- 1Emergency Department, The Affiliated Hospital of Southwest Jiaotong University, The Third People’s Hospital of Chengdu, Chengdu, Sichuan, China
- 2Department of Geriatrics, West China Hospital, Sichuan University, Chengdu, China
- 3Department of Geriatrics, The Affiliated Hospital of Southwest Jiaotong University, The Third People’s Hospital of Chengdu, Chengdu, Sichuan, China
- 4Department of Cardiology, The Affiliated Hospital of Southwest Jiaotong University, The Third People’s Hospital of Chengdu, Chengdu, Sichuan, China
Purpose: Type 1 diabetes mellitus (T1DM) is associated with different types of infections; however, studies on the causal relationship between T1DM and infectious diseases are lacking. Therefore, our study aimed to explore the causalities between T1DM and six high-frequency infections using a Mendelian randomization (MR) approach.
Methods: Two-sample MR studies were conducted to explore the causalities between T1DM and six high-frequency infections: sepsis, acute lower respiratory infections (ALRIs), intestinal infections (IIs), infections of the genitourinary tract (GUTIs) in pregnancy, infections of the skin and subcutaneous tissues (SSTIs), and urinary tract infections (UTIs). Data on summary statistics for T1DM and infections were obtained from the European Bioinformatics Institute database, the United Kingdom Biobank, FinnGen biobank, and Medical Research Council Integrative Epidemiology Unit. All data obtained for summary statistics were from European countries. The inverse-variance weighted (IVW) method was employed as the main analysis. Considering the multiple comparisons, statistical significance was set at p< 0.008. If univariate MR analyses found a significant causal association, multivariable MR (MVMR) analyses were performed to adjust body mass index (BMI) and glycated hemoglobin (HbA1c). MVMR-IVW was performed as the primary analysis, and the least absolute shrinkage and selection operator (LASSO) regression and MVMR-Robust were performed as complementary analyses.
Results: MR analysis showed that susceptibility to IIs increased in patients with T1DM by 6.09% using the IVW-fixed method [odds ratio (OR)=1.0609; 95% confidence interval (CI): 1.0281–1.0947, p=0.0002]. Results were still significant after multiple testing. Sensitivity analyses did not show any significant horizontal pleiotropy or heterogeneity. After adjusting for BMI and HbA1c, MVMR-IVW (OR=1.0942; 95% CI: 1.0666–1.1224, p<0.0001) showed significant outcomes that were consistent with those of LASSO regression and MVMR-Robust. However, no significant causal relationship was found between T1DM and sepsis susceptibility, ALRI susceptibility, GUTI susceptibility in pregnancy, SSTI susceptibility, and UTI susceptibility.
Conclusions: Our MR analysis genetically predicted increased susceptibility to IIs in T1DM. However, no causality between T1DM and sepsis, ALRIs, GUTIs in pregnancy, SSTIs, or UTIs was found. Larger epidemiological and metagenomic studies are required to further investigate the observed associations between the susceptibility of certain infectious diseases with T1DM.
1 Introduction
Type 1 diabetes mellitus (T1DM) is a chronic autoimmune illness characterized by hyperglycemia caused by insulin deficiency due to the secondary loss of the pancreatic islet βcells (1). T1DM, the most prevalent autoimmune disorder in children and teenagers, currently affects more than 500,000 children globally (2). Long-term hyperglycemia can destroy microvascular and macrovascular systems. Therefore, patients with T1DM may develop multiple organ or tissue diseases, such as cardiovascular disease, cerebrovascular disease, peripheral artery disease, sudden cardiac death, and cognitive dysfunction (2).
Infectious diseases are experienced several times in almost every human. Many people may experience sepsis, a high-mortality syndrome that develops with infection (3). Acute lower respiratory infection (ALRI) was the most common cause of death in children in 2019 and accounted for the deaths of 700,000 children (4). Urinary tract infection (UTI) is a common infectious disease in adults, with more than half of all women experiencing at least one UTI in their life; moreover, UTIs are associated with significantly increased mortality in elderly people (5). Infection of the genitourinary tract (GUTI) is the most common bacterial infection in pregnancy, and GUTIs may cause preterm birth (6). Infection of the skin and subcutaneous tissues (SSTI) is another common infectious disease that can progress to a life-threatening infection (7). A recent nationwide study in the United States found that intestinal infection (II) was one of the most common infectious diseases experienced by patients in hospital (8).
Infectious diseases cause a significant burden on families and society. Recently, there has been much debate regarding whether T1DM increases the risk of infections. Some studies have found that patients with diabetes mellitus may be more susceptible to infectious diseases (9–11). However, Liberatore et al. argued that there was no strong relationship between T1DM and the occurrence of infections (12), and other studies revealed that the relationship between T1DM and infections is bidirectional (13). Remarkably, these studies had small sample sizes and possible confounding variables. Some recent meta-analyses found a significant positive relationship between T1DM and different infectious diseases (14–16); however, most of the studies pooled in meta-analyses were case-control studies and case series, which cannot negate the possibility of reverse causality. Therefore, we cannot distinguish which is the cause and which is the effect in the relationship between T1DM and infectious diseases through meta-analyses. A recent review argued that patients with poorly controlled diabetes were immunocompromised and hence were at increased risk of experiencing infectious diseases and their complications (17).
Confirming a causal relationship between T1DM and infectious diseases is challenging due to the possibility of reverse causality and confounders (18). Therefore, randomized controlled trials (RCTs) are considered the gold standard for exploring exposure-outcome causality; however, conducting rigorous RCTs is challenging because of the restriction of the medical ethics committees and selection of participants, and the extrapolation of results is limited because of the strict requirements of population selection (19).
The Mendelian randomization (MR) method was used to infer causal associations between exposures and outcomes using single nucleotide polymorphisms (SNPs) as instrumental variables (IVs) (20). Variants were randomly allocated from parents to offspring at conception. Therefore, the MR method was not susceptible to confounding or reverse causation, similar to the random assignment in RCTs (20). In this study, a two-sample MR was performed to investigate the causality of T1DM with high-frequency infections.
2 Materials and methods
2.1 Study design and data sources
2.1.1 Genetic association datasets for T1DM
Summary statistical data for T1DM, including 9,266 cases and 15,574 controls with European ancestry, came from the European Bioinformatics Institute (EBI) database (21) and can be extracted from the genome-wide association study (GWAS) datasets by “GWAS ID” (Table 1). The EBI database is an international, innovative, interdisciplinary, and open dataset in life sciences (22).
2.1.2 Genetic association datasets for six high-frequency infections
As ALRIs, IIs, GUTIs in pregnancy, SSTIs, and UTIs were the most common infectious diseases in children, women, pregnant women, and in-hospital patients, respectively, and all infectious diseases can progress to sepsis; therefore, sepsis, ALRIs, IIs, GUTIs in pregnancy, SSTIs, and UTIs were chosen as the outcomes in our study.
Summary-level GWAS results regarding sepsis were obtained from UK Biobank with adjustment for age and sex. UK Biobank is an open dataset that included more than 500,000 participants for health-related research (23). Summary-level GWAS of ALRIs, IIs, GUTIs, and SSTIs were obtained from the FinnGen biobank. The FinnGen biobank is a well-known open dataset and comprises the genotype and phenotype data from approximately 20,000 Finnish individuals (24). Summary-level GWAS of UTIs were obtained from the Medical Research Council Integrative Epidemiology Unit (MRC-IEU). The MRC-IEU is an openly available dataset that comprises the most advanced population health science research in the United Kingdom, utilizing genetics, population data, and experimental interventions to identify the etiology of chronic disease (25).
All patients and controls (of both sexes) included in these summary-level GWASs were mainly of European descent. The detailed diagnostic criteria and the methods used to recruit participants in these GWASs are available in the original publications. No significant sample overlap was evident between these GWAS datasets. The profiles of GWAS datasets of T1DM and infections are summarized in Table 1.
2.1.3 Informed consent statement and ethics approval statement
Informed consent and ethics approval were unnecessary for this study as consent and ethics approvals have already been obtained in the previous induvial studies. Furthermore, all summary-level GWAS data were obtained from an open dataset (https://gwas.mrcieu.ac.uk/).
2.2 Instruments selection
Three key assumptions for MR analysis should be met (Figure 1) (19). SNPs linked to T1DM at the genome-wide significance levels from a meta-analysis of GWAS were selected as IVs (p< 5 10−8). Additionally, the related linkage disequilibrium was considered (kb=10,000, r2<0.001) (26). Subsequently, F statistic was calculated to evaluate the strength between the IVs and exposure with the following equation: (27), where R2 is the quantity that IVs can represent exposure, k is the number of SNPs selected, and N is the exposure sample size. If F is more than 10, the relationship is believed to be robust enough to minimize the bias induced by weak IVs. Thereafter, the SNPs related to potential confounders of the outcomes were eliminated. SNPs were selected after adjusting for body mass index, chronic obstructive pulmonary disease, fatigue, arteritis, vaginitis, urolithiasis, depression, immunocompromised, long-standing illness, disability or infirmity, and inflammatory skin disease, which may influence the risk of sepsis, ALRIs, IIs, GUTIs in pregnancy, SSTIs, and UTIs in PhenoScanner V2 (http://www.phenoscanner.medschl.cam.ac.uk/) (28). Finally, SNPs that were not directly associated with outcomes (sepsis, ALRIs, IIs, GUTIs in pregnancy, SSTIs, and UTIs) were selected using PhenoScanner V2 (2018 Cardiovascular Epidemiology Unit, University of Cambridge) (28).
