- The First Affiliated Hospital of Chongqing Medical University, Chongqing Key Laboratory of Ophthalmology, and Chongqing Eye Institute, Chongqing, China
Background: Vogt-Koyanagi-Harada (VKH) disease is a complex disease associated with multiple molecular immunological mechanisms. As the underlying mechanism for VKH disease is unclear, we hope to utilize an integrated analysis of key pathways and drug targets to develop novel therapeutic strategies.
Methods: Candidate genes and proteins involved in VKH disease were identified through text-mining in the PubMed database. The GO and KEGG pathway analyses were used to examine the biological functions of the involved pathways associated with this disease. Molecule-related drugs were predicted through Drug-Gene Interaction Database (DGIdb) analysis.
Results: A total of 48 genes and 54 proteins were associated with VKH disease. Forty-two significantly altered pathways were identified through pathway analysis and were mainly related to immune and inflammatory responses. The top five of significantly altered pathways were termed as “inflammatory bowel disease,” “cytokine-cytokine receptor interaction,” “allograft rejection,” “antigen processing,” and “presentation and Herpes simplex infection” in the KEGG database. IFN-γ and IL-6 were identified as the key genes through network analysis. The DGIdb analysis predicted 48 medicines as possible drugs for VKH disease, among which Interferon Alfa-2B was co-associated both with IFN-γ and IL-6.
Conclusions: In this study, systematic analyses were utilized to detect key pathways and drug targets in VKH disease via bioinformatics analysis. IFN-γ and IL-6 were identified as the key mediators and possible drug targets in VKH disease. Interferon Alfa-2B was predicted to be a potentially effective drug for VKH disease treatment by targeting IFN-γ and IL-6, which warrants further experimental and clinical investigations.
Introduction
Vogt-Koyanagi-Harada (VKH) disease is an immune-mediated disorder characterized by chronic, bilateral granulomatous panuveitis, often associated with neurological, audiovestibular and cutaneous manifestations (1). VKH disease is more commonly seen in Asians, Hispanics, Native Americans (2), and rare in Africans (3). Bilateral panuveitis, hearing disorder and meningitis are the main clinical features. Treatment with systemic corticosteroid is the mainstay of VKH disease therapy in the acute uveitic phase (4). However, despite proper treatment with a high-dose of corticosteroid, 79% of patients will experience recurrent attacks and develop chronic disease (5). Moreover, a high-dose of corticosteroid over a prolonged period may lead to side effects such as Cushing syndrome, hyperglycemia, and increased incidence of severe infections (6). Currently, there remain unmet medical needs for novel therapies that can etiologically target the molecules or immune mediators involved in the disease.
Although the exact biological mechanisms are still unclear, an increasing number of candidate genes and proteins have been reported to be involved in the development of VKH disease, which may be possible drug targets for the disease (7, 8). However, it is still challenging to prioritize these drug targets among many genes and proteins. Here, we used integrated bioinformatic analysis to summarize the candidate genes and proteins associated with VKH disease and identify the potential key pathways and drug targets, which may help to develop new therapeutic agents.
Materials and Methods
Identifying Candidate Genes and Proteins Associated With VKH Disease
We manually collected candidate genes and proteins associated with VKH disease by a thorough review of the literature in any language published from May 1981 to November 2019, using a similar approach used earlier by others (9, 10). We used the following terms to search the PubMed database: “idiopathic uveoencephalitis” OR uveoencephalitis OR “uveomeningitic syndrome” OR Vogt-Koyanagi-Harada. Studies were eligible if they included a comparison of the expression levels of a gene or protein between VKH patients and controls. Key exclusion criteria included: (i) studies only conducted in animal models, (ii) meta-analysis of published results, and (iii) review and comment papers. We also matched eligible genes and proteins with our previously published database (UVEOGENE, http://www.uvogene.com) (11). Two researchers were trained in each step with pilot tests before collecting and managing data independently. Regular meetings were held to clear up any misunderstandings or disagreements.
Functional Analysis
Functional analysis was performed based on the DAVID online tools (version 6.8, http://david.ncifcrf.gov). In the gene ontology (GO) database, the analysis of candidate genes and proteins was divided into three categories, termed as biological processes (BP), molecular functions (MF), and cellular components (CC) (12). The GO database provides annotations to describe the properties of genes and gene products of different organisms and shows enriched genes’ potential function. BP is an ordered combination of molecular functions to describe a wide range of biological processes. The MF is used to describe the function of a gene or gene product, and the CC is designed for describing subcellular structures, locations, and macromolecular complexes of genes. We submitted the list of identified candidate genes to the DAVID online tools and obtained the significant enrichment of the above categories. A significant threshold of P < 0.05 was used.
KEGG Pathway Enrichment Analysis
Kyoto Encyclopedia of Genes and Genomes (KEGG) pathway enrichment analysis was performed on candidate genes to enrich significantly altered pathways, using the DAVID online tools regarding the KEGG database. KEGG is an encyclopedia of genes and genomes for understanding high-level biological functions and utilities (13). The KEGG database provides a collection of graphical diagrams (pathway maps), in which some of the known metabolic pathways and regulatory pathways are displayed to describe the linkage, interaction and function of enriched candidate genes across different cells and organisms. In this study, enriched pathways were considered significant if including at least two genes and reaching a significance level of P < 0.05.
Protein-Protein Interaction Analysis
Protein-protein interaction (PPI) analysis was performed based on candidate genes and proteins using the STRING database (https://stringdb.org/). The STRING database aims to collect and integrate the information by integrating known and predicted protein-protein association data from many organisms (14). Default parameters in STRING were used. Cytoscape software (version 3.7.2, California, USA) was used to construct and visualize the PPI network. Cytoscape is a graphical display tool used for visualizing complex biological networks (15). Furthermore, we used the Cytoscape plugin molecular complex detection (MCODE) to explore significant modules in the PPI network using the following scores and parameters: k score= 2, degree cutoff= 2, node score cutoff= 0.2, and maximum depth= 100 (16).
Identification of Hub Genes
CytoHubba plugin in Cytoscape was used to identify hub genes in the PPI network by calculating and analyzing the network structure. Three algorithms were used to generate intersecting genes, including the maximum neighborhood component (MNC), the degree, and the closeness, as described previously (17). Briefly, the degree is the number of the edges of a gene in the network, representing the interaction pairs with others. The closeness could be used to evaluate the genes in the network according to the calculated centrality. The MNC represents the number of nodes in the maximum connected subgraph. The genes generated by the above algorithms’ intersection were more likely to locate in a core position and were considered as the hub genes.
Drug-Gene Interactions
The Drug-Gene Interaction Database (DGIdb, http://dgidb.genome.wustl.edu/) is a drug prediction database, which can be used to screen drugs that potentially target certain genes of interest (18). The DGIdb provides gene-drug interactions and potential druggability according to their gene category. We utilized the DGIdb analysis to obtain all possible gene-drug interactions for the top-two ranked molecules of hub genes. Cytoscape was used to visualize the acquired interactions.
