- 1Medical Oncology Unit, Austin Health, Melbourne, VIC, Australia
- 2Olivia Newton-John Cancer Research Institute, Melbourne, VIC, Australia
- 3La Trobe University School of Cancer Medicine, Melbourne, VIC, Australia
- 4Department of Mathematics and Statistics, La Trobe University, Melbourne, VIC, Australia
- 5Department of Medical Oncology, Westmead and Blacktown Hospitals, Sydney, NSW, Australia
- 6Melanoma Institute Australia, The University of Sydney, North Sydney, NSW, Australia
- 7Department of Medical Oncology, Royal North Shore and Mater Hospitals, Sydney, NSW, Australia
- 8Tissue Pathology and Diagnostic Oncology, Royal Prince Alfred Hospital and NSW Health Pathology, Sydney, NSW, Australia
- 9Faculty of Medicine and Health, The University of Sydney, Sydney, NSW, Australia
- 10Department of Clinical Medicine, Macquarie University, Sydney, NSW, Australia
- 11Department of Medicine, University of Melbourne, Melbourne, VIC, Australia
A significant number of patients (pts) with metastatic melanoma do not respond to anti-programmed cell death 1 (PD1) therapies. Identifying predictive biomarkers therefore remains an urgent need. We retrospectively analyzed plasma DNA of pts with advanced melanoma treated with PD-1 antibodies, nivolumab or pembrolizumab, for five PD-1 genotype single nucleotide polymorphisms (SNPs): PD1.1 (rs36084323, G>A), PD1.3 (rs11568821, G>A), PD1.5 (rs2227981, C>T) PD1.6 (rs10204225, G>A) and PD1.9 (rs2227982, C>T). Clinico-pathological and treatment parameters were collected, and presence of SNPs correlated with response, progression free survival (PFS) and overall survival (OS). 115 patients were identified with a median follow up of 18.7 months (range 0.26 – 52.0 months). All were Caucasian; 27% BRAF V600 mutation positive. At PD-1 antibody commencement, 36% were treatment-naïve and 52% had prior ipilimumab. The overall response rate was 43%, 19% achieving a complete response. Overall median PFS was 11.0 months (95% CI 5.4 - 17.3) and median OS was 31.1 months (95% CI 23.2 - NA). Patients with the G/G genotype had more complete responses than with A/G genotype (16.5% vs. 2.6% respectively) and the G allele of PD1.3 rs11568821 was significantly associated with a longer median PFS than the AG allele, 14.1 vs. 7.0 months compared to the A allele (p=0.04; 95% CI 0.14 – 0.94). No significant association between the remaining SNPs and responses, PFS or OS were observed. Despite limitations in sample size, this is the first study to demonstrate an association of a germline PD-1 polymorphism and PFS in response to anti-PD-1 therapy in pts with metastatic melanoma. Extrinsic factors like host germline polymorphisms should be considered with tumor intrinsic factors as predictive biomarkers for immune checkpoint regulators.
Introduction
Programmed cell death-1 (PD-1) is a member of the CD28 family of co-stimulatory molecules and is expressed on activated CD4+ and CD8+ T cells (1). Upon binding to its ligand, programmed death-ligand 1 and 2 (PD-L1 and PD-L2), PD-1 is responsible for negatively regulating the effector phase of T-cell responses and maintaining immune tolerance (2). Constitutive high level expression of PD-1 on tumor specific T lymphocytes is a major factor restraining an effective anti-tumor immune response in patients with advanced malignancies (3). The monoclonal antibodies targeting PD-1, nivolumab and pembrolizumab, have shown impressive and durable responses in cancer patients resulting in regulatory approval in many cancer subtypes. Despite the success of these agents, a significant proportion of patients do not respond to anti-PD-1 therapy; therefore identifying biomarkers that predict therapeutic efficacy remains an urgent need (4). The human gene for PD-1, PDCD1, is localized on chromosome 2q37 (5). A number of single nucleotide polymorphisms (SNPs) in PDCD1 have been identified and shown to be associated with the development of autoimmune conditions, including Crohn’s disease, systemic lupus erythematosus, type I diabetes, rheumatoid arthritis, and multiple sclerosis (6). In addition, certain PD-1 polymorphisms have been shown to be associated with an improved viral control in patients with chronic viral infections (7). The effect of PD-1 polymorphism in cancer remains unclear, with some studies reporting an increase in the risk of developing some cancer types while others have reported a reduced risk (8–10). Despite the crucial role that the PD-1/PD-L1 pathway plays in limiting anti-tumor immune responses, there are no data exploring the potential influence of PD-1 polymorphisms on the treatment response to anti-PD-1 blockade.
In this study we retrospectively evaluated the association of polymorphisms in PDCD1 with responses and survival in patients with metastatic melanoma treated with anti-PD-1 monoclonal antibodies. We demonstrate that certain PD-1 SNPs may be associated with improved anti-melanoma outcomes after immunotherapy and can potentially serve as biomarkers.
