- 1NHC Key Laboratory of Hormones and Development, Chu Hsien-I Memorial Hospital and Tianjin Institute of Endocrinology, Tianjin Medical University, Tianjin, China
- 2Tianjin Key Laboratory of Metabolic Diseases, Tianjin Medical University, Tianjin, China
- 3Department of Endocrinology, The Second Hospital of Tianjin Medical University, Tianjin, China
- 4Guangdong Breath Test Engineering and Technology Research Center, Shenzhen University, Shenzhen, China
Aims: To determine the effect of red blood cell (RBC) lifespan variability on glycosylated hemoglobin (HbA1c) measurements in type 2 diabetes mellitus (T2DM) individuals and develop a mathematical model for adjusting HbA1c values.
Methods: We tracked glucose levels in 516 T2DM patients from Chu Hsien-I Memorial Hospital, categorized into Construction (n = 416) and Internal (n = 100) cohorts. Additionally, 165 participants from Tianjin diabetic retinopathy screening cohort, serving as the Independent cohort. RBC lifespan was determined using the CO breath test, and Hemoglobin glycation variation index (HGI) was calculated from the difference between measured and estimated HbA1c (eHbA1c). Model efficacy was evaluated using AUC, accuracy, sensitivity, and specificity.
Results: An inflection in the HGI-RBC lifespan model occurred at 66 days, with HbA1c underestimation when RBC lifespan was below 90 days, notably in the ≤ 66 days group. This underestimation increased the risk of cardiovascular and peripheral neuropathy complications. To rectify the impact of the shorter RBC lifespan in T2DM patients, the correction formula was established as HbA1c(c) = -0.05629×RBC lifespan + 1.127×HbA1c + 3.178 (R = 0.7360) in the ≤ 66 day lifespan group and HbA1c(c) = -0.004772 × RBC lifespan + 0.7569 × HbA1c + 2.394 (R = 0.7344) in the 67 to 89 day group. The corrected HbA1c models exhibited satisfactory predictive performance in all cohorts.
Conclusions: Accurate adjustment for the effects of RBC lifespan on HbA1c values in T2DM patients is expected to enhance blood glucose management and the efficacious prevention and treatment of diabetes-associated complications.
Clinical Trial Registration: Chu Hsien-I Memorial Hospital of Tianjin Medical University, identifier ChiCTR2100046557.
Introduction
Type 2 diabetes mellitus (T2DM) poses a significant threat to global human health. The prevalence of diabetes among adults is increasing, standing at 8.8% in 2017 and projected to escalate to 9.9% by 2045 (1). Glycosylated hemoglobin (HbA1c) is acknowledged as the golden criterion for appraising glycemic control during the past two to three months and is widely employed in clinical contexts (2). Clinical evidence reveals that HbA1c markedly influences the risk of chronic complications and the long-term prognosis for individuals with T2DM (3–5). Therefore, accurate assessment of HbA1c is an important basis for guiding clinical adjustment of anti-hyperglycemia treatment. Growing evidence showed that HbA1c levels could be affected by various conditions, especially by the modification of red blood cell (RBC) lifespan and glycation (1, 2). Glucose monitoring methods include Self-Measured Blood Glucose (SMBG), involving periodic fingerstick blood glucose testing, and Continuous Glucose Monitoring (CGM) systems, which employ subcutaneous sensors to provide real-time glucose data (6). Patients’ SMBG records were guided by a team of diabetes specialists, education nurses, and dietitians following the “Trinity Care” management model. Meanwhile, we employed the RBC lifespan tester (ELS Tester, Seekya Biotec Co., Ltd., Shenzhen, China), an innovation by Ma et al. (7–9), to ascertain the RBC lifespan in T2DM patients and subsequently scrutinized the interplay among HbA1c measurement values, average glucose (AG), and RBC lifespan. The CO breath test serves as a dependable and simplified approach for the rapid determination of human RBC lifespan, suitable for clinical use (9), which provides a powerful tool for rapid, accurate, noninvasive, safe, and economical detection of patients’ RBC lifespan (9, 10).
Hemoglobin glycation variation index (HGI) serves as a pivotal metric for gauging the divergence of HbA1c measurement values from the estimated equivalents, which was calculated as HGI = observed HbA1c - estimated HbA1c (eHbA1c) (11). Studies have confirmed that elevated HGI levels are robust predictors of cardiovascular events, Diabetic Kidney Disease, and overall mortality in T2DM individuals (12–14). Our preliminary investigation, albeit with a limited sample size of T2DM patients exhibiting HbA1c detection values below 7%, demonstrated that a diminished RBC lifespan leads to a significant underestimation of blood glucose levels as indicated by the measured HbA1c values (15).
In this study, we conducted a muticenter cohort study and enlarged the sample size, delineated the extent to which RBC lifespan influenced HbA1c measurements, and further established calculation formulas for correcting the impact of RBC lifespan on the HbA1c detection value, HbA1c(c) = -0.05629×RBC lifespan + 1.127×HbA1c + 3.178 (R = 0.7360) in the ≤ 66 day lifespan group and HbA1c(c) = -0.004772 × RBC lifespan + 0.7569 × HbA1c + 2.394 (R = 0.7344) in the 67 to 89 day group. The corrected HbA1c models achieved encouraging predictive performance in all cohorts, promising to deliver enhanced precision in glycemic assessments for clinical applications.
Methods
Participants and study design
This multicenter cohort study enrolled 516 T2DM patients from the “Trinity Care” outpatient clinic at Chu Hsien-I Memorial Hospital, Tianjin Medical University, from September 2022 to January 2023, and 165 T2DM patients from various communities within the Tianjin diabetic fundus disease screening cohort, observed from May 2023 to August 2023. Patients were divided into three cohorts: a construction cohort (n = 416 from Chu Hsien-I Memorial Hospital, Tianjin Medical University), an internal cohort (n = 100 from Chu Hsien-I Memorial Hospital, Tianjin Medical University), and an independent cohort (n = 165 from Tianjin diabetic fundus disease screening cohort). The flowchart of the study was presented in Figure 1.
