- 1State Key Laboratory of Veterinary Etiological Biology, Key Laboratory of Veterinary Parasitology of Gansu Province, Lanzhou Veterinary Research Institute, Chinese Academy of Agricultural Sciences, Lanzhou, China
- 2College of Veterinary Medicine, Northwest A&F University, Yangling, China
- 3School of Science, Fudan University, Shanghai, China
- 4College of Veterinary Medicine, Shanxi Agricultural University, Taigu, China
- 5Faculty of Medicine and Health Sciences, School of Veterinary Medicine and Science, University of Nottingham, Loughborough, United Kingdom
- 6Key Laboratory of Veterinary Public Health of Higher Education of Yunnan Province, College of Veterinary Medicine, Yunnan Agricultural University, Kunming, China
Fasciola gigantica produces excretory-secretory products (ESPs) with immune-modulating effects to promote its own survival. In this study, we performed RNA-seq to gain a comprehensive global understanding of changes in the expression of mRNAs, miRNAs, lncRNAs, and circRNAs in goat peripheral blood mononuclear cells (PBMCs) treated with F. gigantica ESPs. A total of 1,544 differently expressed mRNAs (790 upregulated and 754 downregulated genes), 30 differently expressed miRNAs (24 upregulated and 6 downregulated genes), 136 differently expressed circRNAs (83 upregulated and 53 downregulated genes), and 1,194 differently expressed lncRNAs (215 upregulated and 979 downregulated genes) were identified. Gene Ontology (GO) and Kyoto Encyclopedia of Genes and Genomes (KEGG) enrichment analyses revealed that F. gigantica ESPs altered the expression of genes associated with the host immune response, receptor signaling, disease and metabolism. Results from RNA-seq were validated by qRT-PCR. These findings provide an important resource for future investigation of the role of mRNAs and non-coding RNAs in mediating the immune-modulating effects of F. gigantica ESPs.
Introduction
Fasciolosis, caused by Fasciola gigantica and F. hepatica, is a significant parasitic disease, causing huge economic losses to livestock and serious adverse impact on public health (1–3). Fasciolosis is prevalent in developing countries, particularly in Asia, Africa, Latin America, and the Caribbean (2, 4, 5). The annual global economic losses to livestock production caused by liver fluke infection exceed $3 billion (6). Despite the significant impact of fasciolosis, our knowledge of host defense mechanisms against F. gigantica infection remains limited. Exploring the molecular mechanisms of interaction between the host and liver flukes would enhance our understanding of the host immune response and assist in identifying potential targets for the development of therapeutic interventions against fasciolosis.
Liver flukes employ a raft of strategies to subvert the host’s immune response, including the secretion of excretory-secretory products (ESPs) to modulate the host’s immune responses, to facilitate their own survival and to establish long-term infection (7, 8). In a previous study, by using co-immunoprecipitation coupled with tandem mass spectrometry, we identified 14, 16, 9, and 9 proteins in F. hepatica ESPs (FhESPs) that can bind to cytokines IL2, IL17, IFN-γ, and goat peripheral blood mononuclear cells (PBMCs) (9). In addition, a proteomic analysis of FgESPs interacting with buffalo serum at different infection periods (42, 70, and 98 dpi) identified 18 proteins after F. gigantica infection, including 13 cathepsin proteins, 4 glutathione S-transferase proteins, and 1 calcium-binding protein (10).
To identify the role of these proteins in the long-term survival of F. gigantica, we cloned and expressed the recombinant F. gigantica cathepsin B (rFgCatB), 14-3-3 epsilon (rFg14-3-3e), Ras-related Rab10 (rFgRab10) and thioredoxin peroxidase (rFgTpx) proteins, and characterized their effects on various functions of the goat PBMCs (11–14). In the related liver fluke F. hepatica, tegumental antigen affects the maturation and antigen presentation to host dendritic cells (DCs) (15). Furthermore, F. hepatica fatty acid-binding protein inhibits the activation of Toll-like Receptor (TLR) 4 and suppresses the expression of inflammatory cytokines induced by lipopolysaccharide (LPS) (16).
Although ESPs play a significant role in supporting liver flukes’ evasion of the host immune response, the molecular mechanisms that are mediated by ESPs remain poorly defined. To reveal new aspects of the immuno-modulatory effects of F. gigantica ESPs, we investigated the expression profiles of messenger RNAs (mRNAs), microRNAs (miRNAs), circular RNAs (circRNAs), and long non-coding RNA (lncRNAs) in goat PBMCs following incubation with ESPs of F. gigantica. The results showed that ESPs can regulate the proliferation, immune response, and metabolism of treated PBMCs. Our data provide new information for further in-depth analysis of the role of ESPs in the immune-evasive strategy of F. gigantica.
Materials and Methods
Source and Culture Conditions of the PBMCs
Goat PBMCs were isolated as previously described (11). Briefly, healthy goat venous blood samples mixed with ethylene diamine tetraacetic acid (EDTA) were collected into vacutainer tubes. Goat PBMCs were isolated using a commercial kit (TBD, Tianjin, China) according to the manufacturer’s instructions. The isolated PBMCs were cultured in RPMI 1640 medium containing 10% fetal bovine serum (FBS) and 1% penicillin-streptomycin (Gibco, California, USA) and were incubated in a humidified atmosphere of 5% CO2 at 37°C for 4 h.
Preparation of ESPs
ESPs of F. gigantica were prepared according to a previously described method (15, 17–19). Briefly, adult liver flukes of F. gigantica were obtained from the bile ducts of slaughtered buffaloes naturally infected by F. gigantica and washed three times with phosphate-buffered saline (PBS) at 37°C. The washed adult flukes were then transferred to PBSG buffer (PBS containing glucose, supplemented with 10,000 UI/ml penicillin G, 10 mg/ml streptomycin sulfate, and 25 μg/ml amphotericin B) and incubated in plastic petri dishes (1 worm/2 mL) for 2 h at 37°C. The supernatant was collected three times (i.e., every 2 h over a 6-h period). After incubation, the supernatant was collected and centrifuged at 10,000g for 30 min at 4°C, then filter-sterilized with a 20-μm nylon filter. After filter-sterilization, ESPs were concentrated using Amicon® Ultra-15 centrifugal filter devices (30 K), and the concentration of ESPs was measured by the Bicinchoninic Acid Protein Assay (BCA-1, Sigma-Aldrich, Corp., St. Louis, MO, USA) and stored at −80°C until analysis.
RNA Extraction and RNA-seq
PBMCs (1 × 106) were incubated with 80 µg of F. gigantica ESPs or an equal volume of PBS (controls) at 37°C with 5% CO2 for 24 h. This experiment was performed in triplicate. Total RNA of goat PBMCs was extracted using the Ribo-Zero Kit (Ambion, Austin, Tex, USA) following the manufacturer’s protocol. RNA integrity was evaluated using the Agilent 2100 Bioanalyzer (Agilent Technologies, Santa Clara, CA, USA). Samples with a RNA Integrity Number (RIN) ≥ 7 were included in the analysis. The sequencing libraries were constructed using TruSeq Stranded Total RNA with Ribo-Zero Globin, according to the manufacturer’s instructions. These libraries were sequenced on an Illumina sequencing platform (HiSeq 2500).
Data Processing and Differential Expression Analysis
Raw reads generated from high-throughput sequencing were fastq format sequences. In order to obtain high-quality reads, trimmomatic software was used to remove low-quality bases, N-bases, and low-quality reads (20). HISTA2 was used to align clean reads to the goat reference genome (21). Also, Q20, Q30, and GC-content of the raw data were calculated (22). The “estimateSizeFactors” function of the DESeq R package was used to normalize the read counts (23), and the “nbinomTest” function was used to calculate P values and fold change values. The transcript with P-values < 0.05 and |log2 Fold change| > 1 was deemed to be a differentially expressed (DE) transcript.
miRNA Prediction
The small RNA tags were mapped to a reference sequence by Bowtie (24) to analyze their expression and distribution on the reference genome. The data of miRBase20.0 were used as miRNA reference, and modified software miRDeep2 (25) was used to identify the known miRNAs. The software miREvo (26) and miRDeep2 (25) were combined to predict novel miRNA by exploring the secondary structure, the Dicer cleavage site and the minimum free energy of the small RNA tags unannotated in the former steps.
CircRNA Prediction
In order to generate a SAM file, BWA software was used to align the sequencing reads of each sample to the reference genome (27). Then, the CIRI software was used to scan paired chiastic clipping (PCC) signals, and circRNA sequences were predicted based on junction reads and GT-AG cleavage signals (28).
lncRNA Prediction
Stringtie software was used to assemble the known and new transcripts (29). The candidate lncRNA transcripts were screened by comparing with the gene annotation information of the reference sequence produced by Cuffcompare software (30). The transcripts with coding potential were then screened out using CPC (31), CNCI, Pfam (32), and PLEK (27) in order to obtain lncRNA predicted sequences.
GO and KEGG Enrichment Analysis
Gene Ontology (GO) enrichment was used for functional annotation analysis. GOseq based Wallenius non-central hyper-geometric distribution (33), which adjusts for gene length bias, was implemented for GO enrichment analysis. The Kyoto Encyclopedia of Genes and Genomes (KEGG) (34) database (http://www.genome.jp/kegg/) was utilized to test the statistical enrichment of the target genes in KEGG pathways using KOBAS software (35).
Quantitative Real-Time PCR (qRT-PCR)
qRT-PCR was performed to validate the transcriptome data using the SYBR assay according to the manufacturer’s instructions. The qRT-PCR reactions were carried out using TB Green® Premix Ex Taq™ II (Tli RNaseH Plus) (Takara, Shuzo, Kyoto, Japan) with the following reaction conditions: 95°C for 30 s, followed by 40 cycles at 95°C for 5 s and 60°C for 30 s. The GADPH gene was used for mRNA, circRNA, and lncRNA normalization, while the U6 gene was used for miRNA’s normalization. Three independent replicates were examined for each gene and the relative expression of the target mRNAs, mircRNAs, circRNAs, and lncRNAs was calculated using the 2−ΔΔCT method. All primers used in this study are listed in Table 1.