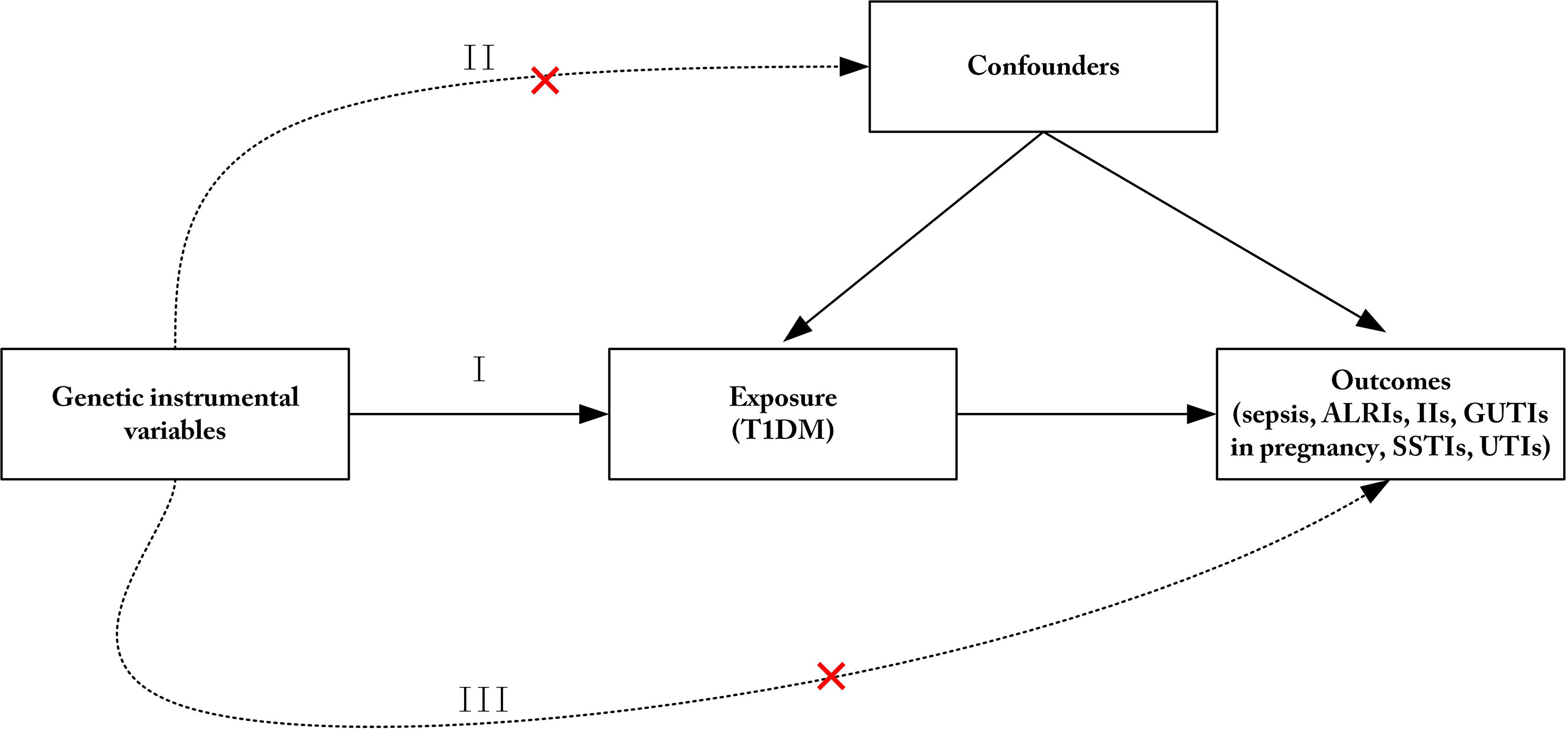
Figure 1 Three principles of the Mendelian randomization study. (I) The genetic instrumental variables (IVs) are strongly associated with T1DM; (II) The genetic IVs do not affect the outcome through the confounders; (III) The genetic IVs do not affect outcomes directly, but only via exposure. ALRIs, acute lower respiratory infections; IIs, intestinal infections; GUTIs, infections of the genitourinary tract; SSTIs, Infections of the skin and subcutaneous tissue; UTIs, urinary tract infections; T1DM, type 1 diabetes mellitus.
We attempted to infer positive-strand alleles using allele frequencies for palindromes across exposure and outcome datasets. SNPs for incompatible alleles and palindromic sequences with intermediate allele frequencies were removed. Proxy SNPs were used when the SNPs were unavailable in the outcomes GWAS datasets, and the minimum linkage disequilibrium r2 was set at 0.8. Final IVs for ensuing MR analysis were then carefully selected.
2.3 MR analysis
The inverse-variance weighted (IVW) method was used as the main analysis approach because of its precise estimate (29). When heterogeneity was statistically significant, the random effect model was utilized. Otherwise, the fixed effect model was utilized. Other MR methods were also performed to guarantee the effectiveness and stability of the outcomes. The maximum likelihood method provides an estimator with the lowest standard error under essentially all conditions compared with other MR methods (30). The penalized weighted median (PWM) estimator is a novel MR method that can provide a consistent estimator when significant heterogeneity exists (31). The weighted median estimator (WME) can control type I errors efficiently to improve the detection ability of causal effects and can provide a stable estimator, even when more than 50% of the information is acquired from invalid IVs (32). The MR-Egger method can identify and correct potential pleiotropy and provide a relatively consistent estimate (33). Considering the multiple comparisons, the Bonferroni method was performed to rectify overall type I errors, and p<0.008 (α = 0.05/6) was considered statistically significant. If a significant causal association was found in univariate MR analyses, multivariable MR (MVMR) analyses would be performed. MVMR-IVW was performed as the primary analysis (34). The least absolute shrinkage and selection operator (LASSO) regression provides the best estimation for moderate-to-high levels of pleiotropy and valid inference in a three-sample setting (35). MVMR-Robust supplies precision estimates in all scenarios (even with 70% pleiotropy), corrects type I error rates, and assesses instrument strength (35). Therefore, we conducted LASSO regression and MVMR-Robust as complementary analyses. MVMR analyses were performed by adjusting for potential confounders, including body mass index (BMI), which had a causality association with T1DM and infectious diseases in previous MR studies (36, 37), and glycated hemoglobin (HbA1c), which reflects the average blood sugar over the past 3 months. We choose HbA1c as a confounder, as numerous studies have found that the status of hyperglycemia in diabetes was responsible for increased morbidity and mortality of infectious diseases rather than diabetes itself (17, 38). Detailed information on the genetic association datasets of the two confounders is presented in Supplementary Table 1.
2.4 Sensitivity analysis
Cochran’s Q statistic (R Foundation for Statistical Computing, Vienna, Austria) was used to identify SNP heterogeneity (34). The MR-Egger intercept (differs on average from zero) method was used to test whether genetic variants of T1DM have pleiotropic effects on infections (33). Mendelian randomization pleiotropy residual sum and outlier (MR-PRESSO) (39) and leave-one-out (40) methods were employed to detect potential outlier SNPs. The forest plot was used to exhibit the single SNP effect size of T1DM on infections. The scatter plot was used to test the causal effects of T1DM on infections. Finally, the funnel plot was used to demonstrate the symmetrical distribution of the selected SNPs. For sensitivity analysis, p<0.05 indicated statistical significance. All data were analyzed using the “TwoSampleMR,” “MRPRESSO,” “MendelianRandomization,” and “MVMR” packages in R software 4.0.5 (R Foundation for Statistical Computing).
3 Results
After the initial screening, 44 SNPs were obtained as IVs. Extensive information on 44 SNPs is presented in Supplementary Table 2. Five SNPs (rs9273363, rs1131017, rs6679677, rs10830227, and rs2111485), which may be associated with confounders, were deleted using the PhenoScanner tool (http://www.phenoscanner.medschl.cam.ac.uk/), and no SNP traits were associated with sepsis, ALRIs, IIs, GUTIs in pregnancy, SSTIs, and UTIs (Table 2). Finally, we obtained 39 SNPs as IVs of T1DM.
3.1 MR Analysis Between T1DM and IIs
3.1.1 Univariate MR analyses
After removing SNP (rs34954) for incompatible alleles, we did not find proxy SNP in the GWAS dataset of IIs. Finally, we obtained 33 SNPs as IVs in the MR analysis of T1DM on IIs. For these IVs, the F statistic was 22.80604. Detailed information about the SNPs of T1DM on IIs is shown in Supplementary Table 3.
