- 1Cellular and Molecular Pathology Laboratory, Faculty of Medicine and Pharmacy of Casablanca, Hassan II University, Casablanca, Morocco
- 2Team Immunity and Cancer, Centre de Recherche en Cancérologie de Marseille (CRCM), Inserm, 41068, CNRS, UMR7258, Institut Paoli-Calmettes, Aix-Marseille University, UM 105, Marseille, France
- 3Mohammed VI Center of Oncology, CHU Ibn Rochd, Faculty of Medicine and Pharmacy of Casablanca, Hassan II University, Casablanca, Morocco
Background: Glioma is the most common type of primary brain tumor in adults. Patients with the most malignant form have an overall survival time of <16 months. Although considerable progress has been made in defining the adapted therapeutic strategies, measures to counteract tumor escape have not kept pace, due to the developed resistance of malignant glioma. In fact, identifying the nature and role of distinct tumor-infiltrating immune cells in glioma patients would decipher potential mechanisms behind therapy failure.
Methods: We integrated into our study glioma transcriptomic datasets from the Cancer Genome Atlas (TCGA) cohort (154 GBM and 516 LGG patients). LM22 immune signature was built using CIBERSORT. Hierarchical clustering and UMAP dimensional reduction algorithms were applied to identify clusters among glioma patients either in an unsupervised or supervised way. Furthermore, differential gene expression (DGE) has been performed to unravel the top expressed genes among the identified clusters. Besides, we used the least absolute shrinkage and selection operator (LASSO) and Cox regression algorithm to set up the most valuable prognostic factor.
Results: Our study revealed, following gene enrichment analysis, the presence of two distinct groups of patients. The first group, defined as cluster 1, was characterized by the presence of immune cells known to exert efficient antitumoral immune response and was associated with better patient survival, whereas the second group, cluster 2, which exhibited a poor survival, was enriched with cells and molecules, known to set an immunosuppressive pro-tumoral microenvironment. Interestingly, we revealed that gene expression signatures were also consistent with each immune cluster function. A strong presence of activated NK cells was revealed in cluster 1. In contrast, potent immunosuppressive components such as regulatory T cells, neutrophils, and M0/M1/M2 macrophages were detected in cluster 2, where, in addition, inhibitory immune checkpoints, such as PD-1, CTLA-4, and TIM-3, were also significantly upregulated. Finally, Cox regression analysis further corroborated that tumor-infiltrating cells from cluster 2 exerted a significant impact on patient prognosis.
Conclusion: Our work brings to light the tight implication of immune components on glioma patient prognosis. This would contribute to potentially developing better immune-based therapeutic approaches.
Introduction
Glioma is the most common type of primary brain tumors in adults, with the most aggressive form known as glioblastoma. Glioma patients are known to be extremely resistant to chemotherapy. Indeed, such a trait is the leading cause of cancer-related mortality and morbidity (1, 2). Patients with glioma have a median overall survival of <16 months (3). The World Health Organization (WHO) classified gliomas into two groups, low-grade glioma (LGG) assembling grades I and II, and high-grade glioma (HGG) of grades III and IV (4, 5). Furthermore, based on histological and molecular features, glioma patients can be stratified into oligodendroglioma or astrocytoma (LGG) and glioblastoma (HGG) with clinically relevant molecular subtypes including mesenchymal, proneural, neural, and classical. Nevertheless, these features can be associated with tumor niche (stromal cells, reactive astrocytes, tumor cells, and immune cells), patient survival, and prognosis (6, 7).
Previous studies on glioma have provided an overview regarding tumor initiation and development (8, 9). As a matter of fact, a variety of chemoattractant and inflammatory cytokines secreted by either cancer or immune cells play a pivotal role in glioma tumor progression by attracting Treg cells into the tumor microenvironment, thus inhibiting NK cell activity and hampering the activation of effector T cells. Moreover, it leads to a plasticity phenomenon that leads to the conversion of tumor-associated macrophages (TAMs) from M1 state to an anti-inflammatory M2 phenotype and T cell transition from Th1 toward a Th2 phenotype which are often associated with poor outcome (6, 10–12). Hence, the cross talk between tumor and the immune cells results in the establishment of an immunosuppressive tumor microenvironment, which promotes tumor escape and cancer progression.
Over the past few years, several immunotherapeutic approaches have been developed (13); however, clinical trials testing vaccines, cell adoptive transfer, and immune checkpoint inhibitors (ICIs) point to a lack of efficacy (14–16).
This failure has shifted the focus toward a more thorough understanding of the distinct components of the glioma microenvironment (17–19). Glioblastomas are very low-immunogenicity tumors (16), enriched with immune-suppressive T cells mainly embedded in the central nervous system (20). As a matter of fact, future treatment strategies should be designed based on an improved understanding of key immune cell interactions with glioma cells.
Recently, computational analyses of RNA-seq data have demonstrated that a glioblastoma microenvironment lacking central memory CD4 T cells or natural killer (NK) cells is further correlated with better prognosis, and the expression levels of ICOS and TNFSF14 were negatively associated with clinical outcome (21). It has also been reported that the glioblastoma microenvironment contains hub genes (CCT3, OLIG2, PSMB9, TRIM21) that were associated with distinct immune cell infiltration characterized by the expression of immune checkpoints and are further involved in cancer development and progression in patients with high-grade glioblastoma (22–24). In low-grade glioma, the expression level of transforming growth factor beta 1 (TGF-β) and programmed cell death ligand 1 (PDL1) was positively correlated with immune risk score, and prognostic hub genes that were positively correlated with immune cell infiltration have also been revealed (25, 26). Notably, T follicular helper (TFH) cells, activated NK cells, and macrophages have been demonstrated to be independent predictors for malignant transformation in low-grade glioma (27). Furthermore, it has been suggested that the expressions of JAK3, IL2Rß, and CD3ε are further associated with the presence of B cell memory and CD8 T cells, which adversely impact immune cell response in the tumor microenvironment (28). As a matter of fact, Qiu et al. recently demonstrated, through their PROMISE model, differences in immune cell abundance between risk groups (29). Although innate immune cells such CD8 T cells were upregulated and further associated with good prognosis, a downregulation of TFH cells was observed in the high-risk group of glioma patients (29). Zhong et al. demonstrated that resting NK cells, CD8+ T cells, TFH cells, gamma delta T cells, and M0 macrophages were negatively related to prognosis. However, their proportion was significantly related to patient’s age and sex (30).
Nevertheless, these studies assessed low-grade glioma or glioblastoma patients individually and did not consider both subtypes as a whole to identify striking resemblance and active involvement of immune genes in glioma patients.
Here we assessed the presence of two distinct groups of glioma patients. The first group, defined as cluster 1, was associated with better patient survival, whereas the second group, cluster 2, exhibited a poor survival. Interestingly, a strong presence of activated NK cells was revealed in cluster 1. In contrast, potent immunosuppressive components such as regulatory T cells, neutrophils, and M0/M1/M2 macrophages were detected in cluster 2, where inhibitory immune checkpoints, such as cytotoxic T-lymphocyte-associated protein 4 (CTLA4), lymphocyte-activation gene 3 (LAG3), and T cell immunoglobulin mucin 3 (TIM3), were also significantly upregulated. Finally, Cox regression analysis further corroborated that tumor-infiltrating cells from cluster 2 exerted a significant impact on patient prognosis. Our results pinpoint that the immune response type impacts the clinical outcome of glioma patients.
Materials and Methods
Data Analysis From TCGA and Independent Datasets
The workflow of our study is shown in Figure 1. Molecular data, including mRNA expression of LGG (n = 516) and GBM (n = 154) patients, were downloaded from The Cancer Genome Atlas (TCGA) data portal (https://www.cbioportal.org/). Clinical data related to glioma patients were downloaded altogether with matrix mRNA gene expression.
Quantification of Immune Signature Enrichment Levels in Glioma Patients
We analyzed 22 immune signatures (e.g., CD8+ T cells, CD4 naive T cells, Treg cells, naïve B cells, memory B cells, plasma cells, CD4 memory resting T cells, CD4 memory activated T cells, follicular helper T cells, gamma delta T cells, resting NK cells, activated NK cells, monocytes, macrophages M0/M1/M2, resting dendritic cells, activated dendritic cells, resting mast cells, activated mast cells, eosinophils, neutrophils). The enrichment level of this immune signature in glioma samples was quantified as the mean expression level of immune signature marker genes. We, therefore, used CIBERSORT (https://cibersort.stanford.edu/index.php) which estimates the relative fraction of each cell type in the signature matrix, such that the sum of all fractions is equal to 1 for a given mixture. The absolute immune fraction score was estimated by the median expression level of all genes in the signature matrix divided by the median expression level of all genes in the mixture.
Hierarchical Clustering
To compare distinct immune cell infiltration genes in glioma patients, unsupervised hierarchical clustering was performed to group the 658 patients into two subgroups termed cluster 1 (n = 293) and cluster 2 (n = 365).
Global Gene Expression Analysis
Differential gene expression (DGE) analysis with RNA-seq data was performed using the R package.
An average raw read count for each gene >1 was applied to determine candidate genes that were reasonably expressed. The expression fold change (FC) denotes upregulation or downregulation according to the FC value. Subsequently, log FC, log CPM, p-value, and the corresponding false discovery rate (FDR) were all reported by the R package. FDR < 0.05, log CPM > 1, and |log FC| > 2 were set as inclusion criteria for DEG selection.
Association of Immune Signature Enrichment Levels With Various Molecular Features
R Programming Environment (version 3.2.5) and Bioconductor were used for preprocessing and data analysis. For data filtering and quality control, the following methods were used. The median rank scores were identified in the dataset, and quartile normalization was performed. Data were visualized as Uniform Manifold Approximation and Projection (UMAP) which engage different parameters such as the distance between points and number of neighbors for a better reduced dimensional representation. We identified the upregulated genes that were significantly associated with immune signature enrichment levels (ISELs) using the Mann–Whitney U test.