Methods
Patients
Patients with metastatic melanoma treated with single agent anti-PD-1 antibodies, nivolumab or pembrolizumab, between January 2014 and June 2016 in three major Australian melanoma centers were studied. Data collected included: baseline demographics, disease stage, disease characteristics; details of PD-1 inhibitor treatment (type, dosage, number of cycles received); prior systemic treatments; and time to endpoint data. End-points evaluated were overall response rate (ORR; defined as CR or PR), progression free survival (PFS) and overall survival (OS). PFS was defined as time between date of commencement of therapy to date of progression or death. Response assessments were made as per the Response Evaluation Criteria in Solid Tumors (RECIST) v1.1 (11).
Genotyping
The SNPs selected for study were those either located in the promoter or untranslated region or coding region of the gene, those previously evaluated in relation to cancer, or those with evidence of functional significance in autoimmune diseases. DNA extracted from baseline blood samples were analyzed by polymerase chain reaction (PCR) and high-resolution melt (HRM) analysis on the Rotor-Gene 6000 (Corbett Life Science). A 20µL reaction mixture contained; 1X PCR Buffer, 2.5mM MgCl2, 800nM total of dNTPs, 250nM forward primer, 250nM reverse primer, 5 µM of SYTO9 intercalating dye (Invitrogen), 0.5 U of HotStarTaq polymerase (Qiagen), 2 ng of genomic DNA and UltraPure™ PCR grade water (ThermoFisher Scientific). The cycling and melting conditions for all PD-1 SNP genotyping assays were as follows; 15 min activation at 95°C followed by 55 PCR cycles of 98°C for 20 seconds, 65°C for 30 seconds and 72°C for 25 seconds, then a post PCR hold at 98°C for 1 min followed by high resolution melting from 68°C to 98°C, 0.2°C per step. All samples were tested in duplicate and analyzed using the Rotor-gene 6000 software.
The study was approved by individual institution ethics committees of Austin Health, Melbourne, Westmead Hospital, Royal North Shore and Mater Hospitals, Sydney and patients either prospectively consented to inclusion or consent was waived as per individual institution ethics committee guidance.
Statistical Analysis
Kaplan-Meier estimates of PFS and OS from commencement of immune checkpoint therapy were calculated separately for each individual SNP. Hazard ratios and corresponding 95% confidence intervals were estimated through univariate and multivariate Cox proportional hazard models. Multivariate logistic regression analysis was used to explore the association between responses and SNP genotype. We performed Fisher’s exact tests per SNP genotypes to assess if responses observed between different SNP genotypes was significant. All analyses were carried out using R version 3.4.0 (12).
Results
Demographics and Efficacy Analyses
A total of 115 patients received either pembrolizumab or nivolumab between January 2014 and June 2016. The median follow-up after commencement of anti-PD-1 therapy was 18.7 months (range 0.26 – 52.0 months). The median age was 71.3 years (29.4 – 92.4), all patients were Caucasian and BRAF V600 mutations were detected in 24% of patients, while NRAS mutations were detected in 25% (Table 1). Thirty-six percent of patients were treatment naïve, 52% being treated with prior ipilimumab. The genotype frequency of SNPs evaluated in this study is consistent those reported in Caucasian populations (13) (Table 2).
In our study the ORR was 43% with 22 patients (19%) obtaining a complete response. The median PFS was 11.0 months (95% CI 5.4 - 17.3) and median OS was 31.1 months (95% CI 23.2 - NA).
Association Between Genotypes and Clinical Outcome
Five PD-1 SNPs were genotyped in this cohort of patients with metastatic melanoma treated with an anti-PD-1 inhibitor. Almost a third of patients, 32 (28%), had the PD1.3 rs11568821 A/G genotype and 83 (72%) the G/G genotype. Patients with the G/G genotype had more complete responses than with A/G genotype (16.5% vs. 2.6%) respectively (Figure 1). No significant association between the remaining SNPs and responses were observed or between SNP genotypes and response (Supplementary Table 1). Kaplan-Meier estimates of PFS were calculated for each SNP (Figure 2) as well as separately for each PD1.3 genotype (Supplementary Figure 1). On univariate analysis, none of the PD-1 SNPs evaluated influenced PFS or OS, however in a multivariate Cox regression model (Supplementary Table 2) including AJCC stage and an interaction term with age, the presence of the G/G PD1.3 SNP was significantly associated with an improved PFS (p= 0.04).
