- 1Beijing Xiaotangshan Hospital, Beijing, China
- 2Tianjin Key Laboratory of Exercise Physiology and Sports Medicine, Institute of Sport, Exercise and Health, Tianjin University of Sport, Tianjin, China
Background: Cardiorespiratory fitness (CRF) is an established risk factor for cardiovascular diseases (CVD). Compared with traditional clinical risk factors for CVD, CRF can better predict health status and possible adverse events. However, few studies have reported the association between multiple metabolic health indicators and CRF as an indicator of CVD risk. Therefore, this study aims to further understand the association between metabolic health indicators and CRF and to provide a theoretical basis for improving the early prevention strategies of CVD in the urban young and middle-aged population.
Methods: A retrospective cross-sectional study was conducted on 889 young and middle-aged urban people who underwent health examinations in Beijing Xiaotangshan Hospital from January 2022 to December 2024. Baseline measurements of physical examination, biochemical examination, and cardiopulmonary exercise testing were obtained. The association between metabolic health indicators and CRF was analyzed. A multiple linear regression analysis was performed to assess the association between each metabolic health indicator and CRF and determine which metabolic health indicators may serve as useful predictors for assessing CRF.
Results: We investigated the association between metabolic health indicators and CRF by adjusting for covariates (age, smoking status, and drinking status) associated with CRF. In multiple linear regression analysis, waist circumference (WC) (β = −0.196, P = 0.010), fasting plasma glucose (FPG) (β = −0.143, P < 0.001), and high-density lipoprotein cholesterol (HDL-C) (β = −0.125, P = 0.005) were significantly associated with VO2peak in young and middle-aged urban men. WC (β = −0.577, P < 0.001) and FPG (β = −0.167, P = 0.002) were significantly associated with VO2peak in young and middle-aged urban women. In addition, WC (men: β = −0.238, P = 0.003; women: β = −0.410, P < 0.001) and FPG (men: β = −0.147, P < 0.001; women: β = −0.123, P = 0.034) were significantly associated with AT in men and women.
Conclusion: Our results showed that WC and FPG were significantly associated with CRF in young and middle-aged urban men and women. This suggests that WC and FPG may serve as useful predictors for assessing CRF within this population.
Introduction
Cardiorespiratory fitness (CRF), also known as cardiorespiratory endurance, refers to the ability of the circulatory system and respiratory system to supply oxygen to skeletal muscles during sustained physical activity, which is a comprehensive reflection of the body’s exercise capacity (1). CRF is not only an established risk factor for cardiovascular disease (CVD) but also one of the stronger, or perhaps strongest, predictors of prognosis in the overall population and in many groups with specific CVD risk factors or established CVD conditions (2). Studies have shown that low CRF is independently associated with a 2- to 4-fold increased risk of cardiovascular disease events even after adjustment for age, BMI, blood pressure, lipid profiles, and blood glucose levels, and its predictive power surpasses that of most traditional clinical risk factors for CVD (3, 4). It is more and more often underlined that CRF assessment should be included as part of routine diagnostic procedures in all health centers (5). Unfortunately, there has been a marked decline in CRF in adults since 1980, with the magnitude of the decline gradually increasing over time. Declines were larger for men than for women and for young adults (< 40 years) than for middle-aged adults (≥ 40 years), suggestive of a corresponding decline in population health (6).
Cardiopulmonary exercise testing (CPET) is an objective, quantitative, and non-invasive CRF assessment method (7). It can integrate and analyze the continuous dynamic changes of the respiratory, circulatory, blood, and metabolic system functions during exercise to assess overall health status and CVD risk (8). Important indicators such as peak oxygen uptake (VO2peak) and anaerobic threshold (AT) are derived from CPET. Specifically, measuring VO2peak (ml/min/kg) by CPET in the laboratory is the gold standard for objectively measuring CRF (9). VO2peak can reflect the upper limit of the overall function of the cardiopulmonary system and is a strong predictor of CVD mortality, while AT can reveal the dynamic balance between metabolic efficiency and cardiovascular health, offering targeted insights for early risk prediction and rehabilitation guidance. The combination of the two can more comprehensively assess CRF and CVD risk. Although CPET is the only method that can accurately measure VO2peak and AT, its popularity is low due to the need for professional equipment and certified medical personnel, the time required for a single test of 30 to 60 minutes, and the fact that it is performed at only a few medical centers (10). Therefore, finding a simpler method to estimate the VO2peak and AT is of great significance for the indirect assessment of CRF.
Several studies have found that low CRF is closely related to metabolic diseases (11–14), such as obesity, diabetes, hypertension, and other diseases. However, these studies are mostly limited to observing the effect of CRF on a certain metabolic disease, and few studies have explored which metabolic health indicators may serve as useful predictors for assessing CRF. So far, CVD remains the leading cause of death worldwide (15), and the prevention of CVD is considered a global public health priority (2). Therefore, our goal in this study was to analyze the association between metabolic health indicators and CRF in the urban young and middle-aged population and to provide a theoretical basis for improving the early prevention strategies of cardiovascular diseases in this population.
Methods
Participants
We conducted a retrospective cross-sectional study based on data obtained from medical records. We included young and middle-aged people aged 18–59 living in the Beijing urban area who underwent health examinations (including physical examination, biochemical examination, and CPET) in Beijing Xiaotangshan Hospital from January 2022 to December 2024. A total of 58 people were excluded based on the following criteria: (a) body mass index (BMI) < 18.5 kg/m2; (b) missing physical examination component data; (c) missing biochemical examination component data; (d) missing CPET component data; (e) unable to cooperate with health examination and sample collection. Ultimately, 889 participants (559 men and 330 women) were included in the study. G*power 3.1.9.7 was used for post-hoc power analysis of the multiple linear regression model, and all results showed power ≥ 0.8, indicating that the sample size was appropriate and the multiple linear regression model had sufficient statistical power (Supplementary Figure 1).
Throughout the study, we did not impose any interventions on participants and did not adversely affect their rights and health. At the same time, we always ensured that participants’ privacy and personal information were fully protected. Protocols used in this study were in accordance with International Ethical Guidelines (according to the Declaration of Helsinki) and approved by the Ethics Committee of Beijing Xiaotangshan Hospital (Approval No. 2024–24). All methods were carried out in accordance with relevant guidelines and regulations. In this study, patient consent was not required as it was approved with a waiver.