Cell Proliferation Assay by CCK-8 Kit
The effect of F. gigantica ESPs on goat PBMC’s proliferation was investigated using the CCK-8 kit (Beyotime, Haimen, Jiangsu, China) as previously described (13). Briefly, goat PBMCs were incubated with F. gigantica ESPs at concentrations of 5, 10, 20, 40, and 80 µg/ml. Forty-eight hours later, 10 µl of CCK-8 reagent were added to each cell culture and incubated for 4 h. The OD450 of each well was measured using a microplate reader (Bio-Rad, California, USA).
Statistical Analysis
Student’s t-test was used to analyze the data from qRT-PCR experiments using GraphPad Prism 5 software (GraphPad Software, Inc., San Diego, CA, USA). All data are presented as means ± standard deviations (SDs).
Results
ESPs of F. gigantica Promoted the Proliferation of PBMCs
F. gigantica ESPs promoted the proliferation of goat PBMCs in a concentration-dependent manner (Figure 1). Significant differences were detected between cells treated with 20 (p < 0.1), 40 (p < 0.0001) and 80 (p < 0.0001) µg/ml ESPs, when compared with untreated (control) cells. However, no significant differences were detected between cells exposed to lower concentrations (5 or 10 µg/ml) and control cells.
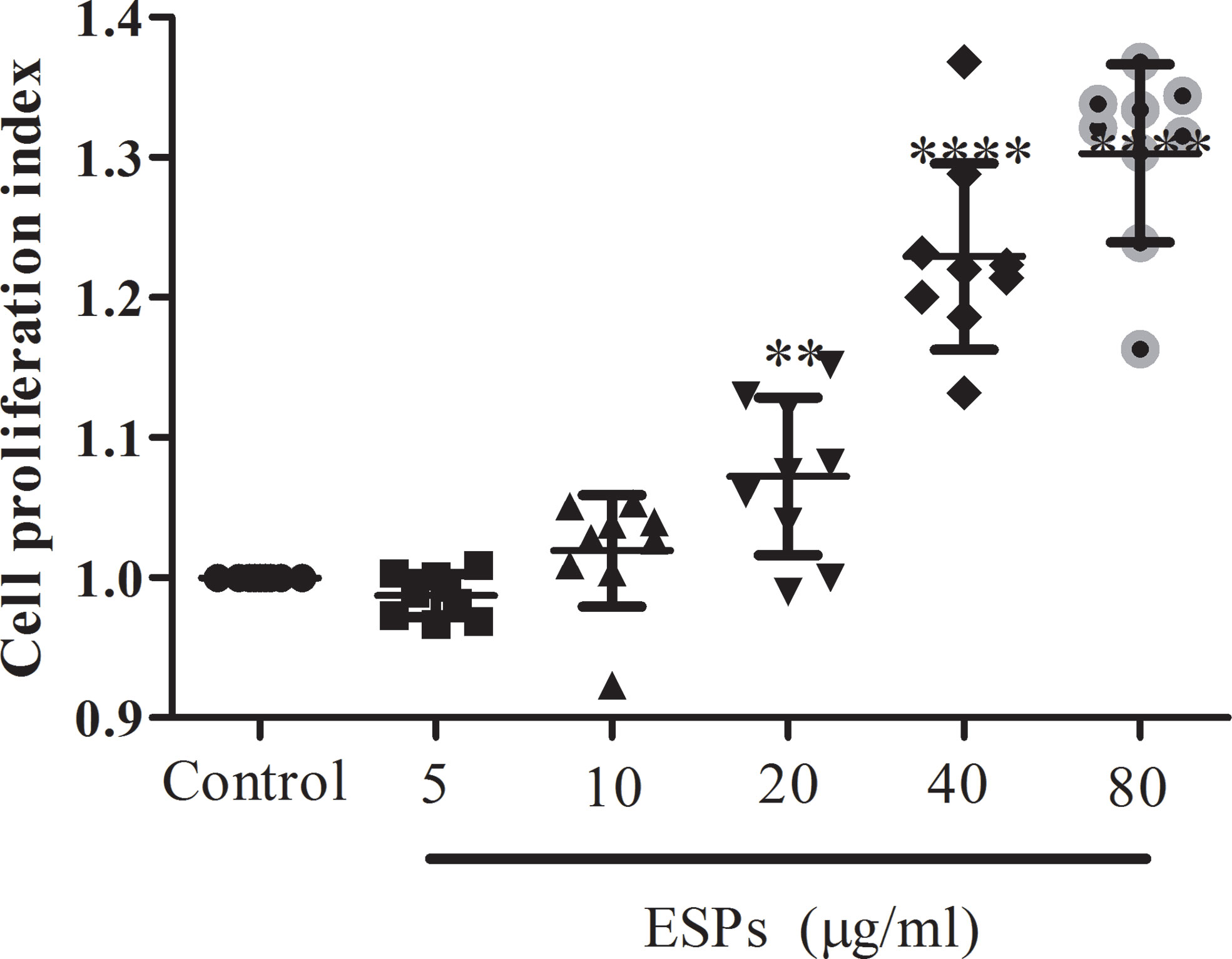
Figure 1 The ESPs of F. gigantica promote goat PBMC’s proliferation in a dose-dependent manner. PBMCs were treated with F. gigantica ESPs at the indicated range of concentrations for 48 h. Cell proliferation was quantified as described in the methods (n = 3). The error bars represent standard deviations. ** p < 0.01; **** p < 0.0001 compared to control untreated PBMCs.
DE mRNAs, miRNAs, circRNAs, and lncRNAs
The volcano plots (Figure 2) and heat maps (Figure 3) clearly showed the number and hierarchical clustering of differentiated mRNAs (Figures 2A and 3A), miRNAs (Figures 2B and 3B), circRNAs (Figures 2C and 3C), and lncRNAs (Figures 2D and 3D) in ESP-treated and control (untreated) groups. Our analysis identified 1,544 differently expressed mRNAs (including 790 upregulated and 754 downregulated, Figure 2A and Supplementary Table S1), 30 differently expressed miRNAs (including 24 upregulated and 6 downregulated, Figure 2B and Supplementary Table S2), 136 differently expressed circRNAs (including 83 upregulated and 53 downregulated, Figure 2C and Supplementary Table S3), and 1,194 differently expressed lncRNAs (including 215 upregulated and 979 downregulated, Figure 2D and Supplementary Table S4).
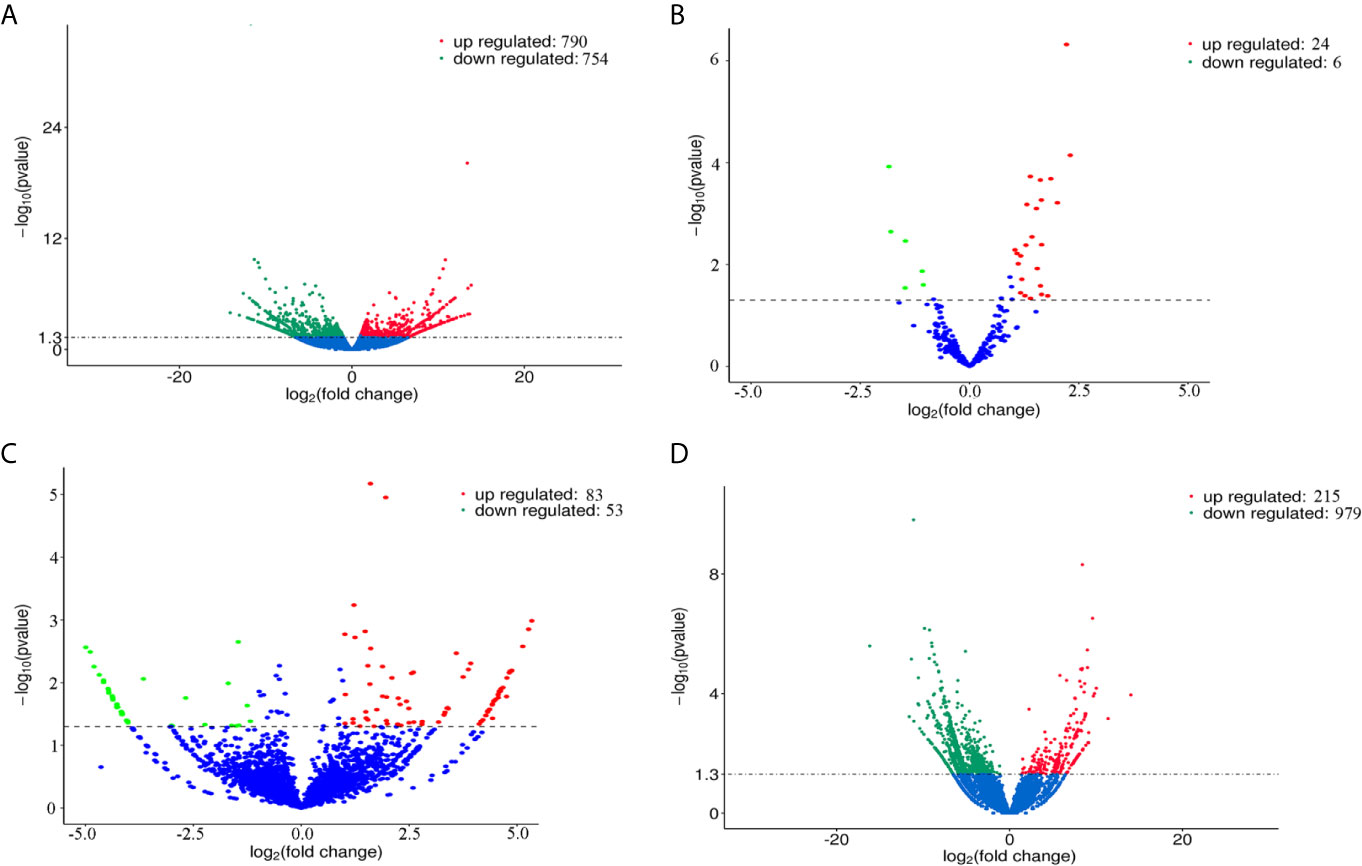
Figure 2 Volcano plots showing the differentially expressed mRNAs (A), miRNAs (B), circRNAs (C), and lncRNAs (D) detected in goat PBMCs after treatment with F. gigantica ESPs. The negative log10 transformed P values (y-axis) are plotted against the average log2 fold changes in expression (x-axis). Data points representing RNAs that were not identified as differentially expressed are shown in blue. Transcripts that are differentially expressed after treatment with ESPs (corrected P value < 0.05) with an absolute log2 fold change (|FC|) greater than or less than 1 are shown as red (upregulated) and green (downregulated) dots.
