- 1Bone Marrow Transplant, Virginia Commonwealth University Massey Cancer Center, Virginia Commonwealth University, Richmond, VA, United States
- 2Virginia Commonwealth University Center for the Study of Biological Complexity, Virginia Commonwealth University, Richmond, VA, United States
- 3Center for International Blood and Marrow Transplant Research, Minneapolis, MN, United States
- 4Department of Microbiology and Immunology, Virginia Commonwealth University, Richmond, VA, United States
- 5School of Pharmacy, Virginia Commonwealth University, Richmond, VA, United States
- 6Mayo Clinic, Rochester Minnesota and King Faisal Research Hospital, Riyadh, Saudi Arabia
- 7Department of Internal Medicine, Virginia Commonwealth University, Richmond, VA, United States
- 8Department of Psychiatry & Statistical Genomics, Virginia Commonwealth University, Richmond, VA, United States
- 9Department of Physics, Virginia Commonwealth University, Richmond, VA, United States
Alloreactivity compromising clinical outcomes in stem cell transplantation is observed despite HLA matching of donors and recipients. This has its origin in the variation between the exomes of the two, which provides the basis for minor histocompatibility antigens (mHA). The mHA presented on the HLA class I and II molecules and the ensuing T cell response to these antigens results in graft vs. host disease. In this paper, results of a whole exome sequencing study are presented, with resulting alloreactive polymorphic peptides and their HLA class I and HLA class II (DRB1) binding affinity quantified. Large libraries of potentially alloreactive recipient peptides binding both sets of molecules were identified, with HLA-DRB1 generally presenting a greater number of peptides. These results are used to develop a quantitative framework to understand the immunobiology of transplantation. A tensor-based approach is used to derive the equations needed to determine the alloreactive donor T cell response from the mHA-HLA binding affinity and protein expression data. This approach may be used in future studies to simulate the magnitude of expected donor T cell response and determine the risk for alloreactive complications in HLA matched or mismatched hematopoietic cell and solid organ transplantation.
Introduction
Graft-vs.-host Disease (GVHD) represents a significant cause of morbidity and mortality in stem-cell transplant (SCT) recipients (1). GVHD in an HLA-matched allogeneic stem cell transplant is the archetype of an adaptive immune response with donor derived T cells responding to recipient antigens presented on shared HLA class I and class II antigens (2–4). HLA matching has been the bedrock principle of donor selection in SCT, and this is particularly so when the donor is not a close relative (5, 6). Improvements in the fidelity of HLA matching between unrelated transplant donors and recipients has yielded incremental benefits in patient outcomes, with improvements in survival resulting from both a reduction in GVHD risk, as well as reduction in graft loss and optimization of relapse risk. Nevertheless, GVHD remains a therapeutic challenge, and there is little that can be done to predict the outcomes of specific donor-recipient pairs.
This challenge may be surmounted by accounting for genomic variation between the donors and recipients which yields the peptides presented on HLA molecules, known as minor histocompatibility antigens (mHA) (7). While mHA have had a recognized pathophysiologic role in allogeneic SCT outcomes, especially in GVHD pathogenesis, it has not been possible to apply the notion to clinical practice because mHA characterization is a cumbersome process (8–11). Two developments in the past decade have potentially changed this situation. First, the emergence of next generation DNA sequencing techniques, such as single nucleotide polymorphism mapping (12, 13) and whole exome sequencing (WES) to identify the potential antigenic differences (14, 15). The second is the development of machine learning algorithms which allow determination of the binding affinity that different antigens may have for specific HLA molecules (16–18). These two techniques have been combined to develop algorithms that may be used to determine the complex array of recipient antigens that a given donor's T cells may encounter in a recipient (19, 20). Studies reporting exome-wide or other genomic disparities in donors and recipients, have demonstrated a large body of DNA sequence differences between transplant donors and recipients, independent of relatedness and HLA matching (13–15). These large genomic differences have been translated to peptides and HLA class I binding affinities for the resulting peptides determined (19). This too yields large libraries of antigens which may be analyzed by either simulating alloreactive donor T cell responses to these recipient antigens or by more conventional statistical methodology to determine predictive power for alloreactive T cell responses (21, 22). To date, these models have examined recipient peptide presentation on HLA class I and studied the resulting associations. Thus far, no obvious linear relationship has been identified between the magnitude of antigen burden and occurrence of clinical alloreactivity.
As noted above, HLA-matched SCT remains fraught with uncertainty as patients with HLA-matched donors continue to have disparate outcomes (23, 24). A quantitative model of transplant alloreactivity would allow a more complete understanding of the molecular immunology of SCT, potentially help to identify the most suitable donors for specific recipients, and allow personalized determination of the optimal level of immunosuppression. A central assumption in one such quantitative model, the dynamical system model of T cell responses, is that alloreactivity (such as GVHD) risk is a function of the cumulative mHA variation in the context of the HLA type of each donor-recipient pair (DRP) and may thus be regarded as an alloreactivity potential for that pair (14, 19, 25). Clinical outcomes partially depend on the cumulative donor T cell responses to the burden of polymorphic recipient peptides. Previous work applying this dynamical system model to HLA class I presented molecules demonstrates that there are large differences in the simulated T cell responses between different HLA matched DRP (21, 22). In this hypothesis developing paper, previously reported findings of WES of SCT DRP are extended with an analysis of the HLA class II presentation of polymorphic peptides. A comparison of the difference in magnitude of the derived peptide libraries presented on the HLA class I and HLA class II molecules in the DRP is presented. Next, a hypothetical quantitative model is developed which may allow the prediction of alloreactive T cell responses to similar large antigen arrays and their eventual application in clinical medicine. The mathematics introduced in the previously reported dynamical systems model of alloreactive T cell responses is generalized to include both HLA class I and HLA class II presented peptides. The model is expanded to account for different variables which may influence antigen-driven proliferation of T cells, including their own state of antigen-responsiveness and the cytokine milieu. This model may, in the future, permit successful simulation of alloreactive T cell responses between different donors and recipients in SCT.
Methods
Whole Exome Sequencing
After obtaining approval from the institutional review board (IRB) at the Virginia Commonwealth University (VCU), whole exome sequencing (WES) was performed on previously cryopreserved DNA samples from 77 HLA-matched DRP (Supplementary Table 1) as previously described (14, 21). Patients with recurrent or high-risk hematological malignancies undergoing allogeneic SCT at VCU were included in this retrospective study. DNA samples were de-identified by clinical research staff, and submitted for sequencing. The VCU IRB waived the need for informed consent on all adult participants as samples were all archived and previously de-identified, with only VCU BMT clinical research staff retaining access to any patient specific information involved in the retrospective analysis. The Sequencing team did not have access to the sample identity and clinical team did not have access to exome sequencing data. Nextera Rapid Capture Expanded Exome Kit was used to extract exomic regions from the deidentified DNA samples, which were then multiplexed and sequenced on an Illumina HiSeq 2500 to achieve an average coverage of ~90X per sample. 2X100 bp sequencing reads were then aligned to the human reference genome using BWA aligner. Duplicate read alignments were detected and removed using Picard tools. Single nucleotide polymorphisms (SNPs) in both the donor and recipients' exomes were determined using GATK HaplotypeCaller walker. GATK best practices were then implemented to filter and recalibrate the SNPs; and store them in variant call file (VCF) format. To identify SNPs unique to the recipient and absent in the donor the results from the GATK pipeline in VCF format were then parsed through the in-house TraCS (Transplant pair Comparison System) set of perl scripts. TraCS traverses through the genotypes of the called SNPs, systematically excluding identical SNPs or editing them to align with the graft-vs.-host (GVH) direction thereby generating a new VCF with SNPs for a particular DRP in the GVH direction (SNP present in the recipient, absent in the donor; R+/D−). All non-synonymous single-nucleotide polymorphisms (nsSNPs) present in the recipient and donor were identified and recorded in the.vcf format. Subsequent processing of the.vcf files was done using custom python scripts to remove synonymous mutations, eliminate duplicates, and record the coordinates of the SNPs. Non-synonymous SNPs that exist in the recipient but not in the donor were recorded and identified as a potential source of alloreactive antigens. Non-synonymous, single nucleotide polymorphisms (nsSNP) in each DRP would correspond to potential antigens due to the resulting amino acid substitution in oligopeptides which bind HLA in that DRP (Figure 1A).
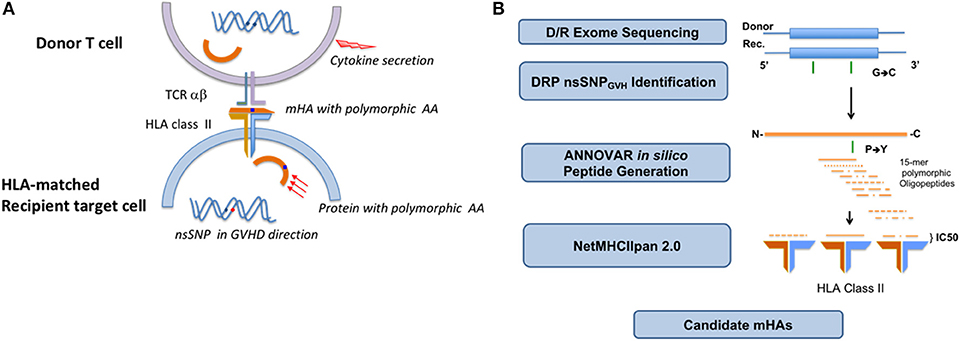
Figure 1. Non-synonymous single nucleotide polymorphisms present in the recipient and absent in the donor yield alloreactive peptides which may be presented to the donor T cells on HLA class I and II molecules. HLA class II presentation and CD4+ T cell recognition and response depicted (A). Schematic depicting the analytic sequence from exome sequencing to HLA class II mHA prediction (B).