Gene-Set Enrichment Analysis
We used FunRich to identify the KEGG pathways that were significantly associated with the genes having an important expression correlation with the immune signature using a threshold of FDR < 0.05.
Survival Analysis
We compared the overall survival (OS) between clusters and in the glioma cohort. We used Kaplan–Meier survival curves to show the survival time differences and the log-rank test to assess the significance of survival time differences. Statistical analyses were carried out with GraphPad Prism 5.0 software (GraphPad Software, Inc., La Jolla, CA, USA). Single comparisons between two groups were performed with Student’s t-test.
Univariate and multivariate Cox regression analyses were used on tumor-infiltrating immune gene signature and clinical parameters, including IDH status and age in TCGA cohorts.
Cox regression analyses were used to evaluate the prognostic effect of the immune signature on glioma patient prognosis and were performed using the R Programming Environment (version 3.2.5) and Bioconductor. To evaluate the difference between groups, the hazard ratios HR ≥ 1 and p-value of ≤0.05 were considered significant.
Results
Independent Immunostimulatory and Immunosuppressive Profiles of Glioma Patients
To identify the role of distinct tumor-infiltrating immune cells in glioma patients, a transcriptomic dataset from the Cancer Genome Atlas (TCGA) cohort (153 GBM and 512 LGG patients) was analyzed in this study. A pretreatment step of glioma transcriptomic data was carried out by emerging LGG and HGG databases, followed by a harmonization step. The unsupervised hierarchical clustering after the enrichment analysis enabled us to demonstrate the presence of two distinct clusters (groups of patients). The first group defined as cluster 1 was characterized by an immunostimulatory profile gathering monocytes, naïve B cells, plasma cells, naïve CD4+ T cells, activated mast cells, NK cells, and T follicular helper cells, whereas the second group (cluster 2) was enriched with immunosuppressive immune cells such as neutrophils, M0/M1/M2 macrophages, regulatory T cells (Tregs), resting mast cells, resting NK cells, resting CD4 memory T cells, and memory B cells (Figure 2A).
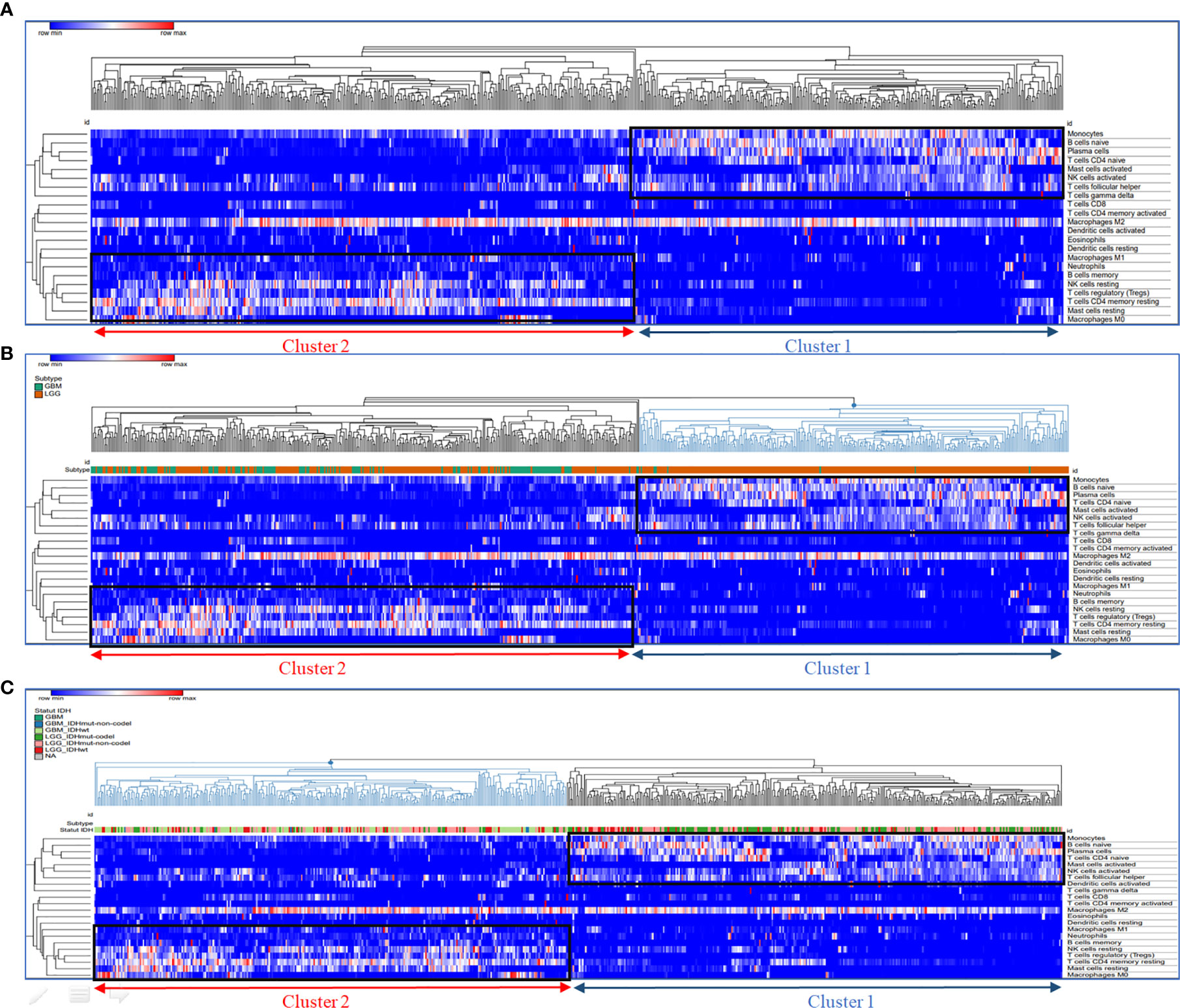
Figure 2 Segregation of enriched immune cell subsets by unsupervised hierarchical clustering. Hierarchical clustering of immune cell frequency in glioblastoma samples. RNA sequencing (RNA-Seq) gene expression data was analyzed with CIBERSORT to quantify the amount of different immune cells in the TCGA glioblastoma samples. Hierarchical clustering of these quantities after normalization is shown in the heat map. (A) Hierarchical clustering of immune cell frequency in glioblastoma samples, (B) Glioma Subtype groups (GBM and LGG) localisation in cluster 1 and 2; (C) Glioma IDH mutation status localisation in cluster 1 and 2. The cluster of samples enriched in immunosuppressive immune cells are represented in cluster 2 (red); the cluster of samples enriched in activated immune cells are represented in cluster 1 (blue). Hierarchical clustering was performed using Euclidean distance and complete linkage methods.
We further integrated clinicopathological parameters such as IDH status and glioma subtypes (LGG and GBM) to this hierarchical clustering, to assess whether the two clusters were correlated with these parameters. As shown in the hit map (Figures 2B, C), cluster 1 contains mainly LGG patients with only six GBM patients inside. However, the majority of GBM patients were grouped in (cluster 2). Moreover, a greater part of LGG patients with IDH mutant and 1p19q codeletion (codel) were gathering in cluster 1. Further, LGG patients with IDH wild-type or IDH mutant with 1p19q non-codeletion (non-codel) were likewise distributed within two clusters.
In order to confirm these results, we used high-dimension reduction algorithm that is based on manifold learning and nonlinear dimensionality reduction, used here as an effective preprocessing step to boost the performance of clustering. These results were confirmed either by increasing the distance between points (near neighbors) or by adding UMAP parameters (Canberra, Cosine, Dice) that control the balance of local versus global structure in our data. Indeed, we could observe the same clusters, which clearly suggest that this clustering depends on patients’ immune profiles rather than clinicopathological parameters (Figures S1A, B and Figures S2A, B).
Difference in Immune Cell Abundance Within the Clustered Patients
To confirm that unsupervised clustering could mostly be driven by the immune enrichment profile in patients with gliomas associated with the tumor microenvironment, we used this time UMAP to score the intensity of each infiltrated immune cell population within the clustered patients (Figure 3). As a matter of fact, we observed more naive B cells, plasma cells, naive CD4 T cells, TFH cells, monocytes, activated mast cells, and activated NK cells in cluster 1 than in cluster 2. When compared to cluster 1, cluster 2 seems to gather more less memory B cells, CD4 memory resting T cells, CD4 memory activated T cells, regulatory T cells, resting NK cells, M0/M1/M2 macrophages, resting dendritic cells, resting mast cells, and neutrophils. According to the Wilcoxon test, there were statistically significant differences in the immune cell subtypes between the two clusters (p < 0.05; FC > 2) (Figure 4), which confirm the immunosuppressive trait of cluster 2 relative to cluster 1. Indeed, these results highlight the weight of the immune profile on patients.
