- 1Instituto René Rachou, Fundação Oswaldo Cruz - FIOCRUZ, Belo Horizonte, Brazil
- 2Novo Nordisk Foundation Center for Protein Research, Faculty of Health and Medical Sciences, University of Copenhagen, Copenhagen, Denmark
- 3Environmental Genomics, Instituto Tecnológico Vale, Belém, Brazil
The study of molecular host–parasite interactions is essential to understand parasitic infection and adaptation within the host system. As well, prevention and treatment of infectious diseases require a clear understanding of the molecular crosstalk between parasites and their hosts. Yet, large-scale experimental identification of host–parasite molecular interactions remains challenging, and the use of computational predictions becomes then necessary. Here, we propose a computational integrative approach to predict host—parasite protein—protein interaction (PPI) networks resulting from the human infection by 15 different eukaryotic parasites. We used an orthology-based approach to transfer high-confidence intraspecies interactions obtained from the STRING database to the corresponding interspecies homolog protein pairs in the host–parasite system. Our approach uses either the parasites predicted secretome and membrane proteins, or only the secretome, depending on whether they are uni- or multi-cellular, respectively, to reduce the number of false predictions. Moreover, the host proteome is filtered for proteins expressed in selected cellular localizations and tissues supporting the parasite growth. We evaluated the inferred interactions by analyzing the enriched biological processes and pathways in the predicted networks and their association with known parasitic invasion and evasion mechanisms. The resulting PPI networks were compared across parasites to identify common mechanisms that may define a global pathogenic hallmark. We also provided a study case focusing on a closer examination of the human–S. mansoni predicted interactome, detecting central proteins that have relevant roles in the human–S. mansoni network, and identifying tissue-specific interactions with key roles in the life cycle of the parasite. The predicted PPI networks can be visualized and downloaded at http://orthohpi.jensenlab.org.
Introduction
Parasites are responsible for many diseases that result in millions of deaths each year. For instance, the World Health Organization published data in 2016 estimating that Plasmodium falciparum alone was responsible for around 214 million malaria cases, and 438,000 deaths worldwide (1). As well, around 7 million people worldwide were reported to be infected with Trypanosoma cruzi, which causes Chagas disease that results in life-long morbidity and disability and more than 7,000 deaths per year (1). Another highly prevalent disease, Leishmaniasis accounts for 20 to 30 thousand deaths a year and is caused by protozoan parasites of the Leishmania genus (1). Similarly, Schistosomiasis, a neglected parasitic disease of high relevance in this work, is mainly caused by five species of the genus Schistosoma. The disease has an estimated prevalence of 200 million cases worldwide (2). The available treatment for schistosomiasis is limited, and the development of resistance is a concern. Thus, there is an urgent need to develop novel drugs or vaccines.
The development of vaccines or treatments has been impeded by the lack of understanding of the parasites infection and survival mechanisms. Typically, parasites have complex life cycles with several morphological stages and infect distinct host cell types and tissues. For that, parasites display a resourceful capacity to live in different environmental conditions (intra and extracellular parasites) and also resist the immunological response of hosts (3). For example, extracellular parasites remodel tissues to migrate and evade the immune system (4). Similarly, intracellular parasites shape cellular processes and remodel host cells to adjust their niche during infection (5). The manipulation of these processes and pathways happens through molecular interactions that parasites use to their advantage.
The study of molecular host–parasite interactions is essential to understand parasitic infection, local adaptation within the host, and pathogenesis. These complex interactions can be described as a network (6). Pathogens affect their hosts partly by interacting with host proteins, which defines a molecular interplay between the parasite survival mechanisms and the host's defense and metabolic systems (7). Understanding this molecular crosstalk can provide insights into specific interactions that could be targeted to avoid the pathological outcomes resulting from the parasitism (8).
Intra-species protein–protein interactions (PPIs) have been studied in depth and there exist large datasets containing experimentally or computationally predicted interactions (9, 10). However, the number of available datasets providing host–pathogen PPIs is limited and challenged by the intrinsic difficulties of analyzing simultaneously both host and pathogen systems in high-throughput experiments (11). Thus, host–pathogen PPIs have mainly been predicted computationally using distinct strategies such as approaches based on sequence (8, 12–15), structure (16, 17), and gene expression (18). Homology-based prediction is one of the most common approaches to predict host–pathogen PPIs. These approaches have been extensively used to infer intra-species interactions (10, 14, 19–22) as well as host–pathogen PPIs (13, 15, 17, 23) based on the assumption that interactions between proteins in one species can be transferred to homolog proteins in another species (interologs).
In this work, we have followed a similar prediction strategy to identify common and specific mechanisms of parasitic infection and survival across 15 human parasites, namely Trypanosoma brucei, Trypanosoma cruzi, Trichinella spiralis, Schistosoma mansoni, Giardia lamblia, Plasmodium falciparum, Plasmodium vivax, Plasmodium knowlesi, Cryptosporidium hominis, Cryptosporidium parvum, Toxoplasma gondii, Leishmania braziliensis, Leishmania mexicana, Leishmania donovani, and Leishmania infantum. Our computational prediction approach is based on orthology transfer. However, we have constrained the method by (1) incorporating only high-confidence intra-species interactions, and interactions mined from the scientific literature (10), (2) using fined-grained orthology assignments instead of simple sequence similarity, and (3) including parasite-specific biological context such as lifestyle (uni- or multi-cellular) and tissue infection.
The objective of these constraints was to reduce the number of falsely predicted interactions, increase reliability, and thereby provide a better understanding of the parasites' molecular mechanisms of interaction with the host. The evaluation of the predicted host–parasite PPIs requires repositories of high-throughput experimental data that are not yet existent. However, we present here extended literature references supporting some of the predicted interactions relevant in the host–parasite molecular crosstalk. We also evaluated the predicted PPIs based on the functional relevance by identifying significantly enriched processes annotated in the human proteins predicted to interact with parasites. Finally, we propose that this approach can be applied to any host–pathogen system to predict relevant molecular interactions and define the context in which they unfold.
Materials and Methods
Proteome Filtering: Adding Parasite-Specific Biological Context
The first step in the prediction pipeline is to filter both the host and parasite proteomes according to the specific characteristics of the studied parasite (Figure 1A). All the human eukaryotic parasites (18 parasites) available in the STRING database were included in our analysis. Firstly, for the interaction to happen, proteins in the parasite need to be secreted or membrane proteins depending on the type of parasite (unicellular or multicellular). When analyzing parasites such as helminths (multicellular and extracellular) we used the soluble secretome. Conversely, in the case of unicellular parasites, we used both the soluble secretome and membrane proteins.
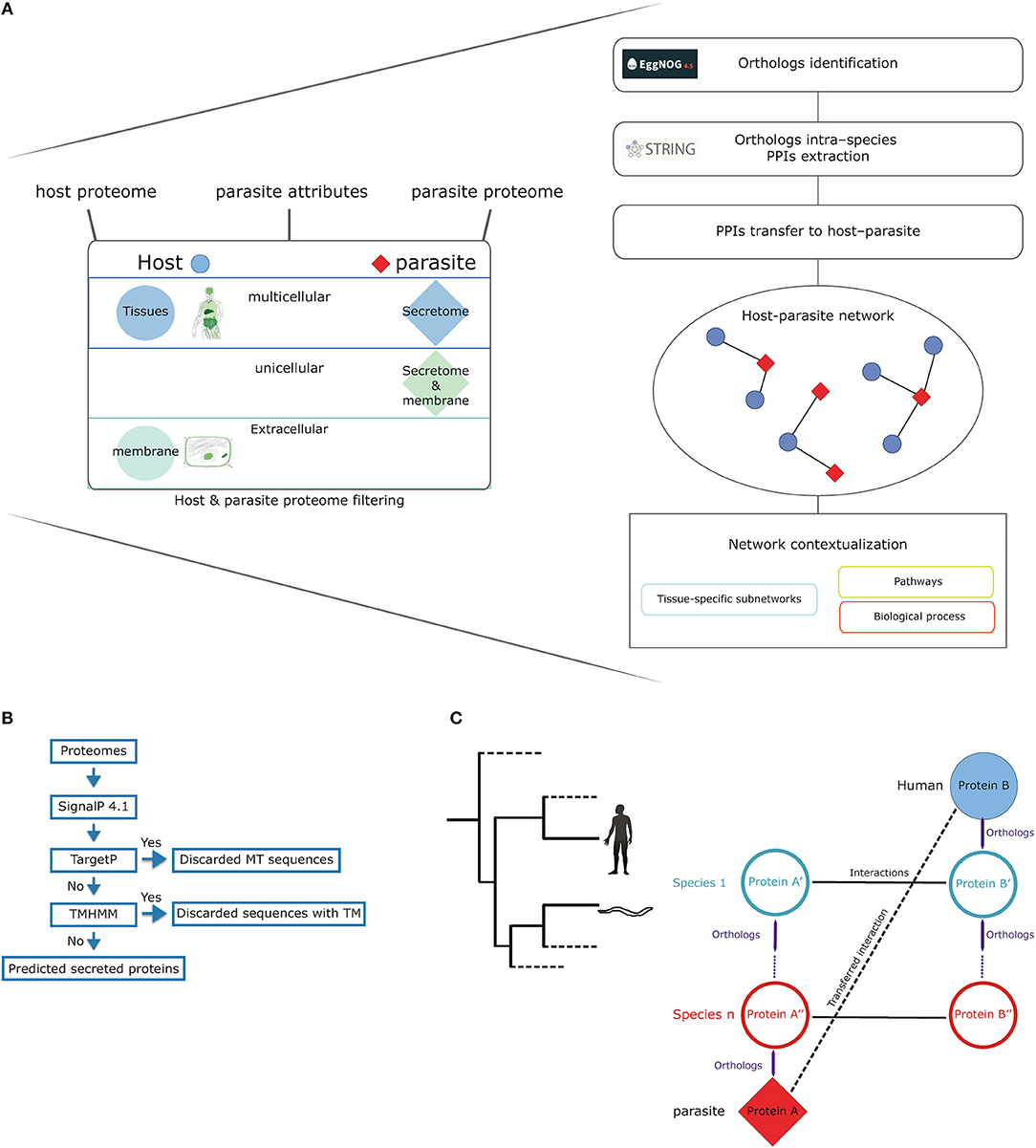
Figure 1. Method workflow. (A) The method input consists of the host and parasite proteomes, parasite attributes (uni- or multicellular), and tropism (tissues). Our approach filters the host proteome according to the specified list of tissues using TISSUES database and limits it to only cellular membrane and extracellular proteins extracted from the COMPARTMENTS database. The parasite proteome is filtered to include membrane and secreted proteins (unicellular parasites) or only secreted proteins (multicellular parasites). The next step in the pipeline uses the eggNOG database to identify orthologous proteins for both the filtered proteomes of both the host and the parasite. The workflow continues with the extraction of intra-species protein–protein interactions from STRING database for all the orthologous proteins. These intra-species interactions are then transferred to the host–parasite system as long as the interacting proteins have an orthologous host and parasite proteins. (B) Workflow for soluble secretome prediction in parasites (MT, mitochondrial; TM, transmembrane; TMHMM, transmembrane hidden markov model). (C) A simple scheme of how the orthology transfer is implemented.