In silico Determination of Alloreactive Peptide Sequences
HLA class I bound 9-mer peptides were generated as previously described (19). Briefly, the SNPs in the VCF were annotated either as synonymous or non-synonymous using Annovar. For HLA class I binding affinity determination, the corresponding amino acid polymorphisms along with flanking regions of each protein were extracted using Annovar to build peptide libraries of 17-mers for each DRP, with the SNP encoded AA occupying the central position. This library was further expanded by sliding a 9-mer window over each 17-mer such that the polymorphic amino-acid position changes in each 9-mer. This resulted in the generation of 9 nona-meric peptides/SNP. The HLA class I binding affinity and IC50 values, which quantify the interactions between all these 9-mers for each DRP and all six HLA class I donor molecules (HLA-A, B, and C) was determined; NetHMCpan version 2.8 was run iteratively in parallel mode on a linux cluster using custom python scripts. Parsing the NetMHCPan output, unique peptide-HLA combinations present in the recipient but not in the donor, i.e., possessing a GVHD vector, were identified and organized in order of declining mHA-HLA affinity. To derive the peptide sequences bound to HLA class II molecules an average peptide length of 15 amino acids was used (26). Each of the nsSNPs could potentially be incorporated into the alloreactive peptide of 15 amino acids. The position of the nsSNP encoded polymorphic amino acid in the peptide could vary from the N-terminus to the C-terminus of the peptide. The possible library of peptides will thus be contained within a 29-mer oligopeptide (Figure 1B). Thus, there are 15 different HLA-II binding peptides that could potentially be generated from each nsSNP identified by WES. ANNOVAR was used to generate 29-mer peptides for each nsSNP respectively to study HLA class II presentation. In ANNOVAR, a sliding window method was used with the “seq_padding” option of the “annotate_variation” function to generate the 15 different 15-mers resulting from each nsSNP. Tissue expression of the proteins from which the peptides were derived was determined as previously described (22). Briefly, the Genotype-Tissue Expression (GTEx) portal V6 has publicly available expression level information (Reads/kilobase of transcripts/million mapped reads, RPKM values; http://www.gtexportal.org/home/) for a variety of human tissues over a large number of genes. Since the gene-ids for the proteins that generate the peptides in the DRP peptide library are known, the RPKM values from the GTEx portal for the specific gene across the whole array of tissues of interest were parsed in, namely, skin, lung, salivary gland, esophagus, small intestine, stomach, colon, and liver.
Calculating HLA Binding Affinity of Alloreactive Peptides
Once the peptide library was created for each DRP, the HLA types for the recipient were tabulated from the medical records. HLA matching was performed using high resolution typing for the unrelated donor SCT recipients, and in related donor recipients intermediate resolution typing was performed for HLA class I, and high resolution typing for class II antigens. For class II HLA, HLA-DRB1 alleles for each patient were recorded. Each patient's HLA-DRB1 allele types (and HLA class I alleles, as previously described) along with peptide library were analyzed using NetMHCIIpan 2.0 to derive the binding affinity of each peptide-HLA complex. This was given as an IC50 (half-maximal inhibitory concentration) for each peptide, measured in nano-Molar. This measure of binding affinity provided the concentration of peptide required to displace 50% of a standard peptide from the HLA type to which it would have been bound.
Data Analysis
Peptides present in the recipient but absent in the donor, generated from the ANNOVAR sliding window with IC50 values for all the different patient HLA types were tabulated and duplicates were deleted. Any peptide with the same amino acid sequence but different SNP position along the peptide must have generated from a different area of the exome and was therefore retained in the enumeration. When compiling the peptides binding to different HLA alleles, the patients with homozygous allele for DRB1 had their peptide values doubled to simulate having double the normal number of allele-specific HLA bound peptides presented. Analysis of the number of strongly bound (SB; IC50 ≤ 50 nM) and bound peptides (BP; IC50 ≤ 500 nM) for each patient-HLA allele combination was done by listing the peptides in descending order of binding affinity, as measured by IC50 levels (Tables 1A,B). HLA class I and HLA class II bound peptides were compared numerically for this perspective paper.
Results and Discussion
HLA Bound Alloreactive Peptides
Whole exome sequencing (WES) was performed on the cohort of 77 donor recipient pairs (DRP) of which 75 were evaluable for this analysis. SNPs were identified, following which alloreactive peptide binding affinities to HLA class I and HLA-DRB1 molecules were derived. There was marked variability in the number of peptides presented on the different HLA class I & II molecules between individuals in the cohort. An average of 1,085 ± 513 alloreactive peptides strongly bound (IC50 < 50 nM) all the HLA class I molecules/DRP, vs. 8,320 ± 11,158 peptides binding the two HLA class II molecules/DRP. When compared to an arbitrary sample with a standard deviation of 100 peptides, the variability observed in the test sample was highly significant (P ≤ 0.0001).
In considering the HLA class II bound peptides, HLA matched unrelated donor (MUD) DRP exhibited a higher number of HLA-DRB1-bound peptide (BP); mean: 39,584 alloreactive peptides in HLA matched related donors (MRD) vs. 67,987 in MUD (t-test P < 0.001). When only the strongly bound (SB) peptides are analyzed, this trend while present, no longer remains statistically significant, mean SB 6,077 alloreactive peptides in MRD vs. 9,535 in MUD (p = 0.168) (Figures 2A,B). This is consistent with the larger burden of exome variation in MUD transplant recipients. Significantly more MUD DRP had BP > the median 52,983 peptides for the whole cohort (34/49 vs. 4/26, Fishers Exact test p < 0.0001), as well as SB >4,245 (30/49 vs. 8/26, p = 0.012), when compared to MRD DRP. There was marked variability in the HLA DRB1 allele binding affinity in the various peptides as well as the tissue expression of the proteins from which peptides were derived (Table 1A). This is likely an effect of the randomness observed in exome sequence variation, and the variation in HLA binding affinity of the resulting alloreactive peptides with different HLA molecules, and illustrates the potential for variability in alloreactive antigen presentation between different donors and recipients who undergo SCT.
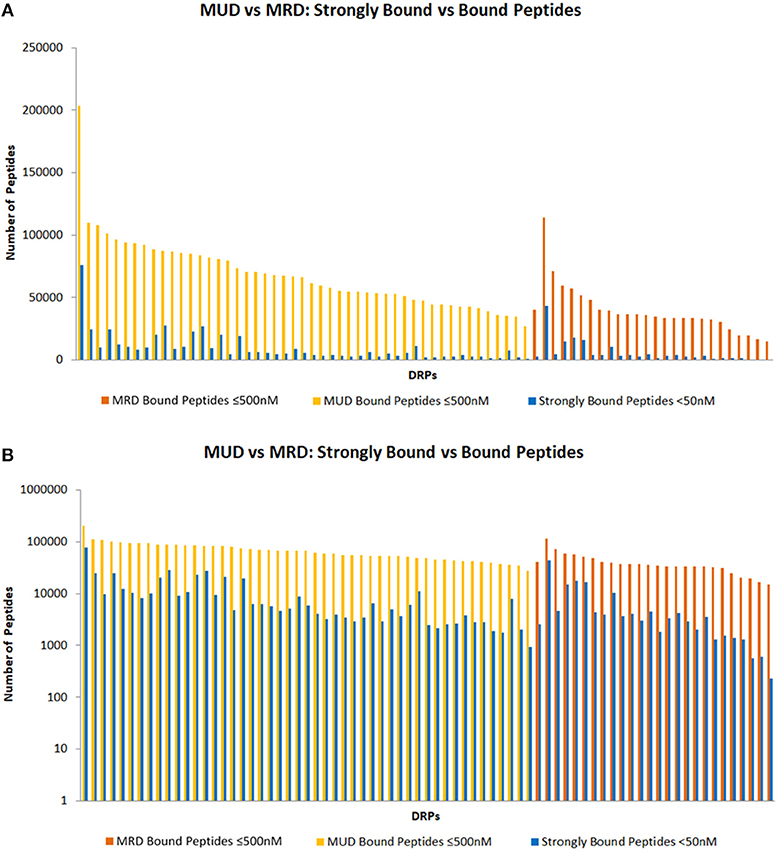
Figure 2. HLA class II bound peptides in HLA MRD and MUD DRP. Depicting SB and BP on standard (A) and logarithmic scales (B).
Computing Donor T Cell Responses From Recipient Antigen Arrays
These data illustrate the large potential that HLA class I and HLA class II molecules have for recipient peptide antigen presentation in the context of allogeneic SCT. Further complexity is added to the antigen driven donor T cell responses by variable tissue expression and different peptide cleavage potential (27, 28). Mass spectrometry studies have in general corroborated the presence of a large number of HLA class I and II bound peptides with several thousand unique peptides identified in studies of both malignant as well as non-malignant cell lines (29–31). These mass spectrometry studies also demonstrate significant variability in the distribution of presented peptides across different HLA class I and class II molecules, with antigen processing and post translational modification playing a role in antigen presentation, in addition to the antigen affinity of the HLA molecules (10, 32). All considered, the magnitude of this antigen burden across the patient population makes it difficult to predict which individual patient will have a poor outcome when utilizing simple statistically determined associations. However, while in and of themselves these parameters may not be definitive for GVHD prediction, given the uniformly large magnitude of mHA identified in the patient cohort examined, these measures if appropriately analyzed, may give insight into the quantitative principles governing the alloreactive T cell immune responses. To accomplish this, it is imperative to understand the quantitative principles at work in donor immune response and use these principles to develop methodology to simulate transplants with different donors in silico. The mHA prediction methodology presented previously and extended herein, augmented by analysis of peptide cleavage sites to more accurately determine the probability of the generation of specific HLA binding alloreactive peptides may allow prediction of alloreactivity potential for different DRP in the future (28). As a first step toward this goal, it was previously shown that donor CD8+ T cell growth simulations may identify patients at risk for moderate to severe GVHD, however these associations were relatively weak (22). While one possible explanation for this is the stochastic nature of alloreactive antigen presentation on HLA molecules (both alloreactive and non-alloreactive peptides may bind HLA), an important limitation in the special case of the model described (HLA class I antigen presentation) was its lack of information on HLA class II mHA presentation and consequent inability to simulate CD4+ helper T cell responses in the donor-recipient pairs involved. Normally, CD4+ T helper cells play an important role in the homing of cytotoxic T cells to infected tissues, and in the case of GVHD to the target tissues (33–35). In the transplant setting, T helper cells will recognize their target alloreactive antigens bound to HLA class II molecules; notably, these differ from the antigens recognized by CD8+ cytotoxic T cells and presented by HLA class I. The T helper cells initiate signaling by secretion of appropriate cytokines (IFN-?, IL-2, IL-12, IL-17 etc.) and set up the homing signal for the cytotoxic T cells to invade the target tissue, which cause tissue injury through direct cytolytic activity. In the present study we estimate the magnitude of alloreactive antigen burden encountered by donor cytotoxic T cells and helper T cells in HLA matched DRP. While this estimate is limited due to lack of protein cleavage site information, and absence of mass spectrometry verification, it may yet allow a more accurate calculation of the likelihood that a patient may develop T cell mediated tissue injury following SCT, then is possible with the current standard of conventional HLA matching.