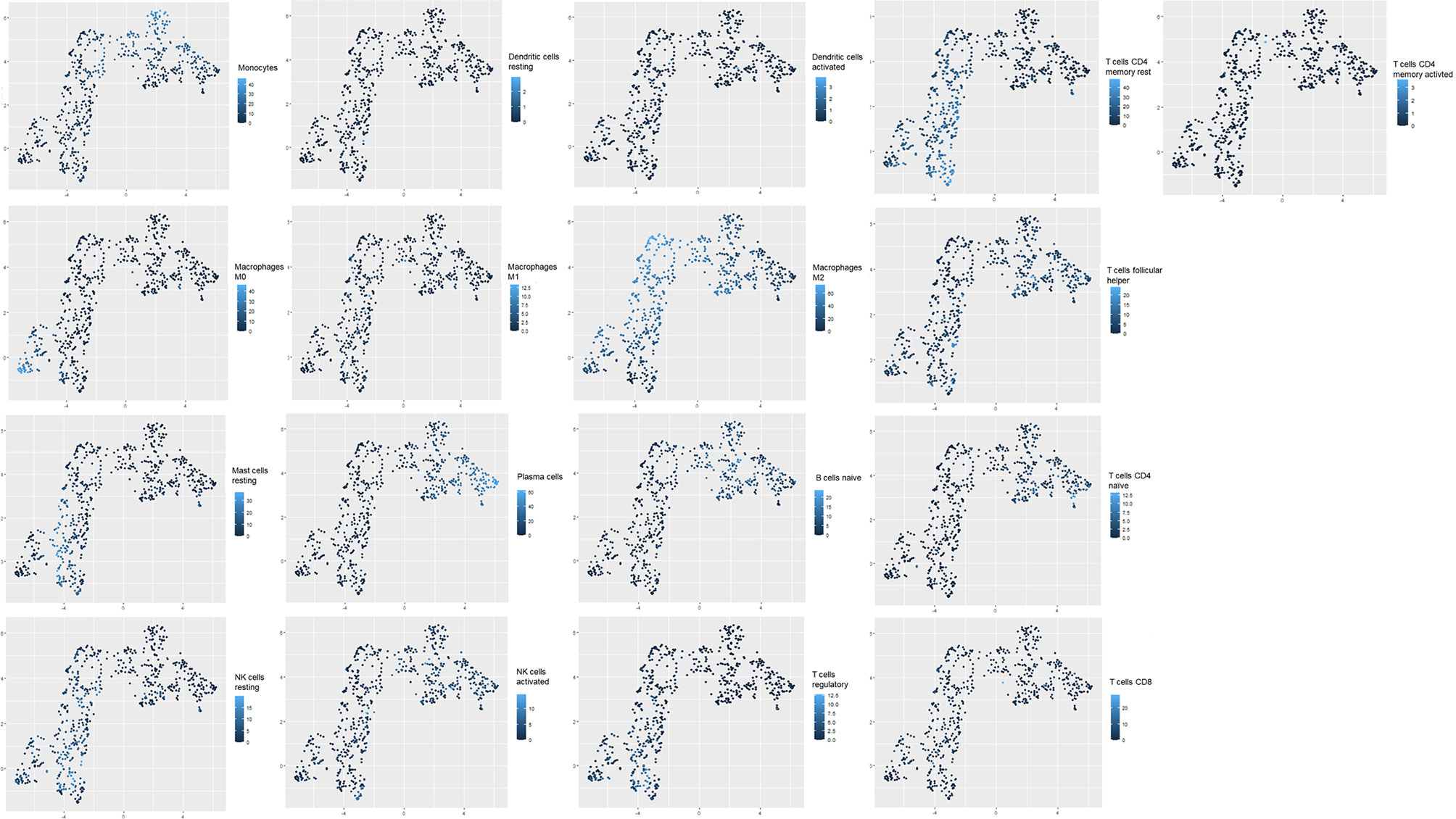
Figure 3 Uniform Manifold Approximation and Projection (UMAP) plots of transcriptomic profiles from glioblastoma datasets. Clustering of tumor-infiltrating immune cell populations (T regulatory cells, dendritic cells, macrophages M0, M1, and M2, CD8 T cells, activated CD4 T cells, activated NK cells, resting NK cells, B cells, T follicular helper cells); intensity of immune cell frequency within the clustered patients (cluster 1 and cluster 2). Light blue reflects a high enrichment intensity, and dark blue is associated with low infiltration intensity.
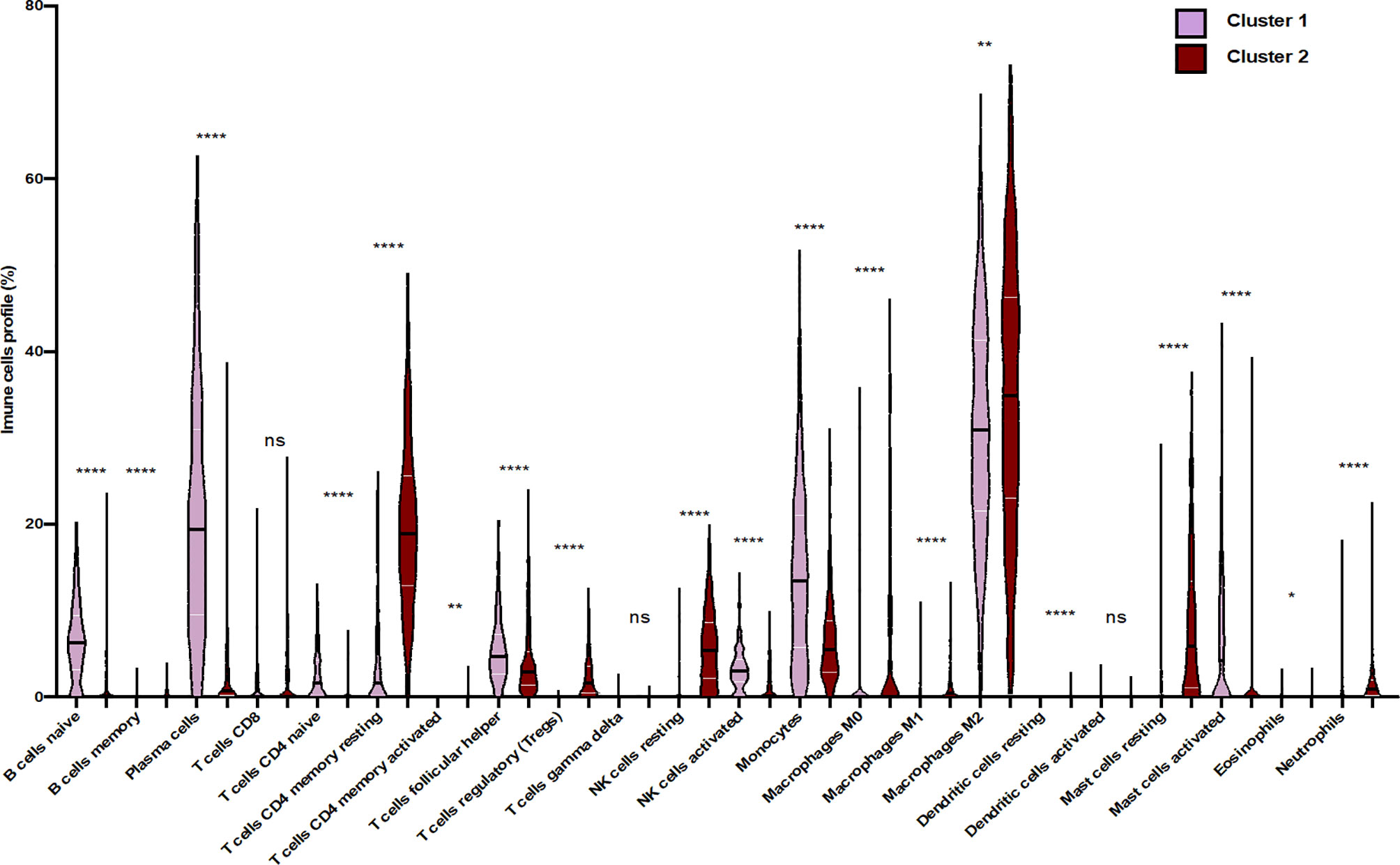
Figure 4 Differential distribution of immune cell proportion within the two clusters of glioma patients. Violin plots present the distribution of each immune subset. Violin plots show median values (black horizontal lines) in glioblastoma data. The Wilcoxon test shows statistically significant differences in the immune cell subtypes between the two clusters (*p < 0.05, **p < 0.01, ****p < 0.0001, ns, statistically no significant).
Association of Immune Checkpoints Overexpression With Immunosuppressive Status
Considering the critical role of the immune checkpoint inhibitors such as programmed-death receptor-1 (PD-1) and cytotoxic T lymphocyte antigen-4 (CTLA-4), not only in the negative regulation of T-cell activity but also in modulating T-cell migration into tissues (31), we aimed to compare the expression level of immune checkpoints between cluster 1 and cluster 2. As illustrated in Figure 4, PDL-1, CTLA-4, TIM-3, LAG-3, band T lymphocyte-associated (BTLA), inducible T cell costimulator (ICOS), inducible T cell costimulator ligand (ICOSL), and GATA-binding protein 3 (GATA-3) expressions were significantly higher in cluster 2 than in cluster 1 (p value <0.05; FC > 2).
Furthermore, a significant correlation between the expressions of PD-1, BTLA, and ICOS has been observed. In addition, our results showed that infiltration of immune cells such as CD8 T cells was associated with the expression of GATA3. Activated CD4 memory T cells and macrophages (M1/M2) were importantly correlated with expressions of BTLA and ICOS [r (-1 to +1), p<0.05]. Moreover, regulatory T cells were highly correlated with CD4 memory T cells, resting NK cells, macrophages (M0), and resting mast cells. Likewise, activated NK cells were associated with plasma cells, B cells naïve, follicular helper T cells, eosinophils, and activated mast cells (Figure 5 and Figure S3).