To identify soluble and membrane proteins in parasites, we used different available bioinformatics tools to predict subcellular localization (Figure 1B). SignalP (24) was used to identify classically secreted proteins that were then scanned for the presence of mitochondrial sequences using TargetP (25) and transmembrane helices by the transmembrane identification based on hidden Markov model (TMHMM) method (26). We filtered host proteins to consider only those located on the cellular membrane and extracellular space by using the COMPARTMENTS database, which provides high-confidence information on cellular localization of proteins (confidence score >3) (27).
Additionally, we included context on the specific tissue tropism of the studied parasite by limiting the predictions to host proteins expressed in tissues relevant in the parasite's life cycle, since parasites need to migrate through distinct host tissues to complete their life cycles. Tissue tropism information for each parasite was based in information available on https://www.cdc.gov/parasites/. For this purpose, we used the TISSUES database (28), which provides protein profiles of tissue expression. Protein-tissue associations in this database are also scored in a similar way to the COMPARTMENTS database, which allowed us to use only high-confidence associations (confidence score >3).
The reason for choosing these specific databases is, that they have been designed to work well together. In addition to the wide range of evidence types considered and the consistent scoring schemes, the protein identifiers are synchronized across all of these databases (COMPARTMENTS, TISSUES, eggNOG, and STRING), which enables a smooth integration and a dynamic recovery of orthologs, mapped tissues, and compartments.
Orthologs Identification and PPIs Transfer
Host–parasite PPIs were predicted using orthology-based transfer. This approach relies on cross-species data integration to predict inter-species protein–protein interactions. Conserved intra-species interactions from multiple organisms, namely interologs, are transferred to the host–parasite system when orthologous proteins exist in these species. For example, an intra-species interaction between protein A and protein B is transferred if the host and the parasite have orthologs to A and B, respectively (Figure 1C).
To obtain intra-species PPIs, we used the STRING database (10). STRING provides PPIs from a variety of sources and evidence types, each with associated confidence scores, which allows us to expand the list of high-confidence PPIs beyond known physical interactions. The interaction file used contains confidence scores for the individual evidence channels (Neighborhood, Gene fusion, Co–occurrence, Co–expression, Experiments, Databases, and Text-mining), which are further subdivided into direct and transferred evidence. The transferred scores come from the orthology transfer performed by the STRING database itself (interologs); we excluded these to only include direct evidences. Consequently, we needed to recalculate the combined score following how scores are combined within the STRING database (Equation 1).
where i is the different evidence channels (Neighborhood, Gene Fusion, Co–occurrence, Co–expression, Experiments, Databases, and Text-mining) and p is the prior probability of two proteins being linked, which is the same value as the one used in the STRING database (p = 0.063). The recalculated score was then used to filter for only high-confidence interactions (scorecombined > 0.7).
For each of the interactions from STRING, we used the fine-grained orthologs functionality derived from phylogenetic analysis in eggNOG database (29) to identify orthologous proteins in human and in the parasites. We transferred an interaction as long as it involved proteins that had an orthologous protein in the parasite and another one in human among the ones retained in the filtered proteomes. Several metrics were collected to facilitate the analyses of the predicted interactions: maximum confidence score transferred, maximum confidence score transferred from the Experiments channel in STRING, the species from which the interactions were transferred, and the eggNOG non-supervised orthologous groups (NOGs) the proteins belong to.
Domain-Domain and Linear Motif-Domain Annotations
To know which of the predicted interactions may be physical rather than only functional associations we annotated our interaction predictions with domain–domain interaction predictions from iPfam (30) and 3did (31), and linear motif–domain interactions from ELM database (32). These databases provide predictions based on structural information from the Protein Data Bank (33). Human and parasites protein domains were predicted using Pfam scan, which combines the HMMER tool (34), and the domain models from Pfam version 31 (35). Linear motif–domain interactions are predicted using the regular expressions provided in the ELM database. We decided that a protein-protein interaction is supported by domain-domain and/or linear motif-domain interactions whether the interacting domains or linear motif-domain interactions reported by the databases (iPfam, 3did, ELM) appeared in the predicted host-parasite interaction. These data are available in the web resource: https://orthohpi.jensenlab.org/ the tab separated files (tsv) downloadable in the web contain a column (#11) indicating which of the predicted interactions are supported by interacting domains or domain-motif pairs.
Network Analysis
Once we obtained the predicted host–parasite PPI networks, we used the topology of the network to identify relevant proteins that may play critical roles in the host–parasite crosstalk. There are several centrality measures that can be used to reveal node importance based on different node attributes such as degree. These different measures correlate to some extent and may highlight other nodes (36). Here, we used betweenness centrality to pinpoint proteins whose targeting would most disrupt this communication (37) but provide the code to generate several correlation measures (Supplementary File 1) with the provided networks in OrthoHPI website (http://orthohpi.jensenlab.org).
To identify key biological processes enriched in the predicted host–parasite PPI networks, we performed functional enrichment analysis using Gene Ontology terms (biological processes) (38) and Reactome pathway annotations (39). The lack of existing annotations in the parasite species limited the analysis to only the human proteins predicted to interact. This analysis revealed common and specific biological processes and pathways targeted by parasites. The functional enrichment was performed using Fisher's exact test and correction for multiple testing (Benjamini–Hochberg; FDR < 0.05). The enrichment was calculated using as background only the filtered proteome.
To overcome the lack of functional characterization of the studied parasites, we also investigated the functional classification of COGs (40) provided by the eggNOG database (29). These annotations classify COGs into broad functional categories that can be used to characterize the proteins grouped in these clusters (Supplementary Figure 1). These categories were transferred to the parasite proteins in the network and when the category was “Function unknown” we assigned as putative functions the categories of their interaction partners in human (Supplementary Table 1).
Web Interface
To provide access to the predictions generated by our approach, we developed a web interface for OrthoHPI (http://orthohpi.jensenlab.org/). This web site provides interactive, predicted host–parasite PPI networks, which are visualized with d3.js (https://d3js.org/) and allow the user to easily navigate the full networks as well as the tissue-specific ones. The nodes in these networks represent parasite and human proteins and their sizes correspond to their betweenness centrality in the network. The edges between nodes show predicted molecular host–parasite interactions and are weighted using the maximum score transferred from STRING database. The predictions can be downloaded in tab-separated values file format or in Graph Modeling Language format, which is compatible with Cytoscape (41).
Results
Computational Prediction of 15 Host–Parasite Interactomes
We applied our integrative orthology-based approach to predict the host–parasite PPIs networks for 18 different eukaryotic parasites and obtained predicted PPIs for 15 of them (Table 1 and Figure 1). PPIs for Entamoeba histolytica, Trichomonas vaginalis, and Leishmania major could not be predicted due to the lack of orthologs. Our predictions returned a total of 27,352 interactions for 14,340 proteins (12,218 host proteins, 2,122 parasite proteins) being T. gondii and C. hominis the largest and the smallest predicted PPIs networks, respectively (Table 2). The large differences in the number of proteins in parasites and host after filtering process depend on whether the parasite is unicellular (filtering process included membrane and secreted proteins) or multicellular (filtering process included only secreted proteins) and in the number of human tissues related with the parasites tropism (Table 2). Our prediction approach transferred most of the STRING high-confidence intra-species interactions from model organisms (M. musculus, S. cerevisiae, D. melanogaster, D. discoideum, etc.) and Homo sapiens (Supplementary Figure 2).
The resulting host–parasite PPIs networks were analyzed to identify central proteins in the networks, tissue-specific connections, and enriched biological processes and pathways. The lack of experimentally validated host–parasite interactions that could be used as a gold standard prevented direct validation of the quality of the predicted interactions. Instead, we evaluated the plausibility of the network by looking at the known functions in which the involved proteins participate. The analyses are divided into two: a global study of the common mechanisms targeted by the studied parasites and the shared human interactors. We also provide a study case focusing on a closer examination of the human–S. mansoni predicted interactome.
Common and Specific Mechanisms Targeted by the Studied Parasites
We used annotations from both biological processes GO terms and Reactome pathways in human to get an overview of the shared pathways targeted by the studied parasites (Figure 2A). In total, 1,910 GO terms (biological process) were identified in human proteins targeted by parasites across all the interactomes (Supplementary Table 2). In the analysis, we found the biological process Protein folding enriched across 14 interactomes (Figure 2A). This biological process has been identified already as enriched and crucial in several host–pathogen systems, including mouse–P. falciparum (18); human–M. tuberculosis (42); B. glabrata–S. mansoni (43); L. salmonis–F. margolisi (44), based on transcriptomics and proteomics data. The protein folding biological process appears to be a conserved natural response to the infection and is related to a response to stress.
