- 1South China Sea Fisheries Research Institute, Chinese Academy of Fishery Sciences, Guangzhou, China
- 2Guangdong Provincial Key Laboratory of Fishery Ecology and Environment, Guangzhou, China
- 3College of the Environment and Ecology, Xiamen University, Xiamen, China
- 4Key Laboratory of the Ministry of Education for Coastal and Wetland Ecosystems, Xiamen University, Xiamen, China
- 5Department of Environmental Science and Engineering, Xiamen University Tan Kah Kee College, Zhangzhou, China
- 6Laboratory of Marine Biology and Ecology, Third Institute of Oceanography, Ministry of Natural Resources, Xiamen, China
The biological trait analysis (BTA) is regarded as a promising approach to unravel how ecosystem functions respond to human-induced disturbances. This study considered the four sampling locations associated with different human activities in Daya Bay, that is, the domestic and industrial sewage discharge area (SED), mariculture area (MRC), nuclear power plants thermal discharge area (NTD), and an area with relatively low human disturbance as a reference (REF). Thirty modalities of nine traits were selected in BTA. Our results showed a clear shift in the functional structure of macrobenthic communities between the sampling locations, except for the case between NTD and REF. The trait composition in the communities did not highlight any seasonal patterns. Bioturbation, longevity, tolerance, body size, feeding habit, and environmental position were the key traits to characterize the functional structure of macrobenthic communities and demonstrated predictable responses along the environmental gradients. Water depth, DO, Chl-a, NH4+, and petroleum contaminants in sediments were the main variables influencing the trait composition. In addition, the taxonomic index (H′) and functional diversity index (Rao’s Q) showed clear differences among the sampling locations. Although there were no significant differences between NTD and REF in terms of the trait composition and functional diversity, a potential function loss in NTD still can be detected through the integrated analysis with taxonomic diversity. We suggest that the traits (except for fragility, larval development, and living habits) selected and the diversity indices (H′ and Rao’s Q) could serve as promising indicators of ecological conditions in Daya Bay.
Introduction
Macrobenthic fauna are key components in marine ecosystems and drive important processes such as sediment reworking, bio-irrigation, nutrient cycling, and organic matter decomposition (Widdicombe et al., 2004; Lindqvist 2014; Bonaglia et al., 2017). As their limited migration ability, relatively long life span, and sensitivity to environmental changes, benthic macrofauna are usually regarded as effective indicators for ecosystem health assessment (Snelgrove 1998; Patricio et al., 2009; Borja et al., 2010). Traditionally, biodiversity and how it is related with environmental change and ecosystem health have often been measured and quantified through taxonomy-based approaches, which include taxonomic diversity indices and multivariate methods, based on species richness, abundance, and biomass (Vandewalle et al., 2010; Strong et al., 2015). But these approaches do not take ecosystem functions into account and ignore that different species may perform similar functional roles in communities and ecosystems (Frid et al., 2000; Dolédec and Bonada 2013). Furthermore, ecosystem processes and services are primarily determined by the functional identity, rather than by taxonomic identity (Bellwood et al., 2012; Naeem et al., 2012). Since the beginning of this century, the trait-based approach, characterizing organisms by a suite of functional identities (functional traits), has received an increasing recognition in the marine community ecology (Bremner et al., 2003; Gagic et al., 2015; Barton et al., 2016; Beauchard et al., 2017; Degen et al., 2018).
A representative trait-based approach is biological trait analysis (BTA) which uses a set of biological traits of organisms to depict the variation patterns of the functional structure of communities along environmental gradients (Bremner et al., 2006). Generally, the functional structure of a community consists of two components, which can be quantified by different indices (Dias et al., 2013). The first component, the “community-weighted mean trait value” (CWM), can be calculated for each trait as the average trait value in a community weighted by the relative abundance/biomass of the species carrying each value (Garnier et al., 2004). This index is usually used to identify the dominant traits in a community and can be particularly useful to understand the response of the biotic communities to the changes in disturbances and environmental gradients due to the environmental selection for certain traits (Vandewalle et al., 2010; Ricotta and Moretti 2011). The other component, the functional diversity (FD), describes the extent of trait differences among coexisting species within a community (Petchey and Gaston 2006). A general assumption is that the stressed communities would lead to a decrease in FD (Mason et al., 2013), thereby decreasing the diversity in resource use strategies (Strong et al., 2015). FD can be further decomposed into three complementary subcomponents, that is, functional richness, functional evenness, and functional divergence (Mason et al., 2005). An increasing number of indices have been proposed or are being developed for the quantification of one or more of these subcomponents, for example, FRic, FEve, FDiv, FDis, FR, and Rao’s Q. FRic (functional richness index), FEve (functional evenness index), and FDiv (functional divergence index), respectively, measure the three subcomponents of functional diversity (Mason et al., 2005; Villeger et al., 2008). Functional dispersion index (FDis) measures the mean distance in the multidimensional trait space of individual species to the centroid of all species (Laliberte and Legendre 2010). FR (functional redundancy index) corresponds to the average number of species sharing similar sets of traits (Mouillot et al., 2014). Rao’s Q (Rao’s quadratic entropy) incorporates the pairwise functional differences of species weight by their relative abundance (Schmera et al., 2017). Among these indices, CWM and Rao’s Q (Rao’s quadratic entropy) are most frequently used in previous studies (Ricotta and Moretti, 2011).
Coastal areas host more than half of the planet’s human population, depending on the ecosystems and the services they provide (Barbier et al., 2008; Halpern et al., 2008). Most coastal ecosystems have been somewhat altered by human activities at all spatial scales (Lotze et al., 2006; Halpern et al., 2008; Halpern et al., 2015; Cloern et al., 2016). Benthic communities are extremely susceptible to human disturbances, which include domestic and industrial sewage and extensive mariculture, resulting in eutrophication, oxygen deficiency, and contaminant enrichment in sediments (Villnas et al., 2011; Franzo et al., 2016). This situation could become worse, given the weak hydrodynamic force in enclosed and semi-enclosed bays; therefore, the concentration of pollutants in sediments often reaches up to the orders of magnitude higher than in the overlying water (Trannum et al., 2004; Cardellicchio et al., 2007). Recently, more and more nuclear power plants (NPPs) have been or are being constructed for the surge in energy demand in China (Ye et al., 2017). Thermal discharge and the use of radionuclides pose substantial risks to aquatic organisms living nearby after the operation of NPP (Shiah et al., 2006; Teixeira et al., 2009; Thinova and Trojek 2009). BTA has been applied in the marine macrobenthic community to assess human impacts such as bottom trawling (Tillin et al., 2006; Bolam et al., 2014), pollution (Oug et al., 2012; Gusmao et al., 2016; van der Linden et al., 2016; Nasi et al., 2018; Egres et al., 2019; Nunes de Souza et al., 2021), dredging, and dredged material disposal (Cooper et al., 2008; Bolam et al., 2016). Most of the research studies focus on Europe, South America, and Arctic region; the application of BTA on the macrobenthic community is relatively scarce in Asia. Above all, previous studies only consider the impact of singular human activities, while the comparisons on the effects between different human activities are rarely involved.
Daya Bay is embedded in the key economic development zone in south China. It is surrounded by petrochemical industries, mariculture, NPP, and urban development (Wu et al., 2009; Liu et al., 2018), which makes it an ideal paradigm for studying the response of macrobenthic communities to multiple human activities. Numerous previous studies demonstrated that human activities had severely changed the biotic and abiotic environment in Daya Bay (Gao and Chen 2008; Wu et al., 2009; Wang et al., 2011; Ma et al., 2014; Arbi et al., 2017). The mariculture area in Daya Bay has expanded from 440 ha in 1988 to 14,000 ha in 2005, a nearly 600-fold growth over the past decades (Wu et al., 2009). The annual average Chl-a concentration increased by 1.9 mg/m3, and harmful algal blooms increased significantly (Song et al., 2009). The cooling sea water from NPP has been discharged at a rate of 95 m3/s and with a temperature of 65°C since 2002, and sea water +1°C area can cover approximately 56 km2 (Yu et al., 2010). The thermal discharges decreased the abundance and diversity of phytoplankton and zooplankton and consequently declined the fishery production (Hao et al., 2016). With the rapid development of the petrochemical industries in Daya Bay, around 1,150 m3/h sewage, containing COD, petroleum hydrocarbons, heavy metals, sulfide, ammonia, and so on, is directly discharged into the bay (Xu et al., 2014).
In this study, BTA was used to illustrate the functional responses of macrobenthic communities to human activities. We targeted three major human activities (domestic and industrial sewage discharge, mariculture, and NPP thermal discharge) in Daya Bay and aimed to 1) evaluate potential differences in the functional traits of macrobenthic communities influenced by different types of human activities; 2) identify the most influential environmental variables impacting the functional trait patterns; and 3) discern the most responsive traits to environment stress.
Materials and Methods
Study Area
Daya Bay is a semi-enclosed bay located in the northern edge of the South China Sea, with a subtropical marine climate. It covers an area of 600 km2 and has an average water depth of 10 m. The minimal sea surface temperature occurs in winter (average 17.3°C) and the maximum in summer (average 29.3°C). It is mainly influenced by the East Asian Monsoon system, whereby southwest winds prevail in summer and northeast winds in winter. The average annual rainfall is 1984 mm, 80% of which happens in spring and summer (wet season) and 20% in autumn and winter (dry season) (Wang et al., 2008). Tidal current is the dominant current component in Daya Bay which is of the irregular semi-diurnal type. Despite the low velocity (2–6 cm/s), there also exist two types of seasonal circulation: cyclonic circulation in summer and anticyclonic circulation in winter (Wu et al., 2007). There are mariculture areas (shellfish farming that has lasted over the past decades) in the northeast coast, petrochemical industries (operated since 2001) in the northwest coast, and two nuclear power plants (operated since 1994 and 2002, respectively) in the western coast. Aotou, located in the northwest coast, is the most densely populated town in Daya Bay, with more than 60,000 residents. Over the past decades, the ecological environment of Daya Bay has been severely influenced by the aforementioned types of human activities (Wang et al., 2008; Chen et al., 2010; Qu et al., 2018; Ke et al., 2019).
