- 1Department of Epidemiology, School of Public Health, University of São Paulo, São Paulo, Brazil
- 2Center of Clinical and Epidemiological Research at University of São Paulo, São Paulo, Brazil
- 3Department of Preventive Medicine, Federal University of São Paulo, São Paulo, Brazil
- 4Department of Internal Medicine, Medical School, University of São Paulo, São Paulo, Brazil
Background: Adverse intrauterine environment—reflected by low birth weight (LBW)—has been linked to insulin resistance and type 2 diabetes later in life. Whether β-cell function reduction and insulin resistance could be detected even in middle-aged adults without overt diabetes is less investigated. We examined the association of LBW with β-cell function and insulin sensitivity in non-diabetic middle-aged adults from the Brazilian Longitudinal Study of Adult Health (ELSA-Brasil).
Methods: This is a cross-sectional analysis of 2,634 ELSA-Brasil participants aged between 34 and 59 years, without diabetes. Participants were stratified according to LBW defined as <2.5 kg and their clinical data were compared. HOMA-IR, HOMA-β, HOMA-adiponectin, TyG index, QUICKI and TG/HDL were calculated and their association with LBW were tested using multiple linear regression including adjustments suggested by Directed Acyclic Graphs and propensity score matching was applied.
Results: The sample (47.4 ± 6.3 years) was composed of 57.5% of women and 9% had LBW. Subjects with LBW and normal-weight reported similar BMI values at the age of 20 years and current BMI was slightly lower in the LBW group. In average, cardiometabolic risk profile and also indexes of β-cell function and insulin sensitivity were within normal ranges. In regression analysis, log-transformed HOMA-β—but not with the other indexes—was associated with LBW (p = 0.014) independent of sex, skin color, prematurity, and family history of diabetes. After applying propensity-score matching in a well-balanced sample, HOMA-AD and TG/HDL indexes were associated with LBW.
Conclusion: The association between LBW and insulin sensitivity markers may occur in healthy middle-aged adults before overt glucose metabolism disturbances. Our data are coherent with the detection of early life events consequent with insulin resistance markers that could contribute to the risk of glucose metabolism disturbances.
Introduction
Diabetes mellitus remains one of the most relevant public health concerns worldwide due to its micro and macrovascular complications (1). The etiology of type 2 diabetes mellitus (T2DM) is multifactorial, involving genetic, environmental, and lifestyle factors (2) and is commonly accompanied by excess body adiposity.
Based on the Developmental Origins of Health and Disease (DOHaD) theory, cardiometabolic disorders in adulthood might have their origins early in life stemming from intrauterine insults, namely, maternal and fetal undernutrition (3), maternal smoking, alcohol consumption or health conditions in perinatal life (4). As an adaptation to survive under adverse gestational conditions, fetal programming occurs, resulting in structural and functional changes in body organs and systems (5, 6).
Low birth weight (LBW), a proxy of intrauterine adversity, has been associated with adult-onset diseases, namely, obesity, T2DM and the metabolic syndrome (7, 8). It has been reported that LBW is associated with decreased β-cell mass and reduced function, resulting in a low insulin response to glucose levels (9, 10). Additional underlying mechanisms have been related to evidence of decreased insulin sensitivity in the genesis of glucose metabolism disturbance, concomitant with progressive β-cell dysfunction during adulthood (11, 12). Studies show that perinatal stress may affect insulin action in peripheral organs with reduced glucose uptake, and decreased expression of GLUT4 glucose transport by muscle and adipose cells (13–15). This condition becomes particularly worrisome considering the tendency of weight gain associated with our current environment and lifestyle. In this context, greater awareness of glucose metabolism abnormalities is important for early identification of risk later in adult life.
A number of studies have associated LBW with T2DM (16, 17), although identifying an association of birth weight with impaired insulin sensitivity and β-cell function before the onset of diabetes, the focus of interest of the present study, would be more opportune for preventive measures.
The Brazilian Longitudinal Study of Adult Health (ELSA-Brasil) is a large cohort study of adult health in Brazil, designed to investigate risk factors associated with diabetes and cardiovascular disease (18, 19). Therefore, the ELSA-Brasil represents an opportunity to investigate associations of early life events with outcomes in adulthood. The present study examined the association of LBW with parameters of β-cell function and insulin sensitivity in non-diabetic participants of the ELSA-Brasil.
Methods
Study Design and Population
A cross-sectional analysis was carried out of baseline data from the multicenter ELSA-Brasil study, whose methodological details have been reported elsewhere (18, 19). The baseline assessment was conducted from August 2008 to December 2010 and included 15,105 employees aged 35–74 years from six Brazilian universities and research institutions. The present analysis drew on the baseline data of 5,061 participants of both sexes, aged 35–74 years from the São Paulo center. The study was approved by the local Ethics Committee and informed consent was obtained from all participants.
Eligibility Criteria
To be eligible for the present study, participants had to be aged <60 years (to reduce recall bias), non-diabetic and have preserved renal function. Of the 5,061 participants, the following subjects were excluded: 1,036 with diabetes (self-reported or in use of antidiabetic medications or newly diagnosed), 210 with glomerular filtration rate <60 mL/min/1.73 m2 or macroproteinuria, and 623 aged ≥60 years (20). Thirty-nine participants were subsequently excluded for being underweight (BMI <18.5 kg/m2) and 186 because they were born with macrosomia (birth weight >4.0 kg). A further 333 participants were excluded for missing data on exposure (birth weight) or outcome (plasma glucose, insulin and lipids) variables. Therefore, a total of 2,634 participants were included in the present study (Figure 1).
