- 1Department of Pediatric and Adolescent Medicine, Li Ka Shing Faculty of Medicine, The University of Hong Kong, Hong Kong, China
- 2Shenzhen Key Laboratory of Reproductive Immunology for Peri-Implantation, Fertility Center, Shenzhen Zhongshan Urology Hospital, Shenzhen, China
- 3ALOM Intelligence Limited, Hong Kong, China
Recurrent reproductive failure (RRF), such as recurrent pregnancy loss and repeated implantation failure, is characterized by complex etiologies and particularly associated with diverse maternal factors. It is currently believed that RRF is closely associated with the maternal environment, which is, in turn, affected by complex immune factors. Without the use of automated tools, it is often difficult to assess the interaction and synergistic effects of the various immune factors on the pregnancy outcome. As a result, the application of Artificial Intelligence (A.I.) has been explored in the field of assisted reproductive technology (ART). In this study, we reviewed studies on the use of A.I. to develop prediction models for pregnancy outcomes of patients who underwent ART treatment. A limited amount of models based on genetic markers or common indices have been established for prediction of pregnancy outcome of patients with RRF. In this study, we applied A.I. to analyze the medical information of patients with RRF, including immune indicators. The entire clinical samples set (561 samples) was divided into two sets: 90% of the set was used for training and 10% for testing. Different data panels were established to predict pregnancy outcomes at four different gestational nodes, including biochemical pregnancy, clinical pregnancy, ongoing pregnancy, and live birth, respectively. The prediction models of pregnancy outcomes were established using sparse coding, based on six data panels: basic patient characteristics, hormone levels, autoantibodies, peripheral immunology, endometrial immunology, and embryo parameters. The six data panels covered 64 variables. In terms of biochemical pregnancy prediction, the area under curve (AUC) using the endometrial immunology panel was the largest (AUC = 0.766, accuracy: 73.0%). The AUC using the autoantibodies panel was the largest in predicting clinical pregnancy (AUC = 0.688, accuracy: 78.4%), ongoing pregnancy (AUC = 0.802, accuracy: 75.0%), and live birth (AUC = 0.909, accuracy: 89.7%). Combining the data panels did not significantly enhance the effect on prediction of all the four pregnancy outcomes. These results give us a new insight on reproductive immunology and establish the basis for assisting clinicians to plan more precise and personalized diagnosis and treatment for patients with RRF.
Introduction
Pregnancy is a complex biological process that poses a great challenge to the maternal immune system. The unique immunology of the maternal–fetal interface was recognized, since the “fetal allograft” concept was first described by Sir Peter Brian Medawar in the early 1950s (1). Correct and precise cross-talk between fetus and mother is an important basis for the apposition, adhesion, implantation, and growth of the embryo in the uterus (2). The abnormal frequencies and functions of maternal immune cells are associated with reproductive failure, especially in cases of recurrent reproductive failure (RRF), such as recurrent pregnancy loss (RPL) and repeated implantation failure (RIF) (3).
In the conventional medical procedure, the patients with RRF are assessed and given a score, based on biomarkers that have been demonstrated to be of relevance to the disease. The treatment for the patients is based on the classification or scores (4, 5). However, the etiologies of RRF are highly heterogeneous and the complex underlying interactions between the biomarkers make the creation of a personalized treatment strategy based on all known parameters impossible for the clinicians. Therefore, the design of a model that can accurately predict the outcome of treatment methods would be highly beneficial to the clinicians, enabling the choice of lower-risk treatments, thus alleviating the financial burden of the treatment cost and reducing treatment time.
In the field of assisted reproductive technology (ART), predictive models have been applied as decision aids to embryo, egg, sperm selection, and pregnancy outcome prediction, and for the intrinsic evaluation of various factors related to clinical outcomes (6, 7). Presently, the validity of the applied models has been demonstrated by analyzing the correlation between factors and the treatment outcome or etiology (8). However, the varying degrees of accuracy and limitations of the applied models have inhibited their use in the routine implementation of in vitro fertilization (IVF) procedures (6). To address this problem, more complex Artificial Intelligence (A.I.) systems, such as artificial neural networks (ANNs), have been introduced in ART fields (9, 10). A.I. systems are advantageous due to their significant information processing properties in terms of non-linearity, high levels of parallelism, noise and fault tolerance, as well as learning, generalization, and adaptive capabilities (11). Nevertheless, few studies that focus on the pregnancy outcome prediction in patients with RRF exist.
Sparse coding is a common machine learning technique used to extract features from raw data. The core of sparse coding involves establishing a sparse representation of the raw data to form a linear combination of basic elements called “atoms,” which collectively form a library known as “dictionary.” The advantages of using sparse coding include: (1) training a learning model by adopting a relatively lower number of features from raw data, which, in turn, lowers the computational cost during model training; (2) increased interpretability of the learning results as critical features that can be identified efficiently from the dictionary (12). It has been demonstrated that sparse coding can be applied in genome-wide association studies, neuroimaging, and oncology for object detection and classification tasks (13–16). Sparse coding techniques have not been comprehensively studied in the area of reproductive medicine, and its application in immunological profile analysis of patients with RRF remains to be explored.
The remainder of this article is organized as follows. A literature review in the field of A.I. and reproductive medicine is presented in the next section, followed by the methodology and demonstration of sparse coding application to analyze multidimensional clinical data of patients with RRF and predict their pregnancy outcomes. The results on the performance of the model are then introduced and finally, the concluding remarks are presented in the discussion.
Overview of A.I. Application in Reproductive Failure
Artificial Intelligence in Predicting Pregnancy Outcomes of Patients With Infertility
Machine learning is a subset of A.I. that enables computer algorithms to model the relationship between a set of observable data (input data) and another set of variables (output data) (17). It provides the ability to interpret and understand data and to develop predictive models based on experience. Machine learning methods include ANN, Support Vector Machines (SVM), C4.5, Classification and Regression Tree (CART), Random Forest (RF), K-Nearest-Neighbor (KNN), and so on. ANNs and SVMs are widely used in biomedical problems analysis. Machine learning methods can provide more options and richer task information for problem solving. At the same time, machine learning methods are gaining popularity in clinical decision-making (18–20).