Anthropometric measurements
The general information of the participants was collected through a face-to-face diagnostic interview, including age, gender, marital status, smoking status, drinking status, and disease history. Weight and height were measured using a calibrated electronic scale while the participants were shoeless and wearing light clothing. BMI (kg/m2) was calculated as weight (kg) divided by height squared (m2). Waist circumference (WC) was taken midway between the lowest rib margin and the highest iliac crest at the end of expiration, and the maximum circumference around the buttocks while wearing thin clothing was taken as hip circumference (HC). Systolic blood pressure (SBP) and diastolic blood pressure (DBP) in both upper limbs of seated participants were measured using an Omron electronic sphygmomanometer (Omron Healthcare Co. Ltd., Kyoto, Japan), with the higher side as the measurement result, and heart rate (HR) was measured at the same time. The mean of two measurements separated by a 5-minute interval was taken as a valid determination of SBP, DBP, and HR.
Biochemical examination
Venous blood samples were obtained from the antecubital vein after an overnight fasting period of at least eight hours to measure fasting plasma glucose (FPG), total cholesterol (TC), triglyceride (TG), high-density lipoprotein cholesterol (HDL-C), and low-density lipoprotein cholesterol (LDL-C). These assays were performed using an automatic biochemistry analyzer (Roche Cobas C 710, supplied by Beijing Barry Medical Equipment Co., Ltd., Beijing, China) according to the manufacturer’s instructions.
Cardiorespiratory fitness
We used the Quark PFT Ergo cardiopulmonary exercise testing system produced by COSMED, Italy, to perform CPET to assess CRF. Participants tried to avoid alcohol, tea, or caffeinated beverages for three days before the test. Before the test, the tester had to ask the participants to sign an informed consent form, which included informing them of the purpose, implementation process, and precautions of CPET and reminding them of the potential discomfort and risks related to exercise. Before the test, the instrument’s gas, capacity, and flow rate were calibrated. Then, the testers transferred the participants safely to the cycle ergometer, adjusted the height suitable for pedaling, connected to the 12-lead electrocardiogram, and placed the cuff on the participants for automatic blood pressure detection and the mask for respiratory gas analysis. The tester set the power increment rate of the cycle ergometer according to the participant’s age, gender, and estimated functional status so that they could reach the symptom-limiting maximum extreme exercise within 6 to 10 minutes. During the whole exercise process, the testers closely monitored the status of the participants (16). Finally, we selected peak oxygen uptake (VO2peak) and anaerobic threshold (AT) as our research indicators.
Metabolic syndrome and its components
The diagnosis of metabolic syndrome (MS) and its components is based on the diagnostic criteria of the guideline for the prevention and treatment of type 2 diabetes mellitus in China (2020 edition) (17). Three or more of the following five components can be diagnosed (1): Abdominal obesity: male WC ≥ 90 cm; female WC ≥ 85 cm; (2) Hyperglycemia: FPG ≥ 6.1 mmol/L or 2-hour postprandial blood glucose ≥ 7.8 mmol/L and/or diabetes has been diagnosed and treated; (3) Hypertension: blood pressure ≥ 130/85 mmHg (1 mmHg = 0.133 kPa) and (or) hypertension has been confirmed and treated; (4) Fasting TG ≥ 1.70 mmol/L; and (5) Fasting HDL-C < 1.04 mmol/L.
Statistical analysis
Shapiro-Wilk test and histograms were used to detect the types of distributions of continuous variables. Continuous variables conforming to the normal distribution were described as mean ± standard deviation (SD), otherwise described as median and interquartile range. Categorical variables were described as frequencies (proportions). For continuous variables with a normal distribution, one-way ANOVA was used to compare multiple groups. For continuous variables with a skewed distribution, the Kruskal-Wallis H test was used to compare multiple groups. To correct for multiple testing, the Bonferroni correction was used. The chi-square test was used to compare the categorical variables. The Pearson or Spearman correlation coefficients were calculated to determine the association between metabolic health indicators and CRF. Multiple linear regression models were used to estimate the beta coefficients (β) of different metabolic health indicators on the VO2peak values. Different models were applied: Model I was crude without any adjustment, and Model II was adjusted for age, smoking status, and drinking status. The collinearity of the variables in the models was assessed by calculating the variance inflation factor (VIF). Considering the difference between metabolic health indicators and CRF in men and women, the participants were divided into two groups according to sex, and the data were analyzed separately. Statistical analyses were performed using SPSS 27.0 (IBM Corp., Armonk, NY, USA). Differences were considered statistically significant at P < 0.05.
Results
Retrospective cross-sectional data were available for 889 participants. The participants consisted of 559 men (62.88%) and 330 women (37.12%), with a mean age of 42.17 years (23–56 years). Regarding metabolic health indicators, SBP, DBP, BMI, WC, FPG, TG, HDL-C, and LDL-C significantly differed between men and women (P < 0.05). Regarding CRF, VO2peak was significantly higher in men than women (P < 0.001). In terms of health-related behaviors, smoking and drinking rates were significantly higher in men than women (P < 0.001). The demographic and clinical characteristics of the study participants were summarized in Table 1.
The Pearson or Spearman correlation coefficient was calculated to analyze the association between metabolic health indicators and CRF (Table 2, Figure 1). In males, VO2peak was negatively correlated with SBP, DBP, BMI, WC, FPG, TC, and TG (P < 0.05), and positively correlated with HDL-C (P < 0.05). AT was negatively correlated with SBP, DBP, BMI, WC, FPG, and TG (P < 0.05), and positively correlated with HDL-C (P < 0.05). In females, VO2peak was negatively correlated with SBP, DBP, BMI, WC, FPG, TG, and LDL-C (P < 0.05), and positively correlated with HDL-C (P < 0.05). AT was negatively correlated with SBP, DBP, BMI, WC, FPG, TC, TG, and LDL-C (P < 0.05), and positively correlated with HDL-C (P < 0.05).
In the multiple linear regression model, VO2peak and AT were used as dependent variables, and metabolic health indicators were used as independent variables. Variables with no correlation or with multicollinearity were not included in the regression model. Finally, we analyzed the predictive effects of SBP, DBP, BMI, WC, FPG, TC, TG, and HDL-C on VO2peak and AT in males and females, respectively (Tables 3, 4). Model I was crude without any adjustment, and Model II was adjusted for age, smoking status, and drinking status.