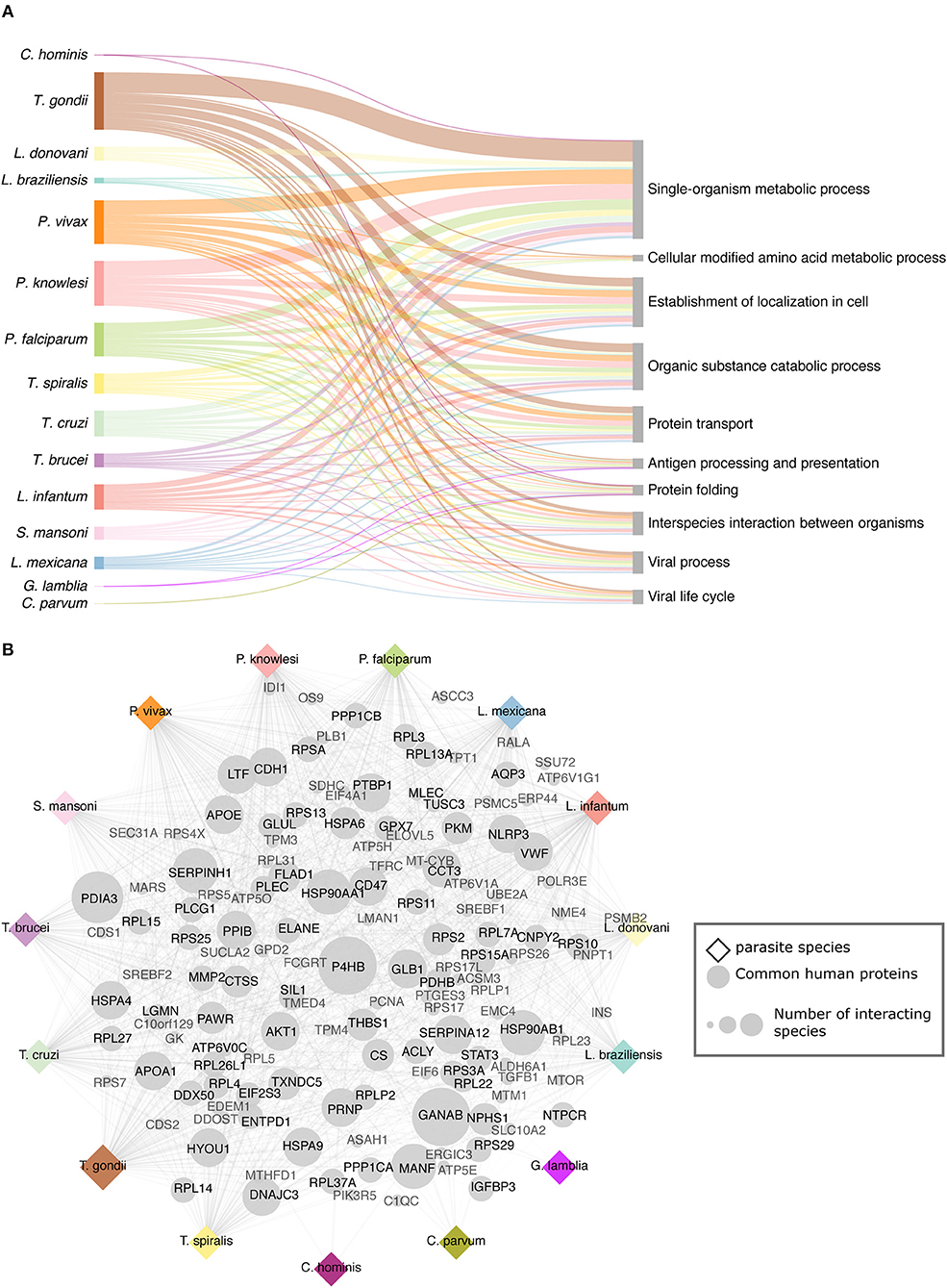
Figure 2. Targeted host processes and proteins. (A) Common and most relevant human biological processes (GO BP) targeted by the parasites. (B) Common human proteins across interactomes some of which have been already linked to pathogenic activity. Both the targeted processes and pathways and these common host interacting proteins may open new therapeutic possibilities.
Different lifestyles (intra- and extracellular parasites) may define specific targeted pathways in the host. We analyzed the common processes and pathways enriched in intracellular and extracellular parasites specifically. The human GO terms and pathways enrichment analysis on networks for the extracellular parasites did not suggest any lifestyle-specific targeted mechanisms. However, the specific lifestyle requirements of the intracellular parasites revealed two (thioester and acyl-CoA metabolic process) exclusive biological processes related to exploitative mechanisms to acquire nutrients from the cytosol of host cells (i.e., de novo lipid synthesis) carrying out a type of sustainability interactions. In terms of host immune response to parasitic infection we obtained a Reactome pathway common to intracellular parasites: antigen presentation: folding, assembly, and peptide loading of class I MHC. This pathway belongs to the human innate immune defense by acting as pattern-recognition receptors, in particular against intracellular pathogens.
The host–parasite interactome of the intracellular P. falciparum has been to some extent studied both experimentally and computationally (13, 17, 23, 45–47). In order to establish a successful infection, P. falciparum proteins interact with a variety of human proteins on the surface of different cell types, as well as with proteins inside the host cells (47). For instance, the parasitophorous vacuole protein called ETRAMP5 was found to interact with the human apoliproteins ApoA, ApoB, and ApoE, which may be involved in the invasion of liver cells by sporozoites (47). Alternatively, this interaction may be important for parasite survival inside red blood cells or hepatocytes (47). Similarly, our results predict the interaction of human APOB with P. falciparum endoplasmin (PFL1070C) and phospholipase (PFF1420w) both parasitophorous vacuole proteins. These interactions were also predicted in liver tissue, which may be related to the parasitophorous vacuole function.
Cell signaling and cell adhesion have also been identified as a relevant biological processes targeted in the interaction between human and P. falciparum proteins. For instance, the parasite chaperone PFI0875w was found to interact with many prominent regulation and signaling host proteins such as members of the TNF pathway (13). Our results predicted the interaction between PFI0875w and human HSP70 protein, which promotes TNF-mediated apoptosis. Similarly, cell adhesion is crucial for host cell invasion and has been shown previously in receptor-mediated viral infections. For example, human protein CD55 (complement decay-accelerating factor) was related to infection by coxsackievirus (23). In P. falciparum protein TRAP (PFC0640w) was found to interact with leucine-rich proteins involved in cell adhesion (13). In our study, the same protein TRAP and a reticulocyte binding protein (PFD0110w) were predicted to interact with receptor proteins involved as well in viral infection like integrins and may play a role in the initial process of cell invasion by P. falciparum (48).
Across the Leishmania species, we identified a genus-specific biological processes related to lipid and fatty acid metabolism. In species that cause mucocutaneous leishmaniasis (L. braziliensis, L. mexicana), we found nine human specific processes, interestingly many of them related to cellular oxygen levels such as: cellular response to decreased oxygen levels, cellular response to oxygen levels, negative regulation of cellular respiration, and cellular response to hypoxia. Regarding visceral leishmaniasis (L. donovani, L. infantum) we found 40 specific GO terms associated with a relevant biological process in the host–parasite interaction such as regulation of defense response to virus by virus and receptor-mediated endocytosis.
In helminths (S. mansoni, and T. spiralis) we identified 24 specific biological processes, some of which were involved in specific process related to blood tissue, for example, platelet degranulation and blood vessel development. Several helminth parasites imbibe host blood, including hookworms, the flukes, and the major livestock nematode parasites (50). Helminth migration through different organs requires the degradation of the extracellular matrix and the disruption of cell junctions by some secreted proteolytic enzymes, causing damage along the path of the migration (51). According to our results, we found some GO terms enriched probably related to helminth migration such as extracellular matrix disassembly and regulation of cell adhesion to be enriched.
We studied the common host proteins to all or most of the studied parasites (Figure 2B). In all the inferred interactomes, GANAB (neutral alpha glucosidase AB) and P4HB (protein disulfide isomerase) proteins were predicted to interact with parasite proteins. GANAB protein is related to the host defense mechanisms and P4HB is relevant in the internalization of broad spectrum of pathogens such as Leishmania, HIV, dengue virus, and rotavirus.
The Human–S. mansoni Interactome Reveals Relevant Central Proteins
We identified 695 interactions (491 host proteins, 74 S. mansoni proteins), and 178 were supported by domain-domain and domain-linear motif interactions (https://orthohpi.jensenlab.org/). In this analysis, we use the topological characteristics of the predicted human–S. mansoni PPI network to identify central proteins. Network centrality helped to prioritize proteins by identifying nodes with a relevant role in the communication flow in the network, which may translate into biological relevant essentiality (52, 53). In the human–S. mansoni interactome network (Figure 3) the nodes with the highest centrality are shown in Table 3 and Figure 3. Within the Gene Ontology biological process, we found 325 gene ontology terms enriched in S. mansoni targets in the host (Supplementary Table 2). GO terms associated with human proteins involved with host–parasite interaction were also retrieved (Table 4). PPIs for each term are available in Supplementary Table 3.