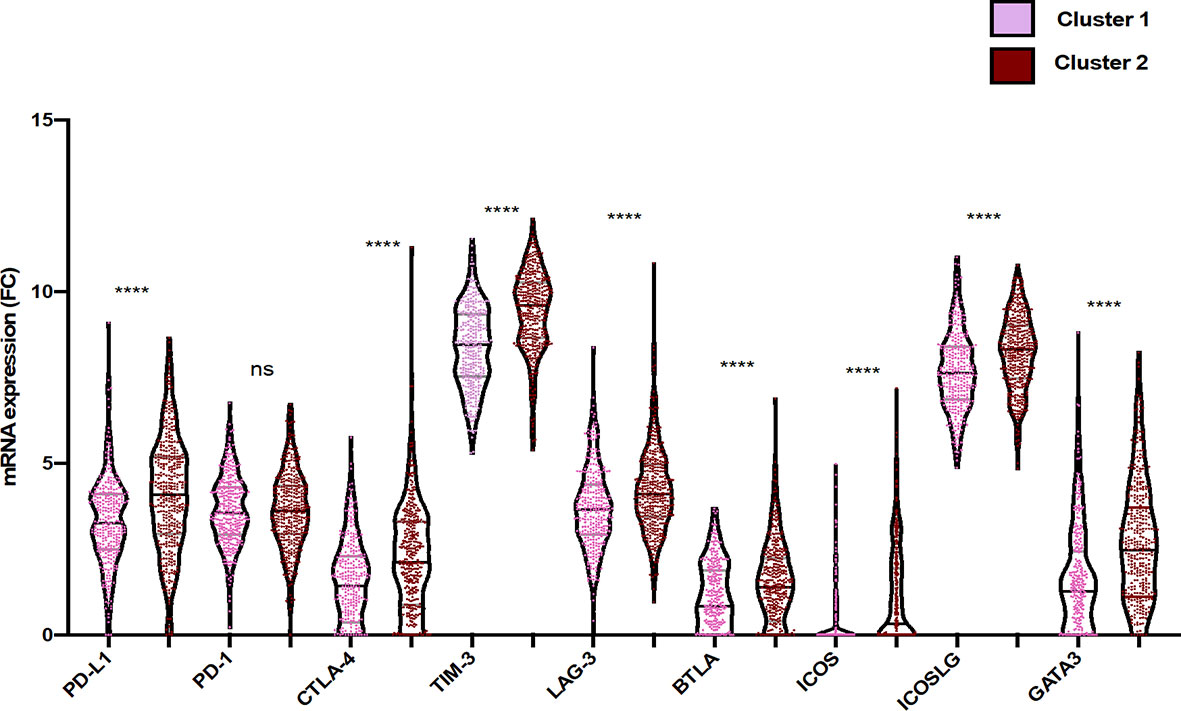
Figure 5 Differential gene expression of immune checkpoint within the two clusters of glioma patients. Violin plots represent the relative mRNA gene expression in the two clusters (pink: cluster 1; red: cluster 2). Each dot represents a sample. Violin plots show median values (black horizontal lines) in glioblastoma data. The two-tailed independent test was applied. p values of differences between groups are shown above (p < 0.05, ****p < 0.0001, ns, statistically no significant).
Our results suggest that NK cells, plasma cells, naïve B cells, follicular helper T cells, eosinophils, and activated mast cells cooperatively exhibit a balance of inhibitory and activating signals in cluster 1 where the cells expressed fewer immune checkpoints.
Relevant Activator and Inhibitor Markers to Guide Characterization of Glioma Clusters
To further deepen our investigation on the identified clusters, we performed differential expression gene (DEG) analysis based on 10,000-gene fold change expression within each cluster. As a matter of fact, we used as a fold change cutoff FC > 0.5 and FC < -0.5; all downregulated genes and upregulated genes are shown in the volcano plot (Figure 6).
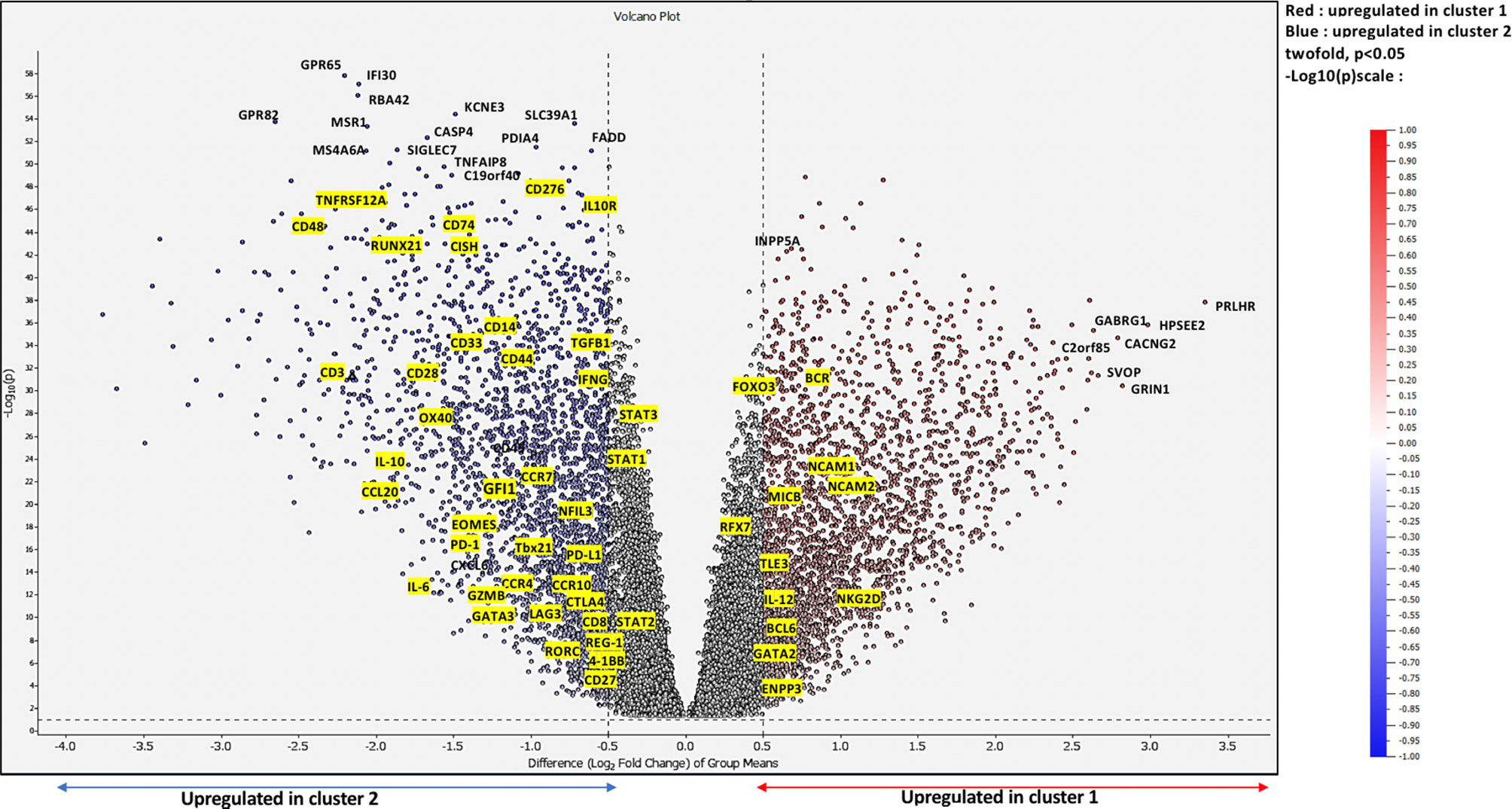
Figure 6 Differentially expressed genes at a Bonferroni-corrected p-value. Volcano plot showing upregulated genes in cluster 1 shown in red vs. upregulated genes in cluster 2 shown in blue while non-DE genes are in black.
Our results demonstrated that, on the one hand, genes such as neural cell adhesion molecule 1 (NCAM1), neural cell adhesion molecule 2 (NCAM2), MHC class I polypeptide-related sequence B (MICB), killer cell lectin-like receptor K1 (NKG2D), and interleukin 12 (IL-12) described as markers of activation were exclusively upregulated in cluster 1. On the other hand, the expressions of genes such as TGFβ, IL-10, interleukin 6 (IL-6), GATA3, regenerating family member 1 (REG-1), RAR related orphan receptor C (RORC), LAG3, CTLA4, cluster of differentiation 276 (CD276), cytokine-inducible SH2-containing protein (CISH), and cluster differentiation (CD44), further associated with the inhibition of T cell effector activity, were upregulated in cluster 2, where immunosuppressive populations are strongly enriched, as we previously demonstrated. Indeed, we could clearly observe the consistent link between the upregulated genes in each immune cluster component.
Immune Cell-Dependent Prognostic Drivers of Glioma Patients’ Survival
To assess whether the difference in immune components and other upregulated genes within cluster 1 and cluster 2 would reliably affect patient prognosis, we performed Kaplan–Meier (K-M) analysis between the two clusters. As a matter of fact, cluster 2 was strikingly associated with poor survival (at 50% of overall survival (OS), the survival median was at roughly 25 months) (p < 0.0001), which fits our results (Figure 7).