In the male-adjusted model with VO2peak as the dependent variable, the P-P plot showed that the residuals were approximately normally distributed, the degree of multicollinearity among the variables was low (all VIFs < 5; Tolerance > 0.2) (Supplementary Table 1), and the multiple linear regression model was statistically significant (adjusted R2 = 0.187, F = 15.238, P < 0.001). Among the variables included in the model, WC (β = −0.196, 95% CI: −0.195 to −0.026, P = 0.010), FPG (β = −0.143, 95% CI: −1.101 to −0.317, P < 0.001), and HDL-C (β = 0.125, 95% CI: 0.539 to 3.039, P = 0.005) had statistically significant effects on VO2peak. In the male-adjusted model with AT as the dependent variable, the P-P plot showed that the residuals were approximately normally distributed, the degree of multicollinearity among the variables was low (all VIFs < 5; Tolerance > 0.2) (Supplementary Table 2), and the multiple linear regression model was statistically significant (adjusted R2 = 0.122, F = 9.605, P < 0.001). Among the variables included in the model, WC (β = −0.238, 95% CI: −0.126 to −0.026, P = 0.003) and FPG (β = −0.147, 95% CI: −0.647 to −0.183, P < 0.001) had statistically significant effects on AT.
In the female-adjusted model with VO2peak as the dependent variable, the P-P plot showed that the residuals were approximately normally distributed, the degree of multicollinearity among the variables was low (all VIFs < 6; Tolerance > 0.2) (Supplementary Table 1), and the multiple linear regression model was statistically significant (adjusted R2 = 0.412, F = 26.612, P < 0.001). Among the variables included in the model, WC (β = −0.577, 95% CI: −0.334 to −0.164, P < 0.001) and FPG (β = −0.167, 95% CI: −2.026 to −0.459, P = 0.002) had statistically significant effects on VO2peak. In the female-adjusted model with AT as the dependent variable, the P-P plot showed that the residuals were approximately normally distributed, the degree of multicollinearity among the variables was low (all VIFs < 6; Tolerance > 0.2) (Supplementary Table 2), and the multiple linear regression model was statistically significant (adjusted R2 = 0.310, F = 17.399, P < 0.001). Among the variables included in the model, WC (β = −0.410, 95% CI: −0.183 to −0.057, P < 0.001) and FPG (β = −0.123, 95% CI: −1.200 to −0.047, P = 0.034) had statistically significant effects on AT.
In this study, the Kruskal-Wallis H test was used to analyze the influence of the number of MS components on CRF in the urban young and middle-aged population (Table 5, Figure 2), and Bonferroni correction was used for pairwise comparison between the two groups (Supplementary Figure 2). In males, participants with 2 or ≥ 3 MS components had lower VO2peak and AT than those with 1 or 0 MS components (Adj. P < 0.05). In females, participants with 1, 2 or ≥ 3 MS components had lower VO2peak and AT than those with 0 MS components (Adj. P < 0.05). When the number of MS components was ≥ 3, VO2peak and AT were lower than those in participants with 1 MS component (Adj. P < 0.05).
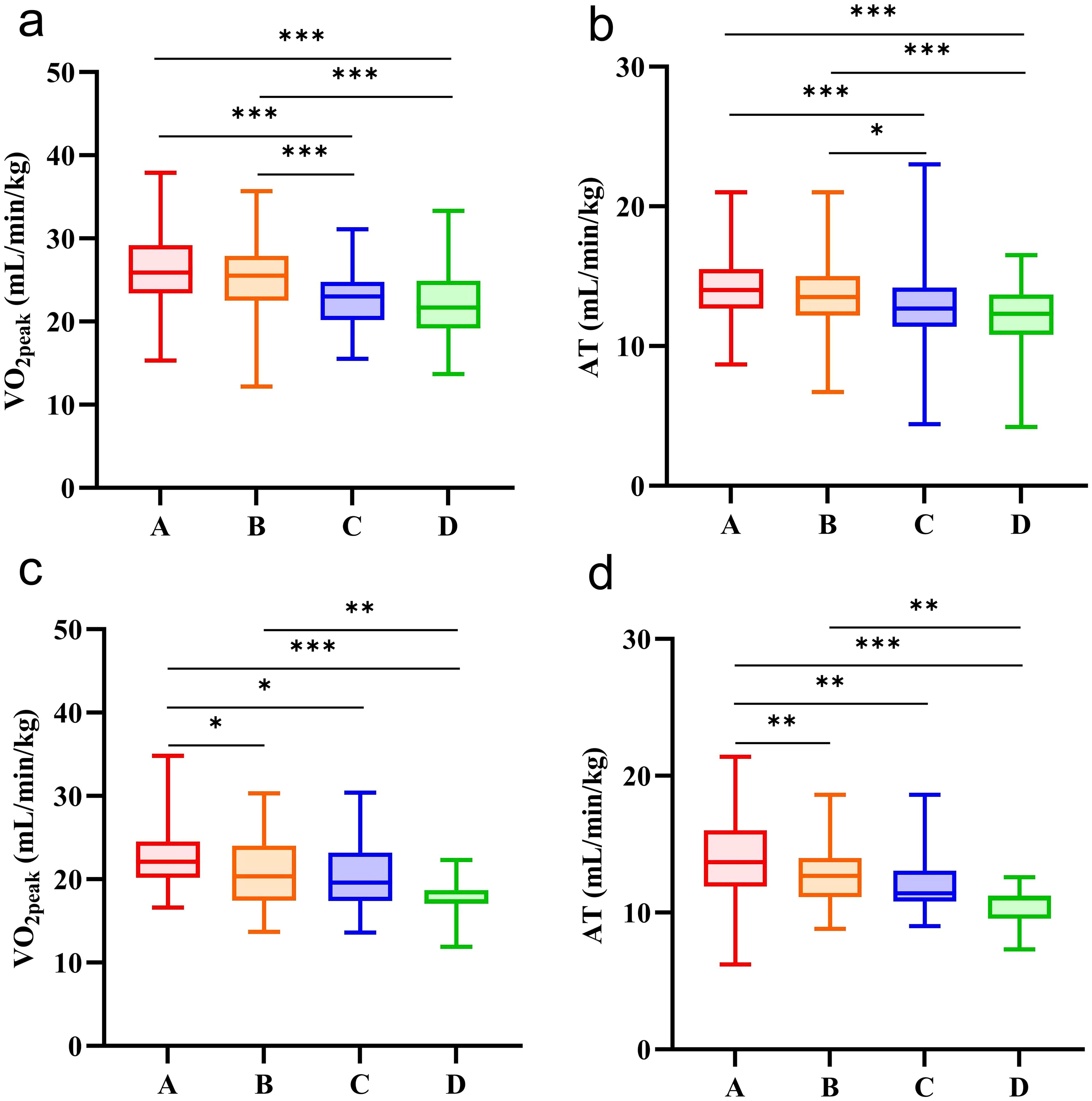
Figure 2. CRF in participants with different numbers of MS components. (a) comparison of VO2peak in male participants with different numbers of MS components. (b) comparison of AT in male participants with different numbers of MS components. (c) comparison of VO2peak in female participants with different numbers of MS components. d, comparison of AT in female participants with different numbers of MS components. A the number of MS components (n = 0); B the number of MS components (n = 1); C the number of MS components (n = 2); D the number of MS components (n ≥ 3). *, the comparison between the two groups was statistically significant (Adj. P < 0.05). **the comparison between the two groups was statistically significant (Adj. P < 0.01). ***the comparison between the two groups was statistically significant (Adj. P < 0.001).