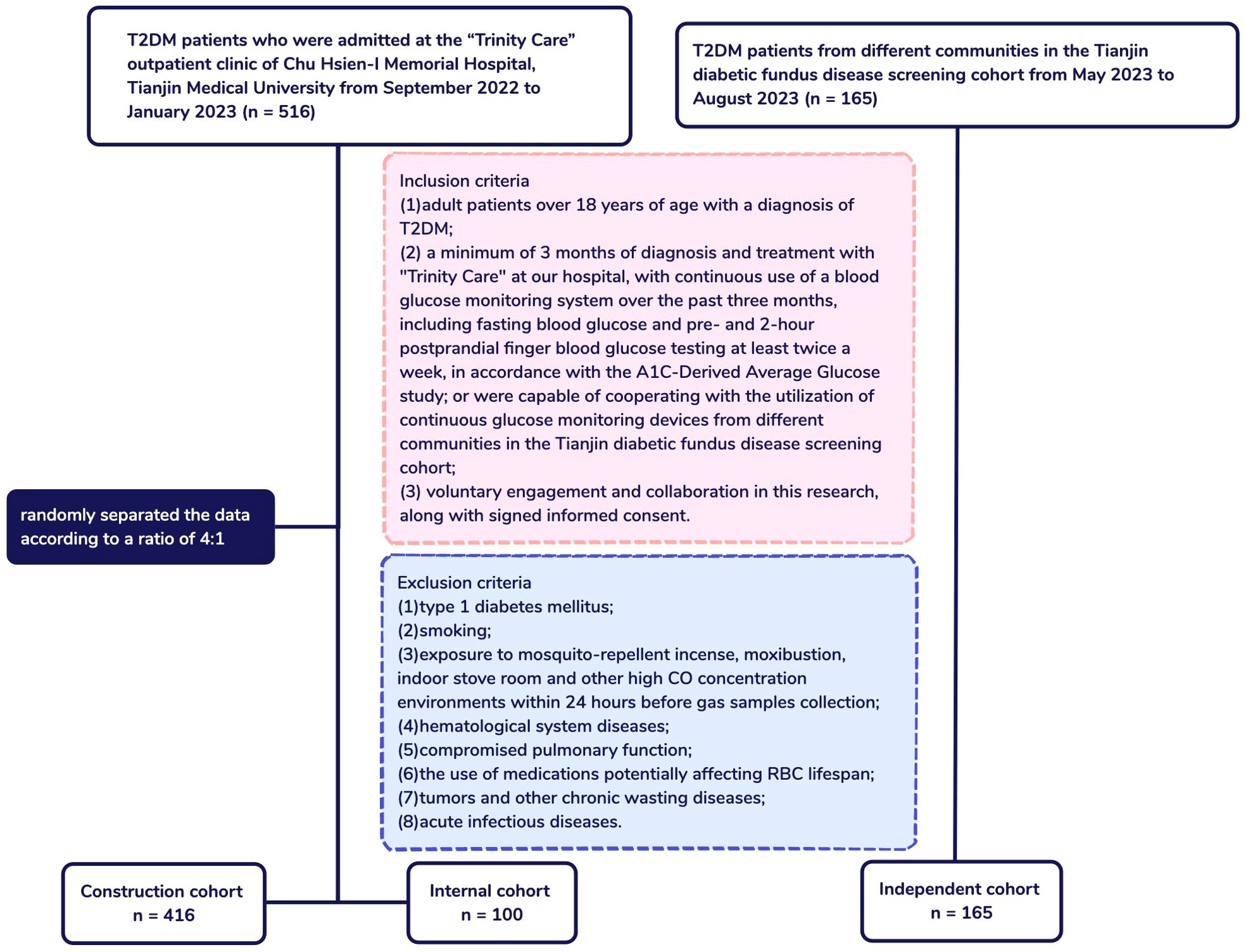
Figure 1. Flowchart of patients and study design. T2DM, Type 2 diabetes mellitus; ADAG, A1C-Derived Average Glucose; CO, Carbon monoxide.
The inclusion criteria encompassed the following: (1) patients aged 18 years or older with a diagnosis of T2DM; (2) a minimum duration of 3 months of diagnosis and treatment with “Trinity Care” at our hospital, with continuous use of a blood glucose monitoring system for the preceding three months, including fasting blood glucose and pre- and 2-hour postprandial finger blood glucose testing at least twice weekly, in accordance with the A1C-Derived Average Glucose study (16); or were capable of cooperating with the utilization of continuous glucose monitoring devices from different communities in the Tianjin diabetic fundus disease screening cohort; (3) voluntary engagement and collaboration in this research, along with provision of signed informed consent. The exclusion criteria included: (1) type 1 diabetes mellitus; (2) smoking; (3) exposure to mosquito-repellent incense, moxibustion, indoor stove room and other high CO concentration environments within 24 hours before gas samples collection; (4) hematological system diseases; (5) compromised pulmonary function; (6) the use of medications potentially affecting RBC lifespan; (7) tumors and other chronic wasting diseases; (8)acute infectious diseases.
This study was registered with the online Chinese Clinical Trial Registry under the identifier ChiCTR2100046557 and was approved by the ethics committee of Chu Hsien-I Memorial Hospital affiliated with Tianjin Medical University (Tianjin, China). Written informed consents were obtained from all patients prior to their participation in the study.
Data acquisition and laboratory inspection
We garnered clinical data, encompassing age, gender, diabetes duration, medications, and incidence of complications (fundus lesions, cardiovascular diseases, peripheral neuropathy). A Beckman Coulter AU5800 automated biochemical analyzer (Beckman Coulter, Brea, CA, USA) was employed to assay liver and kidney functions, blood lipid concentrations, and other biochemical indicators. The HbA1c levels of patients were determined by means of TOSOH G8 (Tosoh Bioscience, Tokyo, Japan). Chu Hsien-I Memorial Hospital patients’ SMBG were recorded by iHealth Smart blood glucose meter under the guidance of a team of diabetes specialists, education nurses, and dietitians following the “Trinity Care” management model. Different communities Patients’ CGM were conducted by “Sibright” CGM system. The AG was translated to the eHbA1c in accordance with the formula: eHbA1c = (AG + 2.5944)/1.5944 (16). The HGI was computed as the discrepancy between the measured and the eHbA1c (HGI = measured HbA1c - eHbA1c) (11). Alveolar gas samples were also collected to measure RBC lifespan by trained personnel.
RBC lifespan measurement
For the measurement of RBC lifespan using Levitt’s CO breath test, the procedure followed a protocol from a recently published study (9). In conclusion, gas samples from the alveoli of each participant were obtained between 8:00 and 10:00 AM after a period of overnight fasting and subsequent 20-minute rest. Participants were directed to inhale deeply, retain their breath for 10 seconds, and then exhale into the collection apparatus via a mouthpiece. The ELS Tester, an automated device manufactured by Seekya Biotec Co., Ltd., Shenzhen, China, was utilized to assess CO levels. This data was subsequently applied to compute the RBC lifespan employing Levitt’s methodology, as follows:
(9).
Statistical analysis
Multiple imputation was used to impute missing data for age, liver and kidney function, and blood lipid concentrations. Construction cohort and Internal cohort allocation were in a 4:1 ratio, achieved via simple randomization within the SPSS. The normalcy of data distribution was evaluated using the Kruskal-Wallis test, while group differences were compared utilizing the Chi-square test. The relationship between continuous variables was modeled using Spearman correlation analysis and restrictive spline regression. For estimating the correction formulas, multivariate linear regression models were engaged. The performance of the correction formulas were verified by ROC curve and calibration curve.
The statistical analyses were performed using IBM SPSS version 25.0, GraphPad Prism version 9.1.1, and R version 4.0.3. A p-value of less than 0.05 from two-tailed tests was deemed to indicate statistical significance.
Results
Cohort characteristics
Based on the inclusion and exclusion criteria, our study included 516 T2DM patients who were attendees at the “Trinity Care” outpatient clinic of Chu Hsien-I Memorial Hospital and 165 T2DM patients from various communities in the Tianjin diabetic fundus disease screening cohort, respectively. In the 516‐patient cohort, 416 and 100 patients were randomly assigned to the construction and internal cohorts, respectively (Figure 1). Table 1 exhibited the traits of the study participants.