Results
Genes and Proteins Acquisition
After screening a total of 1,323 articles from PubMed, we obtained 128 eligible articles. Based on the inclusion and exclusion criteria, we identified 48 genes and 54 proteins associated with VKH disease (Table 1). The expression of these candidate genes was reported to be significantly changed in VKH disease as compared with healthy individuals. Besides, among these candidate genes, 36 genes overlapped with genes recorded in the Uveogene database for VKH disease (11). These 36 genes had been reported to have susceptible single nucleotide polymorphisms and loci for VKH disease in the Uveogene database. Figure 1 illustrates the flow chart of this study.
GO Analysis of Genes and Proteins
GO enrichment analysis was performed with the DAVID online tools to examine the identified genes and proteins’ biological characteristics. The analysis of BP (biological processes) showed a total of 251 functions, 200 of which were significantly enriched (P < 0.05). The top-ranked functions included the categories “immune response,” “inflammatory response,” “positive regulation of T cell proliferation,” “positive regulation of tyrosine phosphorylation of Stat3 protein,” and “interferon-gamma-mediated signaling pathway.” The CC (cellular components) analysis included 29 functions, of which 26 were significantly enriched (P < 0.05). For the CC analysis, the identified genes and proteins were mostly enriched in the “external side of plasma membrane,” “extracellular space,” “integral component of luminal side of endoplasmic reticulum membrane,” “extracellular region,” and “MHC class II protein complex.” The MF (molecular functions) analysis included 37 functions, 26 of which were significantly enriched (P < 0.05). Changes in MF were significantly enriched in “cytokine activity,” “peptide antigen binding,” “MHC class II receptor activity,” “growth factor activity,” and “chemokine activity.” The top ten functional enrichment analyses of GO (BP, MF, CC) are shown in Figure 2, and the significant GO (BP, MF, CC) are provided in Supplementary Table S1. The corresponding genes enriched in GO analysis (Figure 2) are listed in Supplementary Table S2.
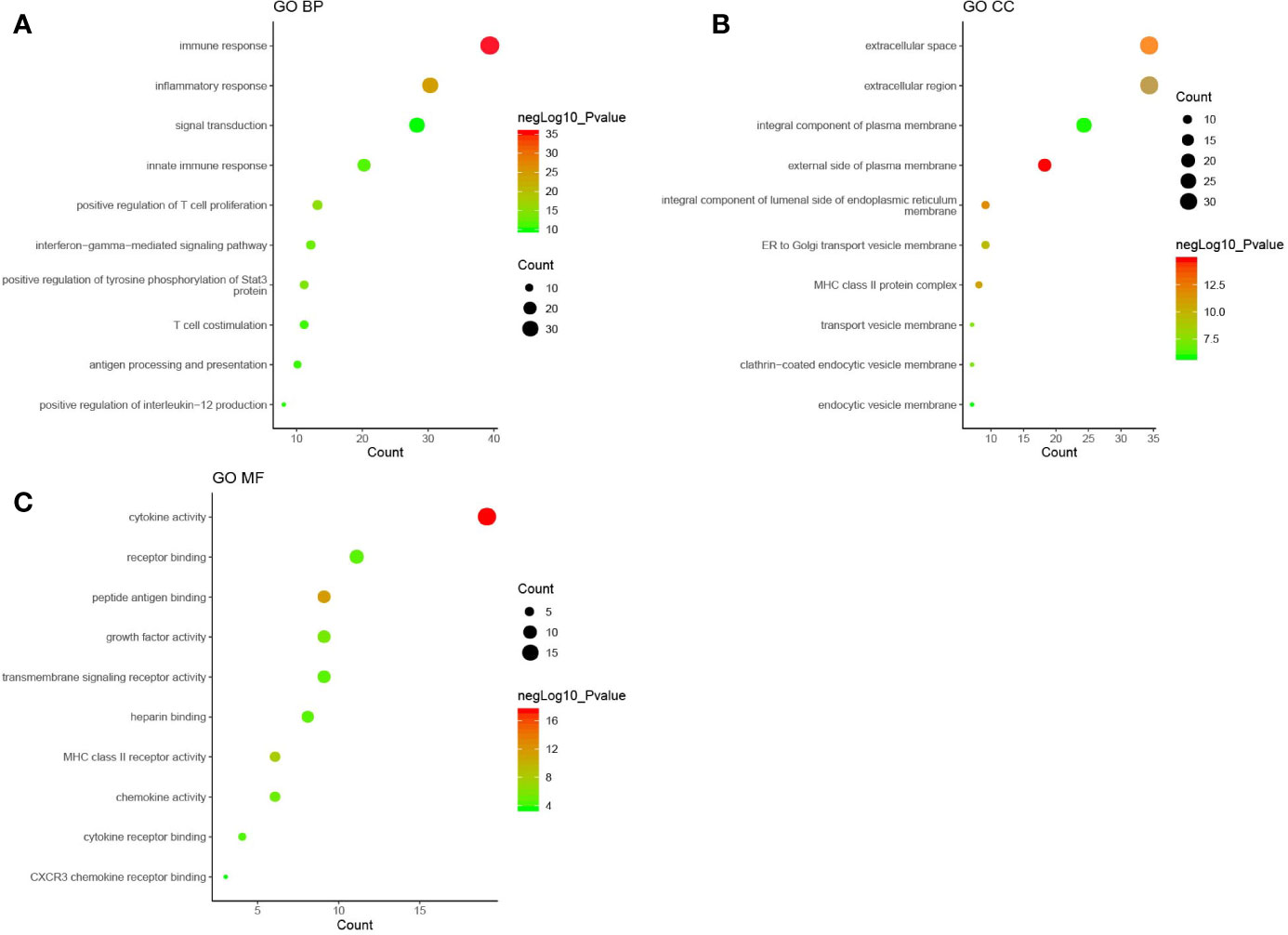
Figure 2 The top ten functional enrichment analyses of gene ontology (GO). (A): biological processes (BP); (B): cellular components (CC); (C): molecular functions (MF). Each circle in Figure 2 represented a pathway of GO analysis. The pathway highlighted in red represented more significant P-values, whereas the pathway highlighted in green represented less significant P-values. The size of circle represented the count of pathway, and a larger circle indicated a larger count enriched in the pathway.
KEGG Analysis of Genes and Proteins
The DAVID online tools were utilized for the KEGG enrichment pathway analysis to show the potential involvement of pathways related to identified candidate genes and proteins. The analysis of KEGG identified 42 significantly altered pathways (P < 0.05) (Supplementary Table S3). The top-ranked pathways were mainly involved in categories termed as “inflammatory bowel disease (IBD),” “cytokine-cytokine receptor interaction,” “allograft rejection,” “antigen processing,” and “presentation and Herpes simplex infection” (Figure 3). The corresponding genes enriched in the KEGG pathway (Figure 3) are listed in Supplementary Table S4. Additionally, the IBD pathway was the most significant in the enrichment analysis with 19 genes involved (Figure 4). The cytokine-cytokine receptor interaction had the largest number of genes, 27 genes enriched in the pathway (Figure 5). Moreover, several genes, including IFN-γ, IL6, IL12, IL4, IL23R and IL21, were shared by the IBD pathway and the cytokine-cytokine receptor interaction pathway and were related to the signaling transductions by members of the interleukin-family indicating that these members of the interleukin-family might play an important role in the pathogenesis of VKH disease.