Discussion
Low CRF in adults is associated with greater risks for congestive heart failure, stroke, type 2 diabetes mellitus, some cancers, and neuropsychological disturbances (e.g., dementia, anxiety, and depression) (18, 19). Most importantly, improvements in CRF over time are associated with reduced mortality and morbidity. This provides a clear signal that having higher levels of CRF is essential for maintaining population health (20). The assessment of CRF requires a standardized CPET in the laboratory. Compared with traditional metabolic indicators, CPET can provide a more comprehensive physiological function assessment and earlier risk warning. However, CPET needs to be equipped with a gas metabolism analyzer, electrocardiogram monitoring system, etc., which not only incurs high costs but also requires a strict standardization process, making it difficult to popularize in grassroots hospitals, which limits the wide application to a certain extent (16). The traditional metabolic health indicators are easy to obtain through routine physical examination, with low cost and high penetration rate (21). So far, few studies have reported the association between multiple metabolic health indicators and CRF as an indicator of CVD risk. Therefore, we bridged this gap through this study and finally found that WC and FPG may serve as useful predictors for assessing CRF in young and middle-aged urban men and women. We propose a strategy of combining metabolic health indicators with CPET: traditional indicators (such as WC and FPG) for primary screening and CPET for in-depth assessment in high-risk populations to optimize the CVD risk stratification model.
Obesity has increasingly become one of the important problems that seriously threaten human physical and mental health in the 21st century. There is a large amount of epidemiological evidence that there is a robust negative correlation between obesity and CRF, and this association still exists after adjusting for the level of physical activity (6, 22). BMI (kg/m2) is calculated as weight (kg) divided by height squared (m2), which is one of the standards commonly used in international clinical practice to measure the degree of obesity (23). However, some studies have pointed out that BMI is an imperfect measure (24), and it cannot excellently assess fat distribution and the cardiometabolic risk associated with increased obesity (25). As a special form of obesity, abdominal obesity is closely related to the morbidity and mortality risk of CVD (26). WC is a simple method to assess abdominal obesity that is easy to standardize and clinically apply (25), and to some extent, it can make up for the deficiency that BMI cannot assess fat distribution (27). A cross-sectional study of 807 men and 633 women in Finland found that individuals with the same BMI category and normal WC had better CRF than those with higher WC (22). A cross-sectional study that only included young men showed that CRF was more closely related to WC than BMI (28). This study found that WC was negatively correlated with VO2peak and AT in young and middle-aged urban men and women. Multiple linear regression analysis showed that WC was one of the important negative factors of VO2peak and AT in the urban young and middle-aged population. These studies have shown that WC, as a simple assessment tool in clinical practice, may serve as a useful predictor for assessing CRF levels and CVD risk.
Diabetes mellitus (DM) is a group of metabolic diseases characterized by hyperglycemia resulting from defects in insulin secretion, insulin action, or both (29). Type 2 diabetes mellitus (T2DM) is the most common DM worldwide, accounting for nearly 90% of DM prevalent cases (30). Because FPG is minimally disturbed by diet (31), it is considered an important reference tool for judging blood glucose levels and diagnosing diabetes compared with random blood glucose levels (32). This study found that FPG was negatively correlated with VO2peak and AT in young and middle-aged urban men and women. Multiple linear regression analysis showed that FPG was one of the important negative factors of VO2peak and AT in the urban young and middle-aged population. Recent studies have also shown that DM has a significant adverse effect on CRF. Although this effect is partially mitigated in people with high physical activity levels, it is still significant. In addition, CRF impairment is greater in patients with T2DM compared with type 1 diabetes mellitus (T1DM) (33). In patients with T2DM, CRF impairment is more significant in women than in men, with similar results in adolescents (34). Many factors, such as insulin resistance, skeletal muscle and microvascular system dysfunction, may lead to this phenomenon. As a vasoactive hormone, insulin has been shown to increase skeletal and myocardial perfusion in rodents and healthy humans (35–37), and this perfusion response is attenuated in insulin-resistant states (38, 39). In addition, studies have found that microvascular blood flow is significantly reduced in insulin-resistant animal models and human participants (40). These studies have demonstrated the role of insulin in the heart, skeletal muscle, and microvascular system, and insulin is one of the important factors affecting CRF. In addition to insulin’s role in regulating perfusion, hyperglycemia can also lead to the production of advanced glycation end products (AGEs) (41). These accrue in the wall of vessels, leading to integral loss of the structure of the vessel wall and underlying basement membrane, as well as proinflammatory signaling contributing to systemic vascular dysfunction, which may also lead to decreased CRF (42–44). To sum up, these findings show that high blood glucose can significantly reduce CRF and increase the risk of CVD, especially for people with T2DM, and also suggest that people with high blood glucose should regularly carry out exercise rehabilitation training to maintain or improve their CRF.