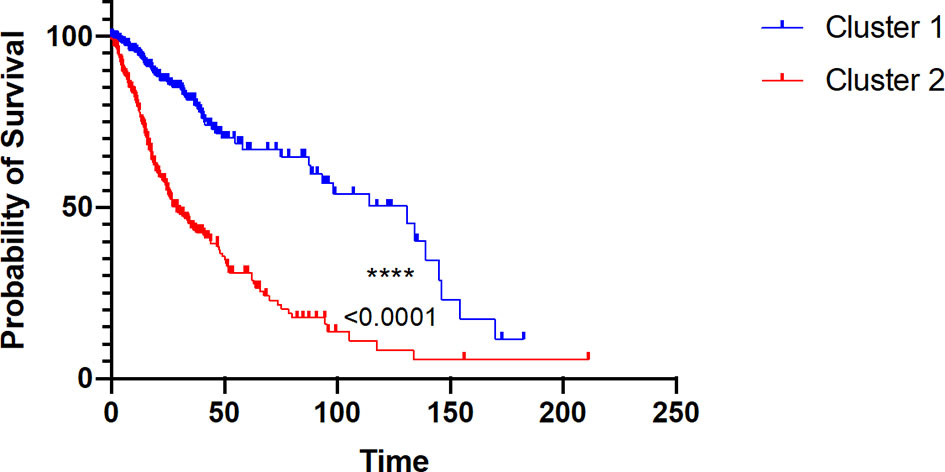
Figure 7 Kaplan–Meier survival probability of the two clusters of glioma patients. Red curve represents overall survival (OS) of cluster 2 patients. Blue curve represents overall survival (OS) of cluster 1 patients, with p value < 0.0001.
Then, we conducted a univariate Cox regression analysis based on clinical features and tumor-infiltrating immune cell parameters of cluster 2 patients for assessing prognostic factors that independently influence glioma patient survival (Table 1).
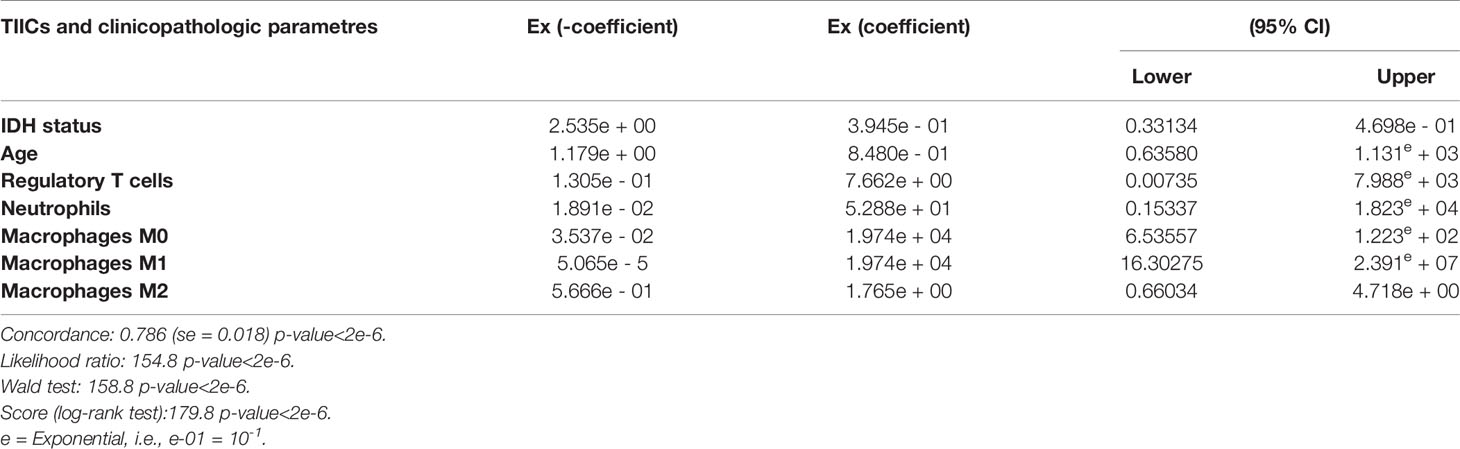
Table 1 Multivariate analysis of the mean tumor-infiltrating immune cells in cluster 2 and glioma clinicopathologic features (IDH status, age).
However, we have demonstrated by multivariate Cox regression analysis that regulatory T cells (Treg), neutrophils, and macrophages M0/M1/M2 are key drivers of cluster 2 patient survival, since the Ex coefficients were respectively 7.662e+00, 5.288e+01, 1.974e+04, 1.974e+04, and 1.765e+00; p < 2 e-6; and hazard ratio (HR) ≥ 1 (Table 1). These results might shed light on the importance of immune infiltrate when establishing patient diagnosis, a key feature that might influence glioma patient overall survival.
Discussion
The tumor microenvironment plays an important role in glioma pathogenesis. Here, we provided the immune signature, which might impact glioma patient prognosis. Using LM22 CIBERSORT, followed by unsupervised hierarchical clustering and UMAP dimensional reduction algorithms, we identified two clusters in glioma patients. These clusters were associated with immune infiltration character which is basically related to some specific expressed genes inside the tumor microenvironment and further associated with either good or poor prognosis. Thus, even though clinico-pathological parameters were added to the unsupervised clustering, those two groups of patients were identified independently of other prognostic parameters such as IDH status and glioma subtype. Then, we employed different methods to confirm our unsupervised clustering. Indeed, our data highlight the strength of the defined immune cells shown in terms of hierarchically clustering our patients.
As a matter of fact, we found that cluster 1 was highly correlated with naive B cell, plasma cell, naive CD4 T cell, T follicular helper cell, NK cell, and monocyte infiltration. It has been previously reported that B cell infiltration in melanoma, breast, and lung cancers was positively correlated with a better survival (32, 33). Indeed, naive B cells and plasma cells can enhance an efficient immune response by activating cytotoxic T cell response (34). Furthermore, B cells have been described as a central player in the response to immune checkpoint blockade in patients with metastatic melanoma and renal cell carcinoma, through the activation of T cells and the generation of IgG subclasses of antibodies (35–37). Moreover, combining radiation, PD-L1 inhibition, and B cell-based vaccine that consists of 4-1BBL+B cell activation promoted glioma cell regression by 80% in mice (38).
Besides, the presence of cytotoxic immune cells such as NK cells is associated with a good prognosis in glioma patients (39). Considering the role of NK cells in glioma immunosurveillance, many clinical trials focus on CAR-NK cells for the treatment of glioblastoma patients (40, 41).
Naïve CD4 T cells can proliferate and differentiate into an effector ThCTL subpopulation which contributes to an effective antitumor response through antigen recognition of peptides presented by MHC class II molecules following CTLA4 blockade (42). Yet, it has been reported that naïve T cells are missing in the glioblastoma microenvironment due to their sequestration in the bone marrow (43). Our data consolidate the thought that cluster 1 is enriched by antitumoral cell subsets with immunostimulatory characteristics.
Our results not only showed that cluster 2 was poorly enriched in activated and cytotoxic T cells but also underlined the high prevalence of M0 and M1 macrophages and neutrophils. Moreover, Treg cells and M2 macrophages were highly enriched in this group of glioma patients. Moreover, Treg accumulation in the tumor microenvironment was associated with antitumor T cell repression (44), poor prognosis (45), and resistance to vascular endothelial growth factor (VEGF) therapy in glioblastoma (46). The predominance of M2 macrophages in the glioma microenvironment has been described to be associated with poor survival and resistance to radiotherapy, whereas M1 macrophages have been reported to be less abundant in high-grade glioma and expression of M1 marker C–C motif chemokine ligand 3 (CCL3) was further associated with a good survival (47, 48). Moreover, clinical data suggest that neutrophils were associated with poor prognosis and tumor progression in glioma patients (49, 50). Altogether, these studies and our results come to strengthen the hypothesis that the immune infiltrate components should be taken into consideration when establishing patients’ diagnosis. These data show that cluster 2 is infiltrated by pro-tumoral cells with immunosuppressive properties.
According to the results of patients responding to anti-PD-1/anti-PD-L1 immunotherapy, Mellman and colleagues described two categories of microenvironment immune profile. The first is “immune inflamed tumor” that exhibits a preexisting antitumor immune response and provides a more favorable environment for T cell activation and renewal. The second profile is named “non-inflamed tumors” where cells are in a pausing state either in the parenchyma or in the tumor stroma and release more immunosuppressive cytokines (51). Our observations demonstrated that the immune components of cluster 1 patients presented similar features to “immune inflamed tumor” such as the abundance of IL-12, MICB, NCAM1, NCAM2, and NKG2D while the second group (cluster 2) seemed to exhibit some of the “non-inflamed tumors” characteristics such as the expressions of TIM3, PDL1, IL-10, and TGF-β.
Differential expression genes revealed the top genes that are, according to the literature, distinctively associated with an antitumor immune response in cluster 1 (MICB, NKG2D, NCAM1, NCAM2, and IL-12). As a matter of fact, MICB overexpression by tumor cells activates cytotoxic lymphocyte functions such as lysis and elimination of cancer cells through NKG2D receptor activation (52, 53). Furthermore, NKG2D receptor and NCAM upregulation triggers cytotoxic effector functions and provides costimulatory signals for NK cells, CD8 T cells, and ɣϨT cells (54–56). Moreover, IL-12, which plays a key role in changing tumor microenvironment composition from one that contains less differentiated Th0 cells to one that has more inflammatory Th1 cells, was also upregulated (57). Besides, TLE3 upregulation may also induce cell cycle arrest and tumor growth suppression via inhibition of MAPK and AKT pathways (58, 59). On the other hand, GATA2 upregulation induces cell surface PDL-1 and PDL-2 expression in brain tumors, and its inhibition has been reported to stimulate chemotherapy-mediated apoptosis in human AML cells overexpressing GATA2 (60, 61). Further, BCL6 overexpression has pro-survival and proliferative activities of glioma cells; its expression in monocytes/macrophage lineage reduces the antitumor immune response and increases the immunosuppressive microenvironment. It has been demonstrated recently that BCL6 deletion in Treg cells significantly inhibited tumor progression (62–64).