Comparing HLA Class I and II Bound Alloreactive Peptides
How big a difference might the additional CD4+ T cell simulations make to the alloreactivity predictions of CD8+ T cell simulations performed earlier (22). To estimate this, the in silico derived HLA-DRB1 binding peptide libraries were compared to the numbers of BP and SB on all Class I HLA alleles for the same patients. On average, the number of alloreactive peptides bound to the two HLA-DRB1 alleles with an IC50 < 500 nM, was far greater than the number bound to the HLA class I loci (all 6 HLA-A, B, & C alleles). Significantly more peptides bound HLA DRB1 molecules compared to all the HLA class I molecules combined; BP for HLA DRB1 median 52,983 compared with BP for all HLA class I molecules 4,532, with a median ratio BP-HLA class I/BP-HLA DRB1 per DRP of 0.09 (range: 0.03–0.29; t-test p < 0.0001). The same association was observed with SB with a median ratio of 0.23 per DRP (range: 0.02–4.48; p = 0.0001) (Figure 3A). There was correlation between the number of BP and SB for both HLA class I and to a lesser extent in HLA class II molecules in the DRP studied; Pearson correlation coefficient, R 0.71, p < 0.0001 for HLA DRB1 & 0.94, p < 0.0001 for all HLA class I molecules together (Figure 3B). There was little overlap in the binding affinities of various alloreactive peptides to different HLA class I molecules (Table 1B). The difference observed in HLA class I and II antigen presentation using this computational methodology, may be related to the longer peptide length (15 amino acids) usually presented on the dimeric HLA class II molecules and used for calculations in this study. This increases the size of the peptide pool on offer (9-mer alloreactive peptides/SNP for HLA I vs. 15-mer for HLA II), and consequently the likelihood that alloreactive peptides will be presented. Therefore, validation of these findings utilizing mass spectrometry will be an important next step in this investigation.
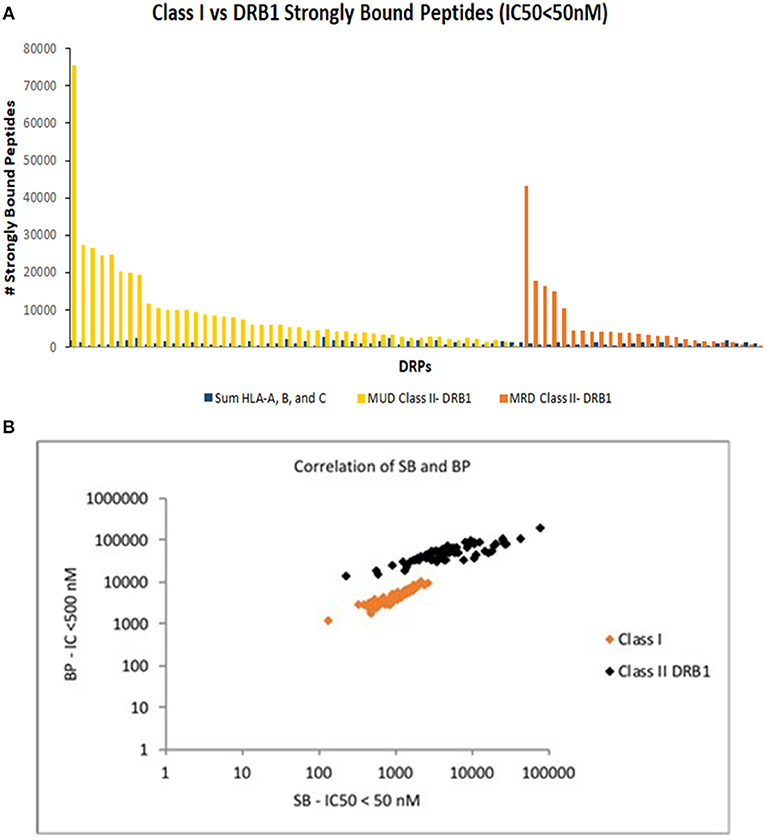
Figure 3. Comparison of HLA class I & class II bound peptides in HLA MRD and MUD DRP. Depicting SB peptides on standard scale in descending order and differentiated by donor type (A). (B) Correlation of SB and BP for both HLA class I and class II molecules in MRD and MUD DRP.
Tissue expression of the proteins from which the peptides presented on HLA class I and class II were derived was also determined. In a subset of patients analyzed (n = 32), analogous to the variability in the number of peptides presented on HLA molecules. Indeed, there was a close correlation between the total number of SB peptides and the SB peptides expressed in each organ (R = 0.99 for both HLA class I and HLA class II molecules). There were differences observed in the expression levels of the proteins of origin for the alloreactive peptides (Tables 1A,B). However, when ordered by expression level as determined by RPKM values reported in the GTEX data base, the number of alloreactive peptides presented in different organs within an individual tended to be relatively similar (Supplementary Figure 1), hinting at the ubiquitous nature of proteins bearing polymorphisms. These overall counts of expressed peptides notwithstanding there is variation in the level of expression of different proteins, identifying this as another critical variable in simulating T cell responses.
T Cell Clonal Proliferation in Response to mHA-HLA Complexes: The Logistic Equation of Growth
The relative differences in HLA class I and HLA class II peptide presentation observed here may contribute to the T cell subset repertoire diversity observed under normal circumstances (36). Previous work has shown there to be far greater diversity in the T cell repertoire of CD4+ T cells than in the CD8+ T cells in the post-transplant period in both allogeneic and autologous SCT (37), particularly when the memory T cell subsets are compared (38). In fact, CD4+ T cell diversity has been found to be about several times greater than CD8+ T cell diversity (39). This may be due to the ability of HLA class II molecules to present a larger number of peptide sequences compared to HLA class I molecules. The antigen-binding region of HLA Class II molecules consists of both an invariant α and a variable β domain, whereas that of HLA Class I molecules contains only α domains. This may allow HLA class II molecules to bind a wider range of peptide sequences (6, 40). This differential antigen presentation likely contributes to the quantitative difference observed between the two classes of T cells and may be understood using the dynamical systems approach. In this model, growth equations have been used to simulate the cytotoxic T cell growth in response to HLA class I presented antigen,
This iterating equation describes the logistic growth of a CD8+ T cell clone Tx in a polyclonal T cell graft infused into a recipient (Figure 4, Supplementary Table 2). N0(Tx) is the T cell count at the time of transplantation (assumed to be 1 for this equation), Nt(Tx) is the T cell count after t iterations (time) following SCT. Nt−1(Tx) represents the T cell count for the previous iteration and K is the proliferation constant that will determine the T cell count at the asymptote (steady state conditions after infinite iterations), K (Tx), representing the maximum T cell count the system would support (carrying capacity); r is the growth rate. In the logistic equation, the steady state count for each T cell clone (KBZ) will be proportional to the product of the binding affinity of the target peptide mHA (peptide y) for the HLA molecule (afmHA = 1/IC50 in Koparde et al., in this paper, By for peptide y) and the affinity of T cell clone, Tx's T cell receptor for the mHA-HLA complex (afTCR = 1/IC50 in Koparde et al., now Zx for T cell clone Tx) (22, 41). In its current form this equation assumes uniform expression levels of the T cell receptors. Presumably in activated T cells, TCR may have a higher levels of expression. Since this equations describes the expansion of T cell clones in response to the cognate antigens, it is likely that higher level of TCR expression may increase the probability of the T cell clones interacting with the APC that present the relevant antigens. In this model, the parameter r, determines the growth rate of the specific clone and reflects the effect of the co-stimulatory molecules and cytokines driving T cell proliferation. This iterating equation gives instantaneous T cell count (magnitude of the proliferative response) in response to antigens presented. The tissue expression of proteins from which peptide y is derived (Py) is a coefficient/multiplier for the steady state T cell population KBZ, and may be estimated by RNA sequencing techniques, and reported as Reads or Fragments Per Kilobase of transcript per Million mapped reads (RPKM or FPKM) (42). In real-world situations the term Py will have a time modifier, et, associated with it, as protein expression and antigen amount declines over time because of tissue injury. This time relationship will be ignored for simplicity at this time. It is important to recognize that in HLA class I-presented antigen-driven T cell expansion, this term is utilized in its entirety given that HLA class I molecules are loaded using peptides derived from proteins present in the cytosol. This however is not the case for HLA class II molecules, which generally present antigens endocytosed from the extracellular environment (43). This means that when calculating helper T cell growth, the term P will be modified to P.c, with a constant, c, reflecting the attenuation of antigen concentration given its “scavenged” nature as opposed to direct cytosolic presence, in other words, 0 < c < 1 (for CD8+ T cells, c = 1). Thus, the equation for determining helper T cell growth will take the general form,
Adjusting the variable P means that the absolute magnitude of the steady state T cell population for each of the dominant (high-ranked) helper T cell clones will be smaller than that for each of the dominant cytotoxic T cell clones, nevertheless because of the greater number of possible antigens presented by HLA class II molecules there may be a greater number of CD4+ T cell clones, and thus greater clonal diversity of helper T cells when compared to cytotoxic T cells. This also means that in a Power law clonal frequency distribution analysis (44, 45), the contribution of the highest-ranking (most numerous) T cell clones to the entire repertoire will be higher with cytotoxic T cells (38). Conversely, in the T helper cell population there will be a larger number of high-ranking clones which contribute a larger component of the overall repertoire. Given the greater number of antigens there may be greater competition between the clones, which in a model accounting for competition between clones will lead to slower growth of helper T cell clones, a relatively frequent clinical observation (46). Also, given the restriction of HLA class II molecules to antigen presenting cells the absolute magnitude of steady-state helper T cell clonal populations will be smaller; however, since HLA class I molecules are expressed on all nucleated cells, cytotoxic T cells get a proliferative signal from many different cell types, therefore steady state T cell clonal counts can be further augmented. From an evolutionary and T cell response sensitivity and specificity standpoint, it is logical that the cytotoxic T cell-recruiting signal provided by CD4+ T helper cells should be more sensitive, triggered by a greater variety of antigens, but when it comes to actual tissue destruction by CD8+ cytotoxic T cells, a more fine-tuned HLA class I bound, shorter peptide with greater specificity required for presentation, provides the necessary stimulus. This would come from the prevention of non-specific binding of peptide antigens to the more “discriminating” HLA class I molecules.