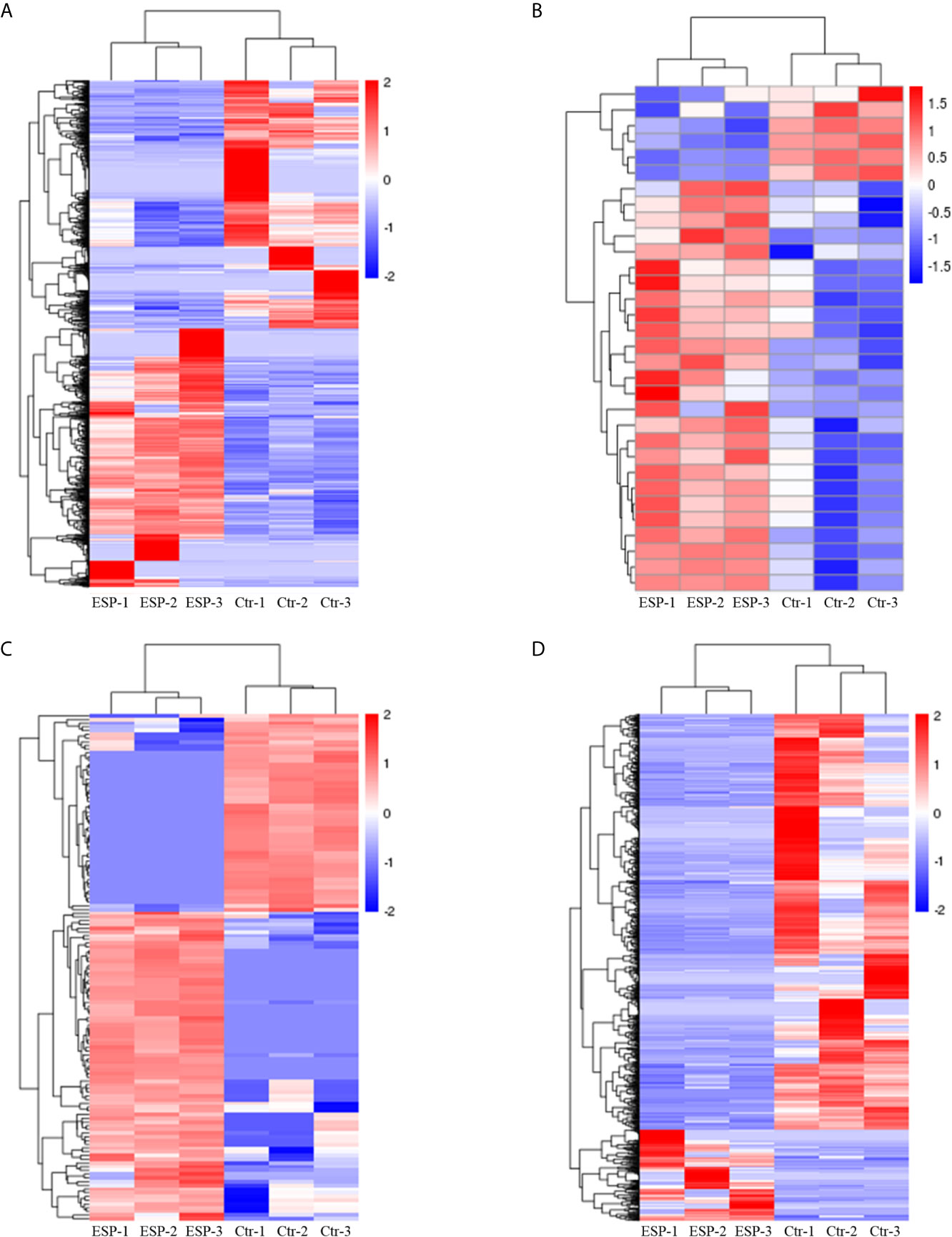
Figure 3 Hierarchical clustering heatmaps of the differentially expressed genes mRNAs (A), miRNAs (B), circRNAs (C), and lncRNAs (D). Each column represents the value for one separate experiment of the control and ESP-treated goat PBMCs. Each raw represents the individual RNAs. The colors represent the level of expression of the RNAs in the sample with red and blue denoting higher and lower expression levels, respectively.
Validation of DE mRNAs, miRNAs, circRNAs, and lncRNAs by qRT-PCR
To validate the transcriptome data, a total of 12 genes were randomly selected for qRT-PCR verification, including three mRNAs (HDAC1, MCM2, CD47) (Figure 4A), three miRNAs [16a-3p (chi-miR-16a-3p), 211 (chi-miR-211), 155-3p (chi-miR-155-3p)] (Figure 4B), three circRNAs (7223 (novel_circ_0017223), 7701 (novel_circ_0007701), 3459 (novel_circ_0003459) (Figure 4C), and three lncRNAs [5943 (ENSCHIT00000005943), 2500 (LNC_012500), 2081 (ENSCHIT00000002081)] (Figure 4D). The qRT-PCR analysis showed that the trend of the data of qRT-PCR expression analysis was consistent with that of the data obtained by transcriptome analysis, supporting the accuracy of the transcriptomic data.
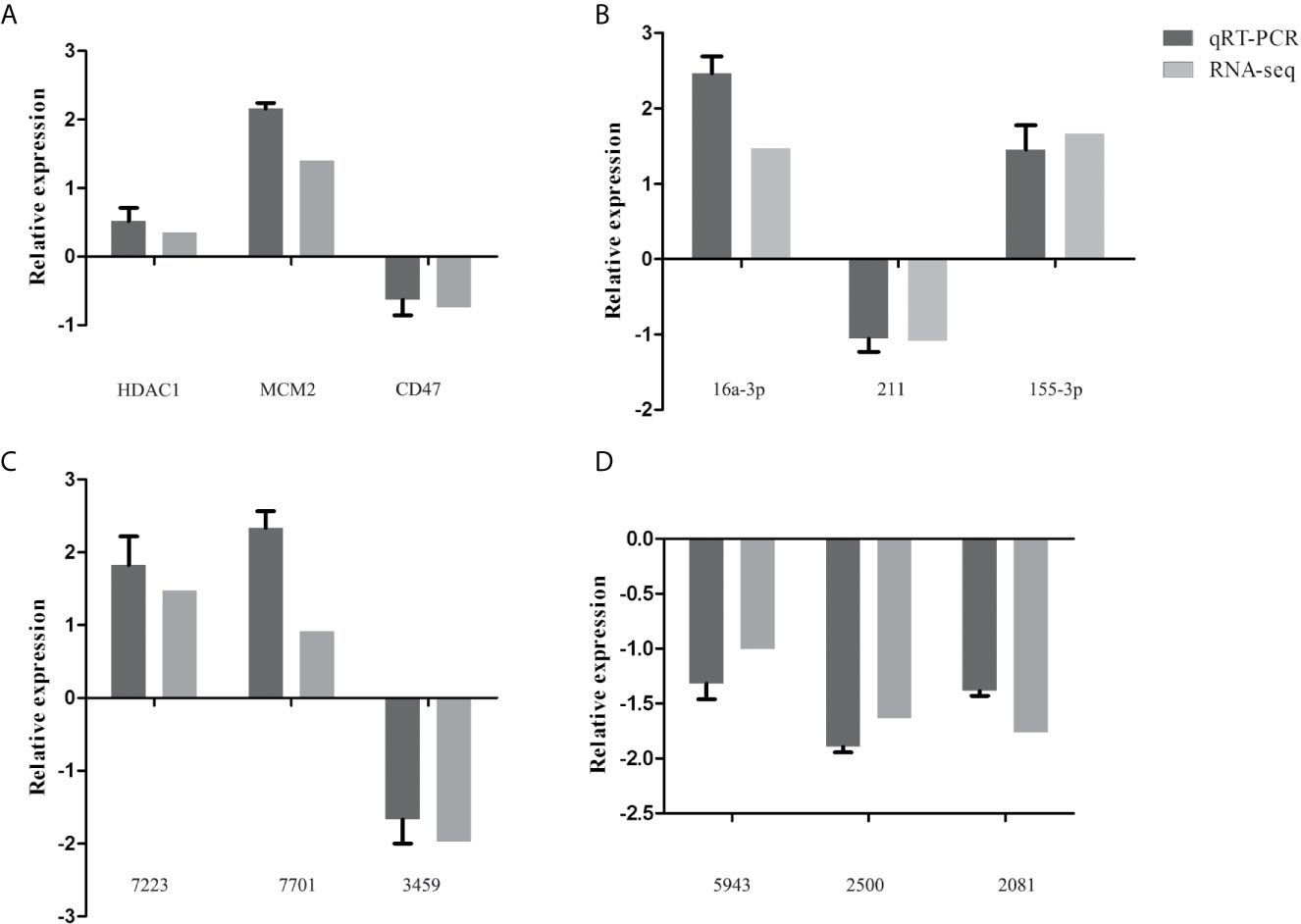
Figure 4 Validation of the expression of representative differentially expressed mRNAs (HDAC1, MCM2, CD47) (A), miRNAs [16a-3p (chi-miR-16a-3p), 211 (chi-miR-211), 155-3p (chi-miR-155-3p)] (B), circRNAs [7223 (novel_circ_0017223), 7701 (novel_circ_0007701), 3459 (novel_circ_0003459)] (C), and lncRNAs [5943 (ENSCHIT00000005943), 2500 (LNC_012500), 2081 (ENSCHIT00000002081)] (D) using qRT-PCR. The x-axis shows the names of the selected RNAs and the y-axis shows the relative expression levels. The GADPH gene was used for mRNA, circRNAs, and lncRNA normalization, while the U6 gene was used for miRNA’s normalization.