HDL-C is generally described as “good cholesterol”, which can participate in the reverse transport of cholesterol, has antioxidant, anti-inflammatory, and anti-thrombotic properties (45), and its anti-atherosclerotic function is vital in promoting cardiovascular health. Liu et al. (46) reported a positive association between CRF and HDL-C in men after adjustment for age, smoking status, and drinking status. Musa et al. (47) reported similar findings. In addition, HDL-C has been demonstrated to be a reliable and consistent predictor of CVD independent of other risk factors (48, 49). However, current epidemiological studies have shown that in CVD patients, the association between HDL-C concentrations and all-cause mortality exhibited a U-shaped association for both men and women, with both extremely high and low concentrations being associated with high all-cause mortality risk (50, 51). Moreover, niacin (52) and cholesteryl ester transfer protein inhibitors (53) increased HDL-C but did not show clinical benefit. This study found a positive correlation between CRF and HDL-C in urban young and middle-aged men. Further multiple linear regression analysis showed that higher levels of HDL-C were significantly associated with CRF. However, based on the above studies, we believe that the association between HDL-C and CRF should not be prematurely concluded, and further exploration is needed in the future. At the same time, we also found that HDL-C had no significant effect on VO2peak in multiple linear regression analysis of young and middle-aged women in urban areas. The possible reasons include (1): Higher estrogen levels in females may partially replace the protective effect of HDL-C by enhancing vascular endothelial function and anti-inflammatory effects, thus weakening the direct association between HDL-C and VO2peak (54) (2). Regarding fat distribution, compared with men, women have a higher proportion of subcutaneous fat and lower metabolic activity, which may reduce the association between HDL-C and visceral fat inflammation and weaken the contribution of HDL-C to VO2peak (55) (3). Regarding HDL particle characteristics, male HDL particles are smaller and have stronger anti-inflammatory and antioxidant capacities, which directly supports the efficiency of oxygen transport during exercise, while female HDL particles exhibit the opposite characteristics (56).
The number of MS components is significant in evaluating the severity of metabolic disorders, so we analyzed the relationship between the number of MS components and CRF. The results showed that CRF decreased significantly with the increase in the number of MS components (especially when the number of male MS components was 2 or ≥ 3 or the number of female MS components was 1, 2, or ≥ 3). This result indicates that a variety of metabolic disorders may be involved in the decline of CRF, including obesity, hyperglycemia, hypertension, hyperlipidemia, etc., and these components may have a superimposed effect on each other. Particular attention should be paid when there is abdominal obesity or hyperglycemia, especially when the two coexist because we found that WC and FPG were the most significant factors affecting CRF in metabolic health indicators through multiple linear regression analysis. Therefore, the results of this study can be used to develop individualized intervention programs in clinical practice to reduce the severity of metabolic disorders and optimize CRF, thereby reducing the risk of CVD.
This study found that WC and FPG may serve as useful predictors for assessing CRF in young and middle-aged urban men and women. However, it should be emphasized that the bidirectional association between CRF and metabolic risk factors has been well established (57). The existing evidence demonstrates a dynamic interplay between the two: on the one hand, metabolic dysfunction may impair CRF through mechanisms such as insulin resistance and mitochondrial dysfunction (34); on the other hand, the improvement of CRF can improve metabolic indicators by ameliorating vascular endothelial function and augmenting skeletal muscle oxidative capacity (58). Despite this bidirectional association, our findings retain significant implications (1): In primary care, WC and FPG measurements could serve as practical screening tools for identifying individuals with low CRF, enabling early intervention (2). This study suggests that future intervention trials could target these specific metabolic health indicators for combined intervention (such as WC reduction combined with FPG control) to explore whether this method is more effective than exercise intervention alone in improving CRF (3). From a clinical point of view, the results of this study highlight the necessity of a dual targeting strategy: both using metabolic health indicators for CRF risk stratification and breaking the vicious cycle of metabolic deterioration by enhancing CRF.
The study also has some limitations. First, this is a retrospective cross-sectional study, so we only report correlations and cannot draw further conclusions about causation. Second, the number of participants in this study is small, and participants are limited to one geographic area. Therefore, the results of this study may not be generalized to rural populations or ethnically diverse groups. In the future, we need to expand the geographical area and collect more data to verify the consistency of the research conclusions in different regions. Third, as a retrospective cross-sectional study, this study failed to determine the required sample size based on a priori power analysis. Although the test power is evaluated by post-hoc power analysis, the estimation of effect size in retrospective design may be interfered with selection bias and uncontrolled confounding factors, resulting in bias in the evaluation of statistical power. Finally, the CRF of all participants was measured by a cycle ergometer, but the CRF measured by a cycle ergometer may be 5–10% lower than the CRF measured by treadmill testing (59), which makes it difficult to reflect the limit of cardiopulmonary fitness. However, the load of the cycle ergometer is controllable, the data is stable, and the applicable population is wider (such as those with insufficient motor skills, lower limb joint injury, and poor coordination), so it is the first choice.
Conclusion
Our results showed that WC and FPG were significantly associated with CRF in young and middle-aged urban men and women. This suggests that WC and FPG may serve as useful predictors for assessing CRF within this population. In the future, further studies with a larger sample size of participants are needed to determine the validity of our findings.
Data availability statement
The original contributions presented in the study are included in the article/Supplementary Material. Further inquiries can be directed to the corresponding authors.
Ethics statement
The studies involving humans were approved by the Ethics Committee of Beijing Xiaotangshan Hospital. The studies were conducted in accordance with the local legislation and institutional requirements. The participants provided their written informed consent to participate in this study.
Author contributions
CW: Writing – original draft. NY: Writing – original draft. QZ: Project administration, Writing – review & editing. YL: Project administration, Writing – review & editing. LZ: Methodology, Writing – review & editing. ZL: Methodology, Writing – review & editing. RZ: Data curation, Writing – review & editing. JQ: Data curation, Writing – review & editing. AX: Data curation, Writing – review & editing. YF: Validation, Writing – review & editing. ZX: Validation, Writing – review & editing. LW: Supervision, Writing – review & editing. JS: Supervision, Writing – review & editing. DH: Supervision, Writing – review & editing.
Funding
The author(s) declare that financial support was received for the research and/or publication of this article. This work was supported by the Beijing Municipal Administration of Hospitals Incubating Program (Code: PX2024074).
Acknowledgments
The authors thank all the study participants for their kind participation and cooperation.
Conflict of interest
The authors declare that the research was conducted in the absence of any commercial or financial relationships that could be construed as a potential conflict of interest.