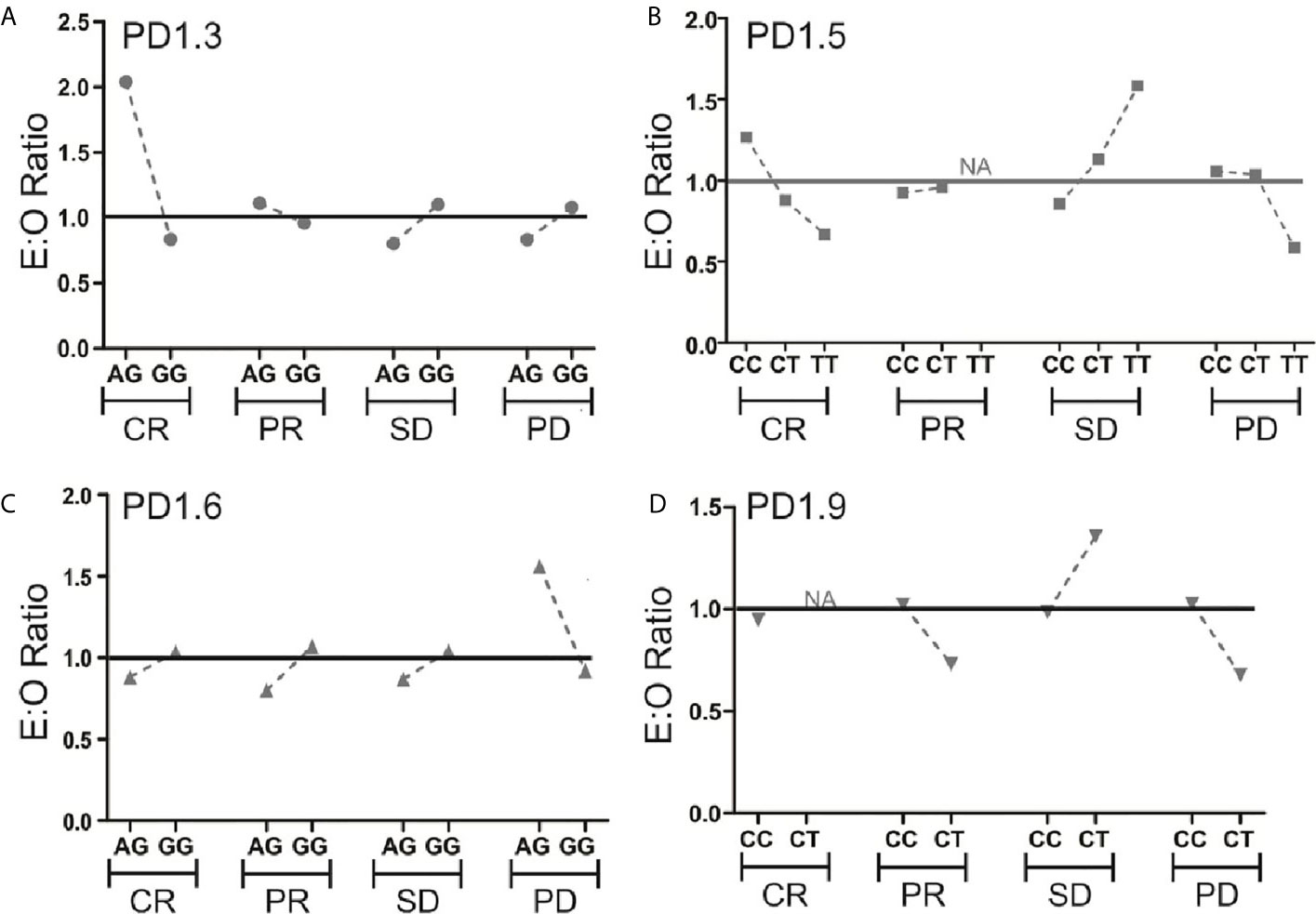
Figure 1 Expected (E)-to-observed (O) ratio of responses according to PD-1 SNP. Responses for all 4 SNPs (A–D) are shown as complete response (CR), partial response (PR), stable disease (SD) or progressive disease (PD) by dividing number of expected responses by number of observed responses within each genotype. The solid line at y=1 represents a theoretical E:O ratio of 1:1. NA signifies data points where one genotype had 0 events.
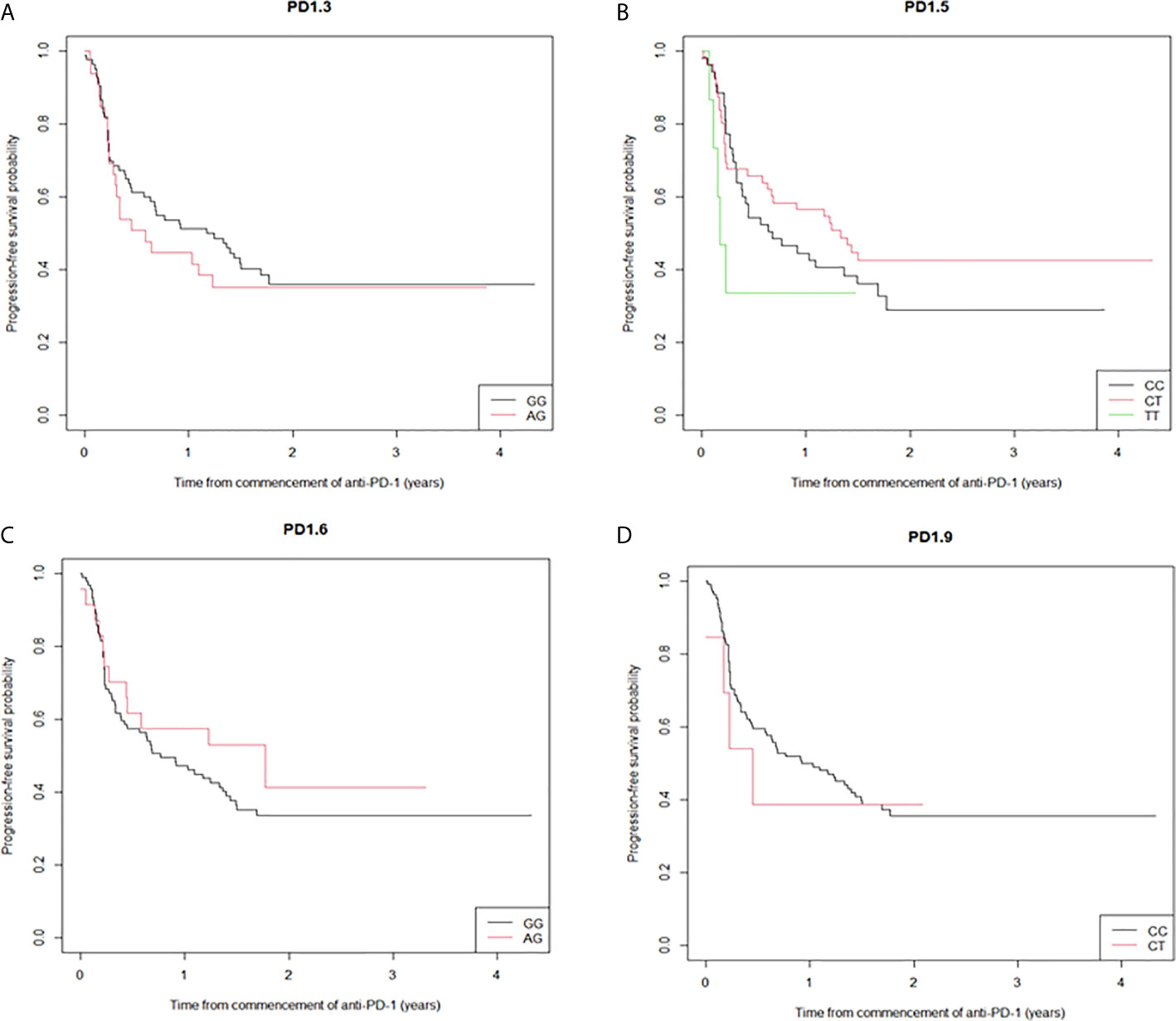
Figure 2 Kaplan-Meier curves of progression-free survival stratified by the individual SNPs. (A) the median PFS for PD1.3 rs11568821 GG allele was 14.1 months versus 7.0 months for the AG allele (HR 0.836 (95%CI (0.50-1.39) p=0.49); (B) the median PFS for PD1.5 rs2227981 CT allele 16 months (HR 0.79 (95%CI (0.48-1.27) p=0.329), 2.1 months for the TT allele (HR 1.78 (95%CI (0.70-4.57) p=0.228) versus 8.1 months for the CC allele; (C) the median PFS for PD1.6 rs10204525 21.3 months for the AG allele versus 9.2 months for the GG allele (HR 0.75 (95%CI (0.40-1.40) p=0.364); (D) the median PFS for PD1.9 rs2227982 CC allele was 11.0 months versus 5.4 months for the CT allele (HR 0.73 (95%CI (0.27-2.01) p=0.543).
Discussion
This is the first study to evaluate the association between response to PD-1 inhibitors and germline polymorphisms in PDCD1 in patients with metastatic melanoma. The ORR in our patient cohort was similar to those reported in clinical trials of anti-PD1 therapies in metastatic melanoma (14, 15). We identified that the G allele of PD1.3 rs11568821 was significantly associated with longer PFS in anti-PD-1 treated patients. Germline SNPs in immune-regulatory genes have been widely conducted, but mainly in the context of cancer-risk, as nicely summarized by Wagner et al. (16). Only a handful of studies have linked responses to immunotherapy or the advent of immune-related adverse events (irAEs) during treatment to SNPs in various immune-regulatory genomic regions, including CTLA4 and PDCD1 (17–20). The PDCD1 SNPs PD1.5 (rs2227981) and PD1.9 (rs2227982) are both located within exon 5 of the PDCD1. PD1.5 is a synonymous polymorphism while PD1.9 is a non-synonymous SNP resulting in the amino acid substitution from valine to alanine at codon 215. The PD1.1 SNP G/A (rs36084323) is located in the promoter region, and was very uncommon in our patient population as previously reported (21). PD1.3 (rs11568821) is a guanine (G) to adenine (A) SNP in intron 4 at nucleotide +7146. This substitution alters the binding of runt-related transcription factor 1 (RUNX1) transcription factors affecting transcriptional regulation