GO and KEGG Analysis of the DE mRNAs
To categorize and annotate the biological functions of the DE transcripts, GO enrichment analysis was performed. The GO terms were classed into three categories including biological process, cellular component and molecular function. The top 30 GO terms are shown in Figure 5. The DE mRNAs were significantly enriched in cellular component including the intracellular organelle part, nucleus and nuclear part (Figure 5A). Among these, the upregulated mRNAs were also significantly enriched in cellular component including the intracellular, intracellular part and organelles (Supplementary Figure S1A), and the downregulated mRNAs were significantly enriched in biological processes, such as the immune system process, regulation of localization, and regulation of the immune system process (Supplementary Figure S1B). The KEGG pathway enrichment was used to identify the significantly enriched pathways of the DE mRNAs. The top 20 pathways are shown in Figure 5B. Systemic lupus erythematosus, alcoholism, DNA replication and cell cycle were the most enriched pathways. Among them, the upregulated mRNAs were also significantly enriched in Systemic lupus erythematosus, alcoholism, DNA replication and cell cycle (Supplementary Figure S1C). The downregulated mRNAs were significantly enriched in Staphylococcus aureus infection, lysosome, complement and coagulation cascades, and phagosome (Supplementary Figure S1D).
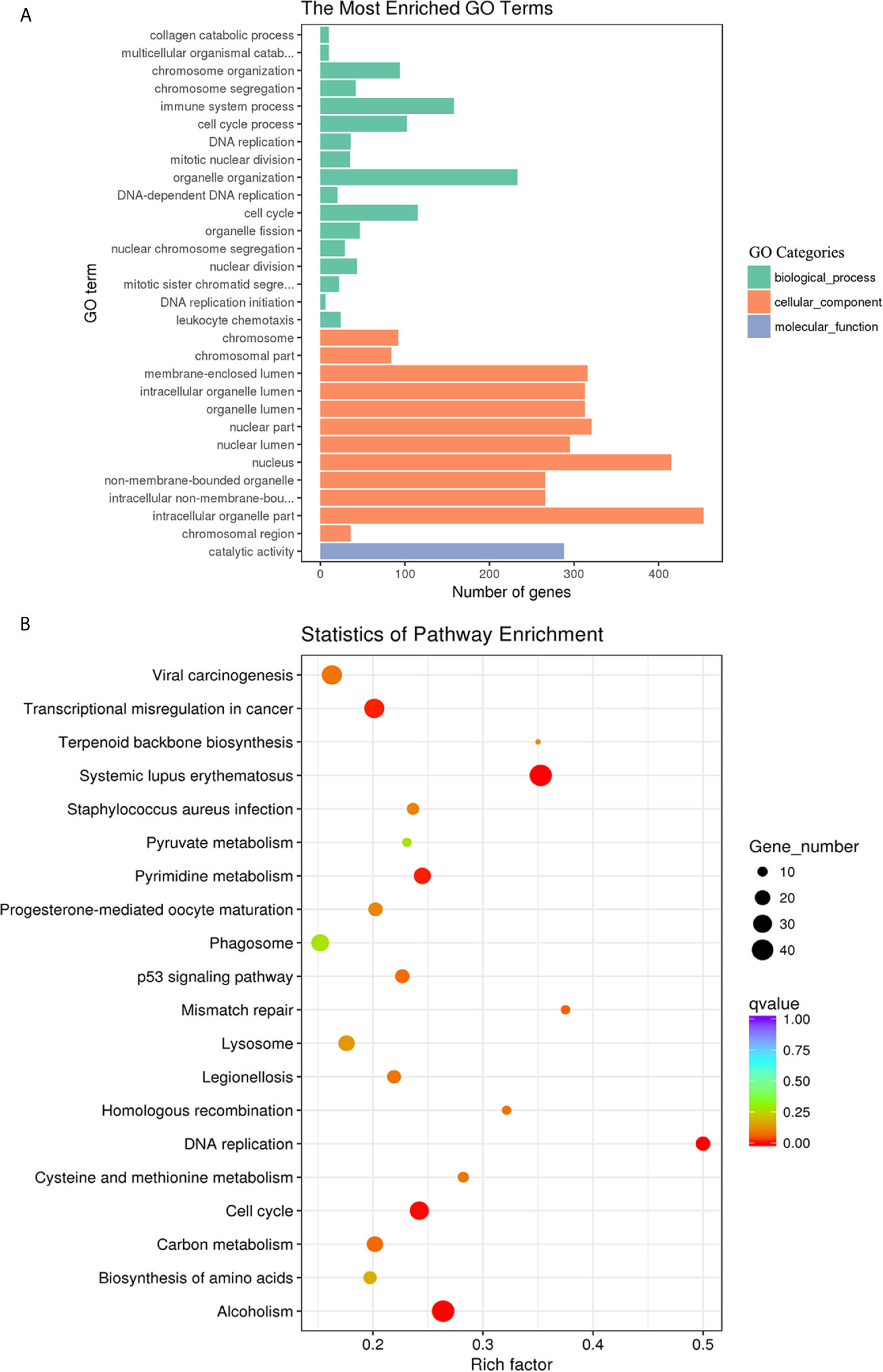
Figure 5 GO enrichment and KEGG pathway analyses of the differentially expressed mRNAs in goat PBMCs. (A) The 30 most enriched GO terms in the biological process, cellular component, and molecular function categories. (B) Scatterplot of KEGG pathway analysis of the top 20 pathways. The x-axis represents the pathway enrichment. The y-axis denotes the names of the significantly enriched pathways. The P-values are indicated by variations from blue to red. A deeper blue color indicates a greater significant difference.
GO and KEGG Analysis of the Target Genes of miRNAs
GO and KEGG pathway analysis was used to probe the main functions and the involved pathways of the DE miRNAs. As shown in Figure 6, the DE miRNAs were significantly enriched in biological processes, such as cell differentiation, cellular developmental process, and regulation of nervous system development. The most enriched pathway of the DE miRNA was the TGF-beta signaling pathway.
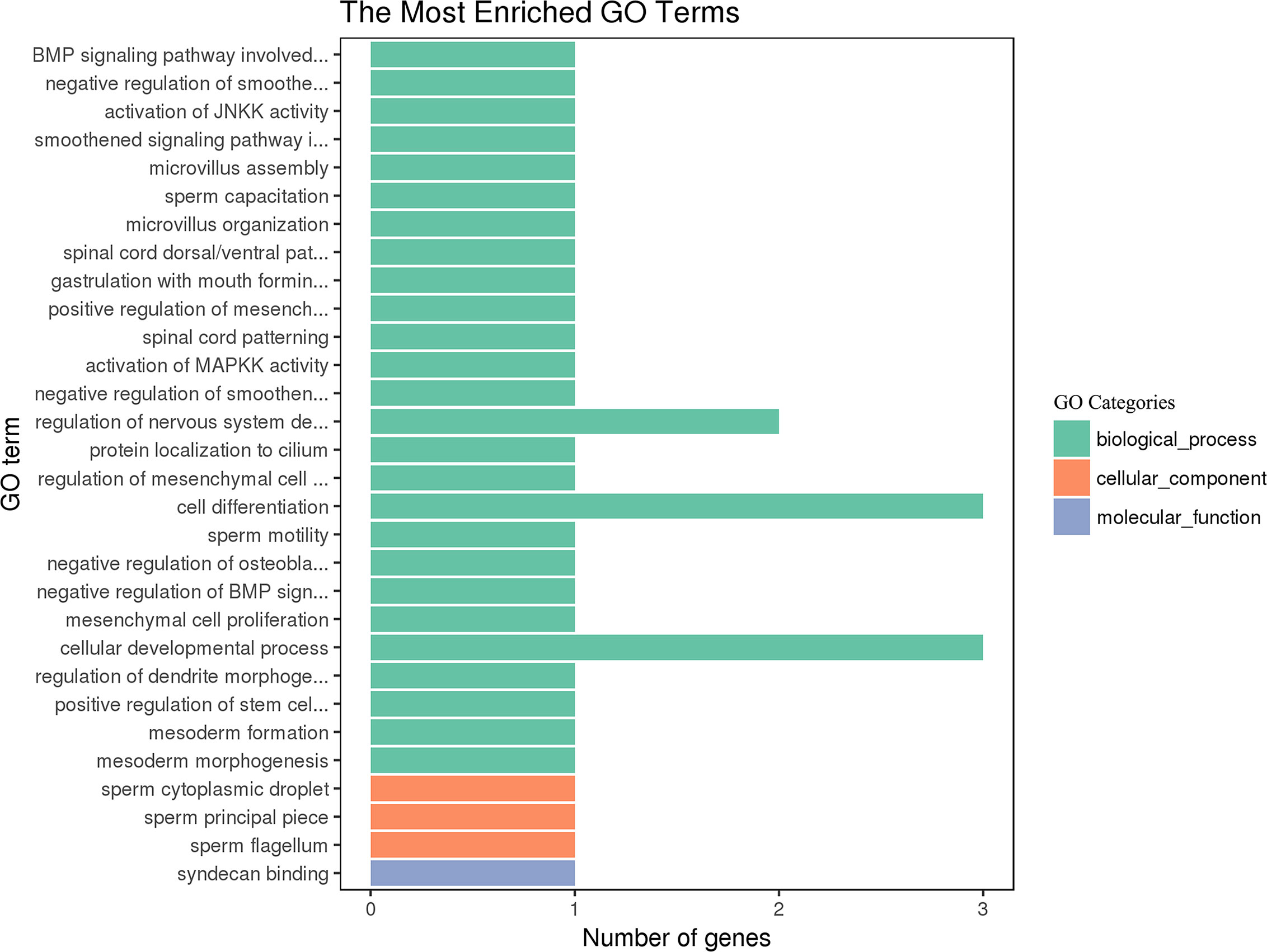
Figure 6 GO enrichment of the differentially expressed miRNAs in goat PBMCs. The 30 most enriched GO terms in the biological process, cellular component and molecular function categories. The y-axis represents the significantly enriched GO terms and the x-axis shows the number of genes in each GO term.
GO and KEGG Analysis of the Target Genes of circRNAs
The DE circRNAs were significantly enriched in biological processes, such as positive regulation of cellular process, positive regulation of biological process, and regulation of cellular metabolic process (Figure 7A). The most enriched pathways of the DE circRNAs involved included lysine degradation, the TNF signaling pathway, the B cell receptor signaling pathway, and hepatitis C (Figure 7B).
