- 1Cytokine Signaling Unit, Department of Immunology, Institut Pasteur, Paris, France
- 2INSERM U1221, Department of Immunology, Institut Pasteur, Paris, France
- 3Center of Bioinformatics, Biostatistics and Integrative Biology, Institut Pasteur, Paris, France
- 4Translational Immunology Laboratory, Department of Immunology, Institut Pasteur, Paris, France
- 5Department of Neurology, Pitié-Salpêtrière Hospital, Paris, France
Background: Interferon beta (IFNβ) has been prescribed as a first-line disease-modifying therapy for relapsing-remitting multiple sclerosis (RRMS) for nearly three decades. However, there is still a lack of treatment response markers that correlate with the clinical outcome of patients.
Aim: To determine a combination of cellular and molecular blood signatures associated with the efficacy of IFNβ treatment using an integrated approach.
Methods: The immune status of 40 RRMS patients, 15 of whom were untreated and 25 that received IFNβ1a treatment (15 responders, 10 non-responders), was investigated by phenotyping regulatory CD4+ T cells and naïve/memory T cell subsets, by measurement of circulating IFNα/β proteins with digital ELISA (Simoa) and analysis of ~600 immune related genes including 159 interferon-stimulated genes (ISGs) with the Nanostring technology. The potential impact of HLA class II gene variation in treatment responsiveness was investigated by genotyping HLA-DRB1, -DRB3,4,5, -DQA1, and -DQB1, using as a control population the Milieu Interieur cohort of 1,000 French healthy donors.
Results: Clinical responders and non-responders displayed similar plasma levels of IFNβ and similar ISG profiles. However, non-responders mainly differed from other subject groups with reduced circulating naïve regulatory T cells, enhanced terminally differentiated effector memory CD4+ TEMRA cells, and altered expression of at least six genes with immunoregulatory function. Moreover, non-responders were enriched for HLA-DQB1 genotypes encoding DQ8 and DQ2 serotypes. Interestingly, these two serotypes are associated with type 1 diabetes and celiac disease. Overall, the immune signatures of non-responders suggest an active disease that is resistant to therapeutic IFNβ, and in which CD4+ T cells, likely restricted by DQ8 and/or DQ2, exert enhanced autoreactive and bystander inflammatory activities.
Introduction
Multiple sclerosis (MS) is an autoimmune and inflammatory disease of the central nervous system (CNS), leading to axonal demyelination, neuronal dysfunction, and neurodegeneration. These damages result from repeated attacks of several innate and adaptive immune cell types which have crossed blood–CNS barriers and exert a pathogenic activity together with resident activated microglia and macrophages (1, 2). Relapsing-remitting MS (RRMS), the most common form of the disease mainly affecting young adults with a female to male ratio of ~2.5–3 (3, 4), has been treated by type I interferon beta (IFNβ) for nearly three decades. To date, IFNβ and its highly stable pegylated form remain widely prescribed as a first-line disease-modifying therapy. Recent meta-analyses performed in a ‘real-world’ setting have confirmed the long-term efficacy of IFNβ in delaying disability and disease progression, and decreasing mortality risk (5, 6). However, ~30% of patients are or become non-responsive to the treatment while still being potentially subject to side effects (7). Given the increasing number of novel and targeted therapeutic options for RRMS, including injectable or oral first-line therapies (8, 9), it is critical to identify IFN treatment response biomarkers and better understand the mechanisms of disease onset and pathogenesis.
Susceptibility to MS is under the influence of genetic heritability [~50% of overall risk (10)] as well as of environmental and lifestyle factors (11). Non-genetic factors such as gender, Epstein–Barr virus infection, smoking, low vitamin D, or adolescent obesity are considered to contribute to, or to potentially trigger, disease onset. Recent large-scale genome-wide association studies uncovered up to 233 independent genetic associations with MS, 30 of these mapping across the MHC region (10, 12, 13). Most variants are related to the adaptive immunity, with a group of HLA class II allelic risk variants, dominated by HLA DRB1*15:01 (OR~3.9) (13–15). Moreover, the interaction between the latter variant and non-genetic risk factors leads to a much higher susceptibility to develop MS (11).
Immune dysregulation is a hallmark of MS pathogenesis. Key players are CD4+ and CD8+ T cells that drive autoreactive and deleterious responses within the CNS while also promoting activation of myeloid and B cells. Notably, the association between specific HLA class II variants and MS points not only to the critical role of autoreactive CD4+ T cells whose T cell receptor is restricted by these variants, but also to antigen-presenting cells expressing the variants and among them B cells. In fact, B cells were reported to drive T cell autoproliferation in RRMS patients bearing the HLA-DR15 haplotype and to contribute to autoimmune and pro-inflammatory cytokine responses (16–18). Their key role in MS pathophysiology is demonstrated by the impressive therapeutic effect of anti-CD20-based treatments (19).
Autoreactive cells can be activated by CNS and non-CNS derived antigens through various mechanisms such as molecular mimicry following viral reactivation, recognition of neo-autoantigens and/or bystander activation (1, 17, 20). In addition, autoreactivity and pro-inflammatory T cell responses can be promoted by dysfunctional regulatory mechanisms, such as those involving peripheral CD4+ regulatory T cells (Tregs) and type 1 regulatory T cells (Tr1s) (21–23). Reduced thymic output of naïve Treg cells in RRMS patients may also indicate a defect of central tolerance mechanisms or an alteration of Treg homeostasis (24, 25). Conversely, effector CD4+ T helper subsets such as Th17 and Th1/Th17 are increased in the periphery, display enhanced pro-inflammatory cytokine and gene expression programs, and may be more resistant to Treg activity (26–28). Circulating cytotoxic CD28− CD4+ T cells were also found to be expanded and to correlate with disease activity (29–31). Finally, CD8+ T cells are considered to play an important role in MS pathogenesis. These cells are enriched in cerebrospinal fluid (CSF) and CNS lesions and can be detected in the periphery with an activated effector/migratory phenotype (2, 32).
The therapeutic activity of IFNβ is largely attributed to the induction of a global anti-inflammatory program although its direct antiviral and pro-apoptotic activities may also contribute (20, 33, 34). Various mechanisms of action involving almost all immune cell types have been proposed. Among the major immunomodulatory effects of IFNβ treatment are the restoration or induction of regulatory T and B cell responses (22, 35–37), the reduced differentiation of inflammatory Th17 and B cells, and the attenuation of monocyte activation (33, 36, 38, 39). Many studies have also shown the strong promoting activity of IFNα/β on IL-10 expression (33, 40, 41).
Different findings have been reported on the frequency of circulating Treg, naïve and memory CD4+ and CD8+ T cell subsets in untreated and IFN-treated RRMS. The rapid evolution of phenotyping procedures, heterogeneous clinical features of patients, and the duration of IFN treatment may account for data variability. To date, no single blood biomarker can predict the therapeutic efficacy of IFNβ nor disease activity (42). On this basis, we have explored the possibility that a combination of cellular and molecular blood biomarkers may prove valuable for patient stratification. We compared the immune status of IFN responders and non-responders with that of untreated RRMS patients and healthy donors. We used an integrated approach by analyzing circulating CD4+ and CD8+ T cell subsets, mRNA expression of IFN-stimulated genes (ISGs) and other immune genes, and allelic variation of HLA class II genes. Results of this exploratory study show converging immune signatures in non-responders, suggesting dysregulation of the immune response and higher disease activity in these patients.
Materials and Methods
MS Patients and Healthy Controls
RRMS patients of Caucasian ethnicity were diagnosed according to the 2010 McDonald criteria (43) and were recruited at the hospital Pitié-Salpêtrière, Paris. Untreated patients did not receive any immunomodulatory or immunosuppressive treatment at least 3 months prior to blood collection. Patients treated with IFNβ1a Avonex (30 µg, IM, once a week) were considered as non-responders if they experienced one or more relapses during the last year of treatment. Blood was collected at least two days after IFN administration on lithium heparin-tubes for flow cytometry and gene expression assays and on EDTA-tubes for genomic DNA extraction and plasma cytokine analysis. Exclusion criteria were disease activity, steroidal anti-inflammatory or immunosuppressive drugs, antibiotics, acute or chronic infectious diseases, autoimmune and inflammatory diseases other than MS, and cancer. The study was approved by the CPP 2014/17NICB and the CNIL MMS/CWR/AR1411558. Healthy controls were from the CoSImmGEn cohort of the ICAReB platform (Clinical Investigation and Access to BioResources, Institut Pasteur) and EFS (Etablissement Français du Sang, Paris). For HLA class II genotyping, controls were from the Milieu Interieur (MI) cohort composed of 1,000 healthy donors, French citizens with metropolitan French origin for three generations (https://clinicaltrials.gov; NCT01699893 and NCT03905993, ANR-10-LABX-69-01). MI healthy controls and RRMS patients provided written informed consent including genetic analyses. Clinical and demographic characteristics of participants are shown in Table 1.
Flow Cytometry
Blood (200 µl, sampling <6 h) was washed with PBS at 1,500 rpm, 5 min, room temperature (RT). Cell pellet was incubated with antibodies premix for 20 min at RT then with viability dye (500 µl, 1/1,000) at 4°C for 30 min (eF506, eBioscience). After washing cells with cold PBS, red cells were lysed and leukocytes were fixed in 2 ml of lysis buffer (BD biosciences) for 15 min at RT in the dark. Stained cells were acquired in 200 µl PBS using MACSQuant® Analyzer 10 (Miltenyi Biotech). CD4+ and CD8+ T cell subsets were analyzed using FlowJo™10 by gating on CD3+ cells after exclusion of dead cells and doublets. The following antibodies were used in two eight-color panels: anti-CD3-Vioblue (BW264/5), anti-CD4-APC-vio770 (VIT4), anti-CD45RA-FITC (T6D1, anti-CD8b-PE-Cy7 (SIDI8BEE), anti-CD25-PerCPeF710 (CD25-4E3), anti-CD27-PerCPvio700 (M-T271), anti-HLADR-PE (clone AC122), anti-CD127-APC (MB15-18C9) (Miltenyi Biotec, eBioscience). Treg, CD4+ and CD8+ T cell subsets were gated using the appropriate FMO control.
