- 1Department of Endocrinology, The Third Hospital of Hebei Medical University, Shijiazhuang, China
- 2Key Laboratory of Orthopedic Biomechanics of Hebei Province, The Third Hospital of Hebei Medical University, Shijiazhuang, China
- 3Trauma Medicine Center, Peking University People’s Hospital, Beijing, China
- 4National Center for Trauma Medicine, Peking University People's Hospital, Beijing, China
Objective: The objective of this study was to analyze the quantitative association between advanced glycation end products (AGEs) and adjusted FRAX by rheumatoid arthritis (FRAX-RA) in postmenopausal type 2 diabetic (T2D) patients. The optimal cutoff value of AGEs was also explored, which was aimed at demonstrating the potential value of AGEs on evaluating osteoporotic fracture risk in postmenopausal T2D patients.
Methods: We conducted a cross-sectional study including 366 postmenopausal participants (180 T2D patients [DM group] and 186 non-T2D individuals [NDM group]). All the subjects in each group were divided into three subgroups according to BMD. Physical examination, dual-energy x-ray absorptiometry (DXA), and serum indicators (including serum AGEs, glycemic parameters, bone turnover markers and inflammation factors) were examined. The relationship between FRAX-RA, serum laboratory variables, and AGEs were explored. The optimal cutoff value of AGEs to predict the risk of osteoporotic fracture was also investigated.
Results: Adjusting the FRAX values with rheumatoid arthritis (RA) of T2D patients reached a significantly increased MOF-RA and an increasing trend of HF-RA. AGEs level was higher in the DM group compared to the NDMs, and was positively correlated with MOF-RA (r=0.682, P<0.001) and HF-RA (r=0.677, P<0.001). The receiver operating characteristic curve analysis revealed that the area under the curve was 0.804 (P<0.001), and the optimal AGEs cut-off value was 4.156mmol/L. Subgroup analysis for T2D patients revealed an increase in TGF-β, IL-6 and SCTX in the osteoporosis group, while a decreased PINP in the osteoporosis group compared to the other two subgroups. AGEs were positively associated with FBG, HbA1c, HOMA-IR, S-CTX, IL-6 and TGF-β in T2D patients, and negatively associated with PINP.
Conclusions: RA-adjusted FRAX is a relevant clinical tool in evaluating fracture risk of postmenopausal T2D patients. Our study analyzed the relationship between AGEs and FRAX-RA, and explored the threshold value of AGEs for predicting fracture risk in postmenopausal T2D patients. AGEs were also associated with serum bone turnover markers and inflammation factors, indicating that the increasing level of AGEs in postmenopausal T2D patients accelerated the expression of inflammatory factors, which led to bone metabolism disorders and a higher risk of osteoporotic fractures.
Introduction
Osteoporosis is prevalent in type 2 diabetes mellitus (T2D) postmenopausal patients, which affects human health, life quality and increases the socioeconomic burden (1). T2D patients have bone mineral density (BMD) that is either unchanged or slightly higher than normal, but they exhibit skeletal fragility independent of BMD (2, 3), even after accounting for some factors (such as body mass index [BMI] and falls) (4, 5), which indicates patients with T2D have a higher fracture risk due to bone fragility independent of BMD. Besides, Diabetes status was associated with low muscle mass and low muscle strength, and the association depended on BMI (6). The concomitance of sarcopenia and osteoporosis which was so-called “osteosarcopenia”, may lead to an increase in fracture risk of T2D than the non-diabetic ones (7). Older adults with osteosarcopenia have to be regarded as the most at-risk population for fractures (8). Thus, the unadjusted fracture risk assessment tool (FRAX) mostly depends on dual-energy x-ray absorptiometry (DXA) detection could also underestimate the fracture risk in T2D patients (9, 10).
Approximately 70% of bone strength is determined by BMD, while collagen fiber composition depends on bone tissue’s tensile strength and ductility. Collagen molecular crosslinking can be divided into beneficial enzyme-catalyzed immature bivalent cross-linking and mature trivalent crosslinking, and unfavorable non-enzyme-catalyzed crosslinking, such as advanced glycation end products (AGEs). AGEs are the spontaneous reaction products between extracellular sugars and amino acid residues on collagen fibers (11). The accumulation of AGEs in the bone can reduce skeletal hardness biomechanical properties (12). Previous studies showed a significantly increased AGEs level in T2D patients (13, 14), which was related to low bone quality and high fracture risk in postmenopausal women (15). Meanwhile, the accumulation of AGEs is associated with impaired bone microarchitecture. It has been reported that AGEs bone content correlated with worse bone microarchitecture in trabecular, including lower volumetric BMD, bone volume fraction, and increased separation/spacing (16). Bone microarchitecture could be regarded as an independent predictor of fracture risk (17). Although the FRAX includes some diseases related to osteoporosis, other risk factors were not accounted for, such as falls, the duration and dosage of glucocorticoids, the etiology and type of diabetes, or other secondary osteoporosis. FRAX base on BMD may not always accurately predict the fracture risk of T2D patients. Therefore, we speculate that abnormal cross-linking of collagen molecules may be an important factor contributing to impaired bone quality and increased skeletal fragility, which increased the fracture risk in postmenopausal T2D patients.
In this study, we adopted a method previously reported by both Hu et al. and Leslie et al. (18, 19), rheumatoid arthritis (RA) was selected as an analogous variable of T2D to obtain the FRAX predictive value for fracture risk. Thus, the objective of this study was to analyze the quantitative association between AGEs and adjusted FRAX by RA (FRAX-RA) in postmenopausal T2D patients. The optimal cutoff value of AGEs was also explored, which was aimed at demonstrating the potential influence of AGEs on osteoporotic fracture risk in postmenopausal T2D patients. Moreover, we tried to use HR-pQCT to verify the status of bone microstructure of T2DM patients in “High-AGEs” or “Low-AGEs” group defined by its cut off value in a small-size sample.
Materials and methods
Subject recruitment
We collected 180 postmenopausal T2D patients (DM group) and 186 healthy individuals (NDM group) who were recruited from the Endocrinology Department of the Third Hospital of Hebei Medical University from October 2019 to May 2020. Each cohort was divided into three subgroups (non-diabetic subjects with normal BMD [Control], non-diabetic subjects with osteopenia [OPN], non-diabetic subjects with osteoporosis [OP], diabetic patients with normal BMD[DMN], diabetic patients with osteopenia [DMOPN], diabetic patients with osteoporosis [DMOP]) according to BMD. All subjects submitted written informed consent prior to participating in this study, which was authorized by the Third Hospital of Hebei Medical University’s ethical committee.