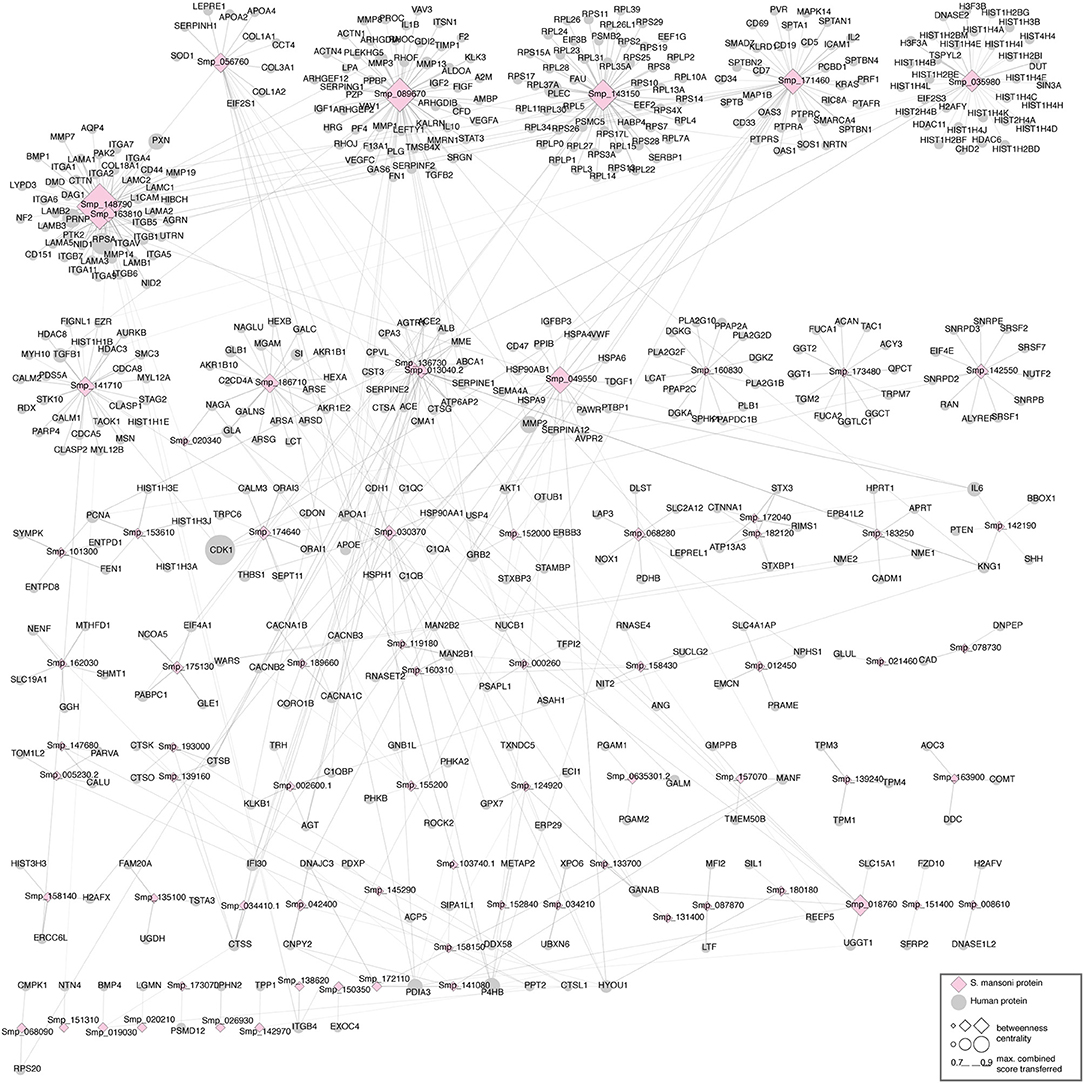
Figure 3. Human–S. mansoni predicted interactome. The layout of the nodes in the network (ellipses: human proteins, diamonds: S. mansoni proteins) uses Markov Clustering to group and locate the nodes. The tools used to visualize the networks in this article are: Cytoscape (41) and the clusterMaker app (49). The central nodes are calculated using betweenness centrality and highlighted in the figure with a higher size.
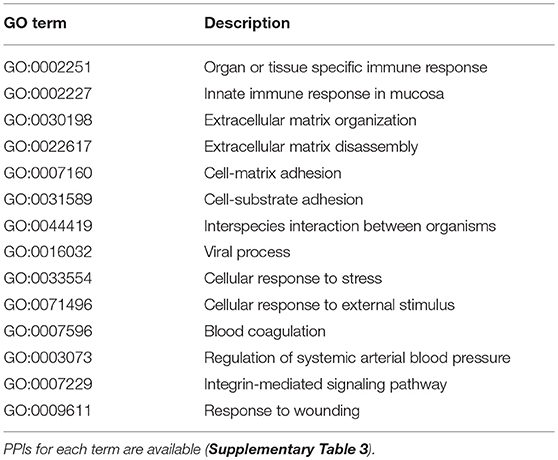
Table 4. GO term enrichment analysis of the most relevant biological process in the H. sapiens—S. mansoni interactome.
The human–S. mansoni interaction network is not static and the tissue expression data used to filter the host proteome can also be used to give context to the predicted molecular associations. Modeling spatial context into the predicted network allowed the identification of relevant interactions associated to tissues through the parasite's life cycle. 1,096 interactions were identified in five tissues related with the parasite tropism (Intestine 114, lung 191, blood 195, liver 443, skin 153) (see https://orthohpi.jensenlab.org/) (Table 5). Top 10 of highest centrality proteins were also identified in every tissue network (Table 5) and two central proteins were conserved across five tissues: Smp_089670 (Alpha-2 macroglobulin) and Smp_171460 (Cell adhesion molecule).
Tissue-specific interactions are relevant to know the specificities in different relevant tissues related to S. mansoni lifecycle. In total, we found 375 tissue-specific interactions (skin 44, lung 66, liver 188, intestine 40, blood 37) (Figure 4) (Supplementary Table 4). Eight interactions were conserved across tissues (blood, intestine, liver, lung, skin) (Supplementary Table 4). Four S. mansoni proteins (Smp_089670, Smp_018760, Smp_049550, Smp_056760), which were part of the eight conserved interactions across tissues are central proteins in the whole interactome human–S. mansoni. P4HB a common targeted host protein across the 15 interactomes appeared in six of the eight interactions conserved across five tissues.
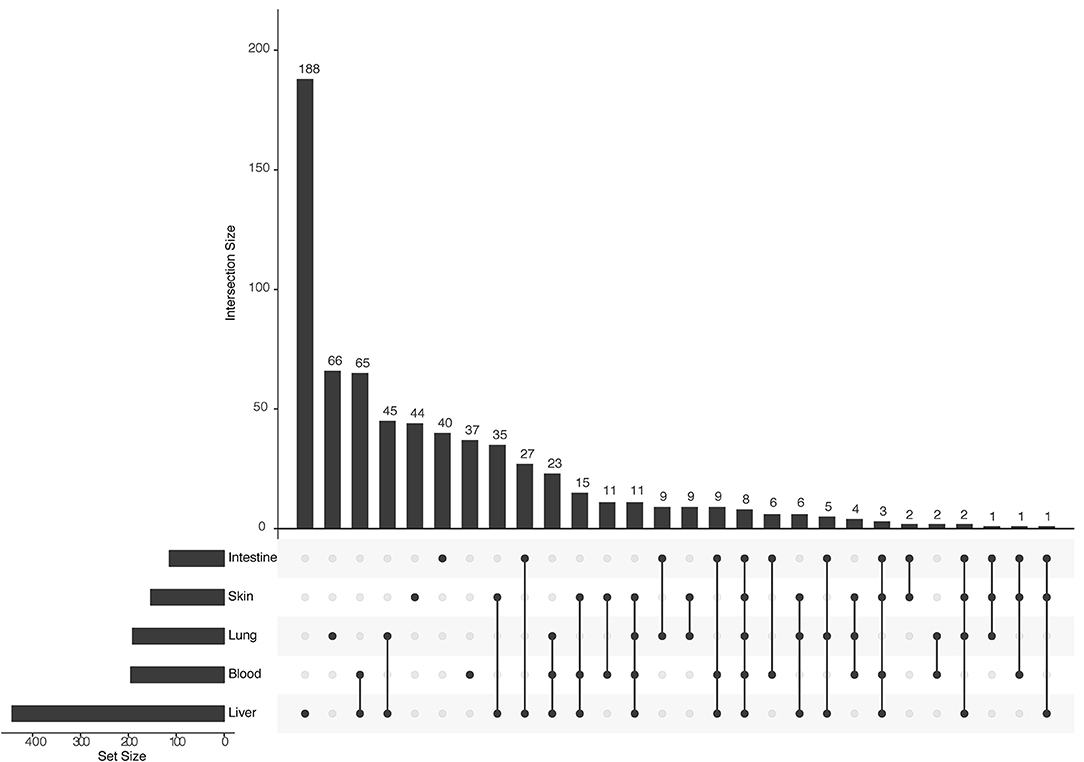
Figure 4. Upset plot of tissue-specific interactions in the human–S. mansoni predicted interactome. The bar chart on the left indicates the total of interactions in each of the five tissues associated with S. mansoni tropism: Liver is the tissue with the most interactions (443 interactions). The upper bar chart indicates the intersection size of shared interactions across tissues and tissue-specific interactions. Blood and Liver are the tissues with more shared interactions (65 interactions) and liver is the tissue with the most tissue-specific interactions (188 interactions).
The OrthoHPI Web Resource
We developed a web resource (http://orthohpi.jensenlab.org) to provide all the predicted interactomes for all the studied parasites (Figure 5). The aim of OrthoHPI is to facilitate the analysis of the predicted host–parasite PPI networks, provide easy access to the full and tissue-specific networks and produce visual, navigable, and interactive networks easily manipulated by users.
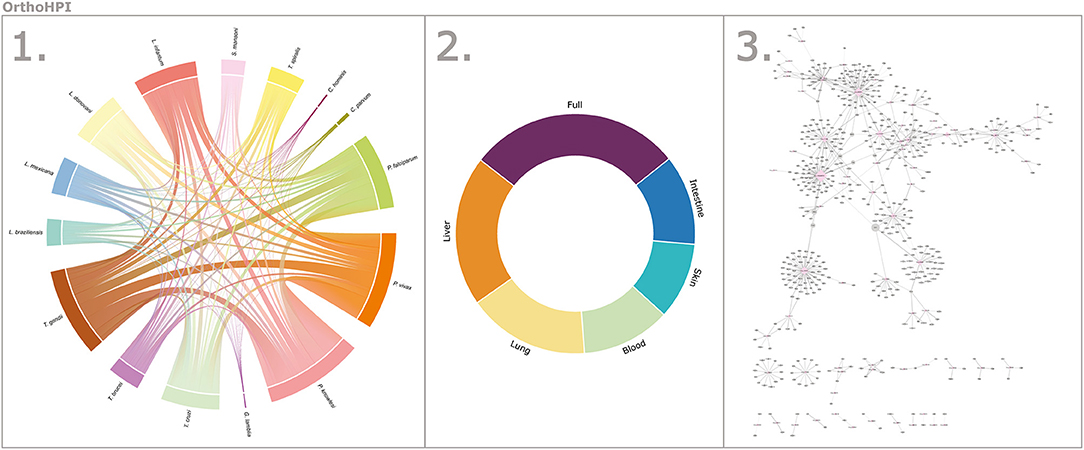
Figure 5. The OrthoHPI web resource. The website (http://orthohpi.jensenlab.org) allows you to visualize the predicted interactomes for all the studied parasites in three simple steps. (1) Select parasite, (2) Choose to visualize the full network or a tissue–associated interactions, and (3) Visualize the interactive network and download the interactions.
