- 1Department of Geriatrics, National Key Clinical Specialty, Guangzhou First People’s Hospital, School of Medicine, South China University of Technology, Guangzhou, China
- 2Center for Bioinformatics and Genomics, Department of Global Biostatistics and Data Science, Tulane University, New Orleans, LA, United States
- 3Shunde Hospital of Southern Medical University (The First People’s Hospital of Shunde), Foshan City, China
- 4Department of Endocrinology and Metabolism, The Third Affiliated Hospital of Southern Medical University, Guangzhou, China
- 5Department of Endocrinology and Metabolism, The Fifth Affiliated Hospital of Guangzhou Medical University, Guangzhou, China
Strong relationships have been found between appendicular lean mass (ALM) and bone mineral density (BMD). It may be due to a shared genetic basis, termed pleiotropy. By leveraging the pleiotropy with BMD, the aim of this study was to detect more potential genetic variants for ALM. Using the conditional false discovery rate (cFDR) methodology, a combined analysis of the summary statistics of two large independent genome wide association studies (GWAS) of ALM (n = 73,420) and BMD (n = 10,414) was conducted. Strong pleiotropic enrichment and 26 novel potential pleiotropic SNPs were found for ALM and BMD. We identified 156 SNPs for ALM (cFDR <0.05), of which 74 were replicates of previous GWASs and 82 were novel SNPs potentially-associated with ALM. Eleven genes annotated by 31 novel SNPs (13 pleiotropic and 18 ALM specific) were partially validated in a gene expression assay. Functional enrichment analysis indicated that genes corresponding to the novel potential SNPs were enriched in GO terms and/or KEGG pathways that played important roles in muscle development and/or BMD metabolism (adjP <0.05). In protein–protein interaction analysis, rich interactions were demonstrated among the proteins produced by the corresponding genes. In conclusion, the present study, as in other recent studies we have conducted, demonstrated superior efficiency and reliability of the cFDR methodology for enhanced detection of trait-associated genetic variants. Our findings shed novel insight into the genetic variability of ALM in addition to the shared genetic basis underlying ALM and BMD.
Introduction
Sarcopenia, a “geriatric giant” characterized by progressive loss of muscle mass and strength, is associated with increased risk of falls, frailty and disability. Appendicular lean mass (ALM) is a major index that defines sarcopenia (1), a condition that causes heavy economic burden to healthcare systems, the annual cost of which in the United States alone is more than 18 billion dollars (2). Sarcopenia is a highly heritable complex disease, lean mass (LM), having a heritability estimated to be 0.52–0.60 (3, 4). Previous genome wide association studies (GWAS) have identified a number of loci and genes associated with LM. For example, loci are present in TRHR (5), GLYAT (6), PRDM16 (7) and TNRC6B (8). Furthermore, the latest large-scale GWAS has just identified and replicated five loci strongly associated with LM at a genome-wide level of significance (9), and a recent ALM GWAS study identified 1059 conditionally independent variants from 799 loci with a genome-wide significance level (p <5 × 10−9) using the UKB cohort (10). These findings help us better understand the genetic background of sarcopenia, but because of the high heritability of LM, the current findings are extremely limited, a missing heritability is evident. To further elucidate the genetic mechanisms of sarcopenia, deeper exploration of LM-associated genetic variants are required.
Epidemiological and clinical studies have shown multiple relationships between LM and bone mineral density (BMD). Both are complex traits with high degrees of genetic determination. The estimated heritability of BMD is 0.75–0.83 (11, 12). Sarcopenia and osteoporosis are both diseases related to aging, the loss of muscle mass and strength decreases the mechanical load on bone, thus causing a reduction in BMD (13). It was reported that hip BMD is positively associated with skeletal muscle strength and mass (13, 14). BMD and LM share common risk factors, such as aging, long-term confinement in bed and inflammatory factors. The same molecular pathways were also reported to be associated with both osteoporosis and sarcopenia, for example, the Wnt signaling pathway (15, 16). The underlying mechanism of the association between LM and BMD remains unclear. We hypothesize that it might partially result from the pleiotropic effect, namely that a single gene can influence multiple traits. In 2017, the pleiotropic effect of LM and BMD were first reported at the locus SREBF1/TOM1L2 in the pediatric population (17). Although the findings were limited, it strongly supported our hypothesis of pleiotropy between LM and BMD. By leveraging the power of pleiotropy and using appropriate analytical methods we may ascertain a greater number of potential pleiotropic variants in addition to trait-associated variants for ALM and/or BMD.
Recently, an analytical method—pleiotropy-informed conditional false discovery rate (cFDR)—was successfully applied to GWAS datasets of correlated phenotypes. According to the simulation, while comparing to the unconditional FDR method, cFDR method was able to increase the discovery of non-null trait-associated single nucleotide polymorphisms (SNP) by 15–20 fold during single phenotype analysis (18). The cFDR methodology provides the opportunity to identify a greater number of novel potential variants in addition to pleiotropic variants for highly correlated phenotypes without recruiting additional samples (18, 19). In our recent studies, we successfully implemented the cFDR methodology on a number of correlated phenotypes. Not only did we discover novel potential genetic variants for single phenotypes, we also uncovered potential common variants for highly-associated traits, such as BMD with height, birth weight with type 2 diabetes, and BMD with coronary heart disease (19–21). In this study, we utilized the cFDR methodology in two independent large scale GWAS datasets of ALM and BMD, aiming to find additional novel potential genetic variants for ALM in addition to potential common variants for both ALM and BMD.