Publisher’s note
All claims expressed in this article are solely those of the authors and do not necessarily represent those of their affiliated organizations, or those of the publisher, the editors and the reviewers. Any product that may be evaluated in this article, or claim that may be made by its manufacturer, is not guaranteed or endorsed by the publisher.
Supplementary material
The Supplementary Material for this article can be found online at: https://www.frontiersin.org/articles/10.3389/fendo.2025.1489152/full#supplementary-material
References
1. Raghuveer G, Hartz J, Lubans DR, Takken T, Wiltz JL, Mietus-Snyder M, et al. Cardiorespiratory fitness in youth: an important marker of health: A scientific statement from the american heart association. Circulation. (2020) 142:e101–18. doi: 10.1161/CIR.0000000000000866
2. Lavie CJ, Ozemek C, Carbone S, Katzmarzyk PT, and Blair SN. Sedentary behavior, exercise, and cardiovascular health. Circ Res. (2019) 124:799–815. doi: 10.1161/CIRCRESAHA.118.312669
3. Mandsager K, Harb S, Cremer P, Phelan D, Nissen SE, and Jaber W. Association of cardiorespiratory fitness with long-term mortality among adults undergoing exercise treadmill testing. JAMA Netw Open. (2018) 1:e183605. doi: 10.1001/jamanetworkopen.2018.3605
4. Myers J, de Souza E Silva CG, Arena R, Kaminsky L, Christle JW, Busque V, et al. Comparison of the FRIEND and wasserman-hansen equations in predicting outcomes in heart failure. J Am Heart Assoc. (2021) 10:e021246. doi: 10.1161/JAHA.121.021246
5. Ross R and Myers J. Cardiorespiratory fitness and its place in medicine. Rev Cardiovasc Med. (2023) 24:14. doi: 10.31083/j.rcm2401014
6. Lamoureux NR, Fitzgerald JS, Norton KI, Sabato T, Tremblay MS, and Tomkinson GR. Temporal trends in the cardiorespiratory fitness of 2,525,827 adults between 1967 and 2016: A systematic review. Sports Med. (2019) 49:41–55. doi: 10.1007/s40279-018-1017-y
7. Chinese Society of Cardiology, Chinese Medical Association, Professional Committee of Cardiopulmonary Prevention and Rehabilitation of Chinese Rehabilitation Medical Association, and Editorial Board of Chinese Journal of Cardiology. Chinese expert consensus on exercise rehabilitation of stab le angina pectoris. Zhonghua Xin Xue Guan Bing Za Zhi. (2023) 51:1033–42. doi: 10.3760/cma.j.cn112148-20230814-00076
8. Herdy AH, Ritt LEF, Stein R, de Araújo CGS, Milani M, RS M, et al. Cardiopulmonary exercise test: background, applicability and interpretation. Arq Bras Cardiol. (2016) 107:467–81. doi: 10.5935/abc.20160171
9. Ross RM. ATS/ACCP statement on cardiopulmonary exercise testing. Am J Respir Crit Care Med. (2003) 167:1451. doi: 10.1164/ajrccm.167.10.950
10. Koen M, Kubota Y, Tokita M, Kato K, Takahashi H, Akutsu K, et al. Relationship of maximum walking speed with peak oxygen uptake and anaerobic threshold in male patients with heart failure. Heart Vessels. (2023) 38:1344–55. doi: 10.1007/s00380-023-02289-y
11. Zhao Y, Qie R, Han M, Huang S, Wu X, Zhang Y, et al. Independent and joint associations of non-exercise cardiorespiratory fitness and obesity with risk of type 2 diabetes mellitus in the Rural Chinese Cohort Study. Nutr Metab Cardiovasc Dis. (2022) 32:929–36. doi: 10.1016/j.numecd.2022.01.005
12. Sloan RA, Kim Y, Kenyon J, Visentini-Scarzanella M, Sawada SS, Sui X, et al. Association between estimated cardiorespiratory fitness and abnormal glucose risk: A cohort study. J Clin Med. (2023) 12:2740. doi: 10.3390/jcm12072740
13. Zhao Y, Fu X, Ke Y, Wu Y, Qin P, Hu F, et al. Independent and joint associations of estimated cardiorespiratory fitness and its dynamic changes and obesity with the risk of hypertension: A prospective cohort. J Hum Hypertens. (2024) 38:413–9. doi: 10.1038/s41371-024-00910-9
14. Patel PH, Gates M, Kokkinos P, Lavie CJ, Zhang J, and Sui X. Non-exercise estimated cardiorespiratory fitness and incident hypertension. Am J Med. (2022) 135:906–14. doi: 10.1016/j.amjmed.2022.01.048
15. Roth GA, Mensah GA, Johnson CO, Addolorato G, Ammirati E, Baddour LM, et al. Global burden of cardiovascular diseases and risk factors, 1990–2019. J Am Coll Cardiol. (2020) 76:2982–3021. doi: 10.1016/j.jacc.2020.11.010
16. Balady GJ, Arena R, Sietsema K, Myers J, Coke L, Fletcher GF, et al. Clinician’s Guide to cardiopulmonary exercise testing in adults: a scientific statement from the American Heart Association. Circulation. (2010) 122:191–225. doi: 10.1161/CIR.0b013e3181e52e69
17. Chinese Elderly Type 2 Diabetes Prevention and Treatment of Clinical Guidelines Writing Group, Geriatric Endocrinology and Metabolism Branch of Chinese Geriatric Society, Geriatric Endocrinology and Metabolism Branch of Chinese Geriatric Health Care Society, Geriatric Professional Committee of Beijing Medical Award Foundation, and National Clinical Medical Research Center for Geriatric Diseases (PLA General Hospital). Clinical guidelines for prevention and treatment of type 2 diabetes mellitus in the elderly in China (2022 edition). Zhonghua Nei Ke Za Zhi. (2022) 61:12–50. doi: 10.3760/cma.j.cn112138-20211027-00751
18. Kodama S, Saito K, Tanaka S, Maki M, Yachi Y, Asumi M, et al. Cardiorespiratory fitness as a quantitative predictor of all-cause mortality and cardiovascular events in healthy men and women: a meta-analysis. JAMA. (2009) 301:2024–35. doi: 10.1001/jama.2009.681