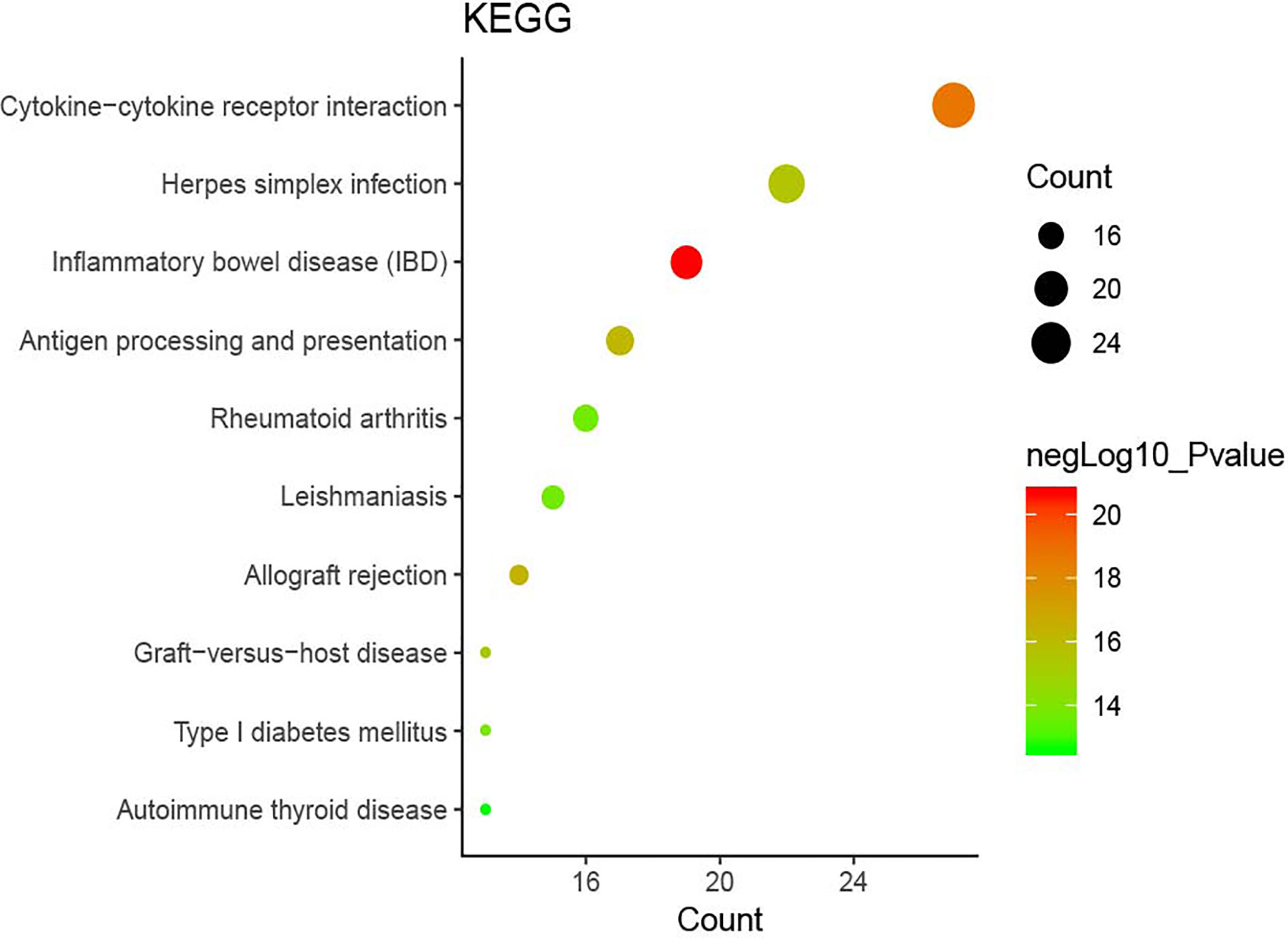
Figure 3 The top ten pathway enrichment analyses from the Kyoto Encyclopedia of Genes and Genomes (KEGG) database.
PPI Network Analysis and Related Gene Modules
The PPI network consisted of STRING and Cytoscape, which were used to determine the most important genes and proteins clusters. All the 87 nodes and 754 edges in the PPI network are shown in Figure 6. Besides, the MCODE plugin in Cytoscape identified two main modules. Cluster 1 (score = 23.28) consisted of 26 nodes and 291 edges, and cluster 2 (score = 6.889) consisted of 10 nodes and 31 edges (Figure 6). In cluster 1, several genes, including IFN-γ, IL6, IL4, IL23R and IL21, were significantly enriched. These genes were also related to the enriched pathways, including the IBD pathway and the cytokine-cytokine receptor interaction pathway. In cluster 2, the most enriched genes were related to the human leukocyte antigen (HLA) family, including HLA-A, HLA-B, HLA-DQA1, HLA-DQB1, HLA-DRA, HLA-DPB1 and HLA-DRB5.