Discussion
The efficacy of treatments for parasitic diseases is still limited, and in many cases, parasites develop resistance. Thus, there is an urgent need to discover novel drugs or vaccines for these neglected diseases. However, our understanding of host–parasite molecular crosstalk is still very limited, as only a few systematic experimental studies have so far been performed. Being this the main reason to predict PPIs using computational approaches.
Here, we predicted host–parasite PPI networks for 15 diverse parasitic species and studied the functional relevance of these interactions by analyzing enriched processes among the human proteins predicted to interact with parasite proteins. The different enrichment analyses allowed us to compare and identify commonalities and specificities across the studied parasites. Additionally, these analyses revealed biological processes and metabolic pathways previously described in the literature as being affected during parasitic infection, which helped to validate functionally the predicted interactions. In the case of the most studied parasite from our list, namely P. falciparum, we were able to compare the list of GO terms enriched in the human-P. falciparum predicted PPI network with an already published functional study (13). In this study, the authors report a list of GO biological processes in human that are most affected by the interaction with P. falciparum (100 GO terms). From this list, 51 terms were in common with our results. The overlapping terms were related mainly with intracellular process in general such as to intracellular transport, signal transduction, cell cycle, actin filament-based process among others. The remaining non-overlapping 49 terms were related with more specific biological process targeted by P. falciparum such as cell death, apoptosis, intracellular protein kinase cascade among other (Supplementary Table 5).
The 15 parasites included in our study are different regarding phylogeny and biology, for example, distinct forms of invasion and the invasion of different types of host tissues (Table 1). Nonetheless, when combining all the predicted PPI networks, we were able to identify common core pathways and biological processes, which were targeted in the host by all the studied parasites. Indeed, it has been previously shown that evolutionarily distinct parasites can target the same pathways as a result of convergent evolution, for example in Arabidopsis pathogens, such as the bacterium Pseudomonas syringae and the eukaryote Hyaloperonospora arabidopsidis (54).
Eukaryotic pathogens are able to synthesize a number of nutrients required for de novo growth; however, it is more advantageous for them to conserve energy and take host derived resources (55, 56). The enriched processes in intracellular parasites are related to the acquisition of host lipids necessary for the parasites to assemble a large amount of new membranes during replication within host cells. The uptake of host lipids may be associated with how Leishmania species deplete membrane cholesterol and disrupt lipid rafts in host macrophages during the invasion process (57).
Concerning the host immune response to parasitic infection we found one Reactome pathway that is common to intracellular parasites: antigen presentation, folding, assembly, and peptide loading of class I MHC. This suggests that innate sensing of parasites is important for the induction of pro-inflammatory responses aimed at controlling infection (56, 58) and belong to the innate immune defense by acting as pattern-recognition receptors, in particular against intracellular pathogens (59) and parasites as the analyzed in this work (60).
In mucocutaneous leishmaniasis, the enriched process make sense, since in skin infected wounds, low oxygen tensions (hypoxic conditions) prevail, whereas oxygen supply promotes wound healing, and helps to control infections (61). Hypoxia can have multiple effects on host–pathogen interactions, many intracellular pathogens successfully adapt to hypoxic conditions and according to our results, the enriched process are showing a kind of response from the host based on the proteins that are part of these enriched biological process.
For intracellular parasites, the first challenge during infection is to gain access to the intracellular environment. Cell invasion starts when parasites contact the surface of the host cell and cellular receptors mediate parasite internalization (62). Consistent with this, we found the GO term receptor-mediated endocytosis to be significantly enriched in the PPI networks for Leishmania parasites.
Some parasites, such as schistosomes, are in constant contact with blood as they inhabit the host's veins. Blood coagulation is triggered by several pathways, which are targeted by blood-feeding parasites to inhibit coagulation and prolong blood flow (50). In helminths, we found specific biological process (blood vessel development and platelet degranulation) related to the inhibition of blood coagulation, which is also related to proteinases that facilitate the invasion of host tissues and digest host proteins. Additionally, parasite proteinases help pathogens evade the host immune response and prevent blood coagulation (63). Helminths-specific enriched Reactome pathways targeted two host mechanisms, sphingolipid metabolism, and glycosphingolipid metabolism, which are known to have a key role in the interaction host–helminths.
In addition to metabolic pathways and GO terms shared by parasites, certain host proteins targeted by parasites were also recurring across interactomes. One of them is protein disulfide isomerase (P4HB), which plays a key role in the internalization of certain pathogens (64). For example, during the host invasion process of L. chagasi increased levels of P4HB were found to induced phagocytosis in the promastigote phase and inhibition of expression of this gene reduced phagocytosis (65). The role of P4HB has also been associated with other pathogens such as HIV, dengue virus, or rotavirus (66–68). In dengue virus, P4HB was linked to a reduction of β1 and β3 integrins allowing for the entry of the virus (68) and in MA104 cells, thiol blockers, and P4HB inhibitors decreased the entry of rotavirus (66). The other recurring human protein is GANAB, which is related to the alteration of eosinophil proteome (69). Strikingly, eosinophils are important mediators of allergies, asthma, and adverse drug reactions, and are also related to the host defense mechanisms against helminth infections, which are characterized by eosinophilia (70–72). Straub et al. (69) conducted a comparative proteomic analysis of eosinophils (healthy vs. hypereosinophilia from acute fascioliasis), and GANAB was one of the four proteins significantly upregulated in the Fasciola-infected patient.
These results show that GANAB and P4HB are relevant proteins not only in our 15 interactomes but also in other host-pathogen systems, which confirm these proteins as possible hallmarks of the host–parasite interaction. Other host proteins that were not recurring across the 15 interactomes but common in other interactomes (Figure 2B) such as HYOU1, PDIA3, HSPA9, CTSS, etc., have been already also found deregulated upon pathogenic infection (73–78).
According to our network topology results, we chose betweenness centrality as a measure to predict central proteins in the human-S. mansoni interactome, there are multiple network centrality measures, and they do correlate to some degree (79). We have generated a correlation analysis between different centrality measures for human-S. mansoni interactome (Supplementary Figure 3). In this case, we chose betweenness centrality because we are interested in highlighting nodes that are central to information flow in the network, which may translate into more relevant nodes for the human-S. mansoni interactome. We identified central proteins in the human–S. mansoni PPI network (Table 3) involved in crucial biological processes needed for invasion and survival of the parasite: protease activity regulation, inhibition of blood coagulation, cell adhesion, and migration (80).
Our results predict that the S. mansoni protein Alpha-2 macroglobulin (Smp_089670) interacts with host proteins involved in extracellular matrix organization such as SERPINE1 or metalloproteases (MMP2, MMP3, MMP13, MMP8, and MMP1). Interactions with the extracellular matrix (ECM) components influence several biological processes and ultimately the fate of the host cell (81). Parasites encounter the ECM as a barrier and they deploy several mechanisms to overcome it such as cell adhesion, induction of ECM degradation, and regulation of the immune response (4, 82, 83). For example, Alpha-2 macroglobulin inhibits the predicted five metalloproteases by a trapping mechanism that limits their access to substrates (80). Laminin subunit beta 1 (Smp_148790) interacts with host protein PLEC, which strengthens cells and tissues by acting as a cross-linking element of the cytoskeleton (84). This interaction may compromise cell and tissue integrity (cell junction) and may be used by the parasite to migrate across different host tissues. The alteration of cell junction and tissue remodeling has been observed in some helminth parasite infections (85). The cell adhesion protein (Smp_171460) is also involved in migration and has interacting partners belonging to the metalloprotease, collagenase, and laminin families, according our results. Histone H2A, another central protein has been identified as part of the secretome in S. mansoni mainly from eggs (86). This protein has moonlight functions outside of the nucleus in parasites and has evolved additional functions in invasion and interaction with the host (87).
Among the central proteins, we found several S. mansoni immunoreactive proteins, namely protein disulphide-isomerase, heat shock protein 70, and eukaryotic translation elongation factor. These proteins were experimentally supported as immunoreactive in a study using adult worm protein extracts probed with pooled sera of infected and non-infected (naturally resistant) individuals from an S. mansoni endemic area (88). Neutral alpha-glucosidase, a central protein in the S. mansoni network, was also detected as immunoreactive in S. mekongii in mouse and patient sera (89). These results showed that probably central proteins are more related to the stimulation of the immune response that non-central proteins in the host–S. mansoni interactome. Functional significance of a protein is related to its position in the PPI network, as deletion of hub proteins (central proteins) have more impact than non-hubs in the interactome, explaining the function essentiality of the central nodes (52). In our case probably are relevant proteins to keep the host–parasite interaction since pathogens may have adapted to “attack” proteins involved in specific pathways, most importantly in immunity and defense mechanisms (53) as evidenced in this work.