Field Sampling and Sample Processing
Three stations were set in the four sampling locations associated with different human activities, respectively, resulting in a total of 12 stations (Figure 1). These locations are domestic and industrial sewage discharge area (SED), mariculture area (MRC), sea water +1°C area induced by NPP thermal discharge (NTD), and an area in the mouth of the bay with relatively low human disturbance as a reference (REF). Sampling surveys were conducted seasonally (November in 2017, January, April, and July in 2018, representing autumn, winter, spring, and summer, respectively). At each station, four replicate sediment samples were collected using a 0.05-m2 Van Veen grab and washed through a 0.5-mm mesh sieve, and the residues were transferred to the sample containers with 5% formalin buffer in situ for faunal analysis. In the laboratory, animals were identified to the lowest possible taxon and enumerated under a dissecting microscope. Taxonomic data from the four replicates were pooled to obtain faunal composition at each sampling station.
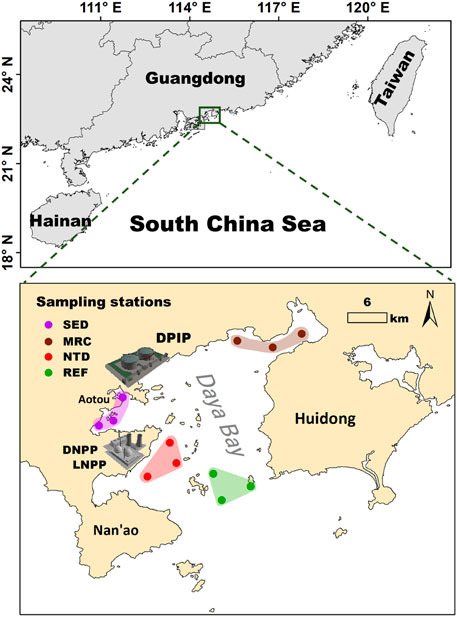
FIGURE 1. Location of sampling stations in Daya Bay. Stations are classified as sewage discharge (SED, dots in the purple shadow), mariculture (MRC, dots in the brown shadow), NPP thermal discharge (NTD, dots in the red shadow), and reference (REF, dots in the green shadow). DPIP, Daya Bay Petrochemical Industry Park; DNPP, Daya Bay Nuclear Power Plant; LNPP, Ling’ao Nuclear Power Plant.
The biological and physicochemical samplings were carried out simultaneously. A set of human activity–related environmental variables were selected. One extra sediment sample from the surface up to 10 cm depth was obtained at each station for the analyses of grain size, total organic carbon (TOC), petroleum contaminants (PCs), and heavy metals including As and Hg, which were mainly influenced by the anthropogenic input (Zhao et al., 2016). A total of 1,000 ml water sample was collected from the bottom layer per station for measurements of phosphate (PO43-), ammonia (NH4+), and chlorophyll a (Chl-a). The water samples were stored in PE bottles with special fixatives. All samples were preserved under 4°C prior to analyses. Water depth (Dep) was measured by a handheld depth sounder (Hondex PS-7). Temperature (T), salinity (Sal), and dissolved oxygen (DO) were measured in situ using a handheld multiparameter meter (YSI Pro Plus). In the laboratory, the sediment grain size was determined using a granulometer (Mastersizer 2000) capable of measuring particle sizes from 0.02 to 2,000 μm. Shepard’s sediment classification method was used to describe the types of sediment (Shepard, 1954). The analytical methods of remaining variables refer to the Marine Monitoring Specification (GB 17378.4-2007; GB 17378.5-2007).
Biological Trait Analysis
Nine biological traits subdivided into 30 modalities were used to describe the functional properties of macrobenthic communities in Daya Bay (Table 1). The traits included morphological (body size and fragility), life history (larval development and longevity), and behavioral (living habit, feeding habit, bioturbation, tolerance, and environmental position) characteristics. The selection of these traits and their implications of ecosystem functions were based on the recent reviews (Beauchard et al., 2017; Degen et al., 2018; Degen and Faulwetter 2019; Lam–Gordillo et al., 2020). Information regarding the biological traits were gathered from literature (Oug et al., 2012; Queirós et al., 2013; Jumars et al., 2015), online databases (http://www.marlin.ac.uk/biotic/; http://www.marinespecies.org/; https://www.univie.ac.at/arctictraits/; http://www.marinespecies.org/traits/index.php; http://polytraits.lifewatchgreece.eu/polytraits), and local specialists. When little or no information is available for a taxon, we referred to the information from the same genus or family. A “fuzzy coding” procedure was used to code for each taxon according to their affinity for the modalities of each trait (Chevenet et al., 1994; Bremner et al., 2003). Fuzzy-coding procedure was used to account for multiple trait modalities of an individual taxon, that is, a scoring range of 0–3 was adopted, where 0 expresses no affinity for the given trait category, 1 or 2 express partial affinity, and 3 expresses exclusive affinity. In present study, to avoid bias among different traits, the affinity scores for each trait were standardized so that the sum was equal to 1 (Darr et al., 2014).
Data Analysis
The principal component analysis (PCA) was used to investigate the variation in environmental variables (Z-score normalized) among the sampling locations. The quantification of the functional structure of communities was proceeded after obtaining the taxa matrix (table L, taxa by stations) and the fuzzy coded trait matrix (table Q, traits by taxa). CWM was calculated for each of the 30 trait modalities. The trait modality standardized scores of each taxon were multiplied by its abundance for every station and then summed over all taxa present at each station to generate a CWM table (traits by stations) with the overall frequency of each trait modality per station. The trait composition among the different sampling locations was explored with the fuzzy correspondence analysis (FCA) based on the CWM table. FCA is an ordination method appropriate for fuzzy coded data (Bremner et al., 2006). In FCA, the correlation ratio (cr) indicates the contribution of each trait to the overall variance, whereas traits with a CR value greater than 0.1 can be considered as the traits with the greatest influence on variance between stations (Liu et al., 2019). Then the CWM table was calculated by Bray–Curtis measure to construct the resemblance matrix. Based on this resemblance matrix, the permutational multivariate analysis of variance (PERMANOVA main test, with 9,999 permutations) was performed to test whether the trait composition of macrobenthic communities showed significant differences among the sampling locations/seasons. The similarity percentage (SIMPER) analysis based on the CWM table and taxa matrix with Bray–Curtis measure was performed to determine the dominant traits and species/taxonomic unit characterizing the dissimilarity among the communities.
FD was estimated by Rao’s quadratic entropy (Rao’s Q) because it incorporates functional richness, functional evenness, and functional divergence (Mouillot et al., 2013). To compare the relationship between functional diversity and taxonomic diversity, the classical taxonomic diversity index, that is, the Shannon–Wiener diversity index (H′, based on log2), was also calculated.
In order to unravel the trait-environment relationships, the BIOENV analysis was used to assess which environmental variables correlate best with the CWM values. The RLQ and fourth-corner methods were further applied to assess which traits response to environmental gradients, based on the following three tables, that is, R table (environmental variables by stations), L table, and Q table (Dray et al., 2014).
Non-parametric Kruskal–Wallis tests and Wilcoxon tests were performed to test whether the median values of environmental variables and indices (functional and taxonomic) showed significant differences between the sampling locations and between seasons. Kruskal–Wallis tests, Wilcoxon tests, PCA, FCA, and the calculation of functional and taxonomic diversity indices were run in R-3.5.3 (R Core Team, 2019) using the packages “stats,” “factoextra,” “vegan” (Oksanen et al., 2019), “ade4” (Dray and Dufour, 2007), and “FD” (Laliberté et al., 2014). PERMANOVA main test, SIMPER, and BIOENV were performed in PRIMER 7 (with PERMANOVA+).
Results
Environmental Variables
For environmental variables, notable differences among the sampling locations and among seasons were observed (Supplementary Table S1). Compared with the stations in SED and MRC, the stations in NTD and REF had higher values of water depth and salinity, whereas they showed a lower content of PCs and Hg in sediments (p < 0.05). SED showed a higher concentration of Chl-a than the other sampling locations (p < 0.05) and a higher content of TOC in sediments than MRC. Salinity, temperature, DO, Chl-a, PO43−, and NH4+ differed significantly between seasons (p < 0.05). The highest value of salinity occurred in autumn. The highest content of PO43− and NH4+ occurred in spring, while the highest concentration of Chl-a occurred in summer. The sediment types in the four sampling locations were all silty clay.
The first two principal components of the PCA accounted for 51.0–56.0% of the total variation in environmental conditions over the studied stations (Figure 2). The seasonal variation of the environmental conditions of the sampling locations was clear. But, in general, SEDs were associated with higher concentration of Chl-a and higher contents of petroleum PCs and Hg in sediments, NTD had greater percentages of silt and clay, REF was characterized by greater water depth. MRC had relatively large intra-group variations and did not show concordant characteristics among seasons.
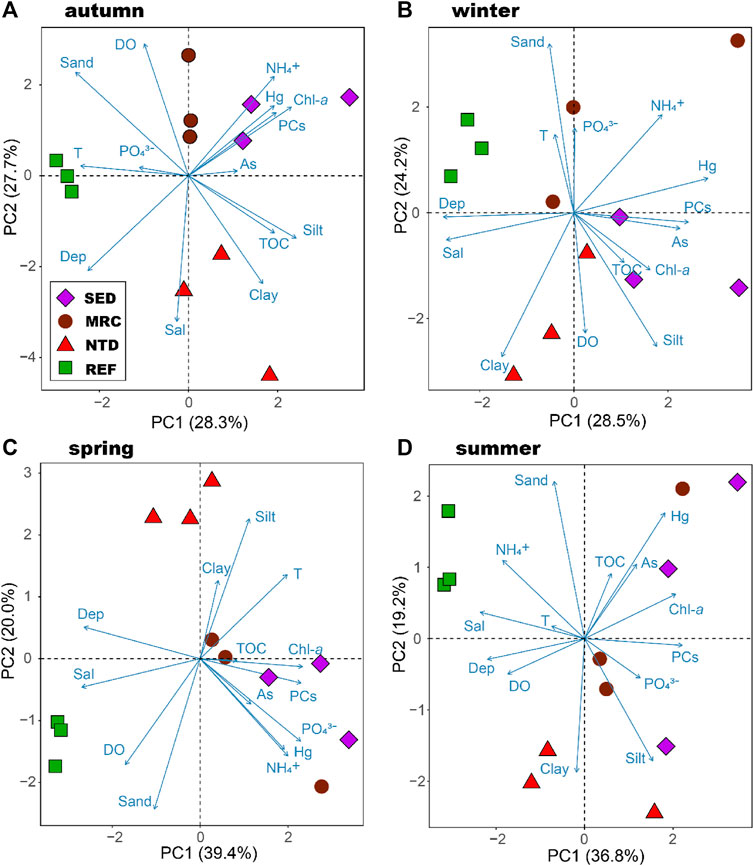
FIGURE 2. Principal component analysis of the relevant environmental variables in Daya Bay. SED, sewage discharge area; MRC, mariculture area; NTD, NPP thermal discharge area; REF, reference area. The Bering Sea.