Inclusion and exclusion criteria
The subjects were chosen based on the following criteria: 1) All subjects were aged between 45 and 80, natural menopause for more than 3 years or menopause caused by surgery (operating time after 40 years old); 2) the WHO’s (1999) diabetes criteria: diabetic symptoms (polydipsia, polyuria, polyphagia, weight loss) + blood glucose level at any time ≥ 11.1mmol/L or fasting glucose ≥ 7.0mmol/L or 2hours postprandial glucose≥11.1mmol/L. Type 1 diabetes mellitus were excluded from this study; 3) the WHO’s osteoporosis criteria: the diagnosis of osteoporosis in postmenopausal women is based on the T value. T value ≥ -1SD was normal bone mineral density, -1SD < T value < -2.5SD was osteopenia; T value ≤ -2.5SD was osteoporosis.
Subjects with these conditions were excluded: severe heart, liver, and kidney disease, thyroid and parathyroid disease, autoimmune disease, rheumatism, long-term use of hormones and thiazide diuretics, use of antidiabetic drugs that may affect bone metabolism for more than three months (metformin, thiazolidinediones, glucagon-like peptide-1 receptor agonist, sodium-glucose cotransporter 2 inhibitor), long-term stay in bed or chronic smoking (smoking for more than 15 years, averaging more than 15 cigarettes a day), BMI is less than 20kg/m2.
Laboratory assessment
We collected data from all subjects (including age, menopausal age, weight, and height), measured serum concentrations of fasting plasma glucose (FPG), glycosylated hemoglobin (HbA1c) and fasting insulin (FIns) by using standard laboratory techniques, measured serum AGEs, insulin, 25-hydroxyvitamin D3 (25-OHD3), procollagen type I N-peptide (PINP), serum C-terminal telopeptide of type I collagen (S-CTX) by the enzyme-linked immunosorbent assay (ELISA) kit (Cusabio, Wuhan, China), measured serum concentrations of insulin, Interleukin-1β (IL-1β), IL-6, tumor necrosis factor-α (TNF-α) and transforming growth factor-β (TGF-β) by the ELISA kit (Excellbio, shanghai, China). BMI was determined using the following equation: BMI=Weight/Height2 (kg/m2). The following formula was used to calculate the insulin resistance index (HOMA-IR): HOMA-IR = FPG * FIns/22.5.
BMD assessment
We evaluated the level of areal BMDs at the lumbar spine (LS, L2-L4), proximal femur (femoral neck and total hip) for each individual using a DXA device (Hologic, USA). The measurements were all taken by the same technician to ensure consistent and reliable results, and the CVs were 1.73% across the board.
Fracture risk assessment tool
The predicted 10-year risk of major and hip osteoporotic fractures was determined using the Asian-China Assessment System (https://www.sheffield.ac.uk/FRAX/tool.aspx?country=2). The FRAX algorithm includes risk factors of age, gender, height, weight, previous fracture history, parents’ history of fragility fractures, smoking status, long-term corticosteroid use, RA history, daily alcohol consumption, secondary OP, and femoral neck bone density. The history of RA was replaced in the algorithm for the current study to calculate FRAX-RA.
Bone microarchitectural measurements
HR-pQCT was used to verify the status of bone microstructure of T2DM patients in both “High-AGEs” (AGEs>4.156mmol/L) or “Low-AGEs” (AGEs<4.156mmol/L) group defined by its cut off value. We chose 14 subjects aged 50-60yr without fracture history (8 in High-AGEs group and 6 in Low-AGEs group) underwent HR-pQCT of the nondominant distal radius and tibia (Xtreme CT II; Scanco Medical AG, Bassersdorf, Switzerland) according to the manufacturer’s standard in vivo acquisition protocol (68 kVp, 1470 μA, matrix size of 2304×2304) (20). The reference line was placed at the endplates of the distal radius and tibia in all tested participants. The scan region was 10.2mm in length, and was fixed starting at 9.0 mm and 22.0 mm proximal to the reference lines of the radius and tibia respectively. The measured parameters were as follows: cortical thickness (Ct.Th, mm); cortical porosity (Ct.Po, %); trabecular bone volume fraction (Tb.BV/TV, %), number (Tb.N, 1/mm), thickness (Tb.Th, mm) and separation (Tb.Sp, mm). The measurements were all taken by the same technician to ensure consistent and reliable results.
Statistical analyses
All statistical analysis was performed using SPSS version 21.0. We used the Kolmogorov-Smirnov test to confirm the normal distribution of variables for each group. The median (interquartile range) was used to express data for non-continuous variables, whereas the mean ± SD was used to express data for continuous variables. The Student’s T-Test is utilized to compare 2 groups that adhere to the normal distribution and uniform variance, and the Wilcoxon test is used to compare 2 groups that do not obey the normal distribution. We used the ANOVA or Friedman test to compare the quantitative variables among groups. Pearson or Spearman correlation tests were used to determine relationships between variables. In order to determine or assess the best AGE cutoff value for predicting or evaluating the risk of osteoporotic fracture, receiver operating characteristic (ROC) curves were used. Maximum sensitivity and specificity for fracture risk are achieved by the cut-off value. Estimating the area under the curve was served to evaluate the test’s discriminatory ability. A difference with a P value of 0.05 or lower is considered statistically significant for all statistical tests.
Results
Baseline features of the subjects
The general characteristics of the subjects are displayed in Table 1. T2D patients had higher BMI compared to non-diabetics (P=0.034), while the two groups were comparable in age and menopause duration. Analysis of subgroups indicates that BMI in DMOP group was considerably lower than in DMN and DMOPN groups (P<0.05), and OP group had significantly lower BMI than the Control and OPN groups (P<0.05).
BMD, FRAX, and RA-FRAX comparation among DM and NDM groups
DM group had substantially higher BMD than the non-diabetics (P<0.05), as shown in Figure 1A. The probabilities of major osteoporotic fractures (MOF) and hip osteoporotic fractures (HF) in T2D patients were lower than the non-diabetics (P<0.05, Figure 1B). Then, in order to obtain MOF-RA and HF-RA, we altered the FRAX values of T2D patients by choosing RA as an analogous variable. A significant increase of MOF-RA in DM group was found than the NDM group (P<0.05), while DM group tends to have higher HF-RA than NDM group (Figure 1C).
