- 1Department for Biosystems Science and Engineering, ETH Zürich, Basel, Switzerland
- 2aiNET GmbH, ETH Zürich, Basel, Switzerland
- 3Department of Biomedicine, University Hospital Basel, Basel, Switzerland
- 4Department of Internal Medicine, Clinical Immunology, University Hospital Basel, Basel, Switzerland
- 5Department of Immunology, University of Oslo, Oslo, Norway
The adaptive immune system recognizes antigens via an immense array of antigen-binding antibodies and T-cell receptors, the immune repertoire. The interrogation of immune repertoires is of high relevance for understanding the adaptive immune response in disease and infection (e.g., autoimmunity, cancer, HIV). Adaptive immune receptor repertoire sequencing (AIRR-seq) has driven the quantitative and molecular-level profiling of immune repertoires, thereby revealing the high-dimensional complexity of the immune receptor sequence landscape. Several methods for the computational and statistical analysis of large-scale AIRR-seq data have been developed to resolve immune repertoire complexity and to understand the dynamics of adaptive immunity. Here, we review the current research on (i) diversity, (ii) clustering and network, (iii) phylogenetic, and (iv) machine learning methods applied to dissect, quantify, and compare the architecture, evolution, and specificity of immune repertoires. We summarize outstanding questions in computational immunology and propose future directions for systems immunology toward coupling AIRR-seq with the computational discovery of immunotherapeutics, vaccines, and immunodiagnostics.
Introduction
The adaptive immune system is responsible for the specific recognition and elimination of antigens originating from infection and disease. Molecular recognition of antigens is achieved through the vast diversity of antibody (B-cell receptor) and T-cell receptors (TCRs). The genetic diversity of these adaptive immune receptors is generated through a somatic recombination process that acts on their constituent V, D, and J segments (1, 2). During the gene rearrangement process, additional sequence diversity is created by nucleotide deletion and addition, resulting in a potential diversity of >1013 unique B- and T-cell immune receptor sequences (3–6). The adaptive immune repertoire often refers to the collection of all antibody and T-cell immune receptors within an individual and represents both the ongoing and the past immune status of an individual. Current threats, for example of pathogenic nature, are countered by B- and T-cell clonal expansion and selection (7), whereas past ones are archived in immunological memory compartments (8). Immune repertoires are highly dynamic. They are constantly evolving within the repertoire sequence space, which is defined as the set of all biologically achievable immune receptor sequences. Repertoire dynamics and evolution span several orders of magnitude in size (germline gene to clonal diversity), physical components (molecular to cellular dynamics), and time (short-lived responses to immunological memory that can persist for decades) (9–14).
The quantitative resolution of immune repertoires has been fueled by the advent of high-throughput sequencing (2, 15–20). Since 2009, high-throughput adaptive immune receptor repertoire sequencing (AIRR-seq) has provided unprecedented molecular insight into the complexity of adaptive immunity by generating data sets of 100 millions to billions of reads (6, 21, 22). The exponential rise in immune repertoire data has correspondingly led to a large increase in the number of computational methods directed at dissecting repertoire complexity (Figures 1 and 2) (23). Immune repertoire sequencing has catalyzed the field of computational and systems immunology in the same way that genomics and transcriptomics have for systems and computational biology (23). To date, the computational methods that have been developed and applied to immune repertoires relate to (i) the underlying mechanisms of diversity generation, (ii) repertoire architecture, (iii) antibody evolution, and (iv) molecular convergence.
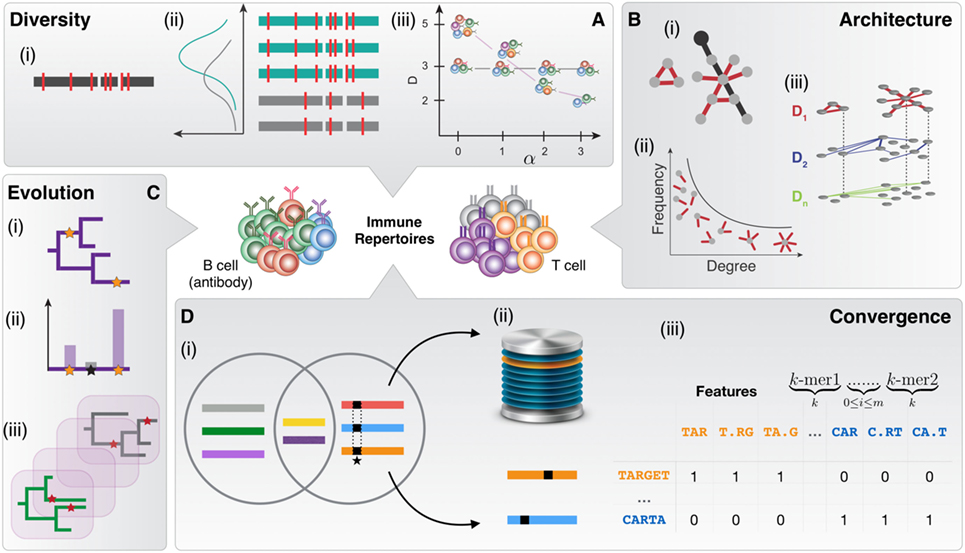
Figure 1. The immune repertoire space is defined by diversity, architecture, evolution, and convergence. (A) Diversity measurements are based on (i) the accurate annotation of V (D) J segments using deterministic and probabilistic approaches with population-level or individualized germline gene reference databases. (ii) Probabilistic and hidden Markov models allow inference of recombination statistics. (iii) Measurement of clonotype diversity using diversity profiles. (B) Analysis of repertoire architecture relies predominantly on (i) clonal networks that are constructed by connecting nucleotide or amino acid sequence nodes by similarity edges. The sequence similarity between clones is defined via a string distance [e.g., Levenshtein distance (LD)], resulting in undirected Boolean networks for a given threshold (nucleotides/amino acids). An example of the global characterization of the network is the diameter, shown by black edges. An example of the local parameters of the network is the degree (n = 1) related to the individual clonal node in black. (ii) Degree distribution is a global characteristic of immune repertoire networks, which can be used for analyzing clonal expansion. (iii) Several similarity layers decompose the immune repertoire along its similarity layers. Layer D1 captures clonal nodes similar by edit distance 1 (1 nt/a.a. different), D2 of distance 2 and so forth. (C) Assessing evolution of antibody lineages. (i) Reconstruction of phylogenetic trees. Stars indicate somatic hypermutation. (ii) Probabilistic methods for the inference of mutation statistics in antibody lineage evolution. (iii) Simulation of antibody repertoire evolution for benchmarking antibody-tailored phylogenetic inference algorithms. (D) Naive and antigen-driven cross-individual sequence similarity and convergence in immune repertoires. (i) The Venn diagram shows sequences shared in the two repertoires (circles). Signature-like sequence features are highlighted by black squares. (ii) Database of convergent or antigen-specific immune receptor sequences. (iii) K-mer sequence decomposition and classification of immune receptor sequences.
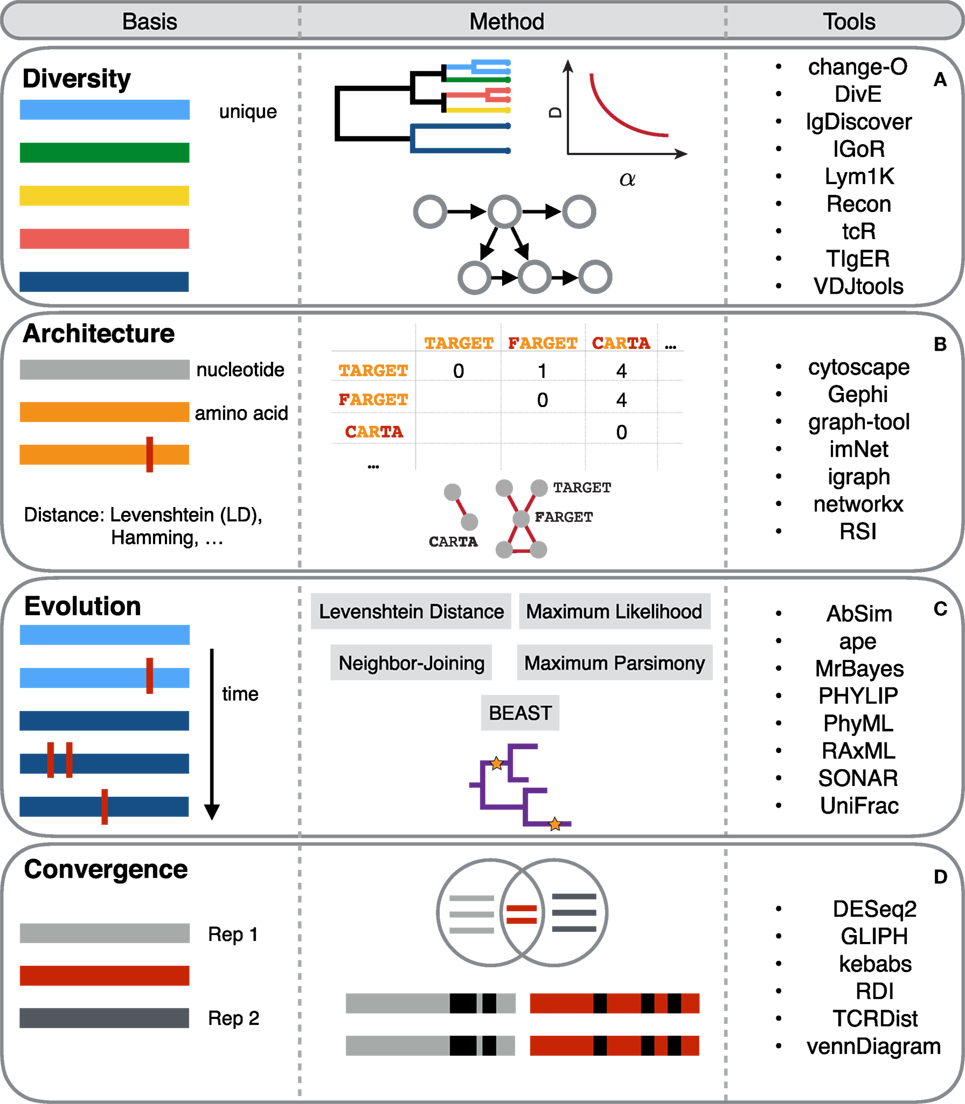
Figure 2. An overview of selected computational tools used in immune repertoire analyses. Each horizontal colored bar colored bar in the Basis column represents a unique antibody or T-cell receptor (TCR) sequence. Vertical red bars represent sequence differences or somatic hypermutation. The Method column describes the general concept of the computational methods and how these are applied to immune repertoires. The Tools column highlights exemplary key resources for performing computational analysis in the respective analytical sections [rows (A–D)].
This review provides an overview of the computational methods that are currently being used to dissect the high-dimensional complexity of immune repertoires. We will treat only those methods that are downstream of data preprocessing although currently there is no consensus on standard operating preprocessing procedures, and please refer to recent reviews on these subjects (2, 17, 24). Specifically, this review centers on computational, mathematical, and statistical approaches used to analyze, measure, and predict immune repertoire complexity. The description of these methods will be embedded within the main areas of immune repertoire research. Given that the genetic structure of antibody and TCRs is very similar, the majority of the methods illustrated in this review can be applied both in the context of antibody and T-cell studies. Exceptions to this rule are stated explicitly.
Measuring Immune Repertoire Diversity
The immense diversity is one of the key features of immune repertoires and enables broad antigen recognition breadth (Figures 1A and 2A). The maximum theoretical amino acid diversity of immune repertoires is ≈10140 (calculated as 20110 × 2). The calculation takes into account the 20 unique amino acids, the 110 amino acids long variable region of immune receptors, and the 2 variable regions composing each receptor (IGVL-IGVH or TCRVα-TCRVβ) (25). However, this enormous diversity is restricted in humans and mice by a starting set of V, D, and J gene segments leading to a potential diversity of about 1013–1018 (3–6, 26–30). Only a fraction of the potential diversity is represented at any point in time in any given individual: the number of B- and T-cells is restricted (human: 1011–12) and the number of different clones, depending on clone definitions, reaches about 109 in humans and 106–7 in mice (3, 5, 6, 31). The study of immune repertoire diversity ranges from the study of (i) the diversity of the building blocks of immune repertoires (V, D, and J segments) and antibody lineage reconstruction (ii) to the mathematical modeling of VDJ recombination and (iii) to the estimation of the theoretical and biologically available repertoire frequency diversity (32). Together, these subfields of repertoire diversity analysis have expanded our analytical and quantitative insight into the creation of naive and antigen-driven antigen receptor diversity.