Methods and Materials
ALM and BMD GWAS Datasets
ALM and BMD GWAS summary statistics were obtained from the Genetic Factors for Osteoporosis Consortium (GEFOS). Both sets of data were released in 2017. The ALM datasets contain summary statistics from adolescent populations of European, African American and Korean descent (n = 73,420), including 2,699,262 SNPs (9). The BMD datasets contain summary statistics of total body BMD (excluding the head) from European and African American populations in four pediatric cohort studies (n = 10,414), including a total of 2,276,810 SNPs (17). Genetic factors are the principal determinants of BMD at any age, with BMD in childhood highly correlated with peak BMD in adulthood (22). ALM and BMD GWASs are independent with no overlapping subjects. The inclusion criteria and phenotypic characteristics of ALM and BMD GWASs are described in detail in the original publications (9, 17)
Data Processing
Based on HapMap 3 genotypes and the linkage disequilibrium (LD) SNP pruning method, we pruned ALM and BMD data using PLINK software. The linkage disequilibrium (LD) value for each pair of SNPs was calculated. For SNP pairs with r2 >0.2, the SNP with the smaller minor allele frequency (MAF) was deleted so that no pairs of SNPs with high LD remained (18). After pruning, there were 1,098,760 SNPs remaining for ALM and 996,515 SNPs for BMD. The ALM data was then combined with the BMD data based on common SNPs, resulting in 976,149 SNPs remaining for subsequent cFDR analysis. The direction of effect of an SNP on ALM was ascertained from the “Effect” entry in the original ALM data, and ascertained by the “Beta” value for BMD in the original BMD data. If the Beta value of the SNP was higher than 0.00, the direction of effect of the SNP was termed “+”, if the Beta value was lower than 0.00, the direction was “−”. “+” indicates a positive effect of the reference allele, “−” indicating a negative effect of the reference allele. Genomic control was conducted in the original GWASs so that the estimated variance of each SNP would not be inflated due to the structure of the population (9, 17).
Statistical Analysis
Estimation of Pleiotropic Enrichment
To evaluate whether the primary phenotype was related to the phenotype under study by the null hypothesis, a quantile–quantile plot (Q–Q plot) was created using the “ggplot2” module in R software. Q–Q plot is a graphical tool that represents pleiotropic enrichment between the nominal and empirical traits. Nominal −log10(p) values are plotted on the Y-axis and the empirical quantiles (−log10(q)) on the X-axis. The Q–Q curve is plotted for the quantile of nominal −log10(p) values for association of the subset of variants that are below differential significance thresholds in the conditional trait. Pleiotropic enrichment can then be observed intuitively as each curve gradually deviates up leftwards from the expected identify line.
Calculation of cFDR and Conjunction cFDR (ccFDR)
The cFDR methodology was developed from that of traditional FDR (23). Its value relates to the false probability that a random SNP is not associated with the principal phenotype given that the p-value of this SNP for the principal phenotype and conditional phenotype are both less than two pre-defined trait-specific significance thresholds (18). In this study, we computed a cFDR value for each SNP while referencing ALM as the principal phenotype and BMD as the conditional phenotype (ALM|BMD), then in reverse, with BMD conditional on ALM (BMD|ALM). The ccFDR was computed as the maximum cFDR value of the two phenotypes, referring to the false possibility that the SNP is associated with neither of the two phenotypes, and used to identify potential pleiotropic SNPs that are associated with both phenotypes. cFDR and ccFDR thresholds were set to 0.05. SNPs with cFDR or ccFDR values lower than 0.05 were considered significantly associated with the corresponding phenotype or both phenotypes, with the false discovery rate lower than 0.05. The detailed procedure for calculating cFDR and ccFDR is described in previous publications (19, 20). To mark the chromosomal positions and significance of cFDR or ccFDR values of the SNPs, we used R software to plot cFDR and ccFDR Manhattan plots. Significant cFDR or ccFDR SNPs were plotted above the red line at 1.3 (corresponding to cFDR or ccFDR values lower than 0.05).
Annotation of Novel Potential Pleiotropic SNPs and Potential ALM-Associated SNPs
To verify whether the SNPs significant in cFDR and ccFDR were novel, we compared them with previous GWAS findings. SNPs that had been confirmed to be associated with only ALM and BMD were not available, so we downloaded the SNPs that had been confirmed to be associated with LM and BMD in previous GWASs (Supplementary Table 2), and SNPs associated with LM (Supplementary Table 4) from the European Bioinformatics Institute website (https://www.ebi.ac.uk/gwas, Accessed Jan 2020). Based on HapMap3 and using R2 0.8 as a threshold (24), we then inputted SNPs significant in ccFDR or cFDR and the SNPs previously confirmed by GWAS into the SNP Annotation and Proxy Search tool (SNAP, http://archive.broadinstitute.org/mpg/snap) and Ldlink (https://ldlink.nci.nih.gov/?tab=home) to perform LD analysis. Only SNPs not clustered in the same LD block with previous GWAS findings were considered as potential novel SNPs, otherwise they were regarded as replication of previous GWAS findings. LD block refers to genomic segment consisting of adjacent genetic polymorphisms with high probability (e.g. R2 >0.8) of non-random association of alleles.
Gene Expression Validation Analysis
Gene expression profiling of mRNA levels in vastus lateralis muscle biopsies was used to explore whether genes corresponding to potential ALM-associated SNPs were expressed differentially between young and old adults (25). A Wilcoxon Rank Sum test, which is a rank-based non-parametric test that compares two groups of expression profiles with no assumption of normality, was computed for the mRNA levels of the genes in vastus lateralis muscle biopsies from 16 young adults (eight men and eight women, mean age: 24 years) and 12 old adults (six men and six women, mean age: 84 years). Details of the gene expression profiling of the muscle biopsies and how it was conducted are described in the original publication (25).
In addition to the vastus lateralis muscle biopsies, gene expression validation analysis was also performed on bone tissue for genes corresponding to potential pleiotropic SNPs that had been identified by ccFDR. Using gene expression profiling in iliac bone biopsies from 84 postmenopausal Caucasian women (26), the correlation coefficients were calculated of whole body BMD against mRNA levels of genes significant by ccFDR. Details of the gene expression profiling of iliac bone biopsies is described in the original publication (26).
Protein–Protein Interaction Analysis and Functional Term Enrichment Analysis
SNPs identified by cFDR were mapped to their corresponding genes using the online tool SNPinfo (https://snpinfo.niehs.nih.gov/). The ALM-associated genes found to be significant by cFDR were inputted into the online database STRING 10.0 (http://string-db.org) to perform protein-protein interaction (PPI) analysis, enabling further exploration of the biological functional role of the proteins produced by ALM-associated genes. Functional term enrichment analysis was conducted using the Web-Based Gene Set Analysis Toolkit (27), assisting in partial validation of results by discovering gene sets in gene ontology (GO) terms and KEGG canonical pathways related to muscle development and/or bone metabolism. GO categories were pre-filtered for redundancy using the online tool REVIGO (28). For GO and KEGG enrichment analysis, an adjusted p value (adjP) lower than 0.05 was regarded as significant. adjP is the p value adjusted by multiple test adjustments.