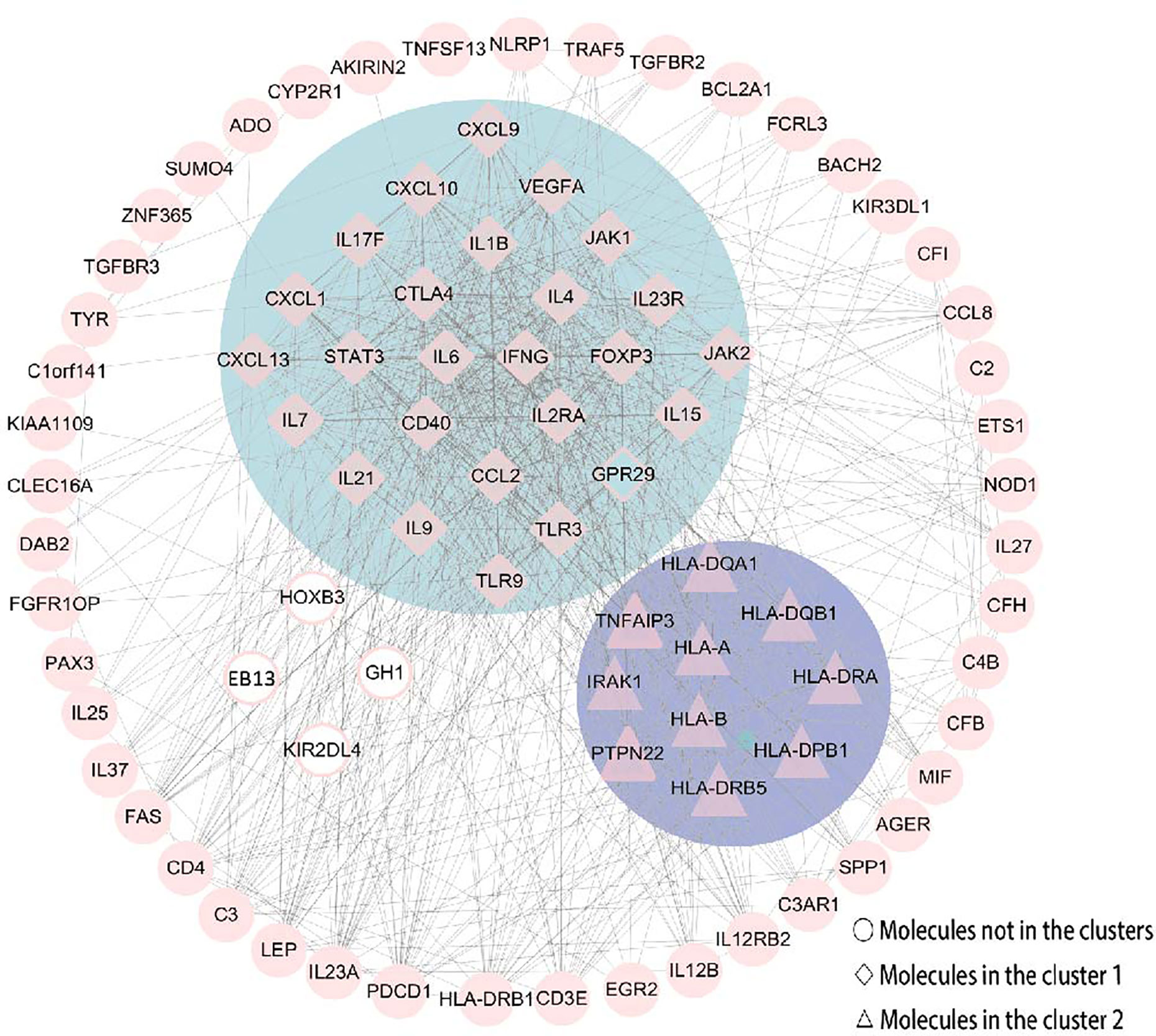
Figure 6 The PPI network was constructed by STRING and Cytoscape. There were 87 nodes and 754 edges in the PPI network. Besides, two modules were recognized by MCODE in Cytoscape. Cluster 1 (score = 23.28) was the most powerful module containing 26 nodes and 291 edges. Cluster 2 (score = 6.889) consisted of 10 nodes and 31 edges. Molecules represented as a pink diamond in the circle with a blue background represents genes belonging to cluster 1, pink triangles in the circle with a purple background represent genes belonging to cluster 2, and genes in the white background do not belong to either cluster. HOXB3, KIR2DL4, GH1, EBI3 and GPR29 in pink graphics were predicted genes using interactions between submitted genes in the PPI network by Cytoscape, and other nodes were of submitted genes in the PPI network and showed interactions between multiple genes.
Hub Genes Recognition
CytoHubba was utilized to search for the key genes and calculated all the molecular nodes and edges. Consequently, 102 target genes were involved in the PPI network complex, forming 87 nodes and 754 edges (Figure 7). To search for the important nodes in the PPI network, all nodes were ranked by the three algorithms, including the degree, closeness and MNC provided by cytoHubba. The cytoHubba plugin Cytoscape was used to analyze the hub genes in the PPI network, and the following genes with the top ten grades were identified as hub genes: IL6, IFN-γ, IL4, CTLA4, IL1B, STAT3, CCL2, CD40, FOXP3 and IL2RA. Among these, IFN-γ and IL-6 were the top two of the ten grades and considered to be the key genes in this model, given the fact that the products of genes were at the core of the PPI network (Figure 7). The descriptions of gene symbols and the details shown in Figure 7 are provided in Supplementary Table S5.
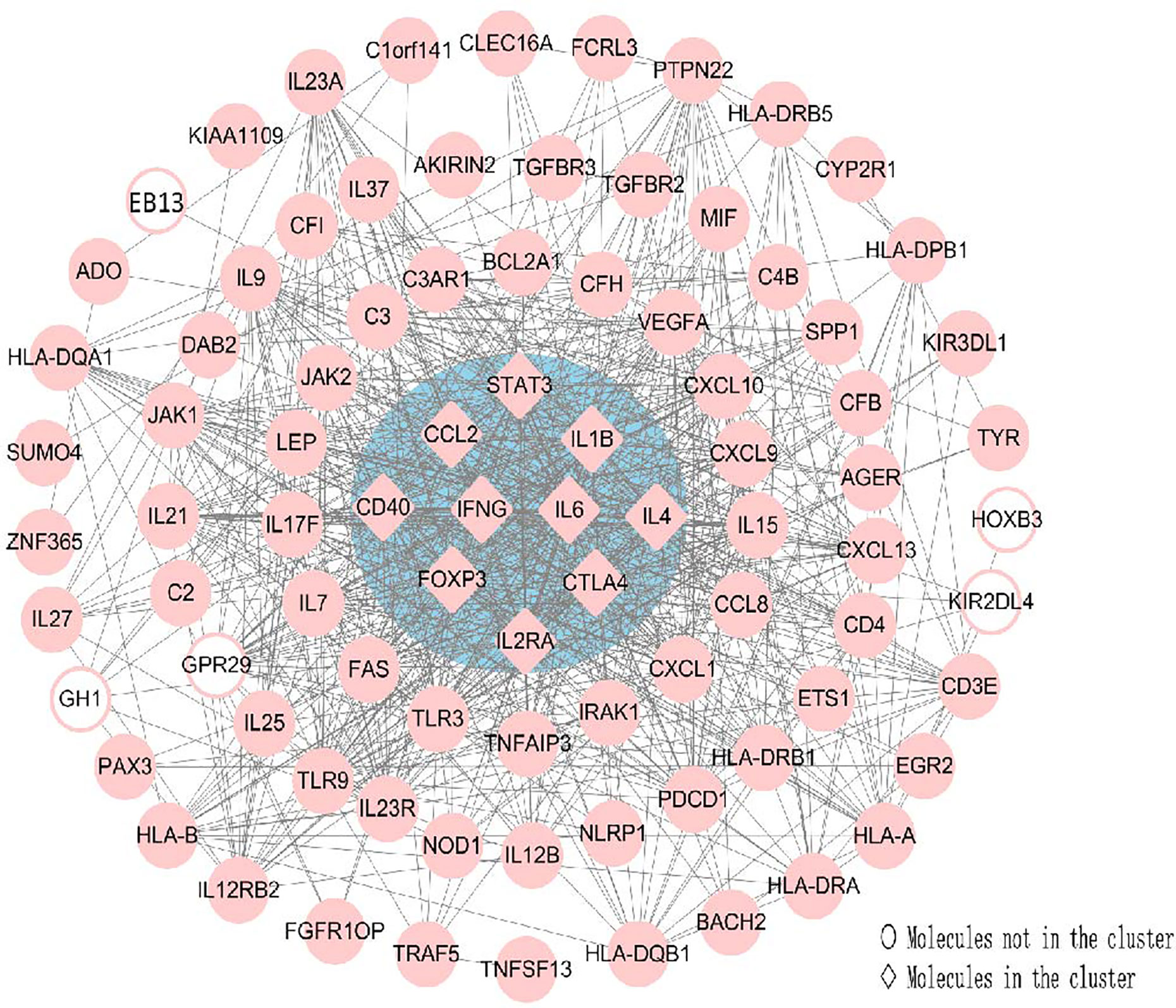
Figure 7 Using Cytohubba, a plugin Cytoscape, the top 10 genes were identified as hub genes. This analysis revealed two key genes: IFN-γ and IL-6 in the cluster. All the gene nodes and edges were calculated. Molecules represented as pink diamonds in the circle with a blue background represented genes belonging to the cluster showing the top ten genes in the network by the Cytoscape plugin Cytohubba, and pink circles without a colored background represent genes not belonging to this cluster. HOXB3, KIR2DL4, GH1, EBI3 and GPR29 in pink graphics were predicted genes using interactions between submitted genes in the PPI network by Cytoscape, and other nodes were of submitted genes in the PPI network and showed interactions between multiple genes.
