- Department of Thoracic Surgery, National Cancer Center/National Clinical Research Center for Cancer/Cancer Hospital, Chinese Academy of Medical Sciences and Peking Union Medical College, Beijing, China
Recent publications have revealed that N6-methyladenosine (m6A) modification is critically involved in tumorigenesis and metastasis. However, the correlation of m6A modification and immune infiltration in early-stage lung adenocarcinoma (LUAD) is still uncertain. We performed NMF clustering based on 23 m6A regulators and identify three distinct m6A clusters and three m6A related genes clusters (m6A cluster-R) in early-stage LUAD. The immune infiltrating levels were calculated using CIBERSORT, MCPcounter and ssGSEA algorithms. And we established the m6A-predictive score to quantify m6A modified phenotypes and predict immunotherapeutic responses. Based on the TME characteristics, different immune profiles were also identified among three m6A gene-related clusters. And the m6A-R-C2 was related to a favorable overall survival (OS), whereas m6A-R-C3 had unfavorable overall survival. The m6A-predictive score was built according to the expression levels of m6A-related genes, and patients could be stratified into subgroups with low/high scores. Patients with high scores had poor overall survival, enhanced immune infiltration, high tumor mutation burden and increased level of somatic mutation. Besides, patients with high scores had unfavorable overall survival in the anti-PD-1 cohort, whereas the overall survival of high-score patients was better in the adoptive T cell therapy cohort. Our work highlights that m6A modification is closely related to immune infiltration in early-stage LUAD, which also contributes to the development of more effective immunotherapy strategies.
Introduction
Lung adenocarcinoma (LUAD) is one of the most prevalent cancers around the world, accounting for approximately 40% of lung cancer patients (1). The study of LUAD has raised considerable concerns because it has a high rate of invasiveness and metastasis, which is the main cause of tumor-associated death (2, 3). Although with the rapid progress of various treatments, such as surgery, radiotherapy and chemotherapy, LUAD patients’ prognosis is still very poor (4–6). Some radiological approaches, such as low-dose computerized tomography (CT), are implemented to screen for LUAD and truly reduced the mortality of patients, but radiological approaches cannot benefit every patient and the diagnostic accuracy still has room for improvement (7, 8). Also, in the treatment of early-stage LUAD, chemotherapy could not reach the satisfactory efficacy among patients with negative driver gene mutation and the use of immunotherapy has remained largely unknown (9, 10). Thus, we need an in-depth investigation of detailed molecular mechanisms to identify patients with a high probability of death, which may contribute to the precise treatment of patients with early-stage LUAD.
N6-methyladenosine (m6A) has been regarded as the most common RNA modification, which primarily focuses on regulating splicing, translation and processing of the specific RNA. And it serves as a critically significant factor in diverse physiological and pathological processes (11–14). Generally speaking, m6A modification is regulated by three regulatory proteins: methyltransferases, binding proteins and demethylases (also known as writers, readers, and erasers) (15). Recent studies have indicated that m6A modification has a strong impact on the occurrence and metastasis of cancer, which suggests that a more comprehensive understanding of m6A modification’s detailed mechanism may benefit patients with cancer (14, 16). For example, Jin et al. have shown that m6A modification induced by METTL3 can increase YAP translation, thus promoting drug resistance and metastasis of non-small cell lung cancer (NSCLC) (17). However, in the early-stage LUAD, the exact roles of m6A modifications and their regulators remain largely unknown. Thus, the exploration of m6A modifications is urgently needed and may contribute to the development of m6A-based therapy.
Increasing evidence has revealed that multiple types of immune cells in the tumor microenvironment (TME) play a critically important role in tumorigenesis and metastasis (18). Furthermore, specific immune checkpoint inhibitors (ICIs), such as anti-PD-1/L1 and anti-CTLA-4, are widely applied in today’s immunotherapy and have been proven to be helpful in LUAD patients with specific immunophenotype (19–21). Thus, identifying particular characteristics of the TME may help to predict the immunotherapeutic responses, which could contribute to the development of more effective therapy in early-stage LUAD patients (22, 23). In addition, several studies have indicated a close relationship between m6A modification and immune infiltrating cells in the TME. Su et al. have revealed that deletion of FTO, an m6A regulatory protein, could inhibit the expression of LILRB4, thus dramatically attenuating the reprogram immune response of the leukemia stem cell. Due to the downregulation of FTO, leukemia cells were more sensitive to T cell cytotoxicity and immune evasion could be avoided (24). Also, Han et al. reported that the knockdown of YTHDF1 in dendritic cells could improve cross-priming of CD8+ T cells and cross-presentation of tumor neoantigens, suggesting YTHDF1 served as a critical biomarker in immunotherapy (25). However, we still lack a more comprehensive study focusing on all of the m6A regulatory proteins in early-stage LUAD. Therefore, exploring the relationship between m6A modification and immune infiltration may help us understand the regulation of the immune system and promote research in tumor immunotherapy.