IFNα/β Measurement
Plasma was obtained by centrifugation of blood at 1,500 rpm, 5 min, RT, and frozen at −80°C. IFNα and IFNβ plasma levels were measured in duplicate by single molecule array (Simoa, Quanterix) digital ELISA using homebrew assays in which capture and detection monoclonal antibodies were 8H1 and 12H5 (Immunoqure AG) for IFNα (41), and 710322-9 and 710323-9 IgG1 for IFNβ (PBL Assay Science) (44).
mRNA Gene Expression
Prewarmed blood (1 ml) was incubated into TruCulture tubes (Myriad RBM) under a final volume of 3 ml, at 37°C, for 22 h. Trizol LS (Qiagen)-lyzed cell pellets were thawed on ice at least 1 h, vortexed twice at 2,250 rpm for 5 min and centrifuged at 3,500 g for 5 min at 4°C. Total RNA was extracted using nucleospin miRNA kit (Macherey-Nagel), eluted in 30 µl RNase-free water and aliquots were stored at −80°C. RNA quality was measured with NanoDrop™2000 (ThermoFisher) and the Agilent 2100 bioanalyzer (RNA 6000 Nano kit). mRNAs were quantified using the Nanostring (nCounter) technology. For that, RNA (100 ng, 20 ng/µl) was hybridized on 12-sample strips at 65°C for 16 h using the Human immunology_V2 (579 genes) codeset and a custom 9 gene codeset (ADAR1, HERC5, ISG15, IRF2, IRF9, RIG-I, HLA-DQA1, HLA-DQB1, HLA-DRB4). RNA/probe complexes were immobilized on a cartridge with the ‘Prep station’ and quantified by the ‘nCounter system’ within 555 fields of views. Data were normalized to internal positive and negative controls. TBP, POLR2A, SDHA, G6PD housekeeping genes were determined with the algorithm ‘gNorm’ (nSolver software V4). Data were analyzed using a background threshold of 15 counts and were log2-transformed for Qlucore Omics Explorer analysis (V3.4). Geomean scores of ISG were determined for each patient according to (45) after normalization of mRNA counts from human_V2 and custom codesets.
HLA Class II Genotyping
Genomic DNA was extracted from 2 ml EDTA-blood of RRMS patients using the Nucleon BACC3 kit (GE-Healthcare) according to the manufacturer’s instructions. Briefly, blood cells were lysed at RT, and pellet was stored at −80°C. Precipitated DNA was airdried for 10 min, resuspended in DNAse/RNAse free water overnight at 4°C and quantified using Qubit dsDNA HS Assay Kit and Qubit 4 Fluorometer (Invitrogen). Class II HLA-DQA1, DQB1, DRB1, and DRB3/4/5 genotypes were determined by single molecule real time sequencing of exons 2–6 and introns 2–5 at 8× resolution (Histogenetics, USA). Genotypes were converted to serotypes according to the 2010 nomenclature of HLA system (46). HLA-DRB1, -DQA1 and -DQB1 typing data of 1,000 healthy donors were a resource of the Milieu Interieur consortium. Alleles were imputed at four-digit resolution from the analysis of 5,699,237 SNPs using SNP2HLA v1.0370 (47).
Statistical Analyses
One-way ANOVA Kruskal–Wallis with Dunn’s correction for multiple comparisons or Mann–Whitney test was utilized for scatter bar plots showing flow cytometry, gene expression and IFNα/β level data (GraphPAD Prism 8). ANOVA multigroup comparison F-test was used for analysis of gene expression shown as heatmaps (Qlucore Omics Explorer V3.4). The distribution of HLA binding and non-binding probes (Nanostring assays) was analyzed using a generalized linear model of the binomial family followed by pairwise comparisons among groups using Tukey-like correction. HLA typing data were analyzed by pairwise comparisons of frequencies between the Milieu Interieur data set (n = 1,000 healthy donors) and other groups using a Fisher’s test. P-values were adjusted to account for multiple testing (Center of Bioinformatics, Biostatistics and Integrative Biology (C3BI, Institut Pasteur).
Results
Peripheral Blood T Cell Phenotypes in IFNβ-Treated RRMS Patients
We investigated cellular and molecular immune phenotypes in IFNβ-treated RRMS patients who were clinically defined as responders (Resp, n = 15) and non-responders (NR, n = 10). All patients received IFNβ1a IM (Avonex, 30 µg, once a week), which minimized possible variation of the treatment response due to dose, frequency, and type of IFNβ. Controls were untreated patients (UT, n = 15) and healthy controls (HC, n = 14) of similar age and sex ratio. All patients presented with a mild disease score (medians EDSS, 0–1.8) and were of Caucasian ethnicity (Table 1 and Materials and Methods).
Number and frequency of regulatory T cells (Tregs), conventional CD4+ T cells (Tconvs), and naïve/memory CD4+ and CD8+ T cell subsets were monitored in whole blood by flow cytometry in the four subject groups (Figures 1A, B for gating strategies and Supplementary Tables 1, 2). Based on differential expression of CD25 and CD127, we found that IFN responders displayed a significantly higher number of Treg and Tconv cells as compared to healthy donors (p < 0.05 and p < 0.005, respectively) with unchanged Treg/Tconv ratio (Figure 1A). In line with this, responders showed an increased number and frequency of total CD4+ T cells while the frequency of CD8+ T cells tended to be decreased in all patient groups (Supplementary Figure 1). Additional gating on Treg cells using CD45RA and HLA-DR identified naïve, memory and activated/terminally differentiated subsets, respectively equipped with enhanced suppressive activity potential (48). Non-responders showed a significant reduction in the number and frequency of naïve Tregs as compared with responders or healthy controls (p < 0.05) and decreased Treg/Tconv ratio (Figure 1A), while responders showed a trend towards increased number and frequency of memory and activated Treg subsets (Supplementary Figure 2D). Finally, untreated patients tended to have decreased number and frequency of activated Tregs (Supplementary Figure 2D).
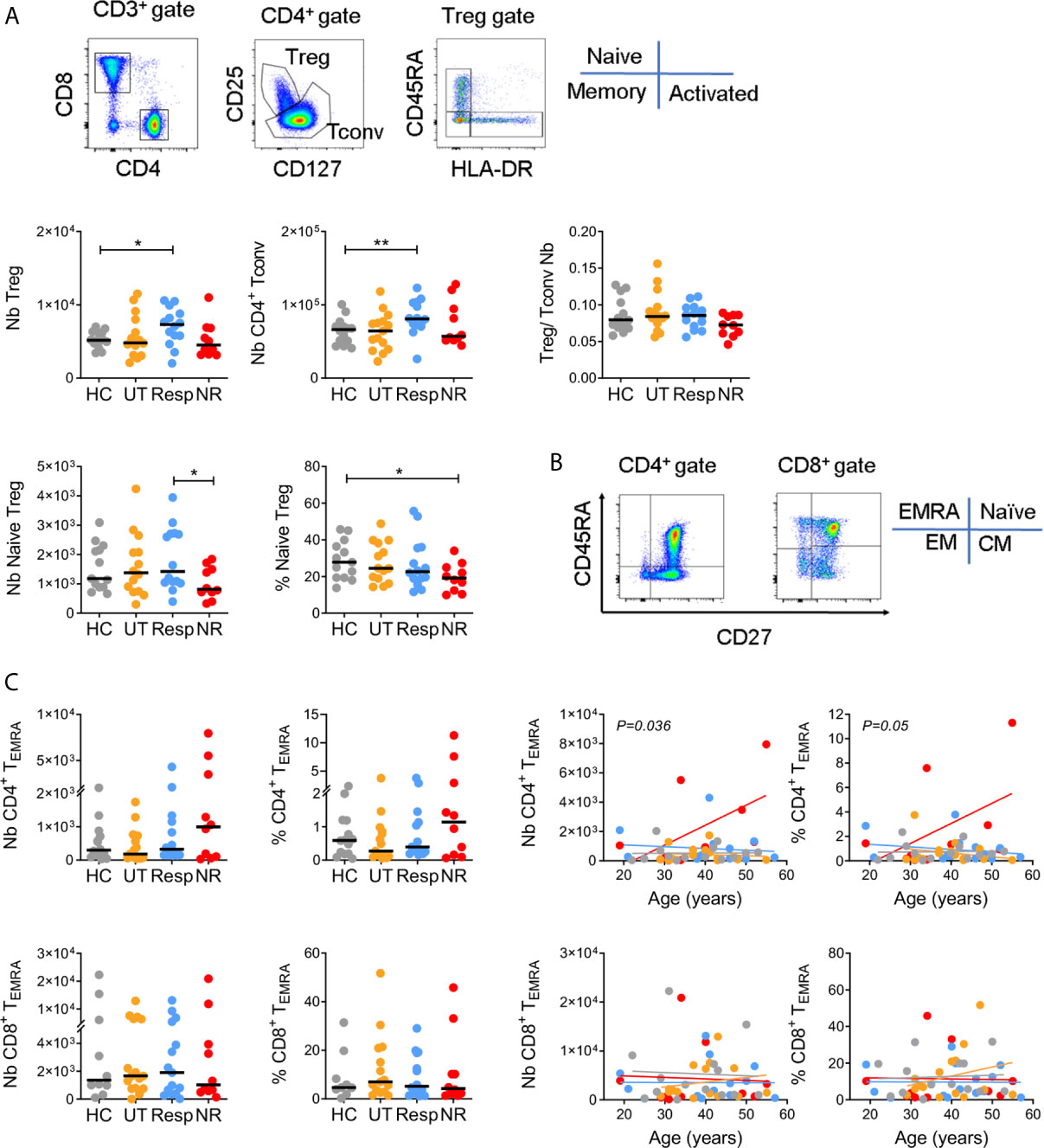
Figure 1 CD4+ T cell phenotypes in IFN responders and non-responders. (A) Gating strategy for CD4+ Treg and Tconv subsets. Upper panels: number (Nb) and Treg/Tconv ratios. Lower panels: number and frequency (%) of naive (CD45RA+ HLA-DR-) Tregs. Controls (HC = 13), untreated (UT = 14), responders (Resp = 14), non-responders (NR = 10). (B) Gating strategy for naïve (TNaive, CD45RA+ CD27+), central memory (TCM, CD45RA− CD27+), effector memory (TEM, CD45RA− CD27−), and terminally differentiated effector memory (TEMRA, CD45RA+ CD27−) subsets. (C) Left panels: number and frequency of CD4+ and CD8+ TEMRA. HC = 9–14, UT = 15, Resp = 14, NR = 10. Right panels: linear regressions between TEMRA cells and age. Indicated p values were obtained from NR and Resp comparison. (A, B) Horizontal lines represent medians. Mann–Whitney test, *p < 0.05, **p < 0.005.