The concept of a neural network is derived from the structure and function of biological neural networks. In particular, ANNs propose a system with stacked layers of interconnected processors, or nodes, that can form increasingly complex features in each successive layer (21). Raw information is supplied into the input layer and passes through the implicit layer by a weighted connection system. Finally, the output values of the transformed features are generated in the output layer, to predict the outcome. In a clinical setting, the input layer can represent medical data, the output layer can represent prognostic subclasses and multiple implicit layers can represent feature detectors, used to capture higher-order correlations. The SVM algorithm classifies the input data by calculating support vectors that construct hyperplanes in a higher-dimensional space, where the features are separable. C4.5, CART, and RF are three decision trees with non-parametric characteristics that map characteristics to outcomes using a partitioning procedure that recursively divides the source set of each node or branch point into unrelated subsets based on the value of a particular characteristic. KNN is an instance-based learning method that assigns classes to the data, based on nearest-neighbor decision rules.
On the basis of big data training iterations, dimensionality reduction can be applied to a large number of influential factors by using various machine learning methods for modeling and prediction. Simultaneously, relevant attributes with high influence can be extracted, and a prediction model with relatively high accuracy can be obtained. Hassan et al. (21) evaluated the predictive ability of five different machine learning models, namely, Multilayer Perceptron (MLP), SVM, C4.5, CART, and RF, on the success rate of IVF pregnancies. A feature selection algorithm for climbing features (attributes), combined with automatic classification using a machine learning technique, was used to reduce the number of most influential attributes to 19 for MLP, 16 for RF, 17 for SVMs, 12 for C4.5, and 8 for CART, in order to analyze and predict IVF pregnancies in a more accurate manner. The most influential factors were summarized as: age, fertility factor index, basal sinus follicle count, number of mature eggs, sperm collection method, gametes, in vitro fertilization rate, 14-day follicle count, and embryo transfer date. Vogiatzi et al. (8) used the ANN approach to validate the efficiency of an ANN based on correlated parameters of live birth as a comprehensive tool for predicting clinical outcomes in patients undergoing ART. The ANN was constructed using 12 statistically significant parameters from the initial integration with a cumulative sensitivity of 76.7% and a specificity of 73.4%. The standard deviation of the performance metrics evaluated between the training and the testing sets was low in the validation process, pointing to the stability of the constructed ANN. The constructed ANN, based on statistically significant live birth outcome variables, is a stable and efficient system with increased performance metrics. The validation of the system led to the recognition of its clinical value as a medical decision aid and provided a reliable method for the routine practice of IVF units in a user-friendly environment. Elson et al. (22) developed a decision tree based on a combination of clinical, morphological, and biochemical parameters predicting successful pregnancy outcomes that assisted the expected management of women with tubal ectopic pregnancies. Significant differences were detected in maternal age, initial serum β-hCG, and progesterone among pregnant women who required surgery or recovered on their own. Analysis utilizing a decision tree can be used as an estimation guide for the probability of successful prediction individually.
Machine learning methods have, in general, high prediction accuracy; however, the final model prediction accuracy can vary. Commonly used classifiers include SVMs, recursive partitioning, RF, adaptive augmentation, and KNN. Hafiz et al. (23) used data mining techniques to predict the implantation outcome of IVF and intracytoplasmic sperm injection (ICSI), which were found to be superior to other comparable methods using RF and recursive partitioning, with the corresponding area under the ROC curve (AUC) values reaching 84.23 and 82.05%, respectively. Ghaeini et al. (24) proposed an ICSI outcome prediction model based on decision trees and SVMs. The input variables of the model included parameters such as the medical history of the couple, hormone testing, and cause of infertility. The output variable was the occurrence of a clinical pregnancy. The accuracies of the decision tree method and SVMs were 70.3 and 75.7%, respectively. The performance of the SVM method was superior to the performance of the decision tree method.
Artificial Intelligence in Predicting Pregnancy Outcomes of Patients With RRF
Currently, research on predictive models for pregnancy outcomes in patients with RRF is limited and has mainly focused on classifying patients for better clinical management, ignoring the effects of relevant immune factors on pregnancy outcomes of the patients. Bruno et al. (25) used machine learning to stratify patients with RPL into different risk categories, validated their appropriate prognosis and potential treatments through diagnostic workup, provided a decision-support system tool to stratify RPL patients, and objectively addressed their appropriate clinical management. Immune factors were not accounted for and pregnancy outcomes were not predicted. Li et al. (26) suggested that RPL may be related to abnormally elevated amounts of uterine natural killer (uNK) cells. They pointed out the difficulty of counting uNK and stromal cells under histochemical sections, because of the close morphological proximity of stromal cells to epithelial cells. This paper was the first to report on the ability to distinguish between different cell morphologies and accurately count them using image recognition techniques. Researchers can greatly benefit from this method in analyzing immunohistochemical images. Nevertheless, its application is limited and is unable to provide predictions, based on cell counts alone. Mora-Sanchez et al. (27) concluded that the degree of allelic sharing of human leukocyte antigen (HLA) genes is related to RPL, combining immunogenetics with A.I. to create a personalized tool to elucidate the genetic causes of unexplained infertility and a gamete matching platform that could improve pregnancy success.
The representative literature on the development of predictive models for pregnancy outcomes in recent infertile and patients with RRF is summarized in Table 1. Notably, a limited amount of models were observed that were established for the prediction of pregnancy outcome of patients with RRF. The published models were based only on genetic markers or common indices. To investigate the impact of immune factors on pregnancy outcomes in patients with RRF, we applied A.I. for the analysis of the medical information of patients with RRF, including immune indices for pregnancy outcomes prediction.