Regarding tissue-specific network analysis, we found that in the blood-specific PPI network, there are H. sapiens proteins targeted by S. mansoni involved in biological processes such as response to stress, blood coagulation, regulation of immune process among others (Supplementary Table 4). Two interactions between alpha 2 macroglobulin (Smp_089670) and coagulation factors like platelet factor four (ENSP00000296029) and Kallikrein (ENSP00000314151), could result in inhibition of the coagulation factors, which may facilitate the migration of the parasite through the broken tissue/vessels (80). Equally compelling is the predicted lungs-specific interaction between the host protein FZD10 (ENSP00000229030), previously implicated in the inflammatory response in the alveolus (90), and the parasite protein Wnt (Smp_151400).
Here, we identified relevant PPIs in the human–S. mansoni interactome related with different stages of the host–parasite interaction such as: adhesion, invasion, feeding, migration, immune evasion, and interactions with specific environments like tissue-specific interactions. Thus, the reported interactions can be used as a starting point in follow up experiments that could lead to better understanding of the disease pathogenesis and the parasite's biology, as well as open new frontiers in the identification of novel therapeutic targets against neglected diseases.
Conclusion
In the present study, we provide 15 predicted host–parasite interactomes, a functional analysis of the main common and specific processes targeted by diverse parasites, and an in-depth analysis of the human–S. mansoni PPI network. As well, we highlighted biological processes, pathways, and tissue-specific interactions that may be essential in the life cycle of the parasites. Moreover, our results highlight mechanisms and specific components that may be candidates for the study of new druggable targets.
One of the advantages of our prediction method is that it combines an orthology method with biological context relevant in the parasite's life cycle, which provides higher quality by reducing noise. Further, we integrate high-quality information from well-known and benchmarked sources of orthology prediction, intra-species protein-protein interactions, and protein location. The approach is scalable, and can be applied to many different host–pathogen systems.
Our study could also be used to assign functions to parasite's hypothetical proteins, assuming that clustered proteins tend to have similar functions and functionally related proteins can interact with each other (91–93). This application would be useful in parasite genomics, considering that a large number of parasite proteins are annotated as hypothetical proteins, and our networks provides a useful resource for annotation of those proteins.
Despite the limitations of homology prediction methods that tend to yield a high number of false positives, our approach constrained the predictions by including parasite-specific biological context, which filtered out some of the interactions otherwise transferred by orthology but biologically inconsistent with the life cycle of the parasite. Unfortunately, the lack of experimental data limited the options to benchmark the accuracy of the method. However, we provide several evidence from scientific literature of known host-parasite protein-protein interactions and established biological processes targeted by the parasites, which we also predicted in this study. Hence, we believe that the predicted interactions provide a base for hypothesis generation and help to focus follow up experiments that can prove the interaction and also find new druggable targets (11). Additionally, the common and specific biological processes identified help to understand parasitic invasion, infection, and persistence in the host and thereby the biology of parasitic diseases.
Author Contributions
YC-A, AS, GO, and LJ conceived and designed the experiments. YC-A performed the analysis of the biological relevance of the PPIs and contributed to the development of the pipeline. AS developed the pipeline and the web resource. All authors participated in reviewing and editing the manuscript and approved the final manuscript.
Funding
This work was supported by grants to GO from the National Institutes of Health-NIH/Fogarty International Center (TW007012), GO is a CNPq fellow (307479/2016-1), and CAPES (REDE 21/2015 CAPES/MINCYT) to YC-A, EMBO short-term fellowship (400–2015) to YC-A. Work at The Novo Nordisk Foundation Center for Protein Research (CPR) is funded in part by a generous donation from the Novo Nordisk Foundation (Grant number NNF14CC0001).
Conflict of Interest Statement
The authors declare that the research was conducted in the absence of any commercial or financial relationships that could be construed as a potential conflict of interest.
Supplementary Material
The Supplementary Material for this article can be found online at: https://www.frontiersin.org/articles/10.3389/fimmu.2019.00212/full#supplementary-material
Supplementary File 1. Python script to analyze node centrality in the networks.
Supplementary Figure 1. Intraspecies eggNOG groups functional annotation transferred. Functions annotated in eggNOG to the most contributing clusters of orthologs groups (COGs) used in the orthology transfer method.
Supplementary Figure 2. Species that contributed the most to the PPI transfer. Most of the interologs transferred to the host–parasite system corresponds to model organisms, which account for most of the high confidence PPI in the STRING database.
Supplementary Figure 3. Correlation analysis between different centrality measures for human-S. mansoni interactome.
Supplementary Table 1. Annotation for parasites proteins with unknown function.
Supplementary Table 2. Human GO terms enriched targeted by 15 parasites. Biological process targeted by parasites across 15 interactomes. Numbers represent the proteins associated with each GO term.
Supplementary Table 3. PPIs of relevant human GO terms enriched in the human–S. mansoni interactome. These PPIs belong to enriched GO terms from Table 4.
Supplementary Table 4. Tissue-specific and shared interactions across five tissues in the human–S. mansoni interactome.
Supplementary Table 5. Comparison of human GO terms enriched targeted by P. falciparum. This comparison was made between results from Wuchty (13) and our results.
References
2. Adenowo AF, Oyinloye BE, Ogunyinka BI, Kappo AP. Impact of human schistosomiasis in sub-Saharan Africa. Braz J Infect Dis. (2015) 19:196–205. doi: 10.1016/j.bjid.2014.11.004
3. Cuesta-Astroz Y, Oliveira FS, de Nahum LA, Oliveira G. Helminth secretomes reflect different lifestyles and parasitized hosts. Int J Parasitol. (2017) 47:529–44. doi: 10.1016/j.ijpara.2017.01.007
4. Piña-Vázquez C, Reyes-López M, Ortíz-Estrada G, de la Garza M, Serrano-Luna J. Host-parasite interaction: parasite-derived and -induced proteases that degrade human extracellular matrix. J Parasitol Res. (2012) 2012:748206. doi: 10.1155/2012/748206
5. Nelson MM, Jones AR, Carmen JC, Sinai AP, Burchmore R, Wastling JM. Modulation of the host cell proteome by the intracellular apicomplexan parasite Toxoplasma gondii. Infect Immun. (2008) 76:828–44. doi: 10.1128/IAI.01115-07
6. Dallas T, Park AW, Drake JM. Predicting cryptic links in host-parasite networks. PLoS Comput Biol. (2017) 13:e1005557. doi: 10.1371/journal.pcbi.1005557
7. Salzet M, Capron A, Stefano GB, Capron A, Dessaint JP, Capron A, et al. Molecular crosstalk in host–parasite relationships: parasitol. Today (2000) 16:536–40. doi: 10.1016/S0169-4758(00)01787-7
8. Taylor CM, Fischer K, Abubucker S, Wang Z, Martin J, Jiang D, et al. Targeting protein-protein interactions for parasite control. PLoS ONE (2011) 6:e18381. doi: 10.1371/journal.pone.0018381
9. Stark C, Breitkreutz BJ, Chatr-Aryamontri A, Boucher L, Oughtred R, Livstone MS, et al. The BioGRID interaction database: 2011 update. Nucleic Acids Res. (2011) 39:D698–704. doi: 10.1093/nar/gkq1116
10. Szklarczyk D, Franceschini A, Wyder S, Forslund K, Heller D, Huerta-Cepas J, et al. STRING v10: protein-protein interaction networks, integrated over the tree of life. Nucleic Acids Res. (2015) 43:D447–52. doi: 10.1093/nar/gku1003
11. Cuesta-Astroz Y, Oliveira G. Computational and experimental approaches to predict host–parasite protein–protein interactions. In: von Stechow L, Santos Delgado A, Editors. Methods in Molecular Biology. New York, NY: Humana Press (2018). p. 153–73. doi: 10.1007/978-1-4939-8618-7_7
12. Kotelnikova E, Kalinin A, Yuryev A, Maslov S. Prediction of protein-protein interactions on the basis of evolutionary conservation of protein functions. Evol Bioinform Online (2007) 3:197–206. doi: 10.1177/117693430700300029
13. Wuchty S. Computational prediction of host-parasite protein interactions between P. falciparum and H sapiens PLoS ONE (2011) 6:e26960. doi: 10.1371/journal.pone.0026960
14. Yu H, Luscombe NM, Lu HX, Zhu X, Xia Y, Han J-DJ, et al. Annotation transfer between genomes: protein-protein interologs and protein-DNA regulogs. Genome Res. (2004) 14:1107–18. doi: 10.1101/gr.1774904
15. Zhou H, Gao S, Nguyen N, Fan M, Jin J, Liu B, et al. Stringent homology-based prediction of H. sapiens-M. tuberculosis H37Rv protein-protein interactions. Biol. Direct (2014) 9:5. doi: 10.1186/1745-6150-9-5