and PD-1 expression (21–24). Additionally this SNP results in impaired PD-1-mediated inhibition of T cell proliferation and interferon gamma (IFNγ) production and augments lymphocyte activity (25). Our findings suggest that aberrant regulation of PD-1 expression leads to the observed increased efficacy of anti-PD-1 therapy in patients carrying this distinct polymorphism. In keeping with such a mechanism pre-clinical work demonstrates that a lower PD-1 expression level on tumor specific T lymphocytes is predictive for a response to anti-PD-1 blockade in mouse tumor models (26). It could also be speculated that the frequency of exhausted PD-1+ T cell subpopulations that can be reinvigorated by anti-PD-1 blockade may differ between individuals based on the presence of certain PD-1 germline polymorphisms but functional effects of PD1.3 in the cancer setting remains to be established. Of interest, several population-based studies conducted largely in the Asian population and meta-analysis of available data have shown a significantly lower cancer risk associated with the PD1.3 (rs11568821) SNP (8, 27, 28), while other studies could not confirm these findings (29). The risk for the development of melanoma and the here examined SNPs is unclear, and we have not been able to find well annotated WGS data in sufficiently large cohorts of melanoma patients to cross-reference observed-to-expected minor allele frequency (MAF) as derived from the 1000 Genomes project. TCGA is providing healthy tissue WGS data from only minor sample populations, WGS data from healthy tissue, and even the recently published Pan-cancer analysis of whole genomes provides only information for a small set of melanoma patients, not all of which are of Caucasian origin (30). Multiple other limitations for GWAS studies exist, including lifestyle and additional factors that may contribute to cancer risk and most of these limitations are true for our study as well. The MAF frequencies as listed in Table 2 demonstrate that a very large patient number beyond the here presented one would be necessary for a sufficiently powered (0.8) study. Additionally, the number of SNPs within each study needs to be corrected for further increasing the necessary sample size for significance. Hence, the here detected associations (and non-associations), while interesting, need confirmation in much larger cohorts, and efforts to establish these are currently underway (31). Another additional challenge is to distinguish between predictive vs prognostic associations, hence study control arms (where available from historical trials) would need to be typed for SNP occurrence as well.