Naïve/memory CD4+ and CD8+ T cells were analyzed based on differential expression of CD45RA and CD27 (Figure 1B). Alterations in numbers and frequencies of naïve, central (CM) and effector memory (EM) T cell subsets were moderate among subject groups (Supplementary Figures 2A, B and Supplementary Tables 1, 2). However and notably, non-responders displayed a higher number and frequency of terminally differentiated effector memory cells (CD4+ TEMRA) than other subject groups, in particular with age as compared to responders (p < 0.05 for cell number, Figure 1C). This was not the case for CD8+ TEMRA, which suggests a selective accumulation of CD4+ TEMRA cells in non-responders. Of note, given the strong association between CD4+ TEMRA cells and CMV seroprevalence in healthy donors (47), we measured CMV-specific IgG in non-responders and responders but found no significant difference between the two groups (not shown).
Altogether, alterations of circulating T cell subsets were mainly observed in non-responders within the Treg and CD4+ TEMRA compartments although we have to point out that differences were statistically significant only without correcting for multiple comparisons between subject groups.
Circulating IFNα/β Proteins and ISG Expression in IFNβ-Treated Patients
Next, we measured the plasma level of IFNβ and IFNα proteins and ISG expression in blood cells of IFN-treated patients. We first assessed patient adherence to the treatment by measuring circulating IFNβ using a Simoa digital ELISA. The level of IFNβ was similar, not statistically different, between responders and non-responders (median 120 and 75 pg/ml, respectively) and was undetectable in most untreated patients (Figure 2A). Circulating endogenous IFNα was also measured using anti-pan-IFNα antibodies of very high affinity (49). Interestingly, non-responders exhibited a moderate but significant increase in IFNα (2.2 fg/ml, 0.47–101) as compared to untreated patients (0.47 fg/ml, 0.47–18.6, p < 0.05), and healthy donors (0.47 fg/ml, 0.47–2.9, p < 0.005).
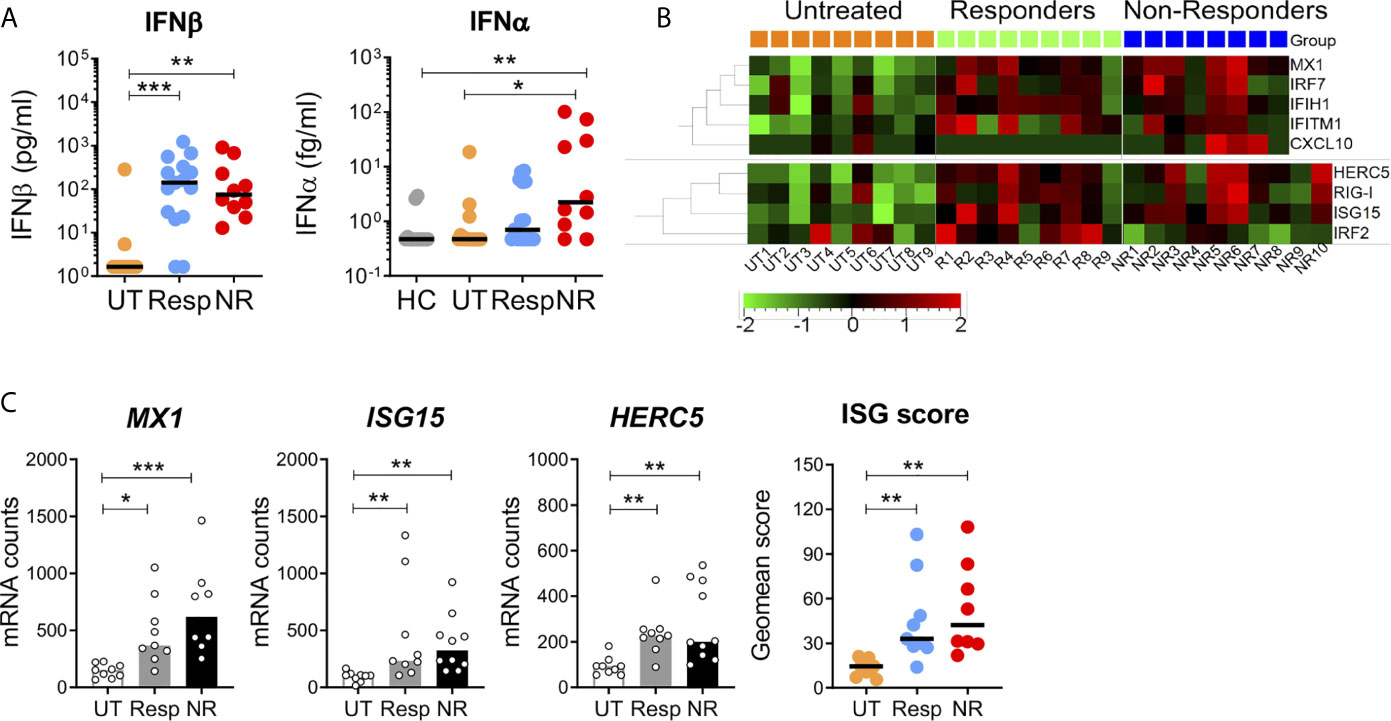
Figure 2 Circulating IFNα/β proteins and interferon-stimulated gene expression in blood cells. (A) The plasma levels of IFNα and IFNβ were measured by digital ELISA (Simoa). Lower limits of detection were 0.47 fg/ml and 1.64 pg/ml for IFNα and IFNβ, respectively. UT = 13–15, Resp = 15, NR = 10, HC = 14. (B) ISG mRNA expression in blood cells of untreated, responders and non-responders was measured with the Nanostring technology. Heatmap depicts relative mRNA counts after one-way Anova F-test, p < 0.05. Upper panel: human V2 codeset, UT = 9, Resp = 9, NR = 8. Lower panel: custom codeset, NR = 10. (C) Canonical ISGs (MX1, ISG15, HERC5) and geomean scores in patient groups. Kruskal–Wallis test with Dunn’s correction for multiple comparisons, *p < 0.05, **p < 0.005, ***p < 0.0005.
ISG induction was investigated in responders and non-responders with the Nanostring digital technology that allows direct mRNA counting by probe hybridization using the human immunology V2 codeset (579 genes) and a custom codeset (nine genes). Baseline mRNA expression of 159 ISGs was compared between the two groups and untreated patients. Among these, 153 ISGs were selected from previous Nanostring data obtained with in vitro IFNβ-stimulated blood of 25 healthy donors of the Milieu Interieur (MI) cohort (50). Analysis of ISG expression by hierarchical gene clustering and multiple comparison showed the upregulation of nine genes in IFN-treated patients (Figure 2B). Among these, three (MX1, ISG15, and HERC5) are canonical type I IFN-induced genes. Consistent with similar levels of circulating IFNβ in responders and non-responders, the three gene ISG scores did not significantly differ in the two groups but was higher than in the untreated patients (Figure 2C). A different analytical strategy, based on the high fold change of ISG induction (FC > 10) in MI healthy donors, revealed a 17 gene signature in IFN-treated patients. However, a large proportion of these genes were not canonical ISGs (Supplementary Figure 3). Altogether, these results point to MX1, ISG15, and HERC5 as good ISG markers for monitoring the IFN biological response in treated patients.
Altered Gene Expression in IFNβ Non-Responders, Including HLA Class II Genes
The expression of genes other than ISGs was analyzed by hierarchical clustering in patient groups (Figure 3A). Non-responders differed from responders and untreated patients by 15 downregulated genes (cluster A) and two upregulated genes. Of interest, significant reductions (p < 0.05) were observed for genes encoding cell surface receptors involved in the regulation of adaptive and innate immune responses (right scatter bar graphs). For instance, CD46 and TGFBR1 control peripheral induction of Foxp3− Tr1 and Foxp3+ Treg cells, respectively (23, 48). Interestingly, CD46-mediated induction of Tr1 cells has been reported to be altered in MS (23). IL-4R plays a crucial role in type 2 immunity, notably by promoting Th2 and B cell differentiation and by restraining neutrophil inflammatory function (51). IL-4R was also reported to dampen IL-1 response by upregulating the decoy receptor IL-1R2 (52). Consistently, a lower expression of IL4R and IL1R2 was observed in non-responders. Conversely, the two cytokine encoding genes, CSF1 and SPP1, were upregulated in non-responders. Interestingly, SPP1 (OPN) was found to be more expressed in MS patients and to correlate with disease activity (53). Most genes contained in cluster B were upregulated in both responders and non-responders as compared to untreated patients and thus were mainly indicative of a response to the IFN treatment. Yet, a few genes were significantly more expressed in responders (Supplementary Figure 4B).