19. Benjamin EJ, Muntner P, Alonso A, Bittencourt MS, Callaway CW, Carson AP, et al. Heart disease and stroke statistics-2019 update: A report from the american heart association. Circulation. (2019) 139:e56-e528. doi: 10.1161/CIR.0000000000000659
20. Ehrman JK, Brawner CA, Al-Mallah MH, Qureshi WT, Blaha MJ, and Keteyian SJ. Cardiorespiratory fitness change and mortality risk among black and white patients: henry ford exercise testing (FIT) project. Am J Med. (2017) 130:1177–83. doi: 10.1016/j.amjmed.2017.02.036
21. Wilson PW, D’Agostino RB, Levy D, Belanger AM, Silbershatz H, and Kannel WB. Prediction of coronary heart disease using risk factor categories. Circulation. (1998) 97:1837–47. doi: 10.1161/01.cir.97.18.1837
22. Duvigneaud N, Matton L, Wijndaele K, Deriemaeker P, Lefevre J, Philippaerts R, et al. Relationship of obesity with physical activity, aerobic fitness and muscle strength in Flemish adults. J Sports Med Phys Fitness (2008) 48:201–10. doi: 10.1016/j.ijms.2008.01.003
23. Suwała S and Junik R. Body mass index and waist circumference as predictors of above-average increased cardiovascular risk assessed by the SCORE2 and SCORE2-OP calculators and the proposition of new optimal cut-off values: cross-sectional single-center study. J Clin Med. (2024) 13:1931. doi: 10.3390/jcm13071931
24. Prillaman M. Why BMI is flawed - and how to redefine obesity. Nature. (2023) 622:232–3. doi: 10.1038/d41586-023-03143-x
25. Ross R, Neeland IJ, Yamashita S, Shai I, Seidell J, Magni P, et al. Waist circumference as a vital sign in clinical practice: a Consensus Statement from the IAS and ICCR Working Group on Visceral Obesity. Nat Rev Endocrinol. (2020) 16:177–89. doi: 10.1038/s41574-019-0310-7
26. Jun JE, Kang M, Jin S-M, Kim K, Hwang Y-C, Jeong I-K, et al. Additive effect of low skeletal muscle mass and abdominal obesity on coronary artery calcification. Eur J Endocrinol. (2021) 184:867–77. doi: 10.1530/EJE-20-0885
27. Wang S, Shi S, Huang Y, Huang H, and Zhong VW. Severity of abdominal obesity and cardiometabolic diseases in US adults. Public Health. (2024) 227:154–62. doi: 10.1016/j.puhe.2023.12.010
28. Fogelholm M, Malmberg J, Suni J, Santtila M, Kyröläinen H, and Mäntysaari M. Waist circumference and BMI are independently associated with the variation of cardio-respiratory and neuromuscular fitness in young adult men. Int J Obes (Lond). (2006) 30:962–9. doi: 10.1038/sj.ijo.0803243
29. American Diabetes Association. Diagnosis and classification of diabetes mellitus. Diabetes Care. (2014) 37 Suppl 1:S81–90. doi: 10.2337/dc14-S081
30. Ahmad E, Lim S, Lamptey R, Webb DR, and Davies MJ. Type 2 diabetes. Lancet. (2022) 400:1803–20. doi: 10.1016/S0140-6736(22)01655-5
31. Moebus S, Göres L, Lösch C, and Jöckel KH. Impact of time since last caloric intake on blood glucose levels. Eur J Epidemiol. (2011) 26:719–28. doi: 10.1007/s10654-011-9608-z
32. American Diabetes Association. Standards of medical care in diabetes–2014. Diabetes Care. (2014) 37 Suppl 1:S14–80. doi: 10.2337/dc14-S014
33. Alvares TS, Souza LVM DE, Soares RN, and Lessard SJ. Cardiorespiratory fitness is impaired in type 1 and type 2 diabetes: A systematic review, meta-analysis, and meta-regression. Med Sci Sports Exerc. (2024) 56:1553–62. doi: 10.1249/MSS.0000000000003451
34. Abushamat LA, McClatchey PM, Scalzo RL, Schauer I, Huebschmann AG, Nadeau KJ, et al. Mechanistic causes of reduced cardiorespiratory fitness in type 2 diabetes. J Endocr Soc. (2020) 4:bvaa063. doi: 10.1210/jendso/bvaa063
35. Barrett EJ, Wang H, Upchurch CT, and Liu Z. Insulin regulates its own delivery to skeletal muscle by feed-forward actions on the vasculature. Am J Physiol Endocrinol Metab. (2011) 301:E252–263. doi: 10.1152/ajpendo.00186.2011
36. Liu Z, Liu J, Jahn LA, Fowler DE, and Barrett EJ. Infusing lipid raises plasma free fatty acids and induces insulin resistance in muscle microvasculature. J Clin Endocrinol Metab. (2009) 94:3543–9. doi: 10.1210/jc.2009-0027
37. Kusters YHAM and Barrett EJ. Muscle microvasculature’s structural and functional specializations facilitate muscle metabolism. Am J Physiol Endocrinol Metab. (2016) 310:E379–387. doi: 10.1152/ajpendo.00443.2015
38. Baron AD, Tarshoby M, Hook G, Lazaridis EN, Cronin J, Johnson A, et al. Interaction between insulin sensitivity and muscle perfusion on glucose uptake in human skeletal muscle: evidence for capillary recruitment. Diabetes. (2000) 49:768–74. doi: 10.2337/diabetes.49.5.768
39. Clerk LH, Vincent MA, Barrett EJ, Lankford MF, and Lindner JR. Skeletal muscle capillary responses to insulin are abnormal in late-stage diabetes and are restored by angiotensin-converting enzyme inhibition. Am J Physiol Endocrinol Metab. (2007) 293:E1804–1809. doi: 10.1152/ajpendo.00498.2007
40. McClatchey PM, Williams IM, Xu Z, Mignemi NA, Hughey CC, McGuinness OP, et al. Perfusion controls muscle glucose uptake by altering the rate of glucose dispersion in vivo. Am J Physiol Endocrinol Metab. (2019) 317:E1022–36. doi: 10.1152/ajpendo.00260.2019
41. Vlassara H and Uribarri J. Advanced glycation end products (AGE) and diabetes: cause, effect, or both? Curr Diabetes Rep. (2014) 14:453. doi: 10.1007/s11892-013-0453-1