Effect of RBC lifespan on AG, HbA1c and HGI in T2DM patients
In the 416 T2DM patients with accessible AG and HbA1c data, a positive correlation was observed between HbA1c levels and RBC lifespan (r = 0.1092, p = 0.0259), while AG exhibited an inverse relationship with RBC lifespan (r = -0.2752, p < 0.0001) (Figure 2). The tested HbA1c may not be a precise indicator of the glycemic status of T2DM individuals and hyperglycemia may lead to a reduced RBC lifespan in this patient population.
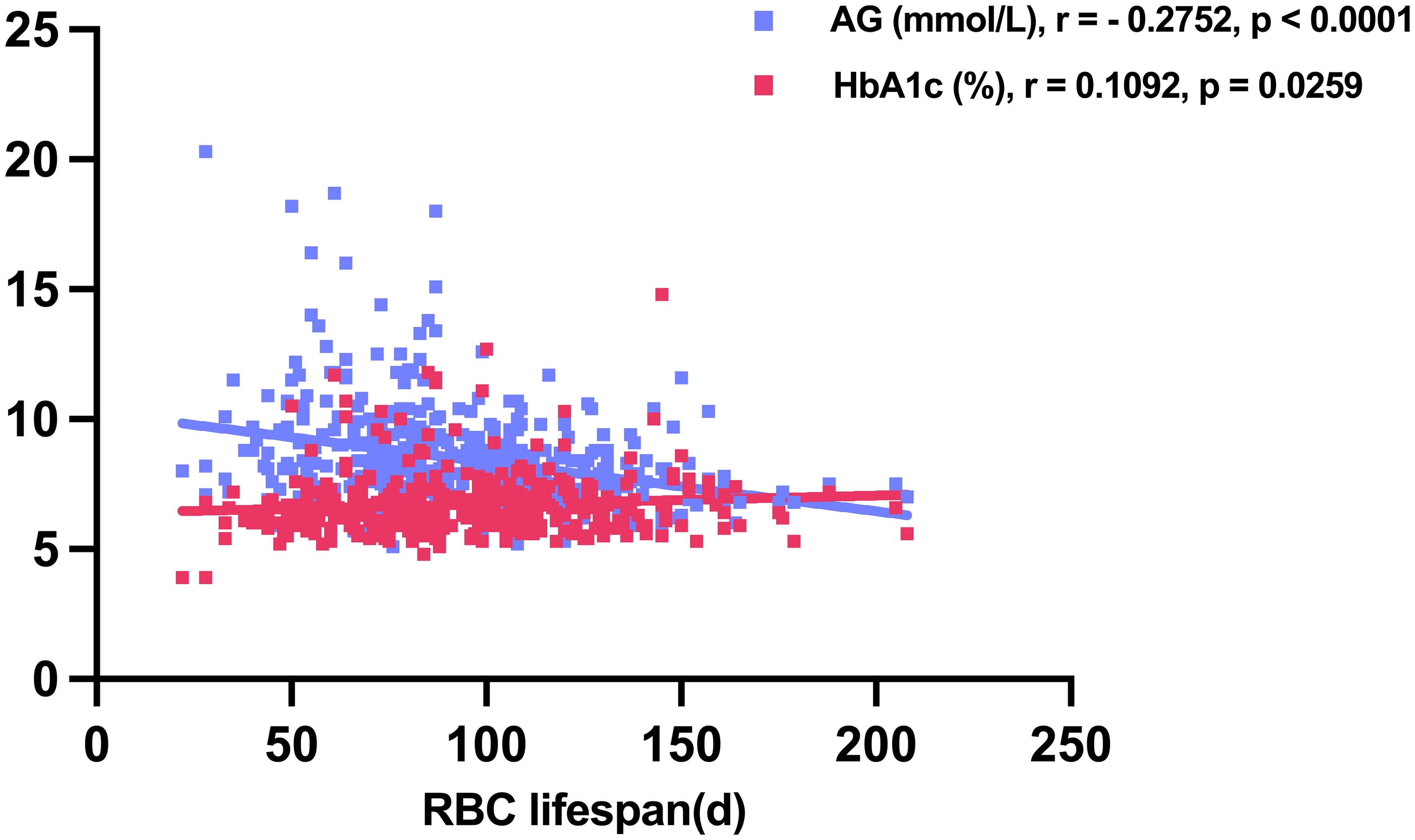
Figure 2. Average glucose (AG) and glycosylated hemoglobin (HbA1c) levels in relation to red blood cell (RBC) lifespan in construction cohort.
The HGI is a critical parameter for determining the deviation of HbA1c measurement values from estimated HbA1c values (17). The translation of AG to eHbA1c based on the formula: eHbA1c = (AG + 2.5944)/1.5944 (16). We examined the correlation between RBC lifespan and HGI within our construction cohort (Figures 3A, B). Consistent with results published before, a diminished RBC lifespan could cause an underestimation of HbA1c values among T2DM patients (12). At the same time, we observed a curve inflection point at RBC lifespan of 66 days (Figure 3B). Previous research has conventionally considered the RBC lifespan range from 90 to 120 days (18). Our study categorized construction group patients into three groups based on RBC lifespan: ≤ 66 days, between 67 and 89 days, and ≥ 90 days. No significant disparities in age, diabetes duration, liver function, and blood lipids were observed across the whole construction cohort and the three subgroups (Table 2). A shorter RBC lifespan could substantially elevate serum creatinine levels (p = 0.0128) and blood urea nitrogen (p = 0.0363) in patients, even when such levels were within the normal range. However, the reduction in RBC lifespan may pose a potential threat to renal function in patients with T2DM, which should not be overlooked.