Drug-Gene Interactions
IFN-γ and IL-6, as the identified key genes, were entered into the DGIdb to obtain potential drugs. 48 drugs were identified in the DGIdb analysis; the information about the source, scores, and interaction type of target drugs is provided in the Supplementary Table S6. Most of the target drugs were inhibitors, monoclonal antibodies and immunomodulatory agents. Among these identified drugs, Interferon Alfa-2B was predicted to act on both IFN-γ and IL-6 (Figure 8).
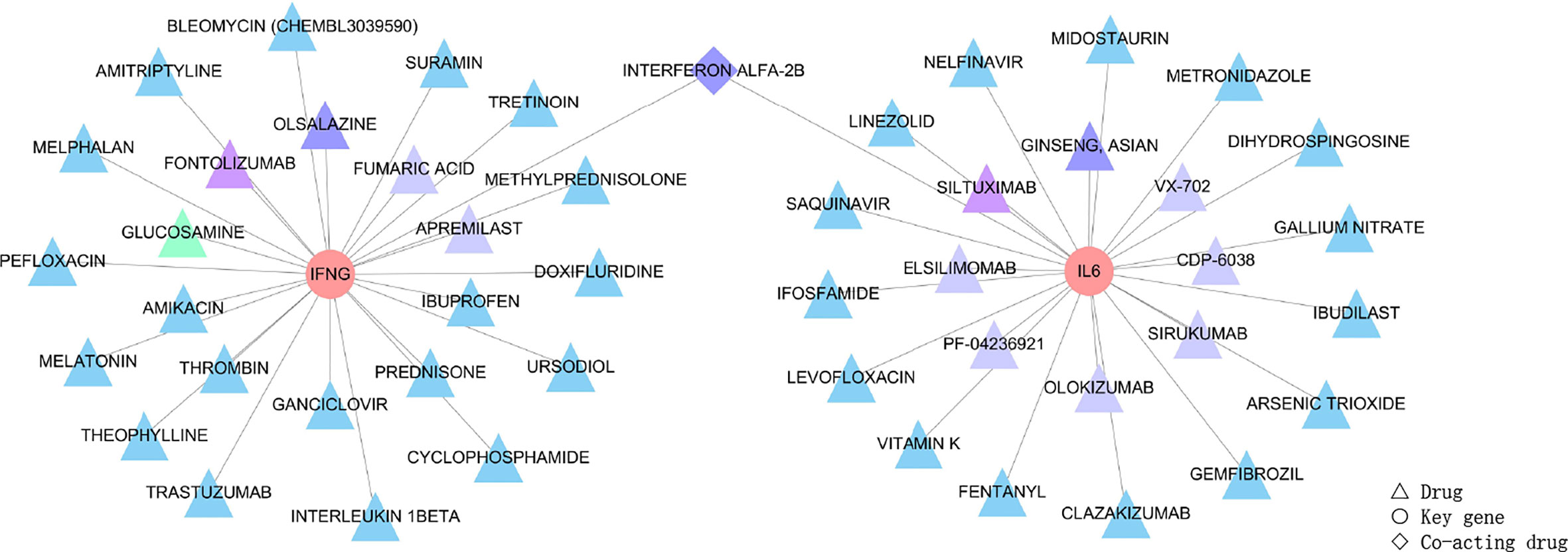
Figure 8 Drug targeting the two genes, IFN-γ and IL-6, shown as pink circles. 48 drugs as the main therapeutic agents shown in triangles and expressed in different groups according to scores. Firstly, the triangle in green represented GLUCOSAMINE obtained five scores through the DGIdb analysis, and it was the most relevant drug. Secondly, triangles in purple, including FONTOLIZUMAB and SILTUXIMAB acquired four scores, and these were the second most relevant drugs. Thirdly, drugs with three scores were OLSALAZINE and GINSENG ASIAN as shown by dark blue triangles, and INTERFERON ALFA-2B shown as dark blue diamonds since Interferon Alfa-2B was able to target both of the two key genes. Moreover, most drugs obtained two scores and are shown as light blue triangles (the target drugs with scores can be seen in Supplementary Table S6). Lastly, eight drugs, including FUMARIC ACID, APREMILAST, VX-702, CDP-6038, SIRUKUMAB, OLOKIZUMAB, ELSILIMOMAB and PF-04236921, obtained one score and are shown as blue-grey triangles. The scores of 48 drugs identified by the DGIdb show the importance of drugs, with a higher score indicating greater importance.
Discussion
In the present study, we used systematic analyses to show key pathways and potential VKH disease drugs. Two significant modules and a top ten hub genes were detected with IFN-γ and IL-6 as key genes. 48 target drugs were potentially useful drugs for the treatment of VKH disease. Interferon Alfa-2B targeted both IFN-γ and IL-6 and predicted a potentially useful drug to treat VKH, and warrants further experimental and clinical investigations.
The results of GO enrichment analysis indicated that immune response and inflammatory response play a significant role in VKH disease, which confirms earlier studies in this field (19–23). There still are many mediators related to these pathways, which have not been reported to be associated with VKH disease. Our enrichment analysis suggests that these molecules along with their involved pathways are closely linked with the development and pathogenic processes of VKH disease, which warrants further experimental investigations. Various mediators, including HOXB3, GH1, KIR2DL4 have not been reported in VKH disease, but these were predicted to have a high relevance for VKH disease as shown by our PPI network analysis. Previous studies have suggested that HOXB3, GH1 and KIR2DL4 are involved in autoimmune disease such as Thyroid-associated orbitopathy (TAO), Type 1 diabetes (T1DM), and Systemic lupus erythematosus (SLE) (24–26). Their functional role in VKH disease requires further studies. We also identified a variety of pathways related to certain autoimmune diseases such as the IBD pathway, the Toll-Like receptor pathway, the CD27–CD70 pathway and the CD40–CD40L pathway which have been shown to be associated with systemic lupus erythematosus (SLE), multiple sclerosis (MS), rheumatoid arthritis (RA), systemic sclerosis (SSc), Sjögren’s syndrome (SS), psoriasis, uveitis and other autoimmune diseases (11, 27–29). These findings suggest a certain degree of shared pathogenic pathways between VKH disease and other autoimmune diseases.