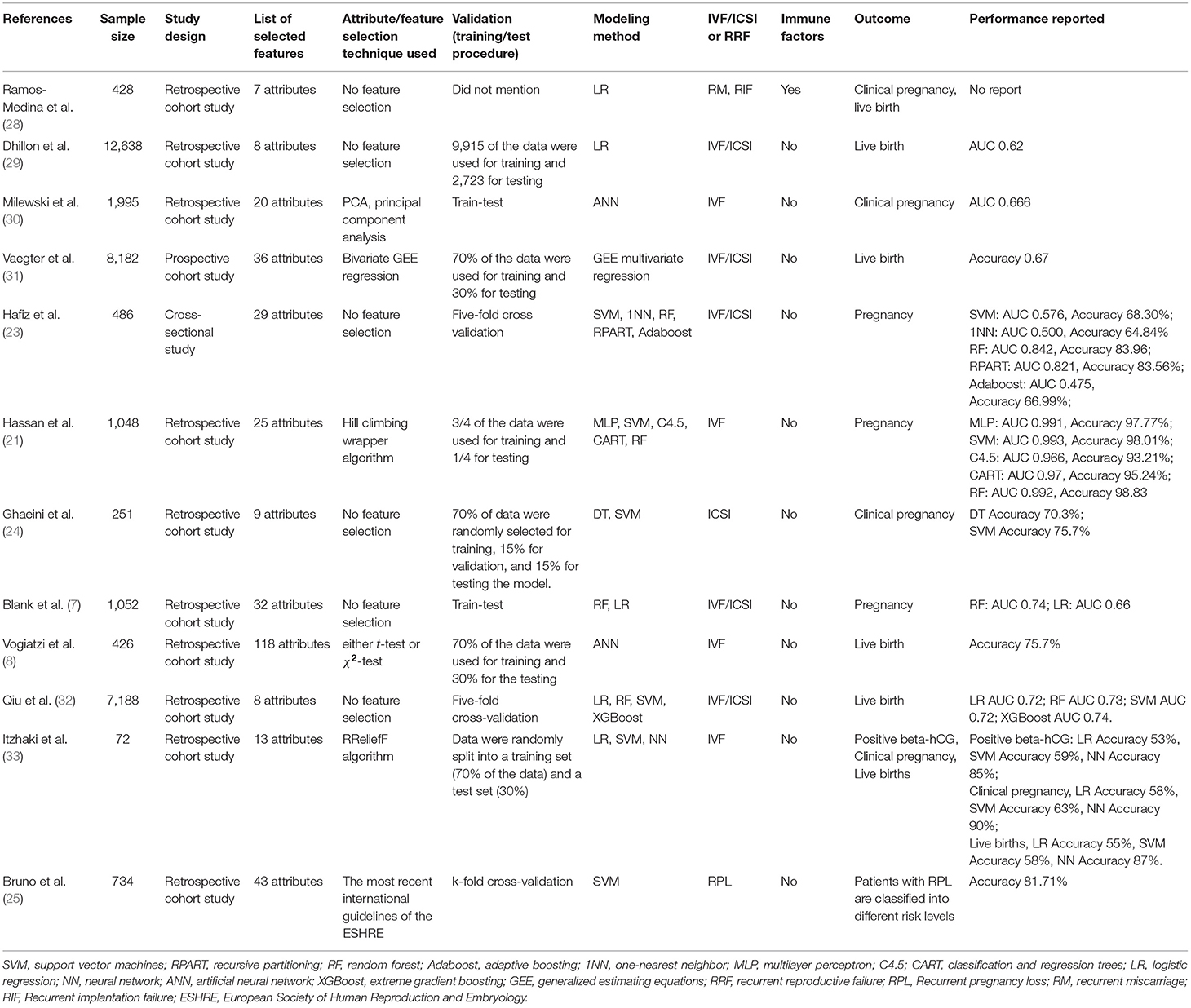
Table 1. Recent representative literature related to predictive models for patients with infertility or RRF.
Experimental Methods
Data Acquisition
Medical data from 942 patients with RRF, including RPL or RIF, who visited the Fertility Center of Shenzhen Zhongshan Urology Hospital, China, from February 2015 to November 2019, were retrospectively extracted from the electronic medical record (EMR) system. RPL is defined as two or more spontaneous abortions prior to 20 weeks of gestation. RIF refers to infertile couples who experienced failure to conceive after multiple IVF cycles. During the process of diagnosis and laboratory detection, the medical data were generated and stored in the EMR system. Out of 942 samples, 381 were excluded, due to missing values. Finally, 561 data samples were included in the analysis.
Data Processing
Data processing, model design, and programming were all carried out in MATLAB 2017b (The MathWorks, Inc.). Due to diversity in clinical testing and reporting period, concatenation of data within 90 days prior to serum human chorionic gonadotropin (hCG) test was performed for each patient. Z-score normalization was used for numerical data and one-hot encoding for categorical data.
Model Training and Performance
The initialization of the dictionary matrix (Wd) was carried out using uniformly distributed numbers. Each column of Wd was normalized to a magnitude of 1. The processed and normalized data set (X) and dictionary matrix were used as input into the Iterative Shrinkage and Thresholding-based Algorithm with coordinate descent to obtain the sparse representation (Z) (34). Tanh and ReLu were selected as the activation functions of the hidden layers for the sparse representation. Softmax was used in the output layer for linear classification to obtain the prediction results. The cost of prediction was calculated using the sum of least squares between the prediction result and the true label. The Wd matrix was updated through backpropagation. Forward- and back-propagation were repeated until the optimal dictionary matrix was obtained (i.e., lowest cost). The data set was divided into training data set (90%) and testing data set (10%) for each data panel. The performance of the model was evaluated on the testing data set. The evaluation metrics included receiver operating characteristic (ROC) curves, accuracy, sensitivity, and specificity. The true positive (TP), true negative (TN), false positive (FP), and false negative (FN) rates were used for the calculation of the abovementioned metrics as follows:
Results
Clinical Characteristics of Samples
Following the literature review and in combination with the expertise of clinicians, six panels with 64 variables were considered as input variables. Three immune-related data panels, including the autoantibodies, peripheral immunology, and endometrial immunology panels were considered. Other IVF-related data panels contributing to the pregnancy outcome, including basic characteristics, hormones, and embryo panels were also considered. The clinical characteristics used in this study along with their respective description explaining their physical implications, type of the values, and their range in the collected data set are listed in Table 2. The mean age at the time of conception was 34.67 years and significantly different between the live birth group and non-live birth group. The average body mass index (BMI) was 21.69 kg/m2, with no statistically significant differences detected (21.55 vs. 21.79). Statistically significant differences were detected between some of the 64 variables, between the groups of patients who did and did not achieve a live birth (Table 2).