So far efforts to identify predictive biomarkers to anti-PD-1 therapy have mainly focused on tumor intrinsic factors. PD-L1 expression on melanoma cells has shown to enrich for responders to treatment with anti-PD-1 antibodies however in all studies evaluating anti-PD-1 therapies, a significant proportion of PD-L1 negative patients benefitted from treatment (32–34). This can be explained by the spatial and temporal heterogeneous PD-L1 expression in tumor tissue and the discordance in PD-L1 expression between metastatic tissue of the same patient (35, 36). Furthermore, apart from inherent technical issues with IHC, varying IHC cut-offs to define PD-L1 positivity, oncogenic versus induced PD-L1 expression, staining of tumor versus immune cells and the dynamic nature of PD-L1 expression complicates its use as a reliable biomarker (37, 38). Similarly patients with tumors that harbor higher numbers of non-synonymous single nucleotide polymorphisms leading to an increased number of neoepitopes have a trend to a better response to anti-PD-1 treatment (39). In addition, clinical pathological factors including peripheral blood markers (38, 40) were also be shown of potential predictive value. More recently it has been shown that the composition of intestinal microbiome can influence the response to anti-PD-1 therapy (41). Given the complexity of anti-tumor immune responses it is now recognized that no single biomarker can predict the success to anti-PD-1 blockade. Nonetheless a variety of factors that are associated with more or less favorable outcomes are being identified and aggregating such tumor-intrinsic features and tumor-extrinsic factors is likely to lead to models (or algorithms) that can assist identifying those patients most likely to respond to anti-PD-1 therapy and those likely to need alternative approaches including combination immunotherapies. Our study identifies the G allele of PD1.3 rs11568821 as the potential first germline SNP biomarker for the efficacy of anti-PD-1 therapies.
In conclusion, this is the first study that potentially demonstrates an association of a germline PD-1 polymorphism and longer PFS in response to anti-PD-1 therapy in patients with metastatic melanoma. Unfortunately, no PBMCs are available from the study cohort, so we could not pinpoint therapy-predictive value of SNPs with PD-1 expression. Additionally, the relative short follow-up time and limited study cohort size (n=115) available for this study emphasizes the importance of confirmative study cohorts. Future studies should additionally include SNPs or SNV in other relevant genes that may influence response to anti-PD-1 treatment or combination immunotherapies that may be used upfront or sequentially if the initial therapy fails. Despite these limitations these results suggest that PD-1 rs11568821 may be a biomarker for identifying patients likely to benefit from anti-PD-1 therapies. Together with other immune-markers this will help in establishing patient-specific cancer- immunomaps for patient stratification and prediction of response.
Data Availability Statement
The raw data supporting the conclusions of this article will be made available by the authors, without undue reservation.
Ethics Statement
The study was approved by individual institution ethics committees of Austin Health, Melbourne, Westmead Hospital, Royal North Shore and Mater Hospitals, Sydney and patients either prospectively consented to inclusion or consent was waived as per individual institution ethics committee guidance. The ethics committee waived the requirement of written informed consent for participation.
Author Contributions
AB, OK, AD, and SbP contributed to conception and design of the study. AB, OK and SgP wrote the manuscript. AD, CS, and AMu wrote sections of the manuscript. All authors contributed to sample collection, data gathering and analysis and manuscript revision. All authors contributed to the article and approved the submitted version.
Conflict of Interest
MC has a consultant advisory role with BMS, MSD, Amgen, Novartis, Pierre Fabre, Roche, Sanofi, Merck and Co, Ideaya, Regeneron, Nektar, Eisai and Q biotics and OncoSec. AMM is a consultant advisor to BMS, MSD, Novartis, Roche, Pierre-Fabre, QBiotics. RAS has received fees for professional services from Qbiotics, Novartis, Merck Sharp & Dohme, NeraCare, AMGEN Inc., Bristol-Myers Squibb, Myriad Genetics, and GlaxoSmithKline. JC has sat on advisory boards for Novartis and GSK. GL is consultant advisor for Aduro Biotech Inc, Amgen Inc, Array Biopharma inc, Boehringer Ingelheim International GmbH, Bristol-Myers Squibb, Hexel AG, Highlight Therapeutics S.L., Merck Sharpe & Dohme, Novartis Pharma AG, OncoSec, Pierre Fabre, QBiotics Group Limited, Regeneron Pharmaceuticals Inc, SkylineDX B.V., and Specialised Therapeutics Australia Pty Ltd.