In this study, we systematically analyzed the relationship between immune infiltrating levels and m6A modification clusters by using the genomic and transcriptomic data of 1230 early-stage LUAD patients. We have utilized nonnegative matrix factorization (NMF) clustering and identified three m6A clusters with different immune phenotypes, indicating m6A modification served as a non-negligible factor in affecting individual TME profiles. Furthermore, we also constructed the m6A-predictive score, which can be used to evaluate m6A modification, predict immune infiltrating levels and patients’ immunotherapeutic responses, suggesting its indispensable utility in clinical diagnosis and treatment.
Materials and Methods
The Collection of Available Datasets
We downloaded the genomic and clinical information of the early-stage LUAD from the GEO database (http://www.ncbi.nlm.nih.gov/geo/) and the TCGA database via UCSC Xena (https://xena.ucsc.edu/). According to the staging system of the American Joint Committee on Cancer (AJCC), LUAD of stage IA, IB, IIA and IIB could be defined as early-stage LUAD. 1230 patients were analyzed in this study, including patients from TCGA-LUAD (n = 374), GSE29013 (n = 22) (26), GSE30219 (n = 81) (27), GSE31210 (n = 226) (28), GSE37745 (n = 89) (29), GSE50081 (n = 127) (30) and GSE72094 (n = 311) (31). The baseline information of all of the early-stage LUAD patients was presented in Table S2. As for the TCGA-LUAD dataset, we obtained the copy number alteration data and the DNA methylation data (data of FMR1 was lack) from the cBioportal database (https://www.cbioportal.org/) and the somatic mutation data from the UCSC Xena. We analyzed the somatic mutation data using the “maftools” R package (version 2.6.05) (32). And we transformed the RNA-seq data of the TCGA-LUAD from the fragments per kilobase million (FPKM) format to transcripts per kilobase million (TPM) and log2(TPM + 1) format. Due to the same microarray platform (Affymetrix Human Genome U133 Plus 2.0 Array) used by GSE29013, GSE30219, GSE31210, GSE37745 and GSE50081, we obtained the raw CEL data and used the “gcrma” R package (version 2.62.0) to correct the background and normalize. Next, we used the ComBat function of the sva R package (version 3.38.0) to combine these five GSE datasets into a meta-GEO cohort (33). To increase the comparability among all the datasets, we utilized the scale transformation in the meta-GEO and GSE72094 cohorts before constructing the m6A-predictive score. Besides, we averaged expression values of genes that had multiple probe set signals.
Consensus Clustering With NMF
We collected the 23 m6A regulators via retrieving previous publications correlated with m6A modification (Table S1) (13, 34–37). According to 23 m6A regulators’ expression levels, NMF clustering was performed to stratify distinct m6A clusters. The “NMF” R package (version 0.23.0) was utilized with the brunet algorithm and 50 nruns in this analysis (38). And we performed another NMF clustering according to the expression of overlapping differentially expressed genes (DEGs) with the lee method. The m6A related gene clusters (m6A cluster-R) were determined. According to the results of the NMF clustering (cophenetic, residuals, dispersion and rss coefficients), we chose the best number of clusters.
GSVA, GSEA and GO/KEGG Enrichment Analysis
We used the “GSVA” R package (version 1.38.2) to perform Gene set variation analysis (GSVA), aiming to analyze different biological processes among all m6A related clusters (39). The Hallmarker gene set was used as the biological signatures and was obtained via the MSigDB database v7.2 (40). Gene Set Enrichment Analysis (GSEA) was applied via the “clusterProfiler” R package (version 3.18.1) and P.adjust < 0.05 was considered statistically significant (41). Gene Ontology (GO) and Kyoto Encyclopedia of Genes and Genomes (KEGG) analysis were also performed via the “clusterProfiler” R package (version 3.18.1). And the threshold of the GO analysis was P.adjust < 0.05 and the threshold of the KEGG analysis was P < 0.05 and P.adjust < 0.2.