Model Performance on Immunological Data Panels
We tested the sparse coding model using various data panels including autoantibodies (Figure 1), peripheral immunology (Figure 2), endometrial immunology (Figure 3), and the combination of all three immunological data panels (Figure 4). A summary of prediction accuracy using various data panels is shown in Table 3. Four output labels were used for prediction, namely, biochemical pregnancy, clinical pregnancy, ongoing pregnancy, and live birth. The ROC curves during the model training generally had AUC values exceeding 0.9, for each immunological panel. The AUC values decreased during testing. In terms of predicting biochemical pregnancy, the AUC of prediction using the endometrial immunology panel was higher (AUC = 0.766, accuracy: 73.0%) compared to the AUC of the panel using autoantibodies (AUC = 0.447, accuracy: 70.3%) and peripheral immunology panel (AUC = 0.697, accuracy: 72.4%). The AUC of prediction using the autoantibodies panel was higher in predicting clinical pregnancy (AUC = 0.688, accuracy: 78.4%), ongoing pregnancy (AUC = 0.802, accuracy: 75.0%), and live birth (AUC = 0.909, accuracy: 89.7%), compared to the AUC of the panels using peripheral immunology and endometrial immunology panel. Combining all three immunological data panels did not result in an increase of the AUCs of all four pregnancy outcomes.
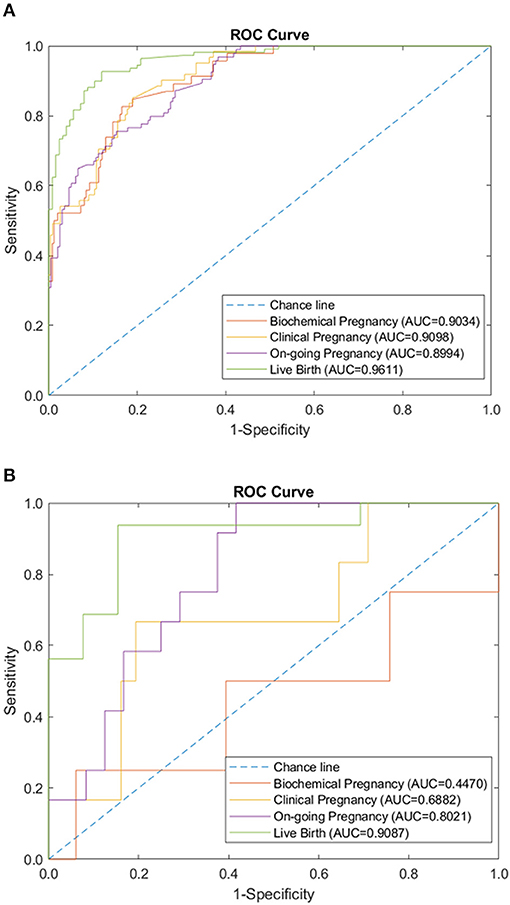
Figure 1. Autoantibodies panel performance of sparse coding in predicting pregnancy outcomes at different pregnancy periods. (A) ROC plot of the training data set. (B) ROC plot of the testing data set.
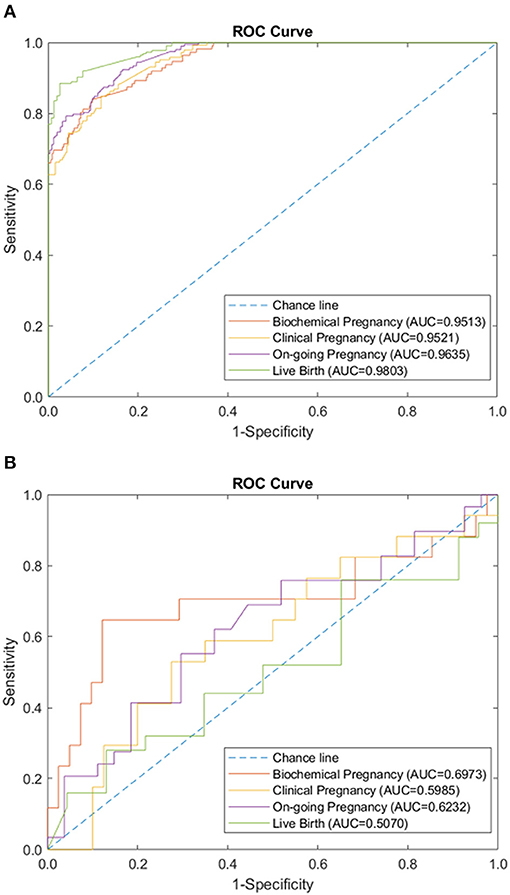
Figure 2. Peripheral immunology panel performance of sparse coding in predicting pregnancy outcomes at different pregnancy periods. (A) ROC plot of the training data set. (B) ROC plot of the testing data set.
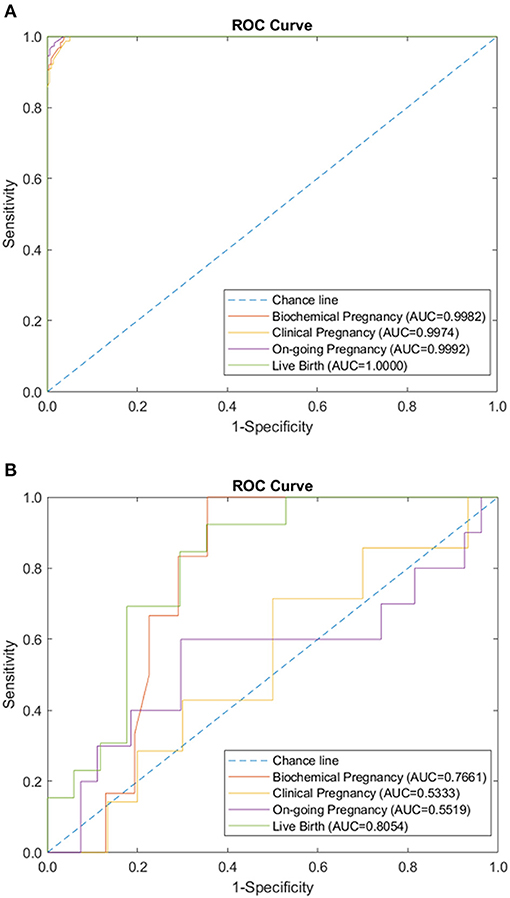
Figure 3. Endometrial immunology panel performance of sparse coding in predicting pregnancy outcomes at different pregnancy periods. (A) ROC plot of the training data set. (B) ROC plot of the testing data set.