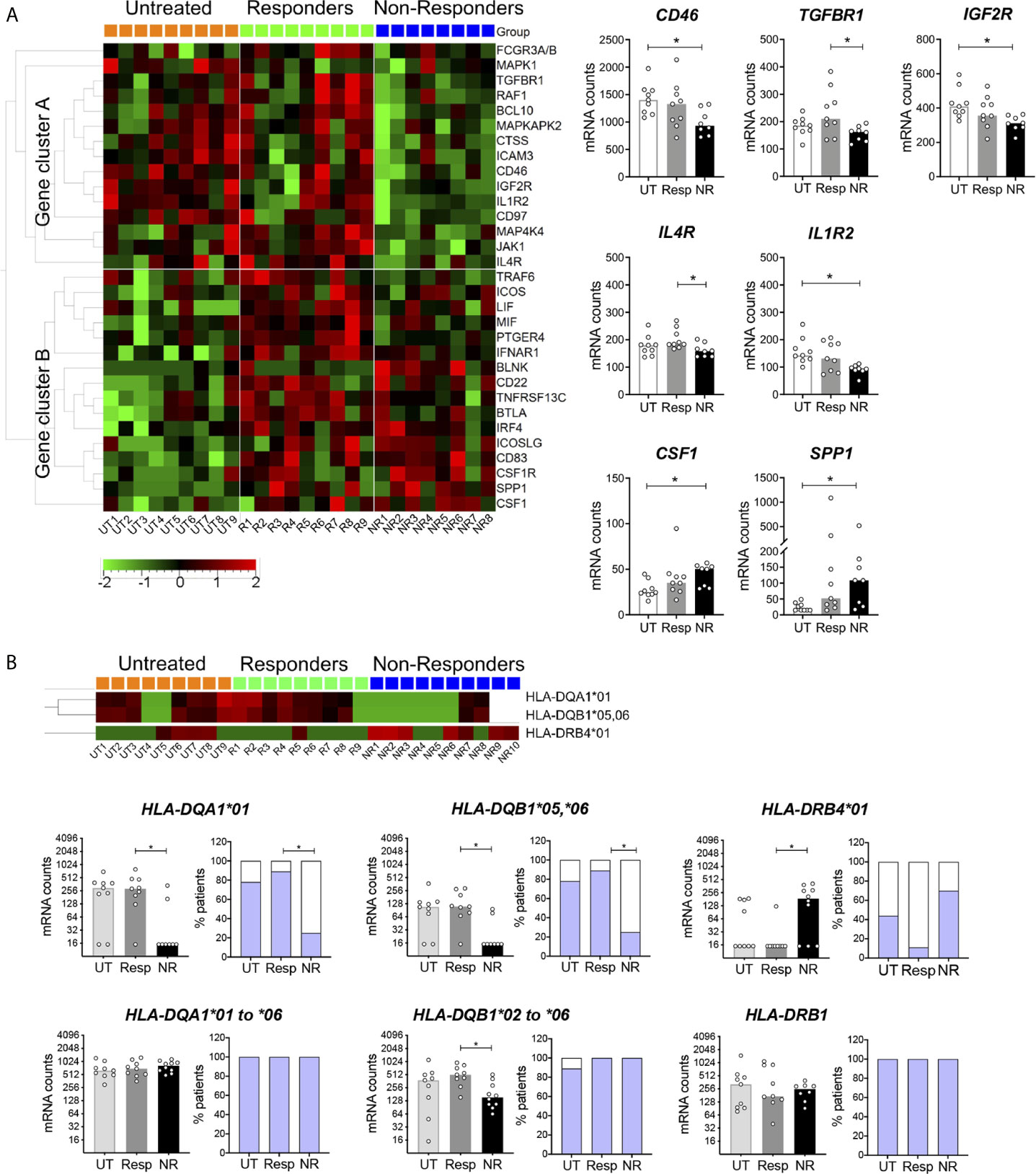
Figure 3 Differentially expressed genes other than ISGs in IFN non-responders. (A) Heatmap of mRNA expression in blood cells of patients measured with the Nanostring technology as in Figure 2. One-way Anova F-test, p < 0.05. UT = 9, Resp = 9, NR = 8–10. Right panels: genes encoding cell surface receptors and cytokine in non-responders. Kruskal–Wallis test with Dunn’s multiple comparisons, *p < 0.05. (B) Differential HLA-DQA1, DQB1, and DRB4 mRNA expression in non-responders. Kruskal–Wallis test with Dunn’s multiple comparisons, *p < 0.05. UT = 9, Resp = 9, NR = 8. Two-colored bar graphs show the proportion of patients for which HLA probe binding was observed (blue) or not (white). Pairwise comparison test followed by Tukey-like adjustment for multiple comparisons, *p < 0.05.
HLA gene expression was analyzed in patient groups, in particular classical HLA class II (HLA-DRA, DRB1, DRB3, DRB4, DQA1, DQB1, DPA1, DPB1) and class I (HLA-A, B, C) genes and non-classical class II genes (HLA-DMA, DMB, DOB). Based on sequence alignments, most probes were gene but not allele specific. However, DQA1 and DRB4 probes were preferentially directed against the *01 allele group, and the DQB1 probe was mainly directed against the *05 and *06 allele groups. We observed in the three patient groups a binary (all or none) mode of binding of these probes (Figure 3B) as well as in healthy controls (not shown). Notably, non-responders strongly differed from responders by a significantly lower frequency of DQA1*01, DQB1*05,*06 probe binding and a higher frequency of DRB4*01 probe binding (p < 0.05, Figure 3B, upper panels). Of note, the lack of binding of both DQA1 and DQB1 probes was observed in the same subjects (not shown). We designed additional probes targeting all DQA1 and DQB1 allele groups and confirmed mRNA expression in non-responders, even if fewer DQB1 mRNA counts were observed (Figure 3B, lower panels). Altogether, these data indicated differential allelic variation of HLA-DQA1, DQB1, and DRB4 in non-responders. To further examine this possibility, IFN-treated patients were genotyped for a series of HLA class II genes.
Increased Carriage of HLA-DQB1 Variants Encoding DQ8 and DQ2 Serotypes in IFNβ Non-Responders
Typing of HLA-DQA1, DQB1, DRB1, and DRB3,4,5 was achieved by sequencing at high resolution the available gDNA from responders (n = 10) and non-responders (n = 9). To increase statistical power, HLA typing data from the 1,000 MI healthy donors were utilized as a control reference population. First, the profile of HLA-DQA1, DQB1, and DRB1 four-digit allelic variants, obtained by imputation from a genome-wide SNP study of the MI cohort (47), was compared to that reported in a European-American reference cohort of healthy donors [n = 1,899 (54)]. The profiles of allele frequencies were similar between the two cohorts for DQB1 and DRB1, but not for DQA1, with the notable absence of DQA1*01:04 and DQA1*03:02 variants in the MI study (Figure 4A). Further analyses were focused on DQB1, DRB1, and DRB3,4,5 that encode DQβ and DRβ chains of the HLA α/β heterodimer. HLA-DQ and -DR serotypes were assigned from genotypes, and frequencies of serotype pairs were compared between responders, non-responders, and the MI 1,000 healthy controls. Strikingly, non-responders showed a marked and significant enrichment of genotypes corresponding to DQ2/DQ8 serotypes (p < 0.05, Figure 4B) and tended to carry DQA1 genotypes including DQA1*03 and DQA1*02:01 variants (Supplementary Table 3). Non-responders were also more frequently positive for DQ2/DQ7 serotypes though this appeared to be driven by the increased usage of DQ2 and not DQ7 (Figure 4C). In accordance with known genetic linkage between specific HLA class II gene variants (55), non-responders carrying DR4/DR7 serotypes also carried the DR53 serotype (Figure 4B). As opposed to these findings, responders and MI healthy controls displayed a high diversity in the usage of DQβ and DRβ chains. Overall, this genotyping analysis strongly suggests that non-responders utilize a distinct repertoire of HLA-DQ and possibly HLA-DR molecules.