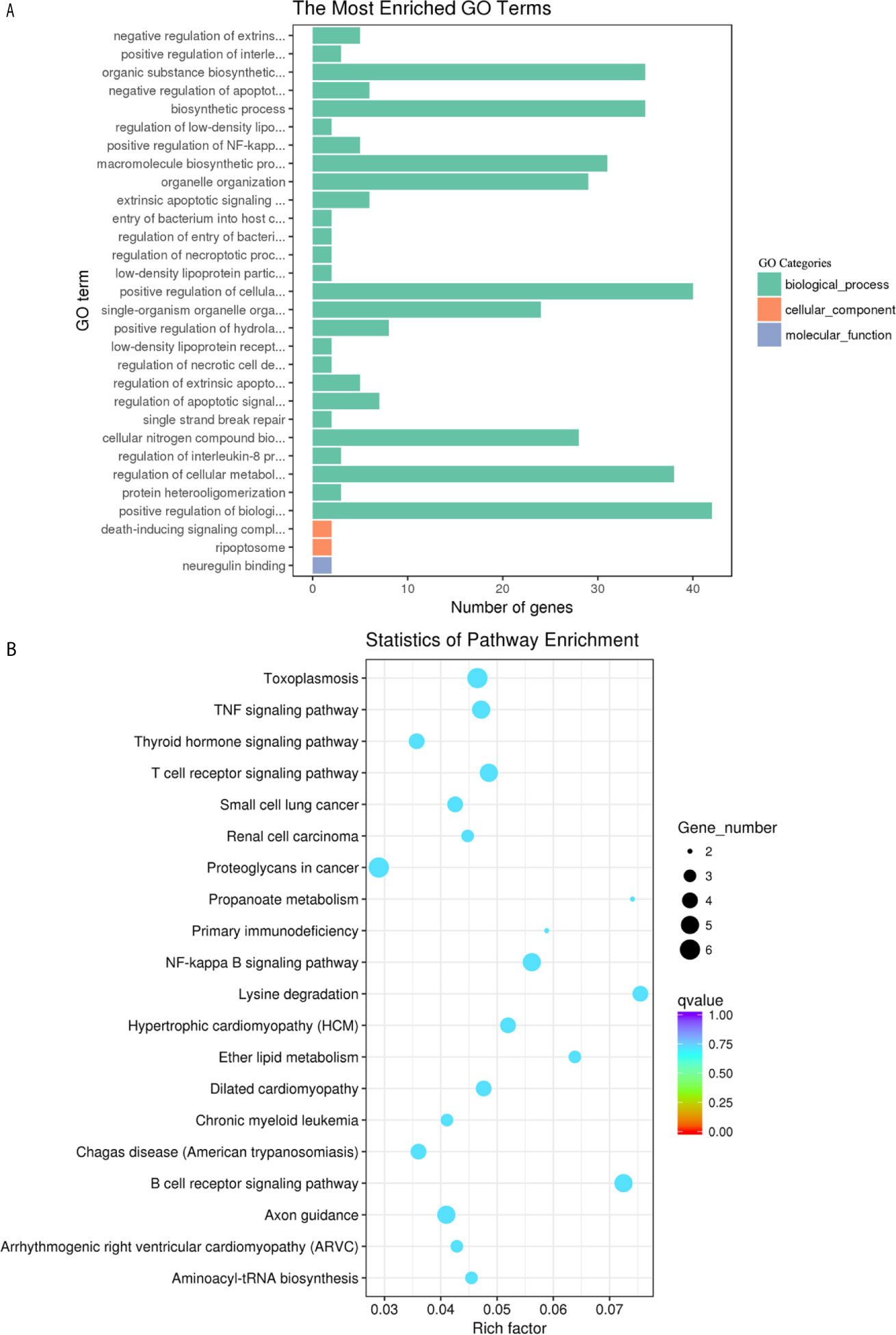
Figure 7 GO enrichment and KEGG pathway analyses of target genes of the differentially expressed circRNAs in goat PBMCs. (A) The 30 most enriched GO terms in the biological process, cellular component and molecular function categories of target genes of differentially expressed circRNAs. (B) Scatterplot of KEGG pathway analysis of the top 20 predominant pathways. The x-axis denotes the pathway enrichment. The y-axis represents the names of the significantly enriched pathways. The P-values are indicated by variations from blue to red. A deeper blue color indicates a greater significant difference.
GO and KEGG Analysis of the Target Genes of lncRNAs
The biological functions of the DE lncRNAs were mainly involved in biological processes, such as negative regulation of biological process, organelle organization and macromolecular complex subunit organization (Figure 8A). Among them, the upregulated lncRNAs were also significantly enriched in biological process and cellular component, such as macromolecular complex subunit organization, chromosome and chromosome organization (Supplementary Figure S2A). The downregulated lncRNAs were significantly enriched in the categories biological process and molecular function, such as enzyme binding, kinase binding and single-organism cellular localization (Supplementary Figure S2B). The DE lncRNAs were significantly enriched in Type I diabetes mellitus, Influenza A and Allograft rejection (Figure 8B). Among them, the upregulated DE lncRNA were also significantly enriched in Influenza A, Type I diabetes mellitus and Graft-versus-host disease (Supplementary Figure S2C). The downregulated DE lncRNAs were significantly enriched in measles, rheumatoid arthritis, influenza A, and the chemokine signaling pathway (Supplementary Figure S2D).
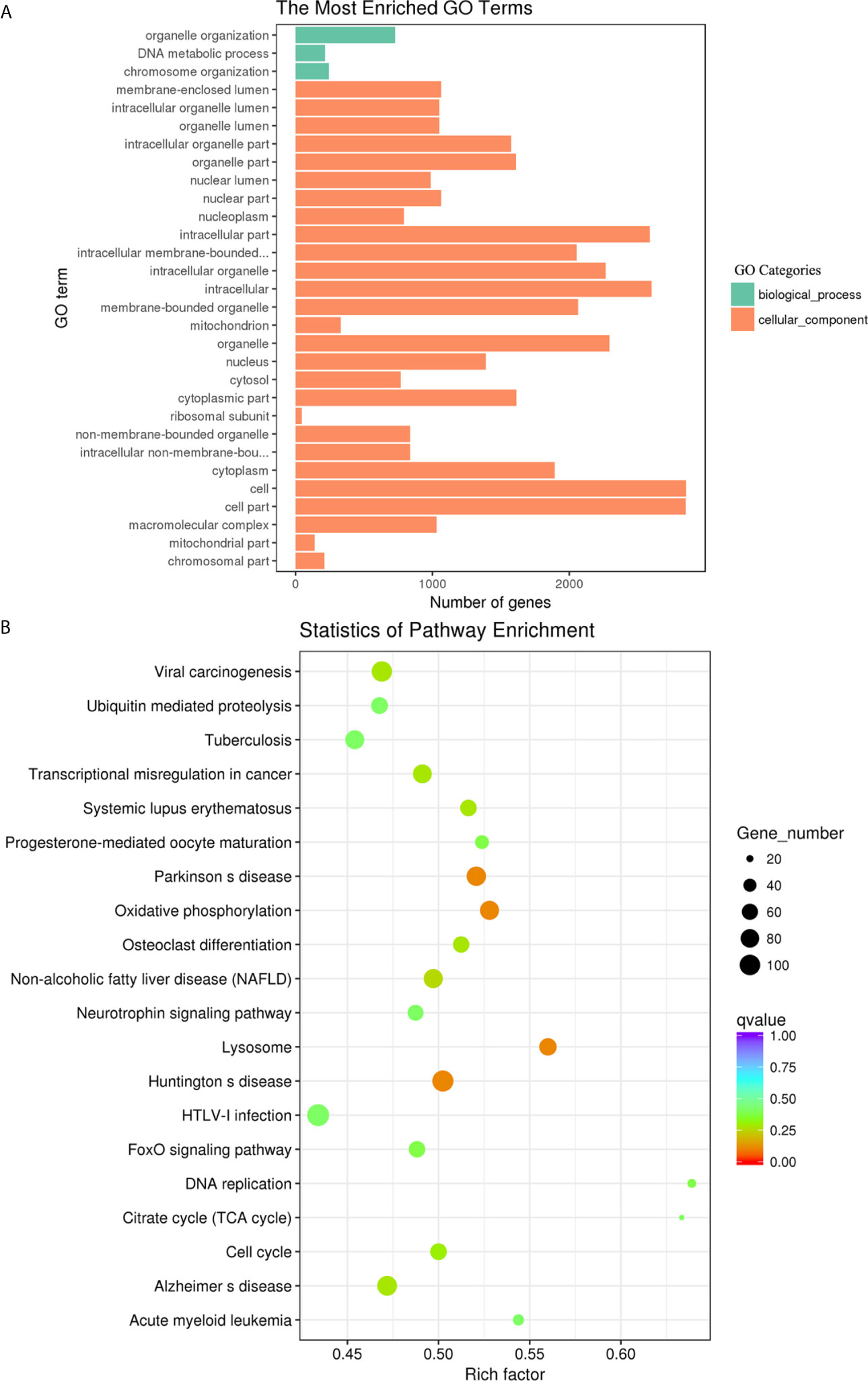
Figure 8 GO enrichment and KEGG pathway analyses of target genes of the differentially expressed lncRNAs in goat PBMCs. (A) The 30 most enriched GO terms in the biological process, cellular component and molecular function categories of target genes of differentially expressed lncRNAs. (B) Scatterplot of KEGG pathway analysis of the top 20 predominant pathways. The x-axis refers to pathway enrichment. The y-axis represents the names of the significantly enriched pathways. The P-values are expressed by variations from blue to red. A deeper blue color indicates a greater significant difference.