Clinical and Laboratory Data
Participants were interviewed using standardized questionnaires (21). Self-reported data regarding demographics, socioeconomic status and health conditions were obtained. Variables of interest were age (years), sex (male, female), skin color (black, white, brown, yellow or indigenous, further stratified into white and non-white categories), family history of diabetes and hypertension (yes or no) and maternal educational level of participant.
Prematurity (yes or no) and birth weight (kg) were self-reported when possible. Birth weight was also categorized into “<2.5 kg”, “2.5–4.0 kg”, “>4.0 kg” or “unknown”. All participants were also asked to provide their body weight at 20 years of age.
Weight and height were measured and body mass index (BMI) then calculated as weight in kilograms divided by height in meters squared to express nutritional status. Waist circumference was measured at the midpoint between the last rib and the iliac crest using an inelastic tape. Blood pressure was measured using an Omron HEM 705CPINT device (Omron Co, Kyoto, Japan) after a 5-minute rest in a sitting position. Three measurements were taken at 1-min intervals and mean values calculated. After overnight fasting, blood samples were collected and participants then underwent a 2-hour 75 g oral glucose tolerance test. Fasting and 2-hour plasma glucose and insulin were determined. Aliquots were frozen at −80°C for further determinations (22, 23).
Plasma glucose was measured by the hexokinase method (ADVIA Chemistry; Siemens, Deerfield, Illinois, USA), and glycated hemoglobin determined by high-pressure liquid chromatography (Bio-Rad Laboratories, Hercules, California, USA) according to the National Glycohemoglobin Standardization Program certified method. Insulin (Siemens, Tarrytown, USA) and adiponectin (Enzo Life Sciences, Farmingdale, NY, USA) were determined using enzyme-linked immunoenzymatic assays.
The HOMA-β and HOMA-IR indexes were used to assess β-cell function and insulin sensitivity, respectively, and were calculated using the equations:
Additionally, insulin sensitivity was evaluated by HOMA-adiponectin (HOMA-AD), the Triglycerides–glucose index (TyG index), QUICKI (Quantitative Insulin Sensitivity Check Index) and the Triglyceride-to-HDL-c ratio (TG/HDL-c), using the following equations: HOMA-AD = fasting glucose (mmol/L) × fasting insulin (mU/L)/22.5 × adiponectin (µg/ml); TyG index = log [(fasting triglycerides (mg/dl) × fasting glucose (mg/dl)]/2; QUICKI = 1/(log insulin (µUI/ml) + (log fasting glucose (mg/dl) and TG/HDL-c.
Adiponectin was measured in a sub-sample of 1,000 participants. After applying exclusion criteria, 742 participants were included with available adiponectin data.
Total cholesterol was assessed using the enzymatic colorimetric method (ADVIA Chemistry; Siemens, Deerfield, Illinois, USA). HDL-c was determined by the homogeneous colorimetric method without precipitation, and triglycerides by the glycerophosphate peroxidase method according to the Trinder assay (ADVIA Chemistry; Siemens, Deerfield, Illinois, USA). LDL-c concentrations were calculated using the Friedewald equation.
Definitions for Analyses
Birth weight (exposure variable) was classified into three categories: low birth weight (<2.5 kg), normal birth weight (2.5–4.0 kg) and macrosomia (>4.0 kg). Prematurity was defined by an affirmative answer to the question: “Were you a premature baby, in other words, were you born earlier than expected?”. Outcomes were HOMA-β, HOMA-IR, HOMA-AD, TyG index, QUICKI and TG/HDL-c, analyzed as continuous variables.
Nutritional status was classified into underweight (BMI <18.5 kg/m2), normal weight (18.5–24.9 kg/m2), overweight (25.0–29.9 kg/m2) and obesity (>30.0 kg/m2). Hypertension was diagnosed when systolic or diastolic blood pressure levels were ≥140 or 90 mmHg, respectively, or when participant was in use of antihypertensive drugs.
Diabetes was diagnosed according to the American Diabetes Association criteria (24), as follows: fasting plasma glucose ≥126 mg/dl or 2-hour post challenge >200 mg/dl or glycated hemoglobin ≥6.5%. Prediabetes (yes or no) was defined as fasting plasma glucose between 100 and 125 mg/dl or 2-hour post challenge between 140 and 199 mg/dl or glycated hemoglobin 5.7–6.4%.
Statistical Analysis
Distribution normality was tested for continuous variables and those with non-normal distribution (HOMA-β, HOMA-IR, HOMA-AD, QUICKI, and TG/HDL-c) were log-transformed before analysis to achieve normality. Continuous variables with a normal distribution were expressed as mean ± standard deviation (SD) and compared using Student´s t-test. Non-normally distributed variables were expressed as median and interquartile range and compared using the Wilcoxon rank test. Categorical variables were expressed as absolute and relative frequencies and compared by the chi-squared test.
Associations of exposure (LBW) and outcome (HOMA-β, HOMA-IR, HOMA-AD, TyG index, QUICKI, and TG/HDL-c) variables were initially analyzed by simple linear regression. Directed Acyclic Graphs (DAG) were used to build theoretical models and analyze independent associations of exposure with outcomes in multiple linear regression analyses. The DAG is a causal diagram which allows scientific evidence regarding the relationships among variables to be incorporated in graphics software to reach the ideal set of covariables (minimum sufficient adjustment) for the model to prevent biases and overadjustments (25, 26). Figures were created by DAGitty software, version 3.0 (www.dagitty.net) included in the Supplementary Material (Figures S1A, B).