The risk of IIs was found to be increased in patients with T1DM by 6.09% using the IVW method (OR=1.0609; 95% CI 1.0281–1.0947, p=0.0002), increased by 7.09% using the MR-Egger method (OR=1.0709; 95% CI, 1.0161–1.1286; p=0.0158), increased by 6.35% using the WME method (OR=1.0635; 95% CI, 1.0143–1.115; p=0.0108), increased by 6.12% using the Maximum likelihood method (OR=1.0612; 95% CI, 1.0281–1.0954; p=0.0002), and increased by 6.12% using the PWM method (OR=1.0634; 95% CI, 1.0141–1.115; p=0.0112). The results are shown in Table 3.
No heterogeneity (MR-Egger, p=0.4049; IVW, p=0.4447) and no potential pleiotropy (MR-Egger, intercept=-0.0041; p=0.6633) were observed in the univariate MR analysis. The MR-PRESSO test showed no outliers. The funnel plot showed the selected SNPs were distributed symmetrically (Figure 2A). The scatter plot demonstrated the causality between T1DM and IIs (Figure 2B). The forest plot displays the effect size for every single SNP on the risk of IIs and shows that causality existed between T1DM and the occurrence of IIs (Figure 2C). The “Leave-one-out plot” suggested that no SNP had an important impact on the estimated causal association (Figure 2D).
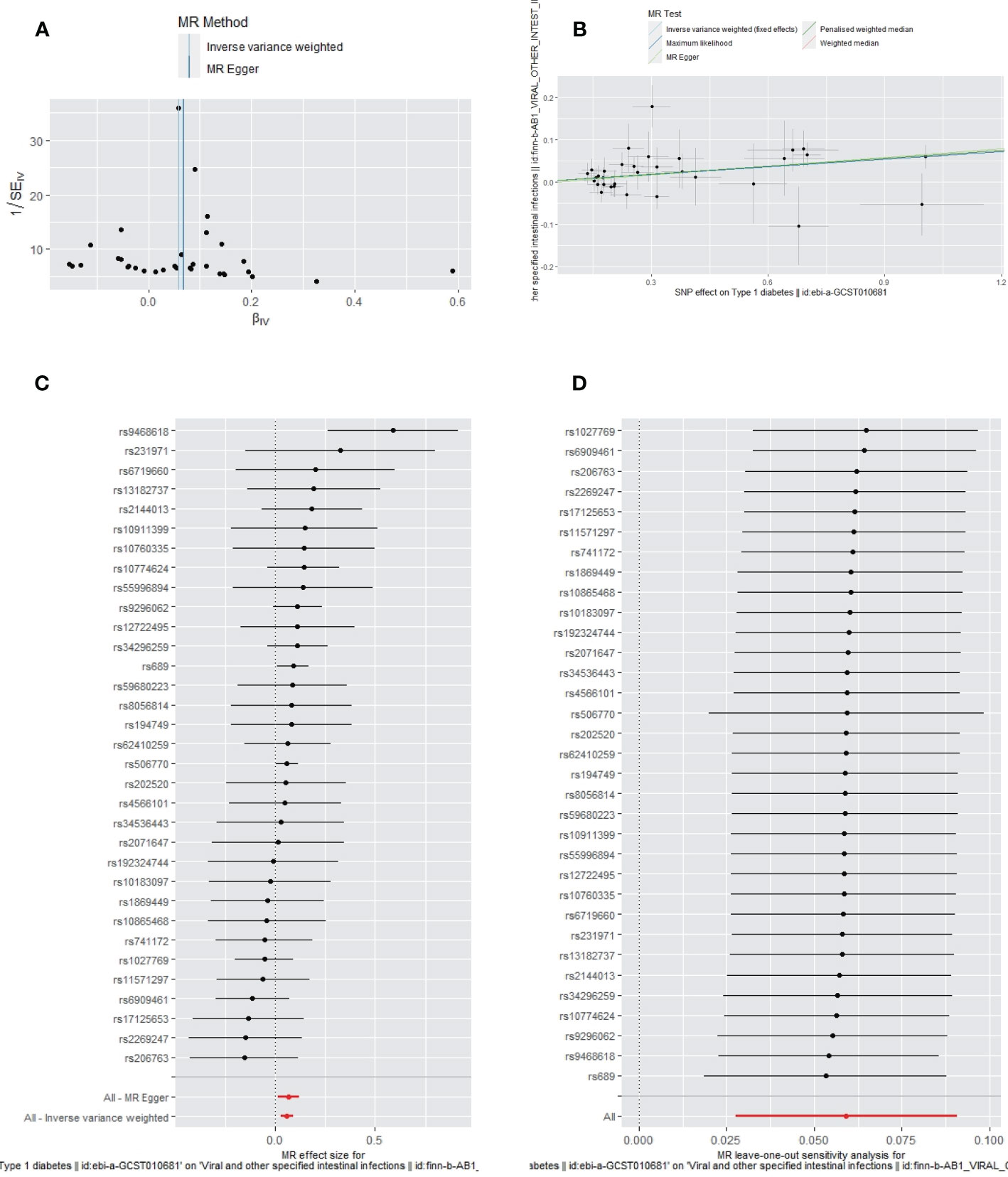
Figure 2 The funnel plot (A), scatter plot (B), Forest plot (C) and leave-one-out plot (D) of the genetic risk of T1DM on IIs.
3.1.2 MVMR analyses
The MVMR-IVW estimates also showed that T1DM had a significant causal association with IIs after adjusting for BMI and HbA1c (OR=1.0942; 95% CI, 1.0666–1.1224; p<0.0001). No heterogeneity (MR-IVW, p=0.9580) was observed. The LASSO regression (OR=1.0942; 95% CI, 1.0666 - 1.1224; p<0.001) and MVMR-Robust (MVMR-Robust, OR= 1.0932; 95% CI, 1.0608 - 1.1263; p<0.05) results were consistent with those of the MVMR-IVW analyses. The F-statistic was 45.4855, and the number of valid instruments was 88.
3.2 MR analysis between T1DM and sepsis, ALRIs, GUTIs in pregnancy, SSTIs, and UTIs
One SNP (rs34954) was removed for incompatible alleles when combining the GWAS data of sepsis, ALRIs, GUTIs in pregnancy, and SSTIs separately with the selected IVs of T1DM. No SNP was removed for incompatible alleles when combining the GWAS data of UTIs and the selected IVs of T1DM. No proxy SNP was found in the GWAS dataset of ALRIs, GUTIs in pregnancy, SSTIs, and UTIs. SNP (rs34296259) was proxied by rs190824943 in the GWAS dataset of sepsis. Finally, we obtained 38, 33, 33, 33, and 26 SNPs, respectively, as IVs in the MR analysis of T1DM on sepsis, ALRIs, GUTIs in pregnancy, SSTIs, and UTIs separately. For these selected IVs, F statistics were more than 10. Detailed information about SNPs of T1DM on sepsis, ALRIs, GUTIs in pregnancy, SSTIs, and UTIs are separately presented in Supplementary Tables 4-8.
The primary IVW-fixed method showed no causality between T1DM and sepsis, ALRIs, GUTIs in pregnancy, SSTIs, or UTIs. The other MR method (MR-Egger, WME, maximum likelihood, and PWM) results were consistent with IVWs (Table 3).
Apart from a significant heterogeneity in the analysis of T1DM on SSTIs, no other heterogeneity was observed in the analysis of T1DM on sepsis, ALRIs, GUTIs in pregnancy, or UTIs. No potential pleiotropy or outliers were observed in the analysis of T1DM on sepsis, ALRIs, GUTIs in pregnancy, UTIs, and SSTIs (Table 4). The funnel plots, the scatter plots, the forest plots, and the leave-one-out plots of T1DM for sepsis, ALRIs, GUTIs in pregnancy, SSTIs, and UTIs are displayed in Figures 3–7 separately.