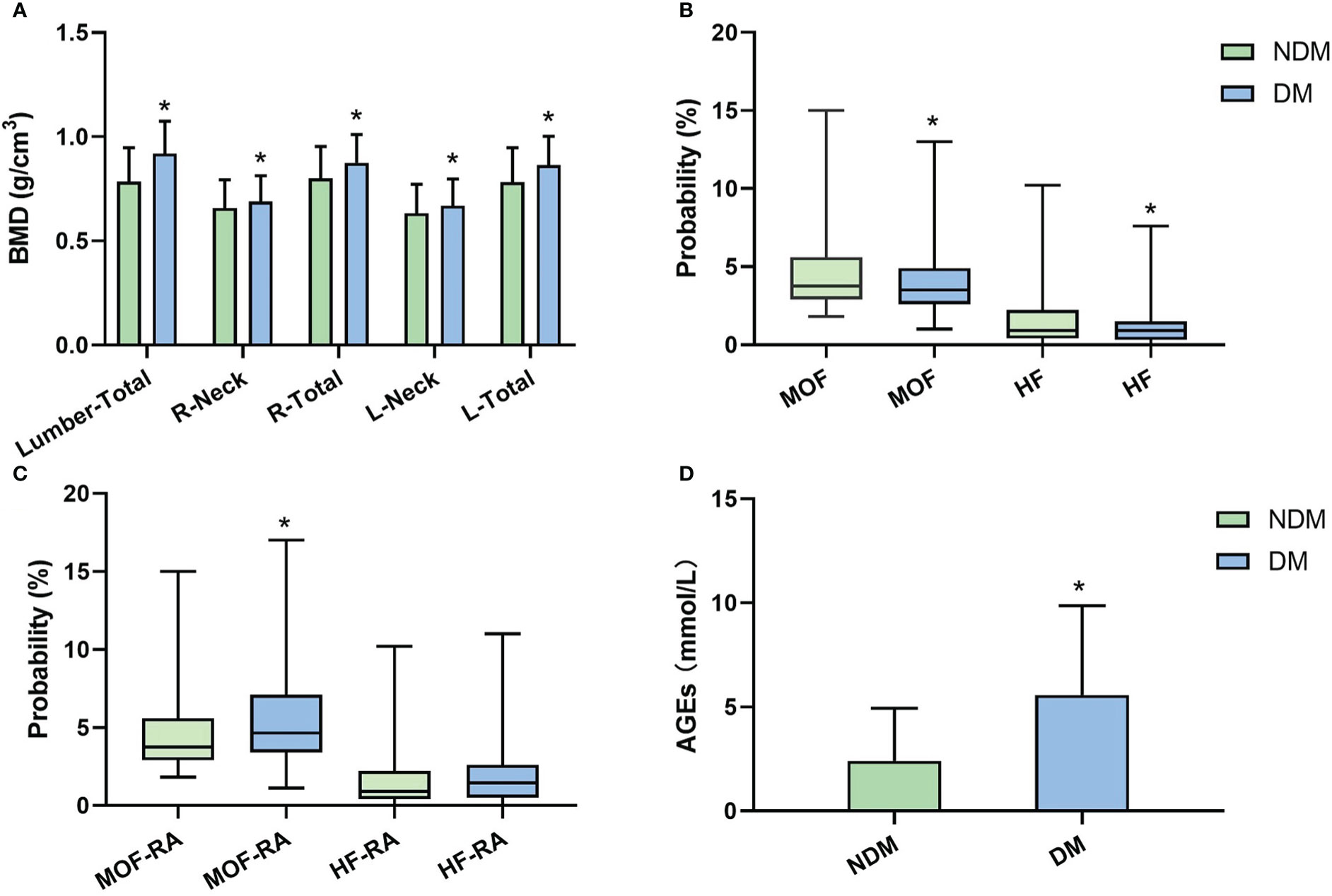
Figure 1 BMD, FRAX values (without correction), FRAX values (corrected by rheumatoid arthritis [RA]), and AGEs level between NDM and DM groups. (A) BMD comparation in each area; (B) major osteoporotic fractures (MOF) and hip osteoporotic fractures (HF) comparation; (C) Adjusted MOF by RA (MOF-RA) and adjusted MOF by RA (HF-RA) comparation; (D) AGEs comparation. (*P<0.05 compared to the NDM group).
AGEs level comparison between DM and NDM groups
In comparison to non-diabetics, we found that DM patients had considerably higher AGEs levels (P<0.05, Figure 1D). According to Pearson correlation analysis, AGEs level was positively correlated with MOF-RA (r=0.682, P<0.001) (Figure 2A) and HF-RA (r=0.677, P<0.001) (Figure 2B).
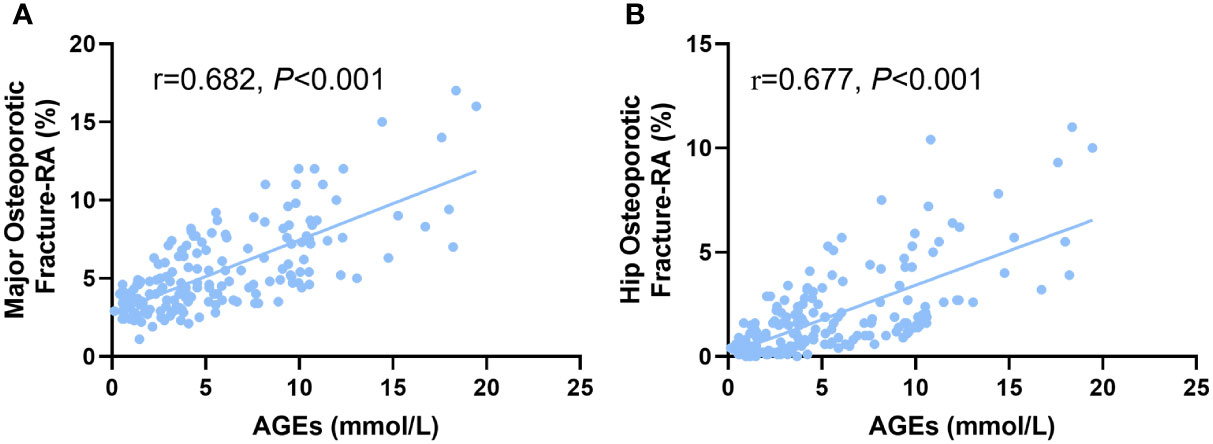
Figure 2 The correlation of AGEs with (A) major osteoporotic fractures and (B) hip osteoporotic fractures adjusted by rheumatoid arthritis (RA) in postmenopausal T2D patients.
Evaluation of the AGEs optimal cutoff value to predict osteoporotic fracture risk
To determine the ideal AGE cut-off value for evaluating fracture risk in postmenopausal T2D patients, the ROC curve was used. As shown in Figure 3, the area under ROC curve (AUC) was recorded as 0.804 (95% confidence interval [CI]:0.749-0.858, P<0.001), and the optimal AGEs cut-off value leading to a high fracture risk was 4.156mmol/L. This suggests postmenopausal T2D patients have an increased risk of fracture when AGEs level is higher than 4.156mmol/L. We then tried to verify our AGEs cut-off value by measuring bone microstructure in T2D postmenopausal women. A total of 14 subjects aged 50-60yr without fracture history underwent HR-pQCT examination in nondominant distal radius and tibia, 8 in High-AGEs group and 6 in Low-AGEs group. The Ct.Po was increased in the High-AGEs group than the Low-AGEs group at tibia. And the results of radius were consistent with tibia (P<0.05, Supplementary Figures 1D, 2D). No difference was found in Ct.Th and trabecular parameters (Tb.BV/TV, Tb.N, Tb.Th, and Tb.Sp) between these two groups in both tibia and radius (Supplementary Figures 1A–C, E–H, 2A–C, E–H).