Based on the DAGs, the association of LBW with HOMA-β and parameters of insulin sensitivity were adjusted for sex, skin color, family history of diabetes, and prematurity.
Considering the difference in sample size between groups with normal birth weight and LBW, and potential selection bias due to the nature of the study, propensity score matching was employed to create more comparable groups (27, 28). The nearest neighbor-matching algorithm within a caliper of 0.1 SD of logit function of propensity score was used. First, for the propensity score matching, a multiple logistic regression model was used, adjusted for DAG-based covariates (sex, skin color, family history of diabetes, and prematurity), and the probability of each participant having LBW versus normal birth weight was estimated. Balance between the groups was assessed by comparing each covariate. When standardized mean difference fell in the −0.1 to 0.1 range, groups were considered balanced. This matching reduced all covariate imbalance in the sample. The matched sample was then submitted to multiple linear regression in order to analyze associations of LBW (exposure as independent variable) with β-cell function and insulin sensitivity markers (outcomes as dependent variables) adjusted for the same DAG-based covariates. Tests were performed using “MatchIt”, “rbounds”, “Matching”, “twang” and “survey” packages in the R statistical environment.
All analyses were performed using the R Project for Statistical Computing software (R version 3.5.2) and statistical significance was set at a p-value of 0.05.
Results
For the study sample of 2,634 participants, mean age was 47.4 ± 6.3 years, 57.5% were women and 59.3% reported white skin color. In general, the cardiometabolic parameters of the sample were within normal ranges (systolic and diastolic blood pressures of 116.6 ± 14.9 and 74.2 ± 10.4 mmHg, respectively), except for overweight (26.8 ± 4.6 kg/m2) and prediabetic (plasma glucose of 102.0 ± 7.7 mg/dl) status.
A total of 238 (9.0%) participants reported LBW and 145 (5.5%) were born preterm. LBW participants were predominantly women (61.7%), had white skin color (52.2%), and reported low maternal educational level (62.0%).
Participants with LBW had a higher rate of low educational level compared to those reporting normal birth weight (16.1% versus 10.8%, p = 0.007). LBW and normal-weight groups reported similar BMI values at the age of 20 years and current BMI was slightly lower in the LBW group, with borderline significance (p = 0.075, Table 1). Mean values of waist circumference were higher in the normal birth weight than the LBW group (88.2 ± 11.6 versus 86.0 ± 11.7 cm, p = 0.008), but both values were, on average, within normal ranges. Blood pressure levels and lipid metabolism variables were similar for the two groups. No differences in beta-cell secretion and insulin sensitivity indexes were found between the groups.
On multiple linear regression analyses, associations of LBW with markers of beta-cell function and insulin sensitivity were tested. LBW was associated with log-transformed HOMA-β values (p = 0.014), but not with the other indexes of insulin sensitivity (Table 2).
Propensity-Score Matching—Variable Balance
Initially, the sample contained 238 participants with LBW. After applying the propensity-score matching, the final samples included 227 matched participants for HOMA-β, HOMA-IR, TyG, QUICKI and TG/HDL analysis and 64 matched participants for HOMA-AD analysis. The matching approach made all covariates appropriately balanced (standardized mean difference of between −0.1 and 0.1) for further analyses. Variable balance was compared before and after matching to assess the improvement of pairing (Supplementary Table S1).
Associations of LBW With β-Cell Function
After propensity-score matching, the multiple linear regression model, adjusted for sex, skin color, family history of diabetes and prematurity, showed no association between LBW and HOMA-β (ß 0.003, 95%CI −0.038–0.045 p = 0.107) (Table 3).
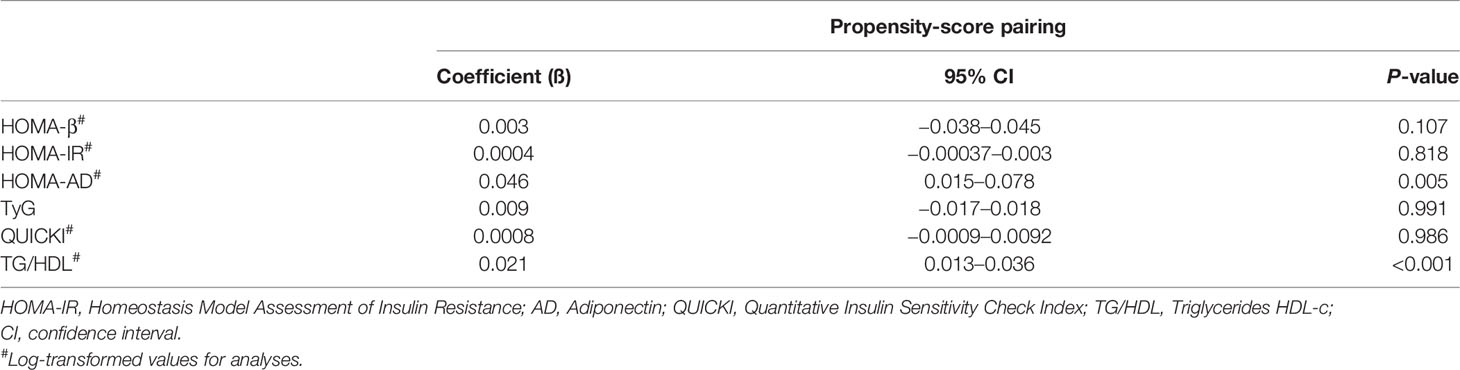
Table 3 Estimates of associations of LBW with parameters of β-cell function and insulin sensitivity after propensity-score matching in ELSA-Brasil participants.