Results
Pleiotropic Enrichment Assessment and Novel Potentially Pleiotropic SNPs Identified by ccFDR
As presented in Figure 1, the curves gradually diverge from the expected baseline, with strong pleiotropic enrichment observed for ALM SNPs across different levels of association with BMD (ALM|BMD) in addition to the converse, for BMD SNPs conditioning on ALM (BMD|ALM). A total of 55 potential pleiotropic SNPs mapping to five chromosomes were identified by ccFDR that associated with both ALM and BMD (Supplementary Figure 1 and Supplementary Table 1). We compared the results from this study with the 12 pleiotropic SNPs reported by a recent bivariate GWAS meta-analysis of pleiotropic effect on LM and BMD (Supplementary Table 2). Interestingly, two previously reported pleiotropic SNPs, rs12284933 and rs754388, were replicated in the present study (SNPs marked * in Supplementary Table 1). Twenty seven potentially pleiotropic SNPs identified by ccFDR resided in the same LD block as the previously reported 12 pleiotropic SNPs (SNPs marked ** in Supplementary Table 1). These 29 SNPs identified by ccFDR are considered a successful replication of previous GWAS findings. A total of 26 novel potential pleiotropic SNPs mapping to five chromosomes were identified by ccFDR for ALM and BMD (Table 1). Some 16 of these 26 novel potential pleiotropic SNPs have the same direction of effect on both ALM and BMD. Of the 26 novel potential pleiotropic SNPs, there are eight SNPs located in LRP5, seven in PPP6R3, four near RUNX2 and CLIC5, and three SNPs near BMP2 and FUSIP1P2. Interestingly, the loci PPP6R3 and RIN3 have been previously reported in a previous GWAS analysis to be associated with both BMD and LM (17).
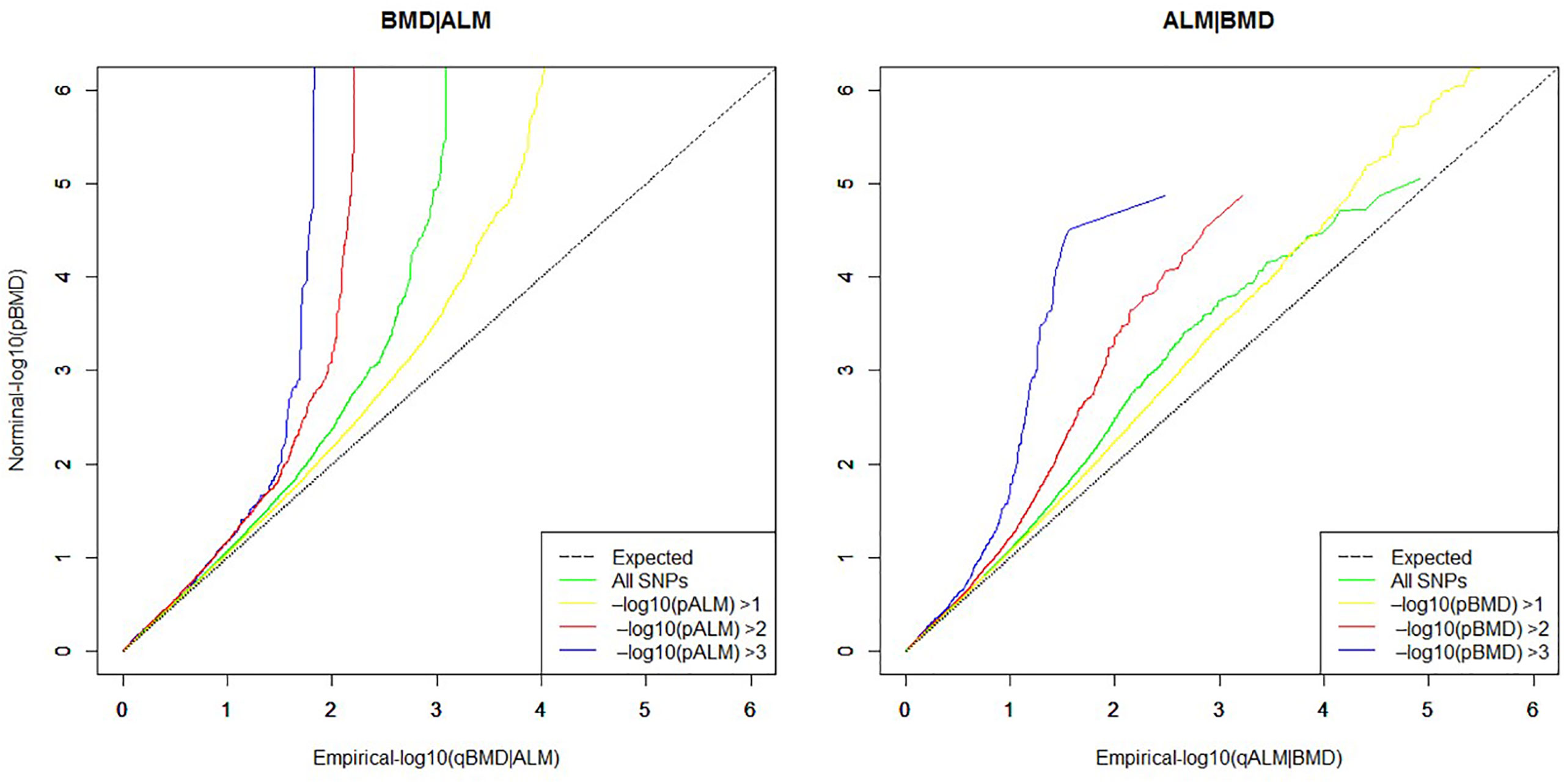
Figure 1 Quantile–quantile plots (Q–Q plots) for ALM as a function of significance of association with BMD (ALM|BMD), and as the reverse, BMD as a function of significance of association with ALM (BMD|ALM).