The remaining authors declare that the research was conducted in the absence of any commercial or financial relationships that could be construed as a potential conflict of interest.
Acknowledgments
This work was supported by funds from the Operational Infrastructure Support Program provided by the Victorian Government, Australia and Melanoma Research Victoria (MRV). AMM is supported by a Cancer Institute NSW Fellowship. A. Behren is the recipient of a Fellowship from the Victorian Government Department of Health and Human Services acting through the Victorian Cancer Agency. We would like to thank Sudheer Veduruparthi for his assistance in SNP genotyping. RS is supported by a National Health and Medical Research Council of Australia (NHMRC) Program Grant (APP1093017) and Practitioner Fellowship (APP1141295).
Supplementary Material
The Supplementary Material for this article can be found online at: https://www.frontiersin.org/articles/10.3389/fimmu.2021.672521/full#supplementary-material
Supplementary Table 1 | Relationship between responses vs genotype.
Supplementary Table 2 | Multivariate Cox model for PFS.
Supplementary Figure 1 | Kaplan-Meier curves of progression-free survival stratified by the individual genotype for SNP 1.3, GG allele versus AG allele respectively (A) Complete response (B) Partial response (C) Stable disease (D) Progressive disease.
References
1. Iwai Y, Hamanishi J, Chamoto K, Honjo T. Cancer Immunotherapies Targeting the PD-1 Signaling Pathway. J Biomed Sci (2017) 24(1):26. doi: 10.1186/s12929-017-0329-9
2. Topalian SL, Drake CG, Pardoll DM. Targeting the PD-1/B7-H1 (Pd-L1) Pathway to Activate Anti-Tumor Immunity. Curr Opin Immunol (2012) 24(2):207–12. doi: 10.1016/j.coi.2011.12.009
3. Ahmadzadeh M, Johnson LA, Heemskerk B, Wunderlich JR, Dudley ME, White DE, et al. Tumor Antigen–Specific Cd8 T Cells Infiltrating the Tumor Express High Levels of PD-1 and are Functionally Impaired. Blood (2009) 114(8):1537–44. doi: 10.1182/blood-2008-12-195792
4. Fox BA, Schendel DJ, Butterfield LH, Aamdal S, Allison JP, Ascierto PA, et al. Defining the Critical Hurdles in Cancer Immunotherapy. J Trans Med (2011) 9(1):214. doi: 10.1186/1479-5876-9-214
5. Shinohara T, Taniwaki M, Ishida Y, Kawaichi M, Honjo T. Structure and Chromosomal Localization of the Human Pd-1 Gene (Pdcd1). Genomics (1994) 23(3):704–6. doi: 10.1006/geno.1994.1562
6. Gianchecchi E, Delfino DV, Fierabracci A. Recent Insights Into the Role of the PD-1/PD-L1 Pathway in Immunological Tolerance and Autoimmunity. Autoimmun Rev (2013) 12(11):1091–100. doi: 10.1016/j.autrev.2013.05.003
7. Zheng L, Li D, Wang F, Wu H, Li X, Fu J, et al. Association Between Hepatitis B Viral Burden in Chronic Infection and a Functional Single Nucleotide Polymorphism of the PDCD1 Gene. J Clin Immunol (2010) 30(6):855–60. doi: 10.1007/s10875-010-9450-1
8. Dong W, Gong M, Shi Z, Xiao J, Zhang J, Peng J. Programmed Cell Death-1 Polymorphisms Decrease the Cancer Risk: A Meta-Analysis Involving Twelve Case-Control Studies. PLoS One (2016) 11(3):e0152448. doi: 10.1371/journal.pone.0152448
9. Ma Y, Liu X, Zhu J, Li W, Guo L, Han X, et al. Polymorphisms of Co-Inhibitory Molecules (Ctla-4/Pd-1/Pd-L1) and the Risk of non-Small Cell Lung Cancer in a Chinese Population. Int J Clin Exp Med (2015) 8(9):16585.
10. Bossart S, Thurneysen S, Rushing E, Frontzek K, Leske H, Mihic-Probst D, et al. Case Report: Encephalitis, With Brainstem Involvement, Following Checkpoint Inhibitor Therapy in Metastatic Melanoma. Oncologist (2017) 22(6):749–53. doi: 10.1634/theoncologist.2016-0366
11. Eisenhauer E, Therasse P, Bogaerts J, Schwartz L, Sargent D, Ford R, et al. New Response Evaluation Criteria in Solid Tumors: Revised Recist Guideline (Version 1.1). Eur J Cancer (2009) 45(2):228–47. doi: 10.1016/j.ejca.2008.10.026
12. Team RC. “A Language and Environment for Statistical Computing”. In: R Foundation for Statistical Computing, 2015. Vienna, Austria (2016).