16. Davis FP, Barkan DT, Eswar N, McKerrow JH, Sali A. Host-pathogen protein interactions predicted by comparative modeling. Protein Sci. (2007) 16:2585–96. doi: 10.1110/ps.073228407
17. Lee S-A, Chan C, Tsai C-H, Lai J-M, Wang F-S, Kao C-Y, et al. Ortholog-based protein-protein interaction prediction and its application to inter-species interactions. BMC Bioinformatics (2008) 9 (Suppl. 12):S11. doi: 10.1186/1471-2105-9-S12-S11
18. Reid AJ, Berriman M. Genes involved in host-parasite interactions can be revealed by their correlated expression. Nucleic Acids Res. (2013) 41:1508–18. doi: 10.1093/nar/gks1340
19. Lo Y-S, Chen Y-C, Yang J-M. 3D-interologs: an evolution database of physical protein- protein interactions across multiple genomes. BMC Genomics (2010) 11:S7. doi: 10.1186/1471-2164-11-S3-S7
20. Murakami Y, Mizuguchi K. Homology-based prediction of interactions between proteins using averaged one-dependence estimators. BMC Bioinformatics (2014) 15:213. doi: 10.1186/1471-2105-15-213
21. Wang F, Liu M, Song B, Li D, Pei H, Guo Y, et al. Prediction and characterization of protein-protein interaction networks in swine. Proteome Sci. (2012) 10:2. doi: 10.1186/1477-5956-10-2
22. Yellaboina S, Dudekula DB, Ko MS. Prediction of evolutionarily conserved interologs in Mus musculus. BMC Genomics (2008) 9:465. doi: 10.1186/1471-2164-9-465
23. Ramakrishnan G, Srinivasan N, Padmapriya P, Natarajan V. Homology-based prediction of potential protein-protein interactions between human erythrocytes and Plasmodium falciparum. Bioinform Biol Insights (2015) 9:195–206. doi: 10.4137/BBI.S31880
24. Petersen TN, Brunak S, von Heijne G, Nielsen H. SignalP 4.0: discriminating signal peptides from transmembrane regions. Nat Methods (2011) 8:785–6. doi: 10.1038/nmeth.1701
25. Emanuelsson O, Nielsen H, Brunak S, von Heijne G. Predicting subcellular localization of proteins based on their N-terminal amino acid sequence. J Mol Biol. (2000) 300:1005–16. doi: 10.1006/jmbi.2000.3903
26. Krogh A, Larsson B, von Heijne G, Sonnhammer EL. Predicting transmembrane protein topology with a hidden Markov model: application to complete genomes. J Mol Biol. (2001) 305:567–80. doi: 10.1006/jmbi.2000.4315
27. Binder JX, Pletscher-Frankild S, Tsafou K, Stolte C, O'Donoghue SI, Schneider R, et al. Compartments: unification and visualization of protein subcellular localization evidence. Database (2014) 2014:bau012. doi: 10.1093/database/bau012
28. Santos A, Tsafou K, Stolte C, Pletscher-Frankild S, O'Donoghue SI, Jensen LJ. Comprehensive comparison of large-scale tissue expression datasets. PeerJ (2015) 3:e1072v1. doi: 10.7717/peerj.1054
29. Huerta-Cepas J, Szklarczyk D, Forslund K, Cook H, Heller D, Walter MC, et al. eggNOG 4.5: a hierarchical orthology framework with improved functional annotations for eukaryotic, prokaryotic and viral sequences. Nucleic Acids Res. (2016) 44:D286–93. doi: 10.1093/nar/gkv1248
30. Finn RD, Miller BL, Clements J, Bateman A. iPfam: a database of protein family and domain interactions found in the Protein Data Bank. Nucleic Acids Res. (2014) 42:D364–73. doi: 10.1093/nar/gkt1210
31. Mosca R, Céol A, Stein A, Olivella R, Aloy P. 3did: a catalog of domain-based interactions of known three-dimensional structure. Nucleic Acids Res. (2014) 42:D374–9. doi: 10.1093/nar/gkt887
32. Dinkel H, Van Roey K, Michael S, Kumar M, Uyar B, Altenberg B, et al. ELM 2016—data update and new functionality of the eukaryotic linear motif resource. Nucleic Acids Res. (2016) 44:D294–300. doi: 10.1093/nar/gkv1291
33. Berman HM, Battistuz T, Bhat TN, Bluhm WF, Bourne PE, Burkhardt K, et al. The protein data bank. Acta Crystallogr D Biol Crystallogr. (2002) 58:899–907. doi: 10.1107/S0907444902003451
34. Finn RD, Clements J, Eddy SR. HMMER web server: interactive sequence similarity searching. Nucleic Acids Res. (2011) 39:W29–37. doi: 10.1093/nar/gkr367
35. Finn RD, Coggill P, Eberhardt RY, Eddy SR, Mistry J, Mitchell AL, et al. The Pfam protein families database: towards a more sustainable future. Nucleic Acids Res. (2016) 44:D279–85. doi: 10.1093/nar/gkv1344
36. Valente TW, Coronges K, Lakon C, Costenbader E. How correlated are network centrality measures? Connect (2008) 28:16–26.
37. Lu L, Zhang M. Edge betweenness centrality. Encycl Syst Biol. (2013) 1:647–8. doi: 10.1007/978-1-4419-9863-7_874
38. Ashburner M, Ball CA, Blake JA, Botstein D, Butler H, Cherry JM, et al. Gene ontology: tool for the unification of biology. Gene Ontol Consort Nat Genet. (2000) 25:25–9. doi: 10.1038/75556
39. Croft D, O'Kelly G, Wu G, Haw R, Gillespie M, Matthews L, et al. Reactome: a database of reactions, pathways and biological processes. Nucleic Acids Res. (2011) 39:D691–7. doi: 10.1093/nar/gkq1018
40. Tatusov RL. The COG database: new developments in phylogenetic classification of proteins from complete genomes. Nucleic Acids Res. (2001) 29:22–8. doi: 10.1093/nar/29.1.22
41. Christmas R, Avila-Campillo I, Bolouri H, Schwikowski B, Anderson M, Kelley R, et al. Cytoscape: a software environment for integrated models of biomolecular interaction networks. AACR Educ B. (2005) 2005:12. doi: 10.1101/gr.1239303
42. VGaston K Mazandu NJM, Maz GK U, Rapano HA. Using host-pathogen functional interactions for filtering potential drug targets in Mycobacterium tuberculosis. Mycobact Dis. (2013) 3:126. doi: 10.4172/2161-1068.1000126
43. Lockyer AE, Emery AM, Kane RA, Walker AJ, Mayer CD, Mitta G, et al. Early differential gene expression in haemocytes from resistant and susceptible biomphalaria glabrata strains in response to Schistosoma mansoni. PLoS ONE (2012) 7:e51102. doi: 10.1371/journal.pone.0051102
44. Poley JD, Sutherland BJG, Fast MD, Koop BF, Jones SRM. Effects of the vertically transmitted microsporidian Facilispora margolisi and the parasiticide emamectin benzoate on salmon lice (Lepeophtheirus salmonis). BMC Genomics (2017) 18:630. doi: 10.1186/s12864-017-4040-8
45. Durmuş Tekir S, Çakir T, Ardiç E, Sayilirbaş AS, Konuk G, Konuk M, et al. PHISTO: pathogen-host interaction search tool. Bioinformatics (2013) 29:1357–8. doi: 10.1093/bioinformatics/btt137
46. Dyer MD, Murali TM, Sobral BW. Computational prediction of host-pathogen protein-protein interactions. Bioinformatics (2007) 23:i159–66. doi: 10.1093/bioinformatics/btm208
47. Vignali M, McKinlay A, LaCount DJ, Chettier R, Bell R, Sahasrabudhe S, et al. Interaction of an atypical Plasmodium falciparum ETRAMP with human apolipoproteins. Malar J. (2008) 7:211. doi: 10.1186/1475-2875-7-211
48. Grove J, Marsh M. The cell biology of receptor-mediated virus entry. J Cell Biol. (2011) 195:1071–82. doi: 10.1083/jcb.201108131
49. Morris JH, Apeltsin L, Newman AM, Baumbach J, Wittkop T, Su G, et al. clusterMaker: a multi-algorithm clustering plugin for Cytoscape. BMC Bioinformatics (2011) 12:436. doi: 10.1186/1471-2105-12-436
50. Knox DP. Proteinase inhibitors and helminth parasite infection. Parasite Immunol. (2007) 29:57–71. doi: 10.1111/j.1365-3024.2006.00913.x
51. Faz-López B, Morales-Montor J, Terrazas LI. Role of macrophages in the repair process during the tissue migrating and resident helminth infections. Biomed Res Int. (2016) 2016:8634603. doi: 10.1155/2016/8634603
52. Abedi M, Gheisari Y. Nodes with high centrality in protein interaction networks are responsible for driving signaling pathways in diabetic nephropathy. PeerJ (2015) 3:e1284. doi: 10.7717/peerj.1284
53. Crua Asensio N, Muñoz Giner E, de Groot NS, Torrent Burgas M. Centrality in the host–pathogen interactome is associated with pathogen fitness during infection. Nat Commun. (2017) 8:14092. doi: 10.1038/ncomms14092
54. Mukhtar MS, Carvunis A-R, Dreze M, Epple P, Steinbrenner J, Moore J, et al. Independently evolved virulence effectors converge onto hubs in a plant immune system network. Science (2011) 333:596–601. doi: 10.1126/science.1203659
55. Olszewski KL, Morrisey JM, Wilinski D, Burns JM, Vaidya AB, Rabinowitz JD, et al. Host-parasite interactions revealed by Plasmodium falciparum metabolomics. Cell Host Microbe (2009) 5:191–9. doi: 10.1016/j.chom.2009.01.004
56. Swann J, Jamshidi N, Lewis NE, Winzeler EA. Systems analysis of host-parasite interactions. Wiley Interdiscip Rev Syst Biol Med. (2015) 7:381–400. doi: 10.1002/wsbm.1311
57. Walker DM, Oghumu S, Gupta G, McGwire BS, Drew ME, Satoskar AR. Mechanisms of cellular invasion by intracellular parasites. Cell Mol Life Sci. (2014) 71:1245–63. doi: 10.1007/s00018-013-1491-1
58. Binns D, Dimmer E, Huntley R, Barrell D, O'Donovan C, Apweiler R. QuickGO: a web-based tool for gene ontology searching. Bioinformatics (2009) 25:3045–6. doi: 10.1093/bioinformatics/btp536
59. Areschoug T, Gordon S. Scavenger receptors: role in innate immunity and microbial pathogenesis. Cell Microbiol. (2009) 11:1160–9. doi: 10.1111/j.1462-5822.2009.01326.x
60. Goldszmid RS, Sher A. Processing and presentation of antigens derived from intracellular protozoan parasites. Curr Opin Immunol. (2010) 22:118–23. doi: 10.1016/j.coi.2010.01.017
61. Mahnke A, Meier RJ, Schatz V, Hofmann J, Castiglione K, Schleicher U, et al. Hypoxia in Leishmania major skin lesions impairs the NO-dependent leishmanicidal activity of macrophages. J Invest Dermatol. (2014) 134:2339–46. doi: 10.1038/jid.2014.121
62. Overath P, Stierhof YD, Wiese M. Endocytosis and secretion in trypanosomatid parasites - tumultuous traffic in a pocket. Trends Cell Biol. (1997) 7:27–33. doi: 10.1016/S0962-8924(97)10046-0