Functional Structure of Macrobenthic Communities
A total of 163 taxa, including 80 polychaetes, 50 molluscs, 25 crustaceans, 3 echinoderms, 2 sipunculid, 1 platyhelminth, 1 nemertean and 1 chordate, were identified during the four sampling surveys in Daya Bay. Taxa matrix and fuzzy coded trait matrix are shown in Supplementary Tables S2, S3. FCA depicted the patterns in the trait composition for the stations grouped by the sampling locations and seasons (Figures 3A,B). The first two FCA axes accounted for 56.4% of the variance in the trait composition between the stations, 38.1% along axis 1, and 18.3% along axis 2. The traits, that is, bioturbation, longevity, tolerance, body size, and feeding habit were significantly related to axis 1, and environmental position was strongly correlated with both axes (Table 2).
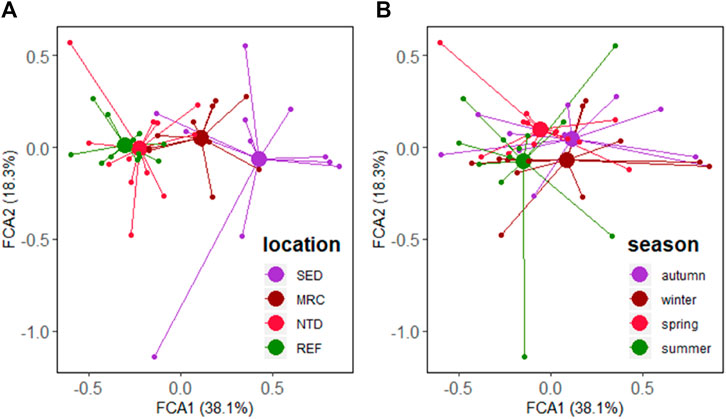
FIGURE 3. FCA ordinations of the trait composition of macrobenthic communities group by (A) location and (B) season in Daya Bay. SED, sewage discharge area; MRC, mariculture area; NTD, NPP thermal discharge area; REF, reference area.
For the trait composition of macrobenthic communities, a significant difference among the sampling locations was confirmed by the PERMANOVA main test (Pseudo-F = 9.587, p < 0.001). However, no significant difference among seasons was detected (Pseudo-F = 1.329, p = 0.215). Therefore, the comparisons of the trait composition between seasons will not be carried out in the following analyses. Pair-wise tests showed that there were significant differences between the sampling locations (t = 2.031–4.455, p < 0.01), excluding the case between NTD and REF (t = 1.268, p = 0.152).
The average values of CWM for each sampling period were plotted to visualize the functional composition of macrobenthic communities in the sampling locations (Figure 4). The SIMPER test performed on CWM values showed that the macrobenthic communities of the four sampling locations were all characterized by the following trait modalities (Table 3), that is, fragile (F1), pelagic larval development (LD1), short longevity (A1), borrower (LH3), deposit feeder (FH1), and high tolerance (T3). Nevertheless, SED and MRC exhibited a higher percentage of small body size (S1) than NTD and REF, and MRC, NTD, and REF had higher percentages of small-medium body size (S2) than SED; NTD, and REF had higher percentages of medium longevity (A2) and biodiffusor (B2) than SED and MRC, while SED was dominated by upward conveyor (B3) and MRC was dominated by surficial modifier (B1); REF had a higher percentage of intermediate tolerance (T2) than the other sampling locations; SED had a higher percentage of epifauna (EP2) than the other sampling locations. The SIMPER test performed on taxa matrix showed that (Table 4) the macrobenthic communities of SED were represented by two Polychaeta species (Polydora sp. and Prionospio polybranchiata); MRC were represented by one Nemertea species and six Polychaeta species (Cirratulidae, Goniada maculata, Mediomastus chinensis, Notomastus latericeus, Paraprionospio cristata, and Sigambra hanaokai); NTDs were represented by one Nemertea species and five Polychaeta species (Aglaophamus lyrochaeta, Cirratulidae, Cossura dimorpha, M. chinensis, and S. hanaokai); REFs were represented by one Nemertea species, nine Polychaeta species (A. dibranchis, A. lyrochaeta, Cirratulidae, Glycera sp., Laonice cirrata, Listriolobus brevirostris, Lumbrineris sp., M. chinensis, and S. hanaokai), one Crustacea species (Photis hawaiensis), and two Echinodermata species (Amphiodia (Amphispina) obtecta and Protankyra bidentata).
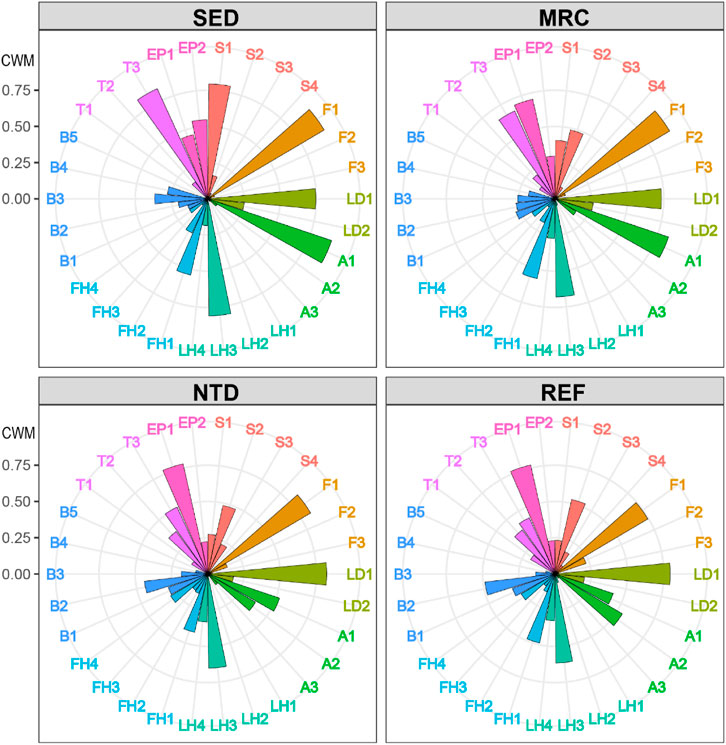
FIGURE 4. Community-weighted means of trait modalities (CWMs), average values for sampling locations. SED, sewage discharge area; MRC, mariculture area; NTD, NPP thermal discharge area; REF, reference area. See Table 1 for the meaning of the trait codes.
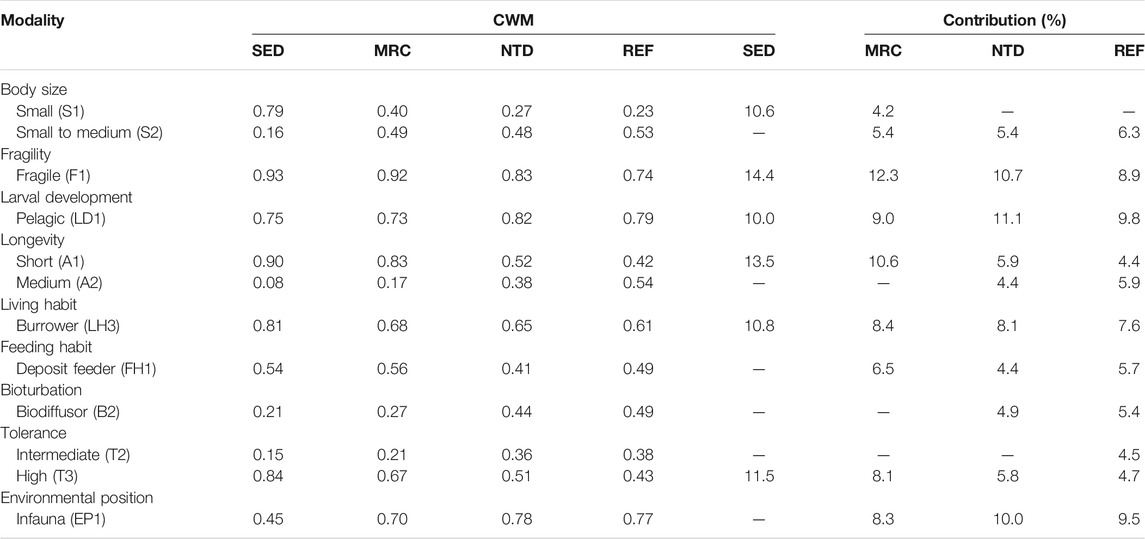
TABLE 3. Average values and contributions of community-weighted means of trait modalities (CWMs) to the similarity of macrobenthic communities in sewage discharge area (SED), mariculture area (MRC), NPP thermal discharge area (NTD), and reference area (REF), based on SIMPER analysis (cutoff applied at 70%).