Accurate quantification of repertoire diversity relies first and foremost on the correct annotation of sequencing reads. Read annotation encompasses multiple steps: (i) calling of V, D, and J segments, (ii) subdivision into framework (FR) and complementarity-determining regions (CDRs), (iii) identification of inserted and deleted nucleotides in the junction region, and (iv) the quantification of the extent of somatic hypermutation (for antibodies). VDJ annotation tools were recently reviewed by Greiff et al. and Yaari and Kleinstein (17, 24). An updated version is currently maintained on the B-T.CR forum.1 The B-T.CR forum is an AIRR-seq community platform for community-edited Wiki pages related to data sets and analysis tools as well as scientific exchange on current relevant topics in AIRR-seq (33, 34).
Accurate antigen receptor germline gene genotyping is crucial for predicting adaptive immunity (personalized and precision medicine) in the genetically diverse human population (30, 35–38). All VDJ annotation tools rely, at least partly, on a reference database of germline gene alleles. A reference database that is not identical to that of the individual from which the sequencing data is being annotated bears the potential of inaccurate annotation. This could affect, for example, the accuracy of the calling of V, D, and J genes and alleles as well as the quantification of somatic hypermutation. Antibody gene allele variation has also been linked to differential effectiveness of the humoral immune response (30, 35). Indeed, an increasing number of human germline gene alleles—representing one or several single-nucleotide polymorphisms—has been recently detected (30, 37, 39–41). These discoveries call into question the widely adopted practice of using one central germline reference database containing a more or less static set of non-personalized germline gene alleles. To address this problem, Corcoran et al. developed a software package (IgDiscover), which employs a cluster identification approach to reconstruct de novo from an AIRR-seq data set the corresponding V-gene germline database—all without a priori knowledge of existing germline gene databases (36). By doing so, they detected extensive individual germline gene differences among rhesus macaques (36). Complementarily, Gadala-Maria et al. developed TiGER (Tool for Ig Genotype Elucidation via Rep-Seq), which detects novel alleles based on the mutation pattern analysis (37). In contrast to IgDiscover, TiGER uses initial VDJ allele assignments with existing databases and software. Extending the analysis of germline gene diversity to the population level, Yu et al. built Lym1K, which is a database that combines validated alleles with novel alleles found in the 1000 Genomes Project (42, 43). In addition to database-centered approaches, probabilistic annotation enabled the detection of novel IgV genes and led to the discovery that substitution and mutation processes are (although reproducible across individuals) segment and allele dependent, thus further refining VDJ annotation and downstream diversity measurement (4, 44–47).
As a direct application in fundamental immunology, the advent of AIRR-seq has enabled the opportunity to describe quantitatively the statistical properties of VDJ recombination. Indeed, the ability to generate large data sets allowed several studies to show evidence of biases in VDJ recombination, as some germline gene frequencies (and combinations thereof) were found to occur more often than others (6, 21, 48–50). To mathematically model the process of VDJ recombination in both B- and T-cells, Elhanati et al. and Murugan et al. have employed techniques borrowed from statistical physics (maximum entropy, Hidden Markov, and probabilistic models) (4, 5, 45) to uncover the amount of diversity information inherent to each part of antibody and TCR sequences (entropy decomposition). VDJ recombination probability inference was mostly performed on non-productive sequences (e.g., out-of-frame, containing stop codon) as these receptors were assumed to be exempt from selection, thus representing unselected products of the generation process (4).
The deep sequence coverage of AIRR-seq has also led to the discovery of public clones or clonotypes—sequences that are shared across two or more individuals (6, 51–54). The existence of naive and antigen-associated public clones signifies a predetermined reduction in a priori genetic and antigen-driven immune receptor diversity (6). Although the exact definition of what constitutes a “public clone” is debatable (55), advancements have been made in understanding the generation and structure of public B- and T-cell clonotypes. By quantifying VDJ recombination probabilities as described above, Elhanati et al. have suggested that the emergence of public clonotypes is a direct consequence of the underlying VDJ recombination bias (56). The inference of VDJ recombination statistics of naive B- and T-cell populations may be of use in vaccination studies for helping distinguish public antigen-specific clonotypes from genetically (naive) predetermined ones. If feasible, such an approach might render the need of a healthy control cohort for determining naive public clones superfluous (47, 57). Complementarily, Greiff et al. have demonstrated extensive VDJ recombination bias by support vector machine analysis. Specifically, it showed that both public and private clones possess predetermined sequence signatures independent of mouse strain, species, and immune receptor type (antibody, TCR). These sequence signatures were found in both naive and antigen-selected B-cell compartments, which might suggest that naive recombination bias exerts a stronger diversity-constricting effect than antigen-driven evolution (58).
While the above-described methods of immune repertoire diversity analysis are relatively new, the quantification and comparison of clonotype diversity have been already studied in the era preceding high-throughput sequencing platforms by borrowing and adapting from mathematical ecology (59–62). The first step to quantifying clonal repertoire diversity is the definition of clonotype. Definitions of clonotype used in the literature range from the exact amino acid CDR3 to clusters of (e.g., CDR3) sequences to the sequence of entire variable chain regions (IGVL-IGVH or TCRVα-TCRVβ) using methods ranging from likelihood-based lineage inference to distance-based measures. A complete list of clonotyping tools has been compiled on the B-T.CR forum.2 The debate on what constitutes a clonotype is ongoing and beyond the scope of this review. The interested reader is kindly referred to two extensive reviews (17, 63) and a recent report by Nouri and Kleinstein, who have developed a flexible user-defined method for clonotype identification (64).
To measure clonotype diversity, diversity indices are used [detailed reviews on diversity indices have been recently published in Ref. (17, 24)]. Briefly, diversity indices enable the comparison of repertoire diversity by parameterizing the repertoire space. They thus overcome the problem of clonally distinct repertoires (65). Several dedicated software packages exist for diversity index calculations (66–69). Briefly, the Diversity (αD) of a repertoire of S clones is usually calculated as follows: (Hill-Diversity), where fi is the frequency of the ith clone weighted by the parameter α. Special cases of this Diversity function correspond to popular diversity indices in the immune repertoire field: species richness (α = 0), and the exponential Shannon-Weiner (α→1), inverse Simpson (α = 2), and Berger–Parker indices (α→∞). The higher the value of alpha, the higher is the influence of the more abundant clones on αD. Thus, each αD value captures a different region (clonal subset) of the clonal frequency distribution (65). Due to the mathematical properties of the Diversity function [Schur concavity (70)], different repertoires may yield qualitatively different αD values depending on the Diversity index used [Figure 1 in Greiff et al. (65)]. Therefore, for any discriminatory diversity comparisons, at least two Diversity indices should be considered. Diversity profiles, which are collections (vectors) of several Diversity indices, have been suggested to be superior to single diversity indices, when comparing clonal diversity (65, 66, 71). Using hierarchical clustering, α-parametrized diversity profiles have been shown to faithfully capture the shape of a repertoire’s underlying clonal frequency distribution, which represents the state of clonal expansion (65). Thus, diversity profiles can serve as a parameterized proxy for a repertoire’s state of clonal expansion. In addition, Mora and Walczak showed that the Rényi entropy (the mathematical foundation of Hill-Diversity profiles) can be constructed, in some cases, from rank-frequency plots (72), thereby establishing a direct mathematical link between clonal frequency distribution and diversity indices. Another interesting novel diversity analysis method is the clonal plane and the polyclonal monoclonal diversity index developed by Afzal et al. (73). These two related mathematical concepts represent repertoire diversity in a coordinate system spanned by species richness and evenness. This allows a visually straightforward identification of polyclonal and oligoclonal samples.
Although clonal frequency distributions, in most cases, cannot be compared directly across individuals due to restricted clonal overlap, their mathematical description has been the object of several studies. Specifically, clonal frequency distributions were found to be power-law distributed, with a few abundant clones, and a large number of lowly abundant clones (65, 74–76). Furthermore, Schwab et al. showed analytically via numerical simulations that Zipf-like distributions, a subclass of power-law distributions arise naturally if fluctuating unobserved variables affect the system (e.g., a variable external antigen environment influencing the observed antibody repertoire) (77). Indeed, it could be shown that clonotype diversity (or state of clonal expansion) contains antigen-associated information on the host immune status (6, 65, 78).
Given the heavy-tailed distribution of clonal frequencies (large number of low-abundant clones), comprehensive sampling of repertoires is challenging to achieve, thereby hindering cross-sample diversity comparison (65, 74, 77, 79). Indeed, diversity indices are highly sensitive to sample size variation caused by varying PCR and sequencing accuracy and biological and technological sampling depth (60, 62, 80). In general, two main approaches are used to mitigate sampling effects. (i) For the comparison of any two repertoires of unequal sampling size, Venturi et al. devised the following strategy: (a) sequencing reads are drawn randomly n-times without replacement from the repertoire with higher sampling depth (higher cell number and/or higher sequencing depth). (b) The desired diversity measure for each bootstrapped immune repertoire data set is then computed. (c) From the distribution of n diversity measures, the median diversity measure is estimated and compared with the smaller data set. This approach, however, does not aim to estimate the true underlying diversity of a cellular compartment (e.g., B- and T-cell developmental stages, antigen-specific repertoire). (ii) Inferring the true diversity of a repertoire is equivalent to the “missing-species problem,” which describes the challenge to estimate the number of clones (“species”) that have been missed in the sampling step. The quantification of missing (or unseen) species may be performed using diversity index estimators (60, 81, 82). These estimators attempt to estimate the number of missing receptors based on a more or less narrow region of the clonal frequency distribution’s tail. A dedicated diversity estimator, adapted to the microevolutionary and high-diversity case of immune repertoires, was published by Laydon et al. They developed a rarefaction-based method called DivE, for estimating total repertoire size (species richness) (82, 83), which they showed to be both superior to common estimators of species richness such as Chao1 (81, 83) and Good-Turing (60, 84) and capable of estimating a repertoire’s underlying clonal frequency distribution. Complementarily, Kaplinsky and Arnaout developed a maximum likelihood (ML) clone-size distribution-independent algorithm called Recon (reconstruction of estimated clones from observed numbers) that does estimate not only species richness but also any Hill-diversity measure (80). In general, however, gold-standard procedures for estimating repertoire diversity in various sampling scenarios are non-existent. A meta-study benchmarking current diversity index estimators on simulated immune repertoires will be needed to establish reliable guidelines for diversity estimation.
To compare differences between diversity profiles, one should also consider resampling strategies as implemented in the R package Change-O by Gupta et al. These allow the determination of confidence areas around each diversity profile (66, 85) in the presence of differently sized repertoires. Accurate diversity calculation in case of incomplete sampling is of special importance when gaining information on human repertoires, which are often restricted to the isolation of a limited number of B- and T-cells from peripheral blood (17, 83, 86, 87).
Although the quantification of diversity is one of the more mature subfields of computational repertoire immunology, numerous open questions remain: (i) diversity has been measured from many different perspectives (germline gene diversity, state of clonal expansion, clonal size), thus capturing different dimensions of the repertoire diversity space. Is it possible to devise a universal metric that synthesizing different aspects of immune repertoire diversity into one? Such a metric would be very useful for repertoire-based immunodiagnostics. (ii) Hidden Markov and Bayesian (probabilistic) approaches have been used for modeling VDJ recombination. Those approaches, however, capture only short-range sequence interactions. Therefore, recurrent neural network approaches might be more appropriate to model the immune repertoire sequence space given their ability to account for sequence interactions of arbitrary length (88, 89)? (iii) Finally, we still have only very superficial insight into the biological diversity of antigen-specific repertoires and the combination rules of IGVL/IGVH and TCRVα1/TCRVβ chains due to the lack of large-scale data (76, 90–93). Once more extensive data have become available, can we leverage machine learning to uncover the underlying structure of antigen-specific repertoires and the prediction rules of chain pairing? Uncovering these immunological prediction rules is crucial for the knowledge-based development of antibody and T-cell-based immunotherapeutics.
Resolving the Sequence Similarity Architecture of Immune Repertoires
The entirety of similarity relations among immune receptor sequences is called the similarity architecture of an immune repertoire. Thus, unlike immune repertoire diversity, which is based on the frequency profiles of immune clones, sequence similarity architecture captures frequency-independent clonal sequence similarity relations. The similarity among immune receptors directly influences antigen recognition breadth: the more dissimilar receptors are, the larger is the antigen space covered. Given the genetic, cellular, and clonal restrictions of immune repertoire diversity, the similarity architecture of antibody and T-cell repertoires has been a longstanding question and has only recently begun to be resolved. Understanding the sequence architecture of immune repertoires is, for example, crucial in the context of antibody therapeutics discovery for the conception of naive antibody libraries and synthetic repertoires that recapitulate natural repertoires (94).