63. Dzik JM. Molecules released by helminth parasites involved in host colonization. Acta Biochim Pol. (2006) 53:33–64.
64. Ali Khan H, Mutus B. Protein disulfide isomerase a multifunctional protein with multiple physiological roles. Front Chem. (2014) 2:70. doi: 10.3389/fchem.2014.00070
65. Santos CXC, Stolf BS, Takemoto PVA, Amanso AM, Lopes LR, Souza EB, et al. Protein disulfide isomerase (PDI) associates with NADPH oxidase and is required for phagocytosis of Leishmania chagasi promastigotes by macrophages. J Leukoc Biol. (2009) 86:989–98. doi: 10.1189/jlb.0608354
66. Calderon MN, Guerrero CA, Acosta O, Lopez S, Arias CF. Inhibiting rotavirus infection by membrane-impermeant thiol/disulfide exchange blockers and antibodies against protein disulfide isomerase. Intervirology (2012) 55:451–64. doi: 10.1159/000335262
67. Ryser HJ, Levy EM, Mandel R, DiSciullo GJ. Inhibition of human immunodeficiency virus infection by agents that interfere with thiol-disulfide interchange upon virus-receptor interaction. Proc Natl Acad Sci USA. (1994) 91:4559–63. doi: 10.1073/pnas.91.10.4559
68. Wan S-W, Lin C-F, Lu Y-T, Lei H-Y, Anderson R, Lin Y-S. Endothelial cell surface expression of protein disulfide isomerase activates β1 and β3 integrins and facilitates dengue virus infection. J Cell Biochem. (2011) 113:1681–91. doi: 10.1002/jcb.24037
69. Straub C, Burnham JP, White AC, Pazdrak K, Sanchez C, Watanabe LC, et al. Altered eosinophil proteome in a patient with hypereosinophilia from acute fascioliasis. Clin Vaccine Immunol. (2011) 18:1999–2002. doi: 10.1128/CVI.05373-11
70. Abraham D, Leon O, Schnyder-Candrian S, Wang CC, Galioto AM, Kerepesi LA, et al. Immunoglobulin E and eosinophil-dependent protective immunity to larval Onchocerca volvulus in mice immunized with irradiated larvae. Infect Immun. (2004) 72:810–7. doi: 10.1128/IAI.72.2.810-817.2004
71. Meeusen EN, Balic A. Do eosinophils have a role in the killing of helminth parasites? Parasitol Today (2000) 16:95–101. doi: 10.1016/S0169-4758(99)01607-5
72. O'Connell AE, Hess JA, Santiago GA, Nolan TJ, Lok JB, Lee JJ, et al. Major basic protein from eosinophils and myeloperoxidase from neutrophils are required for protective immunity to Strongyloides stercoralis in mice. Infect Immun. (2011) 79:2770–8. doi: 10.1128/IAI.00931-10
73. Chiu H-C, Hannemann H, Heesom KJ, Matthews DA, Davidson AD. High-throughput quantitative proteomic analysis of dengue virus type 2 infected A549 cells. PLoS ONE (2014) 9:e93305. doi: 10.1371/journal.pone.0093305
74. Cortés A, Sotillo J, Muñoz-Antoli C, Fried B, Esteban JG, Toledo R. Altered protein expression in the ileum of mice associated with the development of chronic infections with Echinostoma caproni (Trematoda). PLoS Negl Trop Dis. (2015) 9:e0004082. doi: 10.1371/journal.pntd.0004082
75. Manque PA, Probst CM, Probst C, Pereira MCS, Rampazzo RCP, Ozaki LS, et al. Trypanosoma cruzi infection induces a global host cell response in cardiomyocytes. Infect Immun. (2011) 79:1855–62. doi: 10.1128/IAI.00643-10
76. Morias Y, Abels C, Laoui D, Van Overmeire E, Guilliams M, Schouppe E, et al. Ly6C- monocytes regulate parasite-induced liver inflammation by inducing the differentiation of pathogenic Ly6C+ monocytes into macrophages. PLOS Pathog. (2015) 11:e1004873. doi: 10.1371/journal.ppat.1004873
77. Pando-Robles V, Oses-Prieto JA, Rodríguez-Gandarilla M, Meneses-Romero E, Burlingame AL, Batista CVF. Quantitative proteomic analysis of Huh-7 cells infected with Dengue virus by label-free LC–MS. J Proteomics (2014) 111:16–29. doi: 10.1016/j.jprot.2014.06.029
78. Rinaldi M, Dreesen L, Hoorens PR, Li RW, Claerebout E, Goddeeris B, et al. Infection with the gastrointestinal nematode Ostertagia ostertagi in cattle affects mucus biosynthesis in the abomasum. Vet Res. (2011) 42:61. doi: 10.1186/1297-9716-42-61
79. Ashtiani M, Salehzadeh-Yazdi A, Razaghi-Moghadam Z, Hennig H, Wolkenhauer O, Mirzaie M, et al. A systematic survey of centrality measures for protein-protein interaction networks. BMC Syst Biol. (2018) 12:80. doi: 10.1186/s12918-018-0598-2
80. Protasio AV, Dunne DW, Berriman M. Comparative study of transcriptome profiles of mechanical- and skin-transformed Schistosoma mansoni schistosomula. PLoS Negl Trop Dis. (2013) 7:e2091. doi: 10.1371/journal.pntd.0002091
81. Aumailley M, Gayraud B. Structure and biological activity of the extracellular matrix. J Mol Med. (1998) 76:253–65. doi: 10.1007/s001090050215
82. Geurts N, Opdenakker G, Van den Steen PE. Matrix metalloproteinases as therapeutic targets in protozoan parasitic infections. Pharmacol. Ther. (2012) 133:257–79. doi: 10.1016/j.pharmthera.2011.11.008
83. Silva-Almeida M, Carvalho LO, Abreu-Silva AL, Souza CS, Hardoim DJ, Calabrese KS. Extracellular matrix alterations in experimental Leishmania amazonensis infection in susceptible and resistant mice. Vet Res. (2012) 43:10. doi: 10.1186/1297-9716-43-10
84. Liu CG, Maercker C, Castañon MJ, Hauptmann R, Wiche G. Human plectin: organization of the gene, sequence analysis, and chromosome localization (8q24). Proc Natl Acad Sci USA. (1996) 93:4278–83. doi: 10.1073/pnas.93.9.4278
85. Su C, Cao Y, Kaplan J, Zhang M, Li W, Conroy M, et al. Duodenal helminth infection alters barrier function of the colonic epithelium via adaptive immune activation. Infect Immun. (2011) 79:2285–94. doi: 10.1128/IAI.01123-10
86. Cass CL, Johnson JR, Califf LL, Xu T, Hernandez HJ, Stadecker MJ, et al. Proteomic analysis of Schistosoma mansoni egg secretions. Mol Biochem Parasitol. (2007) 155:84–93. doi: 10.1016/j.molbiopara.2007.06.002
87. Eichenberger RM, Ramakrishnan C, Russo G, Deplazes P, Hehl AB. Genome-wide analysis of gene expression and protein secretion of Babesia canis during virulent infection identifies potential pathogenicity factors. Sci Rep. (2017) 7:3357. doi: 10.1038/s41598-017-03445-x
88. Ludolf F, Patrocínio PR, Corrêa-Oliveira R, Gazzinelli A, Falcone FH, Teixeira-Ferreira A, et al. Serological screening of the Schistosoma mansoni adult worm proteome. PLoS Negl Trop Dis. (2014) 8:e2745. doi: 10.1371/journal.pntd.0002745
89. Thiangtrongjit T, Adisakwattana P, Limpanont Y, Dekumyoy P, Nuamtanong S, Chusongsang P, et al. Proteomic and immunomic analysis of Schistosoma mekongi egg proteins. Exp Parasitol. (2018) 191:88–96. doi: 10.1016/j.exppara.2018.07.002
90. Li Y, Shi J, Yang J, Ma Y, Cheng L, Zeng J, et al. A Wnt/β-catenin negative feedback loop represses TLR-triggered inflammatory responses in alveolar epithelial cells. Mol Immunol. (2014) 59:128–35. doi: 10.1016/j.molimm.2014.02.002
91. Goldberg DS, Roth FP. Assessing experimentally derived interactions in a small world. Proc Natl Acad Sci USA. (2003) 100:4372–6. doi: 10.1073/pnas.0735871100
92. Guo X, Xu Y, Bian G, Pike AD, Xie Y, Xi Z. Response of the mosquito protein interaction network to dengue infection. BMC Genomics (2010) 11:380. doi: 10.1186/1471-2164-11-380
Keywords: computational biology, systems biology, biological networks, parasitology, schistosomiasis, host–parasite interactions
Citation: Cuesta-Astroz Y, Santos A, Oliveira G and Jensen LJ (2019) Analysis of Predicted Host–Parasite Interactomes Reveals Commonalities and Specificities Related to Parasitic Lifestyle and Tissues Tropism. Front. Immunol. 10:212. doi: 10.3389/fimmu.2019.00212
Received: 18 September 2018; Accepted: 24 January 2019;
Published: 13 February 2019.
Edited by:
Thiago Almeida Pereira, Stanford University, United StatesReviewed by:
Gabriel Rinaldi, Wellcome Trust Sanger Institute (WT), United KingdomMarc Torrent, University of Cambridge, United Kingdom
Copyright © 2019 Cuesta-Astroz, Santos, Oliveira and Jensen. This is an open-access article distributed under the terms of the Creative Commons Attribution License (CC BY). The use, distribution or reproduction in other forums is permitted, provided the original author(s) and the copyright owner(s) are credited and that the original publication in this journal is cited, in accordance with accepted academic practice. No use, distribution or reproduction is permitted which does not comply with these terms.
*Correspondence: Guilherme Oliveira, Z3VpbGhlcm1lLm9saXZlaXJhQGl0di5vcmc=
Lars J. Jensen, bGFycy5qdWhsLmplbnNlbkBjcHIua3UuZGs=
†These authors have contributed equally to this work