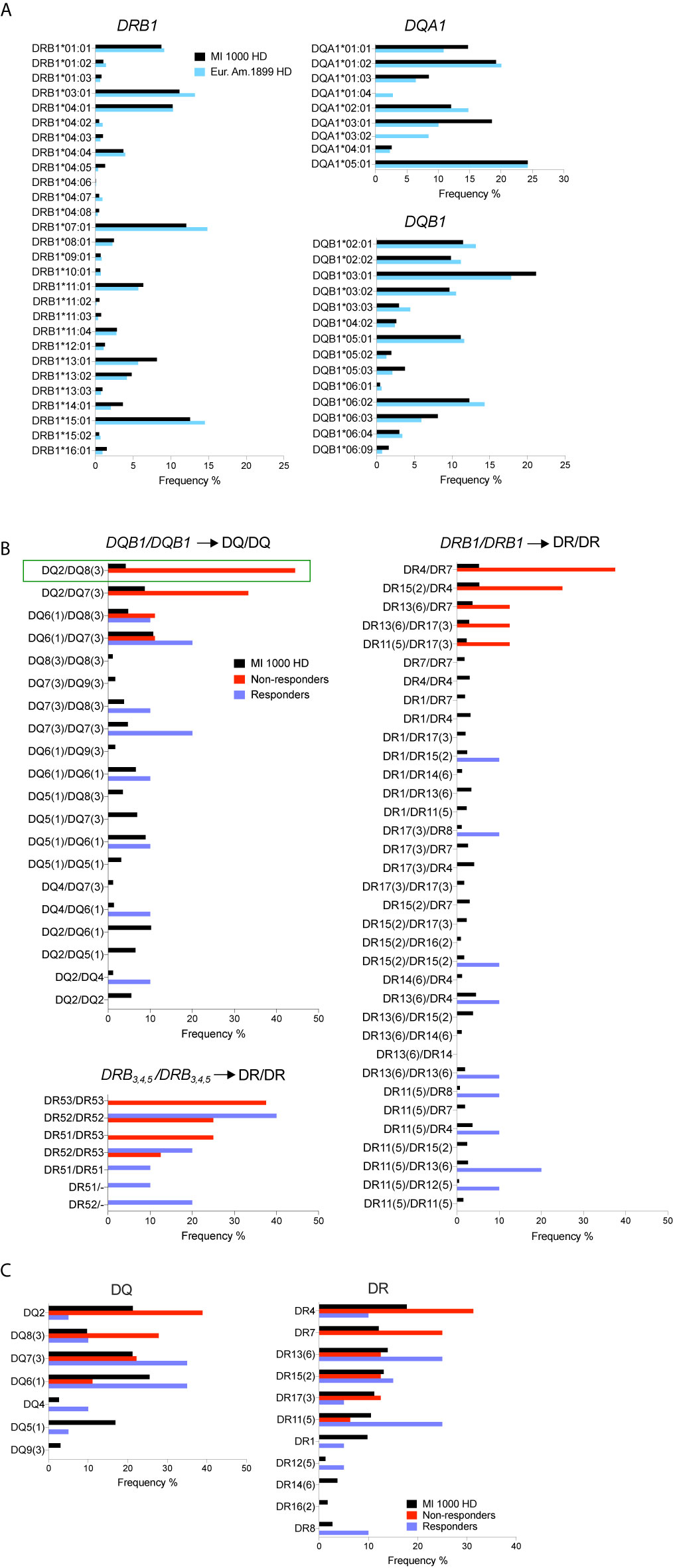
Figure 4 Increased carriage of HLA-DQB1genotypes encoding DQ2/DQ8 serotypes in non-responders. (A) Comparison of HLA-DRB1, DQA1, and DQB1 allele frequencies in the French Milieu Interieur (MI) cohort (n = 1,000) and in a European-American reference cohort [n = 1,899 (54)]. (B) Frequencies of DQ and DR serotype pairs in MI healthy controls, IFN non-responders and responders. (Resp = 10, NR = 9 and 8 for DR53). Pairwise comparison test between groups showed statistically significant difference of the DQ2/DQ8 frequency in NR vs MI HD (green rectangle) with *p < 0.05, adjusted for multiple comparisons. (C) Frequencies of DQ and DR serotypes in MI controls, IFN non-responders and responders.
Discussion
By using an integrated approach and sensitive technologies, we have made new observations related to the therapeutic efficacy of IFNβ in RRMS. Untreated patients and healthy controls globally showed modest differences, possibly explained by the mild MS disease score. On the other hand, non-responders and responders displayed distinct cellular and molecular immune phenotypes. Non-responders were characterized by a reduced number and frequency of naïve Tregs and higher number of CD4+ TEMRA cells, suggesting immune dysregulation in these patients. The mechanism underlying the reduction of naïve Tregs remains to be understood. These cells may have acquired an effector-like phenotype, as for the Th1-like Treg cells described in MS patients (22). Alternatively, the decreased thymic output of naive Tregs reported in untreated patients (24, 25) or an alteration of peripheral Treg homeostasis may be more pronounced in our non-responder patients. In contrast, responders showed a higher number and frequency of total Tregs, which was mainly reflected at the level of memory and activated Treg numbers. In line with this, therapeutic IFNβ has been proposed to promote redistribution within the Treg compartment towards memory Tregs (37, 56). Unexpectedly, we found an increase in the number of total CD4+ T cells in responders. This finding is consistent with studies showing that IFNβ restores thymic function or T cell homeostasis that is altered in untreated patients (35, 57). However, other studies reported fewer circulating T cells in IFN-treated patients (37, 58). Treatment duration and time of blood collection may explain this difference. Indeed, therapeutic IFNβ is known to induce a cytopenia depending on the dose and administration frequency (59). This effect is transient since it was observed during the first 6–12 months and resolved thereafter (60). In our study, responders were long-term treated (median 7 years) once a week, and blood was collected at least two days after IFN administration. In other studies, blood was collected earlier (<24 h) and IFNβ was administered several times per week.
Another T cell phenotype observed in non-responders was the increase in CD4+ TEMRA cells, in particular with age. This accumulation may be cytokine- (61) or HLA class II/antigen-driven, and it would be interesting to know whether some of these cells are autoreactive (17, 20, 30). In humans, most CD4+ TEMRA cells are CD28− (62). Interestingly, memory CD28− CD4+ T cells with a cytotoxic and pro-inflammatory potential were reported to be clonally expanded and to associate with MS progression (29–31). This suggests that CD4+ TEMRA cells enriched in non-responders may exert a deleterious activity.
Many studies have investigated gene expression in blood cells of IFN-treated patients, searching for a signature of IFN bioactivity and treatment response markers. However, no unified view has emerged, possibly due to variability of experimental settings (63–68). One correlate was proposed between elevated baseline ISGs, serum IFNβ prior to treatment and poor clinical outcome (65, 67, 69). In line with this, we found that non-responders displayed an increased expression of some ISGs, in particular MX1, a marker previously used to study IFN non-responders (56, 70, 71). Yet, a three canonical ISG score (MX1, ISG15, HERC5) or other scores based on several ISGs (Supplementary Figure 3) did not allow for distinguishing non-responders from responders but were consistent with similar levels of circulating IFNβ levels and IFNβ bioactivity in the two patient groups. Among other predictive markers associated with poor IFN bioactivity and therapeutic effect is the induction of neutralizing antibodies. Of interest for our study, Avonex was reported to be the least immunogenic preparation, affecting around less than 10% patients during the first 1–3 years of treatment (70, 72–74). Thus, it is likely that a significant impact of neutralizing antibodies was not well appreciated due to the limited number of studied patients (n = 10–15/group).
The analysis of immune related genes other than ISGs led to novel observations. Non-responders were characterized by a cluster of 15 downregulated genes, including cell surface receptors (e.g. CD46, TGFR1, IL4R, IL1R2) and two upregulated cytokines (CSF1, SPP1) with an immunomodulatory function. Among these genes, CD46 and SPP1 have been documented to be involved in MS pathogenesis (23, 53). Together with the alteration of circulating Treg and CD4+ TEMRA cell subsets, these results indicate some level of immune dysregulation in non-responders.
HLA allelic gene variation is known to mainly impact the antigen-binding groove formed by the α/β HLA class II heterodimer, which can result in modifications of the affinity or stability of the peptide-HLA complex and, potentially, the CD4+ T cell repertoire (75). Importantly, we found remarkable differences between patient groups for HLA class II gene variation. First, the binary pattern of HLA-DQA1, DQB1, and DRB4 mRNA expression was clearly altered in non-responders as compared to untreated and responder patients. Second, the profile of HLA class II genotypes markedly differed between non-responders and 1,000 healthy donors (MI cohort).
In European populations, at least six HLA class II risk variants have been reported for MS (14, 15). The strongest one, HLA-DRB1*15:01, is part of the extended haplotype DRB5*01:01-DRB1*15:01-DQA1*01:02-DQB1*06:02 that corresponds to DR51–DR15–DQ6 serotypes. In our study, non-responders did not significantly differ from MI controls and responders for the carriage of DRB1*15:01 encoding the DR15 serotype, but they tended to be enriched for DR4/DR7 and DR53 serotypes.
The most striking result was obtained for DQB1 in non-responders who showed a significant enrichment of DQ8/DQ2 serotypes and mainly carried DQA1 genotypes including DQA1*03 and DQA1*02:01 allelic variants. The enrichment of DQ8 in non-responders is consistent with two recent studies (13, 76). In a meta-analysis of HLA allelic variation performed with multiple MS cohorts of European ancestry, DQB1*03:02, the single allele encoding DQ8, was identified as a dominant risk for MS. DQB1*03:02 was also found to be counteracted by the interaction with DQB1*03:01 (encoding DQ7) in this study (13). Of note, none of our non-responders carried both DQB1*03:02 and DQB1*03:01 alleles (Supplementary Table 3). Using next-generation sequencing, the other study associated two extended haplotypes with MS in European-American patients. The haplotype encoding DR53−DR4−DQ8 serotypes was linked with MS risk, while the other haplotype encoding DR53−DR4−DQ7 serotypes was protective (76). Hence, DQ8 and DQ7, each encoded by a single allele, appear to influence MS susceptibility in an opposite manner. In line with this, our findings support the notion that DQ8 and DQ2 may represent predictive markers of poor MS outcome in IFN-treated patients. In addition, DQ8, DQ2, and DR4−DQ8 serotypes have been strongly associated with type 1 diabetes (77) and celiac disease (78), which suggests some sharing of pathogenic mechanisms between MS and these autoimmune diseases.
Overall, our findings suggest that the disease activity in our IFN non-responder patients is such that it cannot be counteracted by IFNβ bioactivity. Our non-responder patients may suffer from pathogenic CD4+ T cells, likely restricted by DQ8 and DQ2, that may exert autoreactive and bystander inflammatory activities. These findings may be of interest towards improved patient follow-up but warrant further validation with larger cohorts of patients.
Data Availability Statement
The raw data supporting the conclusions of this article will be made available by the authors, without undue reservation.