One powerful approach to interrogate and measure immune repertoire architecture is network analysis (Figures 1B and 2B) (94–100). Networks allow interrogation of sequence similarity and thereby add a complementary layer of information to repertoire diversity analysis. Clonal networks are built by defining each clone (nucleotide or amino acid sequence) as a node (Figure 1B). An edge between clones is drawn if they satisfy a certain similarity condition, which is predefined via a string distance [e.g., Levenshtein distance (LD)], resulting in undirected Boolean networks (94–97, 99, 100). The default distance is usually 1 nucleotide or 1 amino acid difference, but larger distances have also been explored (94). Thus, the construction of clonal networks requires the calculation of an all-by-all distance matrix. While the complete distance matrix can be computed on a single machine with repertoires of clone sizes <10,000, it becomes computationally expensive in terms of time and memory to calculate networks of clone sizes that exceed 105 clones, which is the size of many repertoires in both mice and humans (3, 5, 6). Therefore, Miho et al. have developed a high-performance computing pipeline (imNet), which can compute distance matrices and construct corresponding large-scale repertoire networks (94). This method led to the biological insight that antibody repertoire networks are, in contrast to other systems (101, 102), resistant to subsampling, which is of great importance for the network analysis of human repertoires where limited access to B-cell populations and lymphoid organs restricts complete biological sampling (17, 86). Although networks of a few thousand nodes may be visualized using software suites such as igraph (103), networkx (104), gephi (105), and cytoscape (106), interpretation of the visual graphics is not informative for networks beyond the clonal size of 103 (94). Furthermore, visualization of networks provides only marginal quantitation of the network similarity architecture, thus limiting the quantitative understanding of immune repertoires. Graph properties and network analysis have been recently employed to quantify the network architecture of immune repertoires (94, 100). Architecture analytics may be subdivided into properties that capture the repertoire at the global level (generally one coefficient per network) and those that describe the repertoire at the clonal and thus local level (one coefficient per clone per repertoire, vector of size equal to the clone size) (94).
Global coefficients are, for example, degree distribution, clustering coefficient, diameter, and assortativity (94). The degree of a node is the number of its edges (i.e., the number of similar clones to a certain clone), and a repertoire’s degree distribution quantifies the abundance of node degrees (i.e., clonal similarities) across clones of a repertoire. This degree distribution has been used to describe and classify the networks by type, such as power law (a few highly connected clones and many clones with few connections), which is reminiscent of antigen-driven clonal expansion, or exponential (more even degree distribution across clones, covering extensive sequence space), which is more reflective of naive repertoires (94). The degree distribution thus provides insights into the overall distribution of connectedness (clonal similarities) within a repertoire and its state of clonal sequence expansion. Local characterization allows for the interrogation and correlation of additional clonal-related features, such as frequency and antigen specificity, within the immune repertoire architecture. Local parameters are, for example, degree, authority, closeness, betweenness, and PageRank (94). PageRank, for instance, measures the importance of the similarity between two CDR3 clones within the network. Detailed mathematical descriptions of available network parameters have been described elsewhere (94, 107, 108).
Complementary to networks, which provide a discrete characterization of repertoires, similarity indices, similarity indices have been devised that provide a continuous description of repertoire architecture by quantifying the similarity between all sequences of a repertoire (using distance metrics) on a scale ranging from 0 (zero similarity) to 100% (all sequences are 100% identical) (6, 109). In addition to sequence similarity, the index by Strauli and Hernandez takes the frequency of each sequence into account, thus normalizing sequencing similarity by the frequency of each of the pairwise compared sequences (109).
The assessment of repertoire architecture has only recently started to transition from the visual investigation of clusters of immune receptor sequences to the construction of large-scale networks and the truly quantitative analysis of entire repertoires across similarity layers (>1 amino acid/nucleotide differences). This advance enabled the discovery of fundamental properties of repertoire architecture such as reproducibility, robustness, and redundancy (94). Although the biological interpretation of the mathematical characterization of immune repertoire networks is at an early stage, the universal use of network analysis in the deconvolution of complex systems (107, 108) suggests a great potential in immune repertoire research. Many important questions remain: (i) How can network repertoire architecture be compared across individuals without condensing networks into network indices and potentially losing information? Thus, can discrete and continuous representation of repertoire architecture be merged into one comprehensive mathematical framework? (ii) Can the linking of networks across similarity layers serve to understand the dynamic and potential space of antigen-driven repertoire evolution (94)? (iii) Is the network structure that is observed on the antibody immunogenomic level also maintained on the phenotypic and immunoproteomics level of serum antibodies (110–116)?
Retracing the Antigen-Driven Evolution of Antibody Repertoires
Upon antigen challenge, B-cells expand and hypermutate their antibody variable regions, thus forming a B-cell lineage that extends from the naive unmutated B-cells, to somatically hypermutated memory B-cells (25), to terminally differentiated plasma cells (11). Somatic hypermutation is unique to B-cells and absent in T-cells. Retracing antibody repertoire evolution enables insights into how vaccines (78) and pathogens shape the humoral immune response (117–119).
To infer the ancestral evolutionary relationships among individual B-cells, lineage trees are constructed from the set of sequences belonging to a clonal lineage (Figures 1C and 2C). A clonal lineage is defined as the number of receptor sequences originating from the same recombination event. For building a lineage tree, a common preprocessing step is to group together all sequences with identical V and J genes and CDR3 length. Schramm et al. published a software for the ontogenetic analysis of antibody repertoires, which is designed to enable the automation of antibody repertoire lineage analysis. Importantly, it provides interfaces to phylogenetic inference programs such as BEAST and DNAML (120).
In antibody repertoire phylogenetics, there is no consensus as to which phylogenetic method is optimal for the inference of lineage evolution (17, 121). Most of the current phylogenetic methods rely on assumptions that may be true for species evolution but might be invalid for antibody evolution. One prominent example is the assumption that each site mutates independently of the neighboring nucleotides, which is not the case in antibody evolution (121). In addition, antibodies evolve on time scales that differ by several orders of magnitudes from those of species. These two factors likely decrease the accuracy of clade prediction (clade: set of descendent sequences that all share a common ancestor), thus potentially impacting antibody phylogenetic studies.
Several phylogenetic methods, such as LD, neighbor joining (NJ), maximum parsimony (MP), ML, and Bayesian inference (BEAST), have been used for delineating the evolution of B-cell clonal lineages from antibody repertoire sequencing data (85, 122–124). For general information regarding the methods, refer to the review by Yang and Rannala (125). Briefly, both LD and NJ are distance-based methods that rely upon an initial all-by-all distance matrix calculation and have been implemented in many computational platforms (Clustal, T-REX) and R packages (ape, phangorn) (126–129). Even in the event >105 sequences per sample, the distance matrix calculation in phylogenetics poses less of a problem than in network analysis since a sample’s sequences are grouped by lineage members of identical V–J gene and CDR3 length, thus reducing computational complexity. The relatively short computation time of distance-based methods renders them particularly useful for initial data exploration (125). MP attempts to explain the molecular evolution by non-parametrically selecting the shortest possible tree that explains the data (24). MP trees can be produced using several available tools (e.g., PAUP, TNT, PHYLIP, Rphylip) (130–133). Both ML and BEAST infer lineage evolution using probabilistic methods, which can incorporate biologically relevant parameters such as transition/transversion rate and nucleotide frequencies. A variety of ML tools have been developed (e.g., PhyML, RAxML, and MEGA) (134–136). While multiple phylogenetic tools utilizing Bayesian methods exist (137, 138), this review focuses on BEAST given its recurrent use in antibody repertoire studies (120, 124, 139–141). BEAST traditionally employs a Markov chain Monte Carlo algorithm to explore the tree parameter space. This computationally expensive process limits the practical number of sequences per lineage tree to <103. Despite the extensive computational requirements (both in memory and in run time), BEAST has the advantage of producing time-resolved phylogenies and inferring somatic hypermutation rates (138, 139). The BEAST framework shows, therefore, the highest scientific benefit when applied to experiments examining antibody evolution within the same host across multiple sampling time points (124), as inferred mutation rates and tree heights (duration of evolution) are reported in calendar time.
Yermanos et al. have compared five of the most common phylogenetics reconstruction methods for antibody repertoire analysis in terms of their absolute accuracy and their concordance in clade assignment using both experimental and simulated antibody sequence data (139). Correctly inferring the clades of a phylogenetic tree is crucial for describing the evolutionary relationship between clonally selected and expanded B-cells (i.e., memory B-cells) that belong to a given lineage (i.e., derived from a naive B-cell). Phylogenetic trees inferred by the methods tested (LD, NJ, ML, MP, BEAST) resulted in different topologies as measured by both clade overlap (number of internal nodes sharing the same descendant sequences) and treescape metric (comparison of the placement of the most recent common ancestor of each pair of tips in two trees) (142). These results suggest caution in the interpretation and comparison of results from the phylogenetic reconstruction of antibody repertoire evolution (139).
The accurate reconstruction of antibody phylogenetic trees is tightly linked to the detailed understanding of the physical and temporal dynamics of somatic hypermutation along antigen-driven antibody sequence evolution. Mutation statistics can be inferred probabilistically to account for the fact that the likelihood of mutation is not uniformly distributed over the antibody VDJ region (46, 47). For example, there is a preference to mutate particular DNA motifs called hotspots (length: 2–7 bp) and concentrated in the CDRs over others (coldspots) (4, 121, 143, 144). To uncover the sequence-based rules of somatic hypermutation targeting, Yaari et al. developed S5F, an antibody-specific mutation model. This model provides an estimation of the mutability and mutation preference for each nucleotide in the VDJ region of the heavy chain based on the four surrounding nucleotides (two on either side). The estimated profiles could explain almost half of the variance in observed mutation patterns and were highly conserved across individuals (121). Cui et al. have, in addition, reported two new models that add to the heavy-chain S5F model: the light-chain mouse RS5NF and the light-chain human S5F L chain model (145). In addition, Sheng et al. investigated the intrinsic mutation frequency and substitution bias of somatic hypermutations at the amino acid level by developing a method for generating gene-specific substitution profiles (146). This method revealed gene-specific substitution profiles that are unique to each human V-gene and also highly consistent between human individuals.
The existence of hotspot and coldspot mutation motifs violates the standard assumption of likelihood-based phylogenetics, which is that evolutionary changes at different nucleotide or codon sites are statistically independent. Furthermore, since hotspot motifs are, by definition, more mutable than non-hotspot motifs, their frequency within the B-cell lineage may decrease over time as they are replaced with more stable motifs (147). To explicitly parameterize the effect of biased mutation within a phylogenetic substitution model, Hoehn et al. developed a model that can partially account for the effect of context-dependent mutability of hotspot and coldspot motifs and explicitly model descent from a known germline sequence (148). The resulting model showed a substantially better fit to three well-characterized lineages of HIV-neutralizing antibodies, thus being potentially useful for analyzing the temporal dynamics of antibody mutability in the context of chronic infection. In addition, Vieira et al. assessed the evidence for consistent changes in mutability during the evolution of B-cell lineages (140). By using Bayesian phylogenetic modeling, they showed that mutability losses were about 60% more frequent than gains (in both CDRs and FRs) in anti-HIV antibody sequences (140).
Although computational methods tailored to the phylogenetic analysis of antibody evolution are slowly beginning to surface, many important problems remain. (i) First approaches in coupling clonal expansion information to the inference of phylogenetic trees have been developed (149). Will these additional layers of information enable a better prediction of antibody evolution? (ii) There has been progress in comparing the differences of antibody repertoires in the context of phylogenetic trees using the UniFrac distance measure (150, 151). Briefly, for a given pair of samples, UniFrac measures the total branch length that is unique to each sample. The comparison of tree topologies, however, remains a challenge. This is because each lineage tree is composed of a different number of sequences, and there are thousands, if not more, of simultaneously evolving lineages within a single host. Although methods exists for the comparison of unlabeled phylogenetic trees by, for instance, means of their Laplacian spectra (152), their application and ability to extract meaningful biological conclusions have not yet been realized. (iii) It is unclear to what extent antibody evolution differs between different acute and chronic viral infections, or different antigens. Specifically, is it possible to relate antigen-driven convergence and affinity (6, 50, 117) to phylogenetic antigen-specific signatures (153)?