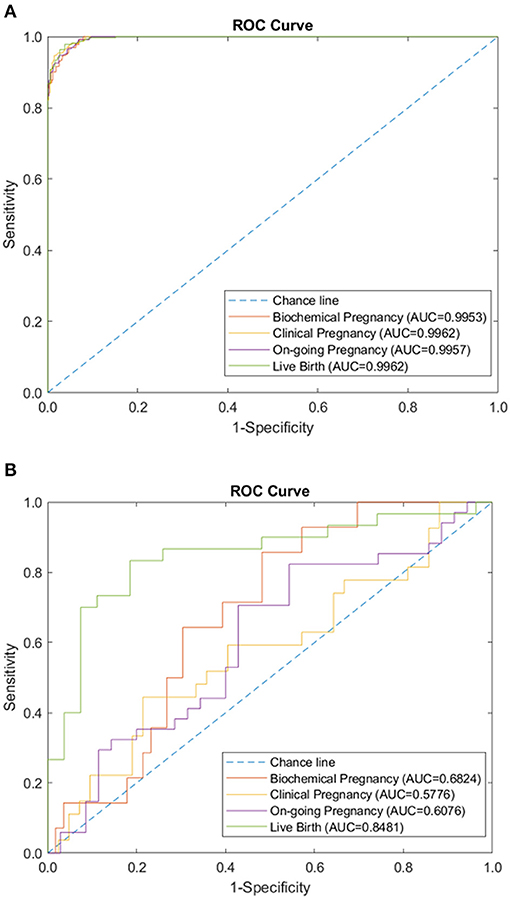
Figure 4. Combination of immunology-related panels (autoantibodies, peripheral immunology, and endometrial immunology) performance of sparse coding in predicting pregnancy outcomes at different pregnancy periods. (A) ROC plot of the training data set. (B) ROC plot of the testing data set.
Performance of Model on Combined Data Panels
Additionally, the sparse coding model was tested using both IVF-related data panels and immunological data panels. The AUC during the training phase was 1.0 for the prediction of all four pregnancy outcomes, using combined data panels. The AUC during the testing phase ranged from 0.661 to 0.793, following a similar lower trend, as in the case of immunological data panels (Figure 5). The use of combined data panels did not significantly enhance the effect on the prediction of all four pregnancy outcomes.
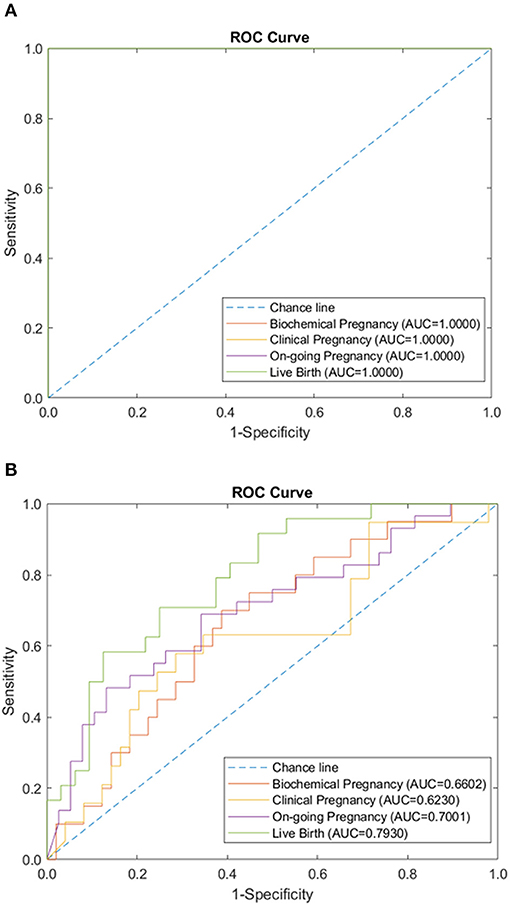
Figure 5. Combination of immunology and IVF-related panels (autoantibodies, peripheral immunology, endometrial immunology, basic patient characteristic, hormone level, and embryo parameter) performance of sparse coding in predicting pregnancy outcomes at different pregnancy periods. (A) ROC plot of the training data set. (B) ROC plot of the testing data set.
Discussion
A machine learning model was developed in this study for the prediction of the pregnancy outcomes for the patients with RRF at any gestational period, namely, biochemical pregnancy, clinical pregnancy, ongoing pregnancy, and live birth. The accuracy of the models for each stage in the testing data set ranged from 54.2 to 89.7%.
We observed that the performance of the endometrial immunology panel in biochemical pregnancy prediction was superior to the autoantibodies and peripheral immunology panel. Consistent with this result, it has been reported that implantation failure in ART is thought to be mainly due to impaired endometrial receptivity (35). In addition, implantation failure and miscarriage occurrence have been reported to have different mechanisms (36). Antiphospholipid syndrome (APS), which is characterized by the presence of anti-cardiolipin autoantibodies (ACAs), is the most common autoimmune disease associated with RPL (37). However, the association between ACAs and RIF is somewhat controversial (38). Anti-thyroid autoantibodies (ATA) have been also demonstrated to correlate with RPL, while, the association between ATA and RIF remains unclear (39–41). Our results showed that the AUC of the model using the autoantibodies panel in predicting clinical pregnancy, ongoing pregnancy, and live birth was the highest, while, the AUC for biochemical pregnancy prediction was the lowest. Subsequently, we can safely conclude that different models are appropriate for pregnancy outcome prediction at different pregnancy periods.