Ethics Statement
The study received administrative and ethical clearance in France from the “Comité de Protection des Personnes Ile de France IV (CPP)” 2014/17NICB and the “Commission Nationale de l’Informatique et des Libertés (CNIL)” MMS/CWR/AR1411558. Studies with healthy controls were approved for the CoSImmGEn cohort, ICAReB platform, Institut Pasteur (CPP 2010-dec.12483, CNIL 1161456), the Etablissement Français du Sang, Paris (CPSL UNT-18/EFS/04), and the Milieu Interieur (https://clinicaltrials.gov; NCT01699893 and NCT03905993, ANR-10-LABX-69-01). The patients provided their written informed consent to participate in this study.
Author Contributions
PD-M performed experiments, analyzed the data, and contributed to the writing. CM-C performed experiments and analyzed the data. PC performed statistical analyses of HLA typing. BC and AM-K contributed to flow cytometry assays. VB, AL, and DD were involved in IFN measurement. CP and EM recruited patients. SP revised the manuscript. FM supervised the work and wrote the manuscript. All authors contributed to the article and approved the submitted version.
Funding
This work was funded by the ARSEP (Aide à la Recherche sur la Sclérose En Plaques), FRM (Fondation pour la Recherche Médicale), INSERM, the Milieu Interieur ANR-10-LABX-69-01, and the Institut Pasteur, Paris.
Conflict of Interest
The authors declare that the research was conducted in the absence of any commercial or financial relationships that could be construed as a potential conflict of interest.
Acknowledgments
We thank the Milieu Interieur consortium and Etienne Patin for HLA class II typing data. We thank Prof. Bertrand Fontaine (Hospital Pitié-Salpêtrière, Paris) for advice in the initiation of the study. DD thanks ImmunoQure AG for sharing antibodies used in the IFNα assay. We acknowledge for support in conducting this study, the Technology Core of the Center for Translational Science, Estelle Mottez from the Clinical Core, the biobanking ICAReB core, Damien Mornico and Natalia Pietrosemoli from the Center of Bioinformatics, Biostatistics and Integrative biology, Institut Pasteur, Paris.
Supplementary Material
The Supplementary Material for this article can be found online at: https://www.frontiersin.org/articles/10.3389/fimmu.2021.628375/full#supplementary-material
Supplementary Figure 1 | Numbers and frequencies of CD3+, CD4+, and CD8+ T cells. Mann-Whitney test, *p < 0.05.
Supplementary Figure 2 | Numbers and frequencies of (A) CD4+ and (B) CD8+ TNaive, TCM and TEM cells in CD4+ and CD8+ CD3+ T cells. (C) Frequencies of Treg and Tconv cells in CD4+ CD3+ cells and ratio. (D) Numbers and frequencies of memory and activated subsets in Treg cells. Mann-Whitney test, *p < 0.05.
Supplementary Figure 3 | ISG signatures in IFN-treated patients. A list of 153 ISG (FC) > 1.5) was determined from previous MI data obtained with 25 healthy controls (50). Baseline ISG geomean scores were calculated in patient groups for strong (FC > 10, n=17 genes), moderate (FC 2.5-7.5) and low ISG induction (FC 1.5–2.5) in UT=9, Resp= 9 and NR=8. Strong ISGs (n=17) were CCL2, CCL8, CXCL10, HERC5, IFI35, IFIH1, IFIT2, IFITM1, IL1RN, IRF7, ISG15, LAMP3, MX1, RIG-I, SERPING1, TNFSF10, TNFSF13B; moderate ISG were ADAR1, BST2, CCR5, CCRL2, CDKN1A, CEACAM1, GBP1, IFI16, IRF5, IRF9, LAG3, LILRB1, MSR1, SLAMF7, SOCS1, STAT1, TAP2; and low ISGs were BLNK, CCND3, CD53, CFB, CTSC, FCER1G, HLA-C, ICAM2, LAIR1, MCL1, PLAU, PLAUR, PSMB10, PSMB8, TAPBP. Kruskal-Wallis test with Dunn’s multiple comparisons. *p < 0.05.
Supplementary Figure 4 | Expression of genes other than ISGs in IFN-treated patients. Additional genes from cluster B in Figure 3 with (A) lower expression in non-responders, (B) higher expression in responders or non-responders. Kruskal-Wallis test with Dunn’s multiple comparisons. *p < 0.05, **p < 0.005.
References
1. Dendrou CA, Fugger L, Friese MA. Immunopathology of Multiple Sclerosis. Nat Rev Immunol (2015) 15:545–58. doi: 10.1038/nri3871
2. Lassmann H. Multiple Sclerosis Pathology. Cold Spring Harb Perspect Med (2018) 8:1–15. doi: 10.1101/cshperspect.a028936
3. Foulon S, Maura G, Dalichampt M, Alla F, Debouverie M, Moreau T, et al. Prevalence and Mortality of Patients With Multiple Sclerosis in France in 2012: A Study Based on French Health Insurance Data. J Neurol (2017) 264:1185–92. doi: 10.1007/s00415-017-8513-0
4. Kingwell E, Marriott JJ, Jette N, Pringsheim T, Makhani N, Morrow SA, et al. Incidence and Prevalence of Multiple Sclerosis in Europe: A Systematic Review. BMC Neurol (2013) 13:128. doi: 10.1186/1471-2377-13-128
5. Palace J, Duddy M, Lawton M, Bregenzer T, Zhu F, Boggild M, et al. Assessing the Long-Term Effectiveness of Interferon-Beta and Glatiramer Acetate in Multiple Sclerosis: Final 10-Year Results From the UK Multiple Sclerosis Risk-Sharing Scheme. J Neurol Neurosurg Psychiatry (2019) 90:251–60. doi: 10.1136/jnnp-2018-318360
6. Kingwell E, Leray E, Zhu F, Petkau J, Edan G, Oger J, et al. Multiple Sclerosis: Effect of Beta Interferon Treatment on Survival. Brain (2019) 142:1324–33. doi: 10.1093/brain/awz055
7. Jakimovski D, Kolb C, Ramanathan M, Zivadinov R, Weinstock-Guttman B. Interferon Beta for Multiple Sclerosis. Cold Spring Harb Perspect Med (2018) 8:1–19. doi: 10.1101/cshperspect.a032003
8. Martin R, Sospedra M, Rosito M, Engelhardt B. Current Multiple Sclerosis Treatments Have Improved Our Understanding of MS Autoimmune Pathogenesis. Eur J Immunol (2016) 46:2078–90. doi: 10.1002/eji.201646485
9. D’Amico E, Zanghi A, Romeo M, Cocco E, Maniscalco GT, Brescia Morra V, et al. Injectable Versus Oral First-Line Disease-Modifying Therapies: Results From the Italian MS Register. Neurotherapeutics (2021). doi: 10.1007/s13311-020-01001-6
10. International Multiple Sclerosis Genetics C. Multiple Sclerosis Genomic Map Implicates Peripheral Immune Cells and Microglia in Susceptibility. Science (2019) 365:eaav7188. doi: 10.1126/science.aav7188
11. Olsson T, Barcellos LF, Alfredsson L. Interactions Between Genetic, Lifestyle and Environmental Risk Factors for Multiple Sclerosis. Nat Rev Neurol (2017) 13:25–36. doi: 10.1038/nrneurol.2016.187
12. International Multiple Sclerosis Genetics C, Wellcome Trust Case Control C, Sawcer S, Hellenthal G, Pirinen M, Spencer CC, et al. Genetic Risk and a Primary Role for Cell-Mediated Immune Mechanisms in Multiple Sclerosis. Nature (2011) 476:214–9. doi: 10.1038/nature10251
13. Moutsianas L, Jostins L, Beecham AH, Dilthey AT, Xifara DK, Ban M, et al. Class II HLA Interactions Modulate Genetic Risk for Multiple Sclerosis. Nat Genet (2015) 47:1107–13. doi: 10.1038/ng.3395
14. Patsopoulos NA, Barcellos LF, Hintzen RQ, Schaefer C, van Duijn CM, Noble JA, et al. Fine-Mapping the Genetic Association of the Major Histocompatibility Complex in Multiple Sclerosis: HLA and non-HLA Effects. PLoS Genet (2013) 9:e1003926. doi: 10.1371/journal.pgen.1003926
15. Hollenbach JA, Oksenberg JR. The Immunogenetics of Multiple Sclerosis: A Comprehensive Review. J Autoimmun (2015) 64:13–25. doi: 10.1016/j.jaut.2015.06.010
16. Li R, Patterson KR, Bar-Or A. Reassessing B Cell Contributions in Multiple Sclerosis. Nat Immunol (2018) 19:696–707. doi: 10.1038/s41590-018-0135-x
17. Jelcic I, Al Nimer F, Wang J, Lentsch V, Planas R, Jelcic I, et al. Memory B Cells Activate Brain-Homing, Autoreactive CD4(+) T Cells in Multiple Sclerosis. Cell (2018) 175:85–100.e23. doi: 10.1016/j.cell.2018.08.011
18. D’Amico E, Zanghi A, Gastaldi M, Patti F, Zappia M, Franciotta D. Placing CD20-Targeted B Cell Depletion in Multiple Sclerosis Therapeutic Scenario: Present and Future Perspectives. Autoimmun Rev (2019) 18:665–72. doi: 10.1016/j.autrev.2019.05.003