Dissecting Naive and Antigen-Driven Repertoire Convergence
Convergence (overlap) of immune repertoires describes the phenomenon of identical or similar immune receptor sequences shared by two or more individuals. Specifically, sequence convergence can either mean that (i) clones (public clonotypes, entire clonal sequence or clonotype cluster) or (ii) motifs (sequence substrings) are shared. Several researchers in the field have endeavored to quantify the extent of naive and antigen-driven repertoire convergence using a large variety of computational approaches that quantify cross-individual sequence similarity (6, 53, 78, 117, 154–156) (Figures 1D and 2D). Repertoire convergence may be of substantial importance for the prediction and manipulation of adaptive immunity (6).
The simplest way to quantify sequence convergence is by clonotype overlap among pairwise samples expressed as a percentage normalized by the clonal size of either one or both of the samples compared (6, 48, 157). In case clonotypes are treated not as single sequences but clusters of sequences, clusters were defined as shared between samples if each sample contributed at least one sequence to the cluster (156). Overlap indices such as Morisita-Horn (158) add additional information to the measurement of clonal overlap by integrating the clonal frequency of compared clones (62, 159, 160). A parameterized version of the Morisita-Horn index, similar to the Hill-diversity, may be used to weigh certain clonal abundance ranges differently (60). Rubelt and Bolen expanded on the idea of an overlap index by incorporating both binned sequence features (e.g., clone sequences, germline genes) and their frequency for measuring the impact of heritable factors on VDJ recombination and thymic selection. Their Repertoire Dissimilarity Index consists of a non-parametric Euclidian-distance-based bootstrapped subsampling approach, which enables the quantification of the average variation between repertoires (50, 161). Importantly, it accounts for variance in sequencing depth between samples. Another clone-based approach was developed by Emerson et al. who mined public TCRβ clonotypes in CMV-positive and CVM-negative individuals to predict their CMV status. To this end, they identified CMV-associated clonotypes by using Fisher’s exact test. Subsequently, these clonotypes were used within the context of a probabilistic classifier to predict an individual’s CMV status. The classifier used dimensionality reduction and feature selection to mitigate the influence of the variance of HLA types across individuals because the distribution of TCRβ clones is HLA dependent (154).
Moving from the clonal to the subsequence level, several groups compared the average distance between repertoires based on their entire sequence diversity (without predetermining feature bins). Specifically, Yokota et al. developed an algorithm for comparing the similarity of immune repertoires by projecting the high-dimensional intersequence relations, calculated from pairwise sequence alignments, onto a low-dimensional space (162). Such low-dimensional embedding of sequence similarity has the advantage of enabling the identification of those sequences that contribute most to intersample (dis)similarity. As previously described, Strauli and Hernandez quantified sequence convergence between repertoires in response to influenza vaccination not only by incorporating genetic distance (Needleman-Wunsch algorithm) but also by incorporating the frequency of each clonal sequence (109). Their approach relies on a statistical framework called functional data analysis (FDA), which is often used for gene expression analysis. In their implementation, FDA models each sample as a continuous function over sampling time points and is thus suitable for the analysis of sequence convergence over a time course experiment. The FDA framework has the advantage of accounting for uneven time point sampling and measurement error, both of which are common characteristics of immune repertoire data sets (2, 17). Bürckert et al. also employed a method borrowed from gene expression analysis (DESeq2) (163) to select for clusters of CDR3s, which are significantly overrepresented within different cohorts of immunized animals (164). These clusters exhibited convergent antigen-induced CDR3 signatures with stereotypic amino acid patterns seen in previously described tetanus toxoid and measles-specific CDR3 sequences.
Given the high-dimensional complexity of the immune repertoire sequence space, sequence distance-based approaches might not suffice for covering the entire complexity of sequence convergence. A greater portion of the sequence space may be covered by sequence-based machine learning (artificial intelligence). Here, the idea is that sequence signatures and motifs are shared between individuals belonging to a predefined class (e.g., different immune status). Sun et al. discriminated the TCRβ repertoire of mice immunized with and without ovalbumin with 80% accuracy by deconstructing it into overlapping amino acid k-mers (165). Sun Cinelli et al. used a one-dimensional Bayesian classifier for the selection of features, which were subsequently used for support vector machine analysis (166). As a third machine learning alternative, Greiff et al. leveraged gapped-k-mers and support vector machines for the classification of public and private clones with 80% accuracy from antibody and TCR repertoires of human and mice. This study used overlapping k-mers to construct sequence prediction profiles, which highlight those convergent sequence regions that contribute most to the identity of a class (public/private clones but also, e.g., also different immune states and antigen specificities) (58). Beyond k-mers, several groups have exploited the addition of additional information such as physicochemical properties (Atchley and Kidera factors) to provide more extensive information to machine learning algorithms (167–171). Finally, a machine learning independent approach using local search graph theory for the detection of disease-associated k-mers was recently published by Apeltsin et al. (172).
One of the longest standing challenges in immunology is whether it is possible to predict antigen specificity from the sequence of the immune receptor (2, 15, 173–175). Sequence-dependent prediction implies that immune receptor sequences specific to one antigen share exclusive sequence signatures (motifs) or have higher intraclass than interclass similarity (class = antigen). Two investigations towards sequenced-based specificity prediction using sequence similarity (sequence distance) approaches have recently been reported (155, 176). In one example, Dash et al. developed a distance measure called TCRDist, which is guided by structural information on pMHC binding (155). Two TCRs sequences were compared by computing a similarity-weighted Hamming distance between CDR sequences, including an additional loop between CDR2 and CDR3. TCRDist was used to detect clusters of highly similar, antigen-specific groups of TCRs that were shared across different mouse or human samples. To predict the antigen specificity of a TCR, it was assigned to the cluster to which it had the highest similarity (as based on the TCRDist), resulting in highly accurate prediction (155). By using a similar approach, Glanville et al. developed GLIPH, a tool that identifies TCR specificity groups using a three-step procedure: (i) determining of shared motifs and global similarity, (ii) clustering based on local and global relationships between TCRs, and (iii) analyzing the enrichment for common V-gene, CDR3 lengths, clonal expansion, shared HLA alleles in recipients, motif significance, and cluster size. This approach yielded also highly accurate prediction of antigen-specific TCRs and led to the design of synthetic TCRs (not existing in biological data) that retained antigen specificity (176).
One of the biggest bottlenecks of learning the underlying principles of antigen-driven repertoire convergence is the scarcity of antigen-specific sequence data. This is not only a problem for machine learning but also a problem for network-based approaches, where one wishes to map antigen-specific information onto generated networks (94, 100). To address this issue for T-cells, Shugay et al. (VDJDB) and Tickotsky et al. (McPAS-TCR) have built dedicated and curated databases. VDJDB gathers >20,000 unique TCR sequences from different species associated with their epitope (>200) and MHC context (177). McPAS-TCR contains more than 5,000 pathogen-associated TCRs from humans and mice (178). For antibodies, Martin has conceived Abysis, which encompasses >5,000 sequences of known function (from literature) from many species (>15) along with, where available, PDB 3D structure information (179). Finally, the Immune Epitope Database has also started capturing epitope-specific antibody and TCR information (>20,000 and >2,000 epitopes) (180).
Significant progress in the understanding of antigen-associated signatures has been made. However, several longstanding questions remain to be answered. (i) The emergence of antigen-driven convergence and phylogenetic evolution are inherently linked. Is it feasible to model both phenomena in a unified computational environment similarly to recent efforts in coupling phylogenetics with the understanding of somatic hypermutation patterns (140, 148)? (ii) Can recently developed models for the inference of VDJ recombination patterns and selection factors be applied to the analysis of antigen-associated sequence signatures (4, 56)? (iii) Do more advanced sequence-based machine learning techniques such as deep neural networks, capable of capturing long-range sequence interactions (out of reach for k-mer-based approaches), improve modeling of the epitope and paratope space (89, 181–186)?
Conclusion
The toolbox of computational immunology for the study of immune repertoires has reached an impressive richness leading to remarkable insights into B- and T-cell development and selection (6, 52, 56, 187, 188), disease, infection, and vaccine profiling (78, 85, 117, 189–192), propelling forward the fields of immunodiagnostics and immunotherapeutics (65, 118, 193). Here, we have discussed computational, mathematical, and statistical methods in the light of underlying assumptions and limitations. Indeed, although considerably matured over the last few years, the field still faces several important and scientifically interesting problems. (i) There exist only few platforms to benchmark computational tools, thus hindering the standardization of methodologies. Recently, a consortium of scientists working in AIRR-seq has convened to establish and implement consensus protocols and simulation frameworks3 (2, 17, 33, 34, 43, 194, 195). (ii) With the exponential increase of both bulk and single-cell data (90, 196), the scalability of computational tools is becoming progressively important. Although advances in this regard have been made in sequence annotation, clonotype clustering, and network construction (64, 94, 197, 198), further efforts especially in the field of phylogenetics are necessary to infer the evolution of large-scale antibody repertoires (139). (iii) Although there exist many approaches, which capture parts of the immune repertoire complexity, a computational approach for the synthesis of many dimensions of the repertoire space at once is missing thus hindering a high-dimensional understanding of the adaptive immune response. (iv) Very few attempts exist yet, which aim to link immune receptor and transcriptomics data (199, 200). Recently, computational tools have been developed that can extract immune receptor sequences from bulk and single-cell transcriptomic data (197, 200–204). Linking immune repertoire and transcriptome may provide a deeper understanding of how antibody and T-cell specificity are regulated on the genetic level with profound implications for synthetic immunology (205–207). (v) Many methods capture a static space of repertoires, but few methods create predictive quantitative knowledge. Increasing the predictive performance of computational methods will help in the antibody discovery from display libraries and immunizations and the design of vaccines and immunodiagnostics (15, 19, 208–210).
Author Conributions
VG and STR conceived and designed the review. All authors wrote the review.
Conflict of Interest Statement
The authors declare that the research was conducted in the absence of any commercial or financial relationships that could be construed as a potential conflict of interest. EM is the founder of aiNET GmbH.
Funding
This work was funded by the Swiss National Science Foundation (Project #: 31003A_170110, to SR), SystemsX.ch – AntibodyX RTD project (to SR); European Research Council Starting Grant (Project #: 679403 to SR). The professorship of STR is made possible by the generous endowment of the S. Leslie Misrock Foundation. We are grateful to ETH Foundation for the Pioneer Fellowship to Enkelejda Miho.