Machine learning algorithms have been widely used in many complex scenarios, such as image analysis, diagnosis, classification, and prognosis (42). Multiple machine learning techniques have been applied to improve the success rate of ART. The A.I. application in reproductive medicine has focused mainly on oocytes evaluation and selection (43), sperm analysis and selection (44), and embryo selection (45). A few studies have attempted to establish models for IVF outcome prediction (23, 46). Typical machine learning techniques such as Deep Artificial Neural Network (DANN) and Convolutional Neural Network (CNN) can be used to handle the high dimensionality data features, but very often these models are hard to interpret due to the “black-box” situation (47), which is usually not favored in biomedical applications. We adopted sparse coding which helps in the creation of an overcomplete information space composed of atom features with high dimensionality, which are critical to our model classifications. Simultaneously, the sparse representation of atoms can highlight the important features of patients using only a few atoms. It also enables us to visualize the features and interpret the classification or prediction results. To our knowledge, this is the first sparse coding-based prediction model based on reproductive big data including basic patient characteristics, hormone levels, immune status, and embryo parameter information for patients with RRF. This model represents an attempt at combining the reproductive immunology parameters with a machine learning algorithm.
In conventional clinical practice, clinicians can only provide the successful pregnancy probability to the patient according to the mean success rate of the fertility center. In addition to predicting pregnancy outcomes, clinicians are also concerned about developing effective treatment strategies based on the medical data of the patient. The models in the majority of the previous studies provided the live birth probability to the clinicians. Given the variation in the probability of success, the clinicians were unable to know how close the status of the patient is related to a successful pregnancy. The clinicians usually plan the treatment strategy according to the medical data of the patient and their experience on the underlying connection between different parameters. Interpreting the underlying relationships between medical data may influence the decision of the clinicians concerning treatment strategies. Future studies can include a thorough analysis of the immune status of the patients, by comparing the atoms which contribute to a successful pregnancy, generated in the sparse representation and assist clinicians to develop more personalized treatment strategies based on the comparison result.
Several limitations of this study need to be considered. First, the entire data set of this study was derived from a single reproductive immunology center. Second, other factors that potentially affect pregnancy outcomes, such as lifestyle (e.g., smoking history) and family genetic history, were not taken into consideration in our study. Finally, the performance of our model is related to the quantity and quality of the data. Therefore, the model presented here needs further study with more multi-center clinical data before full implementation in a clinical setting. Moreover, the current A.I. is mainly used as a support system to improve the accuracy and efficacy of the clinicians, rather than a stand-alone decision-making system. The clinicians should collaborate with algorithm engineers to continually optimize the model, as it is applied in clinical work.
Data Availability Statement
The raw data supporting the conclusions of this article will be made available by the authors, without undue reservation.
Ethics Statement
The studies involving human participants were reviewed and approved by Ethics Committee of Shenzhen Zhongshan Urology Hospital, Shenzhen, China. Written informed consent for participation was not required for this study in accordance with the national legislation and the institutional requirements.
Author Contributions
WT, YZe, and WL were involved in the study design. CH, ZX, and WL were involved in the organization of the entire project, data analysis with a clinical perspective, and manuscript writing. DT, CY, and LW were involved in the establishment of the algorithm. YZh, YL, SY, and LD were involved in collecting and preparing the clinical data. ZL was mainly involved in the literature review about A.I. and reproductive medicine. All authors contributed to the article and approved the submitted version.
Funding
This present study was financially supported by the Clinical Research Fund of Chinese Medical Association (No. 18010110740), the Shenzhen Healthcare Research Project (No. SZXJ2018004), the National Key Research & Developmental Program of China (No. 2018YFC1003900/2018YFC1003904), Chinese National Natural Science Foundation of China (31570898), and Natural Science Foundation of Guangdong Province, China (2016A030313112).
Conflict of Interest
DT, CY, LW, and WL were employed by the company ALOM Intelligence Limited, Hong Kong, China.
The remaining authors declare that the research was conducted in the absence of any commercial or financial relationships that could be construed as a potential conflict of interest.
Acknowledgments
We want to thank Chloe Ran Tu, a summer student from UCLA for her technical assistance and help with data analysis.