19. Hauser SL, Bar-Or A, Comi G, Giovannoni G, Hartung HP, Hemmer B, et al. Ocrelizumab Versus Interferon Beta-1a in Relapsing Multiple Sclerosis. N Engl J Med (2017) 376:221–34. doi: 10.1056/NEJMoa1601277
20. Geginat J, Paroni M, Pagani M, Galimberti D, De Francesco R, Scarpini E, et al. The Enigmatic Role of Viruses in Multiple Sclerosis: Molecular Mimicry or Disturbed Immune Surveillance? Trends Immunol (2017) 38:498–512. doi: 10.1016/j.it.2017.04.006
21. Fletcher JM, Lonergan R, Costelloe L, Kinsella K, Moran B, O’Farrelly C, et al. CD39+Foxp3+ Regulatory T Cells Suppress Pathogenic Th17 Cells and Are Impaired in Multiple Sclerosis. J Immunol (2009) 183:7602–10. doi: 10.4049/jimmunol.0901881
22. Dominguez-Villar M, Baecher-Allan CM, Hafler DA. Identification of T Helper Type 1-Like, Foxp3+ Regulatory T Cells in Human Autoimmune Disease. Nat Med (2011) 17:673–5. doi: 10.1038/nm.2389
23. Astier AL, Meiffren G, Freeman S, Hafler DA. Alterations in CD46-mediated Tr1 Regulatory T Cells in Patients With Multiple Sclerosis. J Clin Invest (2006) 116:3252–7. doi: 10.1172/JCI29251
24. Hug A, Korporal M, Schroder I, Haas J, Glatz K, Storch-Hagenlocher B, et al. Thymic Export Function and T Cell Homeostasis in Patients With Relapsing Remitting Multiple Sclerosis. J Immunol (2003) 171:432–7. doi: 10.4049/jimmunol.171.1.432
25. Haas J, Fritzsching B, Trubswetter P, Korporal M, Milkova L, Fritz B, et al. Prevalence of Newly Generated Naive Regulatory T Cells (Treg) Is Critical for Treg Suppressive Function and Determines Treg Dysfunction in Multiple Sclerosis. J Immunol (2007) 179:1322–30. doi: 10.4049/jimmunol.179.2.1322
26. Cao Y, Goods BA, Raddassi K, Nepom GT, Kwok WW, Love JC, et al. Functional Inflammatory Profiles Distinguish Myelin-Reactive T Cells From Patients With Multiple Sclerosis. Sci Transl Med (2015) 7:287ra74. doi: 10.1126/scitranslmed.aaa8038
27. Hu D, Notarbartolo S, Croonenborghs T, Patel B, Cialic R, Yang TH, et al. Transcriptional Signature of Human Pro-Inflammatory TH17 Cells Identifies Reduced IL10 Gene Expression in Multiple Sclerosis. Nat Commun (2017) 8:1600. doi: 10.1038/s41467-017-01571-8
28. Paroni M, Maltese V, De Simone M, Ranzani V, Larghi P, Fenoglio C, et al. Recognition of Viral and Self-Antigens by TH1 and TH1/TH17 Central Memory Cells in Patients With Multiple Sclerosis Reveals Distinct Roles in Immune Surveillance and Relapses. J Allergy Clin Immunol (2017) 140:797–808. doi: 10.1016/j.jaci.2016.11.045
29. Markovic-Plese S, Cortese I, Wandinger KP, McFarland HF, Martin R. CD4+CD28- Costimulation-Independent T Cells in Multiple Sclerosis. J Clin Invest (2001) 108:1185–94. doi: 10.1172/JCI12516
30. Thewissen M, Somers V, Hellings N, Fraussen J, Damoiseaux J, Stinissen P. CD4+CD28null T Cells in Autoimmune Disease: Pathogenic Features and Decreased Susceptibility to Immunoregulation. J Immunol (2007) 179:6514–23. doi: 10.4049/jimmunol.179.10.6514
31. Peeters LM, Vanheusden M, Somers V, Van Wijmeersch B, Stinissen P, Broux B, et al. Cytotoxic CD4+ T Cells Drive Multiple Sclerosis Progression. Front Immunol (2017) 8:1160. doi: 10.3389/fimmu.2017.01160
32. Nicol B, Salou M, Vogel I, Garcia A, Dugast E, Morille J, et al. An Intermediate Level of CD161 Expression Defines a Novel Activated, Inflammatory, and Pathogenic Subset of CD8(+) T Cells Involved in Multiple Sclerosis. J Autoimmun (2018) 88:61–74. doi: 10.1016/j.jaut.2017.10.005
33. Severa M, Rizzo F, Giacomini E, Salvetti M, Coccia EM. IFN-beta and Multiple Sclerosis: Cross-Talking of Immune Cells and Integration of Immunoregulatory Networks. Cytokine Growth Factor Rev (2015) 26:229–39. doi: 10.1016/j.cytogfr.2014.11.005
34. Zula JA, Green HC, Ransohoff RM, Rudick RA, Stark GR, van Boxel-Dezaire AH. The Role of Cell Type-Specific Responses in IFN-beta Therapy of Multiple Sclerosis. Proc Natl Acad Sci USA (2011) 108:19689–94. doi: 10.1073/pnas.1117347108
35. Venken K, Hellings N, Broekmans T, Hensen K, Rummens JL, Stinissen P. Natural Naive CD4+CD25+CD127low Regulatory T Cell (Treg) Development and Function are Disturbed in Multiple Sclerosis Patients: Recovery of Memory Treg Homeostasis During Disease Progression. J Immunol (2008) 180:6411–20. doi: 10.4049/jimmunol.180.9.6411
36. Dooley J, Pauwels I, Franckaert D, Smets I, Garcia-Perez JE, Hilven K, et al. Immunologic Profiles of Multiple Sclerosis Treatments Reveal Shared Early B Cell Alterations. Neurol Neuroimmunol Neuroinflamm (2016) 3:e240. doi: 10.1212/NXI.0000000000000240
37. Chiarini M, Capra R, Serana F, Bertoli D, Sottini A, Giustini V, et al. Simultaneous Quantification of Natural and Inducible Regulatory T-Cell Subsets During Interferon-Beta Therapy of Multiple Sclerosis Patients. J Transl Med (2020) 18:169. doi: 10.1186/s12967-020-02329-5
38. Ramgolam VS, Sha Y, Jin J, Zhang X, Markovic-Plese S. IFN-Beta Inhibits Human Th17 Cell Differentiation. J Immunol (2009) 183:5418–27. doi: 10.4049/jimmunol.0803227
39. Guarda G, Braun M, Staehli F, Tardivel A, Mattmann C, Forster I, et al. Type I Interferon Inhibits Interleukin-1 Production and Inflammasome Activation. Immunity (2011) 34:213–23. doi: 10.1016/j.immuni.2011.02.006
40. Levings MK, Sangregorio R, Galbiati F, Squadrone S, de Waal Malefyt R, Roncarolo MG. IFN-Alpha and IL-10 Induce the Differentiation of Human Type 1 T Regulatory Cells. J Immunol (2001) 166:5530–9. doi: 10.4049/jimmunol.166.9.5530
41. Corre B, Perrier J, El Khouri M, Cerboni S, Pellegrini S, Michel F. Type I Interferon Potentiates T-cell Receptor Mediated Induction of IL-10-producing CD4(+) T Cells. Eur J Immunol (2013) 43:2730–40. doi: 10.1002/eji.201242977
42. Paul A, Comabella M, Gandhi R. Biomarkers in Multiple Sclerosis. Cold Spring Harb Perspect Med (2019) 9:22. doi: 10.1101/cshperspect.a029058
43. Polman CH, Reingold SC, Banwell B, Clanet M, Cohen JA, Filippi M, et al. Diagnostic Criteria for Multiple Sclerosis: 2010 Revisions to the McDonald Criteria. Ann Neurol (2011) 69:292–302. doi: 10.1002/ana.22366
44. Llibre A, Bilek N, Bondet V, Darboe F, Mbandi SK, Penn-Nicholson A, et al. Plasma Type I IFN Protein Concentrations in Human Tuberculosis. Front Cell Infect Microbiol (2019) 9:296. doi: 10.3389/fcimb.2019.00296
45. Kim H, de Jesus AA, Brooks SR, Liu Y, Huang Y, VanTries R, et al. Development of a Validated Interferon Score Using NanoString Technology. J Interferon Cytokine Res (2018) 38:171–85. doi: 10.1089/jir.2017.0127
46. Marsh SG, Albert ED, Bodmer WF, Bontrop RE, Dupont B, Erlich HA, et al. Nomenclature for Factors of the HLA System, 2010. Tissue Antigens (2010) 75:291–455. doi: 10.1111/j.1399-0039.2010.01466.x
47. Patin E, Hasan M, Bergstedt J, Rouilly V, Libri V, Urrutia A, et al. Natural Variation in the Parameters of Innate Immune Cells Is Preferentially Driven by Genetic Factors. Nat Immunol (2018) 19:302–14. doi: 10.1038/s41590-018-0049-7
48. Miyara M, Yoshioka Y, Kitoh A, Shima T, Wing K, Niwa A, et al. Functional Delineation and Differentiation Dynamics of Human CD4+ T Cells Expressing the FoxP3 Transcription Factor. Immunity (2009) 30:899–911. doi: 10.1016/j.immuni.2009.03.019
49. Rodero MP, Decalf J, Bondet V, Hunt D, Rice GI, Werneke S, et al. Detection of Interferon Alpha Protein Reveals Differential Levels and Cellular Sources in Disease. J Exp Med (2017) 214:1547–55. doi: 10.1084/jem.20161451
50. Urrutia A, Duffy D, Rouilly V, Posseme C, Djebali R, Illanes G, et al. Standardized Whole-Blood Transcriptional Profiling Enables the Deconvolution of Complex Induced Immune Responses. Cell Rep (2016) 16:2777–91. doi: 10.1016/j.celrep.2016.08.011
51. Egholm C, Heeb LEM, Impellizzieri D, Boyman O. The Regulatory Effects of Interleukin-4 Receptor Signaling on Neutrophils in Type 2 Immune Responses. Front Immunol (2019) 10:2507. doi: 10.3389/fimmu.2019.02507