Novel Potential ALM-Associated SNPs Identified by cFDR
In this study, a total of 156 potential ALM-associated SNPs mapping to 15 chromosomes were identified by cFDR (Supplementary Figure 2 and Supplementary Table 3). We compared the SNPs found to be significant by cFDR with previous GWAS findings (Supplementary Table 4) and the latest findings by Pei et al. (10). Eight LM-associated SNPs (rs12284933, rs12741884, rs3765350, rs6591341, rs6726821, rs754388, rs917727 and rs9525638) reported by previous GWASs were replicated by cFDR (SNPs marked * in Supplementary Table 3), 66 ALM SNPs identified as significant by cFDR (SNPs marked ** in Supplementary Table 3) exhibited high LD with previously-confirmed LM-associated SNPs. These 74 SNPs were considered replicates of previous GWAS findings. In total, 82 novel potential SNPs for ALM were identified by cFDR (SNPs marked *** in Supplementary Table 3). Of the 82 novel SNPs, 11 were located in CPED1, nine in LRP5, nine near BMP2 and FUSIP1P2, six in or near PPP6R3 and six SNPs in or near WNT4. Genetic variants in or near CPED1, PPP6R3 and WNT4 have already been reported by previous GWASs to be associated with LM (Supplementary Table 3).
Gene Expression Validation Analysis for Potential Pleiotropic Genes and Potential ALM-Associated Genes
For the novel potential pleiotropic genes associated with both ALM and BMD, mRNA transcripts of four genes (PPP6R3, RIN3, CLIC5 and CLYBL) annotated by 13 novel potential pleiotropic SNPs expressed differentially on vastus lateralis muscle between young and old adults (Bond p.ALM value in the rightmost columns of Table 1). mRNA transcripts of four genes on iliac bone (PPP6R3, CLIC5, RUNX2 and MUS81) annotated by 17 novel potential pleiotropic SNPs were associated with whole body BMD (Bond p.BMD value in the rightmost columns of Table 1). PPP6R3 and CLIC5 were partially validated in both muscle and bone biopsies. For genes corresponding to novel potential ALM-associated SNPs, the mRNA level differences on vastus lateralis muscle between young and old adults were calculated and the p values were presented in the rightmost column of Table 2 and Supplementary Table 3. mRNA transcripts level of seven genes (PPP6R3, CLIC5, TNFSF11, RIN3, CLYBL, SMOC2 and CDC42) annotated by 18 novel potential ALM-associated SNPs expressed differentially on vastus lateralis muscle between young and compared with old adults (p value <0.05). This result suggests that these seven genes may partially participate in the aging of muscle.
Functional Enrichment Analysis of Potential Pleiotropic Genes and Potential ALM-Associated Genes
As shown in Supplementary Table 5, genes corresponding to novel, potentially pleiotropic SNPs were enriched in GO terms for factors that play an important role in muscle development and/or bone metabolism. For example, “osteoblast development” regulates bone formation, “regulation of hormone metabolic process” participates in both muscle development (29) and bone metabolism (30). No KEGG canonical pathway was found for the novel potentially pleiotropic genes, maybe because of the small number of genes that were found. Genes corresponding to novel potentially ALM-associated SNPs were found to be enriched in 10 GO terms (Table 3), of which “regulation of glucocorticoid metabolic process” possessed the lowest adjusted p value. Glucocorticoid participates in the process of ALM development and could induce muscle cell atrophy (31). The results of KEGG enrichment analysis for novel potentially ALM-associated genes are presented in Table 4. In total, 10 KEGG pathways were enriched in, and the majority related to, cancer of different tissues. Cancer occurs in numerous tissues, including muscle, for example, rhabdomyosarcoma and leiomyosarcoma.
Protein–Protein Interaction Analysis for Potential ALM-Associated Genes
As shown in Figure 2, multiple interactions were observed among proteins produced by genes corresponding to potential ALM-associated SNPs. LRP5, CPED1, BMP2, PPP6R3 and WNT4 had at least five novel potential ALM-associated SNPs, proteins produced by them all exhibited direct or indirect interactions with proteins produced by genes corresponding to other potential ALM-associated SNPs, such as WNT16, TNFSF11 and RUNX2. This suggests that these genes operate in a coordinated fashion in their functioning and significance for ALM. As reported in previous studies, LRP5 is a co-receptor of Wnt proteins and is able to transduce signals through the canonical Wnt pathway (32). CPED1 is located near WNT16. It has been reported that SNPs in WNT16 regulate CPED1 expression during lung development (33). The concentration of protein produced by BMP2 could influence myogenesis in mesenchymal cells (34), in addition to BMP2 also being a BMD-associated gene that plays a key role in bone formation.