Most interestingly, upregulated genes in cluster 2 (TGFβ, IL-10, IL-6, GATA3, REG-1, RORC, LAG3, CTLA4, CD276, CISH, and CD44) were related to an activated regulatory T-cell phenotype. Indeed, transcription factors such as GATA3 and RORC are further related to the differentiation of CD4 T cells into Th17 that plays a pivotal role in regulatory T cell generation and recruitment (61–64). TGF-β and IL-10 are anti-inflammatory, which, produced by many cell types, play a crucial role in inhibiting the antitumor immune response in glioma (65–67) by activating the polarization of M0 into M1 or M2 macrophages (68–70). Strikingly, CD276 and nuclear factor interleukin 3-regulated (NFIL3) overexpression is capable of inducing T cell immunosuppression (71, 72). Moreover, the co-expression of PD-1 with other inhibitory receptors, such as PDL-1, TIM-3, LAG3, and CTLA-4, induces effector T cell exhaustion (73, 74). It has also been reported that the PD-1/PDL-1 pathway is significantly correlated with the most aggressive histological subtype of glioma (75, 76). This could reflect the importance of the complex mechanisms established by the tumor microenvironment to suppress the antitumor immune response.
Our survival results highlight the importance of immune parameters in the outcomes of glioma patients and could further be considered as the most suitable predictive feature when adopting new treatment strategies. So far, clinicopathological and genetic features could not be used as predictive parameters in glioma patients’ survival. In fact, glioma patients who have been grouped based on similar clinical characteristics could still present contradictory outcomes (25, 77).
Cox regression results indicated that macrophages M0/M1/M2, neutrophils, and Treg cells might have a detrimental impact in cluster 2 patients’ survival. Our findings shed light on the importance of immune infiltration on glioma patients’ survival. Differential expression of the immune checkpoint inhibitors between cluster 1 and cluster 2 might occur due the presence of macrophages and Treg cells. Indeed, cluster 2 exhibited a high expression of immune checkpoints and was mainly enriched with macrophages M0/M1/M2, neutrophils, and regulatory T cells. In fact, these cells could be involved in the upregulation of checkpoint expression and thus the inhibition of cytotoxic T cell activities (cluster 2). Therefore, understanding the signaling pathways of these cell subsets and their interaction with immune checkpoints would provide more information to define best-suited treatment strategies for glioma patients.
Over the past decade, it has been strongly believed that glioma patients exhibit resistance to adjuvant therapy. As a matter of fact, several resistance mechanisms limit the efficiency of classical treatments (78). Moreover, glioblastoma bears characteristics that contribute to significant therapeutic resistance by preventing adequate control of the entire tumor mass by drugs. Glioblastoma therefore facilitates escape mechanisms by mediating blood–brain barrier junction proteins dysfunction (79). Understanding the different interactions that occur inside the tumor microenvironment is crucial to conceive reliable therapeutic approaches.
Our findings shed light on the importance of using some immune markers to further stratify high- and low-risk glioma patients in order to suggest the adequate treatment for each group. As a matter of fact, Treg and NK cell infiltration rates found within cluster 2 in our study further indicate that this group would probably respond to NK activation treatment or to Treg cell targeting.
Our study comes with important findings which should be further corroborated. As a matter of fact, we have used CIBERSORT to identify critical immune infiltration subtypes. However, given that LM22 involves a limited set of genes and that we still lack evidence for a direct role of each cell subtype within glioma TME, a deepened characterization of immune infiltrates in glioblastoma might be necessary to further strengthen our findings.
Conclusion
Our work brings to light the tight implication of immune components on glioma patient prognosis. Moreover, characterizing immune subsets in the tumor microenvironment could also provide predictive factors that would contribute to potentially developing better immune-based therapeutic approaches.
Data Availability Statement
The raw data supporting the conclusions of this article will be made available by the authors, without undue reservation.
Author Contributions
SA analyzed the data and wrote the manuscript. DC analyzed the data and wrote and revised the manuscript. KA analyzed the data and revised the manuscript. SS and DO contributed to the conception and the design of the study. AB analyzed the data, wrote and revised the manuscript, and supervised the study. All authors contributed to the article and approved the submitted version.
Funding
This work was supported by the Moroccan Ministry of Higher Education and Research and The National Center for Scientific and Technical Research (CNRST) through “PPR1” and Al-khawarizmi grants coordinated by AB.
Conflict of Interest
The authors declare that the research was conducted in the absence of any commercial or financial relationships that could be construed as a potential conflict of interest.
Publisher’s Note
All claims expressed in this article are solely those of the authors and do not necessarily represent those of their affiliated organizations, or those of the publisher, the editors and the reviewers. Any product that may be evaluated in this article, or claim that may be made by its manufacturer, is not guaranteed or endorsed by the publisher.
Supplementary Material
The Supplementary Material for this article can be found online at: https://www.frontiersin.org/articles/10.3389/fimmu.2021.685213/full#supplementary-material
Supplementary Figure 1 | Clustering according to nearest neighbor. (A, B) distance between point based on IDH status and glioma subtype.
Supplementary Figure 2 | Clustering according to Umap parameters. Correlation clustering based on similarity coefficients.
Supplementary Figure 3 | Correlation matrix of 22 immune subsets and 7 immune checkpoints in Glioma patients. The numbers in the scale represent pearson correlation coefficient. Red cycle represents a negative correlation, blue cycle represents a positive correlation, and white boxes represent no correlation between two kinds of cells or checkpoint inhibitors.
References
1. Rabé M, Dumont S, Álvarez-Arenas A, Janati H, Belmonte-Beitia J, Calvo GF, et al. Identification of a Transient State During the Acquisition of Temozolomide Resistance in Glioblastoma. Cell Death Dis (2020) 11:1–14. doi: 10.1038/s41419-019-2200-2
2. Chen R, Smith-Cohn M, Cohen AL, Colman H. Glioma Subclassifications and Their Clinical Significance. Neurotherapeutics (2017) 14:284–97. doi: 10.1007/s13311-017-0519-x
3. Walid M. Prognostic Factors for Long-Term Survival After Glioblastoma. Permanente J (2008) 12:45. doi: 10.7812/tpp/08-027
4. Masui K, Mischel PS, Reifenberger G. Molecular Classification of Gliomas. In: Handbook of Clinical Neurology. Elsevier B.V. (2016). p. 97–120. doi: 10.1016/B978-0-12-802997-8.00006-2
5. Louis DN, Perry A, Reifenberger G, von Deimling A, Figarella-Branger D, Cavenee WK, et al. The 2016 World Health Organization Classification of Tumors of the Central Nervous System: A Summary. Acta Neuropathol (2016) 131:803–20. doi: 10.1007/s00401-016-1545-1
6. Al-kharboosh R, ReFaey K, Lara-Velazquez M, Grewal SS, Imitola J, Quiñones-Hinojosa A. Inflammatory Mediators in Glioma Microenvironment Play a Dual Role in Gliomagenesis and Mesenchymal Stem Cell Homing: Implication for Cellular Therapy. Mayo Clinic Proc: Innovations Qual Outcomes (2020) 4:443–59. doi: 10.1016/j.mayocpiqo.2020.04.006
7. Buerki RA, Chheda ZS, Okada H. Immunotherapy of Primary Brain Tumors: Facts and Hopes. Clin Cancer Res (2018) 24:5198–205. doi: 10.1158/1078-0432.CCR-17-2769
8. Bastola S, Pavlyukov MS, Yamashita D, Ghosh S, Cho H, Kagaya N, et al. Glioma-Initiating Cells at Tumor Edge Gain Signals From Tumor Core Cells to Promote Their Malignancy. Nat Commun (2020) 11:1–17. doi: 10.1038/s41467-020-18189-y