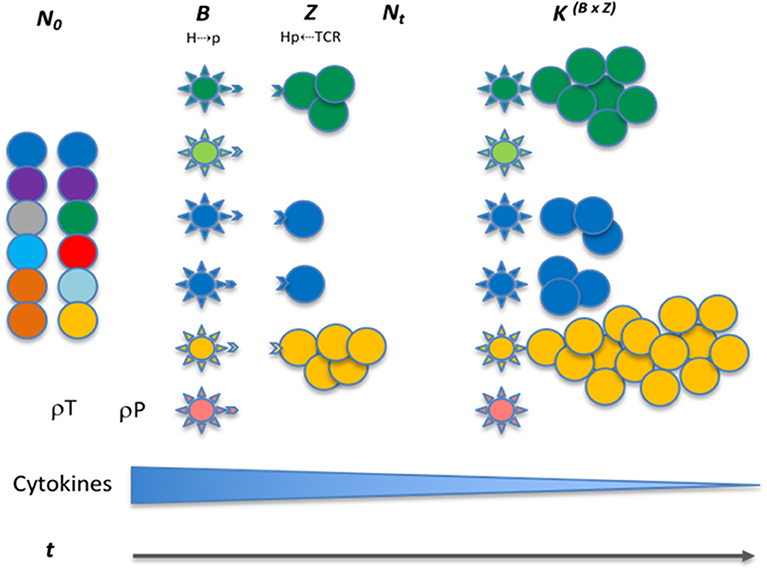
Figure 4. Interaction between donor T cells and recipient antigens presented on APC. Colored circles show different T cell clones with variable antigen affinity. The spiked spheres show antigen presenting cells with HLA molecules, colors indicate unique peptide antigens and correspond to T cell recognition. T cell growth is indicated over time in response to various influences, i.e. peptide affinity for HLA molecules (Vector B), and TCR affinity for HLA-peptide complex (vector Z), both leading to T cell growth. The infused allograft contains T cells with TCR of varying peptide antigen specificity (different colors), these may encounter peptides for which they have affinity (no color difference between APC and T cells), leading to growth. This variability makes antigen response a probability function of the likelihood of T cell presence and peptide antigen presentation (ρP & ρT). The expression level is depicted by more cells presenting the same antigen, making up for weak affinity driving proliferation. The cytokines made by APCs get taken up by the cytokine receptors on the T cells, leading to a diminishing effect as the T cell repertoire expands.
Overlap Between HLA Class I and II Peptide Pools
To determine if there is overlap between HLA class I and II presented peptides, two DRP (one each MRD and MUD), was analyzed to determine the likelihood of peptide presentation from the same proteins on both HLA class I and II molecules. This would result in activation of both CD4+ and CD8+ T cells in the tissues expressing that protein, and greater potential for tissue injury. A comparison of strongly bound peptides (IC50 ≤ 50 nM) demonstrates that these DRP had 143 and 343 genes respectively, that yielded peptides binding both HLA class I and HLA class II. Different degrees of sequence of homology between these 9-mer and 15-mer peptides was observed (Table 2, Supplementary Figure 2). This overlap suggests that if the degree of exome sequence variation in a DRP is sufficiently large, it is plausible that most tissues will potentially present mHA to both helper and cytotoxic T cells. It is also important to note that such protein expression overlap in different tissues in addition to facilitating T helper and cytotoxic T cell interactions, may impact regulatory T cell function such that it serves a protective function against cytotoxic T cells which may recognize alloreactive antigens in a particular tissue. An example of such effect will be the clinical benefit of interleukin-2 observed in chronic GVHD (47).
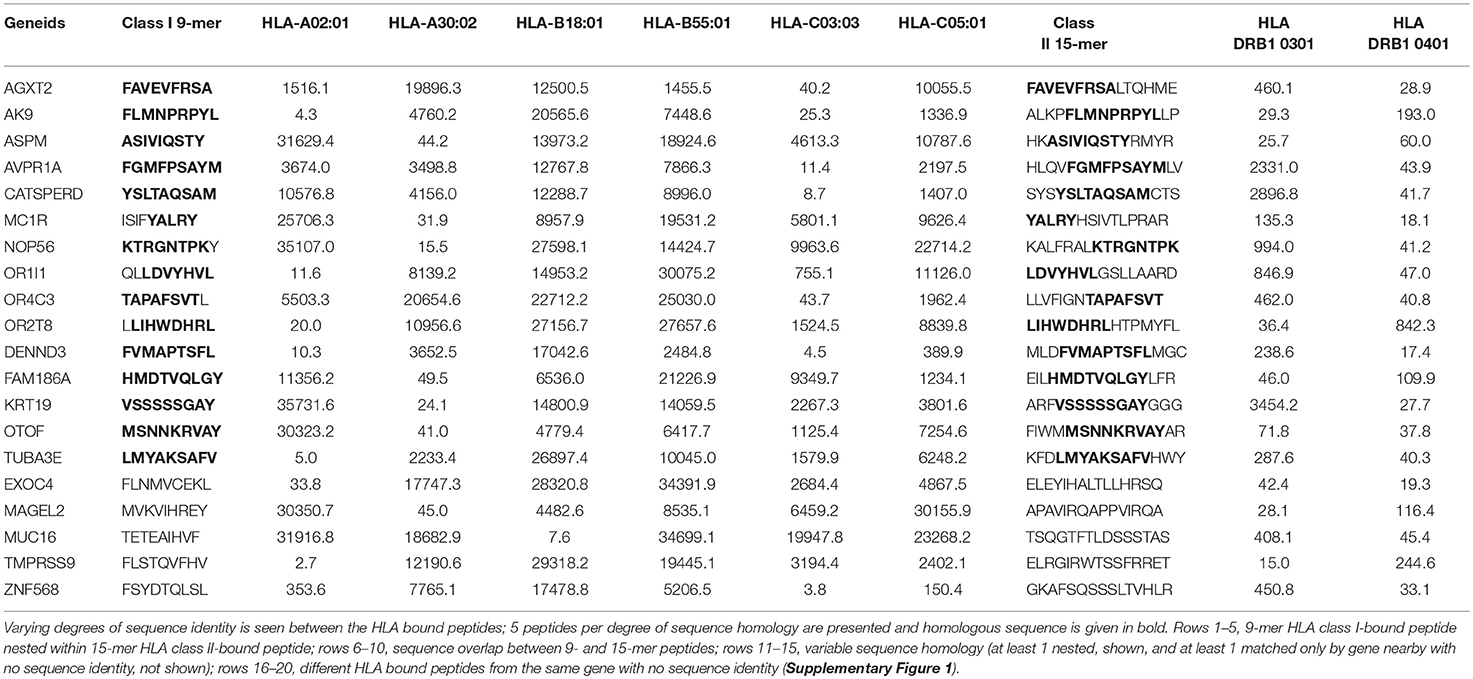
Table 2. MRD DRP 26, polymorphic HLA-bound (both class I & II) peptides derived from the same gene, with binding affinity values in the IC50, 0–50 nM range.
Quantifying mHA-HLA-TCR Interactions: On Matrices, Vectors, and Tensors
Following the above general discussion about T cell behavior, it is necessary to develop a model that will account for the potentially large arrays of antigens being presented in allogeneic SCT as seen in the data sets reported in this paper. As noted earlier, immunotherapy and SCT are fraught with the risk of treatment failure either in the form of relapsed malignancy or immune mediated normal tissue injury (GVHD or graft rejection). Various outcome prediction algorithms and models have been developed using increasingly sophisticated characteristics studied statistically (48, 49). These may allow improvement in clinical outcomes prediction, but often do not provide mechanistic insight into the reason for the observed clinical outcomes. Further, while principles of immune therapy and the mechanisms of T cell action are well known from work on mouse models and in vitro (50, 51), when the antigenic complexity encountered in vivo in human SCT recipients is considered, the existing models do not reliably predict individual clinical outcomes. This is also true of the T cell repertoire that emerges following SCT.
Nevertheless, mathematical methods are available that have long been used in physics to understand natural phenomenon and may be extrapolated to biological systems such as immune response modeling. For example, the concept of vectors and operators has been used to simulate aggregate T cell clonal responses to antigen arrays (21, 22). However, this method is limited in that it requires identification of unique mHA-HLA and it's cognate TCR for application. To overcome this limitation, a related mathematical method, tensor analysis, may be used to simulate the entire T cell clonal response to the vast library of tissue specific antigens presented by the entire spectrum of HLA molecules in an individual. In physics, tensors describe interaction between vector quantities and their components, so they enable determination of variation in vector magnitude and direction and subsequent mapping to a different “state.” In other words, tensors help describe vector transformation when multiple forces are acting upon an object, which itself may be a vector (52–54). It is important to recognize that these methods have been developed for use in “linear” physical systems, however biological systems are seldom linear. They follow non-linear dynamics such as Power laws and exponential growth patterns, which require adaptation of this methodology to account for such behavior and the inherent complexity in biologic systems because of the multiplicity of variables encountered. It is because of it's adaptability that tensor methodology may lend itself to the study of the alloreactive immune response problem. In the example at hand, the donor T cell array infused into the recipient may be considered as a vector, which is modified by the interaction between the T cell receptors (TCR) on the donor T cell clones and the recipient mHA-HLA complexes and is transformed to a new state following SCT. The interacting TCR and mHA-HLA complex in this example may be considered as a tensor, modifying the T cell clonal vector. Tensors remain invariant in different frames of reference and in this application of the concept, the mHA-HLA-TCR interactions, determined by the protein sequences remain constant, regardless of tissues and individuals where the interactions may be occurring. In other words, the unique peptide sequences' affinity to specific HLA molecules and TCR will remain the same across individuals and tissues. In essence, such an alloreactivity tensor comprised of recipient mHA and HLA, in the presence of donor T cell repertoire influences the relative growth of alloreactive T cell clones vs. the non-alloreactive clones. Accordingly, clinical GVHD may or may not manifest.
To understand this notion, consider a basic adaptive immune response to a recipient alloreactive peptide following SCT (or any other antigenic peptide); the first interaction is between the alloreactive recipient peptide and the HLA molecule resulting in the binding and presentation of the peptide on the HLA molecules (Figure 5). Consider two HLA molecules H1 and H2, and two peptides p1 and p2, each recognized by only one of these two HLA molecules; a matrix may be constructed showing the peptides bound to the relevant HLA molecules (55). The possible interactions between the peptides p1 and p2 in a system of two HLA molecules H1 and H2, may be depicted in matrix form as follows.