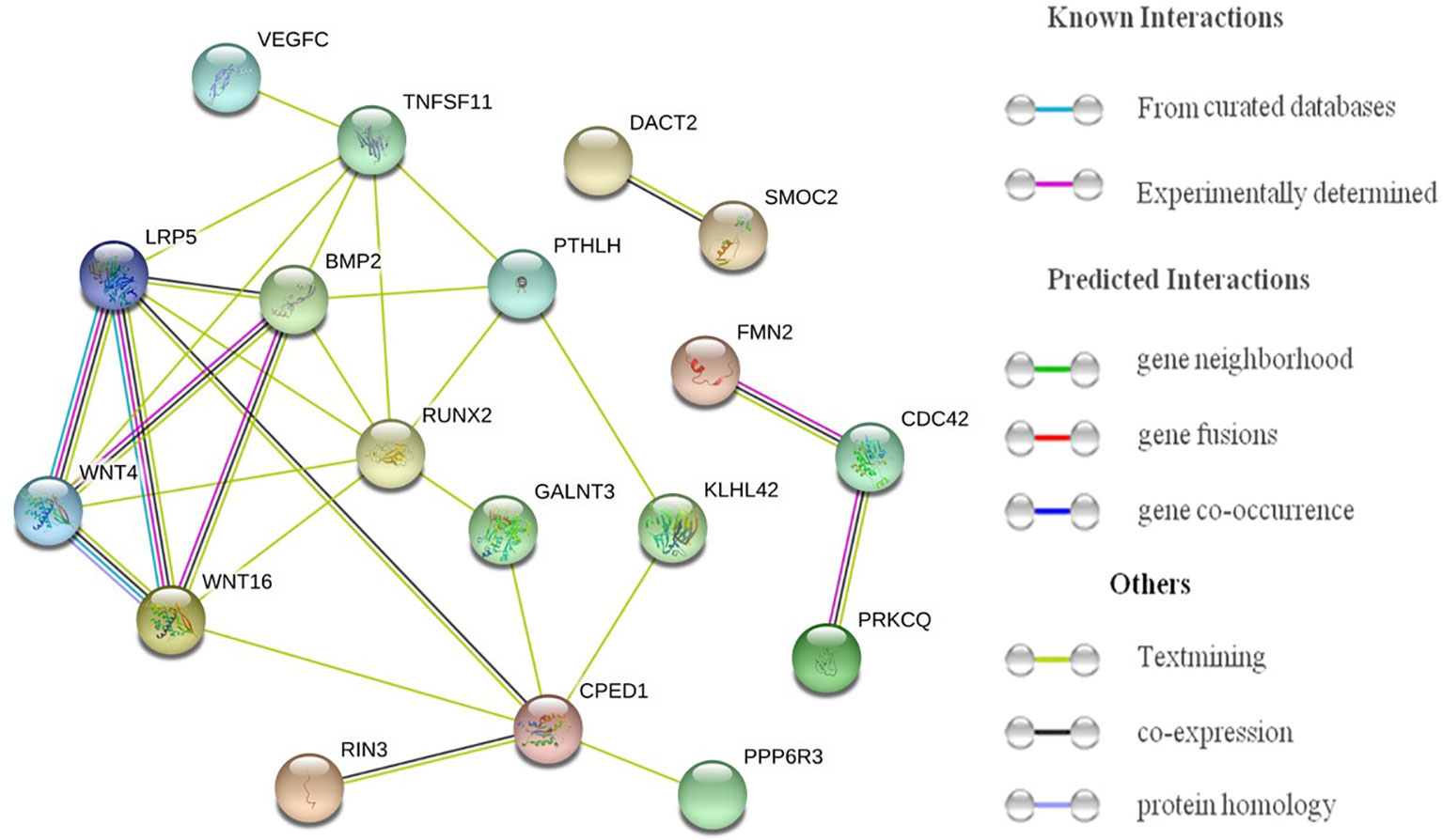
Figure 2 Plot of protein–protein interactions for genes corresponding to potential ALM-associated SNPs.
Discussion
In this study, we implemented cFDR methodology on the summary statistics of a large, independent GWAS of two highly-correlated phenotypes—ALM and BMD, successfully identifying 55 potential pleiotropic SNPs related to the two traits. Of these, 29 had successfully replicated results of previous GWAS findings, with 26 totally novel potential pleiotropic SNPs, identified by ccFDR. The mRNA transcripts of two genes (PPP6R3 and CLIC5) annotated by 11 novel potential pleiotropic SNPs were partially validated in both bone and muscle gene expression validation analysis. Furthermore, by leveraging the power of pleiotropy with BMD, we identified 156 potential ALM-associated SNPs without the recruitment of any additional participants. Of the 156 potential ALM-associated SNPs, 74 have been identified in previous LM GWASs or with high LD in previous LM GWAS findings. Eighty two SNPs were novel potential ALM-associated SNPs identified by cFDR. Seven genes annotated by 18 novel potential ALM-associated SNPs were partially validated in muscle gene expression analysis. Genes found to be significant by ccFDR and/or cFDR were enriched in GO terms and/or KEGG pathways related to muscle development and/or bone metabolism. The present study demonstrated the reliability and enhanced power of cFDR methodology by detecting potential pleiotropic variants in addition to trait-specific variants for complex traits and diseases.
In pleiotropic variant analysis, LRP5 was found to be the gene with the highest number of novel potential pleiotropic SNPs (8 SNPs mapped in total). LRP5 is located on chromosome 11 and plays a key role in bone metabolism. It was confirmed in a large GWAS that LRP5 is associated with BMD (35). In mice, mutations in LRP5 were found to be associated with low BMD and abnormal retinal vasculature (36), while in humans, mutations in LRP5 were found to cause disorders characterized by high BMD and osteoporosis–pseudoglioma syndrome, causing severe osteoporosis and blindness (37). In addition to effects on bone, LRP5 also participates in muscle development. As a co-receptor of Wnt proteins, LRP5 transduces Wnt signaling and regulates the steps in the process of myogenesis, such as myoblast fusion and cell proliferation (32). Given that the highest number of novel potential pleiotropic SNPs was associated with LRP5 in ccFDR analysis, combined with its confirmed role in myogenesis and bone metabolism, we speculate that LRP5 may be a pleiotropic gene for ALM and BMD. Additional experiments should focus on this gene to explore the underlying mechanism of pleiotropy between the two traits.
Besides LRP5, PPP6R3 is also noteworthy as it is annotated by seven novel potential pleiotropic SNPs. It is one of two genes partially validated in both muscle and bone gene expression analysis. PPP6R3 is located on chromosome 11 and can modulate the activity of protein phosphatase catalytic subunits. High expression of transcript of PPP6R3 has been detected in skeletal muscle (38) and bone (39), and the SNPs of PPP6R3 have already been reported in previous GWAS analyses to have pleiotropic effects on LM and BMD in the pediatric population (17). However, we still know little about the exact mechanism of how it affects muscle development or bone metabolism. Additional research is required to investigate its function in the pleiotropy of ALM and BMD.