Discussion
In this study, we performed global RNA-seq to profile the expression of mRNAs, miRNAs, lncRNAs, and circRNAs in goat PBMCs exposed to F. gigantica ESPs. GO and KEGG enrichment analysis revealed that F. gigantica ESPs can influence the expression of transcripts associated with the host immune response, receptor signaling, cell proliferation, disease and metabolism. In previous studies, we have shown that the F. gigantica proteins, rFgRab10, rFgCatB and rFg14-3-34, influence cell proliferation and induce apoptosis in goat PBMCs (11–13). In the present study, we found that ESPs of F. gigantica promote cell proliferation as revealed by the CCK-8 assay (Figure 1) and upregulate cell proliferation-related genes (Table 2) of goat PBMCs. A previous study revealed that miR-211 suppresses epithelial ovarian cancer proliferation and cell-cycle progression by targeting Cyclin D1 and CDK6 (36), and miR-204-5p suppresses proliferation and invasion, and promotes chemotherapeutic sensitivity of colorectal cancer cells by downregulating RAB22A (37). The reduction in the expression of miR-211 and miR-204-5p in the present study implies a possible involvement of F. gigantica ESPs in promoting the proliferation of PBMCs.
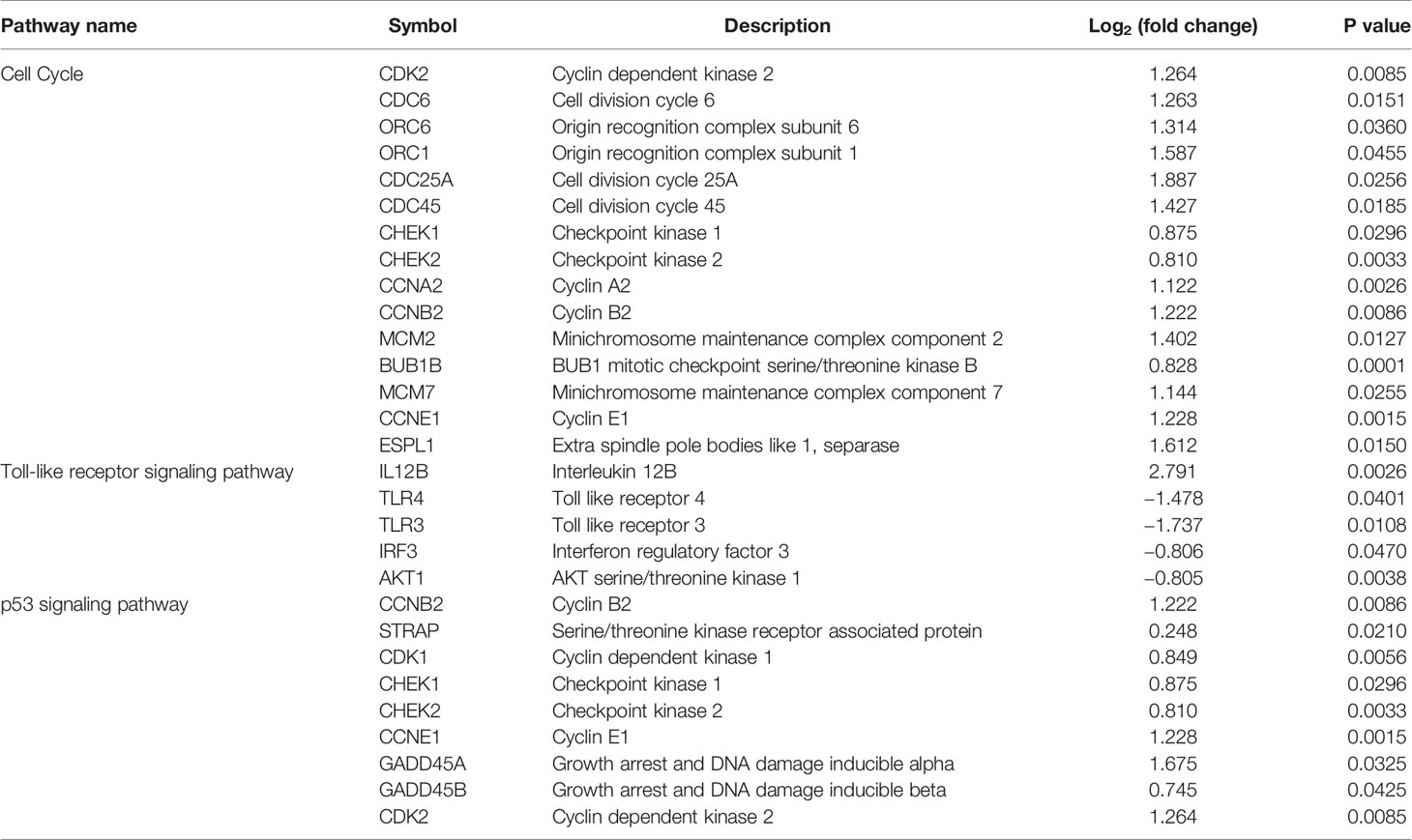
Table 2 The relative expression of the differentially expressed genes in the corresponding key pathways.
TLR3 and TLR4 play a critical role in innate immunity and induction of inflammatory response (38). Previous studies have shown that parasite infections, such as F. hepatica, F. gigantica, Leishmania, and Trypanosoma (39–42), can modulate the expression of TLRs in order to evade the host’s innate immune response. For example, stimulation of human monocyte-derived DCs with live Brugia malayi microfilariae significantly downregulates mRNA expression of TLR3, TLR4, TLR5 and TLR7 (43). In cattle, F. hepatica ESP decreases TLR2/TLR4-mediated immune activation in order to limit excessive inflammation and attenuate the pathological reactions that accompany F. hepatica infection (44). Another study suggested that TLR4 is involved in F. hepatica fatty acid-binding protein (FhFABP) and induces alternative activation of human macrophages (45). FhFABP was suggested to suppress TLR4 activation in macrophages and inhibit the inflammatory cytokines induced following incubation with LPS (16). In the present study, PBMCs incubated with F. gigantica ESPs significantly downregulated mRNA expression of TLR3 and TLR4 (Table 2). TLR3 suppression promotes a Th2 immune response to Schistosoma mansoni infection. In S. mansoni infected TLR3 gene-deficient mice, Th1-associated cytokines (e.g., IL-12) are significantly decreased and Th2-associated cytokines and chemokines are significantly increased (46). rFgCatB protein (component of F. gigantica ESPs) can induce the expression of Th2-associated cytokines (e.g., IL-4, IL-10, and TGF-β) (13). These data suggest that reduced expression of TLR3 skewed the host immune response towards a Th2 response during F. gigantica infection.
Interferon regulatory factor 3 (IRF3) is an important transcription factor involved in the downstream regulation of My88-independent TRIF-dependent TLR signaling (46), which regulates the production of type I IFNs and the induction of inducible nitric oxide synthase (iNOS) expression (47, 48). iNOS catalyzes the conversion of arginine into citrulline and nitric oxide (NO), which protect the host against pathogen invasion (49). In this study, the expression of IRF3 was significantly decreased in PBMCs treated with F. gigantica ESPs. The reduced expression of IRF3 supports the link between inhibition of TRIF-dependent TLR3 signaling and decreased expression of NOS2 during F. hepatica infection (50, 51), which may be one mechanism employed by the liver fluke to avoid damage caused by host-generated NO. These findings suggest a possible mechanism for F. gigantica ESPs in suppressing TLR expression or its signaling cascade.
In this study, the expression of miR-148a-5p was upregulated. miR-148a can inhibit pro-inflammatory cytokines (52). Also, miR-148a-3p, together with miR-30a-5p, can repress pro-inflammatory NF-κB signaling and attenuate inflammation (53). Notably, in the present study, the NF-kB signaling pathway was suppressed. Therefore, the upregulation of miR-148a-5p detected in our study may suggest a role for F. gigantica ESPs in shifting Th1/Th2 towards the Th2 immune response.
Apoptosis of immune cells is an immunosuppressive strategy employed by F. hepatica, wherein the ESPs induce apoptosis in eosinophils via a caspase-dependent mechanism, resulting ROS-mediated mitochondrial-membrane potential loss (54). F. gigantica infection can induce apoptosis in sheep PMBCs via two distinct pathways: the extrinsic death receptor pathway, which is induced by the upregulation of TNF and TNFR1/TNFR2 (40, 50), and the intrinsic mitochondrial pathway (55). In our study, most death receptors and FAS receptor were downregulated, whereas the p53 signaling pathway-related genes were upregulated (Table 2), suggesting that F. gigantica ESPs may induce apoptosis through the p53 signaling pathway, rather than by the Fas/Fasl model. Further investigation of the role of p53 signaling pathway in F. gigantica–induced apoptosis of immune cells is warranted.
Fasciola infection can disrupt liver functions, resulting in disruption of the overall metabolism of infected livestock and losses in productivity (56). KEGG analysis revealed that exposure of goat PBMCs to F. gigantica ESPs upregulated genes related to lipid, nucleotide, amino acid, carbohydrate, and glycan metabolism. Alterations in metabolism during infection with F. gigantica and F. hepatica have been reported in previous studies (57–59). However, the mechanisms by which F. gigantica ESPs alter the host metabolic pathways remain to be elucidated.
Noncoding RNAs (miRNAs, circRNAs, and lncRNAs) participate in the regulation of different biological processes. In this study, a large number of circRNAs and lncRNAs were identified. However, the exact functions of these ncRNAs remain unclear. Pathways analysis indicated that the target genes of lncRNAs were highly enriched in disease-related pathways, such as toxoplasmosis, influenza A, and hepatitis B, and immune-related pathways, such as antigen processing and presentation, the Jak-STAT signaling pathway, and cytokine-cytokine receptor interaction. The target genes of circRNAs were highly enriched in the TNF signaling pathway and Hepatitis C. However, the target genes of miRNAs were only enriched in the TGF-beta signaling pathway. The immune-regulatory roles of these ncRNAs during F. gigantica infection need to be further investigated.
In conclusion, RNA-seq profiling of goat PBMCs treated with F. gigantica ESPs revealed significant changes in the expression of mRNAs, miRNAs, lncRNAs and circRNAs. The abovementioned F. gigantica ESP-induced changes appear to mediate a number of immune-regulatory mechanisms that enable F. gigantica to establish a persistent infection. These data should form the basis for further in-depth investigations of ESP-mediated immune-evasion strategies used by F. gigantica to subvert host immune responses.
Data Availability Statement
The datasets presented in this study can be found in online repositories. The data is deposited in the NCBI repository, accession number is PRJNA686179.
Ethics Statement
This study was reviewed and approved by the Animal Ethics Committee of Lanzhou Veterinary Research Institute, Chinese Academy of Agricultural Sciences (permit no. 2018-012).