Two key genes, IFN-γ and IL-6, were identified in our study by cytoHubba, a plug-in Cytoscape. Lymphocytes produce IFN-γ in response to various immune stimuli. The essential role of IFN-γ in human anti-viral immunity has been illustrated earlier (30, 31). Several studies have indicated that VKH is an autoimmune disease mediated by Th1/IFN-γ and Th17/IL-17 pathways (32). Examples include studies that showed that the expression level of IFN-γ is significantly higher in peripheral blood mononuclear cell (PBMC), aqueous or serum of VKH patients as compared with those in control subjects (33–36). Besides IFN-γ we also identified the cytokine IL-6 as a key player in VKH disease. IL-6 is a four-helix cytokine composed of 184 amino acids (37) with various physiological functions, including regulating the proliferation and differentiation of immune cells. IL-6 modulates almost all aspects of the innate immune system. It has been shown that IL-6 plays a significant role in regulating the balance between IL-17 producing Th17 cells and regulatory T cells (Treg) (38, 39). Both T-cell subsets play an important role in the pathogenesis of VKH disease. Several studies have shown that the concentration of IL-6 in PBMC, monocyte-derived macrophages (MDMs), or aqueous humor from VKH patients is significantly higher than that observed in controls (40–42). This evidence support that IFN-γ and IL-6 are key mediators related to VKH disease, which suggests that they might be an attractive drug target for this disease. According to the analysis of the DGIdb, Interferon Alfa-2B is specific for both IFN-gamma and IL-6. The Drug-Gene Interaction database (DGIdb) mines available resources and predicts potentially effective therapeutic targets or prioritized drug development based on specific genes (18, 43, 44). We performed drug-gene interaction networks through bioinformatics analysis to identify target drugs that may act on both IFN-γ and IL-6. Of the 48 target drugs obtained from the DGIdb, most were inhibitors, monoclonal antibodies or immunomodulators. Among these potential drugs, Interferon Alfa-2B was found to be the drug that could target both IFN-γ as well as IL-6. The DGIdb provides evidence showing that Interferon Alfa-2B affects the expression of both IFN-γ and IL-6 (45, 46). Interferon, a class of cytokines, can interfere with virus replication, reduce cell proliferation, and alter immunity (47, 48). In recent studies, the role of IFN-Alfa in the pathogenesis of autoimmune diseases has been recognized (49, 50). Interferon Alfa-2B is an effective drug for treating autoimmune diseases such as idiopathic thrombocytopenic purpura (ITP) and uveitis (51, 52). The use of Interferon Alfa-2B has also been reported in the treatment of severe chronic uveitis in patients with Behçet’s disease (53) and uveitic cystoid macular edema (54). VKH disease, along with Behçet’s disease and uveitic cystoid macular edema, are anatomically classified as non-infectious posterior or pan-uveitis and can be treated with the same class of immunomodulatory drugs such as cyclosporin A (55, 56). The efficacy of Interferon Alfa-2B in the treatment of VKH disease has not yet been reported, but our analyses highlight the potential of Interferon Alfa-2B for the treatment of this disease, which necessitates further studies.
This study has some major limitations. It should be noted that we were dependent on existing data by integrative bioinformatic analysis, and our analyses were based on currently available information obtained from existing research surveys, suggesting that new information from future studies may influence the results presented here. Due to the lack of available data, dynamic networks’ development is not yet based on a genetic-epigenetic association. Moreover, our analyses are largely exploratory, and these results need to be further confirmed by experimental in vitro and in vivo studies.
Conclusion
In this study, systematic analyses were performed to identify key pathways and drug targets in VKH disease via bioinformatics analysis. Two significant modules and a top ten hub genes in VKH disease were detected with IFN-γ and IL-6 as the top two genes. The study furthermore predicted Interferon Alfa-2B as a potentially useful drug for the treatment of VKH disease.
Data Availability Statement
The raw data supporting the conclusions of this article will be made available by the authors, without undue reservation.
Author Contributions
PY and ZC conceived and designed the study. ZC and WZ did the literature review. ZZ and GS checked data. ZC and ZZ analyzed and interpreted the data. ZC wrote the first draft of the paper. PY supervised the study. All authors contributed to the article and approved the submitted version.
Funding
This study was supported by National Natural Science Foundation Key Program (81930023), Natural Science Foundation Major International (Regional) Joint Research Project (81720108009), Chongqing Outstanding Scientists Project (2019), Chongqing Key Laboratory of Ophthalmology (CSTC, 2008CA5003), Chongqing Science & Technology Platform and Base Construction Program (cstc2014pt-sy10002) and the Chongqing Chief Medical Scientist Project (2018).
Conflict of Interest
The authors declare that the research was conducted in the absence of any commercial or financial relationships that could be construed as a potential conflict of interest.
Supplementary Material
The Supplementary Material for this article can be found online at: https://www.frontiersin.org/articles/10.3389/fimmu.2020.587443/full#supplementary-material
References
1. Chi W, Yang P, Li B, Wu C, Jin H, Zhu X, et al. IL-23 promotes CD4+ T cells to produce IL-17 in Vogt-Koyanagi-Harada disease. J Allergy Clin Immunol (2007) 119(5):1218–24. doi: 10.1016/j.jaci.2007.01.010
2. Greco A, Fusconi M, Gallo A, Turchetta R, Marinelli C, Macri GF, et al. Vogt-Koyanagi-Harada syndrome. Autoimmun Rev (2013) 12(11):1033–8. doi: 10.1016/j.autrev.2013.01.004
3. Damico FM, Kiss S, Young LH. Vogt-Koyanagi-Harada disease. Semin Ophthalmol (2005) 20(3):183–90. doi: 10.1080/08820530500232126
4. Moorthy RS, Inomata H, Rao NA. Vogt-Koyanagi-Harada syndrome. Surv Ophthalmol (1995) 39(4):265–92. doi: 10.1016/s0039-6257(05)80105-5
5. Sakata VM, da Silva FT, Hirata CE, Marin ML, Rodrigues H, Kalil J, et al. High rate of clinical recurrence in patients with Vogt-Koyanagi-Harada disease treated with early high-dose corticosteroids. Graefes Arch Clin Exp Ophthalmol (2015) 253(5):785–90. doi: 10.1007/s00417-014-2904-z