13. Available at: http://grch37.ensembl.org.
14. Schachter J, Ribas A, Long GV, Arance A, Grob J-J, Mortier L, et al. Pembrolizumab Versus Ipilimumab for Advanced Melanoma: Final Overall Survival Results of a Multicentre, Randomised, Open-Label Phase 3 Study (Keynote-006). Lancet (2017) 390(10105):1853–62. doi: 10.1016/S0140-6736(17)31601-X
15. Wolchok JD, Chiarion-Sileni V, Gonzalez R, Rutkowski P, Grob J-J, Cowey CL, et al. Overall Survival With Combined Nivolumab and Ipilimumab in Advanced Melanoma. N Engl J Med (2017) 377(14):1345–56. doi: 10.1056/NEJMoa1709684
16. Wagner M, Jasek M, Karabon L. Immune Checkpoint Molecules-Inherited Variations as Markers for Cancer Risk. Front Immunol (2020) 11:606721. doi: 10.3389/fimmu.2020.606721
17. Chat V, Ferguson R, Simpson D, Kazlow E, Lax R, Moran U, et al. Autoimmune Genetic Risk Variants as Germline Biomarkers of Response to Melanoma Immune-Checkpoint Inhibition. Cancer Immunol Immunother (2019) 68(6):897–905. doi: 10.1007/s00262-019-02318-8
18. Queirolo P, Dozin B, Morabito A, Banelli B, Piccioli P, Fava C, et al. Association of CTLA-4 Gene Variants With Response to Therapy and Long-term Survival in Metastatic Melanoma Patients Treated With Ipilimumab: An Italian Melanoma Intergroup Study. Front Immunol (2017) 8:386. doi: 10.3389/fimmu.2017.00386
19. Nomizo T, Ozasa H, Tsuji T, Funazo T, Yasuda Y, Yoshida H, et al. Clinical Impact of Single Nucleotide Polymorphism in PD-L1 on Response to Nivolumab for Advanced non-Small-Cell Lung Cancer Patients. Sci Rep (2017) 7:45124. doi: 10.1038/srep45124
20. Bins S, Basak EA, El Bouazzaoui S, Koolen SLW, Oomen-de Hoop E, van der Leest CH, et al. Association Between Single-Nucleotide Polymorphisms and Adverse Events in Nivolumab-Treated non-Small Cell Lung Cancer Patients. Br J Cancer (2018) 118(10):1296–301. doi: 10.1038/s41416-018-0074-1
21. Prokunina L, Castillejo-López C, Öberg F, Gunnarsson I, Berg L, Magnusson V, et al. A Regulatory Polymorphism in PDCD1 is Associated With Susceptibility to Systemic Lupus Erythematosus in Humans. Nat Genet (2002) 32(4):666. doi: 10.1038/ng1020
22. Prokunina L, Padyukov L, Bennet A, De Faire U, Wiman B, Prince J, et al. Association of the PD-1.3 A Allele of the PDCD1 Gene in Patients With Rheumatoid Arthritis Negative for Rheumatoid Factor and the Shared Epitope. Arthritis Rheumatol (2004) 50(6):1770–3. doi: 10.1002/art.20280
23. Bertsias GK, Nakou M, Choulaki C, Raptopoulou A, Papadimitraki E, Goulielmos G, et al. Genetic, Immunologic, and Immunohistochemical Analysis of the Programmed Death 1/Programmed Death Ligand 1 Pathway in Human Systemic Lupus Erythematosus. Arthritis Rheumatol (2009) 60(1):207–18. doi: 10.1002/art.24227
24. Kristjansdottir H, Steinsson K, Gunnarsson I, Gröndal G, Erlendsson K, Alarcón-Riquelme ME. Lower Expression Levels of the Programmed Death 1 Receptor on CD4+ Cd25+ T Cells and Correlation With the PD-1.3 A Genotype in Patients With Systemic Lupus Erythematosus. Arthritis Rheumatol (2010) 62(6):1702–11. doi: 10.1002/art.27417
25. Kroner A, Mehling M, Hemmer B, Rieckmann P, Toyka KV, Mäurer M, et al. A PD-1 Polymorphism is Associated With Disease Progression in Multiple Sclerosis. Ann Neurol (2005) 58(1):50–7. doi: 10.1002/ana.20514
26. Ngiow SF, Young A, Jacquelot N, Yamazaki T, Enot D, Zitvogel L, et al. A Threshold Level of Intratumor Cd8+ T Cell PD1 Expression Dictates Therapeutic Response to Anti-PD1. Cancer Res (2015) canres:1082.2015. doi: 10.1158/0008-5472.CAN-15-1082
27. Zhang J, Zhao T, Xu C, Huang J, Yu H. The Association Between Polymorphisms in the PDCD1 Gene and the Risk of Cancer: A Prisma-Compliant Meta-Analysis. Medicine (2016) 95(40):e4423. doi: 10.1097/MD.0000000000004423
28. Hashemi M, Karami S, Sarabandi S, Moazeni-Roodi A, Malecki A, Ghavami S, et al. Association Between PD-1 and PD-L1 Polymorphisms and the Risk of Cancer: A Meta-Analysis of Case-Control Studies. Cancers (Basel) (2019) 11(8):1150. doi: 10.3390/cancers11081150
29. Karami S, Sattarifard H, Kiumarsi M, Sarabandi S, Taheri M, Hashemi M, et al. Evaluating the Possible Association Between Pd-1 (Rs11568821, Rs2227981, Rs2227982) and PD-L1 (Rs4143815, Rs2890658) Polymorphisms and Susceptibility to Breast Cancer in a Sample of Southeast Iranian Women. Asian Pac J Cancer Prev (2020) 21(10):3115–23. doi: 10.31557/APJCP.2020.21.10.3115