42. Yan SF, Ramasamy R, and Schmidt AM. The RAGE axis: a fundamental mechanism signaling danger to the vulnerable vasculature. Circ Res. (2010) 106:842–53. doi: 10.1161/CIRCRESAHA.109.212217
43. Huebschmann AG, Regensteiner JG, Vlassara H, and Reusch JEB. Diabetes and advanced glycoxidation end products. Diabetes Care. (2006) 29:1420–32. doi: 10.2337/dc05-2096
44. Avogaro A, Fadini GP, Gallo A, Pagnin E, and de Kreutzenberg S. Endothelial dysfunction in type 2 diabetes mellitus. Nutr Metab Cardiovasc Dis. (2006) 16 Suppl 1:S39–45. doi: 10.1016/j.numecd.2005.10.015
45. Toth PP, Barter PJ, Rosenson RS, Boden WE, Chapman MJ, Cuchel M, et al. High-density lipoproteins: a consensus statement from the National Lipid Association. J Clin Lipidol. (2013) 7:484–525. doi: 10.1016/j.jacl.2013.08.001
46. Liu Y, Zhu J, Yu J, and Zhang X. Cardiorespiratory fitness and metabolic risk in Chinese population: evidence from a prospective cohort study. BMC Public Health. (2024) 24:522. doi: 10.1186/s12889-024-17742-4
47. Musa DI, Toriola AL, Abubakar NO, Omachi S, Olowoleni VB, and Ayodele KB. Association of adiposity and fitness with triglyceride-to-high-density lipoprotein cholesterol ratio in youth. Ann Pediatr Cardiol. (2023) 16:194–200. doi: 10.4103/apc.apc_1_23
48. Gordon DJ, Probstfield JL, Garrison RJ, Neaton JD, Castelli WP, Knoke JD, et al. High-density lipoprotein cholesterol and cardiovascular disease. Four prospective Am Stud Circ. (1989) 79:8–15. doi: 10.1161/01.cir.79.1.8
49. Emerging Risk Factors Collaboration. Di Angelantonio E, Sarwar N, Perry P, Kaptoge S, Ray KK, Thompson A, Wood AM, Lewington S, Sattar N, et al. Major lipids, apolipoproteins, and risk of vascular disease. JAMA. (2009) 302:1993–2000. doi: 10.1001/jama.2009.1619
50. Madsen CM, Varbo A, and Nordestgaard BG. Extreme high high-density lipoprotein cholesterol is paradoxically associated with high mortality in men and women: two prospective cohort studies. Eur Heart J. (2017) 38:2478–86. doi: 10.1093/eurheartj/ehx163
51. Liu C, Dhindsa D, Almuwaqqat Z, Ko Y-A, Mehta A, Alkhoder AA, et al. Association between high-density lipoprotein cholesterol levels and adverse cardiovascular outcomes in high-risk populations. JAMA Cardiol. (2022) 7:672–80. doi: 10.1001/jamacardio.2022.0912
52. Investigators AIM-HIGH, Boden WE, Probstfield JL, Anderson T, Chaitman BR, Desvignes-Nickens P, et al. Niacin in patients with low HDL cholesterol levels receiving intensive statin therapy. N Engl J Med. (2011) 365:2255–67. doi: 10.1056/NEJMoa1107579
53. Lincoff AM, Nicholls SJ, Riesmeyer JS, Barter PJ, Brewer HB, Fox KAA, et al. Evacetrapib and cardiovascular outcomes in high-risk vascular disease. N Engl J Med. (2017) 376:1933–42. doi: 10.1056/NEJMoa1609581
54. Mendelsohn ME and Karas RH. The protective effects of estrogen on the cardiovascular system. N Engl J Med. (1999) 340:1801–11. doi: 10.1056/NEJM199906103402306
55. Karpe F and Pinnick KE. Biology of upper-body and lower-body adipose tissue–link to whole-body phenotypes. Nat Rev Endocrinol. (2015) 11:90–100. doi: 10.1038/nrendo.2014.185
56. Kontush A, Lindahl M, Lhomme M, Calabresi L, Chapman MJ, and Davidson WS. Structure of HDL: particle subclasses and molecular components. Handb Exp Pharmacol. (2015) 224:3–51. doi: 10.1007/978-3-319-09665-0_1
57. Sloan RA. Estimated cardiorespiratory fitness and metabolic risks. Int J Environ Res Public Health. (2024) 21:635. doi: 10.3390/ijerph21050635
58. Ross R, Blair SN, Arena R, Church TS, Després J-P, Franklin BA, et al. Importance of assessing cardiorespiratory fitness in clinical practice: A case for fitness as a clinical vital sign: A scientific statement from the american heart association. Circulation. (2016) 134:e653–99. doi: 10.1161/CIR.0000000000000461
Keywords: cardiorespiratory fitness, cardiopulmonary exercise testing, metabolic health indicators, urban population, cross-sectional study
Citation: Wang C, Yang N, Zhang Q, Li Y, Zhang L, Liu Z, Zhao R, Qi J, Xiang A, Fu Y, Xiong Z, Wu L, Sun J and Huang D (2025) Association between metabolic health indicators and cardiorespiratory fitness in urban young and middle-aged population: a retrospective cross-sectional study. Front. Endocrinol. 16:1489152. doi: 10.3389/fendo.2025.1489152
Received: 31 August 2024; Accepted: 30 April 2025;
Published: 21 May 2025.
Edited by:
Elisabet Børsheim, University of Arkansas for Medical Sciences, United StatesReviewed by:
Manuel Alberto Guerrero Gutierrez, Autonomous University of Baja California, MexicoTheresia Mina, Nanyang Technological University, Singapore
Copyright © 2025 Wang, Yang, Zhang, Li, Zhang, Liu, Zhao, Qi, Xiang, Fu, Xiong, Wu, Sun and Huang. This is an open-access article distributed under the terms of the Creative Commons Attribution License (CC BY). The use, distribution or reproduction in other forums is permitted, provided the original author(s) and the copyright owner(s) are credited and that the original publication in this journal is cited, in accordance with accepted academic practice. No use, distribution or reproduction is permitted which does not comply with these terms.
*Correspondence: Liang Wu, MTk3Mnd1bGlhbmdAc2luYS5jb20=; Jie Sun, YmluZ3h1ZWhhaXR1bjUyMEAxNjMuY29t; Dan Huang, NTgxNDE0NzRAcXEuY29t
†These authors have contributed equally to this work