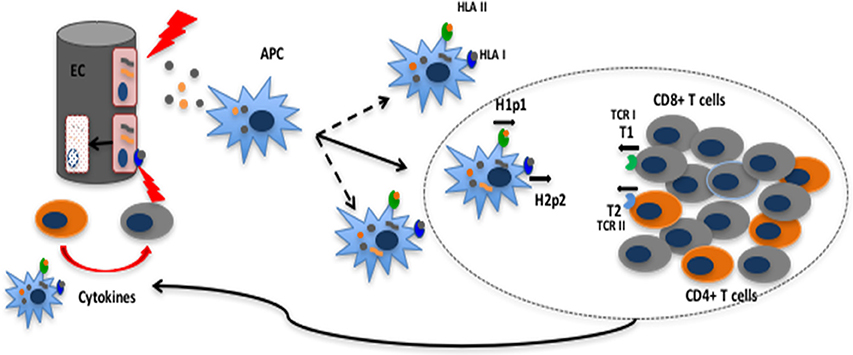
Figure 5. Tissue injury releases polymorphic recipient mHA from epithelial cells (EC); these and endogenous antigens are presented by APC; the APC proliferate and migrate to the lymph node triggering a CD4+ and CD8+ T cell clonal expansion according to the logistic equation of growth. These T cell clones then enter the circulation and migrate to the tissues to initiate tissue injury. Short black arrows in the oval (lymph node) below H1P1 and TCR1 indicate affinity vectors B and Z respectively.
The 0 and 1 represent conditionality of interaction between the peptides and HLA. The matrix on the left-hand side of Equation (3) represents vector quantities, H1p1, H1p2, H2p1, or H2p2, which have a magnitude (binding affinity, expressed in 1/IC50, nM−1) and a “direction” given by the specificity, i.e., unique affinity of the peptide for the HLA molecule. Given affinity of H1 for p1 and H2 for p2, this interaction yields an identity matrix. The interaction between the peptides and HLA molecules constitute a matrix where peptide recognition and binding by an HLA molecule is represented by 1, and the converse situation by 0. Thus, the numbers 1 & 0 represent the selectivity of peptides with a certain sequence (and commensurate length) for specific HLA and vice versa. These two alloreactive HLA-peptide complexes may then be presented to donor T cell clones by the antigen presenting cells, (Figure 5) and specific donor T cell receptors may recognize these unique HLA-peptide combinations and bind. In this example, TCR1 only recognizes H1p1 and, TCR2 only recognizes H2p2. The resulting matrices are given below
The right-hand side of Equation (4) is a tensor with two vector quantities, the affinity of HLA molecule for the peptide and the affinity of the TCR for the peptide-HLA complex, which may be summarized as follows
The matrix depicted in Equation (5), is a tensor of the second rank with two vector quantities, i.e., the affinities B and Z (specific binding between HLA & peptide (B) and between HLA-peptide & TCR (Z)), which are depicted by HpT1,1 and HpT2,2. HpT in this case symbolizes the HLA molecules, peptides and TCR interacting with each other, and the subscripts 1 and 2 are called indices in tensor terminology, identifying interactions between specific molecules (e.g., p1 and p2). The identity matrix reflects the affinity of specific TCR for specific mHA-HLA combinations. It is to be noted that, the same peptides given above may bind other HLA molecules with a different affinity and there may be TCR which bind these alternative antigen complexes with different affinities, constituting different vectors (56) (Figures 6A,B). Along the same lines, a given peptide or TCR may interact with different partners yielding different vector components. For example, in the above matrices, TCR1 may interact with both H1p1 and H1p2, the magnitude of the former will be 1 and the latter, 0. However, given the continuous nature of the IC50s observed for different peptides with different HLA molecules in the analysis presented in this paper it is unlikely that the vector magnitudes are going to be binary in nature. The well-known phenomenon of immune cross reactivity is an example of the vector components which are not binary (57). It is also important to note that the forces (vectors) represented by B (H1p1) and Z (TCR1) may be considered orthogonal (perpendicular) because their direction is imparted by the unique recognition of peptide sequence by HLA, and that of peptide-HLA complex by TCR respectively. Thus, the growth of the T cell clone resulting from this interaction may be considered a “cross” product of these two forces (Sin 90° = 1, for orthogonal vectors) (Figure 6C).
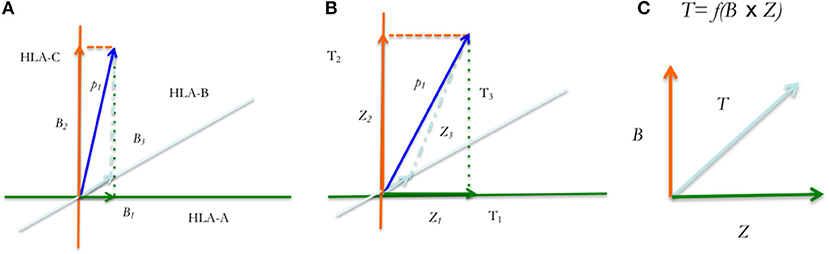
Figure 6. Tensor diagram for the HpT tensor. Peptide p1 binds different HLA molecules, (A–C) with affinities, B1, B2 and B3 (A), while each TCR (TCR1−3) binds these HLA-peptide complexes (with peptide, p1), with affinities Z1, Z2, and Z3 (B). The vectors p1 indicate the average effect of multiple binding affinity vectors. These affinities will remain unaffected in different tissues and individuals. (C) T cell clonal growth in response to the polymorphic peptides is proportional to their product.
T Cell Vector Transformation: Enter Operators
In the SCT context the alloreactivity tensor, HpT, determines the magnitude (and direction) of T cell clonal growth vector in response to antigens. T cell clones with receptors TCR1 and TCR2 respectively will grow in response to the HpT Tensor. It is to be noted that the HLA-peptide driven T cell clonal growth vector is distinct from the TCR affinity vector for HLA-peptide complex, even if one considers that mHA-HLA affinity vector drives T cell clonal growth of the relevant TCR bearing clone. This relationship is analogous to applied force, resulting in motion at a certain velocity and consequent mass displacement which are distinct vector quantities pointing in the same direction (with time being the scalar distinguishing between them; T cell clonal growth is also a time-dependent function). In the above example, the T cell clonal growth vectors, comprising the two T cell clones bearing the T cell receptors TCR1 and TCR2, are termed T1 and T2 respectively. These constitute a vector matrix, which is transformed over time t by the HpT tensor to the vectors T1' and T2'.
In Equation (6), the vector is transformed by the HpT tensor and the logistic operator, previously defined as the logistic equation for T cell growth, which incorporates the term ByZx included in the HpT tensor,
T Cell Growth: The Effect of Co-stimulation, Checkpoints, and Cytokines
In Equation (2) the term r quantifying growth rate is an aggregate measure of different influences on T cells and may be considered a scalar multiple of a tensor quantity. This term represents the cumulative growth effect of the costimulatory and inhibitory molecules present on the T cells and the cytokines present in the environment. In the dynamical system model of T cell growth, the T cell steady state numbers are determined by TCR-mHA-HLA affinity (BZ), also called “Signal 1.” A second critical influence on T cell growth is provided by “Signal 2” mediated by the costimulatory molecule CD28 and inhibitory molecule CTLA4 (S2) may be mathematically represented by, CD28 = 1, CTLA4 = 0. Additionally, the checkpoint mechanism (CP) comprising the PD1 receptors, if engaged may be represented by a variable valued at 0 because no T cell growth will occur, and when absent, valued at 1. Finally, “Signal 3,” (S3) represents the effect of cytokines on T cell growth (Supplementary Figure 3) (58–60). Considering that all these variables contribute to T cell growth, the term r is therefore a composite of the following factors,
Solving this equation for lack of PD1 engagement (1) and the presence of CD28 expression (1) yields,
Solving the equation for CTLA4 expression or PD1 engagement gives r a value of 0, which yields e0 = 1 in Equations (1, 2), consistent with suppression of T cell growth. In other words, the presence of PD1 engagement by PDL-1 or the engagement of CTLA-4 instead of CD28, by CD80 on APC, changes r to zero, eliminating the effect of time t, which changes the value of e to 1 (in Equation 2), leading to growth arrest of the T cell clone.
As for S3, the cytokine mediated signal may also be considered a second order tensor quantity, consisting of a matrix with cytokines and cytokine receptor vectors, because the cytokines and their receptors, have different magnitudes and varying receptor specific effects (directionality) on T cell growth and differentiation. Ignoring the di- or trimerization of cytokine-receptor protein subunits, a simplified version of the cytokine tensor may be constructed as follows,
This is the cytokine tensor, Ck, with the example showing the interaction between IL-12 and IL-10 and their respective receptors. It should be noted that cytokines may bind related receptors with different affinities, providing different vector components. The negative sign means a growth suppressive effect, the net effect of cytokines can either be negative or positive and as a multiple of the CD28-PD1 expression term, the Ck can alter the magnitude and direction of effect of the exponent in Equation (2) (by changing the symbol of r from – to +). Equation (7) therefore is modified to
Further complicating these estimations from a physical standpoint at a cellular level in Equation (8), cytokine exposure will be variable since these effects are “local” to the tissue or lymph nodes. Cytokines likely depend on diffusion via capillary action in the extracellular matrix to create a “field” in which the T cells experience the cytokine effects. These effects on growth are of an exponential nature because of r being an exponent in Equations (1, 2) (61). The receptor expression levels also vary on different cells and confer a direction by means of influencing differentiation and functional specificity to the T cell clones with unique TCR.
Evolution of the T Cell Repertoire: Putting It All Together
The above discussion illustrates the complexity inherent in the multiple factors influencing the T cell responses to antigens presented by HLA molecules. Nevertheless, it makes it clear that despite the complexity, it is possible to describe the immune interactions in mathematical terms, and therefore it is also possible to simulate them, especially when antigen presentation data are available. To do so one may take the example of a random collection of tissue associated peptides. First, consider an alloreactive peptide of any size varying between 7 and 18 amino acids. This peptide will have a choice of binding to HLA class I and II molecules (there are six of each). Therefore, depending on its size and mode of acquisition (extracellular or cytosolic) it will bind to the relevant HLA molecules with a unique binding affinity. It is to be noted that depending on the number of binding HLA molecules and the concentration of competing peptides, there will be a probability function associated with each of these interactions. As demonstrated above in Equations (4, 5), the mHA (polymorphic peptide) binding affinity to available HLA molecules, may be considered to represent the components of the immune response vector to this antigen (or degrees of freedom for the peptide). For most peptides, only one component (one HLA-mHA complex) with the strong interaction will be relevant, and others with weak interactions may be ignored. With the peptide bound to one of the HLA molecules (or more depending on binding affinity with other HLA molecules), it is presented on the APC. If a T cell clone with a TCR which has affinity for the HLA-peptide complex is present (a second probability term), then depending on the CD28/CTLA-4 and PD1 expression levels in the T cell clone, it will grow in the cytokine “field” present in the tissue.