Glucose parameters, bone turnover markers, and inflammation factors comparation among DMN, DMOPN and DMOP groups
First, no differences were found when comparing FBG, HbA1c, insulin, and HOMA-IR among the three groups (P>0.05, Table 2). Then we compared bone turnover markers among the three groups. Results revealed that the DMOP group had lower levels of PINP and 25-OHD3 than the DMN and DMOPN groups (P<0.05, Table 2), while an increase of S-CTX in DMOP group than the other two groups. Next, the comparison of inflammation factors showed that DMOP patients had higher IL-6 and TGF-β levels compared to both DMN and DMOPN groups (P<0.05, Table 2). However, in terms of IL-1β and TNF-α, there were no noticeable differences among the three groups (P>0.05, Table 2).
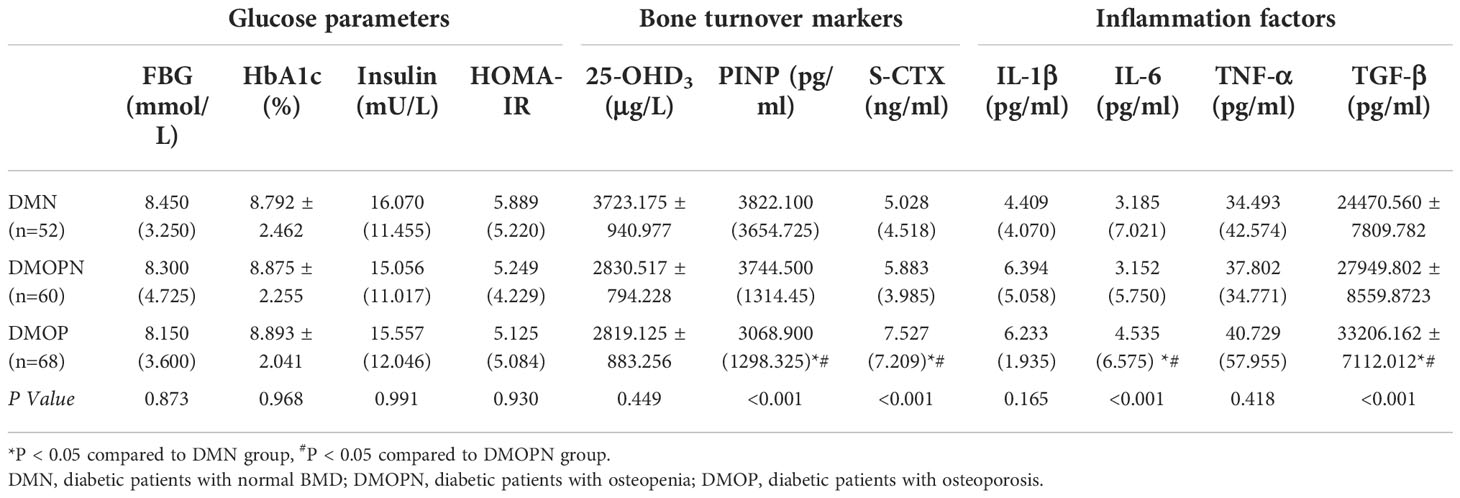
Table 2 Glucose parameters, bone turnover markers, and inflammation factors comparation among DMN, DMOPN and DMOP groups.
Correlations of glycemic parameters, bone turnover markers, and inflammatory factors with AGEs among T2D patients
We used Spearman or Pearson correlation to analyze the relationship among glycemic parameters, bone turnover markers, inflammatory factors, and AGEs in postmenopausal T2D patients. As shown in Table 3, AGEs were positively correlated with FBG, HbA1c, and HOMA-IR levels (r=0.323, r=0.191, r=0.190 respectively, P<0.05). No linear correlations were found between AGEs and insulin. Besides, AGEs were negatively correlated with PINP (r=-0.161, P<0.05) and positively correlated with S-CTX (r=0.167, P<0.05), while no correlation was found between AGEs and 25-OHD3. Serum levels of AGEs were found to be significantly positively correlated with IL-6 and TGF-β (r=0.417, r=0.580 respectively, P<0.05), but no linear correlation was found between IL-1β, TNF-α and AGEs.

Table 3 Correlations of glycemic parameters, bone turnover markers, and inflammatory factors with AGEs among postmenopausal type 2 diabetic patients.
Discussion
Osteoporosis is a frequent metabolic bone disease. Moreover, diabetic patients with osteoporosis would have a greater overall disease burden. Even after adjusting for BMD, BMI, visual impairment and falls, T2D individuals have a higher risk of fragility fractures (21). However, previous studies showed that individuals with T2D show unaltered (22, 23) or paradoxically increased (24, 25) BMD. Our results also showed that BMDs in DM group was significantly higher than the non-diabetics in postmenopausal women, which may partly be due to higher BMI. Therefore, diabetes-related changes in bone metabolism or biochemistry may be independent of other changes in bone microstructure and tissue properties other than BMD (26). Despite the fact that BMD understates the risk of fracture in diabetic patients, it remains to be the gold standard for evaluating bones in this population due to its high accessibility and low cost (27–29).
The most popular tool for assessing fracture risk is FRAX, and it can be used to calculate an individual’s 10-year risk of hip and severe osteoporotic fracture (30). Recent research indicates that T2D considerably raises fracture risk independent of other risk factors (31, 32). However, T2D is not one of the clinical risk variables in the FRAX algorithm. To increase the performance of FRAX in patients with T2D, it is advised to input RA to represent the condition of diabetes (18, 33). In the present study, we used a conventional BMD-based FRAX score to analyze the incidence of MOF and HF in all subjects and found both MOF and HF were significantly lower in DM patients. We subsequently selected RA as the equivalent variable of T2D based on the prior work to increase the precision of FRAX in the fracture risk evaluation of T2D patients (18), and found DM group had a significant increase in MOF-RA and a trend of higher HF-RA than NDM group. This result indicates that adjusting for RA when calculating FRAX may reflect the fracture risk of T2D patients more realistically.