By leveraging the power of pleiotropy with BMD, we identified 82 novel potential ALM-associated SNPs. CPED1 is a gene annotated by 11 of them. Variants near CPED1 and WNT16 have been reported to have pleiotropic effects on LM and BMD in the pediatric population (17), and it has been reported that in Duchenne Muscular Dystrophy patients, up-regulation of CPED1 by muscle-specific microRNAs improved skeletal muscle function (40). In addition to CPED1, BMP2 and LRP5 are each annotated by nine novel potential ALM-associated SNPs as found in cFDR analysis. High concentrations of BMP2 protein can influence myogenesis, osteogenesis and adipogenesis in mesenchymal cells (34), and thus participates in muscle development. As for LRP5, it is a co-receptor of Wnt proteins and regulates the process of myogenesis (32). Six novel potential ALM-associated SNPs are located in or close to WNT4 and two are located in or near WNT16. In KEGG canonical pathway analysis, WNT4, WNT16 and LRP5 were found to be enriched in the Wnt signaling pathway. Studies have demonstrated that Wnt ligands participate in the process of myogenesis, with both canonical and noncanonical Wnt pathways involved in the regulation of muscle formation and body tissue homeostasis (15). Four novel potential ALM-associated SNPs are located close to RUNX2 and CLIC5. RUNX2 is an important determinant of osteoblast differentiation, participating in bone formation and regulating bone metabolism (41). Though RUNX2 is expressed on smooth muscle cells and regulates arterial calcification (42), no relationship between RUNX2 and skeletal muscle has as yet been reported.
SNPs close to CLIC5 have been reported to be associated with heel BMD in a previous GWAS (43). In this gene expression validation analysis, levels of mRNA transcripts of CLIC5 were expressed differentially on vastus lateralis muscle in young compared to old adults, indicating that CLIC5 probably participates in the ageing process of skeletal muscle. Given the high number of novel potentially ALM-associated SNPs these genes possess, combined with the evidence presented above, we hypothesize that CPED1, BMP2, LRP5, WNT4, WNT16, PPP6R3, RUNX2 and CLIC5 may participate in ALM development and are potential ALM-associated genes. Additional studies that replicate these findings could help to establish the validity of these observations.
In addition to total body less head BMD, we also applied the cFDR methodology to the same ALM GWAS data and eBMD GWAS data released from the UK Biobank in 2018 (n = 426,824). The UK biobank replicates many previous BMD GWAS findings. But in the first enrichment analysis (QQ plot), no clear pleiotropic enrichment was found between the two traits. The 2018 eBMD data was estimated from quantitative heel ultrasound measurements. Different skeleton sites do not have the same genetic determination. The degree of pleiotropy in ALM may also be different (44).
Although we succeeded in identifying potential pleiotropic variants for ALM and BMD in addition to novel potential variants for ALM, there were some limitations to our study. Firstly, as empirical research, our analysis aimed to provide a greater number of novel potential pleiotropic or trait-specific SNPs/genes for ALM and/or BMD. Variants identified by cFDR and ccFDR still require to be replicated and/or functional validation research conducted to be robustly confirmed. Secondly, we were not able to obtain raw genotype data and so only analyzed the summary statistics of the GWAS. The contribution of our present findings to the proportion of variability in ALM could not be estimated. Thirdly, we were not able to acquire the bone gene expression profiling data of children, so data from postmenopausal women were used for BMD gene expression analysis. This population is older than children and represents a single sex, thus affecting the results slightly. Lastly, the ALM dataset (n = 73,420) is larger than the BMD dataset (n = 10,414), the effect of imbalanced sample size on the cFDR results was not discussed in the original methodology development paper, it is worth further theoretical investigation, but that is not we can do in the present empirical study.
In conclusion, the cFDR approach increased the effective sample size of existing GWAS data and enhanced the detection of potential genetic variants. By leveraging pleiotropy with correlations in BMD phenotype, combined with the use of the cFDR methodology, we identified 26 novel potential pleiotropic SNPs associated with ALM and BMD, with 82 novel potential ALM-associated SNPs. Genes encompassed by these SNPs play an important role in muscle development and/or bone metabolism. Our findings shed novel insight into the genetic variability of ALM in addition to the shared genetic basis underlying ALM and BMD.
Data Availability Statement
Publicly available datasets were analyzed in this study. The data for appendicular lean mass can be found at: http://www.gefos.org/?q=content/adult-lean-mass-gwas-2017, and data for BMD can be found at: http://www.gefos.org/?q=content/data-release-2017.
Author Contributions
H-LL conceived and initiated the development of this study; she is responsible for general development and design of the study and contributed to critical revisions and finalization of the manuscript; she is a guarantor. CP contributed to the acquisition and analysis of the data and drafted the manuscript. H-WD contributed to the study design and critical revisions. K-JS, XL, Y-QS, S-DH, Q-CC, W-XL, and H-HY contributed to data analysis. FL, JS, and C-PZ contributed to the general study design and development. All authors have given approval to the final version of the manuscript. All authors agree to be accountable for the work and ensure that any questions relating to the accuracy and integrity of the paper are investigated and properly resolved.
Funding
H-LL, CP, and FL were supported by Guangzhou Planed Project of Science and Technology, Guangzhou, China [201903010091, 201704020105], and Medical Science and Technology Foundation of Guangdong Province (CN), [A2019005]. H-WD was partially supported by grants from the National Institutes of Health [AR069055, U19 AG055373, R01 MH104680, R01AR059781 and P20GM109036] and the Edward G. Schlieder Endowment Fund to Tulane University. S-DH and JS were supported by China National Natural Scientific Foundation [81800794, 81770878]] and Natural Science Foundation of Guangdong province doctor initiated (vertical collaboration) project [2017A030310390].
Conflict of Interest
The authors declare that the research was conducted in the absence of any commercial or financial relationships that could be construed as a potential conflict of interest.
Acknowledgments
We acknowledge GEFOS-seq Consortium for their GWAS summary statistics posted online.