Associations of LBW With Insulin Sensitivity
The fully adjusted multiple linear regression model showed that being born with LBW was directly associated with HOMA-AD (ß 0.046, 95% CI 0.015–0.078, p = 0.005) and TG/HDL index (ß 0.021, 95% CI 0.013–0.036, p <0.001). There was no association of LBW with HOMA-IR, TyG or QUICKI (Table 3).
Discussion
We found evidence further supporting the hypothesis that LBW is associated with decreased β-cell function and with insulin resistance in middle-aged non-diabetic participants from the ELSA-Brasil (18, 19). The study findings are strengthened by the facts that several indexes of insulin secretion and sensitivity were used and DAG applied for adjustments and propensity-score matching analysis. An association was found of LBW with HOMA-AD and TG/HDL indexes, after adjustment and propensity-score matching. No association between low birth weight and HOMA-β was found. The results reinforced the possible role of early life events in insulin sensitivity, even with marker values within the normal range in adults born with LBW.
Considering the magnitude of T2DM as a public health concern, causing morbidity and mortality worldwide (29, 30), initiatives to improve prediction and prevention are timely. The present study was prompted by evidence that population-attributable risk of T2DM is associated with increased mortality in adults born with LBW compared to those with normal birth weight (16). Additionally, LBW has been associated with hyperinsulinemia and increased risk of diabetes later in childhood (31, 32). We hypothesized that these abnormalities can affect pancreatic function during the life course, justifying the assessment of beta cell secretion capacity, and peripheral insulin sensitivity before glucose metabolism disturbances emerged. In this context, our study evaluated traditional and novel indexes of β-cell function and insulin sensitivity/resistance.
HOMA-β and HOMA-IR are the most common indexes for estimating insulin secretion and resistance (33). In the present study, HOMA-AD was also calculated to assess insulin sensitivity. This index is a modified version of HOMA-β which incorporates the total serum adiponectin level in the denominator to indirectly adjust to degree of body adiposity. Adiponectin is a protein involved in the pathophysiology of obesity and low levels tend to be observed in obese individuals with ectopic adipose tissue deposition (34). Hypoadiponectinemia has been considered an independent risk factor for the development of T2DM (35). To the best of our knowledge, the present study is the first to assess insulin sensitivity in overweight adults with LBW. HOMA-AD has been evaluated in the pediatric population, individuals with chronic kidney disease and chronic liver disease (36–38). We also calculated TG/HDL ratio, an alternative, low-cost, useful index for clinical practice. These lipid parameters are typically altered in individuals with the metabolic syndrome, in which insulin resistance is the main pathophysiological event. Both HOMA-AD and TG/HDL were associated with LBW in the well-balanced sample after applying propensity-score matching. Considering that most people are born with normal birth weight, as was the case in the present sample, this analysis was valid for improving the reliability of comparisons of subgroups stratified according to birth weight. Several studies have shown that HOMA-AD offers greater accuracy than HOMA-IR for assessing insulin resistance in overweight non-diabetic individuals (39, 40). Given that diabetic individuals were excluded from the study sample, the findings regarding the HOMA-AD and TG/HDL indexes suggest their utility for early detection of insulin resistance in middle-aged adults.
In the natural history of T2DM, insulin resistance precedes the decline of β cell function and is associated with ectopic fat deposition in the liver, muscles and pancreas (41). In turn, weight loss can prevent this condition by improving insulin sensitivity. Our results are consistent with insulin resistance preceding β-cell dysfunction in overweight adults who have not developed a glucose metabolism disturbance. LBW was initially associated with HOMA-β on multiple linear regression. However, after applying propensity-score matching in a well-balanced sample, including for the adiposity parameter (BMI), this association no longer persisted. Therefore, these results revealed an association of LBW with insulin sensitivity markers in middle-aged adults, where an association with HOMA-β can be expected in the long term if a weight loss intervention is not pursued. To our knowledge, no previous studies have reported the use of HOMA-AD and TG/HDL indexes as early markers of insulin sensitivity in adults who still have preserved β-cell function.
Explanations for these findings are based on the reported associations of LBW and glucose metabolism dysfunction, and particularly when these infants also experience catch-up growth in childhood. These individuals are prone to developing obesity, increased visceral adiposity and insulin resistance (42, 43). An elevated number of insulin receptors in their adipocytes and abnormal signaling by phosphorylation of insulin-receptor substrate 1 may result in an anti-lipolysis state (44, 45). Also, it has been shown that each tertile decrease in birth weight was associated with a 1.72 times greater risk of insulin resistance in adults (46). Concordantly, our data favor the hypothesis that once insulin resistance is installed, insulin production will increase and can progress to β-cell failure over time. Other studies support the possibility that decreased β-*cell function can occur without insulin resistance. This was observed in individuals with intrauterine growth restriction who had a marked reduction in number of β cells (47–49). Another study confirmed that adults born with LBW had a 30% reduction in insulin secretion (10). Animal models involving intrauterine energy restriction showed similar results, with a reduction in β cell mass of up to 35% (48). Despite uncertainties over the underlying mechanisms, the present results support the occurrence of early onset of insulin resistance in adults without diabetes.