Footnotes
References
1. Tonegawa S. Somatic generation of antibody diversity. Nature (1983) 302:575–81. doi:10.1038/302575a0
2. Wardemann H, Busse CE. Novel approaches to analyze immunoglobulin repertoires. Trends Immunol (2017) 38(7):471–82. doi:10.1016/j.it.2017.05.003
3. Glanville J, Zhai W, Berka J, Telman D, Huerta G, Mehta GR, et al. Precise determination of the diversity of a combinatorial antibody library gives insight into the human immunoglobulin repertoire. Proc Natl Acad Sci U S A (2009) 106:20216–21. doi:10.1073/pnas.0909775106
4. Elhanati Y, Sethna Z, Marcou Q, Callan CG, Mora T, Walczak AM. Inferring processes underlying B-cell repertoire diversity. Phil Trans R Soc Lond B Biol Sci (2015) 370:20140243. doi:10.1098/rstb.2014.0243
5. Murugan A, Mora T, Walczak AM, Callan CG. Statistical inference of the generation probability of T-cell receptors from sequence repertoires. Proc Natl Acad Sci U S A (2012) 109:16161–6. doi:10.1073/pnas.1212755109
6. Greiff V, Menzel U, Miho E, Weber C, Riedel R, Cook S, et al. Systems analysis reveals high genetic and antigen-driven predetermination of antibody repertoires throughout B cell development. Cell Rep (2017) 19:1467–78. doi:10.1016/j.celrep.2017.04.054
8. Ahmed R, Gray D. Immunological memory and protective immunity: understanding their relation. Science (1996) 272:54–60. doi:10.1126/science.272.5258.54
9. Hammarlund E, Lewis MW, Carter SV, Amanna I, Hansen SG, Strelow LI, et al. Multiple diagnostic techniques identify previously vaccinated individuals with protective immunity against monkeypox. Nat Med (2005) 11:1005–11. doi:10.1038/nm1273
10. Amanna IJ, Carlson NE, Slifka MK. Duration of humoral immunity to common viral and vaccine antigens. N Engl J Med (2007) 357:1903–15. doi:10.1056/NEJMoa066092
11. Manz RA, Thiel A, Radbruch A. Lifetime of plasma cells in the bone marrow. Nature (1997) 388:133–4. doi:10.1038/40540
12. Landsverk OJB, Snir O, Casado RB, Richter L, Mold JE, Réu P, et al. Antibody-secreting plasma cells persist for decades in human intestine. J Exp Med (2017) 214(2):309–17. doi:10.1084/jem.20161590
13. Halliley JL, Tipton CM, Liesveld J, Rosenberg AF, Darce J, Gregoretti IV, et al. Long-lived plasma cells are contained within the CD19−CD38hiCD138+ subset in human bone marrow. Immunity (2015) 43(1):132–45. doi:10.1016/j.immuni.2015.06.016
14. Pollok K, Mothes R, Ulbricht C, Liebheit A, Gerken JD, Uhlmann S, et al. The chronically inflamed central nervous system provides niches for long-lived plasma cells. Acta Neuropathol Commun (2017) 5:88. doi:10.1186/s40478-017-0487-8
15. Calis JJA, Rosenberg BR. Characterizing immune repertoires by high throughput sequencing: strategies and applications. Trends Immunol (2014) 35:581–90. doi:10.1016/j.it.2014.09.004
16. Georgiou G, Ippolito GC, Beausang J, Busse CE, Wardemann H, Quake SR. The promise and challenge of high-throughput sequencing of the antibody repertoire. Nat Biotechnol (2014) 32:158–68. doi:10.1038/nbt.2782
17. Greiff V, Miho E, Menzel U, Reddy ST. Bioinformatic and statistical analysis of adaptive immune repertoires. Trends Immunol (2015) 36:738–49. doi:10.1016/j.it.2015.09.006
18. Baum PD, Venturi V, Price DA. Wrestling with the repertoire: the promise and perils of next generation sequencing for antigen receptors. Eur J Immunol (2012) 42:2834–9. doi:10.1002/eji.201242999
19. Robinson WH. Sequencing the functional antibody repertoire—diagnostic and therapeutic discovery. Nat Rev Rheumatol (2014) 11:171–82. doi:10.1038/nrrheum.2014.220
20. Cobey S, Wilson P, Matsen FA. The evolution within us. Philos Trans R Soc Lond B Biol Sci (2015) 370:20140235. doi:10.1098/rstb.2014.0235
21. Weinstein JA, Jiang N, White RA, Fisher DS, Quake SR. High-throughput sequencing of the zebrafish antibody repertoire. Science (2009) 324:807–10. doi:10.1126/science.1170020
22. DeWitt WS, Lindau P, Snyder TM, Sherwood AM, Vignali M, Carlson CS, et al. A public database of memory and naive B-cell receptor sequences. PLoS One (2016) 11:e0160853. doi:10.1371/journal.pone.0160853
23. Kidd BA, Peters LA, Schadt EE, Dudley JT. Unifying immunology with informatics and multiscale biology. Nat Immunol (2014) 15:118–27. doi:10.1038/ni.2787
24. Yaari G, Kleinstein SH. Practical guidelines for B-cell receptor repertoire sequencing analysis. Genome Med (2015) 7:121. doi:10.1186/s13073-015-0243-2
26. Watson CT, Steinberg KM, Huddleston J, Warren RL, Malig M, Schein J, et al. Complete haplotype sequence of the human immunoglobulin heavy-chain variable, diversity, and joining genes and characterization of allelic and copy-number variation. Am J Hum Genet (2013) 92(4):530–46. doi:10.1016/j.ajhg.2013.03.004
27. Johnston CM, Wood AL, Bolland DJ, Corcoran AE. Complete sequence assembly and characterization of the C57BL/6 mouse Ig heavy chain V region. J Immunol (2006) 176:4221–34. doi:10.4049/jimmunol.176.7.4221
28. Malissen M, Minard K, Mjolsness S, Kronenberg M, Goverman J, Hunkapiller T, et al. Mouse T cell antigen receptor: Structure and organization of constant and joining gene segments encoding the β polypeptide. Cell (1984) 37:1101–10. doi:10.1016/0092-8674(84)90444-6
29. Arden B, Clark SP, Kabelitz D, Mak TW. Human T-cell receptor variable gene segment families. Immunogenetics (1995) 42:455–500. doi:10.1007/BF00172176
30. Watson CT, Glanville J, Marasco WA. The individual and population genetics of antibody immunity. Trends Immunol (2017) 38(7):459–70. doi:10.1016/j.it.2017.04.003
31. Trepel F. Number and distribution of lymphocytes in man. A critical analysis. J Mol Med (1974) 52:511–5.
32. Granato A, Chen Y, Wesemann DR. Primary immunoglobulin repertoire development: time and space matter. Curr Opin Immunol (2015) 33:126–31. doi:10.1016/j.coi.2015.02.011
33. Breden F, Prak Luning TE, Peters B, Rubelt F, Schramm CA, Busse CE, et al. Reproducibility and reuse of adaptive immune receptor repertoire data. Front Immunol (2017) 8:1418. doi:10.3389/fimmu.2017.01418
34. Rubelt F, Busse CE, Bukhari SAC, Bürckert J-P, Mariotti-Ferrandiz E, Cowell LG, et al. Adaptive immune receptor repertoire community recommendations for sharing immune-repertoire sequencing data. Nat Immunol (2017) 18(12):1274–8. doi:10.1038/ni.3873
35. Avnir Y, Watson CT, Glanville J, Peterson EC, Tallarico AS, Bennett AS, et al. IGHV1-69 polymorphism modulates anti-influenza antibody repertoires, correlates with IGHV utilization shifts and varies by ethnicity. Sci Rep (2016) 6:20842. doi:10.1038/srep20842
36. Corcoran MM, Phad GE, Bernat NV, Stahl-Hennig C, Sumida N, Persson MAA, et al. Production of individualized V gene databases reveals high levels of immunoglobulin genetic diversity. Nat Commun (2016) 7:13642. doi:10.1038/ncomms13642
37. Gadala-Maria D, Yaari G, Uduman M, Kleinstein SH. Automated analysis of high-throughput B-cell sequencing data reveals a high frequency of novel immunoglobulin V gene segment alleles. Proc Natl Acad Sci U S A (2015) 112:E862–70. doi:10.1073/pnas.1417683112
38. Ralph DK, Matsen FA IV. Per-sample immunoglobulin germline inference from B cell receptor deep sequencing data. Q-Bio (2017). Available from http://arxiv.org/abs/1711.05843
39. Boyd SD, Gaëta BA, Jackson KJ, Fire AZ, Marshall EL, Merker JD, et al. Individual variation in the germline Ig gene repertoire inferred from variable region gene rearrangements. J Immunol (2010) 184:6986–92. doi:10.4049/jimmunol.1000445
40. Kidd MJ, Chen Z, Wang Y, Jackson KJ, Zhang L, Boyd SD, et al. The inference of phased haplotypes for the immunoglobulin H chain V region gene loci by analysis of VDJ gene rearrangements. J Immunol Baltim (2012) 188:1333–40. doi:10.4049/jimmunol.1102097
41. Kirik U, Greiff L, Levander F, Ohlin M. Parallel antibody germline gene and haplotype analyses support the validity of immunoglobulin germline gene inference and discovery. Mol Immunol (2017) 87:12–22. doi:10.1016/j.molimm.2017.03.012
42. Yu Y, Ceredig R, Seoighe C. A Database of human immune receptor alleles recovered from population sequencing data. J Immunol (2017) 198(5):2202–10. doi:10.4049/jimmunol.1601710
43. Watson CT, Matsen FA, Jackson KJL, Bashir A, Smith ML, Glanville J, et al. Comment on “a database of human immune receptor alleles recovered from population sequencing data”. J Immunol (2017) 198:3371–3. doi:10.4049/jimmunol.1700306
44. Giudicelli V, Chaume D, Lefranc M-P. IMGT/V-QUEST, an integrated software program for immunoglobulin and T cell receptor V-J and V-D-J rearrangement analysis. Nucleic Acids Res (2004) 32:W435–40. doi:10.1093/nar/gkh412
45. Elhanati Y, Marcou Q, Mora T, Walczak AM. repgenHMM: a dynamic programming tool to infer the rules of immune receptor generation from sequence data. Bioinformatics (2016) 32:1943–51. doi:10.1093/bioinformatics/btw112
46. Ralph DK, Matsen FA IV. Consistency of VDJ rearrangement and substitution parameters enables accurate B cell receptor sequence annotation. PLoS Comput Biol (2016) 12:e1004409. doi:10.1371/journal.pcbi.1004409
47. Marcou Q, Mora T, Walczak AM. IGoR: a tool for high-throughput immune repertoire analysis. Q-Bio (2017). Available from: http://arxiv.org/abs/1705.08246
48. Glanville J, Kuo TC, von Büdingen H-C, Guey L, Berka J, Sundar PD, et al. Naive antibody gene-segment frequencies are heritable and unaltered by chronic lymphocyte ablation. Proc Natl Acad Sci U S A (2011) 108:20066–71. doi:10.1073/pnas.1107498108
49. Reddy ST, Ge X, Miklos AE, Hughes RA, Kang SH, Hoi KH, et al. Monoclonal antibodies isolated without screening by analyzing the variable-gene repertoire of plasma cells. Nat Biotechnol (2010) 28:965–9. doi:10.1038/nbt.1673
50. Rubelt F, Bolen CR, McGuire HM, Heiden JAV, Gadala-Maria D, Levin M, et al. Individual heritable differences result in unique cell lymphocyte receptor repertoires of naive and antigen-experienced cells. Nat Commun (2016) 7:11112. doi:10.1038/ncomms11112
51. Shugay M, Bolotin DA, Putintseva EV, Pogorelyy MV, Mamedov IZ, Chudakov DM. Huge overlap of individual TCR beta repertoires. T Cell Biol (2013) 4:466. doi:10.3389/fimmu.2013.00466
52. Covacu R, Philip H, Jaronen M, Almeida J, Kenison JE, Darko S, et al. System-wide analysis of the T cell response. Cell Rep (2016) 14:2733–44. doi:10.1016/j.celrep.2016.02.056
53. Madi A, Shifrut E, Reich-Zeliger S, Gal H, Best K, Ndifon W, et al. T-cell receptor repertoires share a restricted set of public and abundant CDR3 sequences that are associated with self-related immunity. Genome Res (2014) 24:1603–12. doi:10.1101/gr.170753.113
