metabol.2019.06.006
Keywords: dapagliflozin, fecal microbiota, type 2 diabetes, metfomiin, high-fat diet
Citation: Yang M, Shi F-H, Liu W, Zhang M-C, Feng R-L, Qian C, Liu W and Ma J (2020) Dapagliflozin Modulates the Fecal Microbiota in a Type 2 Diabetic Rat Model. Front. Endocrinol. 11:635. doi: 10.3389/fendo.2020.00635
Received: 28 May 2020; Accepted: 05 August 2020;
Published: 17 November 2020.
Edited by:
Jean-François Tanti, INSERM U1065 Centre Méditerranéen de Médecine Moléculaire, FranceReviewed by:
Remy Burcelin, Institut National de la Santé et de la Recherche Médicale (INSERM), FranceMelkam Alamerew Kebede, The University of Sydney, Australia
Copyright © 2020 Yang, Shi, Liu, Zhang, Feng, Qian, Liu and Ma. This is an open-access article distributed under the terms of the Creative Commons Attribution License (CC BY). The use, distribution or reproduction in other forums is permitted, provided the original author(s) and the copyright owner(s) are credited and that the original publication in this journal is cited, in accordance with accepted academic practice. No use, distribution or reproduction is permitted which does not comply with these terms.
*Correspondence: Jing Ma, Y2hlcnJ5MTk5NkBsaXZlLmNu
†These authors have contributed equally to this work and share first authorship