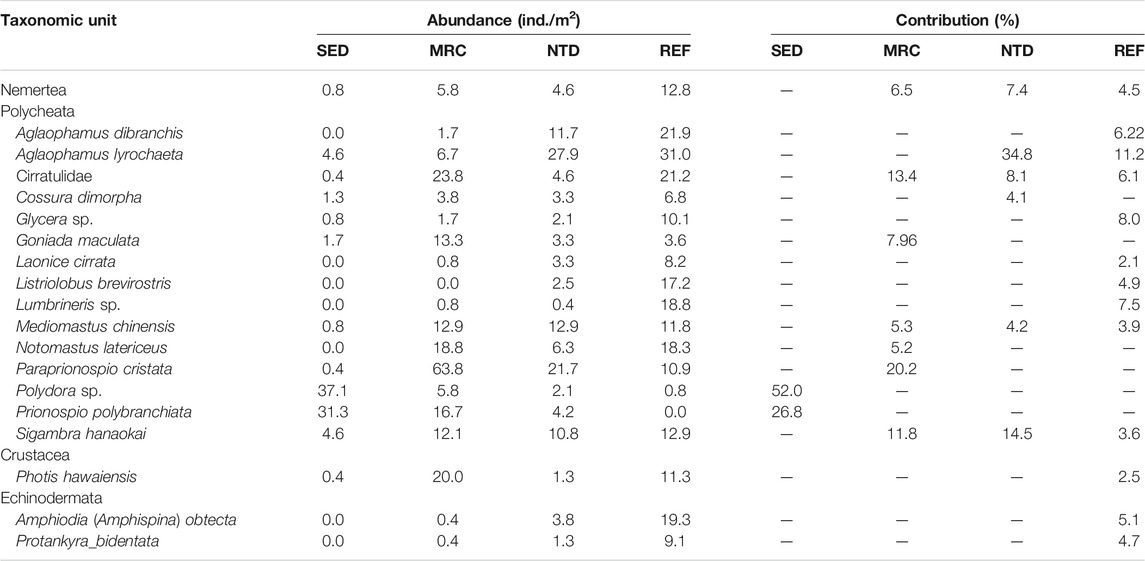
TABLE 4. Average abundance and contributions of taxonomic units to the similarity of macrobenthic communities in sewage discharge area (SED), mariculture area (MRC), NPP thermal discharge area (NTD), and reference area (REF), based on SIMPER analysis (cutoff applied at 70%).
There were significant differences in Shannon–Wiener diversity (H′) and functional diversity (Rao’s Q) among the sampling locations (p < 0.01). The highest H′ and Rao’s Q were both observed in REF, and the lowest values both occurred in SED (Figures 5A,B). The H′ was significantly higher in REF than that in NTD (p = 0.013), but there was no significant difference in Rao’s Q between them (Figures 5A,B). The H′ was slightly higher in MRC than that in NTD, and Rao’s Q was slightly higher in NTD than that in MRC (Figures 5A,B). However, there were no significant differences between MRC and NTD for both indices. Additionally, Rao’s Q responded rapidly as H′ increased in SED, MRC, and NTD, but for REF with relatively higher species diversity, the variation of Rao’s Q was small (Figure 5C).
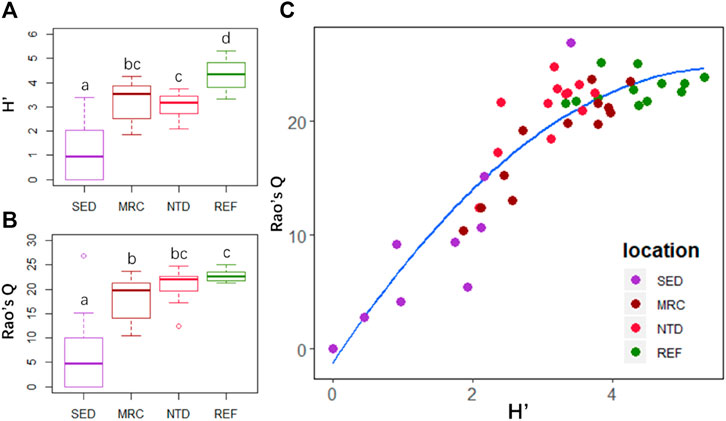
FIGURE 5. Taxonomic and functional diversity indices of macrobenthic communities in the four sampling locations: (A) boxplot of Shannon–Wiener diversity index (H′) and (B) Rao’s quadratic entropy index (Rao’s Q); (C) relationship between H′ and Rao’s Q. SED, sewage discharge area; MRC, mariculture area; NTD, NPP thermal discharge area; REF, reference area. Different lowercase letters indicate significant differences (Wilcoxon test after Kruskal–Wallis test: p < 0.05).
Relationships Between Traits and Environmental Variables
According to the BIOENV analysis, functional trait pattern was best correlated with the combination of Dep, DO, Chl-a, NH4+, and PCs (ρ = 0.470). The combination of RLQ and fourth-corner analysis revealed 14 out of the 30 trait modalities were significantly correlated (p < 0.05) with the environmental variables (Figure 6). Specifically, the frequency of small body size (S1) was positively associated with the contents of NH4+, As, Hg, and PCs, and negatively associated with water depth, salinity, and the percentage of clay. Small-medium (S2) was negatively associated with the contents of NH4+ and Hg. Pelagic larval development (LD1) was more abundant in higher percentage of clay sediments and benthic larval development (LD2) had the opposite trend. Animals with short longevity (A1) were more abundant in PCs/Hg enriched sediments but less abundant in greater water depth, salinity, and clay percentage environments. The frequency of medium longevity (A2) was positively influenced by water salinity, and negatively influenced by PCs, silt, Hg, and NH4+. Filter/suspension feeder (FH2) was positively correlated with NH4+, and predator (FH4) was positively correlated with water salinity. Upward conveyor (B3) and downward conveyor (B4) were more abundant in DO, Chl-a, and PCs enriched environments, while the surficial modifier (B1) was less abundant in DO enriched environments, and downward conveyor (B4) was less abundant in PO43--enriched environments. Animals with high tolerance (T3) were scarce in deeper waters. Epifauna (EP2) was more abundant in NH4+-, As-, and Hg-enriched environments and in fauna (EP1) had the opposite trend.
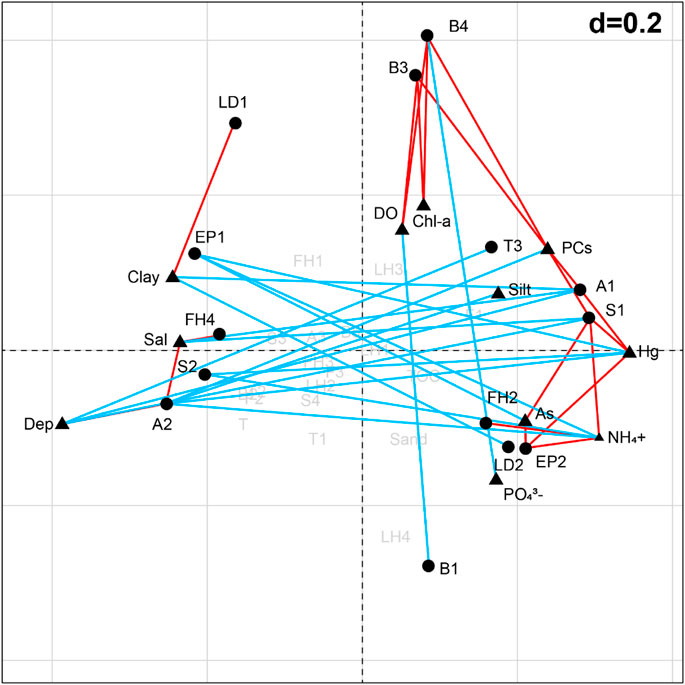
FIGURE 6. Correlations between the environmental variables and trait modalities carried out by fourth-corner on the factorial map of RLQ analysis. Red/blue lines represent positive/negative correlations. Triangles represent the environmental variables; circles represent the trait modalities. See Table 1 for the meaning of the trait codes.
Discussion
Effect of Anthropogenic and Natural Drivers on Environmental Conditions in Daya Bay
Previous studies have confirmed the significant impacts of human activities on Daya Bay environments, for example, petrochemical sewage discharge (Xu et al., 2014; Liu et al., 2018), mariculture (Qi et al., 2019), and thermal discharge (Yu et al., 2010; Hao et al., 2016). In the present study, water depth was associated with environment stress. When water depth increased, the content of nutrients (PO43-, NH4+) in water columns and contaminants (PCs, Hg) in sediments decreased, and vice versa. It can be partly attributed to the human activities such as sewage discharge and mariculture that increase the chemical pollution load located in shallow waters. However, the relatively low Chl-a and TOC contents in MRC may be due to its higher sea water renewal and the dilution and dispersion rates of contaminants. MRC is located in the eastern part of Daya Bay, which is the main channel for water exchange with the outer sea (Wu et al., 2007). Due to the diurnal fluctuations in water temperature, the heating effect of thermal discharge was not reflected from our in situ surveys. Furthermore, the precipitation and the East Asian Monsoon also have an important influence on water quality in Daya Bay. We observed that the higher content of PO43−, NH4+, and Chl-a occurred in wet season (spring and summer). When the East Asian Monsoon prevails, the nutrients are washed out from the land into the bay, causing differences in the water quality between the wet season and dry season (Wu et al., 2009). Additionally, the eastern Guangdong coastal upwelling promotes primary productivity explaining the high Chl-a concentration in summer (Gu et al., 2012). As a result, the environmental conditions of Daya Bay were determined by both anthropogenic and natural factors.
Functional Response of Macrobenthic Communities to Human Activities
Assessments of benthic community responses to human activities are an important task when considering the significance of the impacts on the sustainability of marine ecosystem functions. Trait distribution emerges through evolution and natural selection and is mediated by the environment, biological interactions, and anthropogenic drivers (Barton et al., 2016). Given that the sensitivity of traits to changes in habitat disturbance, the order of extinction of traits matters for assessing the decline in ecosystem functioning (Boersma et al., 2016; Kenny et al., 2018). For instance, organic pollution affects mainly organisms sensitive to oxygen depletion, whereas acidification affects those sensitive calcium depletion (Bolam et al., 2017).