54. Collins AM, Jackson KJL. On being the right size: antibody repertoire formation in the mouse and human. Immunogenetics (2017). doi:10.1007/s00251-017-1049-8
55. Castro R, Navelsaker S, Krasnov A, Du Pasquier L, Boudinot P. Describing the diversity of Ag specific receptors in vertebrates: contribution of repertoire deep sequencing. Dev Comp Immunol (2017) 75:28–37. doi:10.1016/j.dci.2017.02.018
56. Elhanati Y, Murugan A, Callan CG, Mora T, Walczak AM. Quantifying selection in immune receptor repertoires. Proc Natl Acad Sci U S A (2014) 111:9875–80. doi:10.1073/pnas.1409572111
57. Pogorelyy MV, Minervina AA, Chudakov DM, Mamedov IZ, Lebedev YB, Mora T, et al. Method for identification of condition-associated public antigen receptor sequences. Q-Bio (2017). Available from: http://arxiv.org/abs/1709.09703
58. Greiff V, Weber CR, Palme J, Bodenhofer U, Miho E, Menzel U, et al. Learning the high-dimensional immunogenomic features that predict public and private antibody repertoires. J Immunol (2017) 199:2985–97. doi:10.4049/jimmunol.1700594
60. Rempala GA, Seweryn M. Methods for diversity and overlap analysis in T-cell receptor populations. J Math Biol (2013) 67:1–30. doi:10.1007/s00285-012-0589-7
61. Venturi V, Kedzierska K, Tanaka MM, Turner SJ, Doherty PC, Davenport MP. Method for assessing the similarity between subsets of the T cell receptor repertoire. J Immunol Methods (2008) 329:67–80. doi:10.1016/j.jim.2007.09.016
62. Venturi V, Kedzierska K, Turner SJ, Doherty PC, Davenport MP. Methods for comparing the diversity of samples of the T cell receptor repertoire. J Immunol Methods (2007) 321:182–95. doi:10.1016/j.jim.2007.01.019
63. Hershberg U, Prak ETL. The analysis of clonal expansions in normal and autoimmune B cell repertoires. Phil Trans R Soc Lond B Biol Sci (2015) 370:20140239. doi:10.1098/rstb.2014.0239
64. Nouri N, Kleinstein SH. Performance-optimized partitioning of clonotypes from high-throughput immunoglobulin repertoire sequencing data. bioRxiv (2017). doi:10.1101/175315
65. Greiff V, Bhat P, Cook SC, Menzel U, Kang W, Reddy ST. A bioinformatic framework for immune repertoire diversity profiling enables detection of immunological status. Genome Med (2015) 7:49. doi:10.1186/s13073-015-0169-8
66. Gupta NT, Heiden JV, Uduman M, Gadala-Maria D, Yaari G, Kleinstein SH. Change-O: a toolkit for analyzing large-scale B cell immunoglobulin repertoire sequencing data. Bioinformatics (2015) 31(20):3356–8. doi:10.1093/bioinformatics/btv359
67. Oksanen J, Blanchet FG, Kindt R, Legendre P, Minchin PR, O’Hara RB, et al. Vegan: Community Ecology Package. (2015). Available from: http://CRAN.R-project.org/package=vegan
68. Nazarov VI, Pogorelyy MV, Komech EA, Zvyagin IV, Bolotin DA, Shugay M, et al. tcR: An R package for T cell receptor repertoire advanced data analysis. BMC Bioinformatics (2015) 16:175. doi:10.1186/s12859-015-0613-1
69. Shugay M, Bagaev DV, Turchaninova MA, Bolotin DA, Britanova OV, Putintseva EV, et al. VDJtools: unifying post-analysis of T cell receptor repertoires. PLoS Comput Biol (2015) 11:e1004503. doi:10.1371/journal.pcbi.1004503
70. Solomon DL. Unit CUB, Biometrics CUD of, Biology CUD of BS and C. Biometrics Unit Technical Reports: Number BU-573-M: A Comparative Approach to Species Diversity. (1975). Available from: http://ecommons.library.cornell.edu/handle/1813/32672
71. Snir O, Mesin L, Gidoni M, Lundin KEA, Yaari G, Sollid LM. Analysis of celiac disease autoreactive gut plasma cells and their corresponding memory compartment in peripheral blood using high-throughput sequencing. J Immunol (2015) 194:5703–12. doi:10.4049/jimmunol.1402611
72. Mora T, Walczak AM. Renyi entropy, abundance distribution and the equivalence of ensembles. ArXiv Prepr ArXiv160305458 (2016). Available from: http://arxiv.org/abs/1603.05458
73. Afzal S, Gil-Farina I, Gabriel R, Ahmad S, von Kalle C, Schmidt M, et al. Systematic comparative study of computational methods for T-cell receptor sequencing data analysis. Brief Bioinform (2017) 1–13. doi:10.1093/bib/bbx111
74. Mora T, Walczak AM, Bialek W, Callan CG. Maximum entropy models for antibody diversity. Proc Natl Acad Sci U S A (2010) 107:5405–10. doi:10.1073/pnas.1001705107
75. Oakes T, Heather JM, Best K, Byng-Maddick R, Husovsky C, Ismail M, et al. Quantitative characterization of the T cell receptor repertoire of naïve and memory subsets using an integrated experimental and computational pipeline which is robust, economical, and versatile. Front Immunol (2017) 8:1267. doi:10.3389/fimmu.2017.01267
76. Grigaityte K, Carter JA, Goldfless SJ, Jeffery EW, Hause RJ, Jiang Y, et al. Single-cell sequencing reveals αβ chain pairing shapes the T cell repertoire. bioRxiv (2017). doi:10.1101/213462
77. Schwab DJ, Nemenman I, Mehta P. Zipf’s law and criticality in multivariate data without fine-tuning. Phys Rev Lett (2014) 113:068102. doi:10.1103/PhysRevLett.113.068102
78. Jackson KJL, Liu Y, Roskin KM, Glanville J, Hoh RA, Seo K, et al. Human responses to influenza vaccination show seroconversion signatures and convergent antibody rearrangements. Cell Host Microbe (2014) 16:105–14. doi:10.1016/j.chom.2014.05.013
79. Bolkhovskaya OV, Zorin DY, Ivanchenko MV. Assessing T cell clonal size distribution: a non-parametric approach. PLoS One (2014) 9:e108658. doi:10.1371/journal.pone.0108658
80. Kaplinsky J, Arnaout R. Robust estimates of overall immune-repertoire diversity from high-throughput measurements on samples. Nat Commun (2016) 7:11881. doi:10.1038/ncomms11881
81. Chao A, Shen T-J. Nonparametric estimation of Shannon’s index of diversity when there are unseen species in sample. Environ Ecol Stat (2003) 10:429–43. doi:10.1023/A:1021993627070
82. Laydon DJ, Melamed A, Sim A, Gillet NA, Sim K, Darko S, et al. Quantification of HTLV-1 clonality and TCR diversity. PLoS Comput Biol (2014) 10:e1003646. doi:10.1371/journal.pcbi.1003646
83. Laydon DJ, Bangham CRM, Asquith B. Estimating T-cell repertoire diversity: limitations of classical estimators and a new approach. Phil Trans R Soc Lond B Biol Sci (2015) 370:20140291. doi:10.1098/rstb.2014.0291
84. Good IJ. The population frequencies of species and the estimation of population parameters. Biometrika (1953) 40:237–64. doi:10.1093/biomet/40.3-4.237
85. Stern JNH, Yaari G, Heiden JAV, Church G, Donahue WF, Hintzen RQ, et al. B cells populating the multiple sclerosis brain mature in the draining cervical lymph nodes. Sci Transl Med (2014) 6:248ra107. doi:10.1126/scitranslmed.3008879
86. Warren RL, Freeman JD, Zeng T, Choe G, Munro S, Moore R, et al. Exhaustive T-cell repertoire sequencing of human peripheral blood samples reveals signatures of antigen selection and a directly measured repertoire size of at least 1 million clonotypes. Genome Res (2011) 21:790–7. doi:10.1101/gr.115428.110
87. Meng W, Zhang B, Schwartz GW, Rosenfeld AM, Ren D, Thome JJC, et al. An atlas of B-cell clonal distribution in the human body. Nat Biotechnol (2017) 35:879–84. doi:10.1038/nbt.3942
88. Hochreiter S, Schmidhuber J. Long short-term memory. Neural Comput (1997) 9:1735–80. doi:10.1162/neco.1997.9.8.1735
89. Angermueller C, Pärnamaa T, Parts L, Stegle O. Deep learning for computational biology. Mol Syst Biol (2016) 12:878. doi:10.15252/msb.20156651
90. Stubbington MJT, Rozenblatt-Rosen O, Regev A, Teichmann SA. Single-cell transcriptomics to explore the immune system in health and disease. Science (2017) 358:58–63. doi:10.1126/science.aan6828
91. DeKosky B. Paired VH:VL analysis of naïve B cell repertoires and comparison to antigen-experienced B cell repertoires in healthy human donors. Decoding the Antibody Repertoire, Springer Theses (Springer International Publishing) (2017). p. 41–57. https://www.nature.com/articles/nm.3743
92. DeKosky BJ, Ippolito GC, Deschner RP, Lavinder JJ, Wine Y, Rawlings BM, et al. High-throughput sequencing of the paired human immunoglobulin heavy and light chain repertoire. Nat Biotechnol (2013) 31:166–69. doi:10.1038/nbt.2492
93. Howie B, Sherwood AM, Berkebile AD, Berka J, Emerson RO, Williamson DW, et al. High-throughput pairing of T cell receptor α and β sequences. Sci Transl Med (2015) 7:ra131–301. doi:10.1126/scitranslmed.aac5624
94. Miho E, Greiff V, Roskar R, Reddy ST. The fundamental principles of antibody repertoire architecture revealed by large-scale network analysis. bioRxiv (2017). doi:10.1101/124578
95. Bashford-Rogers RJM, Palser AL, Huntly BJ, Rance R, Vassiliou GS, Follows GA, et al. Network properties derived from deep sequencing of human B-cell receptor repertoires delineate B-cell populations. Genome Res (2013) 23:1874–84. doi:10.1101/gr.154815.113
96. Ben-Hamo R, Efroni S. The whole-organism heavy chain B cell repertoire from zebrafish self-organizes into distinct network features. BMC Syst Biol (2011) 5:27. doi:10.1186/1752-0509-5-27
97. Chang Y-H, Kuan H-C, Hsieh TC, Ma KH, Yang C-H, Hsu W-B, et al. Network signatures of IgG immune repertoires in hepatitis B associated chronic infection and vaccination responses. Sci Rep (2016) 6:26556. doi:10.1038/srep26556
98. Hoehn KB, Gall A, Bashford-Rogers R, Fidler SJ, Kaye S, Weber JN, et al. Dynamics of immunoglobulin sequence diversity in HIV-1 infected individuals. Phil Trans R Soc Lond B Biol Sci (2015) 370:20140241. doi:10.1098/rstb.2014.0241
99. Lindner C, Thomsen I, Wahl B, Ugur M, Sethi MK, Friedrichsen M, et al. Diversification of memory B cells drives the continuous adaptation of secretory antibodies to gut microbiota. Nat Immunol (2015) 16:880–8. doi:10.1038/ni.3213
100. Madi A, Poran A, Shifrut E, Reich-Zeliger S, Greenstein E, Zaretsky I, et al. T cell receptor repertoires of mice and humans are clustered in similarity networks around conserved public CDR3 sequences. Elife (2017) 6:e22057. doi:10.7554/eLife.22057
101. Lee SH, Kim P-J, Jeong H. Statistical properties of sampled networks. Phys Rev E (2006) 73:016102. doi:10.1103/PhysRevE.73.016102
102. Sethu H, Chu X. A new algorithm for extracting a small representative subgraph from a very large graph. Phys (2012). Available from: http://arxiv.org/abs/1207.4825
103. Csardi G, Nepusz T. The igraph software package for complex network research, complex system. InterJournal (2006) 1695.
104. Hagberg AA, Schult DA, Swart PJ. Exploring network structure, dynamics, and function using networkx. In: Varoquaux G, Vaught T, Millman J, editors. Proceedings of the 7th Python in Science Conference (SciPy2008). Pasadena, CA USA (2008). p. 11–5.
105. Bastian M, Heymann S, Jacomy M. Gephi: an open source software for exploring and manipulating networks. ICWSM (2009) 8:361–2.