After adjusting by RA, the FRAX score was numerically closer to the realistically fracture risk in T2D patients, but it could not explain the pathogenesis of the increased fracture risk in T2D individuals. Fractures are influenced by a complicated pathophysiological interplay between T2D parameters including a prolonged illness duration (34), diabetic complications, poor glycemic control (35), insulin resistance (36), and the use of insulin or oral antidiabetic medication (37, 38). It is yet unknown how deteriorating glycemic control might alter the characteristics of bone tissue. Hypothesized mechanisms include impaired bone remodeling, bone microvascular insufficiency, alterations in endocrine function, and accumulation of AGEs (21). It’s worth noting that in a prolonged hyperglycinemia state, glucose reacts with proteins to form AGEs, which may degrade bone tissue properties (39–42). The interaction of AGEs with the receptor (RAGE) on osteoblastic lineage cells results in decreased enzymatic collagen maturity, altered collagen fibrils profile, and further disrupts the mineralization process (43, 44). Additionally, the accumulation of AGEs leads to a promotion of inflammation and oxidative stress, which increases the differentiation and activation of osteoclasts (45) and induces osteoblast apoptosis (46, 47). This process also contributes to a low bone turnover state (48, 49). Our result showed that the elevated AGEs level was positively correlated with MOF-RA and HF-RA in postmenopausal women with T2D, indicating AGEs levels are strongly associated with fracture risk in T2D patients. The optimal AGEs cut-off value leading to a high fracture risk was 4.156mmol/L, which suggests postmenopausal T2D patients have an increased fracture risk when the AGEs level is higher than 4.156mmol/L. Previous studies indicated that the impaired bone microarchitecture has a considerable influence on bone strength and is essential in fracture initiation and propagation (50, 51). A meta-analysis reported the increase of cortical porosity is relevant to bone quality decline and increased fracture risk. It was also proved that cancellous bone preferentially accumulates AGEs relative to cortical bone (52). We thus verify the status of bone microstructure of T2DM patients in both High-AGEs or Low-AGEs group defined by its cut off value. The result showed the cortical porosity was increased in the High-AGEs group than the Low-AGEs group at tibia. No difference was found in cortical thickness and trabecular parameters between these two groups in both tibia and radius. These results were consistent with previous studies that AGEs bone content correlated with worse bone microarchitecture (16). However, Hunt et al. observed the trend of higher BV/TV values and greater mineral content in the T2D specimens which increased the bone strength (11). We speculated that the difference was because of Hunt et al. only analyzed cancellous bone structure, and their subjects was male T2D patients, which was quite different from us. Therefore, we suggest that the AGEs level as a correction factor that could improve the capacity of FRAX algorithm to predict fracture risk in T2D postmenopausal women.
Serum bone turnover markers can be used to assess bone loss or formation more sensitive than BMD (53–55). Previous studies demonstrated reduced bone resorption and formation in T2D individuals (56–58), suggesting that hyperglycemia and AGEs crosslinking may impair the function of osteoblasts and osteoclasts, thereby inhibiting bone formation and promoting bone resorption. Correlation analysis in our study also confirmed the AGEs level was positively correlated with glycemic parameters including FBG, HbA1c, HOMA-IR, bone resorption marker S-CTX, and negatively correlated with bone formation marker PINP in postmenopausal T2D patients. These findings imply that deteriorating glycemic control may contribute to the accumulation of AGEs, which interfere with normal osteoblast function and impair osteoblast development. AGEs may also reduce bone resorption by suppressing osteoclastic differentiation as well as changing the structural integrity of matrix proteins.
Patients with T2D have higher levels of AGEs due to hyperglycemia, which can also increase the production of inflammatory cytokines and reactive oxygen species, setting off a vicious cycle of chronic inflammation and bone resorption (59). Activating of RAGE in both osteoclasts (60, 61) and osteoblasts (46, 62) could induce up-regulation of pro-inflammatory cytokines such as IL-1β, IL-6, and TNF-α, which could directly affect bone homeostasis (63, 64). Accumulating evidence indicates that the TGF-β also plays an important role in the osteogenic progress affected by AGEs, especially biologically potent AGE2 and AGE3 (65, 66). Yamaguchi et al. conducted a series of studies and found that AGE2 and AGE3 suppressed stomal ST2 cell growth, differentiation, and mineralization, as well as increased apoptosis of osteoblastic cells by up-regulating TGF-β (67–69). As was shown in a clinical study, T2D patients have increased serum levels of IL-6, TGF-β, and TNF-α (70). We also found elevated levels of IL-6 and TGF-β in postmenopausal T2D patients, both of which positively correlated with AGEs levels. Thus, we hypothesized that the bone fragility and increased fracture risk of T2D patients may be due to AGE-induced IL-6 and TGF-β related inflammatory response. At present, the related mechanism of IL-6 and TGF-β on bone collagen abnormal cross-linking is still incomplete, and further research is needed.
In conclusion, both DXA and FRAX scores underestimated the accurate fracture risk in T2D patients. RA-adjusted FRAX is an efficient clinical tool for determining the risk of fracture in postmenopausal T2D patients. AGEs were also associated with serum bone turnover markers and inflammation factors, indicating that the increasing level of AGEs in postmenopausal T2D patients accelerated the expression of inflammatory factors, which led to bone metabolism disorders and a higher risk of osteoporotic fractures.
Data availability statement
The original contributions presented in the study are included in the article/Supplementary Material. Further inquiries can be directed to the corresponding authors.
Ethics statement
The studies involving human participants were reviewed and approved by Ethics Committee of the Third Hospital of Hebei Medical University. The patients/participants provided their written informed consent to participate in this study.
Author contributions
PX, LG, CL and PH designed the research. LG, CL, PH, NW and XB organized the database. BW and KW performed the statistical analysis. PX, LG, CL and PH wrote the first draft of the manuscript. PX, YL and LG thoroughly revised the manuscript. All authors contributed to manuscript revision and approved the submitted version.
Funding
This study was supported by Basic Research Program for Beijing-Tianjin-Hebei Coordination (No. 19JCZDJC65500[Z]), Returned Overseas Personnel Program of Hebei Province (No. C20190355), Medical application technology program of Hebei Province (No. G2019008) and Osteoporosis Program for Young Doctors (No. GX20191107).
Acknowledgments
We appreciate all of the patients for their agreement to participate in our study.
Conflict of interest
The authors declare that the research was conducted in the absence of any commercial or financial relationships that could be construed as a potential conflict of interest.
Publisher’s note
All claims expressed in this article are solely those of the authors and do not necessarily represent those of their affiliated organizations, or those of the publisher, the editors and the reviewers. Any product that may be evaluated in this article, or claim that may be made by its manufacturer, is not guaranteed or endorsed by the publisher.