30. Consortium ITP-CAoWG. Pan-Cancer Analysis of Whole Genomes. Nature (2020) 578(7793):82–93. doi: 10.1038/s41586-020-1969-6
31. Kirchhoff T, Ferguson R. Germline Genetics in Immuno-oncology: From Genome-Wide to Targeted Biomarker Strategies. Methods Mol Biol (2020) 2055:93–117. doi: 10.1007/978-1-4939-9773-2_4
32. Robert C, Long GV, Brady B, Dutriaux C, Maio M, Mortier L, et al. Nivolumab in Previously Untreated Melanoma Without BRAF Mutation. N Engl J Med (2015) 372(4):320–30. doi: 10.1056/NEJMoa1412082
33. Ribas A, Hamid O, Daud A, Hodi FS, Wolchok JD, Kefford R, et al. Association of Pembrolizumab With Tumor Response and Survival Among Patients With Advanced Melanoma. JAMA (2016) 315(15):1600–9. doi: 10.1001/jama.2016.4059
34. Garon EB, Rizvi NA, Hui R, Leighl N, Balmanoukian AS, Eder JP, et al. Pembrolizumab for the Treatment of non–Small-Cell Lung Cancer. N Engl J Med (2015) 372(21):2018–28. doi: 10.1056/NEJMoa1501824
35. Chabanon RM, Pedrero M, Lefebvre C, Marabelle A, Soria J-C, Postel-Vinay S. Mutational Landscape and Sensitivity to Immune Checkpoint Blockers. Clin Cancer Res (2016) 22(17):4309–21. doi: 10.1158/1078-0432.CCR-16-0903
36. Madore J, Vilain RE, Menzies AM, Kakavand H, Wilmott JS, Hyman J, et al. Pd-L1 Expression in Melanoma Shows Marked Heterogeneity Within and Between Patients: Implications for Anti-PD-1/PD-L1 Clinical Trials. Pigment Cell Melanoma Res (2015) 28(3):245–53. doi: 10.1111/pcmr.12340
37. Patel SP, Kurzrock R. Pd-L1 Expression as a Predictive Biomarker in Cancer Immunotherapy. Mol Cancer Ther (2015) 14(4):847–56. doi: 10.1158/1535-7163.MCT-14-0983
38. Topalian SL, Taube JM, Anders RA, Pardoll DM. Mechanism-Driven Biomarkers to Guide Immune Checkpoint Blockade in Cancer Therapy. Nat Rev Cancer (2016) 16(5):275. doi: 10.1038/nrc.2016.36
39. Hugo W, Zaretsky JM, Sun L, Song C, Moreno BH, Hu-Lieskovan S, et al. Genomic and Transcriptomic Features of Response to Anti-PD-1 Therapy in Metastatic Melanoma. Cell (2016) 165(1):35–44. doi: 10.1016/j.cell.2016.02.065
40. Meng X, Huang Z, Teng F, Xing L, Yu J. Predictive Biomarkers in PD-1/PD-L1 Checkpoint Blockade Immunotherapy. Cancer Treat Rev (2015) 41(10):868–76. doi: 10.1016/j.ctrv.2015.11.001
41. Gopalakrishnan V, Spencer C, Reuben A, Karpinets T, Hutchinson D, Hoffman K, et al. Association of Diversity and Composition of the Gut Microbiome With Differential Responses to PD-1 Based Therapy in Patients With Metastatic Melanoma. 2017 ASCO-SITC Clinical Immuno-Oncology Symposium; Orlando, FL, United States. J Clin Oncol (2017) 35:2. doi: 10.1200/JCO.2017.35.7_suppl.2
Keywords: metastatic melanoma, PD1, polymorphism, predictive biomarker, immunotherapy
Citation: Parakh S, Musafer A, Paessler S, Witkowski T, Suen CSNLW, Tutuka CSA, Carlino MS, Menzies AM, Scolyer RA, Cebon J, Dobrovic A, Long GV, Klein O and Behren A (2021) PDCD1 Polymorphisms May Predict Response to Anti-PD-1 Blockade in Patients With Metastatic Melanoma. Front. Immunol. 12:672521. doi: 10.3389/fimmu.2021.672521
Received: 25 February 2021; Accepted: 21 May 2021;
Published: 09 June 2021.
Edited by:
Fernando Guimaraes, University of Queensland, AustraliaReviewed by:
Anna Vilgelm, The Ohio State University, United StatesJonas Nilsson, Harry Perkins Institute of Medical Research, Australia
Copyright © 2021 Parakh, Musafer, Paessler, Witkowski, Suen, Tutuka, Carlino, Menzies, Scolyer, Cebon, Dobrovic, Long, Klein and Behren. This is an open-access article distributed under the terms of the Creative Commons Attribution License (CC BY). The use, distribution or reproduction in other forums is permitted, provided the original author(s) and the copyright owner(s) are credited and that the original publication in this journal is cited, in accordance with accepted academic practice. No use, distribution or reproduction is permitted which does not comply with these terms.
*Correspondence: Sagun Parakh, sagun.parakh@onjcri.org.au
†These authors have contributed equally to this work