We explored the functional structure of macrobenthic communities in Daya Bay subject to the potential impacts from different human activities, in particular, assessing the potential effects of contaminants and other environmental variables on macrofaunal functional identity and diversity. The FCA carried out on trait composition highlighted the differences in the sampling locations associated with different human activities (see Figure 3A), a result corroborated also by the PERMANOVA main test (Pseudo-F = 9.587, p < 0.001). However, no significant differences were observed between NTD and REF (t = 1.268, p = 0.152). We found that bioturbation, longevity, tolerance, body size, feeding habit, and environmental position, were key traits in characterizing the functional structure of macrobenthic communities (See Table 2). Small body size (<10 mm), short longevity (<2 years), and high tolerance animals were more abundant in SED and MRC (higher proportions in SED), which were represented by the species of Spionidae, that is, Polydora sp., P. polybranchiata, and P. cristata. These are typical features of opportunistic species within nutrient-enriched or stressed habitats (Oug et al., 2012; Nasi et al., 2018). Although fragile animals were dominant in Daya Bay, this was more evident in SED and MRC, indicating they were not suitable for animals with shells, for example, mollusks and crustaceans. The dominant bioturbation mode in SED was upward conveyor (B2). Upward conveyors are efficient at moving deep horizon particles to the sediment surface (Kristensen et al., 2012). Surficial modifiers (B1) in general with filter/suspension feeding habit, a greater amount of food provided by mussel biodeposition can explain why they were more abundant in MRC (Lacoste et al., 2019). The activities of surficial modifiers are restricted to the surficial layer (0–2 cm) of the sedimentary profile (Queirós et al., 2013); therefore, the limited bioturbation potential could lead to the deterioration of sediment quality in MRC. Less infauna (EP1) found in SED was supposedly related to the anoxic sediments there. Comparatively, small-medium body size (S2), medium longevity (A2), and intermediate tolerant (T2) species were more abundant in NTD and REF (higher proportions in REF), indicating their relatively good environmental status. A two-decade study demonstrated that the body size of the macrobenthic fauna became smaller and biodiversity was increasingly simplified in Daya Bay (Wang et al., 2008). It is true, from our findings, that a small Polychaeta species Sigambra hanaokai was formerly rare in Daya Bay and has recently become ubiquitous.
On the other hand, we found a similar functional structure between seasons (Pseudo-F = 1.329, p = 0.215), indicating low temporal variability for the trait composition in macrobenthic communities. While the taxonomic composition was quite different between seasons (Pseudo-F = 1.682, p = 0.006). Most benthic organisms reproduce and settle at specific times of the year (Reiss and Kroncke, 2005), which makes it difficult to compare works conducted in different seasons. Yet the seasonal stability of functional structure seems to make up for this deficiency.
The taxonomic and functional diversity indices showed clear differences between different human activities (Figures 5A,B). The relatively lower taxonomic and functional diversity were found in the disturbed areas (i.e., SED, MRC, and NTD). It is consistent with previous studies which noted that the inner bay of the Daya Bay disturbed by mariculture and sewage pollutions had lower taxonomic richness and diversity (Arbi et al., 2017), and the biomass and species richness near nuclear power plants had dropped dramatically relative to the results of pre-operational surveys (Wang et al., 2008). Compared with MRC, NTD had relatively lower taxonomic diversity but higher functional diversity. This result suggested that thermal discharge might cause a decline in taxonomic diversity but not affect the functional diversity, if the reduced species contribute little to function in the community. Thermal discharge have been shown to affect the abundance, richness, and feeding habit of freshwater macroinvertebrate (Worthington et al., 2015; Han et al., 2017). However, most previous studies have demonstrated that the influence of thermal discharge on marine macrobenthic community was insignificant (Lardicci et al., 1999; Kwon et al., 2017; Jung et al., 2018; Lin et al., 2018), possibly benefitting the huge bulk of seawater. Kwon et al. (2017) assumed that if thermal discharge could exert an influence on the macrobenthic community depending on the season, summer may be a bad one. Lin et al. (2018) admitted that the elevated seawater temperature at the bottom might not be high enough to significantly affect the macrobenthos, but the stratification and elevated temperature could affect the phytoplankton at the bottom. In fact, the shift of pelagic food web in thermal discharge areas has been identified (Chen et al., 2015; Hao et al., 2016). The relatively low proportion of deposit feeder (FH1) in NTD might be a response to this shift. Deposit feeders ingest sediments and use organic matter and microbial organisms in sediments as food, such as benthic diatoms. However, the effects of thermal discharge on benthic diatoms have been demonstrated (Ingleton and McMinn, 2012). Despite the higher level of taxonomic diversity, REF showed little increase in functional diversity over NTD. In other words, there were a larger number of functionally similar species in REF. This indicates that this community potentially has a higher functional redundancy than the other communities. Functional redundancy is a policy that insures against changes in the functioning of the ecosystem due to the loss of species (Dolédec and Bonada, 2013). Although NTP and REF are similar in functional structure, a potential decrease in functional diversity in NTP can be expected when environmental degradation continues. Therefore, the combination of taxonomic and functional diversity can be a reliable method for ecological risk assessment.
In the present study, the trait composition was primarily influenced by water depth, DO, Chl-a, NH4+, and petroleum contaminants (PCs) in sediments. Generally, water depth and sediment type are considered as the main natural factors that obviously influence the distribution of macrobenthic fauna (Bolam et al., 2017; Kenny et al., 2018; Liu et al., 2019). Although natural factors showed influence on the contaminants, given the consistency of the sediment type (silty clay) and the small water depth range (4–16 m) in Daya Bay, we assumed that the human-induced environmental changes were the main factors affecting macrobenthic communities. Combined with the correlations between the environmental variables and trait modalities (Figure 6), we suggested that the traits “body size,” “longevity,” “feeding habit,” “bioturbation,” “tolerance,” “environmental position,” and the diversity indices H′ and Rao’s Q could be served as the promising indicators of how macrobenthic fauna react to the stress caused by human activities in Daya Bay.
Ecosystem Functioning Effects
The present study on macrobenthic communities in Daya Bay showed that the BTA method detected specific functional features correlated with human disturbances, for instance, the change from small body size organisms to larger body size organisms along pollution gradients. Of particular interest is the demonstration of the impact of human activities on body size, feeding habit, and bioturbation. All these traits express the aspects of biological activities that are important for sediment reworking, and nutrient uptake and turnover. It is a first step toward understanding how human disturbances can affect ecosystem functioning. However, the accurate assessment of the magnitude of biogeochemical processes loss in response to functional structure shifts still requires further studies (Gusmao et al., 2016).
Cautions and Limitations
The performance of the BTA relies on the selected traits and metrics used during the analytical process (Bremner, 2008). In the present study, a priori selection of traits was based on a trade-off between their efficacy for describing ecological functioning and the effort required to gather information on the selected traits. Some traits, such as reproductive type and mobility, are especially important to analyze recolonization when disturbances are absent. At present, it seems unrealistic to incorporate all possible functional features that could be of importance. Darr et al. (2014) suggested that biomass-based BTA represent an informative tool to describe the functional structure of macrobenthic community. In contrast, Gusmao et al. (2016) considered that abundance is a more suitable metric than biomass. In fact, the discrepancy may depend on the selected traits. Because some traits (e.g., larval development) are linked to abundance and others (e.g., bioturbation) are directly related to biomass. However, how to effectively integrate abundance and biomass into the corresponding traits is still a challenging question.
Conclusion
In the present study, BTA was used to discern shifts in the functional structure of macrobenthic communities associated with different human activities. The effects of contaminants and other environmental variables on macrofaunal functional identity and diversity were observed. However, the seasonality of trait expressions in communities was not significant. Bioturbation, longevity, tolerance, body size, feeding habit, and environmental position were the traits most affected by the human disturbances. And water depth, DO, Chl-a, NH4+, and petroleum contaminants (PCs) in sediments were the main factors related to the trait composition. Small body size, short longevity, and high tolerance species were more abundant in the contaminated areas (SED and MRC). The taxonomic and functional diversity indices revealed obvious differences among the sampling locations. Although NTD and REF were similar in trait composition, a potential function loss in NTD can be detected through the integrated analysis with taxonomic diversity.
Data Availability Statement
The original contributions presented in the study are included in the article/Supplementary Material; further inquiries can be directed to the corresponding author.
Author Contributions
LC conceived of the study and obtained funding. LC, YR, XC, XZ, SF, and HH were responsible for field and laboratory work. LC conducted the identification of benthic macrofauna and created many of the manuscript elements. YR led the data analysis and writing effort, with significant contributions to these by all authors.
Funding
This work was supported by the National Key Research and Development Program of China (2018YFC1407501), Central Public-interest Scientific Institution Basal Research Fund, South China Sea Fisheries Research Institute, CAFS (No. 2020TS02), and Central Public-interest Scientific Institution Basal Research Fund, CAFS (NO. 2020TD15).
Conflict of Interest
The authors declare that the research was conducted in the absence of any commercial or financial relationships that could be construed as a potential conflict of interest.
Publisher’s Note
All claims expressed in this article are solely those of the authors and do not necessarily represent those of their affiliated organizations, or those of the publisher, the editors, and the reviewers. Any product that may be evaluated in this article, or claim that may be made by its manufacturer, is not guaranteed or endorsed by the publisher.
Acknowledgments
We thank Dr. Senjie Lin of University of Connecticut for providing valuable comments on earlier versions of the manuscript. We are sincerely grateful to the Huizhou Ocean Technology Center for providing us with environmental data. We would like to thank Director Haoliang Liang and Dr. Xingjun Wang for their support in sample collection. All the members involved in this project are to be acknowledged here.
Supplementary Material
The Supplementary Material for this article can be found online at: https://www.frontiersin.org/articles/10.3389/fenvs.2021.766580/full#supplementary-material
Supplementary Table S1 | Environmental variables (mean ± SD) for four seasons at the four sampling locations. Dep, depth; Sal, salinity; T, temperature; PCs, petroleum contaminants in sediments; TOC, total organic carbon in sediments; As, arsenium in sediments; Hg, mercury in sediments.
Supplementary Table S2 | List of abundance of benthic macrofauna recorded at each station of the four sampling locations of Daya Bay in four seasons from 2017 to 2018 (ind./m2).