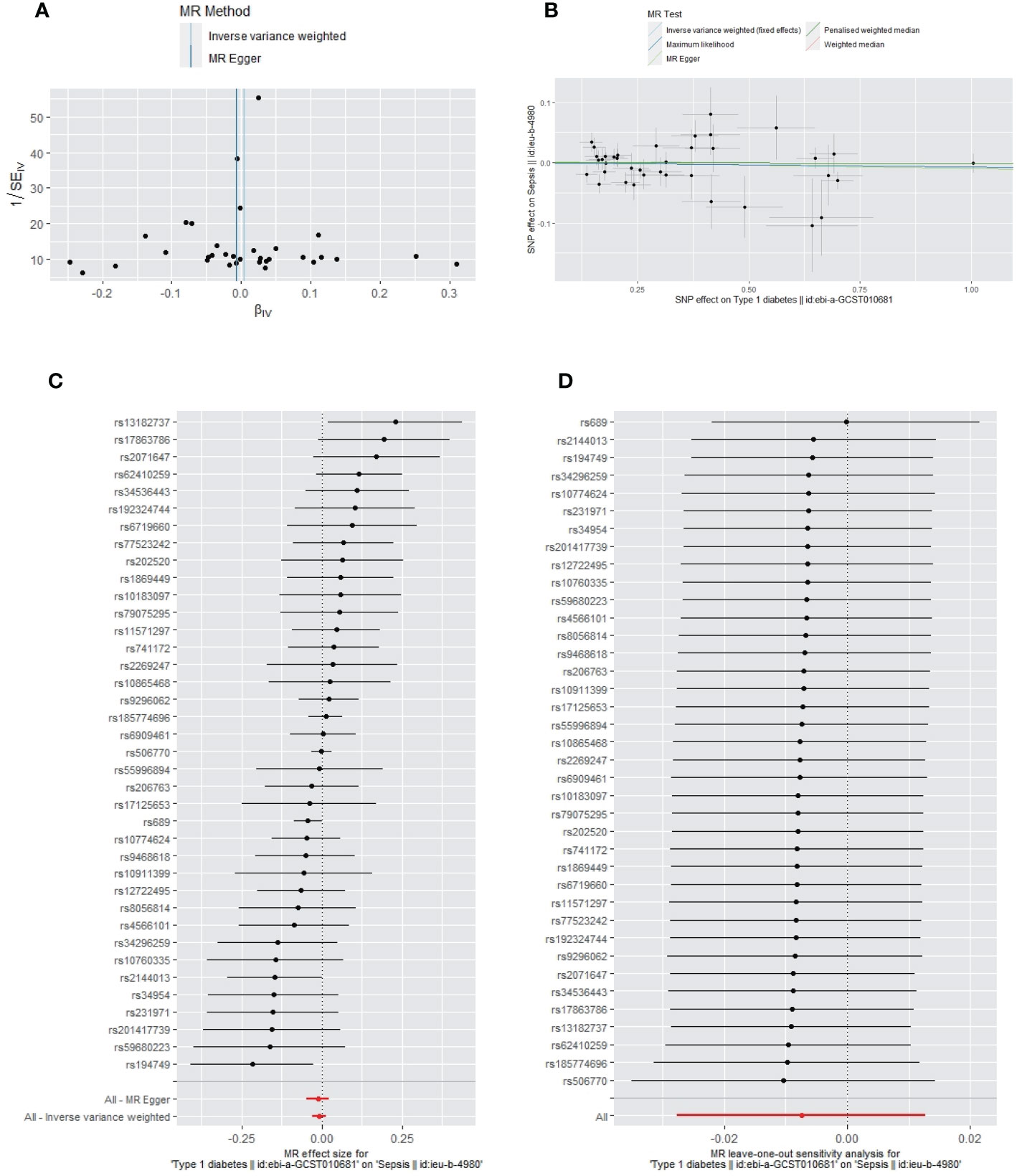
Figure 3 The funnel plot (A), scatter plot (B), Forest plot (C) and leave-one-out plot (D) of the genetic risk of T1DM on sepsis.
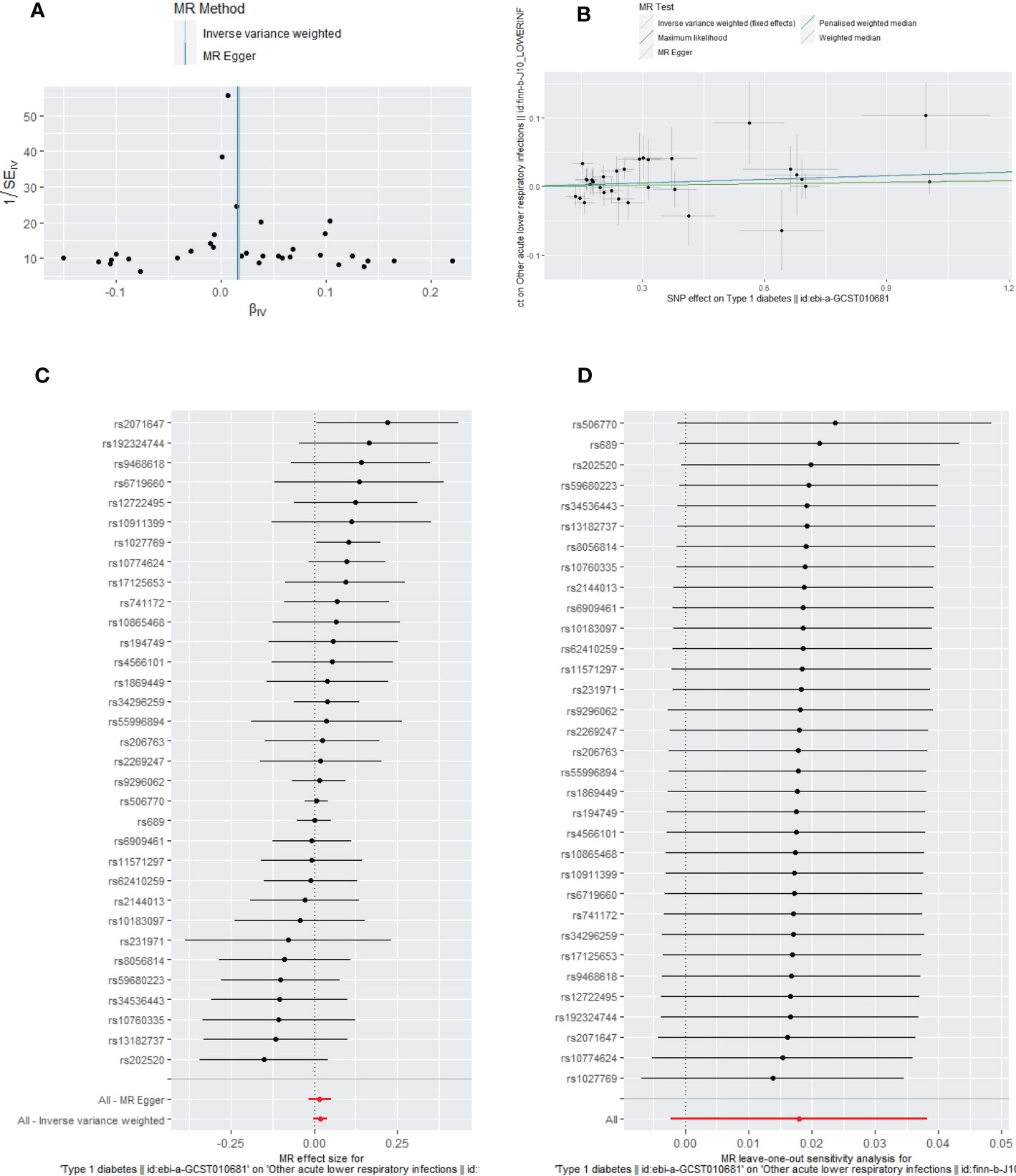
Figure 4 The funnel plot (A), scatter plot (B), Forest plot (C) and leave-one-out plot (D) of the genetic risk of T1DM on ALRIs.
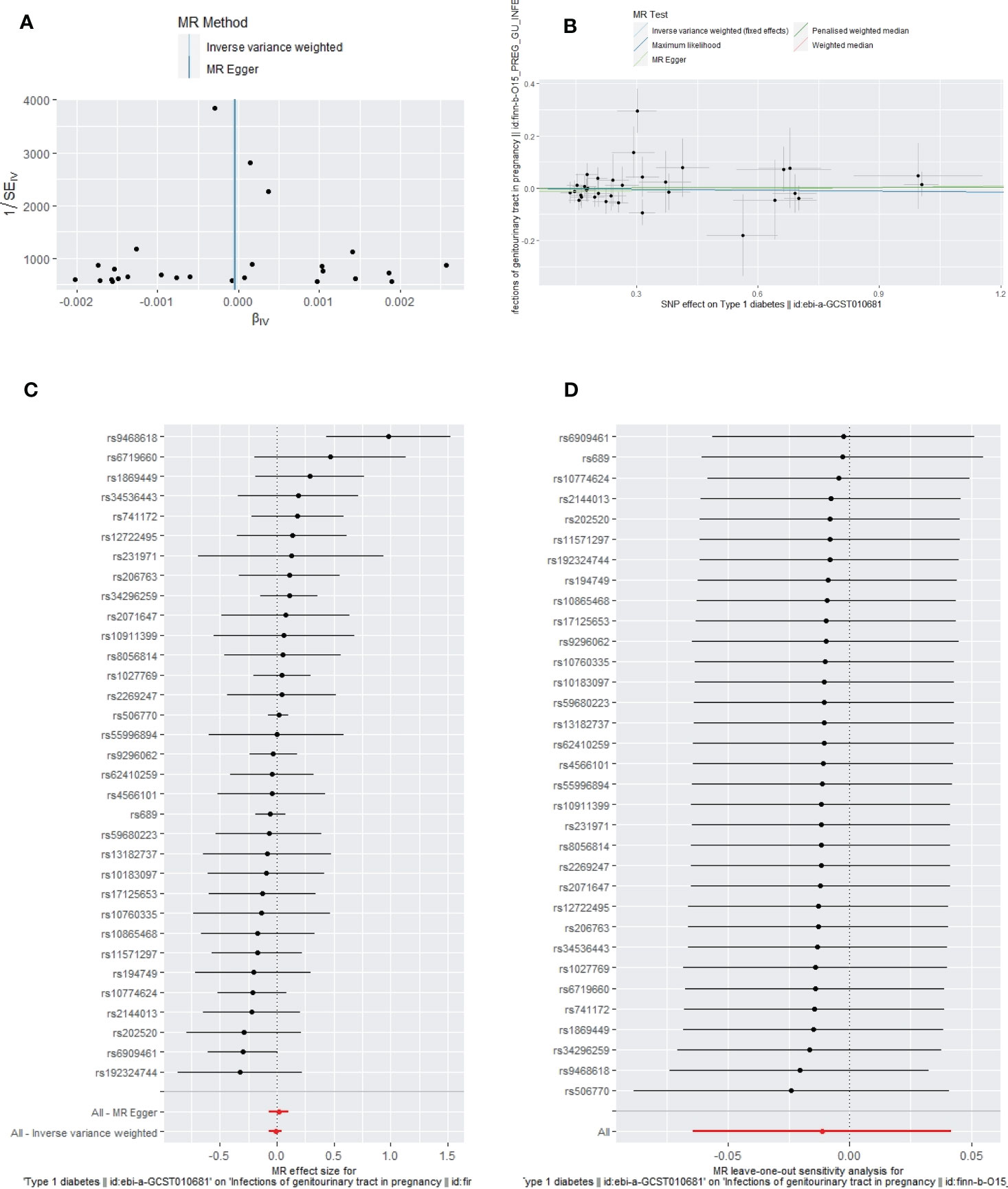
Figure 5 The funnel plot (A), scatter plot (B), Forest plot (C) and leave-one-out plot (D) of the genetic risk of T1DM on GUTIs in pregnancy.