Other indexes could have been useful to assess β cell function such as the OGIS (50) and Matsuda index (51) that require several determinations of plasma glucose and insulin during glucose tolerance tests. More recently, insulin clearance was raised as an important aspect of glucose metabolism and its impairment has been related to the risk of developing T2DM (52–54). Although this method would enhance the β cell function evaluation, measurements for its estimation were not available in the ELSA-Brasil.
The present study has limitations related to recall bias, given that retrospective data were collected regarding early life events. This bias can be reduced by using a sample of middle-aged participants, under 60 years of age. Some studies have shown that perinatal-related events are reliably reported during adult life (55–57). We also use the exposure (LBW) as a categorical variable, possibly reducing the statistical power of the analysis. This approach was chosen to minimize information inaccuracy from participants who were unable to accurately recall their birth weight in kilograms. The use of the propensity-score method decreased the sample size, limiting the ability to find valid associations. Therefore, future studies investigating the association between LBW and HOMA-β and other indexes in larger samples are needed. A cross-sectional analysis of the ELSA-Brasil data was conducted. Further analyses of the follow-up of the sample can allow causality between LBW and the occurrence of glucose metabolism disturbances to be explored.
A strength of this study was the methodological approach employed, including the Directed Acyclic Graph method to identify confounding variables, avoiding over adjustments in the regression models constructed (25, 26). Although a variety of covariates were controlled for, other exposures which occurred during the life course of participants were not included. However, we collected body weight at age 20 in an attempt to define participant body weight trajectory. Another strength was the use of propensity-score matching to reduce potential selection confounders seen in observational studies (27, 28), achieving sufficiently balanced groups in the analysis. Furthermore, the frequency of self-reported LBW found in the sample was comparable to that reported in the Brazilian population at large (58).
In conclusion, LBW was found to be associated with insulin sensitivity markers in adulthood before overt glucose metabolism disturbances emerged. HOMA-AD and TG/HDL indexes appeared to be useful for detecting insulin resistance in overweight adults who had LBW. These findings are relevant in reinforcing the hypothesis that early life events affect glucose metabolism during the life course. Thus, identifying the subset of individuals at risk may be important to allow early implementation of preventive measures.
Data Availability Statement
The original contributions presented in the study are included in the article/Supplementary Material. Further inquiries can be directed to the corresponding author.
Ethics Statement
The study was approved by the National Commission on Ethics Research (CONEP) and the local ethics committee, the Research Ethics Committee (CEP) under registration number 76 of the University of São Paulo (HU-USP). The patients/participants provided their written informed consent to participate in this study.
Author Contributions
Study design, analysis, interpretation and preparation of the manuscript: JB, BA-P and SF. Acquisition of data and critical revision for the manuscript content: PL and IB. All authors listed have made a substantial, direct, and intellectual contribution to the work and approved it for publication.
Funding
The current study was supported by a grant from the São Paulo Research Foundation (Fundação de Amparo à Pesquisa do Estado de São Paulo—FAPESP—Protocol 2009/15041-9). Also it was financed in part by the Coordenação de Aperfeiçoamento de Pessoal de Nível Superior—Brasil (CAPES)—(Finance Code 001). The baseline ELSA-Brasil study was supported by the Brazilian Ministry of Health (Science and Technology Department), the Brazilian Ministry of Science and Technology, and CNPq-National Research Council (# 01 06 0010.00 RS, 01 06 0212.00 BA, 01 06 0300.00 ES, 01 06 0278.00 MG, 01 06 0115.00 SP, 01 06 0071.00 RJ).
Conflict of Interest
The authors declare that the research was conducted in the absence of any commercial or financial relationships that could be construed as a potential conflict of interest.
Publisher’s Note
All claims expressed in this article are solely those of the authors and do not necessarily represent those of their affiliated organizations, or those of the publisher, the editors and the reviewers. Any product that may be evaluated in this article, or claim that may be made by its manufacturer, is not guaranteed or endorsed by the publisher.
Acknowledgments
The authors would like to acknowledge the participation of the 5,061 individuals recruited for this study, without them this study and those based on the ELSA-Brasil cohort would not have been possible.