Supplementary material
The Supplementary Material for this article can be found online at: https://www.frontiersin.org/articles/10.3389/fendo.2022.1013397/full#supplementary-material
References
1. Bommer C, Heesemann E, Sagalova V, Manne-Goehler J, Atun R, Barnighausen T, et al. The global economic burden of diabetes in adults aged 20-79 years: A cost-of-Illness study. Lancet Diabetes Endocrinol (2017) 5(6):423–30. doi: 10.1016/S2213-8587(17)30097-9
2. Karim L, Rezaee T, Vaidya R. The effect of type 2 diabetes on bone biomechanics. Curr Osteoporos Rep (2019) 17(5):291–300. doi: 10.1007/s11914-019-00526-w
3. Hofbauer LC, Busse B, Eastell R, Ferrari S, Frost M, Muller R, et al. Bone fragility in diabetes: Novel concepts and clinical implications. Lancet Diabetes Endocrinol (2022) 10(3):207–20. doi: 10.1016/S2213-8587(21)00347-8
4. Martineau P, Leslie WD. The utility and limitations of using trabecular bone score with frax. Curr Opin Rheumatol (2018) 30(4):412–9. doi: 10.1097/BOR.0000000000000504
5. Rasmussen NH, Dal J. Falls and fractures in diabetes-more than bone fragility. Curr Osteoporos Rep (2019) 17(3):147–56. doi: 10.1007/s11914-019-00513-1
6. Nishimoto K, Doi T, Tsutsumimoto K, Nakakubo S, Kurita S, Kiuchi Y, et al. Relationship between diabetes status and sarcopenia in community-dwelling older adults. J Am Med Dir Assoc (2022) 23(10):1718 e7– e12. doi: 10.1016/j.jamda.2022.07.020
7. Moretti A, Palomba A, Gimigliano F, Paoletta M, Liguori S, Zanfardino F, et al. Osteosarcopenia and type 2 diabetes mellitus in post-menopausal women: A case-control study. Orthop Rev (Pavia) (2022) 14(6):38570. doi: 10.52965/001c.38570
8. Drey M, Sieber CC, Bertsch T, Bauer JM, Schmidmaier R, FiAT intervention Group. Osteosarcopenia is more than sarcopenia and osteopenia alone. Aging Clin Exp Res (2016) 28(5):895–9. doi: 10.1007/s40520-015-0494-1
9. Giangregorio LM, Leslie WD, Lix LM, Johansson H, Oden A, McCloskey E, et al. Frax underestimates fracture risk in patients with diabetes. J Bone Miner Res (2012) 27(2):301–8. doi: 10.1002/jbmr.556
10. Schacter GI, Leslie WD. Dxa-based measurements in diabetes: Can they predict fracture risk? Calcif Tissue Int (2017) 100(2):150–64. doi: 10.1007/s00223-016-0191-x
11. Hunt HB, Torres AM, Palomino PM, Marty E, Saiyed R, Cohn M, et al. Altered tissue composition, microarchitecture, and mechanical performance in cancellous bone from men with type 2 diabetes mellitus. J Bone Miner Res (2019) 34(7):1191–206. doi: 10.1002/jbmr.3711
12. Karim L, Moulton J, Van Vliet M, Velie K, Robbins A, Malekipour F, et al. Bone microarchitecture, biomechanical properties, and advanced glycation end-products in the proximal femur of adults with type 2 diabetes. Bone (2018) 114:32–9. doi: 10.1016/j.bone.2018.05.030
13. Osawa S, Katakami N, Sato I, Ninomiya H, Omori K, Yamamoto Y, et al. Skin autofluorescence is associated with vascular complications in patients with type 2 diabetes. J Diabetes Complications (2018) 32(9):839–44. doi: 10.1016/j.jdiacomp.2018.06.009
14. Hunt HB, Pearl JC, Diaz DR, King KB, Donnelly E. Bone tissue collagen maturity and mineral content increase with sustained hyperglycemia in the kk-ay murine model of type 2 diabetes. J Bone Miner Res (2018) 33(5):921–9. doi: 10.1002/jbmr.3365
15. Yang DH, Chiang TI, Chang IC, Lin FH, Wei CC, Cheng YW. Increased levels of circulating advanced glycation end-products in menopausal women with osteoporosis. Int J Med Sci (2014) 11(5):453–60. doi: 10.7150/ijms.8172
16. Piccoli A, Cannata F, Strollo R, Pedone C, Leanza G, Russo F, et al. Sclerostin regulation, microarchitecture, and advanced glycation end-products in the bone of elderly women with type 2 diabetes. J Bone Miner Res (2020) 35(12):2415–22. doi: 10.1002/jbmr.4153
17. Sornay-Rendu E, Boutroy S, Duboeuf F, Chapurlat RD. Bone microarchitecture assessed by hr-pqct as predictor of fracture risk in postmenopausal women: The ofely study. J Bone Miner Res (2017) 32(6):1243–51. doi: 10.1002/jbmr.3105
18. Hu L, Li T, Zou Y, Yin XL, Gan H. The clinical value of the Ra-adjusted fracture risk assessment tool in the fracture risk prediction of patients with type 2 diabetes mellitus in China. Int J Gen Med (2021) 14:327–33. doi: 10.2147/IJGM.S296399
19. Leslie WD, Johansson H, McCloskey EV, Harvey NC, Kanis JA, Hans D. Comparison of methods for improving fracture risk assessment in diabetes: The Manitoba bmd registry. J Bone Miner Res (2018) 33(11):1923–30. doi: 10.1002/jbmr.3538
20. Boutroy S, Bouxsein ML, Munoz F, Delmas PD. In vivo assessment of trabecular bone microarchitecture by high-resolution peripheral quantitative computed tomography. J Clin Endocrinol Metab (2005) 90(12):6508–15. doi: 10.1210/jc.2005-1258
21. Hunt HB, Miller NA, Hemmerling KJ, Koga M, Lopez KA, Taylor EA, et al. Bone tissue composition in postmenopausal women varies with glycemic control from normal glucose tolerance to type 2 diabetes mellitus. J Bone Miner Res (2021) 36(2):334–46. doi: 10.1002/jbmr.4186
22. Asokan AG, Jaganathan J, Philip R, Soman RR, Sebastian ST, Pullishery F. Evaluation of bone mineral density among type 2 diabetes mellitus patients in south karnataka. J Nat Sci Biol Med (2017) 8(1):94–8. doi: 10.4103/0976-9668.198363
23. Agius R, Galea R, Fava S. Bone mineral density and intervertebral disc height in type 2 diabetes. J Diabetes Complications (2016) 30(4):644–50. doi: 10.1016/j.jdiacomp.2016.01.021
24. Wen Y, Li H, Zhang X, Liu P, Ma J, Zhang L, et al. Correlation of osteoporosis in patients with newly diagnosed type 2 diabetes: A retrospective study in Chinese population. Front Endocrinol (Lausanne) (2021) 12:531904. doi: 10.3389/fendo.2021.531904