52. Mantovani A, Dinarello CA, Molgora M, Garlanda C. Interleukin-1 and Related Cytokines in the Regulation of Inflammation and Immunity. Immunity (2019) 50:778–95. doi: 10.1016/j.immuni.2019.03.012
53. Housley WJ, Pitt D, Hafler DA. Biomarkers in Multiple Sclerosis. Clin Immunol (2015) 161:51–8. doi: 10.1016/j.clim.2015.06.015
54. Klitz W, Maiers M, Spellman S, Baxter-Lowe LA, Schmeckpeper B, Williams TM, et al. New HLA Haplotype Frequency Reference Standards: High-Resolution and Large Sample Typing of HLA DR-DQ Haplotypes in a Sample of European Americans. Tissue Antigens (2003) 62:296–307. doi: 10.1034/j.1399-0039.2003.00103.x
55. Robinson J, Barker DJ, Georgiou X, Cooper MA, Flicek P, Marsh SGE. IPD-IMGT/HLA Database. Nucleic Acids Res (2020) 48:D948–D55. doi: 10.1093/nar/gkz950
56. Chiarini M, Serana F, Zanotti C, Capra R, Rasia S, Rottoli M, et al. Modulation of the Central Memory and Tr1-like Regulatory T Cells in Multiple Sclerosis Patients Responsive to Interferon-Beta Therapy. Mult Scler (2012) 18:788–98. doi: 10.1177/1352458511427720
57. Korporal M, Haas J, Balint B, Fritzsching B, Schwarz A, Moeller S, et al. Interferon Beta-Induced Restoration of Regulatory T-cell Function in Multiple Sclerosis is Prompted by an Increase in Newly Generated Naive Regulatory T Cells. Arch Neurol (2008) 65:1434–9. doi: 10.1001/archneur.65.11.1434
58. Teniente-Serra A, Grau-Lopez L, Mansilla MJ, Fernandez-Sanmartin M, Ester Condins A, Ramo-Tello C, et al. Multiparametric Flow Cytometric Analysis of Whole Blood Reveals Changes in Minor Lymphocyte Subpopulations of Multiple Sclerosis Patients. Autoimmunity (2016) 49:219–28. doi: 10.3109/08916934.2016.1138271
59. Comi G, De Stefano N, Freedman MS, Barkhof F, Polman CH, Uitdehaag BM, et al. Comparison of Two Dosing Frequencies of Subcutaneous Interferon beta-1a in Patients With a First Clinical Demyelinating Event Suggestive of Multiple Sclerosis (REFLEX): A Phase 3 Randomised Controlled Trial. Lancet Neurol (2012) 11:33–41. doi: 10.1016/S1474-4422(11)70262-9
60. Rieckmann P, O’Connor P, Francis GS, Wetherill G, Alteri E. Haematological Effects of Interferon-beta-1a (Rebif) Therapy in Multiple Sclerosis. Drug Saf (2004) 27:745–56. doi: 10.2165/00002018-200427100-00005
61. Geginat J, Sallusto F, Lanzavecchia A. Cytokine-Driven Proliferation and Differentiation of Human Naive, Central Memory, and Effector Memory CD4(+) T Cells. J Exp Med (2001) 194:1711–9. doi: 10.1084/jem.194.12.1711
62. Cossarizza A, Chang HD, Radbruch A, Acs A, Adam D, Adam-Klages S, et al. Guidelines for the Use of Flow Cytometry and Cell Sorting in Immunological Studies (Second Edition). Eur J Immunol (2019) 49:1457–973. doi: 10.1002/eji.201970107
63. Hesse D, Krakauer M, Lund H, Sondergaard HB, Langkilde A, Ryder LP, et al. Breakthrough Disease During Interferon-[Beta] Therapy in MS: No Signs of Impaired Biologic Response. Neurology (2010) 74:1455–62. doi: 10.1212/WNL.0b013e3181dc1a94
64. Martire S, Navone ND, Montarolo F, Perga S, Bertolotto A. A Gene Expression Study Denies the Ability of 25 Candidate Biomarkers to Predict the Interferon-Beta Treatment Response in Multiple Sclerosis Patients. J Neuroimmunol (2016) 292:34–9. doi: 10.1016/j.jneuroim.2016.01.010
65. van Baarsen LG, Vosslamber S, Tijssen M, Baggen JM, van der Voort LF, Killestein J, et al. Pharmacogenomics of Interferon-Beta Therapy in Multiple Sclerosis: Baseline IFN Signature Determines Pharmacological Differences Between Patients. PLoS One (2008) 3:e1927. doi: 10.1371/journal.pone.0001927
66. Reder AT, Velichko S, Yamaguchi KD, Hamamcioglu K, Ku K, Beekman J, et al. IFN-beta1b Induces Transient and Variable Gene Expression in Relapsing-Remitting Multiple Sclerosis Patients Independent of Neutralizing Antibodies or Changes in IFN Receptor RNA Expression. J Interferon Cytokine Res (2008) 28:317–31. doi: 10.1089/jir.2007.0131
67. Axtell RC, de Jong BA, Boniface K, van der Voort LF, Bhat R, De Sarno P, et al. T Helper Type 1 and 17 Cells Determine Efficacy of Interferon-Beta in Multiple Sclerosis and Experimental Encephalomyelitis. Nat Med (2010) 16:406–12. doi: 10.1038/nm.2110
68. Bushnell SE, Zhao Z, Stebbins CC, Cadavid D, Buko AM, Whalley ET, et al. Serum IL-17F Does Not Predict Poor Response to IM IFNbeta-1a in Relapsing-Remitting MS. Neurology (2012) 79:531–7. doi: 10.1212/WNL.0b013e318259e123
69. Comabella M, Lunemann JD, Rio J, Sanchez A, Lopez C, Julia E, et al. A Type I Interferon Signature in Monocytes is Associated With Poor Response to Interferon-Beta in Multiple Sclerosis. Brain (2009) 132:3353–65. doi: 10.1093/brain/awp228
70. Malucchi S, Gilli F, Caldano M, Marnetto F, Valentino P, Granieri L, et al. Predictive Markers for Response to Interferon Therapy in Patients With Multiple Sclerosis. Neurology (2008) 70:1119–27. doi: 10.1212/01.wnl.0000304040.29080.7b
71. Malhotra S, Bustamante MF, Perez-Miralles F, Rio J, Ruiz de Villa MC, Vegas E, et al. Search for Specific Biomarkers of IFNbeta Bioactivity in Patients With Multiple Sclerosis. PLoS One (2011) 6:e23634. doi: 10.1371/journal.pone.0023634
72. Herndon RM, Rudick RA, Munschauer FE 3rd, Mass MK, Salazar AM, Coats ME, et al. Eight-Year Immunogenicity and Safety of Interferon Beta-1a-Avonex Treatment in Patients With Multiple Sclerosis. Mult Scler (2005) 11:409–19. doi: 10.1191/1352458505ms1209oa
73. Gneiss C, Tripp P, Reichartseder F, Egg R, Ehling R, Lutterotti A, et al. Differing Immunogenic Potentials of Interferon Beta Preparations in Multiple Sclerosis Patients. Mult Scler (2006) 12:731–7. doi: 10.1177/1352458506070941
74. Grossberg SE, Oger J, Grossberg LD, Gehchan A, Klein JP. Frequency and Magnitude of Interferon Beta Neutralizing Antibodies in the Evaluation of Interferon Beta Immunogenicity in Patients With Multiple Sclerosis. J Interferon Cytokine Res (2011) 31:337–44. doi: 10.1089/jir.2010.0038
75. Dendrou CA, Petersen J, Rossjohn J, Fugger L. HLA Variation and Disease. Nat Rev Immunol (2018) 18:325–39. doi: 10.1038/nri.2017.143
76. Creary LE, Mallempati KC, Gangavarapu S, Caillier SJ, Oksenberg JR, Fernandez-Vina MA. Deconstruction of HLA-DRB1*04:01:01 and HLA-DRB1*15:01:01 Class II Haplotypes Using Next-Generation Sequencing in European-Americans With Multiple Sclerosis. Mult Scler (2019) 25:772–82. doi: 10.1177/1352458518770019
77. Noble JA. Immunogenetics of Type 1 Diabetes: A Comprehensive Review. J Autoimmun (2015) 64:101–12. doi: 10.1016/j.jaut.2015.07.014
Keywords: multiple sclerosis, type I interferon, T cells, interferon-stimulated genes, HLA class II genes, immune phenotypes, blood biomarkers
Citation: Devi-Marulkar P, Moraes-Cabe C, Campagne P, Corre B, Meghraoui-Kheddar A, Bondet V, Llibre A, Duffy D, Maillart E, Papeix C, Pellegrini S and Michel F (2021) Altered Immune Phenotypes and HLA-DQB1 Gene Variation in Multiple Sclerosis Patients Failing Interferon β Treatment. Front. Immunol. 12:628375. doi: 10.3389/fimmu.2021.628375
Received: 11 November 2020; Accepted: 23 April 2021;
Published: 25 May 2021.
Edited by:
Simona Bonavita, University of Campania Luigi Vanvitelli, ItalyReviewed by:
Antonio Bertolotto, San Luigi Gonzaga University Hospital, ItalyEmanuele D’Amico, University of Catania, Italy
Copyright © 2021 Devi-Marulkar, Moraes-Cabe, Campagne, Corre, Meghraoui-Kheddar, Bondet, Llibre, Duffy, Maillart, Papeix, Pellegrini and Michel. This is an open-access article distributed under the terms of the Creative Commons Attribution License (CC BY). The use, distribution or reproduction in other forums is permitted, provided the original author(s) and the copyright owner(s) are credited and that the original publication in this journal is cited, in accordance with accepted academic practice. No use, distribution or reproduction is permitted which does not comply with these terms.
*Correspondence: Frédérique Michel, ZnJlZGVyaXF1ZS5taWNoZWxAcGFzdGV1ci5mcg==