106. Shannon P, Markiel A, Ozier O, Baliga NS, Wang JT, Ramage D, et al. Cytoscape: a software environment for integrated models of biomolecular interaction networks. Genome Res (2003) 13:2498–504. doi:10.1101/gr.1239303
107. Albert R, Jeong H, Barabasi A-L. Error and attack tolerance of complex networks: article: nature. Nature (2000) 406:378–82. doi:10.1101/187120
109. Strauli NB, Hernandez RD. Statistical inference of a convergent antibody repertoire response to influenza vaccine. Genome Med (2016) 8:60. doi:10.1186/s13073-016-0314-z
110. Wine Y, Boutz DR, Lavinder JJ, Miklos AE, Hughes RA, Hoi KH, et al. Molecular deconvolution of the monoclonal antibodies that comprise the polyclonal serum response. Proc Natl Acad Sci U S A (2013) 110:2993–8. doi:10.1073/pnas.1213737110
111. Wine Y, Horton AP, Ippolito GC, Georgiou G. Serology in the 21st century: the molecular-level analysis of the serum antibody repertoire. Curr Opin Immunol (2015) 35:89–97. doi:10.1016/j.coi.2015.06.009
112. Lavinder JJ, Wine Y, Giesecke C, Ippolito GC, Horton AP, Lungu OI, et al. Identification and characterization of the constituent human serum antibodies elicited by vaccination. Proc Natl Acad Sci U S A (2014) 111:2259–64. doi:10.1073/pnas.1317793111
113. Iversen R, Snir O, Stensland M, Kroll JE, Steinsbø Ø, Korponay-Szabó IR, et al.. Strong clonal relatedness between serum and gut IgA despite different plasma cell origins. Cell Rep (2017) 20:2357–67. doi:10.1016/j.celrep.2017.08.036
114. Chen J, Zheng Q, Hammers CM, Ellebrecht CT, Mukherjee EM, Tang H-Y, et al. Proteomic analysis of pemphigus autoantibodies indicates a larger, more diverse, and more dynamic repertoire than determined by B cell genetics. Cell Rep (2017) 18:237–47. doi:10.1016/j.celrep.2016.12.013
115. VanDuijn MM, Dekker LJ, Van IJcken JWF, Sillevis Smitt PAE, Luider TM. Immune repertoire after immunization as seen by next-generation sequencing and proteomics. Front Immunol (2017) 8:1286. doi:10.3389/fimmu.2017.01286
116. Berger CT, Greiff V, Mehling M, Fritz S, Meier MA, Hoenger G, et al. Influenza vaccine response profiles are affected by vaccine preparation and preexisting immunity, but not HIV infection. Hum Vaccin Immunother (2015) 11:391–6. doi:10.1080/21645515.2015.1008930
117. Wang C, Liu Y, Cavanagh MM, Saux SL, Qi Q, Roskin KM, et al. B-cell repertoire responses to varicella-zoster vaccination in human identical twins. Proc Natl Acad Sci U S A (2015) 112:500–5. doi:10.1073/pnas.1415875112
118. Zhu J, Ofek G, Yang Y, Zhang B, Louder MK, Lu G, et al. Mining the antibodyome for HIV-1–neutralizing antibodies with next-generation sequencing and phylogenetic pairing of heavy/light chains. Proc Natl Acad Sci U S A (2013) 110:6470–5. doi:10.1073/pnas.1219320110
119. Hoehn KB, Fowler A, Lunter G, Pybus OG. The diversity and molecular evolution of B-cell receptors during infection. Mol Biol Evol (2016) 33:1147–57. doi:10.1093/molbev/msw015
120. Schramm CA, Sheng Z, Zhang Z, Mascola JR, Kwong PD, Shapiro L. SONAR: a high-throughput pipeline for inferring antibody ontogenies from longitudinal sequencing of B cell transcripts. B Cell Biol (2016) 7:372. doi:10.3389/fimmu.2016.00372
121. Yaari G, Heiden JV, Uduman M, Gadala-Maria D, Gupta N, Stern JN, et al. Models of somatic hypermutation targeting and substitution based on synonymous mutations from high-throughput Immunoglobulin sequencing data. Front B Cell Biol (2013) 4:358. doi:10.3389/fimmu.2013.00358
122. Barak M, Zuckerman NS, Edelman H, Unger R, Mehr R. IgTree©: creating immunoglobulin variable region gene lineage trees. J Immunol Methods (2008) 338:67–74. doi:10.1016/j.jim.2008.06.006
123. Andrews SF, Kaur K, Pauli NT, Huang M, Huang Y, Wilson PC. High preexisting serological antibody levels correlate with diversification of the influenza vaccine response. J Virol (2015) 89(6):3308–17. doi:10.1128/JVI.02871-14
124. Wu X, Zhang Z, Schramm CA, Joyce MG, Do Kwon Y, Zhou T, et al. Maturation and diversity of the VRC01-antibody lineage over 15 years of chronic HIV-1 infection. Cell (2015) 161:470–85. doi:10.1016/j.cell.2015.03.004
125. Yang Z, Rannala B. Molecular phylogenetics: principles and practice. Nat Rev Genet (2012) 13:303–14. doi:10.1038/nrg3186
126. Schliep KP. Phangorn: phylogenetic analysis in R. Bioinformatics (2011) 27:592–3. doi:10.1093/bioinformatics/btq706
127. Paradis E, Claude J, Strimmer K. APE: Analyses of Phylogenetics and Evolution in R language. Bioinformatics (2004) 20:289–90. doi:10.1093/bioinformatics/btg412
128. Boc A, Diallo AB, Makarenkov V. T-REX: a web server for inferring, validating and visualizing phylogenetic trees and networks. Nucleic Acids Res (2012) 40:W573–9. doi:10.1093/nar/gks485
129. Larkin MA, Blackshields G, Brown NP, Chenna R, McGettigan PA, McWilliam H, et al. Clustal W and clustal X version 2.0. Bioinformatics (2007) 23:2947–8. doi:10.1093/bioinformatics/btm404
130. Swofford D, Begle DP. PAUP: Phylogenetic Analysis Using Parsimony, Version 3.1, March 1993. Illinois: Center for Biodiversity, Natural History Survey (1993).
131. Giribet G. TNT: Tree analysis using New Technology. Syst Biol (2005) 54:176–8. doi:10.1080/10635150590905830
132. Felsenstein J. PHYLIP—Phylogeny Inference Package (version 3.2). Cladistics (1989) 5:164–6. doi:10.1111/j.1096-0031.1989.tb00562.x
133. Revell LJ, Chamberlain SA. Rphylip: an R interface for PHYLIP. Methods Ecol Evol (2014) 5:976–81. doi:10.1111/2041-210X.12233
134. Stamatakis A. RAxML version 8: a tool for phylogenetic analysis and post-analysis of large phylogenies. Bioinformatics (2014) 30:1312–3. doi:10.1093/bioinformatics/btu033
135. Guindon S, Lethiec F, Duroux P, Gascuel O. PHYML Online—a web server for fast maximum likelihood-based phylogenetic inference. Nucleic Acids Res (2005) 33:W557–9. doi:10.1093/nar/gki352
136. Kumar S, Stecher G, Tamura K. MEGA7: molecular evolutionary genetics analysis version 7.0 for bigger datasets. Mol Biol Evol (2016) 33:1870–4. doi:10.1093/molbev/msw054
137. Ronquist F, Huelsenbeck JP. MrBayes 3: Bayesian phylogenetic inference under mixed models. Bioinforma Oxf Engl (2003) 19:1572–4. doi:10.1093/bioinformatics/btg180
138. Bouckaert R, Heled J, Kühnert D, Vaughan T, Wu C-H, Xie D, et al. BEAST 2: a software platform for Bayesian evolutionary analysis. PLoS Comput Biol (2014) 10:e1003537. doi:10.1371/journal.pcbi.1003537
139. Yermanos A, Greiff V, Krautler NJ, Menzel U, Dounas A, Miho E, et al. Comparison of methods for phylogenetic B-cell lineage inference using time-resolved antibody repertoire simulations (AbSim). Bioinformatics (2017) 33(24):3938–46. doi:10.1093/bioinformatics/btx533
140. Vieira MC, Zinder D, Cobey S. Selection and neutral mutations drive pervasive mutability losses in long-lived B cell lineages. bioRxiv (2017). doi:10.1101/163741
141. Pinheiro A, de Mera IG, Alves PC, Gortázar C, de la Fuente J, Esteves PJ. Sequencing of modern lepus VDJ genes shows that the usage of VHn genes has been retained in both oryctolagus and lepus that diverged 12 million years ago. Immunogenetics (2013) 65:777–84. doi:10.1007/s00251-013-0728-3
142. Kendall M, Colijn C. Mapping phylogenetic trees to reveal distinct patterns of evolution. Mol Biol Evol (2016) 33:2735–43. doi:10.1093/molbev/msw124
143. Yeap L-S, Hwang JK, Du Z, Meyers RM, Meng F-L, Jakubauskaitė A, et al. Sequence-intrinsic mechanisms that target AID mutational outcomes on antibody genes. Cell (2015) 163:1124–37. doi:10.1016/j.cell.2015.10.042
144. Betz AG, Rada C, Pannell R, Milstein C, Neuberger MS. Passenger transgenes reveal intrinsic specificity of the antibody hypermutation mechanism: clustering, polarity, and specific hot spots. Proc Natl Acad Sci U S A (1993) 90:2385–8. doi:10.1073/pnas.90.6.2385
145. Cui A, Niro RD, Heiden JAV, Briggs AW, Adams K, Gilbert T, et al. A Model of somatic hypermutation targeting in mice based on high-throughput Ig sequencing data. J Immunol (2016) 197(9):3566–74. doi:10.4049/jimmunol.1502263
146. Sheng Z, Schramm CA, Kong R, NISC Comparative Sequencing ProgramMullikin JC, Mascola JR, et al. Gene-specific substitution profiles describe the types and frequencies of amino acid changes during antibody somatic hypermutation. Front Immunol (2017) 8:537. doi:10.3389/fimmu.2017.00537
147. Sheng Z, Schramm CA, Connors M, Morris L, Mascola JR, Kwong PD, et al. Effects of darwinian selection and mutability on rate of broadly neutralizing antibody evolution during HIV-1 infection. PLoS Comput Biol (2016) 12:e1004940. doi:10.1371/journal.pcbi.1004940
148. Hoehn KB, Lunter G, Pybus OG. A phylogenetic codon substitution model for antibody lineages. Genetics (2017) 206:417–27. doi:10.1534/genetics.116.196303
149. DeWitt WS III, Mesin L, Victora GD, Minin VN, Matsen FA IV. Using genotype abundance to improve phylogenetic inference. Q-Bio (2017). Available from: http://arxiv.org/abs/1708.08944
150. Lozupone C, Knight R. UniFrac: a new phylogenetic method for comparing microbial communities. Appl Environ Microbiol (2005) 71:8228–35. doi:10.1128/AEM.71.12.8228-8235.2005
151. de Bourcy CFA, Angel CJL, Vollmers C, Dekker CL, Davis MM, Quake SR. Phylogenetic analysis of the human antibody repertoire reveals quantitative signatures of immune senescence and aging. Proc Natl Acad Sci U S A (2017) 114(5):1105–10. doi:10.1073/pnas.1617959114
152. Lewitus E, Morlon H. Characterizing and comparing phylogenies from their laplacian spectrum. Syst Biol (2016) 65:495–507. doi:10.1093/sysbio/syv116
153. Horns F, Vollmers C, Dekker CL, Quake SR. Signatures of selection in the human antibody repertoire: selective sweeps, competing subclones, and neutral drift. bioRxiv (2017). doi:10.1101/145052
154. Emerson RO, DeWitt WS, Vignali M, Gravley J, Hu JK, Osborne EJ, et al. Immunosequencing identifies signatures of cytomegalovirus exposure history and HLA-mediated effects on the T cell repertoire. Nat Genet (2017) 49:659–65. doi:10.1038/ng.3822
155. Dash P, Fiore-Gartland AJ, Hertz T, Wang GC, Sharma S, Souquette A, et al. Quantifiable predictive features define epitope-specific T cell receptor repertoires. Nature (2017) 547:89–93. doi:10.1038/nature22383
156. Galson JD, Trück J, Fowler A, Clutterbuck EA, Münz M, Cerundolo V, et al. Analysis of B cell repertoire dynamics following hepatitis B vaccination in humans, and enrichment of vaccine-specific antibody sequences. EBioMedicine (2015) 2(12):2070–9. doi:10.1016/j.ebiom.2015.11.034
157. Chen H. VennDiagram: Generate High-Resolution Venn and Euler Plots. (2016). Available from: https://CRAN.R-project.org/package=VennDiagram.
158. Morisita M. Measuring of the dispersion of individuals and analysis of the distributional patterns. Mem Fac Sci Kyushu Univ Ser E (1959) 2:5–23.
159. Dziubianau M, Hecht J, Kuchenbecker L, Sattler A, Stervbo U, Rödelsperger C, et al. TCR Repertoire analysis by next generation sequencing allows complex differential diagnosis of T cell–related pathology. Am J Transplant (2013) 13:2842–54. doi:10.1111/ajt.12431
160. Rempala GA, Seweryn M, Ignatowicz L. Model for comparative analysis of antigen receptor repertoires. J Theor Biol (2011) 269:1–15. doi:10.1016/j.jtbi.2010.10.001
161. Bolen CR, Rubelt F, Vander Heiden JA, Davis MM. The repertoire dissimilarity index as a method to compare lymphocyte receptor repertoires. BMC Bioinformatics (2017) 18:155. doi:10.1186/s12859-017-1556-5
162. Yokota R, Kaminaga Y, Kobayashi TJ. Quantification of inter-sample differences in T-cell receptor repertoires using sequence-based information. Front Immunol (2017) 8:1500. doi:10.3389/fimmu.2017.01500
163. Love MI, Huber W, Anders S. Moderated estimation of fold change and dispersion for RNA-seq data with DESeq2. Genome Biol (2014) 15:550. doi:10.1186/s13059-014-0550-8
164. Bürckert J-P, Dubois ARSX, Faison WJ, Farinelle S, Charpentier E, Sinner R, et al. Functionally convergent B cell receptor sequences in transgenic rats expressing a Human B cell repertoire in response to tetanus toxoid and measles antigens. Front Immunol (2017) 8:1834. doi:10.3389/fimmu.2017.01834
165. Sun Y, Best K, Cinelli M, Heather JM, Reich-Zeliger S, Shifrut E, et al. Specificity, privacy, and degeneracy in the CD4 T cell receptor repertoire following immunization. Front Immunol (2017) 8:430. doi:10.3389/fimmu.2017.00430
166. Sun Cinelli M, Best K, Heather JM, Reich-Zeliger S, Shifrut E, Friedman N, et al. Feature selection using a one dimensional naïve Bayes’ classifier increases the accuracy of support vector machine classification of CDR3 repertoires. Bioinformatics (2017) 33:951–5. doi:10.1093/bioinformatics/btw771
167. Atchley WR, Zhao J, Fernandes AD, Drüke T. Solving the protein sequence metric problem. Proc Natl Acad Sci U S A (2005) 102:6395–400. doi:10.1073/pnas.0408677102
168. Thomas N, Best K, Cinelli M, Reich-Zeliger S, Gal H, Shifrut E, et al. Tracking global changes induced in the CD4 T cell receptor repertoire by immunization with a complex antigen using short stretches of CDR3 protein sequence. Bioinforma Oxf Engl (2014) 30(22):3181–8. doi:10.1093/bioinformatics/btu523
169. Kidera A, Konishi Y, Oka M, Ooi T, Scheraga HA. Statistical analysis of the physical properties of the 20 naturally occurring amino acids. J Protein Chem (1985) 4:23–55. doi:10.1007/BF01025492
170. Konishi H, Komura D, Katoh H, Atsumi S, Koda H, Yamamoto A, et al. Capturing the difference in humoral immunity between normal and tumor environments from RNA sequences of B-cell receptors using supervised machine learning. bioRxiv (2017):187120.