Supplementary Material
The Supplementary Material for this article can be found online at: https://www.frontiersin.org/articles/10.3389/fendo.2022.842233/full#supplementary-material
References
1. Zimmet P, Alberti KG, Shaw J. Global and Societal Implications of the Diabetes Epidemic. Nature (2001) 414(6865):782–7. doi: 10.1038/414782a
2. Zheng Y, Ley SH, Hu FB. Global Aetiology and Epidemiology of Type 2 Diabetes Mellitus and its Complications. Nat Rev Endocrinol (2018) 14(2):88–98. doi: 10.1038/nrendo.2017.151
3. Jaddoe WV, Witterman JCM. Hypotheses on the Fetal Origins of Adult Diseases: Contributions of Epidemiological Studies. Eur J Epidemiol (2006) 21:91–102. doi: 10.1007/s10654-005-5924-5
4. Alexander BT, Dasinger JH, Intapad S. Fetal Programming and Cardiovascular Pathology. Compr Physiol (2015) 5(2):997–1025. doi: 10.1002/cphy.c140036
5. Barker DJ, Fall CH. Fetal and Infant Origins of Cardiovascular Disease. Arch Dis Child (1993) 68(6):797–9. doi: 10.1136/adc.68.6.797
6. Calkins K, Devaskar SU. Fetal Origins of Adult Disease. Curr Probl Pediatr Adolesc Health Care (2011) 41(6):158–76. doi: 10.1016/j.cppeds.2011.01.001
7. Pilgaard K, Faerch K, Carstensen B, Poulsen P, Pisinger C, Pedersen O, et al. Lowbirth Weight and Premature Birth Are Both Associated With Type 2 Diabetes in a Random Sample of Middle-Aged Danes. Diabetologia (2010) 53(12):2526–30. doi: 10.1007/s00125-010-1917-3
8. Jornayvaz FR, Vollenweider P, Bochud M, Mooser V, Waeber G, Marques-Vidal P. Low Birth Weight Leads to Obesity, Diabetes and Increased Leptin Levels in Adults: The CoLaus Study. Cardiovasc Diabetol (2016) 15:73. doi: 10.1186/s12933-016-0389-2
9. Kaijser M, Bonamy AKE, Akre O, Cnattingius S, Granath F, Norman M, et al. Perinatal Risk Factors for Diabetes in Later Life. Diabetes (2009) 58(3):523–6. doi: 10.2337/db08-0558
10. Jensen CB, Storgaard H, Dela F, Holst JJ, Madsbad S, Vaag AA. Early Differential Defects of Insulin Secretion and Action in 19-Year-Old Caucasian Men Who had Low Birth Weight. Diabetes (2002) 51(4):1271–80. doi: 10.2337/diabetes.51.4.1271
11. Lithell HO, McKeigue, Berglund L, Mohsen R, Lithell UB, Leon DA. Relation of Size at Birth to Non-Insulin Dependent Diabetes and Insulin Concentrations in Men Aged 50-60years. BMJ (1996) 312(7028):406–10. doi: 10.1136/bmj.312.7028.406
12. Barker DJ. The Developmental Origins of Insulin Resistance. Horm Res (2005) 64 Suppl 3:2–7. doi: 10.1159/000089311
13. Dunlop K, Cedrone M, Staples JF, Regnault TRH. Altered Fetal Skeletal Muscle Nutrient Metabolism Following and Adverse In Utero Environment and the Modulation of Later Life Insulin Sensitivity. Nutrients (2015) 7(2):1202–16. doi: 10.3390/nu7021202
14. Thamotharan M, Shin BC, Suddirikku DT, Thamothatan S, Garg M, Devaskar SU. GLUT4 Expression and Subcellular Localization in the Intrauterine Growth-Restricted Adult Rat Female Offspring. Am J Physiol Endocrinol Metab (2005) 288(5):E935–947. doi: 10.1152/ajpendo.00342.2004
15. Barker DJP. The Developmental Origins of Adult Disease. J Am Coll Nutr (2004) 23(6 Suppl):588S–95S. doi: 10.1080/07315724.2004.10719428
16. Leibson CL, Burke JP, Ransom JE, Forsgren J, Melton 3J, Bailey KR, et al. Relative Risk of Mortality Associated With Diabetes as a Function of Birth Weight. Diabetes Care (2005) 28(12):2839–43. doi: 10.2337/diacare.28.12.2839
17. Whincup PH, Kaye SJ, Owen CG, Huxley R, Cook DG, Anazawa S, et al. Birth Weight and Risk of Type 2 Diabetes: A Systematic Review. JAMA (2008) 300(24):2886–97. doi: 10.1001/jama.2008.886
18. Schmidt MI, Duncan BB, Mill JG, Lotufo PA, Chor D, Barreto SM, et al. Cohort Profile: Longitudinal Study of Adult Health (ELSA-Brasil). Int J Epidemiol (2015) 44(1):68–75. doi: 10.1093/ije/dyu027
19. Aquino EM, Barreto SM, Bensenor IM, Carvalho MS, Chor D, Duncan BB, et al. Brazilian Longitudinal Study of Adult Health (ELSA-Brasil): Objectives and Design. Am J Epidemiol (2012) 175(4):315–24. doi: 10.1093/aje/kwr294
20. Kidney Disease Improving Global Outcomes - KDIGO. Clinical Practice Guideline for the Evaluation and Management of Chronic Kidney Disease. Official Journal of the International Society of Nephrology. Kidney Int Suppl (2013) 3(1):7–10. doi: 10.1038/kisup.2012.77
21. Bensenor IM, Griep RH, Pinto KA, Faria CP, Felisbino-Mendes M, Caetano EI, et al. Routines of Organization of Clinical Test and Interviews in the ELSA-Brasil Investigation Center. Rev Saude Publica (2013) 47 Suppl 2:37–47. doi: 10.1590/S0034-8910.2013047003780