Supplementary Material
The Supplementary Material for this article can be found online at: https://www.frontiersin.org/articles/10.3389/fimmu.2021.643894/full#supplementary-material
References
1. Studenski SA, Peters KW, Alley DE, Cawthon PM, McLean RR, Harris TB, et al. The FNIH sarcopenia project: rationale, study description, conference recommendations, and final estimates. J Gerontol A Biol Sci Med Sci (2014) 69(5):547–58. doi: 10.1093/gerona/glu010
2. Janssen I, Shepard DS, Katzmarzyk PT, Roubenoff R. The healthcare costs of sarcopenia in the United States. J Am Geriatr Soc (2004) 52(1):80–5. doi: 10.1111/j.1532-5415.2004.52014.x
3. Arden NK, Spector TD. Genetic influences on muscle strength, lean body mass, and bone mineral density: a twin study. J Bone Miner Res (1997) 12(12):2076–81. doi: 10.1359/jbmr.1997.12.12.2076
4. Hsu FC, Lenchik L, Nicklas BJ, Lohman K, Register TC, Mychaleckyj J, et al. Heritability of body composition measured by DXA in the diabetes heart study. Obes Res (2005) 13(2):312–9. doi: 10.1038/oby.2005.42
5. Liu XG, Tan LJ, Lei SF, Liu YJ, Shen H, Wang L, et al. Genome-wide association and replication studies identified TRHR as an important gene for lean body mass. Am J Hum Genet (2009) 84(3):418–23. doi: 10.1016/j.ajhg.2009.02.004
6. Guo YF, Zhang LS, Liu YJ, Hu HG, Li J, Tian Q, et al. Suggestion of GLYAT gene underlying variation of bone size and body lean mass as revealed by a bivariate genome-wide association study. Hum Genet (2013) 132(2):189–99. doi: 10.1007/s00439-012-1236-5
7. Urano T, Shiraki M, Sasaki N, Ouchi Y, Inoue S. Large-scale analysis reveals a functional single-nucleotide polymorphism in the 5’-flanking region of PRDM16 gene associated with lean body mass. Aging Cell (2014) 13(4):739–43. doi: 10.1111/acel.12228
8. Karasik D, Zillikens MC, Hsu YH, Aghdassi A, Akesson K, Amin N, et al. Disentangling the genetics of lean mass. Am J Clin Nutr (2019) 109(2):276–87. doi: 10.1093/ajcn/nqy272
9. Zillikens MC, Demissie S, Hsu YH, Yerges-Armstrong LM, Chou WC, Stolk L, et al. Large meta-analysis of genome-wide association studies identifies five loci for lean body mass. Nat Commun (2017) 8(1):80. doi: 10.1038/s41467-017-00031-7
10. Pei YF, Liu YZ, Yang XL, Zhang H, Feng GJ, Wei XT, et al. The genetic architecture of appendicular lean mass characterized by association analysis in the UK Biobank study. Commun Biol (2020) 3(1):608. doi: 10.1038/s42003-020-01334-0
11. Videman T, Levalahti E, Battie MC, Simonen R, Vanninen E, Kaprio J. Heritability of BMD of femoral neck and lumbar spine: a multivariate twin study of Finnish men. J Bone Miner Res (2007) 22(9):1455–62. doi: 10.1359/jbmr.070606
12. Ralston SH, Uitterlinden AG. Genetics of osteoporosis. Endocr Rev (2010) 31(5):629–62. doi: 10.1210/er.2009-0044
13. Singh H, Kim D, Bemben MG, Bemben DA. Relationship between muscle performance and DXA-derived bone parameters in community-dwelling older adults. J Musculoskelet Neuronal Interact (2017) 17(2):50–8.
14. Kim IJ, Kang KY. Low Skeletal Muscle Mass is Associated with the Risk of Low Bone Mineral Density in Urban Dwelling Premenopausal Women. Calcif Tissue Int (2017) 101(6):581–92. doi: 10.1007/s00223-017-0314-z
15. Girardi F, Le Grand F. Wnt Signaling in Skeletal Muscle Development and Regeneration. Prog Mol Biol Transl Sci (2018) 153:157–79. doi: 10.1016/bs.pmbts.2017.11.026
16. Amjadi-Moheb F, Akhavan-Niaki H. Wnt signaling pathway in osteoporosis: Epigenetic regulation, interaction with other signaling pathways, and therapeutic promises. J Cell Physiol (2019) 234:14641–50. doi: 10.1002/jcp.28207
17. Medina-Gomez C, Kemp JP, Dimou NL, Kreiner E, Chesi A, Zemel BS, et al. Bivariate genome-wide association meta-analysis of pediatric musculoskeletal traits reveals pleiotropic effects at the SREBF1/TOM1L2 locus. Nat Commun (2017) 8(1):121. doi: 10.1038/s41467-017-00108-3
18. Andreassen OA, Thompson WK, Schork AJ, Ripke S, Mattingsdal M, Kelsoe JR, et al. Improved detection of common variants associated with schizophrenia and bipolar disorder using pleiotropy-informed conditional false discovery rate. PloS Genet (2013) 9(4):e1003455. doi: 10.1371/journal.pgen.1003455
19. Greenbaum J, Wu K, Zhang L, Shen H, Zhang J, Deng HW. Increased detection of genetic loci associated with risk predictors of osteoporotic fracture using a pleiotropic cFDR method. Bone (2017) 99:62–8. doi: 10.1016/j.bone.2017.03.052
20. Peng C, Shen J, Lin X, Su KJ, Greenbaum J, Zhu W, et al. Genetic sharing with coronary artery disease identifies potential novel loci for bone mineral density. Bone (2017) 103:70–7. doi: 10.1016/j.bone.2017.06.016
21. Zeng CP, Chen YC, Lin X, Greenbaum J, Chen YP, Peng C, et al. Increased identification of novel variants in type 2 diabetes, birth weight and their pleiotropic loci. J Diabetes (2016) 9(10):898–907. doi: 10.1111/1753-0407.12510
22. Rizzoli R, Bianchi ML, Garabedian M, McKay HA, Moreno LA. Maximizing bone mineral mass gain during growth for the prevention of fractures in the adolescents and the elderly. Bone (2010) 46(2):294–305. doi: 10.1016/j.bone.2009.10.005
23. Benjamini Y, Drai D, Elmer G, Kafkafi N, Golani I. Controlling the false discovery rate in behavior genetics research. Behav Brain Res (2001) 125(1-2):279–84. doi: 10.1016/S0166-4328(01)00297-2