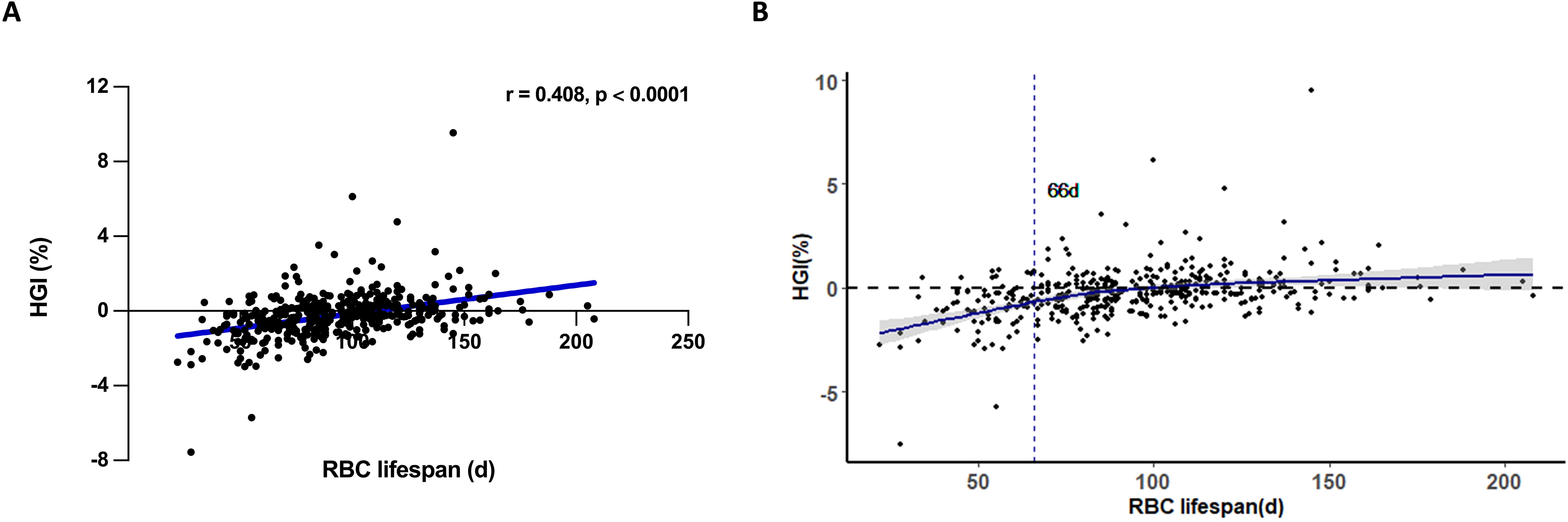
Figure 3. The impact of RBC lifespan upon hemoglobin variation glycation index (HGI) in type 2 diabetes mellitus (T2DM) patients. (A) Spearman correlation analysis of red blood cells (RBC) lifespan and HGI in T2DM patients; (B) Relationship between RBC lifespan and HGI restrictive spline regression fitting curve in T2DM patients. Etimated HbA1c (eHbA1c) = (average glucose (AG) + 2.5944)/1.5944. HGI = HbA1c - eHbA1c.
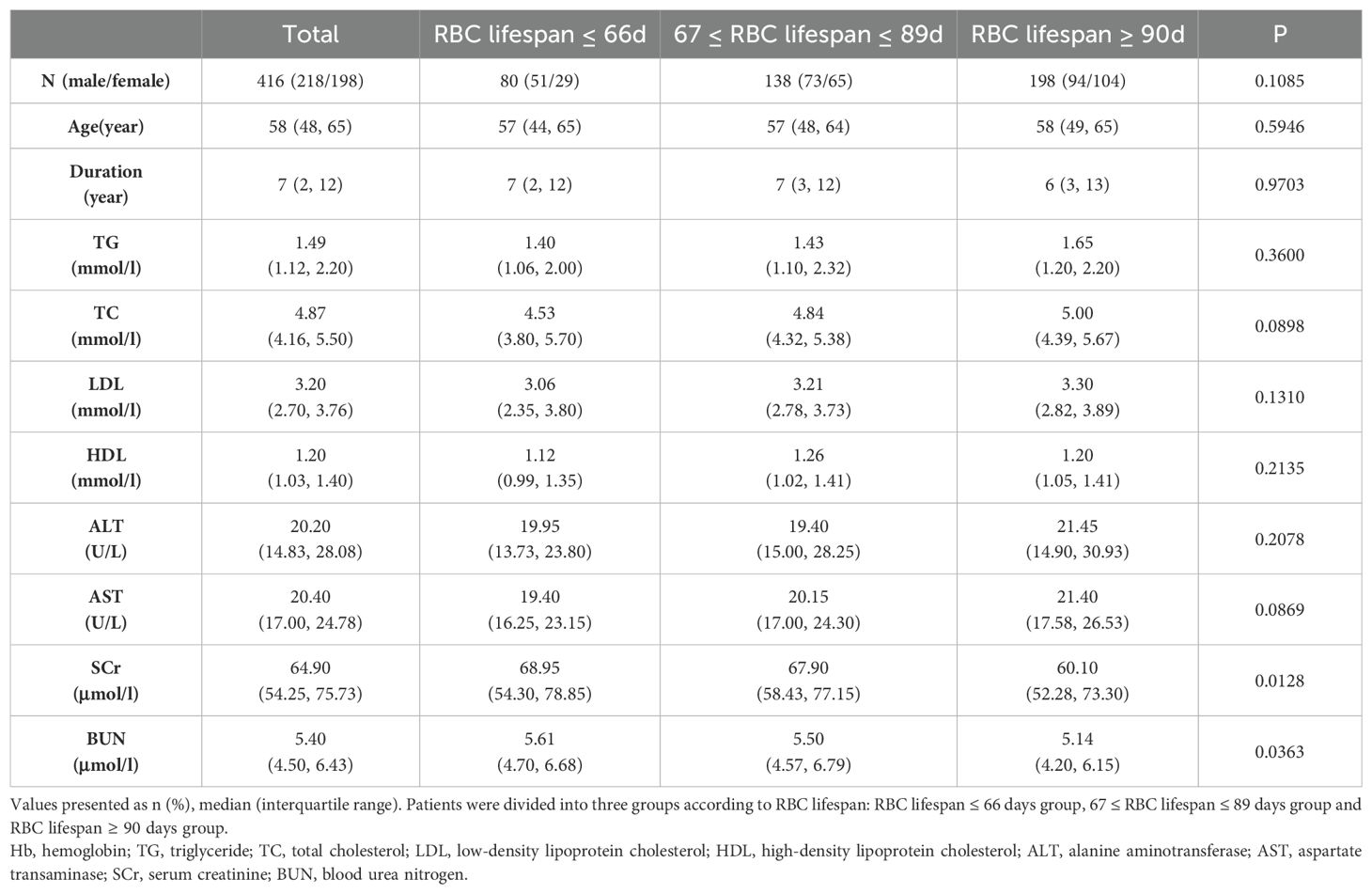
Table 2. General clinical data of type 2 diabetes patients of different red blood cell (RBC) lifespan.
Underestimated HbA1c levels in diverse RBC lifespan groups
To confirm that a shorter RBC lifespan results in an underestimation of the HbA1c measurement value, we assessed the extent of underestimation in HbA1c measurements relative to different RBC lifespans. No significant variation was detected in HbA1c detection values among the three groups (Figure 4A); however, the AG and eHbA1c levels in groups with an RBC lifespan of less than 90 days were notably higher compared to those with a normal RBC lifespan (Figures 4B, C). The median (IQR) HGI of the three groups (Figure 4D) were -0.855 (-1.640, -0.238), -0.415 (-0.963, 0.133), and -0.020 (-0.423, 0.523), respectively. Consequently, the reduction in RBC lifespan could lead to a significant underestimation of blood glucose levels in T2DM patients, particularly in those with an RBC lifespan of ≤ 66 days.