22. Fedeli LG, Vidigal PG, Leite CM, Castilhos CD, Pimentel RA, Maniero VC, et al. Logistics of Collection and Transportation of Biological Samples and the Organization of the Central Laboratory in the ELSA-Brasil. Rev Saude Publica (2013) 47 Suppl 2:63–71. doi: 10.1590/S0034-8910.2013047003807
23. Pereira AC, Bensenor IM, Fedeli LM, Castilhos C, Vidigal PG, Maniero V, et al. Design and Implementation of the ELSA-Brasil Biobank: A Prospective Study in a Brazilian Population. Rev Saude Publica (2013) 47 Suppl 2:72–8. doi: 10.1590/S0034-8910.2013047003822
24. American Diabetes Association. Classification and Diagnosis of Diabetes. Diabetes Care (2015) 38(Suppl 1):S8–S16. doi: 10.2337/dc15-S005
25. Hernán MA, Robins JM. Instruments for Causal Inference: An Epidemiologist’s Dream? Epidemiology (2006) 17(4):360–72. doi: 10.1097/01.ede.0000222409.00878.37
26. Greenland S, Pearl J, Robins JM. Causal Diagrams for Epidemiologic Research. Epidemiology (1999) 10(1):37–48. doi: 10.1097/00001648-199901000-00008
27. Austin PC. An Introduction to Propensity Score Methods for Reducing the Effects of Confounding in Observational Studies. Multivariate Behav Res (2011) 46(3):399–424. doi: 10.1080/00273171.2011.568786
28. Haukoos JS, Lewis RJ. The Propensity Score. JAMA (2015) 314(15):1637–8. doi: 10.1001/jama.2015.13480
29. Meigs JB, Rutter MK, Sullivan LM, Fox CS, D’Agostino RB, Wilson PW. Impact of Insulin Resistance on Risk of Type 2 Diabetes and Cardiovascular Disease in People With Metabolic Syndrome. Diabetes Care (2007) 30(5):1219–25. doi: 10.2337/dc06-2484
30. Wild S, Roglic G, Green A, Sicree R, King H. Global Prevalence of Diabetes. Estimates for the Year 2000 and Projections for 2030. Diabetes Care (2004) 27:1047–53. doi: 10.2337/diacare.27.5.1047
31. Giapros V, Vavva E, Siomou E, Kolios G, Tsabouri S, Cholevas V, et al. Low-Birth-Weight, But Not Catch-Up Growth, Correlates With Insulin Resistance and Resistin Level in SGA Infants at 12 Months. J Matern Fetal Neonat Med (2017) 30(15):1771–6. doi: 10.1080/14767058.2016.1224838
32. Wang G, Divall S, Radovick S, Paige D, Ning Y, Chen Z, et al. Preterm Birth and Random Plasma Insulin Levels at Birth and in Early Childhood. JAMA (2014) 311(6):587–296. doi: 10.1001/jama.2014.1
33. Matthews DR, Hosker JP, Rudenski AS, Naylor BA, Treacher DF, Turner RC. Homeostasis Model Assessment: Insulin Resistance and Beta-Cell Function From Fasting Plasma Glucose and Insulin Concentrations in Man. Diabetologia (1985) 28(7):412–9. doi: 10.1007/BF00280883
34. Bays HE. “Sick Fat”, Metabolic Disease, and Atherosclerosis. Am J Med (2009) 122(1 Suppl):S26–37. doi: 10.1016/j.amjmed.2008.10.015
35. Pittas AG, Joseph N, Greenberg AS. Adipocytokines and Insulin Resistance. J Clin Endocrinol Metab (2004) 89(2):447–52. doi: 10.1210/jc.2003-031005
36. Makni E, Moalla W, Lac G, Aouichaoui C, Cannon D, Elloumi M, et al. The Homeostasis Model Assessment-Adiponectin (HOMA-AD) Is the Most Sensitive Predictor of Insulin Resistance in Obese Children. Ann Endocrinol (Paris) (2012) 73(1):26–33. doi: 10.1016/j.ando.2011.12.002
37. Hung AM, Sundell MB, Egbert P, Siew ED, Shintani A, Ellis CD, et al. A Comparison of Novel and Commonly-Used Indices of Insulin Sensitivity in African American Chronic Hemodialysis Patients. Clin J Am Soc Nephrol (2011) 6(4):767–74. doi: 10.2215/CJN.08070910
38. Michalczuk MT, Kappel CR, Birkhan O, Bragança AC, Alvares-da-Silva MR. HOMA-AD in Assessing Insulin Resistance in Lean Noncirrhotic HCV Outpatients. Int J Hepatol (2012) 2012:576584. doi: 10.1155/2012/576584
39. McLaughlin T, Abbasi F, Cheal K, Chu J, Lamendola C, Reaven G. Use of Metabolic Markers to Identify Overweight Individuals Who Are Insulin Resistant. Ann Intern Med (2003) 139(10):802–9. doi: 10.7326/0003-4819-139-10-200311180-00007
40. Hannon TS, Bacha F, Lee SJ, Janosky J, Arslanian SA. Use of Markers of Dyslipidemia to Identify Overweight Youth With Insulin Resistance. Pediatr Diabetes (2006) 7(5):260–6. doi: 10.1111/j.1399-5448.2006.00199.x
41. Skyler JS, Bakris GL, Bonifacio E, Darsow T, Eckel RH, Groop L, et al. Differentiation of Diabetes by Pathophysiology, Natural History, and Prognosis. Diabetes (2017) 66:241–55. doi: 10.2337/db16-0806
42. Law CM, Shiell AW, Newsome CA, Syddall HE, Shinebourne EA, Fayers PM, et al. Fetal, Infant, and Childhood Growth and Adult Blood Pressure: A Longitudinal Study From Birth to 22 Years of Age. Circulation (2002) 105(9):1088–92. doi: 10.1161/hc0902.104677