6. Su E, Oza VS, Latkany P. A case of recalcitrant pediatric Vogt-Koyanagi-Harada disease successfully controlled with adalimumab. J Formos Med Assoc (2019) 118(5):945–50. doi: 10.1016/j.jfma.2018.12.014
7. Chang R, Chen L, Su G, Du L, Qin Y, Xu J, et al. Identification of Ribosomal Protein S4, Y-Linked 1 as a cyclosporin A plus corticosteroid resistance gene. J Autoimmun (2020) 112:102465. doi: 10.1016/j.jaut.2020.102465
8. Hou S, Liao D, Zhang J, Fang J, Chen L, Qi J, et al. Genetic variations of IL17F and IL23A show associations with Behcet’s disease and Vogt-Koyanagi-Harada syndrome. Ophthalmology (2015) 122(3):518–23. doi: 10.1016/j.ophtha.2014.09.025
9. Pu J, Yu Y, Liu Y, Tian L, Gui S, Zhong X, et al. MENDA: a comprehensive curated resource of metabolic characterization in depression. Brief Bioinform (2020) 21(4):1455–64. doi: 10.1093/bib/bbz055
10. Lee WY, Bachtiar M, Choo CCS, Lee CG. Comprehensive review of Hepatitis B Virus-associated hepatocellular carcinoma research through text mining and big data analytics. Biol Rev Camb Philos Soc (2019) 94(2):353–67. doi: 10.1111/brv.12457
11. Wang Q, Su G, Tan X, Deng J, Du L, Huang X, et al. UVEOGENE: An SNP database for investigations on genetic factors associated with uveitis and their relationship with other systemic autoimmune diseases. Hum Mutat (2019) 40(3):258–66. doi: 10.1002/humu.23702
12. Gene Ontology C. Gene Ontology Consortium: going forward. Nucleic Acids Res (2015) 43(Database issue):D1049–56. doi: 10.1093/nar/gku1179
13. Kanehisa M, Goto S. KEGG: kyoto encyclopedia of genes and genomes. Nucleic Acids Res (2000) 28(1):27–30. doi: 10.1093/nar/28.1.27
14. Szklarczyk D, Morris JH, Cook H, Kuhn M, Wyder S, Simonovic M, et al. The STRING database in 2017: quality-controlled protein-protein association networks, made broadly accessible. Nucleic Acids Res (2017) 45(D1):D362–8. doi: 10.1093/nar/gkw937
15. Shannon P, Markiel A, Ozier O, Baliga NS, Wang JT, Ramage D, et al. Cytoscape: a software environment for integrated models of biomolecular interaction networks. Genome Res (2003) 13(11):2498–504. doi: 10.1101/gr.1239303
16. Bader GD, Hogue CW. An automated method for finding molecular complexes in large protein interaction networks. BMC Bioinf (2003) 4:2. doi: 10.1186/1471-2105-4-2
17. Chin CH, Chen SH, Wu HH, Ho CW, Ko MT, Lin CY. cytoHubba: identifying hub objects and sub-networks from complex interactome. BMC Syst Biol (2014) 8 Suppl:4, S11. doi: 10.1186/1752-0509-8-S4-S11
18. Cotto KC, Wagner AH, Feng YY, Kiwala S, Coffman AC, Spies G, et al. DGIdb 3.0: a redesign and expansion of the drug-gene interaction database. Nucleic Acids Res (2018) 46(D1):D1068–73. doi: 10.1093/nar/gkx1143
19. Du L, Kijlstra A, Yang P. Immune response genes in uveitis. Ocul Immunol Inflamm (2009) 17(4):249–56. doi: 10.1080/09273940902999356
20. Rao NA. Mechanisms of inflammatory response in sympathetic ophthalmia and VKH syndrome. Eye (Lond) (1997) 11(Pt 2):213–6. doi: 10.1038/eye.1997.54
21. Lee RW, Nicholson LB, Sen HN, Chan CC, Wei L, Nussenblatt RB, et al. Autoimmune and autoinflammatory mechanisms in uveitis. Semin Immunopathol (2014) 36(5):581–94. doi: 10.1007/s00281-014-0433-9
22. Das D, Akhtar S, Kurra S, Gupta S, Sharma A. Emerging role of immune cell network in autoimmune skin disorders: An update on pemphigus, vitiligo and psoriasis. Cytokine Growth Factor Rev (2019) 45:35–44. doi: 10.1016/j.cytogfr.2019.01.001
23. Gutcher I, Becher B. APC-derived cytokines and T cell polarization in autoimmune inflammation. J Clin Invest (2007) 117(5):1119–27. doi: 10.1172/JCI31720
24. Miranda ER, Fuller KNZ, Perkins RK, Beisswenger PJ, Farabi SS, Quinn L, et al. Divergent Changes in Plasma AGEs and sRAGE Isoforms Following an Overnight Fast in T1DM. Nutrients (2019) 11(2):386. doi: 10.3390/nu11020386
25. Sawalha AH, Wang L, Nadig A, Somers EC, McCune WJ, Michigan Lupus C, et al. Sex-specific differences in the relationship between genetic susceptibility, T cell DNA demethylation and lupus flare severity. J Autoimmun (2012) 38(2-3):J216–22. doi: 10.1016/j.jaut.2011.11.008
26. Tao W, Ayala-Haedo JA, Field MG, Pelaez D, Wester ST. RNA-Sequencing Gene Expression Profiling of Orbital Adipose-Derived Stem Cell Population Implicate HOX Genes and WNT Signaling Dysregulation in the Pathogenesis of Thyroid-Associated Orbitopathy. Invest Ophthalmol Vis Sci (2017) 58(14):6146–58. doi: 10.1167/iovs.17-22237
27. Chen JQ, Szodoray P, Zeher M. Toll-Like Receptor Pathways in Autoimmune Diseases. Clin Rev Allergy Immunol (2016) 50(1):1–17. doi: 10.1007/s12016-015-8473-z
28. Han BK, Olsen NJ, Bottaro A. The CD27-CD70 pathway and pathogenesis of autoimmune disease. Semin Arthritis Rheumatol (2016) 45(4):496–501. doi: 10.1016/j.semarthrit.2015.08.001
29. Karnell JL, Rieder SA, Ettinger R, Kolbeck R. Targeting the CD40-CD40L pathway in autoimmune diseases: Humoral immunity and beyond. Adv Drug Delivery Rev (2019) 141:92–103. doi: 10.1016/j.addr.2018.12.005
30. Zhang SY, Boisson-Dupuis S, Chapgier A, Yang K, Bustamante J, Puel A, et al. Inborn errors of interferon (IFN)-mediated immunity in humans: insights into the respective roles of IFN-alpha/beta, IFN-gamma, and IFN-lambda in host defense. Immunol Rev (2008) 226:29–40. doi: 10.1111/j.1600-065X.2008.00698.x
31. Commodaro AG, Peron JP, Genre J, Arslanian C, Sanches L, Muccioli C, et al. IL-10 and TGF-beta immunoregulatory cytokines rather than natural regulatory T cells are associated with the resolution phase of Vogt-Koyanagi-Harada (VKH) syndrome. Scand J Immunol (2010) 72(1):31–7. doi: 10.1111/j.1365-3083.2010.02401.x
32. Amadi-Obi A, Yu CR, Liu X, Mahdi RM, Clarke GL, Nussenblatt RB, et al. TH17 cells contribute to uveitis and scleritis and are expanded by IL-2 and inhibited by IL-27/STAT1. Nat Med (2007) 13(6):711–8. doi: 10.1038/nm1585
33. Imai Y, Sugita M, Nakamura S, Toriyama S, Ohno S. Cytokine production and helper T cell subsets in Vogt-Koyanagi-Harada’s disease. Curr Eye Res (2001) 22(4):312–8. doi: 10.1076/ceyr.22.4.312.5510
34. Chen L, Yang P, Zhou H, He H, Ren X, Chi W, et al. Diminished frequency and function of CD4+CD25high regulatory T cells associated with active uveitis in Vogt-Koyanagi-Harada syndrome. Invest Ophthalmol Vis Sci (2008) 49(8):3475–82. doi: 10.1167/iovs.08-1793
35. Horie Y, Kitaichi N, Takemoto Y, Namba K, Yoshida K, Hirose S, et al. Polymorphism of IFN-gamma gene and Vogt-Koyanagi-Harada disease. Mol Vis (2007) 13:2334–8.