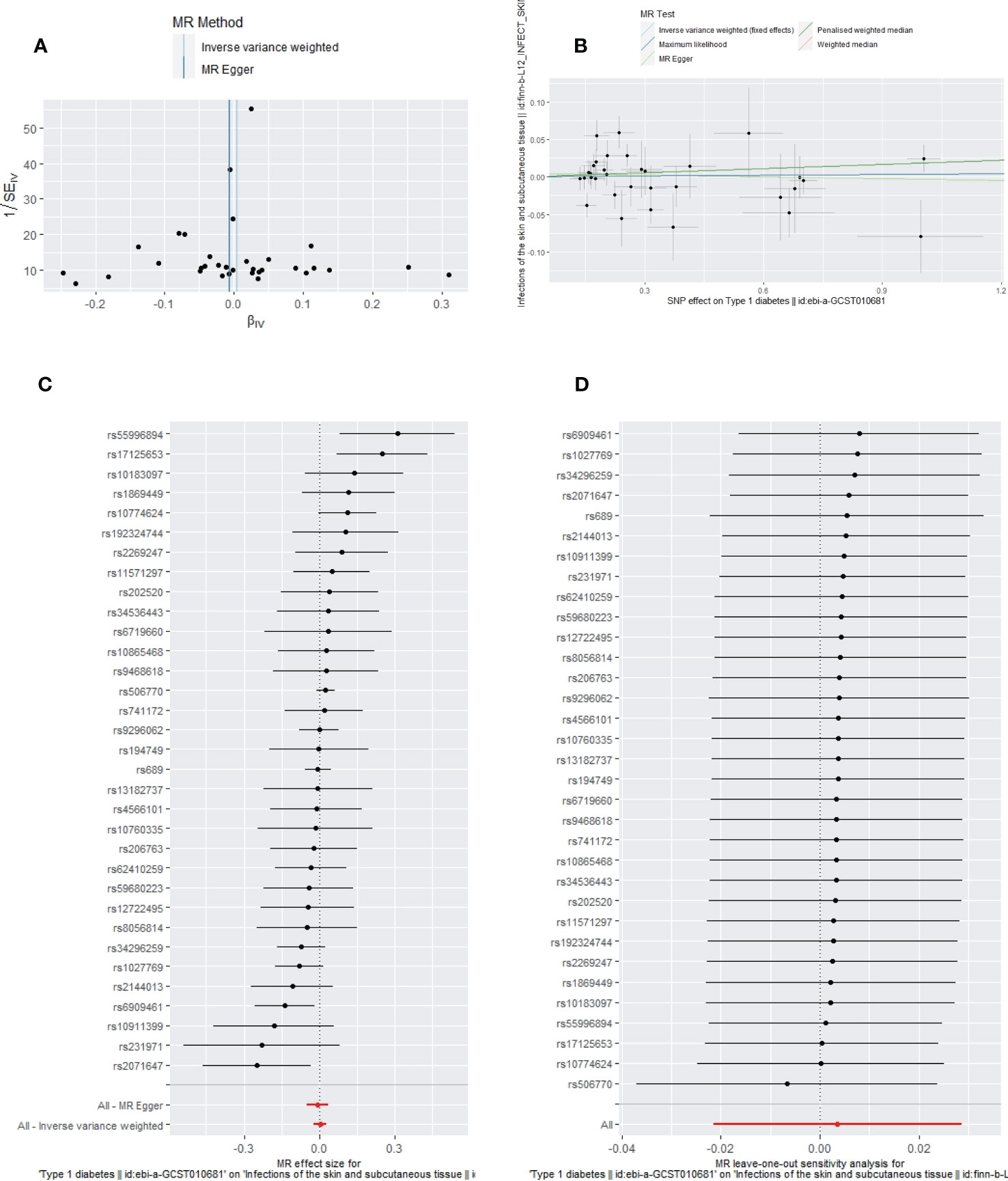
Figure 6 The funnel plot (A), scatter plot (B), Forest plot (C) and leave-one-out plot (D) of the genetic risk of T1DM on SSTIs.
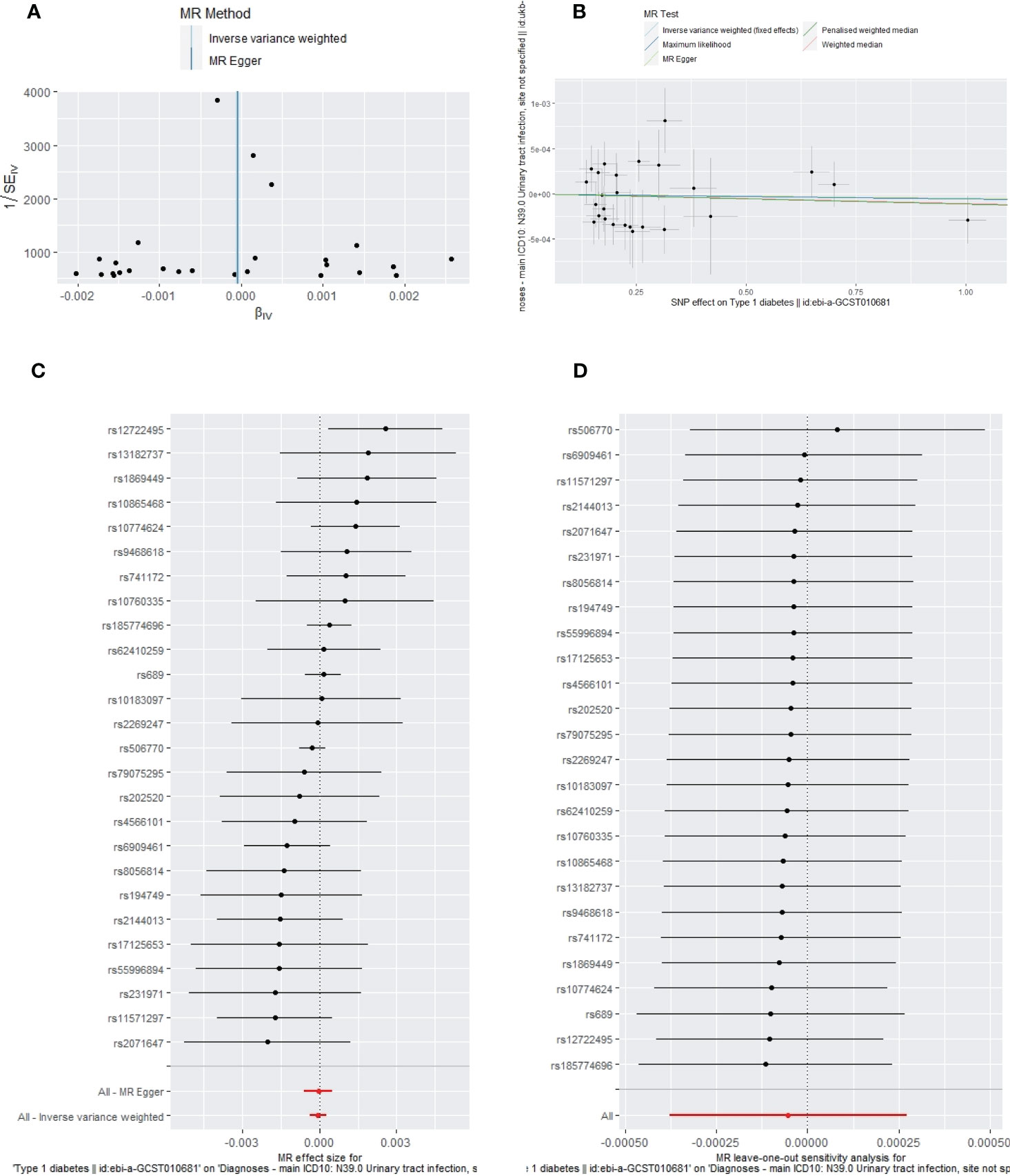
Figure 7 The funnel plot (A), scatter plot (B), Forest plot (C) and leave-one-out plot (D) of the genetic risk of T1DM on UTIs.