References
1. Billington WD. The immunological problem of pregnancy: 50 years with the hope of progress. A tribute to Peter Medawar. J Reprod Immunol. (2003) 60:1–11. doi: 10.1016/S0165-0378(03)00083-4
2. PrabhuDas M, Bonney E, Caron K, Dey S, Erlebacher A, Fazleabas A, et al. Immune mechanisms at the maternal-fetal interface: perspectives and challenges. Nat Immunol. (2015) 16:328–34. doi: 10.1038/ni.3131
3. Liu S, Diao L, Huang C, Li Y, Zeng Y, Kwak-Kim JYH. The role of decidual immune cells on human pregnancy. J Reprod Immunol. (2017) 124:44–53. doi: 10.1016/j.jri.2017.10.045
4. Practice Committee of the American Society for Reproductive Medicine Evaluation and treatment of recurrent pregnancy loss: a committee opinion. Fertil Steril. (2012) 98:1103–11. doi: 10.1016/j.fertnstert.2012.06.048
5. Margalioth EJ, Ben-Chetrit A, Gal M, Eldar-Geva T. Investigation and treatment of repeated implantation failure following IVF-ET. Hum Reprod. (2006) 21:3036–43. doi: 10.1093/humrep/del305
6. Simopoulou M, Sfakianoudis K, Maziotis E, Antoniou N, Rapani A, Anifandis G, et al. Are computational applications the “crystal ball” in the IVF laboratory? The evolution from mathematics to artificial intelligence. J Assist Reprod Genet. (2018) 35:1545–57. doi: 10.1007/s10815-018-1266-6
7. Blank C, Wildeboer RR, DeCroo I. Prediction of implantation after blastocyst transfer in in vitro fertilization: a machine-learning perspective. Fertil Steril. (2019) 111:318–26. doi: 10.1016/j.fertnstert.2018.10.030
8. Vogiatzi P, Pouliakis A, Siristatidis C. An artificial neural network for the prediction of assisted reproduction outcome. J Assist Reprod Genet. (2019) 36:1441–8. doi: 10.1007/s10815-019-01498-7
9. Kaufmann SJ, Eastaugh JL, Snowden S, Smye SW, Sharma V. The application of neural networks in predicting the outcome of in-vitro fertilization. Hum Reprod. (1997) 12:1454–7. doi: 10.1093/humrep/12.7.1454
10. Curchoe CL, Bormann CL. Artificial intelligence and machine learning for human reproduction and embryology presented at ASRM and ESHRE 2018. J Assist Reprod Genet. (2019) 36:591–600. doi: 10.1007/s10815-019-01408-x
11. Basheer IA, Hajmeer M. Artificial neural networks: fundamentals, computing, design, and application. J Microbiol Methods. (2000) 43:3–31. doi: 10.1016/S0167-7012(00)00201-3
12. Deng Y, Dai Q, Zhang Z. An overview of computational sparse models and their applications in artificial intelligence. In: Yang XS, editor. Artificial Intelligence, Evolutionary Computing and Metaheuristics. Studies in Computational Intelligence, Vol. 427. Berlin; Heidelberg: Springer (2013). doi: 10.1007/978-3-642-29694-9_14
13. Roy S, He Q, Sweeney E, Carass A, Reich DS, Prince JL, et al. Subject-specific sparse dictionary learning for atlas-based brain MRI segmentation. IEEE J Biomed Health Inform. (2015) 19:1598–609. doi: 10.1109/JBHI.2015.2439242
14. Li Y, Ngom A. Sparse representation approaches for the classification of high-dimensional biological data. BMC Syst Biol. (2013) 7:1–14. doi: 10.1186/1752-0509-7-S4-S6
15. Codella N, Cai J, Abedini M, Garnavi R, Halpern A, Smith JR. Deep learning, sparse coding, and SVM for melanoma recognition in dermoscopy images. In: Zhou L, Wang L, Wang Q, Shi Y, editors. Machine Learning in Medical Imaging. MLMI 2015. Lecture Notes in Computer Science. Vol. 9352. Cham: Springer (2015). doi: 10.1007/978-3-319-24888-2_15
16. Hang X, Wu FX. Sparse representation for classification of tumors using gene expression data. J Biomed Biotechnol. (2009) 2009:403689. doi: 10.1155/2009/403689
17. Camacho DM, Collins KM, Powers RK, Costello JC, Collins JJ. Next-generation machine learning for biological networks. Cell. (2018) 173:1581–92. doi: 10.1016/j.cell.2018.05.015
18. Deo RC. Machine learning in medicine. Circulation. (2015) 132:1920–30. doi: 10.1161/CIRCULATIONAHA.115.001593
19. Obermeyer Z, Emanuel EJ. Predicting the future — big data, machine learning, and clinical medicine. N Engl J Med. (2016) 375:1216–9. doi: 10.1056/NEJMp1606181
20. Adamker G, Holzer T, Karakis I, Amitay M, Anis E, Singer SR, et al. Prediction of Shigellosis outcomes in Israel using machine learning classifiers. Epidemiol Infect. (2018) 146:1445–51. doi: 10.1017/S0950268818001498
21. Hassan MR, Al-Insaif S, Hossain MI, Kamruzzaman J. A machine learning approach for prediction of pregnancy outcome following IVF treatment. Neural Comput Appl. (2018) 32:2283–97. doi: 10.1007/s00521-018-3693-9
22. Elson J, Tailor A, Banerjee S, Salim R, Hillaby K, Jurkovic D. Expectant management of tubal ectopic pregnancy:Prediction of successful outcome using decision tree analysis. Ultrasound Obstet Gynecol. (2004) 23:552–6. doi: 10.1002/uog.1061
23. Hafiz P, Nematollahi M, Boostani R, Jahromi BN. Predicting implantation outcome of in vitro fertilization and intracytoplasmic sperm injection using data mining techniques. Int J Fertil Steril. (2017) 11:184–90. doi: 10.22074/ijfs.2017.4882
24. Ghaeini MK, Amin-Naseri MR, Aghahoseini M. Prediction of clinical pregnancy occurrence after ICSI using decision tree and support vector machine methods. J Obstetr Gynecol Cancer Res. (2018) 3:149–55. doi: 10.30699/jogcr.3.4.12
25. Bruno V, D'Orazio M, Ticconi C, Abundo P, Riccio S, Martinelli E, et al. Machine learning (ML) based-method applied in recurrent pregnancy loss (RPL) patients diagnostic work-up: a potential innovation in common clinical practice. Sci Rep. (2020) 10:7970. doi: 10.1038/s41598-020-64512-4
26. Li G, Raza SEA, Rajpoot NM. Multi-resolution cell orientation congruence descriptors for epithelium segmentation in endometrial histology images. Med Image Anal. (2017) 37:91–100. doi: 10.1016/j.media.2017.01.006
27. Mora-Sanchez A, Aguilar-Salvador DI, Nowak I. Towards a gamete matching platform: using immunogenetics and artificial intelligence to predict recurrent miscarriage. NPJ Digit Med. (2019) 2:12. doi: 10.1038/s41746-019-0089-x
28. Ramos-Medina R, Garcia-Segovia A, Gil J, Carbone J, Aguaron de la Cruz A, Seyfferth A, et al. Experience in IVIg therapy for selected women with recurrent reproductive failure and NK cell expansion. Am J Reprod Immunol. (2014) 71:458–66. doi: 10.1111/aji.12217
29. Dhillon RK, McLernon DJ, Smith PP, Fishel S, Dowell K, Deeks JJ, et al. Predicting the chance of live birth for women undergoing IVF: a novel pretreatment counselling tool. Hum Reprod. (2016) 31:84–92. doi: 10.1093/humrep/dev268
30. Milewski R, Kuczynska A, Stankiewicz B, Kuczynski W. How much information about embryo implantation potential is included in morphokinetic data? A prediction model based on artificial neural networks and principal component analysis. Adv Med Sci. (2017) 62:202–6. doi: 10.1016/j.advms.2017.02.001
31. Vaegter KK, Lakic TG, Olovsson M, Berglund L, Brodin T, Holte J. Which factors are most predictive for live birth after in vitro fertilization and intracytoplasmic sperm injection (IVF/ICSI) treatments? Analysis of 100 prospectively recorded variables in 8,400 IVF/ICSI single-embryo transfers. Fert Steril. (2017) 107:641–8.e2. doi: 10.1016/j.fertnstert.2016.12.005
32. Qiu J, Li P, Dong M, Xin X, Tan J. Personalized prediction of live birth prior to the first in vitro fertilization treatment: a machine learning method. J Transl Med. (2019) 17:317. doi: 10.1186/s12967-019-2062-5
33. Barnett-Itzhaki Z, Elbaz M, Butterman R, Amar D, Amitay M, Racowsky C, et al. Machine learning vs. classic statistics for the prediction of IVF outcomes. J Assist Reprod Genet. (2020) 37:2405–12. doi: 10.1007/s10815-020-01908-1
34. Gregor K, LeCun Y. Learning fast approximations of sparse coding. In: Proceedings of the 27th International Conference on International Conference on Machine Learning. Haifa: Omnipress (2010). p. 399–406.