Author Contributions
X-QZ, DC, S-SW, and HE conceived and designed the experiments. S-SW and DC performed the experiments. J-JH, W-BZ, A-LT, and G-HZ contributed reagents/materials/analysis tools. S-SW and DC analyzed the data and wrote the paper. HE, J-JH, W-BZ, and X-QZ critically revised the manuscript. All authors read and approved the final version of the manuscript. All authors contributed to the article and approved the submitted version.
Funding
Project financial support was provided by the National Key Basic Research Program (973 Program) of China (grant 2015CB150300), the Agricultural Science and Technology Innovation Program (ASTIP) (grant CAAS-ASTIP-2016-LVRI-03) and Yunnan Expert Workstation (grant 202005AF150041).
Conflict of Interest
The authors declare that the research was conducted in the absence of any commercial or financial relationships that could be construed as a potential conflict of interest.
Acknowledgments
The authors would like to thank Novogene Bioinformatics Technology Co., Ltd (Beijing, China) for performing the sequencing and preliminary data analysis. The funding bodies played no role in the design of the study and collection, analysis, and interpretation of data and in writing the manuscript.
Supplementary Material
The Supplementary Material for this article can be found online at: https://www.frontiersin.org/articles/10.3389/fimmu.2021.653755/full#supplementary-material
Supplementary Table 1 | List of all differentially expressed messenger RNAs (mRNAs).
Supplementary Table 2 | List of all differentially expressed microRNAs (miRNAs).
Supplementary Table 3 | List of all differentially expressed circular RNAs (circRNAs).
Supplementary Table 4 | List of all differentially expressed long non-coding RNA (lncRNAs).
Supplementary Figure 1 | GO enrichment and KEGG pathway analyses of differentially expressed mRNAs in goat PBMCs. The 30 most enriched GO terms of the upregulated (A) and downregulated (B) mRNAs stratified according to the biological process, cellular component and molecular function categories. Scatterplots of the KEGG pathway analysis of the top 20 predominant pathways of the upregulated (C) and downregulated (D) mRNAs. The x-axis denotes the pathway enrichment. The y-axis shows the names of the significantly enriched pathways. The P-values are indicated by variations from blue to red. A deeper blue color indicates a greater significant difference.
Supplementary Figure 2 | GO enrichment and KEGG pathway analyses of target genes of differentially expressed lncRNAs in goat PBMCs. The 30 most enriched GO terms of the target genes of upregulated (A) and downregulated (B) lncRNAs in the biological process, cellular component and molecular function categories. Scatterplots of KEGG pathway analysis of the top 20 predominant pathways of the target genes of upregulated (C) and downregulated (D) lncRNAs. The x-axis shows the pathway enrichment. The y-axis represents the names of the significantly enriched pathways. The P-values are indicated by variations from blue to red. A deeper blue color indicates a greater significant difference.
References
1. Yadav S, Sharma R, Kalicharan A, Mehra U, Dass R, Verma A. Primary experimental infection of riverine buffaloes with Fasciola gigantica. Vet Parasitol (1999) 82(4):285–96. doi: 10.1016/s0304-4017(99)00005-9
2. Mas-Coma S. Epidemiology of fascioliasis in human endemic areas. J Helminthol (2005) 79(3):207–16. doi: 10.1079/joh2005296
3. Mungube EO, Bauni SM, Tenhagen BA, Wamae LW, Nginyi JM, Mugambi JM. The prevalence and economic significance of Fasciola gigantica and Stilesia hepatica in slaughtered animals in the semi-arid coastal Kenya. Trop Anim Health Prod (2006) 38(6):475–83. doi: 10.1007/s11250-006-4394-4
4. Carmona C, Tort JF. Fasciolosis in South America: epidemiology and control challenges. J Helminthol (2017) 91(2):99–109. doi: 10.1017/S0022149X16000560
5. Schwantes JB, Quevedo P, D’Ávila MF, Molento MB, Graichen DAS. Fasciola hepatica in Brazil: genetic diversity provides insights into its origin and geographic dispersion. J Helminthol (2019) 94:e83. doi: 10.1017/S0022149X19000774
6. Kelley JM, Elliott TP, Beddoe T, Anderson G, Skuce P, Spithill TW. Current threat of triclabendazole resistance in Fasciola hepatica. Trends Parasitol (2016) 32(6):458–69. doi: 10.1016/j.pt.2016.03.002
7. Girones N, Valero MA, Garcia-Bodelon MA, Chico-Calero I, Punzon C, Fresno M, et al. Immune suppression in advanced chronic fascioliasis: an experimental study in a rat model. J Infect Dis (2007) 195(10):1504–12. doi: 10.1086/514822
8. Walsh KP, Brady MT, Finlay CM, Boon L, Mills KH. Infection with a helminth parasite attenuates autoimmunity through TGF-beta-mediated suppression of Th17 and Th1 responses. J Immunol (2009) 183(3):1577–86. doi: 10.4049/jimmunol.0803803
9. Liu Q, Huang SY, Yue DM, Wang JL, Wang Y, Li X, et al. Proteomic analysis of Fasciola hepatica excretory and secretory products (FhESPs) involved in interacting with host PBMCs and cytokines by shotgun LC-MS/MS. Parasitol Res (2017) 116(2):627–35. doi: 10.1007/s00436-016-5327-4
10. Huang SY, Yue DM, Hou JL, Zhang XX, Zhang FK, Wang CR, et al. Proteomic analysis of Fasciola gigantica excretory and secretory products (FgESPs) interacting with buffalo serum of different infection periods by shotgun LC-MS/MS. Parasitol Res (2019) 118(2):453–60. doi: 10.1007/s00436-018-6169-z
11. Tian AL, Lu M, Calderón-Mantilla G, Petsalaki E, Dottorini T, Tian X, et al. A recombinant Fasciola gigantica 14-3-3 epsilon protein (rFg14-3-3e) modulates various functions of goat peripheral blood mononuclear cells. Parasit Vectors (2018) 11(1):152. doi: 10.1186/s13071-018-2745-4
12. Tian AL, Lu M, Zhang FK, Calderón-Mantilla G, Petsalaki E, Tian X, et al. The pervasive effects of recombinant Fasciola gigantica Ras-related protein Rab10 on the functions of goat peripheral blood mononuclear cells. Parasit Vectors (2018) 11(1):579. doi: 10.1186/s13071-018-3148-2
13. Chen D, Tian AL, Hou JL, Li JX, Tian X, Yuan XD, et al. The multitasking Fasciola gigantica cathepsin B interferes with various functions of goat peripheral blood mononuclear cells in vitro. Front Immunol (2019) 10:1707. doi: 10.3389/fimmu.2019.01707
14. Tian AL, Tian X, Chen D, Lu M, Calderón-Mantilla G, Yuan XD, et al. Modulation of the functions of goat peripheral blood mononuclear cells by Fasciola gigantica thioredoxin peroxidase in vitro. Pathogens (2020) 9(9):758. doi: 10.3390/pathogens9090758
15. Falcón C, Carranza F, Martínez FF, Knubel CP, Masih DT, Motrán CC, et al. Excretory-secretory products (ESP) from Fasciola hepatica induce tolerogenic properties in myeloid dendritic cells. Vet Immunol Immunopathol (2010) 137(1-2):36–46. doi: 10.1016/j.vetimm.2010.04.007
16. Martin I, Caban-Hernandez K, Figueroa-Santiago O, Espino AM. Fasciola hepatica fatty acid binding protein inhibits TLR4 activation and suppresses the inflammatory cytokines induced by lipopolysaccharide in vitro and in vivo. J Immunol (2015) 194(8):3924–36. doi: 10.4049/jimmunol.1401182
17. Novobilský A, Kasný M, Mikes L, Kovarcík K, Koudela B. Humoral immune responses during experimental infection with Fascioloides magna and Fasciola hepatica in goats and comparison of their excretory/secretory products. Parasitol Res (2007) 101(2):357–64. doi: 10.1007/s00436-007-0463-5
18. El Ridi R, Salah M, Wagih A, William H, Tallima H, El Shafie MH, et al. Fasciola gigantica excretory-secretory products for immunodiagnosis and prevention of sheep fasciolosis. Vet Parasitol (2007) 149(3-4):219–28. doi: 10.1016/j.vetpar.2007.08.024
19. Shi W, He JJ, Mei XF, Lu KJ, Zeng ZX, Zhang YY, et al. Dysregulation of hepatic microRNA expression in C57BL/6 mice affected by excretory-secretory products of Fasciola gigantica. PLoS Negl Trop Dis (2020) 14(12):e0008951. doi: 10.1371/journal.pntd.0008951
20. Bolger AM, Lohse M, Usadel B. Trimmomatic: a flexible trimmer for Illumina sequence data. Bioinformatics (2014) 30(15):2114–20. doi: 10.1093/bioinformatics/btu170
21. Kim D, Langmead B, Salzberg SL. HISAT: a fast spliced aligner with low memory requirements. Nat Methods (2015) 12(4):357–60. doi: 10.1038/nmeth.3317
22. Wang L, Wang S, Li W. RSeQC: quality control of RNA-seq experiments. Bioinformatics (2012) 28(16):2184–5. doi: 10.1093/bioinformatics/bts356
23. Wang L, Feng Z, Wang X, Wang X, Zhang X. DEGseq: an R package for identifying differentially expressed genes from RNA-seq data. Bioinformatics (2010) 26(1):136–8. doi: 10.1093/bioinformatics/btp612
24. Langmead B, Trapnell C, Pop M, Salzberg SL. Ultrafast and memory-efficient alignment of short DNA sequences to the human genome. Genome Biol (2009) 10(3):R25. doi: 10.1186/gb-2009-10-3-r25