25. Vestergaard P. Discrepancies in bone mineral density and fracture risk in patients with type 1 and type 2 diabetes–a meta-analysis. Osteoporos Int (2007) 18(4):427–44. doi: 10.1007/s00198-006-0253-4
26. Eller-Vainicher C, Cairoli E, Grassi G, Grassi F, Catalano A, Merlotti D, et al. Pathophysiology and management of type 2 diabetes mellitus bone fragility. J Diabetes Res (2020) 2020:7608964. doi: 10.1155/2020/7608964
27. Reistetter TA, Graham JE, Deutsch A, Markello SJ, Granger CV, Ottenbacher KJ. Diabetes comorbidity and age influence rehabilitation outcomes after hip fracture. Diabetes Care (2011) 34(6):1375–7. doi: 10.2337/dc10-2220
28. Wang C, Liu J, Xiao L, Liu D, Yan W, Hu T, et al. Comparison of frax in postmenopausal Asian women with and without type 2 diabetes mellitus: A retrospective observational study. J Int Med Res (2020) 48(2): 1–14. doi: 10.1177/0300060519879591
29. Leighton PM, Little JA. Identification of coagulase-negative staphylococci isolated from urinary tract infections. Am J Clin Pathol (1986) 85(1):92–5. doi: 10.1093/ajcp/85.1.92
30. Kanis JA, Harvey NC, Johansson H, Liu E, Vandenput L, Lorentzon M, et al. A decade of frax: How has it changed the management of osteoporosis? Aging Clin Exp Res (2020) 32(2):187–96. doi: 10.1007/s40520-019-01432-y
31. Schousboe JT, Morin SN, Kline GA, Lix LM, Leslie WD. Differential risk of fracture attributable to type 2 diabetes mellitus according to skeletal site. Bone (2022) 154:116220. doi: 10.1016/j.bone.2021.116220
32. Guo Y, Wang Y, Chen F, Wang J, Wang D. Assessment of risk factors for fractures in patients with type 2 diabetes over 60 years old: A cross-sectional study from northeast China. J Diabetes Res (2020) 2020:1508258. doi: 10.1155/2020/1508258
33. Schwartz AV, Vittinghoff E, Bauer DC, Hillier TA, Strotmeyer ES, Ensrud KE, et al. Association of bmd and frax score with risk of fracture in older adults with type 2 diabetes. JAMA (2011) 305(21):2184–92. doi: 10.1001/jama.2011.715
34. Poiana C, Capatina C. Osteoporosis and fracture risk in patients with type 2 diabetes mellitus. Acta Endocrinol (Buchar) (2019) 15(2):231–6. doi: 10.4183/aeb.2019.231
35. Rianon NJ, Smith SM, Lee M, Pervin H, Musgrave P, Watt GP, et al. Glycemic control and bone turnover in older Mexican americans with type 2 diabetes. J Osteoporos (2018) 2018:7153021. doi: 10.1155/2018/7153021
36. Napoli N, Conte C, Pedone C, Strotmeyer ES, Barbour KE, Black DM, et al. Effect of insulin resistance on bmd and fracture risk in older adults. J Clin Endocrinol Metab (2019) 104(8):3303–10. doi: 10.1210/jc.2018-02539
37. Khosla S, Samakkarnthai P, Monroe DG, Farr JN. Update on the pathogenesis and treatment of skeletal fragility in type 2 diabetes mellitus. Nat Rev Endocrinol (2021) 17(11):685–97. doi: 10.1038/s41574-021-00555-5
38. Losada-Grande E, Hawley S, Soldevila B, Martinez-Laguna D, Nogues X, Diez-Perez A, et al. Insulin use and excess fracture risk in patients with type 2 diabetes: A propensity-matched cohort analysis. Sci Rep (2017) 7(1):3781. doi: 10.1038/s41598-017-03748-z
39. Lekkala S, Taylor EA, Hunt HB, Donnelly E. Effects of diabetes on bone material properties. Curr Osteoporos Rep (2019) 17(6):455–64. doi: 10.1007/s11914-019-00538-6
40. Sihota P, Yadav RN, Dhaliwal R, Bose JC, Dhiman V, Neradi D, et al. Investigation of mechanical, material, and compositional determinants of human trabecular bone quality in type 2 diabetes. J Clin Endocrinol Metab (2021) 106(5):e2271–e89. doi: 10.1210/clinem/dgab027
41. Tang SY, Zeenath U, Vashishth D. Effects of non-enzymatic glycation on cancellous bone fragility. Bone (2007) 40(4):1144–51. doi: 10.1016/j.bone.2006.12.056
42. Karim L, Bouxsein ML. Effect of type 2 diabetes-related non-enzymatic glycation on bone biomechanical properties. Bone (2016) 82:21–7. doi: 10.1016/j.bone.2015.07.028
43. de Paula FJ, Horowitz MC, Rosen CJ. Novel insights into the relationship between diabetes and osteoporosis. Diabetes Metab Res Rev (2010) 26(8):622–30. doi: 10.1002/dmrr.1135
44. Hamann C, Kirschner S, Gunther KP, Hofbauer LC. Bone, sweet bone–osteoporotic fractures in diabetes mellitus. Nat Rev Endocrinol (2012) 8(5):297–305. doi: 10.1038/nrendo.2011.233
45. Park SY, Choi KH, Jun JE, Chung HY. Effects of advanced glycation end products on differentiation and function of osteoblasts and osteoclasts. J Korean Med Sci (2021) 36(37):e239. doi: 10.3346/jkms.2021.36.e239
46. Suzuki R, Fujiwara Y, Saito M, Arakawa S, Shirakawa JI, Yamanaka M, et al. Intracellular accumulation of advanced glycation end products induces osteoblast apoptosis Via endoplasmic reticulum stress. J Bone Miner Res (2020) 35(10):1992–2003. doi: 10.1002/jbmr.4053
47. Alikhani M, Alikhani Z, Boyd C, MacLellan CM, Raptis M, Liu R, et al. Advanced glycation end products stimulate osteoblast apoptosis Via the map kinase and cytosolic apoptotic pathways. Bone (2007) 40(2):345–53. doi: 10.1016/j.bone.2006.09.011
48. Shanbhogue VV, Mitchell DM, Rosen CJ, Bouxsein ML. Type 2 diabetes and the skeleton: New insights into sweet bones. Lancet Diabetes Endocrinol (2016) 4(2):159–73. doi: 10.1016/S2213-8587(15)00283-1
49. Starup-Linde J, Hygum K, Harslof T, Langdahl B. Type 1 diabetes and bone fragility: Links and risks. Diabetes Metab Syndr Obes (2019) 12:2539–47. doi: 10.2147/DMSO.S191091
50. Burghardt AJ, Issever AS, Schwartz AV, Davis KA, Masharani U, Majumdar S, et al. High-resolution peripheral quantitative computed tomographic imaging of cortical and trabecular bone microarchitecture in patients with type 2 diabetes mellitus. J Clin Endocrinol Metab (2010) 95(11):5045–55. doi: 10.1210/jc.2010-0226