Thus, consider peptides (p1, p2.. pn) with high affinities B1, B2… Bn for HLA molecules H1, H2… Hn respectively, but with a very low-level affinity for the non-corresponding HLA molecules present in the individual (e.g., the components p1H2, p2Hn, pnH1, not considered here for the sake of simplicity in illustration, but fundamental to the tensor concept) (Figure 6). These mHA-HLA complexes have corresponding T cell receptors TCR1, TCR2… TCRm with affinities, Z1, Z2… Zm, the tensor HpT may be written as follows,
Here n and m are indices which indicate the HLA-peptide affinity (Bi) and TCR binding affinity to the HLA-peptide complex (Zj). This is the alloreactivity tensor, and it reflects the interaction of the alloreactive peptides with the HLA molecules in that individual and transforms the T cell clonal vector comprised of the array of the T cell clones bearing the above TCR <Tm> according to the logistic function.
This results in the transformation of the infused donor T cell repertoire, with T1, T2… Tm being transformed to T1', T2'… Tm' following transplant. The logistic growth equation provides the rule for transformation, so Equation (1) may also be rewritten as follows for the ith HLA-bound-peptide, pi, and the responding jth T cell clone <Tj> in a repertoire comprised of T cell clones T1 thru Tm.
Substituting the value of r from Equation (9) in this equation, we get,
The aggregate alloreactive T cell response at time, t then is
This general equation describes the transforming effect of the alloreactivity tensor and the cytokine tensor on the T cell repertoire following SCT. The risk of alloreactivity developing clinically will in this instance be proportional to Nt(1 → Tm).
Dynamical System Model of Alloreactive T Cell Response and Clinical Observations
Does this model explain observations in clinical transplantation? To determine this one may consider the general problem of HLA mismatched SCT and associated negative clinical outcomes (62, 63). In the dynamical system model this phenomenon may be easily understood; the mismatched HLA epitopes are highly expressed so instead of having a low concentration alloreactive protein (the term P.c in Equation 2) governing T cell clonal growth, T cell clones bearing TCR that recognize epitopes on the mismatched HLA molecules may encounter an order of magnitude higher target concentration with marked amplification of the steady state alloreactive T cell clonal populations. Indeed, polymorphisms impacting the level of HLA expression correlate with the likelihood of GVHD developing (64). Further any other peptides bound to the mismatched HLA will be novel antigen complexes, to which thymic tolerance and negative selection would not have occurred in the donor, so donor-derived T cell clones will recognize these non-self-antigens and proliferate. This would result in a strong aggregate immune response to the mismatched HLA (and its presented peptides), and this response may be significantly larger than a mHA-HLA directed immune response in the HLA matched setting (65).
Despite the ability of this model to explain some common clinical observations (logistic growth of T cells, power law distributions, and CD4/CD8 clonal distribution), it will not be validated unless it explains the random occurrence of GVHD following allografting. A discussion of this has previously been presented (22), where the competition between non-alloreactive and alloreactive peptides for HLA binding and presentation was invoked as a possible reason for this, resulting in a probability distribution (ρHpn) for the alloreactive peptide pn to be presented on HLA molecule H. A further consideration in the development of GVHD from these alloreactive T cell clonal growth simulations is the probability function introduced by peptide cleavage potential, which affects the likelihood of antigen presentation, as well as whether the relevant T cell clones are present following transplantation (ρTm). The probability of peptide cleavage (ρpcl) is determined by the amino-acid sequence at the C terminal of the peptide antigens (66), as such, several peptides in our study may have low likelihood of presentation and may be ignored to simplify the model. The likelihood of alloreactive antigen response (ρGVHD) may then be calculated as
Computed for each alloreactive peptide, the probability of clonal expansion of the mHA-targeting-T cells will be significantly diminished as the number of probability terms are introduced into the computations, which explains why despite many potential alloreactive antigens being present in each donor and recipient not every patient develops GVHD.
Another clinical phenomenon, the T cell growth amplification effect of cytokines is well recognized clinically. This is recognized in both the need for lymphodepletion prior to adaptive immunotherapy and in the cytokine release syndrome seen following it (67, 68). Thus far in the dynamical system model discussed above the cytokine tensor effect has been described as modulating rate of T cell clonal growth. However, cytokines effect not only the rate, but they also effect the magnitude of clonal expansion, amplifying the T cell clonal growth. This may be modeled using the iterating equation
This equation demonstrates the effect of the cytokine tensor, Ck, as a time-dependent function, which in the beginning increases the magnitude of T cell clonal growth for clones expressing the relevant cytokine receptors by an order of magnitude. As the number of T cells increases, this time-dependent effect declines to a steady state level since the cytokines are taken up and utilized by the growing T cell population. This relationship plotted over time demonstrates the familiar T cell antigen response curve and mirrors the effect of antigen presenting cell growth previously described (Supplementary Figure 4) [See Koparde et al (22), for discussion of APC-T cell interactions].
A final consideration in building this model is that the antigen matrices presented above are “identity matrices” with binary values of, 1 along the diagonal of a square matrix and 0 elsewhere. In physiologic conditions, however there will be a continuum of values because of differential binding of peptides to various HLA molecules and cross reactivity of T cell receptors with such antigen complexes, generating random number matrices, rather than identity matrices (69). This will add another element of complexity to the antigen-effector interactions, and possibly provides a rationale for complex GVHD phenotypes observed.
In conclusion, the considerable genetic variation present between HLA matched transplant donors and recipients, when analyzed in silico, yields a putative large array of recipient mHA bound to both HLA class I and class II molecules (Supplementary Table 3). The peptide-HLA antigen findings reported here are likely to be revised and refined in the future as the computational biology approach becomes more sophisticated to account for the variables not considered in this analysis. However, the value of this work lies in the mathematical principles of T cell response to antigen arrays it helps illustrate. This mathematical model may be used to optimize donor selection and titrate immunosuppression, first, by utilizing exome sequencing to determine the alloreactive antigen profile. Second, contemporary high throughput sequencing of T cell receptors would allow identification of antigen-HLA specific motifs (70); extended to the donor T cells this may be used to identify potential alloreactive T cell clones (37, 71). These insights using a combination of next generation sequencing and mathematical models accounting for the complexity of alloreactive immune responses may be utilized to allow greater precision in stem cell donor selection and management of immunosuppression following transplantation in particular and cancer immunotherapy in general.
Ethics Statement
The Virginia Commonwealth University's Institutional Review Board approved this study. Previously cryopreserved DNA samples, de-identified by clinical research staff, were used for this study. The samples were acquired from archived DNA remaining after post-transplant studies in the VCU Molecular Biology laboratory. The VCU IRB waived the need for informed consent on all adult participants as samples were all archived and previously de-identified with only VCU BMT research staff and the study PI retaining access to any PHI involved in retrospective analysis. The Sequencing team did not have access to the sample identity and clinical team did not have access to exome sequencing data.
Author Contributions
VK performed bioinformatic analysis of the sequencing data to identify unique peptides and their HLA binding affinity, as well as tissue expression and wrote the paper. MS performed sequencing on samples identified and procured by CR. AS analyzed the data, performed statistical analysis and wrote the paper. AS, CH, and MJ-L created data files with unique peptides and HLA IC50 values and wrote the paper. AT, MN, and GB developed the WES study. AT developed the vector-operator and tensor models and wrote the manuscript. JR, RQ, MN, SH, DW, BA, and JM critically reviewed the manuscript and provided expert commentary. All the authors contributed to writing the manuscript. Sequencing and Bioinformatics Analysis was performed in the Genomics Core of the Nucleic Acids Research Facilities at VCU, supervised by GB.
Conflict of Interest Statement
The authors declare that the research was conducted in the absence of any commercial or financial relationships that could be construed as a potential conflict of interest.
Acknowledgments
This study conducted at Virginia Commonwealth University's Massey Cancer Center was supported, in part, by research funding from the NIH-NCI Cancer Center Support Grant (P30-CA016059; PI: Gordon Ginder, MD) and by research funding from Virginia's Commonwealth Health Research Board Grant #236-11-13 (PI: MN, PhD). We acknowledge Allison Scalora and David J Kobulnicky for collection of clinical outcomes data and analysis. Data files associated with the manuscript are available at, https://osf.io/d98px/. Additional Supplementary Materials included.
A pre-print version of this paper is available on the Cold Spring Harbor Laboratory server, bioRxiv (72).