43. Law CM, Barker DJ, Osmond C, Fall CH, Simmonds SJ. Early Growth and Abdominal Fatness in Adult Life. J Epidemiol Community Health (1992) 46(3):184–6. doi: 10.1136/jech.46.3.184
44. Ozanne SE, Nave BT, Wang CL, Shepherd PR, Prins J, Smith GD. Poor Fetal Nutrition Causes Long-Term Changes in Expression of Insulin Signaling Components in Adipocytes. Am J Physiol (1997) 273(1 Pt 1):E46–51. doi: 10.1152/ajpendo.1997.273.1.E46
45. Ozanne SE, Dorling MW, Wang CL, Nave BT. Impaired PI 3-Kinase Activation in Adipocytes From Early Growth-Restricted Male Rats. Am J Physiol Endocrinol Metab (2001) 280(3):E534–539. doi: 10.1152/ajpendo.2001.280.3.E534
46. Valdez R, Athens MA, Thompson GH, Bradshaw BS, Stern MP. Birthweight and Adult Health Outcomes in a Biethnic Population in the USA. Diabetologia (1994) 37(6):624–31. doi: 10.1007/BF00403383
47. Economides DL, Proudler A, Nicolaides KH. Plasma Insulin in Appropriate- and Small-for-Gestational-Age Fetuses. Am J Obstet Gynecol (1989) 160(5 Pt 1):1091–4. doi: 10.1016/0002-9378(89)90167-1
48. Garofano A, Czernichow P, Bréant B. In Utero Undernutrition Impairs Beta-Cell Development. Diabetologia (1997) 40(10):1231–4. doi: 10.1007/s001250050812
49. Limesand SW, Rozance PJ, Zerbe GO, Hutton JC, Hay WH Jr. Attenuated Insulin Release and Storage in Fetal Sheep Pancreatic Islets With Intrauterine Growth Restriction. Endocrinology (2006) 147(3):1488–97. doi: 10.1210/en.2005-0900
50. Mari A, Pacini G, Murphy E, Ludvik B, Nolan JJ. A Model-Based Method for Assessing Insulin Sensitivity From the Oral Glucose Tolerance Test. Diabetes Care (2001) 24(3):539–48. doi: 10.2337/diacare.24.3.539
51. Matsuda M, DeFronzo RA. Insulin Sensitivity Indices Obtained From Oral Glucose Tolerance Testing: Comparison With the Euglycemic Insulin Clamp. Diabetes Care (1999) 22(9):1462–70. doi: 10.2337/diacare.22.9.1462
52. Hamley S, Kloosterman D, Duthie T, Dalla Man C, Visentin R, Mason SA, et al. Mechanisms of Hyperinsulinaemia in Apparently Healthy non-Obese Young Adults: Role of Insulin Secretion, Clearance and Action and Associations With Plasma Amino Acids. Diabetologia (2019) 62(12):2310–24. doi: 10.1007/s00125-019-04990-y
53. Borges DO, Patarrão RS, Ribeiro RT, de Oliveira RM, Duarte N, Belew GD, et al. Loss of Postprandial Insulin Clearance Control by Insulin-Degrading Enzyme Drives Dysmetabolism Traits. Metabolism (2021) 118:154735. doi: 10.1016/j.metabol.2021.154735
54. Tura A, Göbl C, Morettini M, Burattini L, Kautzky-Willer A, Pacini G. Insulin Clearance is Altered in Women With a History of Gestational Diabetes Progressing to Type 2 Diabetes. Nutr Metab Cardiovasc Dis (2020) 30(8):1272–80. doi: 10.1016/j.numecd.2020.04.004
55. Chin HB, Baird DD, McConnaughey DR, Weinberg CR, Wilcox AJ, Jukic AM. Long-Term Recall of Pregnancy-Related Events. Epidemiology (2017) 28(4):575–9. doi: 10.1097/EDE.0000000000000660
56. Promislow JHE, Gladen BC, Sandler DP. Maternal Recall of Breastfeeding Duration by Elderly Women. Am J Epidemiol (2005) 161(3):289–96. doi: 10.1093/aje/kwi044
57. Tomeo CA, Rich-Edwards JW, Michels KB, Berkey CS, Hunter DJ, Frazier AL, et al. Reproducibility and Validity of Maternal Recall of Pregnancy-Related Events. Epidemiology (1999) 10(6):774–7. doi: 10.1097/00001648-199911000-00022
Keywords: low birth weight, early life events, beta cell function, insulin sensitivity, prediabetes
Citation: Branda JIF, de Almeida-Pititto B, Bensenor I, Lotufo PA and Ferreira SRG (2022) Low Birth Weight, β-Cell Function and Insulin Resistance in Adults: The Brazilian Longitudinal Study of Adult Health. Front. Endocrinol. 13:842233. doi: 10.3389/fendo.2022.842233
Received: 23 December 2021; Accepted: 14 February 2022;
Published: 14 March 2022.
Edited by:
Raghavendra L.S. Hallur, Pravara Institute of Medical Sciences (Deemed to be University), IndiaReviewed by:
Patricia Cristina Lisboa, Rio de Janeiro State University, BrazilMicaela Morettini, Marche Polytechnic University, Italy
Copyright © 2022 Branda, de Almeida-Pititto, Bensenor, Lotufo and Ferreira. This is an open-access article distributed under the terms of the Creative Commons Attribution License (CC BY). The use, distribution or reproduction in other forums is permitted, provided the original author(s) and the copyright owner(s) are credited and that the original publication in this journal is cited, in accordance with accepted academic practice. No use, distribution or reproduction is permitted which does not comply with these terms.
*Correspondence: Sandra Roberta G. Ferreira, c2FuZHJhZnZAdXNwLmJy