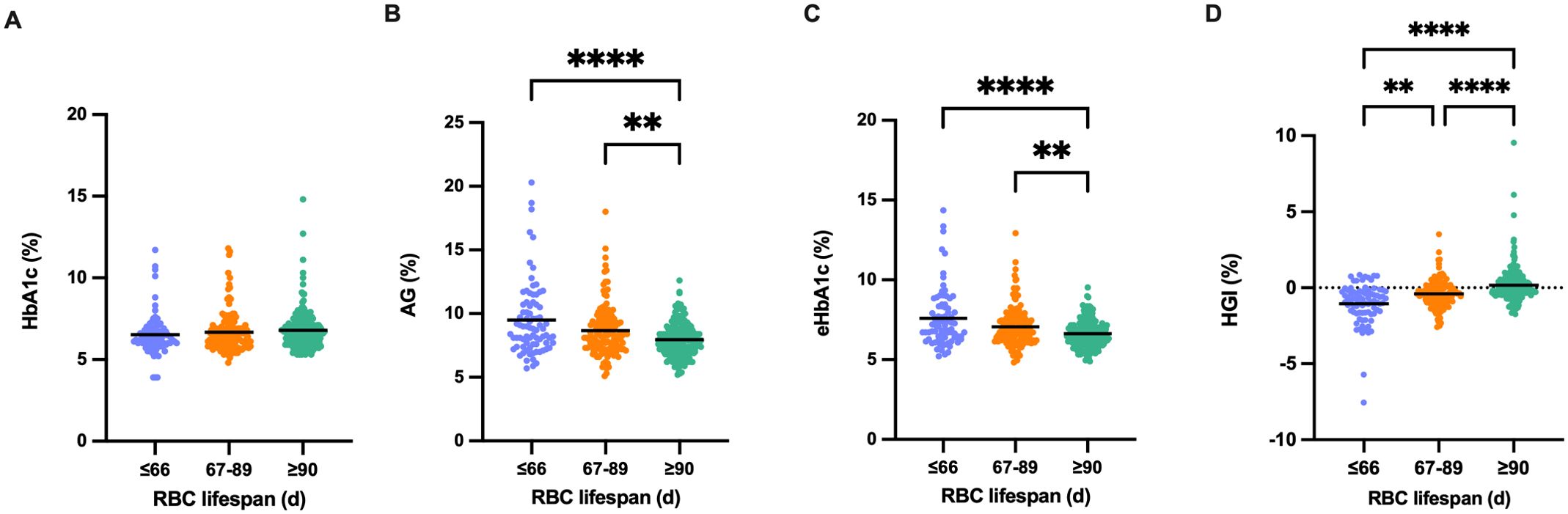
Figure 4. Underestimated glycosylated hemoglobin (HbA1c) levels in diverse red blood cells (RBC) lifespan groups. (A-D). Comparison of HbA1c, average glucose (AG), estimated HbA1c (eHbA1c), and hemoglobin variation glycation index (HGI) in the different RBC lifespan groups. eHbA1c = (AG + 2.5944)/1.5944. HGI = HbA1c - eHbA1c. (**p < 0.01 ****p < 0.0001).
Underestimation of HbA1c values increased the risk of chronic complications in T2DM patients
We established that HbA1c measurement values were subject to varying degrees of underestimation in patients with a RBC lifespan of less than 90 days. The distribution of patients across the groups with RBC lifespan ≤ 66 days, 67 to 89 days, and ≥ 90 days was 19.23%, 33.17%, and 47.60%, respectively (Figure 5A). A diminished RBC lifespan can result in inadequate glycemic control among individuals with T2DM, potentially elevating the risk for developing chronic complications. When the RBC lifespan was less than 90 days, there was a notably elevated risk for cardiovascular disease (OR 1.865, 95% CI 1.224 - 2.833) and peripheral neuropathy (OR 1.599, 95% CI 1.018 - 2.510) (Figure 5B). These results suggested that half (52.40%) of T2DM patients with their HbA1c test values underestimated in varying degrees, and it is imperative to rectify the influence of a shorter RBC lifespan on HbA1c test values to mitigate the risk of chronic complications.
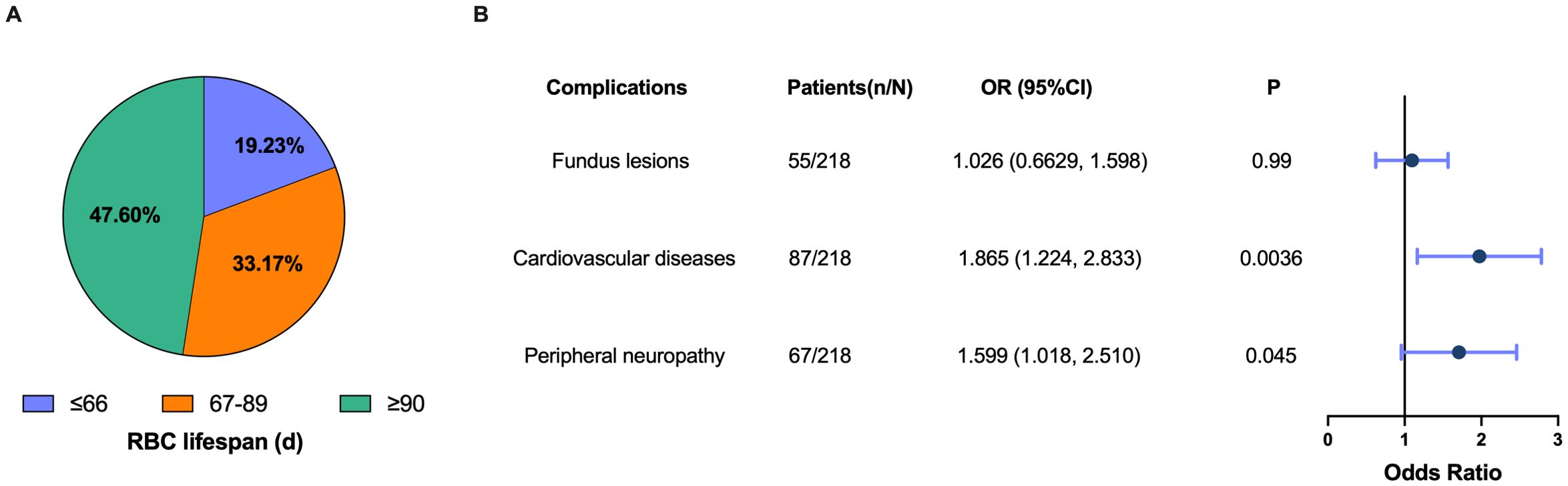
Figure 5. Underestimation of glycosylated hemoglobin (HbA1c) values increased the risk of chronic complications in type 2 diabetes mellitus (T2DM) patients. (A) Proportion of patients in the different red blood cells (RBC) lifespan groups; (B) Forest plot of the association between a shorter RBC lifespan (RBC lifespan < 90 d) and the risk of fundus lesions, cardiovascular disease and peripheral neurology. Dots depicts the point estimate. Horizontal bars depicts 95% confidence interval (CI). OR, odds ratio.