4 Discussion
Based on the first MR study, we investigated the causality of T1DM in six high-frequency infections. There were two main results of the present MR study. First, the risk of IIs increased by 6.09% (OR=1.0609; 95% CI: 1.0281–1.0947, p=0.0002) in patients with T1DM even when a significant threshold was set at 0.008 (corrected by the Bonferroni method). The result was confirmed by sensitivity analyses. In the MVMR analyses, after adjusting for BMI and HbA1c, the causality of T1DM on IIs was still significant (MVMR-IVW, OR=1.0942; 95% CI, 1.0666–1.1224; p<0.0001); these results were consistent with those of the LASSO regression and MVMR-Robust. Second, T1DM may not be responsible for some infections, such as sepsis, ALRIs, GUTIs in pregnancy, SSTIs, and UTIs.
The findings of the present MR study did not support the findings of some observational studies that suggested that patients with T1DM had an increased risk of contracting some infections (41–43). A prospective cohort study reported that patients with T1DM were at a highly increased risk of lower respiratory tract, urinary tract, and skin infection (42). Another retrospective study that adjusted for age and sex also found that patients with T1DM were more susceptible to infection with the SARS-CoV-2 virus (43). Hence, our findings are consistent with those of many previous observational studies (12, 44, 45). One case-control study concluded that patients with T1DM were not susceptible to infections by comparing the plasma levels of immunoglobulins and complement proteins in patients with T1DM and controls (12). A survey with 10 years of follow-up stated that sexual activity rather than the T1DM was linked to an increased risk of UTIs in women (44). Czaja et al. believed that patients with T1DM may have an increased risk of asymptomatic bacteriuria (ASB). Nevertheless, due to impaired immunity caused by T1DM, ASB would not progress to a symptomatic infectious disease (44). Another prospective study showed that the risk of sepsis increased when T1DM was complicated with hind foot ulceration (45). Recently, a meta-analysis discovered that diabetes mellitus did not affect sepsis prognosis, although high blood glucose levels did (46).
T1DM and infections have been linked bidirectionally. As mentioned above, some studies argued that T1DM increased the occurrence of infections, whereas many other studies found that infections also have a marked influence on the onset of T1DM. For example, a study based on a population-based registry in Abruzzo (central Italy) found that multiple bacterial infections before diabetes mellitus could significantly delay the onset of T1DM (47). A review summarized that enterovirus infections, such as coxsackievirus B4, accelerated or promoted the initiation of T1DM (48). Another review also suggested that gut dysbiosis might be associated with the onset of T1DM, and different intestinal bacteria play different roles (49).
T1DM is a chronic disease, and patients with this disease may experience complications, long-term abnormal blood sugar levels, unhealthy diet habits, and different environments; hence, it is challenging for observational studies to adjust for all confounders (2). Therefore, residual confounding or reverse causation bias in observational research may explain our disagreement. The present study used an MR design to confirm the causality pathway from T1DM to six high-frequency infections. As no heterogeneity was observed in the MR analysis of T1DM on IIs, sepsis, ALRIs, GUTIs in pregnancy, and UTIs, we selected the IVW-fixed analysis results as our primary results. As significant heterogeneity was observed in the MR analysis of T1DM on SSTIs, we selected the IVW-random analysis result as the primary result. We also conducted different MR methods as complementary and sensitivity analyses to confirm our results. Moreover, to strictly meet the criteria of an “IVs not linked to confounding factors” and “IVs influence outcomes only through T1DM,” we deleted all SNPs potentially associated with confounders and outcomes according to the trait in PhenoScanner V2.
Approximately 451 million people have diabetes mellitus globally, approximately 5 million deaths were attributed to diabetes mellitus (DM) in 2017, and the number of people with DM may increase to 693 million by 2045 (50). Recently, Wang et al. conducted an MR to explore the causal relationship between T2DM and five high-frequency infectious diseases, including UTIs in pregnancy, ALRIs, sepsis, and SSTIs. They stated that no causal relationships existed (51). The present MR study complemented and extended the abovementioned study by Wang et al. In this study, we not only confirmed that T1DM has no causality with those five high-frequency infectious diseases but also found that T1DM increased the occurrence of IIs due to genetic liability.
Early in 2007, Oikarinen et al. examined enteroviruses in small intestinal tissue of T1DM and suggested that most had a persistent enterovirus infection of the intestinal mucosa (52). A later case-control study confirmed that enterovirus RNA was found more frequently in patients with T1DM than in controls, and the enterovirus was considered to be associated with IIs (53).
The potential mechanism between T1DM and IIs may be explained by the following three pathways. First, many studies have reported dysbiosis of the intestinal microbiomes in patients with T1DM, which would promote inflammation in the gut (49, 54). Second, a review article suggested that a genetically deficient macrophage migration inhibitory factor, one of the mechanisms for the occurrence of T1DM, would induce the Th1 inflammatory cytokines and reduce regulatory T cells (Treg), thereby finally causing colitis (55). Third, celiac disease primarily affects the small intestine, causes weight loss, anemia, and intestinal mucosal damage, and has been found to be associated with T1DM (56, 57). Both celiac disease and T1DM are linked to high-risk human lymphocyte antigens (HLAs) (58). HLAs present antigens to antigen-responsive T cells, resulting in the destruction of the intestinal enterocyte (58).
Based on our positive findings, we provide some prevention strategies for infections in T1DM. First, hand hygiene: good hand hygiene is the most important step in preventing infections. Second, food hygiene: individuals with T1DM should ensure that their food is clean and thoroughly cooked. Third, immunization: individuals with T1DM should receive all recommended vaccinations, especially some vaccines to prevent intestinal infections, such as the rotavirus vaccine, enterohemorrhagic E. coli vaccine, and salmonella vaccine (59). Fourth, blood sugar control: individuals with T1DM should aim to maintain good glycemic control to reduce the risk of infections. Fifth, probiotics are live microorganisms that can help to promote a healthy gut microbiome and prevent intestinal infections. T1DM can take probiotic supplements. Sixth, follow a strict gluten-free diet when T1DM is complicated with celiac disease (60).
The MR study design, which reduces confounders and reverse causality which may influence the findings of epidemiological studies, is a key strength of this work. Additionally, we systematically explored the causalities between T1DM and six common infections. For all the selected IVs, F-statistics were more than 10. Furthermore, no pleiotropy was detected, confirming our results’ accuracy. After a causal association between T1DM and IIs was detected, we performed three different MVMR analyses (MVMR-IVW, LASSO, and MVMR-Robust) to assess the validity of the results by adjusting for BMI and HbA1c. LASSO and MVMR-Robust provided a reliable estimation even with high pleiotropy, and no heterogeneity was observed; the results obtained using the three methods were consistent, demonstrating their credibility. The datasets of exposure and outcomes were mainly composed of European populations; consequently, these findings were less likely to be influenced by population stratification, although it limits the applicability to other ethnic groups. Moreover, we presented a checklist based on the previous work by Woolf et al. (61) to improve our reporting quality, and most of the list items were attained. Since we could not obtain detailed information from the original research, it was challenging to evaluate the quality of the data sources (Supplementary Table 9). We only chose recent datasets provided by widely recognized consortiums to address this limitation. Furthermore, we cannot exclude the possibility that other potential confounders may have influenced our results. We excluded all SNPs associated with more than ten potential confounders possibly associated with the outcomes and performed three MVMR methods adjusting for BMI and HbA1c. However, we cannot rule out all the direct pathways between T1DM and IIs, as pleiotropy could not be controlled entirely. Since some previous studies showed some infectious diseases might cause T1DM, a bidirectional MR analysis should be performed to explore the association of infectious diseases with T1DM. However, none of the six infectious diseases GWAS datasets could supply sufficient SNPs (clumped at p< 5 × 10−8, kb=10,000, and r2<0.001) to perform MR and sensitivity analyses. Therefore, this was one limitation we could not improve and should be performed in future studies.
In conclusion, our study found no convincing evidence of a causal link between T1DM and sepsis, ALRIs, GUTIs in pregnancy, SSTIs, or UTIs in the European population. However, there was a genetic liability in T1DM for increased risk of contracting IIs. Larger epidemiological studies or metagenomic studies are needed to better understand the observed association between susceptibility of certain infectious diseases in patients with T1DM.
Data availability statement
The datasets presented in this study can be found in online repositories. The names of the repository/repositories and accession number(s) can be found below: The datasets [T1DM, sepsis, ALRIs, GUTI susceptibility in pregnancy, SSTIs, UTIs, IIs] for this study can be found in the GWAS summary data [https://gwas.mrcieu.ac.uk/].
Ethics statement
Ethical review and approval was not required for the study on human participants in accordance with the local legislation and institutional requirements. Written informed consent for participation was not required for this study in accordance with the national legislation and the institutional requirements.