Supplementary Table S3 | Fuzzy coded trait data of each taxa of benthic macrofauna recorded at the four sampling locations of Daya Bay in four seasons from 2017 to 2018.
References
Arbi, I., Zhang, J., Liu, S., Wu, Y., and Huang, X. (2017). Benthic Habitat Health Assessment Using Macrofauna Communities of a Sub-Tropical Semi-Enclosed Bay Under Excess Nutrients. Mar. Pollut. Bull. 119, 39–49. doi:10.1016/j.marpolbul.2017.03.042
Barbier, E. B., Koch, E. W., Silliman, B. R., Hacker, S. D., Wolanski, E., Primavera, J., et al. (2008). Coastal Ecosystem-Based Management with Nonlinear Ecological Functions and Values. Science 319, 321–323. doi:10.1126/science.1150349
Barton, A. D., Dutkiewicz, S., Andersen, K. H., Fiksen, Ø., Follows, M. J., Mouw, C. B., Record, N. R., and Rynearson, T. A. (2016). “Report on the “Trait-Based Approaches to Ocean Life” Scoping Workshop,” (Accessed October 5–8, 2015).
Beauchard, O., Veríssimo, H., Queirós, A. M., and Herman, P. M. J. (2017). The Use of Multiple Biological Traits in marine Community Ecology and its Potential in Ecological Indicator Development. Ecol. Indic. 76, 81–96. doi:10.1016/j.ecolind.2017.01.011
Bellwood, D. R., Hoey, A. S., and Hughes, T. P. (2012). Human Activity Selectively Impacts the Ecosystem Roles of Parrotfishes on Coral Reefs. Proc. Biol. Sci. 279, 1621–1629. doi:10.1098/rspb.2011.1906
Boersma, K. S., Dee, L. E., Miller, S. J., Bogan, M. T., Lytle, D. A., and Gitelman, A. I. (2016). Linking Multidimensional Functional Diversity to Quantitative Methods: A Graphical Hypothesis‐evaluation Framework. Ecology 97, 583–593. doi:10.1890/15-0688
Bolam, S. G., Coggan, R. C., Eggleton, J., Diesing, M., and Stephens, D. (2014). Sensitivity of Macrobenthic Secondary Production to Trawling in the English Sector of the Greater North Sea: A Biological Trait Approach. J. Sea Res. 85, 162–177. doi:10.1016/j.seares.2013.05.003
Bolam, S. G., Garcia, C., Eggleton, J., Kenny, A. J., Buhl-Mortensen, L., Gonzalez-Mirelis, G., et al. (2017). Differences in Biological Traits Composition of Benthic Assemblages between Unimpacted Habitats. Mar. Environ. Res. 126, 1–13. doi:10.1016/j.marenvres.2017.01.004
Bolam, S. G., McIlwaine, P. S. O., and Garcia, C. (2016). Application of Biological Traits to Further Our Understanding of the Impacts of Dredged Material Disposal on Benthic Assemblages. Mar. Pollut. Bull. 105, 180–192. doi:10.1016/j.marpolbul.2016.02.031
Bonaglia, S., Brüchert, V., Callac, N., Vicenzi, A., Chi Fru, E., and Nascimento, F. J. A. (2017). Methane Fluxes from Coastal Sediments Are Enhanced by Macrofauna. Sci. Rep. 7, 13145. doi:10.1038/s41598-017-13263-w
Borja, Á., Elliott, M., Carstensen, J., Heiskanen, A.-S., and van de Bund, W. (2010). Marine Management - Towards an Integrated Implementation of the European Marine Strategy Framework and the Water Framework Directives. Mar. Pollut. Bull. 60, 2175–2186. doi:10.1016/j.marpolbul.2010.09.026
Bremner, J., Rogers, S., and Frid, C. (2003). Assessing Functional Diversity in marine Benthic Ecosystems: a Comparison of Approaches. Mar. Ecol. Prog. Ser. 254, 11–25. doi:10.3354/meps254011
Bremner, J., Rogers, S., and Frid, C. (2006). Methods for Describing Ecological Functioning of marine Benthic Assemblages Using Biological Traits Analysis (BTA). Ecol. Indic. 6, 609–622. doi:10.1016/j.ecolind.2005.08.026
Bremner, J. (2008). Species' Traits and Ecological Functioning in marine Conservation and Management. J. Exp. Mar. Biol. Ecol. 366, 37–47. doi:10.1016/j.jembe.2008.07.007
Cardellicchio, N., Buccolieri, A., Giandomenico, S., Lopez, L., Pizzulli, F., and Spada, L. (2007). Organic Pollutants (PAHs, PCBs) in Sediments from the Mar Piccolo in Taranto (Ionian Sea, Southern Italy). Mar. Pollut. Bull. 55, 451–458. doi:10.1016/j.marpolbul.2007.09.007
Chen, X., Gao, H., Yao, X., Fang, H., Chen, Z., and Xu, Z. (2010). Ecosystem-based Assessment Indices of Restoration for Daya Bay Near a Nuclear Power Plant in South China. Environ. Sci. Technol. 44, 7589–7595. doi:10.1021/es1008592
Chen, Z., Xu, S., and Qiu, Y. (2015). Using a Food-Web Model to Assess the Trophic Structure and Energy Flows in Daya Bay, China. Continental Shelf Res. 111, 316–326. doi:10.1016/j.csr.2015.08.013
Chevene, F., Doléadec, S., and Chessel, D. (1994). A Fuzzy Coding Approach for the Analysis of Long-Term Ecological Data. Freshw. Biol 31, 295–309. doi:10.1111/j.1365-2427.1994.tb01742.x
Cloern, J. E., Abreu, P. C., Carstensen, J., Chauvaud, L., Elmgren, R., Grall, J., et al. (2016). Human Activities and Climate Variability Drive Fast-Paced Change across the World's Estuarine-Coastal Ecosystems. Glob. Change Biol. 22, 513–529. doi:10.1111/gcb.13059
Cooper, K. M., Barrio Froján, C. R. S., Defew, E., Curtis, M., Fleddum, A., Brooks, L., et al. (2008). Assessment of Ecosystem Function Following marine Aggregate Dredging. J. Exp. Mar. Biol. Ecol. 366, 82–91. doi:10.1016/j.jembe.2008.07.011
Darr, A., Gogina, M., and Zettler, M. L. (2014). Functional Changes in Benthic Communities along a Salinity Gradient- A Western Baltic Case Study. J. Sea Res. 85, 315–324. doi:10.1016/j.seares.2013.06.003
Degen, R., Aune, M., Bluhm, B. A., Cassidy, C., Kędra, M., Kraan, C., et al. (2018). Trait-based Approaches in Rapidly Changing Ecosystems: A Roadmap to the Future Polar Oceans. Ecol. Indic. 91, 722–736. doi:10.1016/j.ecolind.2018.04.050
Degen, R., and Faulwetter, S. (2019). The Arctic Traits Database - A Repository of Arctic Benthic Invertebrate Traits. Earth Syst. Sci. Data 11, 301–322. doi:10.5194/essd-11-301-2019
Dias, A. T. C., Berg, M. P., de Bello, F., Van Oosten, A. R., Bílá, K., and Moretti, M. (2013). An Experimental Framework to Identify Community Functional Components Driving Ecosystem Processes and Services Delivery. J. Ecol. 101, 29–37. doi:10.1111/1365-2745.12024
Dolédec, S., and Bonada, N. (2013). So What? Implications of Loss of Biodiversity for Ecosystem Functioning. River Conservation Challenges and Opportunities. Madrid: Fundacion BBVA, 169–192.
Dray, S., Choler, P., Dolédec, S., Peres-Neto, P. R., Thuiller, W., Pavoine, S., et al. (2014). Combining the Fourth-Corner and the RLQ Methods for Assessing Trait Responses to Environmental Variation. Ecology 95, 14–21. doi:10.1890/13-0196.1
Dray, S., and Dufour, A. (2007). The ade4 Package: Implementing the Duality Diagram for Ecologists. J. Stat. Soft. 22 (4), 1–20. doi:10.18637/jss.v022.i04
Egres, A. G., Hatje, V., Miranda, D. A., Gallucci, F., and Barros, F. (2019). Functional Response of Tropical Estuarine Benthic Assemblages to Perturbation by Polycyclic Aromatic Hydrocarbons. Ecol. Indic. 96, 229–240. doi:10.1016/j.ecolind.2018.08.062
Franzo, A., Auriemma, R., Nasi, F., Vojvoda, J., Pallavicini, A., Cibic, T., et al. (2016). Benthic Ecosystem Functioning in the Severely Contaminated Mar Piccolo of Taranto (Ionian Sea, Italy): Focus on Heterotrophic Pathways. Environ. Sci. Pollut. Res. 23, 12645–12661. doi:10.1007/s11356-015-5339-0
Frid, R. S., Nicholson, M., Ellis, J. R., and Freeman, S. (2000). “Using Biological Characteristics to Develop New Indices of Ecosystem Health”, in 88th Annual ICES Science Conference: Mini-symposium on Defining the Role of ICES in Supporting Biodiversity Conservation, Copenhagen, Denmark.