Modulation of the impact of shorter RBC lifespan upon HbA1c test values
Based on the linear relationship between HbA1c and eHbA1c, and the different influence laws in RBC lifespan ≤ 66 day group and 67 ≤ RBC lifespan ≤ 89 days, we employed a multivariate linear regression model to formulate mathematical expressions that adjust for the impact of shorter RBC lifespan on HbA1c measurement values. The model incorporated RBC lifespan and measured HbA1c values as independent variables and the estimated HbA1c values as the dependent variable. P < 0.05 in the F tests, which meant that these regression formulas were meaningful. In the group with RBC lifespan ≤ 66 days, HbA1c(c) = -0.05629×RBC lifespan + 1.127×HbA1c + 3.178 (R = 0.7360). In the 67 ≤ RBC lifespan ≤ 89 days group, HbA1c(c) = -0.004772 × RBC lifespan + 0.7569 × HbA1c + 2.394 (R = 0.7344) may be used for correction. The corrected HbA1c models exhibited satisfactory predictive performance and calibration in the construction cohort (AUC 0.7904, 95% CI, 0.7313 - 0.8494), internal cohort (AUC 0.7940, 95% CI, 0.6646 - 0.9234) and independent cohort (AUC 0.7937, 95% CI, 0.7160 - 0.8714) (Figures 6A–D).
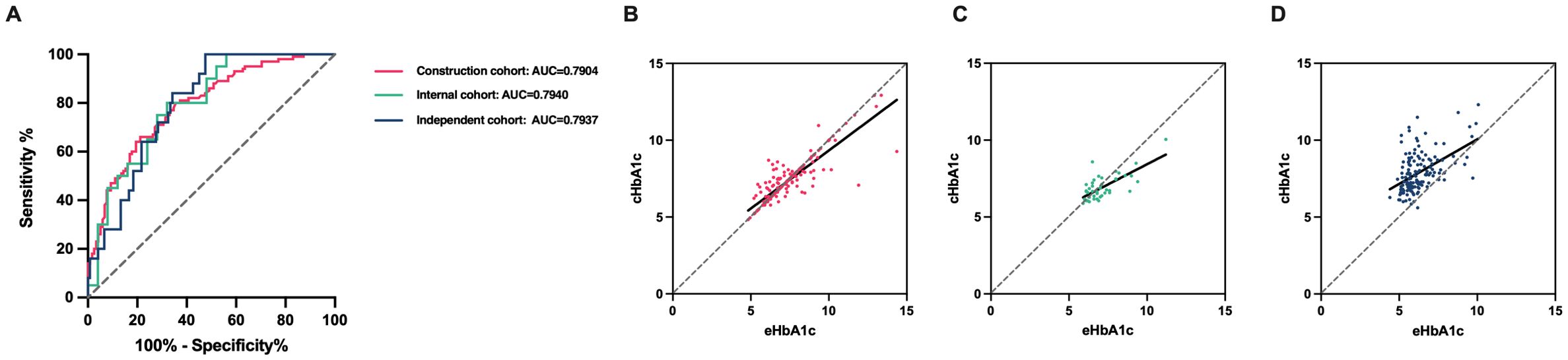
Figure 6. The corrected HbA1c models’ predictive performance and calibration in the different cohorts. (A) Diagnostic performance of the corrected glycosylated hemoglobin (cHbA1c) to detect poor management of blood glucose (estimated HbA1c (eHbA1c) > 7%) of type 2 diabetes mellitus (T2DM) patients in construction cohort, internal cohort and independent cohort. (B-D) Assessing calibration in construction cohort, internal cohort and independent cohort.
Discussion
HbA1c serves as a critical metric for guiding clinicians in adjusting glucose-lowering therapies (2), and the accuracy with which it reflects the actual blood glucose levels in T2DM ndividuals is of profound clinical importance for glycemic management and the prevention of diabetes-related complications (3–5). Our study assessed the effects of variability in RBC lifespan on HbA1c measurement values, clarifying the necessity and significance of correcting this impact in glycemic control. Additionally, we established a mathematical formula to adjust for the influence of RBC lifespan on HbA1c measurement values. We provided accurate information about blood glucose levels in T2DM patients, thereby furthering the advanced management of blood glucose and diabetes-related chronic complications for clinical practice.
To ascertain the impact of RBC lifespan on T2DM patients’ HbA1c and to correct and verify this influence, we incorporated a construction cohort, an internal validation cohort, and an independent test cohort, all of which underwent a standardized HbA1c, AG, and biochemical indices detection protocol. The findings revealed substantial variability in RBC lifespan among T2DM patients. We observed the different correlations between AG, HbA1c and the RBC lifespan, suggesting that measured HbA1c might not accurately represent patients’ glycemic status, and hyperglycemia could contribute to a shortened RBC lifespan in T2DM individuals.
The linear association between RBC lifespan and HGI, which reflected the degree of HbA1c detection value deviating from eHbA1c, strongly indicated that the RBC lifespan variability is a significant cause of large HGI. Research has confirmed that the HGI, or glycosylation gap (Ggap)—an alternative measure to evaluate the discrepancy between HbA1c measurement values and AG—is an independent risk element for microvascular complications in T2DM patients, including diabetic nephropathy (12), diabetic retinopathy (19, 20), and macrovascular diseases (13, 14, 21). The presence of a significant HGI may result in HbA1c measurements that fail to accurately represent the true blood glucose levels of patients, leading to chronic suboptimal glycemic control. Consequently, identifying diabetic patients with large HGI is crucial for diminishing the risk of diabetes-related complications and enhancing their overall prognosis. Our results strongly suggested that RBC lifespan is a suitable parameter to assess HGI. Some studies have also reported that the characteristics of RBC lifespan themselves influence the HGI, including the survival time of RBC, the glycosylation rate of Hb, and genetic factors (16, 22). Malka reported that the variation in the average RBC age among individuals accounts for all the glucose-independent variability in HbA1c (23). Although this study had a robust design, its sample size was very small. Our study with a large sample also supported the conclusion that a shorter RBC lifespan may be the primary driver of HGI in diabetic individuals.
In our study, there were half of T2DM patients with a shorter RBC lifespan, which could substantially raise the risk of cardiovascular disease and peripheral neurology. This result may partly explain those clinical cases with suitable HbA1c levels who suffered from severe diabetic-related chronic complications. These cases may have reached chronic hyperglycemic status. Our study also strongly suggested that in the management of blood glucose levels in T2DM patients, it is essential to adjust for the impact of a shorter RBC lifespan on HbA1c test values to ascertain the actual blood glucose levels and to mitigate the risk of chronic complications. Consequently, a pilot study is warranted to elucidate the causal link between a shortened RBC lifespan and diabetes-related complications.