Author contributions
TX, HW, and X-HC contributed to the conception and design of the study. H-QL and QN organized the database. X-HC and QN performed the statistical analyses. X-HC and H-QL wrote the first draft of the manuscript. All authors contributed to the article and approved the submitted version.
Funding
This study was supported by the Project of Chengdu Science and Technology Department [2021-YF05-00823-SN].
Acknowledgments
Summary-level data for SNPs associated with T1DM were extracted from the EBI database. Summary-level data for the six high-frequency infections were extracted from the UK Biobank, FinnGen biobank, and MRC Integrative Epidemiology Unit. We thank all the participants for sharing the data.
Conflict of interest
The authors declare that the research was conducted in the absence of any commercial or financial relationships that could be construed as a potential conflict of interest.
Publisher’s note
All claims expressed in this article are solely those of the authors and do not necessarily represent those of their affiliated organizations, or those of the publisher, the editors and the reviewers. Any product that may be evaluated in this article, or claim that may be made by its manufacturer, is not guaranteed or endorsed by the publisher.
Supplementary material
The Supplementary Material for this article can be found online at: https://www.frontiersin.org/articles/10.3389/fendo.2023.1135726/full#supplementary-material
References
2. Katsarou A, Gudbjornsdottir S, Rawshani A, Dabelea D, Bonifacio E, Anderson BJ, et al. Type 1 diabetes mellitus. Nat Rev Dis Primers (2017) 3:17016. doi: 10.1038/nrdp.2017.16
3. Evans L, Rhodes A, Alhazzani W, Antonelli M, Coopersmith CM, French C, et al. Surviving sepsis campaign: International guidelines for management of sepsis and septic shock 2021. Crit Care Med (2021) 49:e1063–e143. doi: 10.1097/CCM.0000000000005337
4. Roh EJ, Shim JY, Chung EH. Epidemiology and surveillance implications of community-acquired pneumonia in children. Clin Exp Pediatr (2022) 65:563–73. doi: 10.3345/cep.2022.00374
5. Medina M, Castillo-Pino E. An introduction to the epidemiology and burden of urinary tract infections. Ther Adv Urol (2019) 11:1756287219832172. doi: 10.1177/1756287219832172
6. Ansaldi Y, Martinez de Tejada Weber B. Urinary tract infections in pregnancy. Clin Microbiol Infection (2022). doi: 10.1016/j.cmi.2022.08.015
7. Esposito S, Pagliano P, De Simone G, Pan A, Brambilla P, Gattuso G, et al. Epidemiology, aetiology and treatment of skin and soft tissue infections: final report of a prospective multicentre national registry. J Chemother (2022) 34:524–33. doi: 10.1080/1120009X.2022.2075170
8. Kennedy JL, Haberling DL, Huang CC, Lessa FC, Lucero DE, Daskalakis DC, et al. Infectious disease hospitalizations: United states, 2001 to 2014. Chest (2019) 156:255–68. doi: 10.1016/j.chest.2019.04.013
9. Burekovic A, Dizdarevic-Bostandzic A, Godinjak A. Poorly regulated blood glucose in diabetic patients-predictor of acute infections. Med Arch (2014) 68:163–6. doi: 10.5455/medarh.2014.68.163-166
10. McKane CK, Marmarelis M, Mendu ML, Moromizato T, Gibbons FK, Christopher KB. Diabetes mellitus and community-acquired bloodstream infections in the critically ill. J Crit Care (2014) 29:70–6. doi: 10.1016/j.jcrc.2013.08.019
11. Critchley JA, Carey IM, Harris T, DeWilde S, Hosking FJ, Cook DG. Glycemic control and risk of infections among people with type 1 or type 2 diabetes in a large primary care cohort study. Diabetes Care (2018) 41:2127–35. doi: 10.2337/dc18-0287
12. Liberatore RR Jr., Barbosa SF, Alkimin M, Bellinati-Pires R, Florido MP, Isaac L, et al. Is immunity in diabetic patients influencing the susceptibility to infections? immunoglobulins, complement and phagocytic function in children and adolescents with type 1 diabetes mellitus. Pediatr Diabetes (2005) 6:206–12. doi: 10.1111/j.1399-543X.2005.00136.x
13. Piccolo G, De Rose EL, Bassi M, Napoli F, Minuto N, Maghnie M, et al. Infectious diseases associated with pediatric type 1 diabetes mellitus: A narrative review. Front Endocrinol (2022) 13:966344. doi: 10.3389/fendo.2022.966344
14. Yang S, Zhao B, Zhang Z, Dai X, Zhang Y, Cui L. Association between enterovirus infection and clinical type 1 diabetes mellitus: systematic review and meta-analysis of observational studies. Epidemiol Infect (2021) 150:e23. doi: 10.1017/S0950268821002442
15. Mansori K, Moradi Y, Naderpour S, Rashti R, Moghaddam AB, Saed L, et al. Helicobacter pylori infection as a risk factor for diabetes: a meta-analysis of case-control studies. BMC Gastroenterol (2020) 20:77. doi: 10.1186/s12876-020-01223-0
16. Saha S, Al-Rifai RH, Saha S. Diabetes prevalence and mortality in COVID-19 patients: a systematic review, meta-analysis, and meta-regression. J Diabetes Metab Disord (2021) 20:939–50. doi: 10.1007/s40200-021-00779-2
17. Berbudi A, Rahmadika N, Tjahjadi AI, Ruslami R. Type 2 diabetes and its impact on the immune system. Curr Diabetes Rev (2020) 16:442–9. doi: 10.2174/1573399815666191024085838
18. Agoritsas T, Merglen A, Shah ND, O’Donnell M, Guyatt GH. Adjusted analyses in studies addressing therapy and harm. JAMA (2017) 317:748–59. doi: 10.1001/jama.2016.20029
19. Emdin CA, Khera AV, Kathiresan S. Mendelian randomization. JAMA (2017) 318:1925–6. doi: 10.1001/jama.2017.17219
20. Davey Smith G, Ebrahim S. ‘Mendelian randomization’: can genetic epidemiology contribute to understanding environmental determinants of disease? Int J Epidemiol (2003) 32:1–22. doi: 10.1093/ije/dyg070
21. Forgetta V, Manousaki D, Istomine R, Ross S, Tessier MC, Marchand L, et al. Rare genetic variants of large effect influence risk of type 1 diabetes. Diabetes (2020) 69:784–95. doi: 10.2337/db19-0831
22. Emmert DB, Stoehr PJ, Stoesser G, Cameron GN. The European bioinformatics institute (EBI) databases. Nucleic Acids Res (1994) 22:3445–9. doi: 10.1093/nar/22.17.3445
23. Sudlow C, Gallacher J, Allen N, Beral V, Burton P, Danesh J, et al. UK Biobank: An open access resource for identifying the causes of a wide range of complex diseases of middle and old age. PloS Med (2015) 12:e1001779. doi: 10.1371/journal.pmed.1001779
24. Locke AE, Steinberg KM, Chiang CWK, Service SK, Havulinna AS, Stell L, et al. Exome sequencing of Finnish isolates enhances rare-variant association power. Nature (2019) 572:323–8. doi: 10.1038/s41586-019-1457-z
25. Elsworth B, Lyon M, Alexander T, Liu Y, Matthews P, Hallett J, et al. The MRC IEU OpenGWAS data infrastructure. bioRxiv (2020). doi: 10.1101/2020.08.10.244293
26. Purcell S, Neale B, Todd-Brown K, Thomas L, Ferreira MAR, Bender D, et al. PLINK: A tool set for whole-genome association and population-based linkage analyses. Am J Hum Genet (2007) 81:559–75. doi: 10.1086/519795
27. Staiger D, Stock JH. Instrumental variables regression with weak instruments. Econometrica (1997) 65:557–86. doi: 10.2307/2171753
28. Kamat MA, Blackshaw JA, Young R, Surendran P, Burgess S, Danesh J, et al. PhenoScanner V2: an expanded tool for searching human genotype–phenotype associations. Bioinformatics (2019) 35:4851–3. doi: 10.1093/bioinformatics/btz469
29. Marín-Martínez F, Sánchez-Meca J. Weighting by inverse variance or by sample size in random-effects meta-analysis. Educ Psychol Meas (2009) 70:56–73. doi: 10.1177/0013164409344534
30. Milligan BG. Maximum-likelihood estimation of relatedness. Genetics (2003) 163:1153–67. doi: 10.1093/genetics/163.3.1153
31. He J, Luo X, Xin H, Lai Q, Zhou Y, Bai Y. The effects of fatty acids on inflammatory bowel disease: A two-sample mendelian randomization study. Nutrients (2022) 14:2883. doi: 10.3390/nu14142883
32. Bowden J, Davey Smith G, Haycock PC, Burgess S. Consistent estimation in mendelian randomization with some invalid instruments using a weighted median estimator. Genet Epidemiol (2016) 40:304–14. doi: 10.1002/gepi.21965