Gagic, V., Bartomeus, I., Jonsson, T., Taylor, A., Winqvist, C., Fischer, C., et al. (2015). Functional Identity and Diversity of Animals Predict Ecosystem Functioning Better Than Species-Based Indices. Proc. R. Soc. B. 282, 20142620. doi:10.1098/rspb.2014.2620
Gao, X., and Chen, S. (2008). Petroleum Pollution in Surface Sediments of Daya Bay, South China, Revealed by Chemical Fingerprinting of Aliphatic and Alicyclic Hydrocarbons. Estuar. Coast. Shelf Sci. 80, 95–102. doi:10.1016/j.ecss.2008.07.010
Garnier, E., Cortez, J., Billès, G., Navas, M.-L., Roumet, C., Debussche, M., et al. (2004). Plant Functional Markers Capture Ecosystem Properties During Secondary Succession. Ecology 85, 2630–2637. doi:10.1890/03-0799
Gu, Y., Pan, J., and Lin, H. (2012). Remote Sensing Observation and Numerical Modeling of an Upwelling Jet in Guangdong Coastal Water. J. Geophys. Res. Oceans 117. doi:10.1029/2012jc007922
Gusmao, J. B., Brauko, K. M., Eriksson, B. K., and Lana, P. C. (2016). Functional Diversity of Macrobenthic Assemblages Decreases in Response to Sewage Discharges. Ecol. Indic. 66, 65–75. doi:10.1016/j.ecolind.2016.01.003
Halpern, B. S., Frazier, M., Potapenko, J., Casey, K. S., Koenig, K., Longo, C., et al. (2015). Spatial and Temporal Changes in Cumulative Human Impacts on the World's Ocean. Nat. Commun. 6, 7615. doi:10.1038/ncomms8615
Halpern, B. S., Walbridge, S., Selkoe, K. A., Kappel, C. V., Micheli, F., D’Agrosa, C., et al. (2008). A Global Map of Human Impact on marine Ecosystems. Science 319, 948–952. doi:10.1126/science.1149345
Han, J. S., Wang, J. H., Kim, J. E., Jung, J. H., Bae, Y. J., Choi, J. K., et al. (2017). The Effects of thermal Discharge on Benthic Macroinvertebrate Communities Structure in Buso Stream. Korean J. Environ. Biol. 35, 83–94. doi:10.11626/kjeb.2017.35.1.083
Hao, Y., Tang, D., Boicenco, L., and Wang, S. (2016). Environmental Ecological Response to Increasing Water Temperature in the Daya Bay, Southern China in 1982-2012. Nr 07, 184–192. doi:10.4236/nr.2016.74017
Ingleton, T., and McMinn, A. (2012). Thermal Plume Effects: A Multi-Disciplinary Approach for Assessing Effects of thermal Pollution on Estuaries Using Benthic Diatoms and Satellite Imagery. Estuarine, Coastal Shelf Sci. 99, 132–144. doi:10.1016/j.ecss.2011.12.024
Jumars, P. A., Dorgan, K. M., and Lindsay, S. M. (2015). Diet of Worms Emended: an Update of Polychaete Feeding Guilds. Annu. Rev. Mar. Sci. 7, 497–520. doi:10.1146/annurev-marine-010814-020007
Jung, Y.-H., Kim, H. J., and Park, H.-S. (2018). Thermal Discharge Effects on the Species Composition and Community Structure of Macrobenthos in Rocky Intertidal Zone Around the Taean Thermoelectric Power Plant, Korea. Ocean Polar Res. 40, 59–67.
Ke, Z., Tan, Y., Huang, L., Liu, J., Xiang, C., Zhao, C., et al. (2019). Significantly Depleted 15N in Suspended Particulate Organic Matter Indicating a strong Influence of Sewage Loading in Daya Bay, China. Sci. Total Environ. 650, 759–768. doi:10.1016/j.scitotenv.2018.09.076
Kenny, A. J., Jenkins, C., Wood, D., Bolam, S. G., Mitchell, P., Scougal, C., et al. (2018). Assessing Cumulative Human Activities, Pressures, and Impacts on North Sea Benthic Habitats Using a Biological Traits Approach. ICES J. Mar. Sci. 75, 1080–1092. doi:10.1093/icesjms/fsx205
Kristensen, E., Penha-Lopes, G., Delefosse, M., Valdemarsen, T., Quintana, C., and Banta, G. (2012). What is Bioturbation? The Need for a Precise Definition for Fauna in Aquatic Sciences. Mar. Ecol. Prog. Ser. 446, 285–302. doi:10.3354/meps09506
Kwon, S. H., Yu, O. H., and Lee, H.-G. (2017). Community Structure of Macrobenthos Around the thermal Discharge Area of the Uljin Nuclear Power Plant in the East Sea, Korea. Ocean Polar Res. 39, 125–148. doi:10.4217/opr.2017.39.2.125
Lacoste, É., Weise, A. M., Lavoie, M.-F., Archambault, P., and McKindsey, C. W. (2019). Changes in Infaunal Assemblage Structure Influence Nutrient Fluxes in Sediment Enriched by Mussel Biodeposition. Sci. Total Environ. 692, 39–48. doi:10.1016/j.scitotenv.2019.07.235
Laliberte, E., and Legendre, P. 2010. A Distance-Based Framework for Measuring Functional Diversity from Multiple Traits. Ecology. 91, 299–305. doi:10.1890/08-2244.1
Laliberté, E., Legendre, P., and Shipley, B. (2014). FD: Measuring Functional Diversity from Multiple Traits, and Other Tools for Functional Ecology. R package version 1.0-12.
Lam-Gordillo, O., Baring, R., and Dittmann, S. (2020). Ecosystem Functioning and Functional Approaches on marine Macrobenthic Fauna: A Research Synthesis towards a Global Consensus. Ecol. Indic. 115, 106379. doi:10.1016/j.ecolind.2020.106379
Lardicci, C., Rossi, F., and Maltagliati, F. (1999). Detection of Thermal Pollution: Variability of Benthic Communities at Two Different Spatial Scales in an Area Influenced by a Coastal Power Station. Mar. Pollut. Bull. 38, 296–303. doi:10.1016/s0025-326x(98)00149-0
Lin, J., Zou, X., and Huang, F. (2018). Effects of the thermal Discharge from an Offshore Power Plant on Plankton and Macrobenthic Communities in Subtropical China. Mar. Pollut. Bull. 131, 106–114. doi:10.1016/j.marpolbul.2018.04.005
Lindqvist, S. (2014). Transport by Benthic Macrofauna: Functional Classification and Biogeochemical Response. Gothenburg: University of Gothenburg.
Liu, J.-J., Ni, Z.-X., Diao, Z.-H., Hu, Y.-X., and Xu, X.-R. (2018). Contamination Level, Chemical Fraction and Ecological Risk of Heavy Metals in Sediments from Daya Bay, South China Sea. Mar. Pollut. Bull. 128, 132–139. doi:10.1016/j.marpolbul.2018.01.021
Liu, K., Lin, H., He, X., Huang, Y., Li, Z., Lin, J., et al. (2019). Functional Trait Composition and Diversity Patterns of marine Macrobenthos across the Arctic Bering Sea. Ecol. Indic. 102, 673–685. doi:10.1016/j.ecolind.2019.03.029
Lotze, H. K., Lenihan, H. S., Bourque, B. J., Bradbury, R. H., Cooke, R. G., Kay, M. C., et al. (2006). Depletion, Degradation, and Recovery Potential of Estuaries and Coastal Seas. Science 312, 1806–1809. doi:10.1126/science.1128035
Ma, Y. e., Ke, Z., Huang, L., and Tan, Y. (2014). Identification of Human-Induced Perturbations in Daya Bay, China: Evidence from Plankton Size Structure. Cont. Shelf Res. 72, 10–20. doi:10.1016/j.csr.2013.10.012
Mason, N. W. H., de Bello, F., Mouillot, D., Pavoine, S., and Dray, S. (2013). A Guide for Using Functional Diversity Indices to Reveal Changes in Assembly Processes along Ecological Gradients. J. Veg Sci. 24, 794–806. doi:10.1111/jvs.12013
Mason, N. W. H., Mouillot, D., Lee, W. G., and Wilson, J. B. 2005. Functional Richness, Functional Evenness and Functional Divergence: The Primary Components of Functional Diversity. Oikos 111, 112–118. doi:10.1111/j.0030-1299.2005.13886.x
Mouillot, D., Graham, N. A. J., Villéger, S., Mason, N. W. H., and Bellwood, D. R., (2013). A Functional Approach Reveals Community Responses to Disturbances. Trends Ecol. Evol. 28, 167–177. doi:10.1016/j.tree.2012.10.004
Mouillot, D., Villéger, S., Parravicini, V., Kulbicki, M., Arias-González, J. E., Bender, M., et al. (2014). Functional Over-redundancy and High Functional Vulnerability in Global Fish Faunas on Tropical Reefs. Proc. Natl. Acad. Sci. 111, 13757–13762. doi:10.1073/pnas.1317625111
Naeem, S., Duffy, J. E., and Zavaleta, E. (2012). The Functions of Biological Diversity in an Age of Extinction. Science 336, 1401–1406. doi:10.1126/science.1215855
Nasi, F., Nordström, M. C., Bonsdorff, E., Auriemma, R., Cibic, T., and Del Negro, P. (2018). Functional Biodiversity of Marine Soft-Sediment Polychaetes from Two Mediterranean Coastal Areas in Relation to Environmental Stress. Mar. Environ. Res. 137, 121–132. doi:10.1016/j.marenvres.2018.03.002
Nunes de Souza, J. V., Martins Vieira, M. L., De Assis, J. E., Zanardi - Lamardo, E., Gomes, P. B., and Botelho de Souza, J. R. (2021). Responses of Functional Traits of Macrobenthic Communities on the Presence of Polycyclic Aromatic Hydrocarbons Contamination in Three Tropical Estuaries. Estuarine, Coastal Shelf Sci. 250, 107105. doi:10.1016/j.ecss.2020.107105
Oksanen, J., Guillaume Blanchet, F., Friendly, M., Kindt, R., Legendre, P., McGlinn, D., et al. (2019). vegan: Community Ecology Package. R package version 2.5-6. Available at: https://CRAN.R-project.org/package=vegan.