To accurately gauge whether T2DM patients with a shorter RBC lifespan have achieved the desired blood glucose levels, it is imperative to correct for the influence of RBC lifespan on HbA1c values. Utilizing the linear relationship between estimated HbA1c value and measured HbA1c, and considering the distinct effects observed in patients with an RBC lifespan of ≤ 66 days and those with 67 to 89 days, we employed a multivariate linear regression model to establish mathematical formulas that adjust for the impact of a shorter RBC lifespan on HbA1c measurement values. In patients with RBC lifespan ≤ 66 days, HbA1c(c) = -0.05629×RBC lifespan + 1.127×HbA1c + 3.178 (R = 0.7360). So, for patients with RBC lifespan less than 66 days, this formula helps us obtain a more trustable HbA1c value. In patients with an RBC lifespan of 67 ≤ RBC lifespan ≤ 89 days, HbA1c(c) = -0.004772 × RBC lifespan + 0.7569 × HbA1c + 2.394 (R = 0.7344) may be used for correction. The corrected HbA1c models exhibited satisfactory predictive performance and calibration in the construction cohort, internal cohort and independent cohort. In clinical practice, to precisely assess the blood glucose levels of T2DM individuals, both the tested HbA1c and the values of RBC lifespan are requisite. When a patient’s RBC lifespan is shorter than 90 days, their true HbA1c should be calculated using the aforementioned formulas. These formulas will provide valuable clinical insights to more accurately evaluate patients’ blood glucose levels and guide treatment decisions.
Our study had notable strengths. We enrolled a large and multi-center study to investigate the impact of variability in RBC lifespan on the HbA1cmeasurement value, clarifying the need and importance of correcting this influence in the management of blood glucose. We established the mathematic formulas to rectify the influence of RBC lifespan on HbA1c measurements and validated them across various population cohorts. The correction formula offers a more precise evaluation of glycemic control, particularly in patients with shortened RBC lifespan. This is crucial as standard HbA1c measurements may underestimate blood glucose levels in such cases, potentially leading to inadequate treatment. By providing a more accurate reflection of a patient’s true glycemic status, the formula enables clinicians to tailor treatment plans more effectively. This can result in more appropriate adjustments to diabetes medications and lifestyle interventions. Additionally, the corrected HbA1c values can improve the assessment of a patient’s risk for diabetes-related complications, allowing for better preventive measures and more timely interventions.
However, some questions still remain to be answered: 1) How often do we need to test RBC lifespan in patients with T2DM? 2) Do we need to avoid the menstrual period of women patients when testing their RBC lifespan? We acknowledge that our study has several limitations that need to be addressed in future research. Specifically, we did not account for potential confounding factors such as interindividual differences in hemoglobin glycation rates, anemia, and chronic kidney disease, that may affect the accuracy of HbA1c measurements. Future research should aim to incorporate these variables into more comprehensive models to further enhance the precision of glycemic assessment. Additionally, the study was conducted in Tianjin and the sample size was relatively small, which may limit the generalizability of our results and the statistical power of our analysis. Meanwhile, the specific characteristics of our cohorts may limit the direct applicability of these formulas to other populations. Further validation is necessary to confirm the effectiveness of our correction formulas in different patient populations with varying ethnic backgrounds, different stages of diabetes, and those with comorbid conditions.
In summary, the variation in RBC lifespan among T2DM patients is a crucial factor contributing to the discrepancy between measured and estimated HbA1c levels, known as HGI. A substantial proportion of diabetic individuals with a reduced RBC lifespan exhibited significantly underestimated HbA1c measurement values. Correcting the HbA1c measurements accurately in T2DM patients with a shorter RBC lifespan is essential. This correction is crucial for optimizing blood glucose management and for enhancing the outcomes related to chronic complications of diabetes. This approach will ultimately contribute to the advancement of clinical practices in diabetes care.
Data availability statement
The raw data supporting the conclusions of this article will be made available by the authors, without undue reservation.
Ethics statement
The studies involving humans were approved by Chu Hsien-I Memorial Hospital of Tianjin Medical University (ChiCTR2100046557). The studies were conducted in accordance with the local legislation and institutional requirements. The participants provided their written informed consent to participate in this study. Written informed consent was obtained from the individual(s), and minor(s)’ legal guardian/next of kin, for the publication of any potentially identifiable images or data included in this article.
Author contributions
LZ: Conceptualization, Writing – original draft, Writing – review & editing, Formal Analysis. XG: Formal Analysis, Methodology, Writing – review & editing. XM: Formal Analysis, Funding acquisition, Software, Writing – review & editing. GM: Methodology, Software, Writing – review & editing. JL: Methodology, Software, Writing – review & editing. WW: Methodology, Software, Writing – review & editing. SC: Funding acquisition, Methodology, Software, Writing – review & editing. YM: Conceptualization, Writing – review & editing. PY: Conceptualization, Writing – review & editing. SZ: Conceptualization, Funding acquisition, Writing – original draft, Writing – review & editing.
Funding
The author(s) declare that financial support was received for the research and/or publication of this article. This study was supported by the China Endocrine Metabolism Talent Research Fund [grant number 2022-N-02-07, 2023-N-03-15], the Scientific Research Funding of Tianjin Medical University Chu Hsien-I Memorial Hospital, Tianjin Key Laboratory of Metabolic Diseases [grant number ZXY-ZDSYSZD2022-1], Bethune Charitable Foundation [grant number Z04JKM2022E035], Hubei Chen Xiaoping Science and Technology Development Fund [grant number CXPJJH122012-002] and the Tianjin Key Medical Discipline (Specialty) Construction Project [grant number TJYXZDXK-032A].
Acknowledgments
We thank LetPub (www.letpub.com) for its linguistic assistance during the preparation of this manuscript.
Conflict of interest
The authors declare that the research was conducted in the absence of any commercial or financial relationships that could be construed as a potential conflict of interest.
Publisher’s note
All claims expressed in this article are solely those of the authors and do not necessarily represent those of their affiliated organizations, or those of the publisher, the editors and the reviewers. Any product that may be evaluated in this article, or claim that may be made by its manufacturer, is not guaranteed or endorsed by the publisher.
Supplementary material
The Supplementary Material for this article can be found online at: https://www.frontiersin.org/articles/10.3389/fendo.2025.1500660/full#supplementary-material
Abbreviations
AG, average glucose; CGM, Continuous Glucose Monitoring; eHbA1c, estimated HbA1c; HbA1c, Glycosylated hemoglobin; HGI, Hemoglobin glycation variation index; RBC, red blood cell; SMBG, Self-Measured Blood Glucose; T2DM,Type 2 diabetes mellitus.
References
1. Standl E, Khunti K, Hansen TB, Schnell O. The global epidemics of diabetes in the 21st century: Current situation and perspectives. Eur J Prev Cardiol. (2019) 26:7–14. doi: 10.1177/2047487319881021
2. American Diabetes Association. 6. Glycemic targets: standards of medical care in diabetes-2019. Diabetes Care. (2019) 42:S61–70. doi: 10.2337/dc19-S006
3. Intensive blood-glucose control with sulphonylureas or insulin compared with conventional treatment and risk of complications in patients with type 2 diabetes (UKPDS 33) Vol. 352. Lancet (London, England: UK Prospective Diabetes Study (UKPDS) Group (1998) p. 837–53.