9. Azzarelli R, Simons BD, Philpott A. The Developmental Origin of Brain Tumours: A Cellular and Molecular Framework. Dev (Cambridge) (2018) 145:1–9. doi: 10.1242/dev.162693
10. Gieryng A, Pszczolkowska D, Walentynowicz KA, Rajan WD, Kaminska B. Immune Microenvironment of Gliomas. Lab Invest (2017) 97:498–518. doi: 10.1038/labinvest.2017.19
11. Clavreul A, Menei P. Mesenchymal Stromal-Like Cells in the Glioma Microenvironment: What Are These Cells? Cancers (2020) 12:1–12. doi: 10.3390/cancers12092628
12. Shimato S, Maier LM, Maier R, Bruce JN, Anderson RCE, Anderson DE. Profound Tumor-Specific Th2 Bias in Patients With Malignant Glioma. BMC Cancer (2012) 12:561. doi: 10.1186/1471-2407-12-561
13. Hanaei S, Afshari K, Hirbod-Mobarakeh A, Mohajer B, Amir Dastmalchi D, Rezaei N. Therapeutic Efficacy of Specific Immunotherapy for Glioma: A Systematic Review and Meta-Analysis. Rev Neurosci (2018) 29:443–61. doi: 10.1515/revneuro-2017-0057
14. Yan Y, Zeng S, Gong Z, Xu Z. Clinical Implication of Cellular Vaccine in Glioma: Current Advances and Future Prospects. J Exp Clin Cancer Res (2020) 39:1–18. doi: 10.1186/s13046-020-01778-6
15. Goff SL, Morgan RA, Yang JC, Sherry RM, Robbins PF, Restifo NP, et al. Pilot Trial of Adoptive Transfer of Chimeric Antigen Receptor-Transduced T Cells Targeting Egfrviii in Patients With Glioblastoma. J Immunother (2019) 42:126–35. doi: 10.1097/CJI.0000000000000260
16. Weenink B, French PJ, Smitt PAES, Debets R, Geurts M. Immunotherapy in Glioblastoma: Current Shortcomings and Future Perspectives. Cancers (2020) 12:3–751. doi: 10.3390/cancers12030751
17. Montoya ML, Kasahara N, Okada H. Introduction to Immunotherapy for Brain Tumor Patients: Challenges and Future Perspectives. Neuro-Oncol Pract (2020) 7:465–76. doi: 10.1093/nop/npaa007
18. Khamis ZI, Al-Akkary N, Hua T, Draughon SA, Li Y, Sang Q-XA. Clinical Investigations of Immunotherapy for Human Primary Brain Tumors. Neuroimmunol Neuroinflamm (2020) 2020:1–14. doi: 10.20517/2347-8659.2020.43
19. Sampson JH, Gunn MD, Fecci PE, Ashley DM. Brain Immunology and Immunotherapy in Brain Tumours. Nat Rev Cancer (2020) 20:12–25. doi: 10.1038/s41568-019-0224-7
20. Desland FA, Hormigo A. The CNS and the Brain Tumor Microenvironment: Implications for Glioblastoma Immunotherapy. Int J Mol Sci (2020) 21:1–19. doi: 10.3390/ijms21197358
21. Wu S, Yang W, Zhang H, Ren Y, Fang Z, Yuan C, et al. The Prognostic Landscape of Tumor-Infiltrating Immune Cells and Immune Checkpoints in Glioblastoma. Technol Cancer Res Treat (2019) 18:1–10. doi: 10.1177/1533033819869949
22. Huang S, Song Z, Zhang T, He X, Huang K, Zhang Q, et al. Identification of Immune Cell Infiltration and Immune-Related Genes in the Tumor Microenvironment of Glioblastomas. Front Immunol (2020) 11:585034. doi: 10.3389/fimmu.2020.585034
23. Tang G, Yin W. Development of an Immune Infiltration-Related Prognostic Scoring System Based on the Genomic Landscape Analysis of Glioblastoma Multiforme. Front Oncol (2020) 10:154. doi: 10.3389/fonc.2020.00154
24. Hu L, Han Z, Cheng X, Wang S, Feng Y, Lin Z. Expression Profile Analysis Identifies a Novel Seven Immune-Related Gene Signature to Improve Prognosis Prediction of Glioblastoma. Front Genet (2021) 12:638458. doi: 10.3389/fgene.2021.638458
25. Song LR, Weng JC, Li CB, Huo XL, Li H, Hao SY, et al. Prognostic and Predictive Value of an Immune Infiltration Signature in Diffuse Lower-Grade Gliomas. JCI Insight (2020) 5:1–8. doi: 10.1172/jci.insight.133811
26. Su J, Long W, Ma Q, Xiao K, Li Y, Xiao Q, et al. Identification of a Tumor Microenvironment-Related Eight-Gene Signature for Predicting Prognosis in Lower-Grade Gliomas. Front Genet (2019) 10:1143. doi: 10.3389/fgene.2019.01143
27. Lu J, Li H, Chen Z, Fan L, Feng S, Cai X, et al. Identification of 3 Subpopulations of Tumor-Infiltrating Immune Cells for Malignant Transformation of Low-Grade Glioma. Cancer Cell Int (2019) 19:1–13. doi: 10.1186/s12935-019-0972-1
28. Zhang B, Guan F, Dai B, Zhu G, Mao B, Liang X, et al. Prognostic Value and Immune Infiltration of Novel Biomarkers in Glioma: Evidence From a Comprehensive Analysis of Tumor Microenvironment. In Rev (2020) 1–15. doi: 10.21203/rs.3.rs-125889/v1
29. Qiu H, Li Y, Cheng S, He C, Li J, Li J. Frontiers | A Prognostic Microenvironment-Related Immune Signature via ESTIMATE (PROMISE Model) Predicts Overall Survival of Patients With Glioma. Oncology. doi: 10.3389/fonc.2020.580263
30. Zhong Q-Y, Fan E-X, Feng G-Y, Chen Q-Y, Gou X-X, Yue G-J, et al. A Gene Expression-Based Study on Immune Cell Subtypes and Glioma Prognosis. BMC Cancer (2019) 19:1116. doi: 10.1186/s12885-019-6324-7
31. Brunner-Weinzierl MC, Rudd CE. CTLA-4 and PD-1 Control of T-Cell Motility and Migration: Implications for Tumor Immunotherapy. Front Immunol (2018) 9:2737. doi: 10.3389/fimmu.2018.02737
32. Iglesia MD, Parker JS, Hoadley KA, Serody JS, Perou CM, Vincent BG. Genomic Analysis of Immune Cell Infiltrates Across 11 Tumor Types. J Natl Cancer Inst (2016) 108:1–11. doi: 10.1093/jnci/djw144
33. Liao P, Han S, Qu H. Expression, Prognosis, and Immune Infiltrates Analyses of E2Fs in Human Brain and CNS Cancer. BioMed Res Int (2020) 2020:1–15. doi: 10.1155/2020/6281635
34. Largeot A, Pagano G, Gonder S, Moussay E, Paggetti J. The B-Side of Cancer Immunity: The Underrated Tune. Cells (2019) 8:449. doi: 10.3390/cells8050449
35. Diem S, Fässler M, Bomze D, Ali OH, Berner F, Niederer R, et al. Immunoglobulin G and Subclasses as Potential Biomarkers in Metastatic Melanoma Patients Starting Checkpoint Inhibitor Treatment. J Immunother (2019) 42:89–93. doi: 10.1097/CJI.0000000000000255
36. Helmink BA, Reddy SM, Gao J, Zhang S, Basar R, Thakur R, et al. B Cells and Tertiary Lymphoid Structures Promote Immunotherapy Response. Nature (2020) 577:549–55. doi: 10.1038/s41586-019-1922-8
37. Hollern DP, Xu N, Thennavan A, Glodowski C, Garcia-Recio S, Mott KR, et al. B Cells and T Follicular Helper Cells Mediate Response to Checkpoint Inhibitors in High Mutation Burden Mouse Models of Breast Cancer. Cell (2019) 179:1191–1206.e21. doi: 10.1016/j.cell.2019.10.028
38. Lee-Chang C, Miska J, Hou D, Rashidi A, Zhang P, Burga RA, et al. Activation of 4-1BBL+ B Cells With CD40 Agonism and Ifnγ Elicits Potent Immunity Against Glioblastoma. J Exp Med (2021) 218:1–21. doi: 10.1084/JEM.20200913
39. Ren F, Zhao Q, Huang L, Zheng Y, Li L, He Q, et al. The R132H Mutation in IDH1 Promotes the Recruitment of NK Cells Through CX3CL1/CX3CR1 Chemotaxis and Is Correlated With a Better Prognosis in Gliomas. Immunol Cell Biol (2019) 97:457–69. doi: 10.1111/imcb.12225
40. Burger MC, Zhang C, Harter PN, Romanski A, Strassheimer F, Senft C, et al. CAR-Engineered NK Cells for the Treatment of Glioblastoma: Turning Innate Effectors Into Precision Tools for Cancer Immunotherapy. Front Immunol (2019) 10:2683. doi: 10.3389/fimmu.2019.02683
41. Holl EK, Frazier VN, Landa K, Beasley GM, Hwang ES, Nair SK. Examining Peripheral and Tumor Cellular Immunome in Patients With Cancer. Front Immunol (2019) 10:1767. doi: 10.3389/fimmu.2019.01767
42. Pluhar GE, Pennell CA, Olin MR. CD8+ T Cell–Independent Immune-Mediated Mechanisms of Anti-Tumor Activity. Crit Rev Immunol (2015) 35:153–72. doi: 10.1615/CritRevImmunol.2015013607
43. Schneider JR, Kwan K, Boockvar JA. Missing Naïve T Cells in the Setting of Glioblastoma Are Found to Be Sequestered in the Bone Marrow. Neurosurgery (2019) 85:E104–5. doi: 10.1093/neuros/nyz167
44. Dowling MR, Kan A, Heinzel S, Marchingo JM, Hodgkin PD, Hawkins ED. Regulatory T Cells Suppress Effector T Cell Proliferation by Limiting Division Destiny. Front Immunol (2018) 9:2461. doi: 10.3389/fimmu.2018.02461
45. Sayour EJ, McLendon P, McLendon R, De Leon G, Reynolds R, Kresak J, et al. Increased Proportion of FoxP3+ Regulatory T Cells in Tumor Infiltrating Lymphocytes is Associated With Tumor Recurrence and Reduced Survival in Patients With Glioblastoma. Cancer Immunol Immunother (2015) 64:419–27. doi: 10.1007/s00262-014-1651-7
46. Long Y, Tao H, Karachi A, Grippin AJ, Jin L, Chang Y, et al. Dysregulation of Glutamate Transport Enhances Treg Function That Promotes VEGF Blockade Resistance in Glioblastoma. Cancer Res (2020) 80:499–509. doi: 10.1158/0008-5472.CAN-19-1577