Supplementary Material
The Supplementary Material for this article can be found online at: https://www.frontiersin.org/articles/10.3389/fimmu.2018.02284/full#supplementary-material
References
1. Storb R, Gyurkocza B, Storer BE, Sorror ML, Blume K, et al. Graft-versus-host disease and graft-versus-tumor effects after allogeneic hematopoietic cell transplantation. J Clin Oncol. (2013) 31:1530–8. doi: 10.1200/JCO.2012.45.0247
2. Spierings E, Kim YH, Hendriks M, Borst E, Sergeant R, Canossi A, et al. Multicenter analyses demonstrate significant clinical effects of minor histocompatibility antigens on GvHD and GvL after HLA-matched related and unrelated hematopoietic stem cell transplantation. Biol Blood Marrow Transplant. (2013) 19:1244–53. doi: 10.1016/j.bbmt.2013.06.001
3. Kosugi-Kanaya M, Ueha S, Abe J, Shichino S, Shand FHW, Morikawa T, et al. Long-lasting graft-derived donor T cells contribute to the pathogenesis of chronic graft-versus-host disease in mice. Front Immunol. (2017) 8:1842. doi: 10.3389/fimmu.2017.01842
4. Amir AL, van der Steen DM, Hagedoorn RS, Kester MG, van Bergen CA, Drijfhout JW, et al. Allo-HLA-reactive T cells inducing graft-versus-host disease are single peptide specific. Blood (2011) 118:6733–42. doi: 10.1182/blood-2011-05-354787
5. Ferrara GB, Bacigalupo A, Lamparelli T, Lanino E, Delfino L, Morabito A, et al. Bone marrow transplantation from unrelated donors: the impact of mismatches with substitutions at position 116 of the human leukocyte antigen class I heavy chain. Blood (2001) 98:3150 doi: 10.1182/blood.V98.10.3150
6. Woolfrey A, Klein JP, Haagenson M, Spellman S, Petersdorf E, Oudshoorn M, et al. HLA-C antigen mismatch is associated with worse outcome in unrelated donor peripheral blood stem cell transplantation. Biol Blood Marrow Transplant. (2011) 17:885–92. doi: 10.1016/j.bbmt.2010.09.012
7. Griffioen M, van Bergen CA, Falkenburg JH. Autosomal minor histocompatibility antigens: how genetic variants create diversity in immune targets. Front Immunol. (2016) 7:100. doi: 10.3389/fimmu.2016.00100
8. Terakura S, Murata M, Warren EH, Sette A, Sidney J, Naoe T, et al. A single minor histocompatibility antigen encoded by UGT2B17 and presented by human leukocyte antigen-A*2902 and -B*4403. Transplantation (2007) 83:1242–8. doi: 10.1097/01.tp.0000259931.72622.d1
9. Vogt MH, van den Muijsenberg JW, Goulmy E, Spierings E, Kluck P, Kester MG, et al. The DBY gene codes for an HLA-DQ5-restricted human male-specific minor histocompatibility antigen involved in graft-versus-host disease. Blood (2002) 99:3027–32. doi: 10.1182/blood.V99.8.3027
10. Abelin JG, Keskin DB, Sarkizova S, Hartigan CR, Zhang W, Sidney J, et al. Mass spectrometry profiling of HLA-associated peptidomes in mono-allelic cells enables more accurate epitope prediction. Immunity (2017) 46:315–26. doi: 10.1016/j.immuni.2017.02.007
11. Ofran Y, Kim HT, Brusic V, Blake L, Mandrell M, Wu CJ, et al. Diverse patterns of T-cell response against multiple newly identified human Y chromosome-encoded minor histocompatibility epitopes. Clin Cancer Res. (2010) 16:1642–51. doi: 10.1158/1078-0432.CCR-09-2701
12. Armistead PM, Liang S, Li H, Lu S, Van Bergen CA, Alatrash G, et al. Common minor histocompatibility antigen discovery based upon patient clinical outcomes and genomic data. PLoS ONE (2011) 6:e23217. doi: 10.1371/journal.pone.0023217
13. Martin PJ, Levine DM, Storer BE, Warren EH, Zheng X, Nelson SC, et al. Genome-wide minor histocompatibility matching as related to the risk of graft-versus-host disease. Blood (2017) 129:791–8. doi: 10.1182/blood-2016-09-737700
14. Sampson JK, Sheth NU, Koparde VN, Scalora AF, Serrano MG, Lee V, et al. Whole exome sequencing to estimate alloreactivity potential between donors and recipients in stem cell transplantation. Br J Haematol. (2014) 166:566–70. doi: 10.1111/bjh.12898
15. Pineda S, Sigdel TK, Chen J, Jackson AM, Sirota M, Sarwal MM. Novel non-histocompatibility antigen mismatched variants improve the ability to predict antibody-mediated rejection risk in kidney transplant. Front Immunol. (2017) 8:1687. doi: 10.3389/fimmu.2017.01687
16. Hoof I, Peters B, Sidney J, Pedersen LE, Sette A, Lund O, et al. NetMHCpan, a method for MHC class I binding prediction beyond humans. Immunogenetics (2009) 61:1–13. doi: 10.1007/s00251-008-0341-z
17. Rasmussen M, Fenoy E, Harndahl M, Kristensen AB, Nielsen IK, Nielsen M, et al. Pan-specific prediction of peptide-MHC class I complex stability, a correlate of T cell immunogenicity. J Immunol. (2016) 197:1517–24. doi: 10.4049/jimmunol.1600582
18. Zhang H, Lundegaard C, Nielsen M. Pan-specific MHC class I predictors: a benchmark of HLA class I pan-specific prediction methods. Bioinformatics (2009) 25:83–9. doi: 10.1093/bioinformatics/btn579
19. Jameson-Lee M, Koparde V, Griffith P, Scalora AF, Sampson JK, Khalid H, et al. In silico derivation of HLA-specific alloreactivity potential from whole exome sequencing of stem-cell transplant donors and recipients: understanding the quantitative immunobiology of allogeneic transplantation. Front Immunol. (2014) 5:529. doi: 10.3389/fimmu.2014.00529
20. Granados DP, Sriranganadane D, Daouda T, Zieger A, Laumont CM, Caron-Lizotte O, et al. Impact of genomic polymorphisms on the repertoire of human MHC class I-associated peptides. Nat Commun. (2014) 5:3600. doi: 10.1038/ncomms4600
21. Abdul Razzaq B, Scalora A, Koparde VN, Meier J, Mahmood M, Salman S, et al. Dynamical system modeling to simulate donor T cell response to whole exome sequencing-derived recipient peptides demonstrates different alloreactivity potential in HLA-matched and -mismatched donor-recipient pairs. Biol Blood Marrow Transplant. (2016) 22:850–61. doi: 10.1016/j.bbmt.2015.11.1103
22. Koparde V, Abdul Razzaq B, Suntum T, Sabo R, Scalora A, Serrano M, et al. Dynamical system modeling to simulate donor T cell response to whole exome sequencing-derived recipient peptides: understanding randomness in alloreactivity incidence following stem cell transplantation. PLoS ONE (2017) 12:e0187771. doi: 10.1371/journal.pone.0187771
23. Valcárcel D, Sierra J, Wang T, Kan F, Gupta V, Hale GA, et al. One-antigen mismatched related versus HLA-matched unrelated donor hematopoietic stem cell transplantation in adults with acute leukemia: center for International Blood and Marrow TransplantResearch results in the era of molecular HLA typing. Biol Blood Marrow Transplant. (2011) 17:640–8. doi: 10.1016/j.bbmt.2010.07.022
24. Konuma T, Tsukada N, Kanda J, Uchida N, Ohno Y, Miyakoshi S, et al. Comparison of transplant outcomes from matched sibling bone marrow or peripheral blood stem cell and unrelated cord blood in patients 50 years or older. Am J Hematol. (2016) 91:E284–92. doi: 10.1002/ajh.24340
25. Toor AA, Kobulnicky JD, Salman S, Roberts CH, Jameson-Lee M, Meier J, et al. Stem cell transplantation as a dynamical system: are clinical outcomes deterministic? Front Immunol. (2014) 5:613. doi: 10.3389/fimmu.2014.00613
26. van Niel G, Wubbolts R, Stoorvogel W. Endosomal sorting of MHC class II determines antigen presentation by dendritic cells. Curr Opin Cell Biol. (2008) 20:437–44. doi: 10.1016/j.ceb.2008.05.011
27. Kloetzel PM. Antigen processing by the proteasome. Nat Rev Mol Cell Biol. (2001) 2:179–87. doi: 10.1038/35056572
28. Diez-Rivero CM, Lafuente EM, Reche PA. Computational analysis and modeling of cleavage by the immunoproteasome and the constitutive proteasome. BMC Bioinformatics (2010) 11:479. doi: 10.1186/1471-2105-11-479
29. Chong C, Marino F, Pak H, Racle J, Daniel RT, Müller M, et al. High-throughput and sensitive immunopeptidomics platform reveals profound Interferonγ-mediated remodeling of the Human Leukocyte Antigen(HLA) ligandome. Mol Cell Proteomics (2018) 17:533–48. doi: 10.1074/mcp.TIR117.000383
30. Mommen GP, Marino F, Meiring HD, Poelen MC, van Gaans-van den Brink JA, Mohammed S, et al. Sampling from the proteome to the Human Leukocyte Antigen-DR (HLA-DR) ligandome proceeds via high specificity. Mol Cell Proteomics (2016) 15:1412–23. doi: 10.1074/mcp.M115.055780
31. Berlin C, Kowalewski DJ, Schuster H, Mirza N, Walz S, Handel M, et al. Mapping the HLA ligandome landscape of acute myeloid leukemia: a targeted approach toward peptide-based immunotherapy. Leukemia (2015) 29:647–59. doi: 10.1038/leu.2014.233
32. Godkin AJ, Smith KJ, Willis A, Tejada-Simon MV, Zhang J, Elliott T, et al. Naturally processed HLA class II peptides reveal highly conserved immunogenic flanking region sequence preferences that reflect antigen processing rather than peptide-MHC interactions. J Immunol. (2001) 166:6720–7. doi: 10.4049/jimmunol.166.11.6720
33. Lai HY, Chou TY, Tzeng CH, Lee OK. Cytokine profiles in various graft-versus-host disease target organs following hematopoietic stem cell transplantation. Cell Transplant. (2012) 21:2033–45. doi: 10.3727/096368912X653110
34. Furlan SN, Watkins B, Tkachev V, Cooley S, Panoskaltsis-Mortari A, Betz K, et al. Systems analysis uncovers inflammatory Th/Tc17-driven modules during acute GVHD in monkey and human T cells. Blood (2016) 128:2568–79. doi: 10.1182/blood-2016-07-726547
35. Valbon SF, Condotta SA, Richer MJ. Regulation of effector and memory CD8(+) T cell function by inflammatory cytokines. Cytokine (2016) 82:16–23. doi: 10.1016/j.cyto.2015.11.013