36. Chen W, Lin H, Zhong X, Liu Z, Geng Y, Xie C, et al. Discrepant expression of cytokines in inflammation- and age-related cataract patients. PloS One (2014) 9(10):e109647. doi: 10.1371/journal.pone.0109647
37. Kishimoto T. IL-6: from its discovery to clinical applications. Int Immunol (2010) 22(5):347–52. doi: 10.1093/intimm/dxq030
38. Garbers C, Heink S, Korn T, Rose-John S. Interleukin-6: designing specific therapeutics for a complex cytokine. Nat Rev Drug Discovery (2018) 17(6):395–412. doi: 10.1038/nrd.2018.45
39. Hunter CA, Jones SA. IL-6 as a keystone cytokine in health and disease. Nat Immunol (2015) 16(5):448–57. doi: 10.1038/ni.3153
40. Bonacini M, Soriano A, Cimino L, De Simone L, Bolletta E, Gozzi F, et al. Cytokine Profiling in Aqueous Humor Samples From Patients With Non-Infectious Uveitis Associated With Systemic Inflammatory Diseases. Front Immunol (2020) 11:358. doi: 10.3389/fimmu.2020.00358
41. Liang L, Peng XY, Wang H. Th lymphocyte subsets in patients with Vogt-Koyanagi-Harada disease. Int J Ophthalmol (2019) 12(2):207–11. doi: 10.18240/ijo.2019.02.04
42. Liang L, Tan X, Zhou Q, Tian Y, Kijlstra A, Yang P. TLR3 and TLR4 But not TLR2 are Involved in Vogt-Koyanagi- Harada Disease by Triggering Proinflammatory Cytokines Production Through Promoting the Production of Mitochondrial Reactive Oxygen Species. Curr Mol Med (2015) 15(6):529–42. doi: 10.2174/1566524015666150731095611
43. Shao K, Shen LS, Li HH, Huang S, Zhang Y. Systematic-analysis of mRNA expression profiles in skeletal muscle of patients with type II diabetes: The glucocorticoid was central in pathogenesis. J Cell Physiol (2018) 233(5):4068–76. doi: 10.1002/jcp.26174
44. Liu H, Chen D, Liu P, Xu S, Lin X, Zeng R. Secondary analysis of existing microarray data reveals potential gene drivers of cutaneous squamous cell carcinoma. J Cell Physiol (2019) 234(9):15270–8. doi: 10.1002/jcp.28172
45. Molto L, Carballido J, Manzano L, Reyes E, Olivier C, Alvarez-Mon M. Prophylactic intracavitary treatment with interferon alpha increases interferon gamma production by peripheral blood mononuclear cells in patients with superficial transitional cell carcinoma of the bladder. Br J Cancer (1997) 75(12):1849–53. doi: 10.1038/bjc.1997.315
46. Zhang Y, Khoo HE, Esuvaranathan K. Effects of bacillus Calmette-Guerin and interferon alpha-2B on cytokine production in human bladder cancer cell lines. J Urol (1999) 161(3):977–83. doi: 10.1016/S0022-5347(01)61832-9
47. Chow KT, Gale M Jr. SnapShot: Interferon Signaling. Cell (2015) 163(7):1808–1808 e1801. doi: 10.1016/j.cell.2015.12.008
49. Mathian A, Amoura Z, Adam E, Colaone F, Hoekman MF, Dhellin O, et al. Active immunisation of human interferon alpha transgenic mice with a human interferon alpha Kinoid induces antibodies that neutralise interferon alpha in sera from patients with systemic lupus erythematosus. Ann Rheum Dis (2011) 70(6):1138–43. doi: 10.1136/ard.2010.141101
50. Plskova J, Greiner K, Muckersie E, Duncan L, Forrester JV. Interferon-alpha: a key factor in autoimmune disease? Invest Ophthalmol Vis Sci (2006) 47(9):3946–50. doi: 10.1167/iovs.06-0058
51. Proctor SJ. alpha Interferon therapy in the treatment of idiopathic thrombocytopenic purpura. Eur J Cancer (1991) 27 Suppl. 4:S63–8. doi: 10.1016/0277-5379(91)90577-z
52. Sanchez Roman J, Pulido Aguilera MC, Castillo Palma MJ, Ocana Medina C, Toral Pena A, Lopez-Checa F, et al. [The use of interferon alfa-2r in the treatment of autoimmune uveitis (primary or associated with Behcet’s disease)]. Rev Clin Esp (1996) 196(5):293–8.
53. Demiroglu H, Ozcebe OI, Barista I, Dundar S, Eldem B. Interferon alfa-2b, colchicine, and benzathine penicillin versus colchicine and benzathine penicillin in Behcet’s disease: a randomised trial. Lancet (2000) 355(9204):605–9. doi: 10.1016/S0140-6736(99)05131-4
54. Butler NJ, Suhler EB, Rosenbaum JT. Interferon alpha 2b in the treatment of uveitic cystoid macular edema. Ocul Immunol Inflamm (2012) 20(2):86–90. doi: 10.3109/09273948.2011.645989
55. Nussenblatt RB, Palestine AG, Rook AH, Scher I, Wacker WB, Gery I. Treatment of intraocular inflammatory disease with cyclosporin A. Lancet (1983) 2(8344):235–8. doi: 10.1016/s0140-6736(83)90230-1
Keywords: Vogt-Koyanagi-Harada disease, pathway analysis, network analysis, drug repurposing, uveitis
Citation: Chen Z, Zhong Z, Zhang W, Su G and Yang P (2020) Integrated Analysis of Key Pathways and Drug Targets Associated With Vogt-Koyanagi-Harada Disease. Front. Immunol. 11:587443. doi: 10.3389/fimmu.2020.587443
Received: 28 July 2020; Accepted: 11 November 2020;
Published: 15 December 2020.
Edited by:
Yoshihiko Usui, Tokyo Medical University Hospital, JapanReviewed by:
Chunfu Zheng, Fujian Medical University, ChinaHoward A. Young, National Cancer Institute at Frederick, United States
Vishali Gupta, Post Graduate Institute of Medical Education and Research (PGIMER), India
Copyright © 2020 Chen, Zhong, Zhang, Su and Yang. This is an open-access article distributed under the terms of the Creative Commons Attribution License (CC BY). The use, distribution or reproduction in other forums is permitted, provided the original author(s) and the copyright owner(s) are credited and that the original publication in this journal is cited, in accordance with accepted academic practice. No use, distribution or reproduction is permitted which does not comply with these terms.
*Correspondence: Peizeng Yang, cGVpemVuZ3ljbXVAMTI2LmNvbQ==
†These authors have contributed equally to this work