47. Vidyarthi A, Agnihotri T, Khan N, Singh S, Tewari MK, Radotra BD, et al. Predominance of M2 Macrophages in Gliomas Leads to the Suppression of Local and Systemic Immunity. Cancer Immunol Immunother (2019) 68:1995–2004. doi: 10.1007/s00262-019-02423-8
48. Wang Q, Hu B, Hu X, Kim H, Squatrito M, Scarpace L, et al. Tumor Evolution of Glioma-Intrinsic Gene Expression Subtypes Associates With Immunological Changes in the Microenvironment. Cancer Cell (2017) 32:42–56.e6. doi: 10.1016/j.ccell.2017.06.003
49. Rahbar A, Cederarv M, Wolmer-Solberg N, Tammik C, Stragliotto G, Peredo I, et al. Enhanced Neutrophil Activity Is Associated With Shorter Time to Tumor Progression in Glioblastoma Patients. OncoImmunology (2016) 5:1–8. doi: 10.1080/2162402X.2015.1075693
50. Massara M, Persico P, Bonavita O, Poeta VM, Locati M, Simonelli M, et al. Neutrophils in Gliomas. Front Immunol (2017) 8:1349. doi: 10.3389/fimmu.2017.01349
51. Chen DS, Mellman I. Elements of Cancer Immunity and the Cancer-Immune Set Point. Nature (2017) 541:321–30. doi: 10.1038/nature21349
52. Le Trinh T, Kandell WM, Donatelli SS, Tu N, Tejera MM, Gilvary DL, et al. Immune Evasion by Tgfβ-Induced miR-183 Repression of MICA/B Expression in Human Lung Tumor Cells. OncoImmunology (2019) 8:1–11. doi: 10.1080/2162402X.2018.1557372
53. De Andrade LF, En Tay R, Pan D, Luoma AM, Ito Y, Badrinath S, et al. Antibody-Mediated Inhibition of MICA and MICB Shedding Promotes NK Cell-Driven Tumor Immunity. Science (2018) 359:1537–42. doi: 10.1126/science.aao0505
54. Bauer S, Groh V, Wu J, Steinle A, Phillips JH, Lanier LL, et al. Activation of NK Cells and T Cells by NKG2D, a Receptor for Stress- Inducible MICA. Science (1999) 285:727–9. doi: 10.1126/science.285.5428.727
55. Groh V, Rhinehart R, Randolph-Habecker J, Topp MS, Riddell SR, Spies T. Costimulation of CD8αβ T Cell by NKG2D via Engagement by MIC Induced on Virus-Infected Cells. Nat Immunol (2001) 2:255–60. doi: 10.1038/85321
56. Chraa D, Naim A, Olive D, Badou A. T Lymphocyte Subsets in Cancer Immunity: Friends or Foes. J Leukoc Biol (2019) 105:243–55. doi: 10.1002/JLB.MR0318-097R
57. Powell MD, Read KA, Sreekumar BK, Jones DM, Oestreich KJ. IL-12 Signaling Drives the Differentiation and Function of a TH1-Derived TFH1-Like Cell Population. Sci Rep (2019) 9:1–12. doi: 10.1038/s41598-019-50614-1
58. Xu L, Chen Y, Dutra-Clarke M, Mayakonda A, Hazawa M, Savinoff SE, et al. BCL6 Promotes Glioma and Serves as a Therapeutic Target. Proc Natl Acad Sci USA (2017) 114:15. doi: 10.1073/pnas.1609758114
59. Peng LN, Deng XY, Gan XX, Zhang JH, Ren GH, Shen F, et al. Targeting of TLE3 by Mir−3677 in Human Breast Cancer Promotes Cell Proliferation, Migration and Invasion. Oncol Lett (2020) 19:1409–17. doi: 10.3892/ol.2019.11241
60. Fu Y, Liu CJ, Kobayashi DK, Johanns TM, Bowman-Kirigin JA, Schaettler MO, et al. GATA2 Regulates Constitutive PD-L1 and PD-L2 Expression in Brain Tumors. Sci Rep (2020) 10:1–12. doi: 10.1038/s41598-020-65915-z
61. Wang D, Yang L, Yu W, Wu Q, Lian J, Li F, et al. Colorectal Cancer Cell-Derived CCL20 Recruits Regulatory T Cells to Promote Chemoresistance via FOXO1/CEBPB/NF-κb Signaling. J ImmunoTher Cancer (2019) 7:1–15. doi: 10.1186/s40425-019-0701-2
62. Bettelli E, Carrier Y, Gao W, Korn T, Strom TB, Oukka M, et al. Reciprocal Developmental Pathways for the Generation of Pathogenic Effector TH17 and Regulatory T Cells. Nature (2006) 441:235–8. doi: 10.1038/nature04753
63. Miller SA, Weinmann AS. Common Themes Emerge in the Transcriptional Control of T Helper and Developmental Cell Fate Decisions Regulated by the T-Box, GATA and ROR Families. Immunology (2009) 126:306–15. doi: 10.1111/j.1365-2567.2008.03040.x
64. Hartigan-O’Connor DJ, Hirao LA, McCune JM, Dandekar S. Th17 Cells and Regulatory T Cells in Elite Control Over HIV and SIV. Curr Opin HIV AIDS (2011) 6:221–7. doi: 10.1097/COH.0b013e32834577b3
65. Qi L, Yu H, Zhang Y, Zhao D, Lv P, Zhong Y, et al. IL-10 Secreted by M2 Macrophage Promoted Tumorigenesis Through Interaction With JAK2 in Glioma. Oncotarget (2016) 7:71673–85. doi: 10.18632/oncotarget.12317
66. Liu Z, Kuang W, Zhou Q, Zhang Y. TGF-β1 Secreted by M2 Phenotype Macrophages Enhances the Stemness and Migration of Glioma Cells via the SMAD2/3 Signalling Pathway. Int J Mol Med (2018) 42:3395–403. doi: 10.3892/ijmm.2018.3923
67. Humphries W, Wei J, Sampson JH, Heimberger AB. The Role of Tregs in Glioma-Mediated Immunosuppression: Potential Target for Intervention. Neurosurg Clinics North America (2010) 21:125–37. doi: 10.1016/j.nec.2009.08.012
68. Hosokawa H, Tanaka T, Endo Y, Kato M, Shinoda K, Suzuki A, et al. Akt1-Mediated Gata3 Phosphorylation Controls the Repression of IFNÎ 3 in Memory-Type Th2 Cells. Nat Commun (2016) 7:1–12. doi: 10.1038/ncomms11289
69. Orecchioni M, Ghosheh Y, Pramod AB, Ley K. Macrophage Polarization: Different Gene Signatures in M1(Lps+) vs. Classically and M2(LPS-) vs. Alternatively Activated Macrophages. Front Immunol (2019) 10:1084. doi: 10.3389/fimmu.2019.01084
70. Kanhere A, Hertweck A, Bhatia U, Gökmen MR, Perucha E, Jackson I, et al. Jenner RG. T-Bet and GATA3 Orchestrate Th1 and Th2 Differentiation Through Lineage-Specific Targeting of Distal Regulatory Elements. Nat Commun (2012) 3:1–9. doi: 10.1038/ncomms2260
71. Zhu C, Sakuishi K, Xiao S, Sun Z, Zaghouani S, Gu G, et al. An IL-27/NFIL3 Signalling Axis Drives Tim-3 and IL-10 Expression and T-Cell Dysfunction. Nat Commun (2015) 6:1–12. doi: 10.1038/ncomms7072
72. Yang S, Wei W, Zhao Q. B7-H3, a Checkpoint Molecule, as a Target for Cancer Immunotherapy. Int J Biol Sci (2020) 16:1767–73. doi: 10.7150/ijbs.41105
73. Okoye IS, Houghton M, Tyrrell L, Barakat K, Elahi S. Coinhibitory Receptor Expression and Immune Checkpoint Blockade: Maintaining a Balance in CD8+ T Cell Responses to Chronic Viral Infections and Cancer. Front Immunol (2017) 8:1215. doi: 10.3389/fimmu.2017.01215
74. Verdon DJ, Mulazzani M, Jenkins MR. Cellular and Molecular Mechanisms of CD8+ T Cell Differentiation, Dysfunction and Exhaustion. Int J Mol Sci (2020) 21:1–28. doi: 10.3390/ijms21197357
75. Ghouzlani A, Rafii S, Karkouri M, Lakhdar A, Badou A. The Promising IgSF11 Immune Checkpoint Is Highly Expressed in Advanced Human Gliomas and Associates to Poor Prognosis. Front Oncol (2021) 10:608609. doi: 10.3389/fonc.2020.608609
76. Ghouzlani A, Kandoussi S, Tall M, Reddy KP, Rafii S, Badou A. Immune Checkpoint Inhibitors in Human Glioma Microenvironment. Front Immunol (2021) 12:679425. doi: 10.3389/fimmu.2021.679425
77. Poon MTC, Sudlow CLM, Figueroa JD, Brennan PM. Longer-Term (≥ 2 Years) Survival in Patients With Glioblastoma in Population-Based Studies Pre- and Post-2005: A Systematic Review and Meta-Analysis. Sci Rep (2020) 10:11622. doi: 10.1038/s41598-020-68011-4
78. Auffinger B, Spencer D, Pytel P, Ahmed AU, Lesniak MS. The Role of Glioma Stem Cells in Chemotherapy Resistance and Glioblastoma Multiforme Recurrence. Expert Rev Neurother (2015) 15:741–52. doi: 10.1586/14737175.2015.1051968
Keywords: glioma, tumour-infiltrating immune cell, biomarker, immune response, prognosis
Citation: Ait Ssi S, Chraa D, El Azhary K, Sahraoui S, Olive D and Badou A (2021) Prognostic Gene Expression Signature in Patients With Distinct Glioma Grades. Front. Immunol. 12:685213. doi: 10.3389/fimmu.2021.685213
Received: 24 March 2021; Accepted: 10 August 2021;
Published: 01 September 2021.
Edited by:
Mohamed-Ridha Barbouche, Pasteur Institute of Tunis, TunisiaReviewed by:
Roslyn Kemp, University of Otago, New ZealandVenkata Boddupalli, Yale University, United States
Copyright © 2021 Ait Ssi, Chraa, El Azhary, Sahraoui, Olive and Badou. This is an open-access article distributed under the terms of the Creative Commons Attribution License (CC BY). The use, distribution or reproduction in other forums is permitted, provided the original author(s) and the copyright owner(s) are credited and that the original publication in this journal is cited, in accordance with accepted academic practice. No use, distribution or reproduction is permitted which does not comply with these terms.
*Correspondence: Abdallah Badou, YWJkYWxsYWhiYWRvdUB5YWhvby5jb20=
†These authors have contributed equally to this work