24. Johnson AD, Handsaker RE, Pulit SL, Nizzari MM, O’Donnell CJ, de Bakker PI. SNAP: a web-based tool for identification and annotation of proxy SNPs using HapMap. Bioinformatics (2008) 24(24):2938–9. doi: 10.1093/bioinformatics/btn564
25. Raue U, Trappe TA, Estrem ST, Qian HR, Helvering LM, Smith RC, et al. Transcriptome signature of resistance exercise adaptations: mixed muscle and fiber type specific profiles in young and old adults. J Appl Physiol (1985) (2012) 112(10):1625–36. doi: 10.1152/japplphysiol.00435.2011
26. Reppe S, Refvem H, Gautvik VT, Olstad OK, Hovring PI, Reinholt FP, et al. Eight genes are highly associated with BMD variation in postmenopausal Caucasian women. Bone (2010) 46(3):604–12. doi: 10.1016/j.bone.2009.11.007
27. Wang J, Duncan D, Shi Z, Zhang B. WEB-based GEne SeT AnaLysis Toolkit (WebGestalt): update 2013. Nucleic Acids Res (2013) 41(Web Server issue):W77–83. doi: 10.1093/nar/gkt439
28. Supek F, Bosnjak M, Skunca N, Smuc T. REVIGO summarizes and visualizes long lists of gene ontology terms. PloS One (2011) 6(7):e21800. doi: 10.1371/journal.pone.0021800
29. Morley JE. Hormones and Sarcopenia. Curr Pharm Des (2017) 23(30):4484–92. doi: 10.2174/1381612823666161123150032
30. Khosla S, Oursler MJ, Monroe DG. Estrogen and the skeleton. Trends Endocrinol Metab (2012) 23(11):576–81. doi: 10.1016/j.tem.2012.03.008
31. Schakman O, Kalista S, Barbe C, Loumaye A, Thissen JP. Glucocorticoid-induced skeletal muscle atrophy. Int J Biochem Cell Biol (2013) 45(10):2163–72. doi: 10.1016/j.biocel.2013.05.036
32. Suzuki A, Pelikan RC, Iwata J. WNT/beta-Catenin Signaling Regulates Multiple Steps of Myogenesis by Regulating Step-Specific Targets. Mol Cell Biol (2015) 35(10):1763–76. doi: 10.1128/MCB.01180-14
33. Minelli C, Dean CH, Hind M, Alves AC, Amaral AF, Siroux V, et al. Association of Forced Vital Capacity with the Developmental Gene NCOR2. PloS One (2016) 11(2):e0147388. doi: 10.1371/journal.pone.0147388
34. Rogers MB, Shah TA, Shaikh NN. Turning Bone Morphogenetic Protein 2 (BMP2) on and off in Mesenchymal Cells. J Cell Biochem (2015) 116(10):2127–38. doi: 10.1002/jcb.25164
35. Rivadeneira F, Styrkarsdottir U, Estrada K, Halldorsson BV, Hsu YH, Richards JB, et al. Twenty bone-mineral-density loci identified by large-scale meta-analysis of genome-wide association studies. Nat Genet (2009) 41(11):1199–206. doi: 10.1038/ng.446
36. Charette JR, Earp SE, Bell BA, Ackert-Bicknell CL, Godfrey DA, Rao S, et al. A mutagenesis-derived Lrp5 mouse mutant with abnormal retinal vasculature and low bone mineral density. Mol Vis (2017) 23:140–8.
37. Levasseur R, Lacombe D, de Vernejoul MC. LRP5 mutations in osteoporosis-pseudoglioma syndrome and high-bone-mass disorders. Joint Bone Spine (2005) 72(3):207–14. doi: 10.1016/j.jbspin.2004.10.008
38. Twells RC, Metzker ML, Brown SD, Cox R, Garey C, Hammond H, et al. The sequence and gene characterization of a 400-kb candidate region for IDDM4 on chromosome 11q13. Genomics (2001) 72(3):231–42. doi: 10.1006/geno.2000.6492
39. Ziembik MA, Bender TP, Larner JM, Brautigan DL. Functions of protein phosphatase-6 in NF-kappaB signaling and in lymphocytes. Biochem Soc Trans (2017) 45(3):693–701. doi: 10.1042/BST20160169
40. Liu DZ, Stamova B, Hu S, Ander BP, Jickling GC, Zhan X, et al. MicroRNA and mRNA Expression Changes in Steroid Naive and Steroid Treated DMD Patients. J Neuromuscul Dis (2015) 2(4):387–96. doi: 10.3233/JND-150076
41. Wei J, Shimazu J, Makinistoglu MP, Maurizi A, Kajimura D, Zong H, et al. Glucose Uptake and Runx2 Synergize to Orchestrate Osteoblast Differentiation and Bone Formation. Cell (2015) 161(7):1576–91. doi: 10.1016/j.cell.2015.05.029
42. Kawane T, Qin X, Jiang Q, Miyazaki T, Komori H, Yoshida CA, et al. Runx2 is required for the proliferation of osteoblast progenitors and induces proliferation by regulating Fgfr2 and Fgfr3. Sci Rep (2018) 8(1):13551. doi: 10.1038/s41598-018-31853-0
43. Kim SK. Identification of 613 new loci associated with heel bone mineral density and a polygenic risk score for bone mineral density, osteoporosis and fracture. PloS One (2018) 13(7):e0200785. doi: 10.1371/journal.pone.0200785
Keywords: appendicular lean mass, bone mineral density, pleiotropy, genome wide association study, novel SNPs
Citation: Peng C, Liu F, Su KJ, Lin X, Song YQ, Shen J, Hu SD, Chen QC, Yuan HH, Li WX, Zeng CP, Deng HW and Lou HL (2021) Enhanced Identification of Novel Potential Variants for Appendicular Lean Mass by Leveraging Pleiotropy With Bone Mineral Density. Front. Immunol. 12:643894. doi: 10.3389/fimmu.2021.643894
Received: 19 December 2020; Accepted: 09 March 2021;
Published: 06 April 2021.
Edited by:
Sean X. Leng, Johns Hopkins University, United StatesReviewed by:
Lei Zhang, Soochow University, ChinaXiang Qiu, National Institutes of Health (NIH), United States
Copyright © 2021 Peng, Liu, Su, Lin, Song, Shen, Hu, Chen, Yuan, Li, Zeng, Deng and Lou. This is an open-access article distributed under the terms of the Creative Commons Attribution License (CC BY). The use, distribution or reproduction in other forums is permitted, provided the original author(s) and the copyright owner(s) are credited and that the original publication in this journal is cited, in accordance with accepted academic practice. No use, distribution or reproduction is permitted which does not comply with these terms.
*Correspondence: Hui-Ling Lou, aHVpbGluZ2xvdUAxNjMuY29t