4. Sakurai M, Saitoh S, Miura K, Nakagawa H, Ohnishi H, Akasaka H, et al. HbA1c and the risks for all-cause and cardiovascular mortality in the general Japanese population: NIPPON DATA90. Diabetes Care. (2013) 36:3759–65. doi: 10.2337/dc12-2412
5. Inzucchi SE, Bergenstal RM, Buse JB, Diamant M, Ferrannini E, Nauck M, et al. Management of hyperglycemia in type 2 diabetes, 2015: a patient-centered approach: update to a position statement of the American Diabetes Association and the European Association for the Study of Diabetes. Diabetes Care. (2015) 38:140–9. doi: 10.2337/dc14-2441
6. Lai M, Weng J, Yang J, Gong Y, Fang F, Li N, et al. Effect of continuous glucose monitoring compared with self-monitoring of blood glucose in gestational diabetes patients with HbA1c<6%: a randomized controlled trial. Front Endocrinol. (2023) 14:1174239. doi: 10.3389/fendo.2023.1174239
7. Ma YJ, Zhang HD, Wu CH, Zhu GL, Ji YQ, Huang JL, et al. Rapid CO breath test screening of drugs for protective effects on ribavirin-induced hemolysis in a rabbit model: a pilot study. J Breath Res. (2016) 10:36010. doi: 10.1088/1752-7155/10/3/036010
8. Ma YJ, Zhang HD, Ji YQ, Zhu GL, Huang JL, Du LT, et al. A modified carbon monoxide breath test for measuring erythrocyte lifespan in small animals. BioMed Res Int. (2016) 2016:7173156. doi: 10.1155/2016/7173156
9. Ye L, Ji Y, Zhou C, Luo J, Zhang L, Jing L, et al. Comparison of Levitt’s CO breath test and the 15 N-glycine labeling technique for measuring the lifespan of human red blood cells. Am J Hematol. (2021) 96:1232–40. doi: 10.1002/ajh.26290
10. Chu HW, Ma YJ, Huang ZH. A pilot study: effect of erythrocyte lifespan determined by a modified carbon monoxide breath test on glycosylated hemoglobin interpretation. J Breath Res. (2020) 14:027101. doi: 10.1088/1752-7163/ab75f8
11. Merino-Torres JF, Fajardo-Montañana C, Ferrer-García JC, Piñón-Sellés F. Hemoglobin Glycosylation Index is not related with blood glucose. J Diabetes its Complications. (2003) 17:249–53. doi: 10.1016/s1056-8727(02)00226-x
12. Xin S, Zhao X, Ding J, Zhang X. Association between hemoglobin glycation index and diabetic kidney disease in type 2 diabetes mellitus in China: A cross- sectional inpatient study. Front Endocrinol. (2023) 14:1108061. doi: 10.3389/fendo.2023.1108061
13. Yang J, Shangguan Q, Xie G, Yang M, Sheng G. Sex-specific associations between haemoglobin glycation index and the risk of cardiovascular and all-cause mortality in individuals with pre-diabetes and diabetes: A large prospective cohort study. Diabetes Obes Metab. (2024) 26:2275–83. doi: 10.1111/dom.15541
14. Zhang L, Wang M, Zhang R, Zhong Y, Fan H, Wang M, et al. Hemoglobin glycation index in relationship to the risk of cardiovascular complication in patients with type 2 diabetes: A systematic review and meta-analysis. J Diabetes its Complications. (2020) 34:107673. doi: 10.1016/j.jdiacomp.2020.107673
15. Wang J, Zhang L, Bai Y, Wang X, Wang W, Li J, et al. The influence of shorter red blood cell lifespan on the rate of HbA1c target achieved in type 2 diabetes patients with a HbA1c detection value lower than 7. J Diabetes. (2023) 15:7–14. doi: 10.1111/1753-0407.13345
16. Nathan DM, Kuenen J, Borg R, Zheng H, Schoenfeld D, Heine RJ, et al. Translating the A1C assay into estimated average glucose values. Diabetes Care. (2008) 31:1473–8. doi: 10.2337/dc08-0545
17. Lyu L, Yu J, Liu Y, He S, Zhao Y, Qi M, et al. High hemoglobin glycation index is associated with telomere attrition independent of hbA1c, mediated by TNFα. J Clin Endocrinol Metab. (2022) 107:462–73. doi: 10.1210/clinem/dgab703
18. Inoue K, Tsujimoto T, Yamamoto-Honda R, Goto A, Kishimoto M, Noto H, et al. A newer conversion equation for the correlation between HbA1c and glycated albumin. Endocrine J. (2014) 61:553–60. doi: 10.1507/endocrj.ej13-0450
19. McCarter RJ, Hempe JM, Gomez R, Chalew SA. Biological variation in HbA1c predicts risk of retinopathy and nephropathy in type 1 diabetes. Diabetes Care. (2004) 27:1259–64. doi: 10.2337/diacare.27.6.1259
20. Soros AA, Chalew SA, McCarter RJ, Shepard R, Hempe JM. Hemoglobin glycation index: a robust measure of hemoglobin A1c bias in pediatric type 1 diabetes patients. Pediatr Diabetes. (2010) 11:455–61. doi: 10.1111/j.1399-5448.2009.00630.x
21. Ahn CH, Min SH, Lee DH, Oh TJ, Kim KM, Moon JH, et al. Hemoglobin glycation index is associated with cardiovascular diseases in people with impaired glucose metabolism. J Clin Endocrinol Metab. (2017) 102:2905–13. doi: 10.1210/jc.2017-00191
22. Engström G, Smith JG, Persson M, Nilsson PM, Melander O, Hedblad B. Red cell distribution width, haemoglobin A1c and incidence of diabetes mellitus. J Internal Med. (2014) 276:174–83. doi: 10.1111/joim.12188
Keywords: glycosylated hemoglobin (HbA1c), hemoglobin glycation index (HGI), red blood cell lifespan, type 2 diabetes mellitus, correction model
Citation: Zhang L, Gao X, Meng X, Ma G, Li J, Wang W, Chen S, Ma Y, Yu P and Zhou S (2025) Rectify the impact of shorter red blood cell lifespan upon HbA1c detection values in T2DM patients: modeling and internal-external verification. Front. Endocrinol. 16:1500660. doi: 10.3389/fendo.2025.1500660
Received: 23 September 2024; Accepted: 28 March 2025;
Published: 15 April 2025.
Edited by:
Sandeep Dhindsa, Saint Louis University, United StatesReviewed by:
Inderpreet Madahar, Central Michigan University, United StatesKaleb Mayisso, Hawassa University, Ethiopia
Copyright © 2025 Zhang, Gao, Meng, Ma, Li, Wang, Chen, Ma, Yu and Zhou. This is an open-access article distributed under the terms of the Creative Commons Attribution License (CC BY). The use, distribution or reproduction in other forums is permitted, provided the original author(s) and the copyright owner(s) are credited and that the original publication in this journal is cited, in accordance with accepted academic practice. No use, distribution or reproduction is permitted which does not comply with these terms.
*Correspondence: Saijun Zhou, emhvdXNhaWp1bkB0bXUuZWR1LmNu; Pei Yu, eXVwZWlAdG11LmVkdS5jbg==; Yongjian Ma, bWF5akBzenUuZWR1LmNu
†These authors have contributed equally to this work and share first authorship