35. Fatemi HM, Popovic-Todorovic B. Implantation in assisted reproduction: a look at endometrial receptivity. Reprod Biomed Online. (2013) 27:530–8. doi: 10.1016/j.rbmo.2013.05.018
36. Huang J, Qin H, Yang Y, Chen X, Zhang J, Laird S, et al. A comparison of transcriptomic profiles in endometrium during window of implantation between women with unexplained recurrent implantation failure and recurrent miscarriage. Reproduction. (2017) 153:749–58. doi: 10.1530/REP-16-0574
37. Cervera R, Balasch J. Bidirectional effects on autoimmunity and reproduction. Hum Reprod Update. (2008) 14:359–66. doi: 10.1093/humupd/dmn013
38. Hornstein MD, Davis OK, Massey JB, Paulson RJ, Collins JA. Antiphospholipid antibodies and in vitro fertilization success: a meta-analysis. Fertil Steril. (2000) 73:330–3. doi: 10.1016/S0015-0282(99)00498-7
39. Matalon ST, Blank M, Ornoy A, Shoenfeld Y. The association between anti-thyroid antibodies and pregnancy loss. Am J Reprod Immunol. (2001) 45:72–7. doi: 10.1111/j.8755-8920.2001.450202.x
40. Bussen S, Steck T, Dietl J. Increased prevalence of thyroid antibodies in euthyroid women with a history of recurrent in-vitro fertilization failure. Hum Reprod. (2000) 15:545–8. doi: 10.1093/humrep/15.3.545
41. Negro R, Formoso G, Coppola L, Presicce G, Mangieri T, Pezzarossa A, et al. Euthyroid women with autoimmune disease undergoing assisted reproduction technologies: the role of autoimmunity and thyroid function. J Endocrinol Invest. (2007) 30:3–8. doi: 10.1007/BF03347388
42. Ngiam KY, Khor IW. Big data and machine learning algorithms for health-care delivery. Lancet Oncol. (2019) 20:e262–73. doi: 10.1016/S1470-2045(19)30149-4
43. Cavalera F, Zanoni M, Merico V, Bui TTH, Belli M, Fassina L, et al. A neural network-based identification of developmentally competent or incompetent mouse fully-grown oocytes. J Vis Exp. (2018) 133:56668. doi: 10.3791/56668
44. Goodson SG, White S, Stevans AM, Bhat S, Kao CY, Jaworski S, et al. CASAnova: a multiclass support vector machine model for the classification of human sperm motility patterns. Biol Reprod. (2017) 97:698–708. doi: 10.1093/biolre/iox120
45. Storr A, Venetis C, Cooke S, Kilani S, Ledger W. Time-lapse algorithms and morphological selection of day-5 embryos for transfer: a preclinical validation study. Fertil Steril. (2018) 109:276–83. e3. doi: 10.1016/j.fertnstert.2017.10.036
46. Guvenir HA, Misirli G, Dilbaz S, Ozdegirmenci O, Demir B, Dilbaz B. Estimating the chance of success in IVF treatment using a ranking algorithm. Med Biol Eng Comput. (2015) 53:911–20. doi: 10.1007/s11517-015-1299-2
Keywords: artificial intelligence, recurrent reproductive failure, reproductive immunology, sparse coding, assisted reproductive technology
Citation: Huang C, Xiang Z, Zhang Y, Tan DS, Yip CK, Liu Z, Li Y, Yu S, Diao L, Wong LY, Ling WL, Zeng Y and Tu W (2021) Using Deep Learning in a Monocentric Study to Characterize Maternal Immune Environment for Predicting Pregnancy Outcomes in the Recurrent Reproductive Failure Patients. Front. Immunol. 12:642167. doi: 10.3389/fimmu.2021.642167
Received: 15 December 2020; Accepted: 15 February 2021;
Published: 01 April 2021.
Edited by:
Andrew L. Mellor, Newcastle University, United KingdomReviewed by:
Gerard Chaouat, INSERM U976 Immunologie, Dermatologie, Oncologie, FranceStefano Ugel, University of Verona, Italy
Copyright © 2021 Huang, Xiang, Zhang, Tan, Yip, Liu, Li, Yu, Diao, Wong, Ling, Zeng and Tu. This is an open-access article distributed under the terms of the Creative Commons Attribution License (CC BY). The use, distribution or reproduction in other forums is permitted, provided the original author(s) and the copyright owner(s) are credited and that the original publication in this journal is cited, in accordance with accepted academic practice. No use, distribution or reproduction is permitted which does not comply with these terms.
*Correspondence: Wenwei Tu, d3d0dUBoa3UuaGs=; Yong Zeng, emVuZ3lvbmcxOTY2QGdtYWlsLmNvbQ==; Wai Lim Ling, d2lsbGlhbUBhbG9tLmFp
†These authors have contributed equally to this work