51. Heilmeier U, Joseph GB, Pasco C, Dinh N, Torabi S, Darakananda K, et al. Longitudinal evolution of bone microarchitecture and bone strength in type 2 diabetic postmenopausal women with and without history of fragility fractures-a 5-year follow-up study using high resolution peripheral quantitative computed tomography. Front Endocrinol (Lausanne) (2021) 12:599316. doi: 10.3389/fendo.2021.599316
52. Karim L, Tang SY, Sroga GE, Vashishth D. Differences in non-enzymatic glycation and collagen cross-links between human cortical and cancellous bone. Osteoporos Int (2013) 24(9):2441–7. doi: 10.1007/s00198-013-2319-4
53. Xia N, Cai Y, Wang W, Bao C, Li Y, Xie Q, et al. Association of bone-related biomarkers with femoral neck bone strength. BMC Musculoskelet Disord (2022) 23(1):482. doi: 10.1186/s12891-022-05427-1
54. Jain S, Camacho P. Use of bone turnover markers in the management of osteoporosis. Curr Opin Endocrinol Diabetes Obes (2018) 25(6):366–72. doi: 10.1097/MED.0000000000000446
55. Szulc P. Bone turnover: Biology and assessment tools. Best Pract Res Clin Endocrinol Metab (2018) 32(5):725–38. doi: 10.1016/j.beem.2018.05.003
56. An Y, Liu S, Wang W, Dong H, Zhao W, Ke J, et al. Low serum levels of bone turnover markers are associated with the presence and severity of diabetic retinopathy in patients with type 2 diabetes mellitus. J Diabetes (2021) 13(2):111–23. doi: 10.1111/1753-0407.13089
57. Wang L, Li T, Liu J, Wu X, Wang H, Li X, et al. Association between glycosylated hemoglobin A1c and bone biochemical markers in type 2 diabetic postmenopausal women: A cross-sectional study. BMC Endocr Disord (2019) 19(1):31. doi: 10.1186/s12902-019-0357-4
58. Xu L, Niu M, Yu W, Xia W, Gong F, Wang O. Associations between Fgf21, osteonectin and bone turnover markers in type 2 diabetic patients with albuminuria. J Diabetes Complications (2017) 31(3):583–8. doi: 10.1016/j.jdiacomp.2016.11.012
59. Napoli N, Chandran M, Pierroz DD, Abrahamsen B, Schwartz AV, Ferrari SL, et al. Mechanisms of diabetes mellitus-induced bone fragility. Nat Rev Endocrinol (2017) 13(4):208–19. doi: 10.1038/nrendo.2016.153
60. Yang X, Gandhi C, Rahman MM, Appleford M, Sun LW, Wang X. Age-related effects of advanced glycation end products (Ages) in bone matrix on osteoclastic resorption. Calcif Tissue Int (2015) 97(6):592–601. doi: 10.1007/s00223-015-0042-1
61. Dong XN, Qin A, Xu J, Wang X. In situ accumulation of advanced glycation endproducts (Ages) in bone matrix and its correlation with osteoclastic bone resorption. Bone (2011) 49(2):174–83. doi: 10.1016/j.bone.2011.04.009
62. Gossiel F, Paggiosi MA, Naylor KE, McCloskey EV, Walsh J, Peel N, et al. The effect of bisphosphosphonates on bone turnover and bone balance in postmenopausal women with osteoporosis: The T-score bone marker approach in the trio study. Bone (2020) 131:115158. doi: 10.1016/j.bone.2019.115158
63. Chen H, Liu W, Wu X, Gou M, Shen J, Wang H. Advanced glycation end products induced il-6 and vegf-a production and apoptosis in osteocyte-like mlo-Y4 cells by activating rage and Erk1/2, P38 and Stat3 signalling pathways. Int Immunopharmacol (2017) 52:143–9. doi: 10.1016/j.intimp.2017.09.004
64. Sakamoto E, Mihara C, Ikuta T, Inagaki Y, Kido J, Nagata T. Inhibitory effects of advanced glycation end-products and porphyromonas gingivalis lipopolysaccharide on the expression of osteoblastic markers of rat bone marrow cells in culture. J Periodontal Res (2016) 51(3):313–20. doi: 10.1111/jre.12310
65. Brownlee M. Biochemistry and molecular cell biology of diabetic complications. Nature (2001) 414(6865):813–20. doi: 10.1038/414813a
66. Brownlee M. The pathobiology of diabetic complications: A unifying mechanism. Diabetes (2005) 54(6):1615–25. doi: 10.2337/diabetes.54.6.1615
67. Otsuka E, Yamaguchi A, Hirose S, Hagiwara H. Characterization of osteoblastic differentiation of stromal cell line St2 that is induced by ascorbic acid. Am J Physiol (1999) 277(1):C132–8. doi: 10.1152/ajpcell.1999.277.1.C132
68. Yamaguchi A, Ishizuya T, Kintou N, Wada Y, Katagiri T, Wozney JM, et al. Effects of bmp-2, bmp-4, and bmp-6 on osteoblastic differentiation of bone marrow-derived stromal cell lines, St2 and Mc3t3-G2/Pa6. Biochem Biophys Res Commun (1996) 220(2):366–71. doi: 10.1006/bbrc.1996.0411
69. Okazaki K, Yamaguchi T, Tanaka K, Notsu M, Ogawa N, Yano S, et al. Advanced glycation end products (Ages), but not high glucose, inhibit the osteoblastic differentiation of mouse stromal St2 cells through the suppression of osterix expression, and inhibit cell growth and increasing cell apoptosis. Calcif Tissue Int (2012) 91(4):286–96. doi: 10.1007/s00223-012-9641-2
Keywords: type 2 diabetes mellitus, osteoporosis, fracture risk, FRAX, advanced glycation end products
Citation: Gao L, Liu C, Hu P, Wang N, Bao X, Wang B, Wang K, Li Y and Xue P (2022) The role of advanced glycation end products in fracture risk assessment in postmenopausal type 2 diabetic patients. Front. Endocrinol. 13:1013397. doi: 10.3389/fendo.2022.1013397
Received: 07 August 2022; Accepted: 29 November 2022;
Published: 12 December 2022.
Edited by:
Zhi-Feng Sheng, Central South University, ChinaReviewed by:
Kamyar Asadipooya, University of Kentucky, United StatesAntimo Moretti, University of Campania Luigi Vanvitelli, Italy
Copyright © 2022 Gao, Liu, Hu, Wang, Bao, Wang, Wang, Li and Xue. This is an open-access article distributed under the terms of the Creative Commons Attribution License (CC BY). The use, distribution or reproduction in other forums is permitted, provided the original author(s) and the copyright owner(s) are credited and that the original publication in this journal is cited, in accordance with accepted academic practice. No use, distribution or reproduction is permitted which does not comply with these terms.
*Correspondence: Peng Xue, hebmuxuepeng@163.com; Yukun Li, lykun1962@163.com
†These authors share first authorship