25. Friedländer MR, Mackowiak SD, Li N, Chen W, Rajewsky N. miRDeep2 accurately identifies known and hundreds of novel microRNA genes in seven animal clades. Nucleic Acids Res (2012) 40(1):37–52. doi: 10.1093/nar/gkr688
26. Wen M, Shen Y, Shi S, Tang T. miREvo: An Integrative microRNA evolutionary analysis platform for next-generation sequencing experiments. BMC Bioinf (2012) 13:140. doi: 10.1186/1471-2105-13-140
27. Li A, Zhang J, Zhou Z. PLEK: a tool for predicting long non-coding RNAs and messenger RNAs based on an improved k-mer scheme. BMC Bioinf (2014) 15(1):311. doi: 10.1186/1471-2105-15-311
28. Gao Y, Wang J, Zhao F. CIRI: an efficient and unbiased algorithm for de novo circular RNA identification. Genome Biol (2015) 16(1):4. doi: 10.1186/s13059-014-0571-3
29. Pertea M, Pertea GM, Antonescu CM, Chang TC, Mendell JT, Salzberg SL. StringTie enables improved reconstruction of a transcriptome from RNA-seq reads. Nat Biotechnol (2015) 33(3):290–5. doi: 10.1038/nbt.3122
30. Trapnell C, Roberts A, Goff L, Pertea G, Kim D, Kelley DR, et al. Differential gene and transcript expression analysis of RNA-seq experiments with TopHat and Cufflinks. Nat Protoc (2012) 7(3):562–78. doi: 10.1038/nprot.2012.016
31. Kong L, Zhang Y, Ye ZQ, Liu XQ, Zhao SQ, Wei L, et al. CPC: assess the protein-coding potential of transcripts using sequence features and support vector machine. Nucleic Acids Res (2007) 35(Web Server issue):W345–9. doi: 10.1093/nar/gkm391
32. Finn RD, Mistry J, Schuster-Böckler B, Griffiths-Jones S, Hollich V, Lassmann T, et al. Pfam: clans, web tools and services. Nucleic Acids Res (2006) 34(Database issue):D247–51. doi: 10.1093/nar/gkj149
33. Young MD, Wakefield MJ, Smyth GK, Oshlack A. Gene ontology analysis for RNA-seq: accounting for selection bias. Genome Biol (2010) 11(2):R14. doi: 10.1186/gb-2010-11-2-r14
34. Kanehisa M, Araki M, Goto S, Hattori M, Hirakawa M, Itoh M, et al. KEGG for linking genomes to life and the environment. Nucleic Acids Res (2008) 36(Database issue):D480–4. doi: 10.1093/nar/gkm882
35. Mao X, Cai T, Olyarchuk JG, Wei L. Automated genome annotation and pathway identification using the KEGG Orthology (KO) as a controlled vocabulary. Bioinformatics (2005) 21(19):3787–93. doi: 10.1093/bioinformatics/bti430
36. Xia B, Yang S, Liu T, Lou G. miR-211 suppresses epithelial ovarian cancer proliferation and cell-cycle progression by targeting Cyclin D1 and CDK6. Mol Cancer (2015) 14:57. doi: 10.1186/s12943-015-0322-4
37. Yin Y, Zhang B, Wang W, Fei B, Quan C, Zhang J, et al. miR-204-5p inhibits proliferation and invasion and enhances chemotherapeutic sensitivity of colorectal cancer cells by downregulating RAB22A. Clin Cancer Res (2014) 20(23):6187–99. doi: 10.1158/1078-0432.CCR-14-1030
38. Brubaker SW, Bonham KS, Zanoni I, Kagan JC. Innate immune pattern recognition: a cell biological perspective. Annu Rev Immunol (2015) 33:257–90. doi: 10.1146/annurev-immunol-032414-112240
39. Ashour DS. Toll-like receptor signaling in parasitic infections. Expert Rev Clin Immunol (2015) 11(6):771–80. doi: 10.1586/1744666X.2015.1037286
40. Fu Y, Browne JA, Killick K, Mulcahy G. Network analysis of the systemic response to Fasciola hepatica infection in sheep reveals changes in fibrosis, apoptosis, toll-like receptors 3/4, and B cell function. Front Immunol (2017) 8:485. doi: 10.3389/fimmu.2017.00485
41. Zhang FK, Hou JL, Guo AJ, Tian AL, Sheng ZA, Zheng WB, et al. Expression profiles of genes involved in TLRs and NLRs signaling pathways of water buffaloes infected with Fasciola gigantica. Mol Immunol (2018) 94:18–26. doi: 10.1016/j.molimm.2017.12.007
42. Zhang FK, Zhang XX, Elsheikha HM, He JJ, Zhu XQ. Transcriptomic responses of water buffalo liver to infection with the digenetic fluke. Fasciola Gigantica Parasit Vectors (2017) 10(1):56. doi: 10.1186/s13071-017-1990-2
43. Semnani RT, Venugopal PG, Leifer CA, Mostböck S, Sabzevari H, Nutman TB. Inhibition of TLR3 and TLR4 function and expression in human dendritic cells by helminth parasites. Blood (2008) 112(4):1290–8. doi: 10.1182/blood-2008-04-149856
44. Flynn RJ, Mulcahy G. Possible role for toll-like receptors in interaction of Fasciola hepatica excretory/secretory products with bovine macrophages. Infect Immun (2008) 76(2):678–84. doi: 10.1128/IAI.00732-07
45. Figueroa-Santiago O, Espino AM. Fasciola hepatica fatty acid binding protein induces the alternative activation of human macrophages. Infect Immun (2014) 82(12):5005–12. doi: 10.1128/IAI.02541-14
46. Joshi AD, Schaller MA, Lukacs NW, Kunkel SL, Hogaboam CM. TLR3 modulates immunopathology during a Schistosoma mansoni egg-driven Th2 response in the lung. Eur J Immunol (2008) 38(12):3436–49. doi: 10.1002/eji.200838629
47. Sen GC, Sarkar SN. Transcriptional signaling by double-stranded RNA: role of TLR3. Cytokine Growth Factor Rev (2005) 16(1):1–14. doi: 10.1016/j.cytogfr.2005.01.006
48. Donnelly S, O’Neill SM, Stack CM, Robinson MW, Turnbull L, Whitchurch C, et al. Helminth cysteine proteases inhibit TRIF-dependent activation of macrophages via degradation of TLR3. J Biol Chem (2010) 285(5):3383–92. doi: 10.1074/jbc.M109.060368
49. Bogdan C. Nitric oxide synthase in innate and adaptive immunity: an update. Trends Immunol (2015) 36(3):161–78. doi: 10.1016/j.it.2015.01.003
50. Fu Y, Chryssafidis AL, Browne JA, O’Sullivan J, McGettigan PA, Mulcahy G. Transcriptomic Study on Ovine Immune Responses to Fasciola hepatica Infection. PLoS Negl Trop Dis (2016) 10(9):e0005015. doi: 10.1371/journal.pntd.0005015
51. Flynn RJ, Irwin JA, Olivier M, Sekiya M, Dalton JP, Mulcahy G. Alternative activation of ruminant macrophages by Fasciola hepatica. Vet Immunol Immunopathol (2007) 120(1-2):31–40. doi: 10.1016/j.vetimm.2007.07.003
52. Li G, Tang X, Chen H, Sun W, Yuan F. miR-148a inhibits pro-inflammatory cytokines released by intervertebral disc cells by regulating the p38/MAPK pathway. Exp Ther Med (2018) 16(3):2665–9. doi: 10.3892/etm.2018.6516
53. Patel V, Carrion K, Hollands A, Hinton A, Gallegos T, Dyo J, et al. The stretch responsive microRNA miR-148a-3p is a novel repressor of IKBKB, NF-κB signaling, and inflammatory gene expression in human aortic valve cells. FASEB J (2015) 29(5):1859–68. doi: 10.1096/fj.14-257808
54. Serradell MC, Guasconi L, Masih DT. Involvement of a mitochondrial pathway and key role of hydrogen peroxide during eosinophil apoptosis induced by excretory-secretory products from Fasciola hepatica. Mol Biochem Parasitol (2009) 163(2):95–106. doi: 10.1016/j.molbiopara.2008.10.005
55. Elmore S. Apoptosis: a review of programmed cell death. Toxicol Pathol (2007) 35(4):495–516. doi: 10.1080/01926230701320337
56. Lenton LM, Bygrave FL, Behm CA, Boray JC. Fasciola hepatica infection in sheep: changes in liver metabolism. Res Vet Sci (1996) 61(2):152–6. doi: 10.1016/s0034-5288(96)90091-0
57. Phiri IK, Phiri AM, Harrison LJ. The serum glucose and beta-hydroxybutyrate levels in sheep with experimental Fasciola hepatica and Fasciola gigantica infection. Vet Parasitol (2007) 143(3-4):287–93. doi: 10.1016/j.vetpar.2006.09.001
58. Kozat S, Denizhan V. Glucose, lipid, and lipoprotein levels in sheep naturally infected with Fasciola hepatica. J Parasitol (2010) 96(3):657–9. doi: 10.1645/GE-2104.1
Keywords: Fasciola gigantica, peripheral blood mononuclear cells, RNA-seq, excretory-secretory products, long noncoding RNA
Citation: Wang S-S, Chen D, He J-J, Zheng W-B, Tian A-L, Zhao G-H, Elsheikha HM and Zhu X-Q (2021) Fasciola gigantica–Derived Excretory-Secretory Products Alter the Expression of mRNAs, miRNAs, lncRNAs, and circRNAs Involved in the Immune Response and Metabolism in Goat Peripheral Blood Mononuclear Cells. Front. Immunol. 12:653755. doi: 10.3389/fimmu.2021.653755
Received: 15 January 2021; Accepted: 10 March 2021;
Published: 12 April 2021.
Edited by:
Wanderley De Souza, Federal University of Rio de Janeiro, BrazilReviewed by:
Jiezuan Yang, Zhejiang University, ChinaEduardo José Lopes Torres, Rio de Janeiro State University, Brazil
Carlos Robello, Universidad de la República, Uruguay
Copyright © 2021 Wang, Chen, He, Zheng, Tian, Zhao, Elsheikha and Zhu. This is an open-access article distributed under the terms of the Creative Commons Attribution License (CC BY). The use, distribution or reproduction in other forums is permitted, provided the original author(s) and the copyright owner(s) are credited and that the original publication in this journal is cited, in accordance with accepted academic practice. No use, distribution or reproduction is permitted which does not comply with these terms.
*Correspondence: Hany M. Elsheikha, hany.elsheikha@nottingham.ac.uk; Xing-Quan Zhu, xingquanzhu1@hotmail.com
†These authors have contributed equally to this work