33. Burgess S, Thompson SG. Interpreting findings from mendelian randomization using the MR-egger method. Eur J Epidemiol (2017) 32:377–89. doi: 10.1007/s10654-017-0255-x
34. Bowden J, Spiller W, Del Greco MF, Sheehan N, Thompson J, Minelli C, et al. Improving the visualization, interpretation and analysis of two-sample summary data mendelian randomization via the radial plot and radial regression. Int J Epidemiol (2018) 47:1264–78. doi: 10.1093/ije/dyy101
35. Grant AJ, Burgess S. Pleiotropy robust methods for multivariable mendelian randomization. Stat Med (2021) 40:5813–30. doi: 10.1002/sim.9156
36. Winter-Jensen M, Afzal S, Jess T, Nordestgaard BG, Allin KH. Body mass index and risk of infections: a mendelian randomization study of 101,447 individuals. Eur J Epidemiol (2020) 35:347–54. doi: 10.1007/s10654-020-00630-7
37. Censin JC, Nowak C, Cooper N, Bergsten P, Todd JA, Fall T. Childhood adiposity and risk of type 1 diabetes: A mendelian randomization study. PloS Med (2017) 14:e1002362. doi: 10.1371/journal.pmed.1002362
38. Cheong HS, Chang Y, Kim Y, Joo EJ, Kwon MJ, Wild SD, et al. Glycaemic status, insulin resistance, and risk of infection-related mortality: a cohort study. Eur J Endocrinol (2023) 188:lvad011. doi: 10.1093/ejendo/lvad011
39. Verbanck M, Chen C-Y, Neale B, Do R. Detection of widespread horizontal pleiotropy in causal relationships inferred from mendelian randomization between complex traits and diseases. Nat Genet (2018) 50:693–8. doi: 10.1038/s41588-018-0099-7
40. Stock M, Pahikkala T, Airola A, Waegeman W, De Baets B. Algebraic shortcuts for leave-one-out cross-validation in supervised network inference. Brief Bioinform (2018) 21:262–71. doi: 10.1093/bib/bby095
41. Rizzo A, Paolillo R, Iafusco D, Prisco F, Romano Carratelli C. Chlamydia pneumoniae infection in adolescents with type 1 diabetes mellitus. J Med Microbiol (2012) 61:1584–90. doi: 10.1099/jmm.0.048512-0
42. Muller LM, Gorter KJ, Hak E, Goudzwaard WL, Schellevis FG, Hoepelman AI, et al. Increased risk of common infections in patients with type 1 and type 2 diabetes mellitus. Clin Infect Dis (2005) 41:281–8. doi: 10.1086/431587
43. Heald AH, Jenkins DA, Williams R, Sperrin M, Fachim H, Mudaliar RN, et al. The risk factors potentially influencing hospital admission in people with diabetes, following SARS-CoV-2 infection: A population-level analysis. Diabetes Ther (2022) 13:1007–21. doi: 10.1007/s13300-022-01230-2
44. Czaja CA, Rutledge BN, Cleary PA, Chan K, Stapleton AE, Stamm WE. Urinary tract infections in women with type 1 diabetes mellitus: survey of female participants in the epidemiology of diabetes interventions and complications study cohort. J Urol (2009) 181:1129–35. doi: 10.1016/j.juro.2008.11.021
45. Schofield H, Haycocks S, Robinson A, Edmonds M, Anderson SG, Heald AH. Mortality in 98 type 1 diabetes mellitus and type 2 diabetes mellitus: Foot ulcer location is an independent risk determinant. Diabetes Med (2021) 38:e14568. doi: 10.1111/dme.14568
46. Jiang L, Cheng M. Impact of diabetes mellitus on outcomes of patients with sepsis: an updated systematic review and meta-analysis. Diabetol Metab Syndr (2022) 14:39. doi: 10.1186/s13098-022-00803-2
47. Altobelli E, Petrocelli R, Verrotti A, Chiarelli F, Marziliano C. Genetic and environmental factors affect the onset of type 1 diabetes mellitus. Pediatr Diabetes (2016) 17:559–66. doi: 10.1111/pedi.12345
48. Jaidane H, Sauter P, Sane F, Goffard A, Gharbi J, Hober D. Enteroviruses and type 1 diabetes: towards a better understanding of the relationship. Rev Med Virol (2010) 20:265–80. doi: 10.1002/rmv.647
49. Abdellatif AM, Sarvetnick NE. Current understanding of the role of gut dysbiosis in type 1 diabetes. J Diabetes (2019) 11:632–44. doi: 10.1111/1753-0407.12915
50. Cho NH, Shaw JE, Karuranga S, Huang Y, da Rocha Fernandes JD, Ohlrogge AW, et al. IDF diabetes atlas: Global estimates of diabetes prevalence for 2017 and projections for 2045. Diabetes Res Clin Pract (2018) 138:271–81. doi: 10.1016/j.diabres.2018.02.023
51. Wang H, Guo Z, Zheng Y, Yu C, Hou H, Chen B. No causal relationship between T2DM and the risk of infectious diseases: A two-sample mendelian randomization study. Front Genet (2021) 12:720874. doi: 10.3389/fgene.2021.720874
52. Oikarinen M, Tauriainen S, Honkanen T, Oikarinen S, Vuori K, Kaukinen K, et al. Detection of enteroviruses in the intestine of type 1 diabetic patients. Clin Exp Immunol (2008) 151:71–5. doi: 10.1111/j.1365-2249.2007.03529.x
53. Oikarinen M, Tauriainen S, Oikarinen S, Honkanen T, Collin P, Rantala I, et al. Type 1 diabetes is associated with enterovirus infection in gut mucosa. Diabetes (2012) 61:687–91. doi: 10.2337/db11-1157
54. Paun A, Yau C, Danska JS. Immune recognition and response to the intestinal microbiome in type 1 diabetes. J Autoimmun (2016) 71:10–8. doi: 10.1016/j.jaut.2016.02.004
55. Claesson MH, Nicoletti F, Stosic-Grujicic S, Doria A, Zampieri S. Interactions between infections and immune-inflammatory cells in type 1 diabetes mellitus and inflammatory bowel diseases: evidences from animal models. Clin Exp Rheumatol (2008) 26:S8–11.
56. Salardi S, Volta U, Zucchini S, Fiorini E, Maltoni G, Vaira B, et al. Prevalence of celiac disease in children with type 1 diabetes mellitus increased in the mid-1990 s: an 18-year longitudinal study based on anti-endomysial antibodies. J Pediatr Gastroenterol Nutr (2008) 46:612–4. doi: 10.1097/MPG.0b013e31815d697e
57. Maki M, Hallstrom O, Huupponen T, Vesikari T, Visakorpi JK. Increased prevalence of coeliac disease in diabetes. Arch Dis Child (1984) 59:739–42. doi: 10.1136/adc.59.8.739
58. Goodwin G. Type 1 diabetes mellitus and celiac disease: Distinct autoimmune disorders that share common pathogenic mechanisms. Horm Res Paediatr (2019) 92:285–92. doi: 10.1159/000503142
59. Esposito S, Mariotti Zani E, Torelli L, Scavone S, Petraroli M, Patianna V, et al. Childhood vaccinations and type 1 diabetes. Front Immunol (2021) 12:667889. doi: 10.3389/fimmu.2021.667889
60. Al-Toma A, Volta U, Auricchio R, Castillejo G, Sanders DS, Cellier C, et al. European Society for the study of coeliac disease (ESsCD) guideline for coeliac disease and other gluten-related disorders. United Eur Gastroenterol J (2019) 7:583–613. doi: 10.1177/2050640619844125
61. Woolf B, Di Cara N, Moreno-Stokoe C, Skrivankova V, Drax K, Higgins JPT, et al. Investigating the transparency of reporting in two-sample summary data mendelian randomization studies using the MR-base platform. Int J Epidemiol (2022) 51:1943–56. doi: 10.1093/ije/dyac074
Glossary
Keywords: mendelian randomization, infections, intestinal, sepsis, lower respiratory, pregnancy, skin, urinary tract
Citation: Chen X-H, Liu H-Q, Nie Q, Wang H and Xiang T (2023) Causal relationship between type 1 diabetes mellitus and six high-frequency infectious diseases: A two-sample mendelian randomization study. Front. Endocrinol. 14:1135726. doi: 10.3389/fendo.2023.1135726
Received: 01 January 2023; Accepted: 21 March 2023;
Published: 31 March 2023.
Edited by:
Ping Wang, Department of Radiology, Michigan State University, United StatesReviewed by:
Lyubov E. Salnikova, Vavilov Institute of General Genetics (RAS), RussiaCosmin Mihai Vesa, University of Oradea, Romania
Copyright © 2023 Chen, Liu, Nie, Wang and Xiang. This is an open-access article distributed under the terms of the Creative Commons Attribution License (CC BY). The use, distribution or reproduction in other forums is permitted, provided the original author(s) and the copyright owner(s) are credited and that the original publication in this journal is cited, in accordance with accepted academic practice. No use, distribution or reproduction is permitted which does not comply with these terms.
*Correspondence: Tao Xiang, eHQxMTQyNzUyOTI5QDE2My5jb20=; Han Wang, d2FuZ2hhbkBzd2p0dS5lZHUuY24=