Oug, E., Fleddum, A., Rygg, B., and Olsgard, F. (2012). Biological Traits Analyses in the Study of Pollution Gradients and Ecological Functioning of Marine Soft Bottom Species Assemblages in a Fjord Ecosystem. J. Exp. Mar. Biol. Ecol. 432-433, 94–105. doi:10.1016/j.jembe.2012.07.019
Patrício, J., Neto, J. M., Teixeira, H., Salas, F., and Marques, J. C. (2009). The Robustness of Ecological Indicators to Detect Long-Term Changes in the Macrobenthos of Estuarine Systems. Mar. Environ. Res. 68, 25–36. doi:10.1016/j.marenvres.2009.04.001
Petchey, O. L., and Gaston, K. J. (2006). Functional Diversity: Back to Basics and Looking Forward. Ecol. Lett. 9, 741–758. doi:10.1111/j.1461-0248.2006.00924.x
Pharr, D. Y., Mckenzie, J. K., and Hickman, A. B. (1992). Fingerprinting Petroleum Contamination Using Synchronous Scanning Fluorescence Spectroscopy. Ground Water 30, 484–489. doi:10.1111/j.1745-6584.1992.tb01523.x
Qi, Z., Shi, R., Yu, Z., Han, T., Li, C., Xu, S., et al. (2019). Nutrient Release from Fish Cage Aquaculture and Mitigation Strategies in Daya Bay, Southern China. Mar. Pollut. Bull. 146, 399–407. doi:10.1016/j.marpolbul.2019.06.079
Qu, B., Song, J., Yuan, H., Li, X., Li, N., and Duan, L. (2018). Intensive Anthropogenic Activities Had Affected Daya Bay in South China Sea since the 1980s: Evidence from Heavy Metal Contaminations. Mar. Pollut. Bull. 135, 318–331. doi:10.1016/j.marpolbul.2018.07.011
Queirós, A. M., Birchenough, S. N. R., Bremner, J., Godbold, J. A., Parker, R. E., Romero-Ramirez, A., et al. (2013). A Bioturbation Classification of European marine Infaunal Invertebrates. Ecol. Evol. 3, 3958–3985. doi:10.1002/ece3.769
R Core Team (2019). R: A Language and Environment for Statistical Computing. Vienna, Austria: R Foundation for Statistical Computing. Available at: https://www.R-project.org/.
Reiss, H., and Kröncke, I. (2005). Seasonal Variability of Infaunal Community Structures in Three Areas of the North Sea under Different Environmental Conditions. Estuarine, Coastal Shelf Sci. 65, 253–274. doi:10.1016/j.ecss.2005.06.008
Ricotta, C., and Moretti, M. (2011). CWM and Rao's Quadratic Diversity: a Unified Framework for Functional Ecology. Oecologia 167, 181–188. doi:10.1007/s00442-011-1965-5
Schmera, D., Heino, J., Podani, J., Erős, T., and Dolédec, S. (2017). Functional Diversity: a Review of Methodology and Current Knowledge in Freshwater Macroinvertebrate Research. Hydrobiologia 787, 27–44. doi:10.1007/s10750-016-2974-5
Shepard, F. P. (1954). Nomenclature Based on Sand-silt-clay Ratios. J. Sediment. Res. 24, 151–158. doi:10.1306/d4269774-2b26-11d7-8648000102c1865d
Shiah, F., Wu, T., Li, K., Kao, S., Tseng, Y., Chung, J., et al. (2006). Thermal Effects on Heterotrophic Processes in a Coastal Ecosystem Adjacent to a Nuclear Power Plant. Mar. Ecol. Prog. Ser. 309, 55–65. doi:10.3354/meps309055
Snelgrove, P. V. R. (1998). The Biodiversity of Macrofaunal Organisms in marine Sediments. Biodiversity and Conservation 7, 1123–1132. doi:10.1023/a:1008867313340
Song, X., Huang, L., Zhang, J., Huang, H., Li, T., and Su, Q. (2009). Harmful Algal Blooms (HABs) in Daya Bay, China: An In Situ Study of Primary Production and Environmental Impacts. Mar. Pollut. Bull. 58, 1310–1318. doi:10.1016/j.marpolbul.2009.04.030
Strong, J. A., Andonegi, E., Bizsel, K. C., Danovaro, R., Elliott, M., Franco, A., et al. (2015). Marine Biodiversity and Ecosystem Function Relationships: The Potential for Practical Monitoring Applications. Estuarine, Coastal Shelf Sci. 161, 46–64. doi:10.1016/j.ecss.2015.04.008
Teixeira, T. P., Neves, L. M., and Araújo, F. G. (2009). Effects of a Nuclear Power Plant thermal Discharge on Habitat Complexity and Fish Community Structure in Ilha Grande Bay, Brazil. Mar. Environ. Res. 68, 188–195. doi:10.1016/j.marenvres.2009.06.004
Thinova, L., and Trojek, T. (2009). Data Analysis from Monitoring of Radionuclides in the Nuclear Power Plant Temelin Ecosystem Area. Appl. Radiat. Isot. 67, 1503–1508. doi:10.1016/j.apradiso.2009.02.051
Tillin, H., Hiddink, J., Jennings, S., and Kaiser, M. (2006). Chronic Bottom Trawling Alters the Functional Composition of Benthic Invertebrate Communities on a Sea-Basin Scale. Mar. Ecol. Prog. Ser. 318, 31–45. doi:10.3354/meps318031
Trannum, H. C., Olsgard, F., Skei, J. M., Indrehus, J., Øverås, S., and Eriksen, J. (2004). Effects of Copper, Cadmium and Contaminated Harbour Sediments on Recolonisation of Soft-Bottom Communities. J. Exp. Mar. Biol. Ecol. 310, 87–114. doi:10.1016/j.jembe.2004.04.003
van der Linden, P., Borja, A., Rodríquez, J. G., Muxika, I., Galparsoro, I., Patrício, J., et al. (2016). Spatial and Temporal Response of Multiple Trait-Based Indices to Natural- and Anthropogenic Seafloor Disturbance (Effluents). Ecol. Indic. 69, 617–628. doi:10.1016/j.ecolind.2016.05.020
Vandewalle, M., De Bello, F., Berg, M. P., Bolger, T., Dolédec, S., Dubs, F., et al. (2010). Functional Traits as Indicators of Biodiversity Response to Land Use Changes across Ecosystems and Organisms. Biodivers Conserv 19, 2921–2947. doi:10.1007/s10531-010-9798-9
Villéger, S., Mason, N. W. H., and Mouillot, D. (2008). New Multidimensional Functional Diversity Indices for a Multifaceted Framework in Functional Ecology. Ecology 89, 2290–2301. doi:10.1890/07-1206.1
Villnäs, A., Perus, J., and Bonsdorff, E. (2011). Structural and Functional Shifts in Zoobenthos Induced by Organic Enrichment - Implications for Community Recovery Potential. J. Sea Res. 65, 8–18. doi:10.1016/j.seares.2010.06.004
Wang, Y.-S., Lou, Z.-P., Sun, C.-C., and Sun, S. (2008). Ecological Environment Changes in Daya Bay, China, from 1982 to 2004. Mar. Pollut. Bull. 56, 1871–1879. doi:10.1016/j.marpolbul.2008.07.017
Wang, Z.-H., Mu, D.-H., Li, Y.-f., Cao, Y., and Zhang, Y.-J. (2011). Recent Eutrophication and Human Disturbance in Daya Bay, the South China Sea: Dinoflagellate Cyst and Geochemical Evidence. Estuar. Coast. Shelf Sci. 92, 403–414. doi:10.1016/j.ecss.2011.01.015
Widdicombe, S., Austen, M., Kendall, M., Olsgard, F., Schaanning, M., Dashfield, S., et al. (2004). Importance of Bioturbators for Biodiversity Maintenance: Indirect Effects of Fishing Disturbance. Mar. Ecol. Prog. Ser. 275, 1–10. doi:10.3354/meps275001
Worthington, T. A., Shaw, P. J., Daffern, J. R., and Langford, T. E. L. (2015). The Effects of a thermal Discharge on the Macroinvertebrate Community of a Large British River: Implications for Climate Change. Hydrobiologia 753, 81–95. doi:10.1007/s10750-015-2197-1
Wu, M.-L., Wang, Y.-S., Sun, C.-C., Wang, H., Dong, J.-D., and Han, S.-H. (2009). Identification of Anthropogenic Effects and Seasonality on Water Quality in Daya Bay, South China Sea. J. Environ. Manage. 90, 3082–3090. doi:10.1016/j.jenvman.2009.04.017
Wu, R., Cai, S., Wang, S., and Zhang, W. (2007). Three-dimensional Numerical Simulation of Tidal Current and Residual Current at Daya Bay. J. Trop. Oceanogr. 26, 18–23.
Xu, S. N., Li, C. H., Xu, J. J., Xiao, Y. Y., Lin, L., and Huang, X. P. (2014). Pollution by Heavy Metals in the Petrochemical Sewage Waters of the Sea Area of Daya Bay and Assessment on Potential Ecological Risks. Huan Jing Ke Xue 35, 2075–2084.
Ye, S., Zhang, L., and Feng, H. (2017). Marine Ecological Risk Assessment Methods for Radiation Accidents. J. Environ. Radioact. 180, 65–76. doi:10.1016/j.jenvrad.2017.09.024
Yu, J., Tang, D., Yao, L., Chen, P., Jia, X., and Li, C. (2010). Long-term Water Temperature Variations in Daya Bay, China Using Satellite and In Situ Observations. Terr. Atmos. Ocean. Sci. 21, 393–399. doi:10.3319/tao.2009.05.26.01(oc)
Keywords: biological trait analysis, macrobenthic community, functional diversity, human activity, Daya Bay
Citation: Rao Y, Cai L, Chen X, Zhou X, Fu S and Huang H (2021) Responses of Functional Traits of Macrobenthic Communities to Human Activities in Daya Bay (A Subtropical Semi-Enclosed Bay), China. Front. Environ. Sci. 9:766580. doi: 10.3389/fenvs.2021.766580
Received: 29 August 2021; Accepted: 14 October 2021;
Published: 12 November 2021.
Edited by:
Xiaoping Huang, South China Sea Institute of Oceanology (CAS), ChinaReviewed by:
Pablo Muniz, Universidad de la República, UruguayXinzheng Li, Institute of Oceanology (CAS), China
Copyright © 2021 Rao, Cai, Chen, Zhou, Fu and Huang. This is an open-access article distributed under the terms of the Creative Commons Attribution License (CC BY). The use, distribution or reproduction in other forums is permitted, provided the original author(s) and the copyright owner(s) are credited and that the original publication in this journal is cited, in accordance with accepted academic practice. No use, distribution or reproduction is permitted which does not comply with these terms.
*Correspondence: Lizhe Cai, cailizhe@xmu.edu.cn