171. Ostmeyer J, Christley S, Rounds WH, Toby I, Greenberg BM, Monson NL, et al. Statistical classifiers for diagnosing disease from immune repertoires: a case study using multiple sclerosis. BMC Bioinformatics (2017) 18:401. doi:10.1186/s12859-017-1814-6
172. Apeltsin L, Wang S, Büdingen H-C, Sirota M. A haystack heuristic for autoimmune disease biomarker discovery using next-gen immune repertoire sequencing data. Sci Rep (2017) 7:5338. doi:10.1038/s41598-017-04439-5
173. Torkamani A, Andersen KG, Steinhubl SR, Topol EJ. High-definition medicine. Cell (2017) 170:828–43. doi:10.1016/j.cell.2017.08.007
174. Boyd SD, Crowe JE Jr. Deep sequencing and human antibody repertoire analysis. Curr Opin Immunol (2016) 40:103–9. doi:10.1016/j.coi.2016.03.008
175. Heather JM, Ismail M, Oakes T, Chain B. High-throughput sequencing of the T-cell receptor repertoire: pitfalls and opportunities. Brief Bioinform (2017):bbw138. doi:10.1093/bib/bbw138
176. Glanville J, Huang H, Nau A, Hatton O, Wagar LE, Rubelt F, et al. Identifying specificity groups in the T cell receptor repertoire. Nature (2017) 547:94–8. doi:10.1038/nature22976
177. Shugay M, Bagaev DV, Zvyagin IV, Vroomans RM, Crawford JC, Dolton G, et al. VDJdb: a curated database of T-cell receptor sequences with known antigen specificity. Nucleic Acids Res (2018) 46(D1):D419–27. doi:10.1093/nar/gkx760
178. Tickotsky N, Sagiv T, Prilusky J, Shifrut E, Friedman N. McPAS-TCR: a manually curated catalogue of pathology-associated T cell receptor sequences. Bioinformatics (2017) 33(18):2924–9. doi:10.1093/bioinformatics/btx286
179. Martin ACR. Protein sequence and structure analysis of antibody variable domains. In: Kontermann R, Dübel S, editors. Antibody Engineering. Berlin, Heidelberg: Springer Berlin Heidelberg (2016). p. 33–51. Available from: http://link.springer.com/10.1007/978-3-642-01147-4_3
180. Vita R, Overton JA, Greenbaum JA, Ponomarenko J, Clark JD, Cantrell JR, et al. The Immune Epitope Database (IEDB) 3.0. Nucleic Acids Res (2015) 43:D405–12. doi:10.1093/nar/gku938
181. Ching T, Himmelstein DS, Beaulieu-Jones BK, Kalinin AA, Do BT, Way GP, et al. Opportunities and obstacles for deep learning in biology and medicine. bioRxiv (2017). doi:10.1101/142760
182. Jurtz VI, Rosenberg Johansen A, Nielsen M, Armenteros A, Juan J, Nielsen H, et al. An introduction to deep learning on biological sequence data – examples and solutions. Bioinformatics (2017) 33(22):3685–90. doi:10.1093/bioinformatics/btx531
183. Lee J, Boutz DR, Chromikova V, Joyce MG, Vollmers C, Leung K, et al. Molecular-level analysis of the serum antibody repertoire in young adults before and after seasonal influenza vaccination. Nat Med (2016) 22:1456–64. doi:10.1038/nm.4224
184. Snir O, Chen X, Gidoni M, du Pré MF, Zhao Y, Steinsbø Ø, et al. Stereotyped antibody responses target posttranslationally modified gluten in celiac disease. JCI Insight (2017) 2:93961. doi:10.1172/jci.insight.93961
185. Mamoshina P, Vieira A, Putin E, Zhavoronkov A. Applications of deep learning in biomedicine. Mol Pharm (2016) 13:1445–54. doi:10.1021/acs.molpharmaceut.5b00982
186. Greiff V, Redestig H, Luck J, Bruni N, Valai A, Hartmann S, et al. A minimal model of peptide binding predicts ensemble properties of serum antibodies. BMC Genomics (2012) 13:79. doi:10.1186/1471-2164-13-79
187. Becattini S, Latorre D, Mele F, Foglierini M, Gregorio CD, Cassotta A, et al. Functional heterogeneity of human memory CD4+ T cell clones primed by pathogens or vaccines. Science (2014) 347:400–6. doi:10.1126/science.1260668
188. Kaplinsky J, Li A, Sun A, Coffre M, Koralov SB, Arnaout R. Antibody repertoire deep sequencing reveals antigen-independent selection in maturing B cells. Proc Natl Acad Sci U S A (2014) 111:E2622–9. doi:10.1073/pnas.1403278111
189. Ghraichy M, Galson JD, Kelly DF, Trück J. B-cell receptor repertoire sequencing in patients with primary immunodeficiency: a review. Immunology (2018) 153(2):145–60. doi:10.1111/imm.12865
190. Khavrutskii IV, Chaudhury S, Stronsky SM, Lee DW, Benko JG, Wallqvist A, et al. Quantitative analysis of repertoire-scale immunoglobulin properties in vaccine-induced B-cell responses. Front Immunol (2017) 8:910. doi:10.3389/fimmu.2017.00910
191. Galson JD, Trück J, Clutterbuck EA, Fowler A, Cerundolo V, Pollard AJ, et al. B-cell repertoire dynamics after sequential hepatitis B vaccination and evidence for cross-reactive B-cell activation. Genome Med (2016) 8:68. doi:10.1186/s13073-016-0322-z
192. Ellebedy AH, Jackson KJL, Kissick HT, Nakaya HI, Davis CW, Roskin KM, et al. Defining antigen-specific plasmablast and memory B cell subsets in human blood after viral infection or vaccination. Nat Immunol (2016) 17:1226–34. doi:10.1038/ni.3533
193. Parameswaran P, Liu Y, Roskin KM, Jackson KKL, Dixit VP, Lee J-Y, et al. Convergent antibody signatures in human dengue. Cell Host Microbe (2013) 13:691–700. doi:10.1016/j.chom.2013.05.008
194. Shlemov A, Bankevich S, Bzikadze A, Turchaninova MA, Safonova Y, Pevzner PA. Reconstructing antibody repertoires from error-prone immunosequencing reads. J Immunol (2017) 199(9):3369–80. doi:10.4049/jimmunol.1700485
195. Safonova Y, Lapidus A, Lill J. IgSimulator: a versatile immunosequencing simulator. Bioinformatics (2015) 31(19):3213–5. doi:10.1093/bioinformatics/btv326
196. Friedensohn S, Khan TA, Reddy ST. Advanced methodologies in high-throughput sequencing of immune repertoires. Trends Biotechnol (2016) 35(3):203–14. doi:10.1016/j.tibtech.2016.09.010
197. Bolotin DA, Poslavsky S, Mitrophanov I, Shugay M, Mamedov IZ, Putintseva EV, et al. MiXCR: software for comprehensive adaptive immunity profiling. Nat Methods (2015) 12:380–1. doi:10.1038/nmeth.3364
198. Gupta NT, Adams KD, Briggs AW, Timberlake SC, Vigneault F, Kleinstein SH. Hierarchical clustering can identify B cell clones with high confidence in Ig repertoire sequencing data. J Immunol (2017) 198(6):2489–99. doi:10.4049/jimmunol.1601850
199. Brown SD, Raeburn LA, Holt RA. Profiling tissue-resident T cell repertoires by RNA sequencing. Genome Med (2015) 7:125. doi:10.1186/s13073-015-0248-x
200. Rizzetto S, Koppstein DN, Samir J, Singh M, Reed JH, Cai CH, et al. B-cell receptor reconstruction from single-cell RNA-seq with VDJPuzzle. bioRxiv (2017):181156. doi:10.1101/181156
201. Mangul S, Mandric I, Yang HT, Strauli N, Montoya D, Rotman J, et al. Profiling adaptive immune repertoires across multiple human tissues by RNA sequencing. bioRxiv (2016):089235. doi:10.1101/089235
202. Lindeman I, Emerton G, Sollid LM, Teichmann S, Stubbington MJT. BraCeR: Reconstruction of B-cell receptor sequences and clonality inference from single-cell RNA-sequencing. bioRxiv (2017):185504. doi:10.1101/185504
203. Stubbington MJT, Lönnberg T, Proserpio V, Clare S, Speak AO, Dougan G, et al. T cell fate and clonality inference from single-cell transcriptomes. Nat Methods (2016) 13:329–32. doi:10.1038/nmeth.3800
204. Li B, Li T, Pignon J-C, Wang B, Wang J, Shukla SA, et al. Landscape of tumor-infiltrating T cell repertoire of human cancers. Nat Genet (2016) 48:725–32. doi:10.1038/ng.3581
205. Geering B, Fussenegger M. Synthetic immunology: modulating the human immune system. Trends Biotechnol (2015) 33:65–79. doi:10.1016/j.tibtech.2014.10.006
206. Roybal KT, Lim WA. Synthetic immunology: hacking immune cells to expand their therapeutic capabilities. Annu Rev Immunol (2017) 35:229–53. doi:10.1146/annurev-immunol-051116-052302
207. Jiang N. Immune engineering: from systems immunology to engineering immunity. Curr Opin Biomed Eng (2017) 1:54–62. doi:10.1016/j.cobme.2017.03.002
208. Liu XS, Mardis ER. Applications of immunogenomics to cancer. Cell (2017) 168:600–12. doi:10.1016/j.cell.2017.01.014
209. Ravn U, Gueneau F, Baerlocher L, Osteras M, Desmurs M, Malinge P, et al. By-passing in vitro screening—next generation sequencing technologies applied to antibody display and in silico candidate selection. Nucleic Acids Res (2010) 38:e193. doi:10.1093/nar/gkq789
Keywords: systems immunology, B-cell receptor, T-cell receptor, phylogenetics, networks, artificial intelligence, immunogenomics, antibody discovery
Citation: Miho E, Yermanos A, Weber CR, Berger CT, Reddy ST and Greiff V (2018) Computational Strategies for Dissecting the High-Dimensional Complexity of Adaptive Immune Repertoires. Front. Immunol. 9:224. doi: 10.3389/fimmu.2018.00224
Received: 22 November 2017; Accepted: 26 January 2018;
Published: 21 February 2018
Edited by:
Jacob Glanville, Distributed Bio, United StatesReviewed by:
Benny Chain, University College London, United KingdomClaude-Agnes Reynaud, Institut National de la Santé et de la Recherche Médicale (INSERM), France
Copyright: © 2018 Miho, Yermanos, Weber, Berger, Reddy and Greiff. This is an open-access article distributed under the terms of the Creative Commons Attribution License (CC BY). The use, distribution or reproduction in other forums is permitted, provided the original author(s) and the copyright owner are credited and that the original publication in this journal is cited, in accordance with accepted academic practice. No use, distribution or reproduction is permitted which does not comply with these terms.
*Correspondence: Sai T. Reddy, c2FpLnJlZGR5QGV0aHouY2g=;
Victor Greiff, dmljdG9yLmdyZWlmZkBtZWRpc2luLnVpby5ubw==