36. Wang C, Sanders CM, Yang Q, Schroeder HW Jr, Wang E, Babrzadeh F, et al. High throughput sequencing reveals a complex pattern of dynamic interrelationships among human T cell subsets. Proc Natl Acad Sci USA. (2010) 107:1518–23. doi: 10.1073/pnas.0913939107
37. Kanakry CG, Coffey DG, Towlerton AM, Vulic A, Storer BE, Chou J, et al. Origin and evolution of the T cell repertoire after posttransplantation cyclophosphamide. JCI Insight (2016) 1:e86252. doi: 10.1172/jci.insight.86252
38. Qi Q, Liu Y, Cheng Y, Glanville J, Zhang D, Lee JY, et al. Diversity and clonal selection in the human T-cell repertoire. Proc Natl Acad Sci USA. (2014) 111:13139–44. doi: 10.1073/pnas.1409155111
39. van Heijst JW, Ceberio I, Lipuma LB, Samilo DW, Wasilewski GD, Gonzales AM, et al. Quantitative assessment of T cell repertoire recovery after hematopoietic stem cell transplantation. Nat Med. (2013) 19:372–7. doi: 10.1038/nm.3100
40. Choo SY. The HLA system: genetics, immunology, clinical testing, and clinical implications. Yonsei Med J. (2007) 48:11–23. doi: 10.3349/ymj.2007.48.1.11
41. Tan MP, Gerry AB, Brewer JE, Melchiori L, Bridgeman JS, Bennett AD, et al. T cell receptor binding affinity governs the functional profile of cancer-specific CD8+ T cells. Clin Exp Immunol. (2015) 180:255–70. doi: 10.1111/cei.12570
42. Bassani-Sternberg M, Pletscher-Frankild S, Jensen LJ, Mann M. Mass spectrometry of human leukocyte antigen class I peptidomes reveals strong effects of protein abundance and turnover on antigen presentation. Mol Cell Proteomics (2015) 14:658–73. doi: 10.1074/mcp.M114.042812
43. Neefjes J, Jongsma ML, Paul P, Bakke O. Towards a systems understanding of MHC class I and MHC class II antigen presentation. Nat Rev Immunol. (2011) 11:823–36. doi: 10.1038/nri3084
44. Meier J, Roberts C, Avent K, Hazlett A, Berrie J, Payne K, et al. Fractal organization of the human T cell repertoire in health and after stem cell transplantation. Biol Blood Marrow Transplant. (2013) 19:366–77. doi: 10.1016/j.bbmt.2012.12.004
45. Bolkhovskaya OV, Zorin DY, Ivanchenko MV. Assessing T cell clonal size distribution: a non-parametric approach. PLoS ONE (2014) 9:e108658. doi: 10.1371/journal.pone.0108658
46. Martínez C, Urbano-Ispizua A, Rozman C, Marín P, Rovira M, Sierra J, et al. Immune reconstitution following allogeneic peripheral blood progenitor cell transplantation: comparison of recipients of positive CD34+ selected grafts with recipients of unmanipulated grafts. Exp Hematol. (1999) 27:561–8. doi: 10.1016/S0301-472X(98)00029-0
47. Koreth J, Matsuoka K, Kim HT, McDonough SM, Bindra B, Alyea EP III, et al. Interleukin-2 and regulatory T cells in graft-versus-host disease. N Engl J Med. (2011) 365:2055–66. doi: 10.1056/NEJMoa1108188
48. Pidala J, Anasetti C, Kharfan-Dabaja MA, Cutler C, Sheldon A, Djulbegovic B. Decision analysis of peripheral blood versus bone marrow hematopoietic stem cells for allogeneic hematopoietic cell transplantation. Biol Blood Marrow Transplant. (2009) 15:1415–21. doi: 10.1016/j.bbmt.2009.07.009
49. Koreth J, Pidala J, Perez WS, Deeg HJ, Garcia-Manero G, Malcovati L, et al. Role of reduced-intensity conditioning allogeneic hematopoietic stem-cell transplantation in older patients with de novo myelodysplastic syndromes: an international collaborative decision analysis. J Clin Oncol. (2013) 31:2662–70. doi: 10.1200/JCO.2012.46.8652
50. Hülsdünker J, Zeiser R. Insights into the pathogenesis of GvHD: what mice can teach us about man. Tissue Antigens. (2015) 85:2–9. doi: 10.1111/tan.12497
51. Schroeder MA, DiPersio JF. Mouse models of graft-versus-host disease: advances and limitations. Dis Model Mech. (2011) 4:318–33. doi: 10.1242/dmm.006668
52. Nearing J. Tensors. Mathematical Tools for Physics. Mineola, NY: Dover Publications Inc. (2010). p. 327–59.
53. Feynman, Leighton & Sands. Tensors. The Feynman Lectures On Physics. Chapter 31 (online version). California Institute of Technology (2013).
54. Fleisch D. Higher Rank Tensors. A Students Guide to Vectors and Tensors. Cambridge, UK: Cambridge University Press (2012). p. 132.
55. Jordan T. Matrices. Quantum Mechanics in Simple Matrix Form. Mineola, NY: Dover Publications (2005). p. 19.
56. Birnbaum ME, Mendoza JL, Sethi DK, Dong S, Glanville J, Dobbins J, et al. Deconstructing the peptide-MHC specificity of T cell recognition. Cell (2014) 157:1073–87. doi: 10.1016/j.cell.2014.03.047
57. Hall CE, Koparde VN, Jameson-Lee M, Elnasseh AG, Scalora AF, Kobulnicky DJ, et al. Sequence homology between HLA-bound cytomegalovirus and human peptides: A potential trigger for alloreactivity. PLoS ONE (2017) 12:e0178763. doi: 10.1371/journal.pone.0178763
58. Pietropaolo M, Surhigh JM, Nelson PW, Eisenbarth GS. Primer: immunity and autoimmunity. Diabetes (2008) 57:2872–82. doi: 10.2337/db07-1691
59. Miller DM, Thornley TB, Greiner DL, Rossini AA. Viral infection: a potent barrier to transplantation tolerance. Clin Dev Immunol. (2008) 2008:742810. doi: 10.1155/2008/742810
60. O'Donnell JS, Smyth MJ, Teng MWL. PD1 functions by inhibiting CD28-mediated co-stimulation. Clin Transl Immunol. (2017) 6:e138. doi: 10.1038/cti.2017.15
61. Bonacci B, Edwards B, Jia S, Williams CB, Hessner MJ, Gauld SB, et al. Requirements for growth and IL-10 expression of highly purified human T regulatory cells. J Clin Immunol. (2012) 32:1118–28. doi: 10.1007/s10875-012-9701-4
62. Kekre N, Mak KS, Stopsack KH, Binder M, Ishii K, Brånvall E, et al. Impact of HLA-mismatch in unrelated donor hematopoietic stem cell transplantation: a meta-analysis. Am J Hematol. (2016) 91:551–5. doi: 10.1002/ajh.24342
63. Morishima Y, Kashiwase K, Matsuo K, Azuma F, Morishima S, Onizuka M, et al. Biological significance of HLA locus matching in unrelated donor bone marrow transplantation. Blood (2015) 125:1189–97. doi: 10.1182/blood-2014-10-604785
64. Petersdorf EW, Malkki M, O'hUigin C, Carrington M, Gooley T, Haagenson MD, et al. High HLA-DP expression and graft-versus-host disease. N Engl J Med. (2015) 373:599–609. doi: 10.1056/NEJMoa1500140
65. Gaschet J, Treviño MA, Cherel M, Vivien R, Garcia-Sahuquillo A, Hallet MM, et al. HLA-target antigens and T-cell receptor diversity of activated T cells invading the skin during acute graft-versus-host disease. Blood (1996) 87:2345–53.
66. Altuvia Y, Margalit H. Sequence signals for generation of antigenic peptides by the proteasome: implications for proteasomal cleavage mechanism. J Mol Biol. (2000) 295:879–90. doi: 10.1006/jmbi.1999.3392
67. Muranski P, Boni A, Wrzesinski C, Citrin DE, Rosenberg SA, Childs R, et al. Increased intensity lymphodepletion and adoptive immunotherapy–how far can we go? Nat Clin Pract Oncol. (2006) 3:668–81. doi: 10.1038/ncponc0666
68. Abboud R, Keller J, Slade M, DiPersio JF, Westervelt P, Rettig MP, et al. Severe cytokine-release syndrome after T cell-replete peripheral blood haploidentical donor transplantation is associated with poor survival and Anti-IL-6 therapy is safe and well tolerated. Biol Blood Marrow Transplant. (2016) 22:1851–60. doi: 10.1016/j.bbmt.2016.06.010
69. Kriecherbauer T, Marklof J, Soshnikov A. Random matrices and quantum chaos. Proc Natl Acad Sci USA. (2001) 98:10531–2. doi: 10.1073/pnas.191366198
70. Miyai M, Eikawa S, Hosoi A, Iino T, Matsushita H, Isobe M, et al. Detection and tracking of NY-ESO-1-specific CD8+ T cells by high-throughput T cell receptor β (TCRB) gene rearrangements sequencing in a peptide-vaccinated patient. PLoS ONE (2015) 10:e0136086. doi: 10.1371/journal.pone.0136086
71. Emerson RO, Mathew JM, Konieczna IM, Robins HS, Leventhal JR. Defining the alloreactive T cell repertoire using high-throughput sequencing of mixed lymphocyte reaction culture. PLoS ONE (2014) 9:e111943. doi: 10.1371/journal.pone.0111943
72. Salman A, Koparde V, Hall C, Jameson-Lee M, Roberts C, Serrano M, et al. Determining the quantitative principles of T cell response to antigenic disparity: the case of stem cell transplant donor-recipient exome wide mismatching and HLA bound alloreactive peptides. bioRxiv. (2018). doi: 10.1101/305474
Keywords: stem cell transplantation, GVHD, antigen response, HLA, tensors, vectors and operators, matrices, T cell response
Citation: Salman A, Koparde V, Hall CE, Jameson-Lee M, Roberts C, Serrano M, AbdulRazzaq B, Meier J, Kennedy C, Manjili MH, Spellman SR, Wijesinghe D, Hashmi S, Buck G, Qayyum R, Neale M, Reed J and Toor AA (2018) Determining the Quantitative Principles of T Cell Response to Antigenic Disparity in Stem Cell Transplantation. Front. Immunol. 9:2284. doi: 10.3389/fimmu.2018.02284
Received: 31 May 2018; Accepted: 14 September 2018;
Published: 11 October 2018.
Edited by:
Aurore Saudemont, GlaxoSmithKline, United KingdomReviewed by:
Pietro Crivello, Universitätsklinikum Essen, GermanyLuca Vago, San Raffaele Scientific Institute (IRCCS), Italy
Copyright © 2018 Salman, Koparde, Hall, Jameson-Lee, Roberts, Serrano, AbdulRazzaq, Meier, Kennedy, Manjili, Spellman, Wijesinghe, Hashmi, Buck, Qayyum, Neale, Reed and Toor. This is an open-access article distributed under the terms of the Creative Commons Attribution License (CC BY). The use, distribution or reproduction in other forums is permitted, provided the original author(s) and the copyright owner(s) are credited and that the original publication in this journal is cited, in accordance with accepted academic practice. No use, distribution or reproduction is permitted which does not comply with these terms.
*Correspondence: Amir A. Toor, YW1pci50b29yQHZjdWhlYWx0aC5vcmc=