Estimation of Immune Cell Infiltration: ssGSEA, CIBERSORT and MCPcounter
We utilized different algorithms to evaluate the fraction of immune infiltrating cells, including single sample gene set enrichment analysis (ssGSEA), CIBERSORT and MCPcounter. The “GSVA” R package (version 1.38.2) was used to perform ssGSEA, which could calculate the fraction of twenty-eight immune cells of the TME. The estimated proportion of these immune infiltrating cells was characterized by a normalized score and shown in the heatmap. The CIBERSORT algorithm (http://cibersort.stanford.edu/) was designed to estimate the relative fraction of twenty-two immune cells (42). And we utilized the “MCPcounter” R package (version 1.2.0) to evaluate the abundances of two stromal cells and eight immune cells (43).
Prediction of Immune Response: Immunophenoscore (IPS) and ESTIMATE
Immunophenoscore (IPS) serves as an essential factor in predicting response to anti-PD-1 and anti-CTLA-4 therapies. We calculated the IPS to investigate determinants of tumor immunogenicity, which also revealed cancer antigenomes and intratumoral immune features (44). ESTIMATE algorithm is generally utilized to infer the immune score and the stromal score, which is also useful to indicate the levels of immune infiltration (45). Based on the transcriptional profiles, we calculated the ESTIMATEScore, ImmuneScore and StromalScore to reveal different immune infiltrating levels of each cluster.
DEGs Among Different m6A Clusters
Based on the results of the NMF clustering, three distinct m6A clusters were identified in the meta-GEO cohort. We then identified the DEGs between every two m6A clusters using the “limma” R package (version 3.46.0) (46). We calculated the differential expressed statistics via the lmFit and eBayes functions. We set |fold change| > 1 and P.adjust < 0.01 as the statistically significant threshold to identify DEGs. And we intersected three groups of DEGs to determine the overlapping DEGs among three different m6A clusters.
Construction and Validation of the m6A-Predictive Score
Although the distinct m6A clusters were associated with prognosis and TME of patients with early-stage LUAD, it was not convenient to perform the NMF clustering in each independent cohort. Therefore, a more useful and reliable scoring system was required to analyze the prognosis and immune features of patients with early-stage LUAD. To begin with, we performed univariate Cox regression analysis using the “survival” R package (version: 3.2-10) to screen for the overlapping DEGs with prognostic value (overall survival) in the meta-GEO cohort. We defined P < 0.05 as the statistically significant threshold. The genes with an important prognostic impact were then analyzed with random forest (RF) algorithms using the “randomForestSRC” R package (version 2.10.1) and some genes were selected. Finally, we conducted the multivariate Cox regression analysis of these selected genes to screen for final genes and established the m6A-predictive score. The coefficients of the final genes were extracted from the multivariate Cox regression results. We used the following formula as the m6A-predictive score: score = ∑(Coefi * Expri), where n refers to the number of the key genes, Coefi refers to the coefficient of genei and Expri refers to the expression level of genei. We then calculated the m6A-predictive score of all samples and stratified patients into high- and low-score subgroups according to the median value of the m6A-predictive score. We also validated the efficacy of the m6A-predictive score in the TCGA and GSE72094 cohorts, respectively. We used the “survminer” R package (version 0.4.9) to get the Kaplan–Meier curves in these cohorts. And we evaluated the performance of this scoring system via the time-dependent receiver operating characteristic (ROC) curves using the “survivalROC” R package (version: 1.0.3).
Establishment of the Nomogram
Using univariate and multivariate Cox regression analysis, we investigated whether our scoring system was an independent parameter among other clinical parameters, and the co-variates were composed of age and pathological stage. Then we utilized “rms” R package (version 6.1-1) to build a nomogram, which can predict the prognosis of patients with early-stage LUAD. Next, we used Calibration curves and the time-dependent ROC curves to evaluate this nomogram’ predictive accuracy. Also, we compared the concordance index among all the clinical parameters to analyze the discrimination of our nomogram.
The Performance of m6A-Predictive Score in Immunotherapeutic Cohort
We further integrated two independent immunotherapy cohorts with genomic and clinical data to validate whether patients stratified by high and low m6A-predictive scores had significantly different clinical outcomes (overall survival). The X-tile software (version: 3.6.1) was utilized to identify the optimal cutoff value (47). A two-sided P value was used in the analysis. The data were downloaded from the TIDE database (http://tide.dfci.harvard.edu/) (48). The gene expression data were already normalized via the TIDE database. These cohorts mainly focused on the immunotherapy of patients with melanoma, which included anti-PD-1 antibody intervention in the study of Gide et al. (49) and the utilization of adoptive T cell therapy in the study of Lauss et al. (50).
The Single-Cell Analysis for T Cells
We analyzed the T cells’ single-cell RNA-seq data of LUAD patients via http://lung.cancer-pku.cn/index.php, which contained 12 346 T cells from 14 treatment-naive NSCLC patients (51). The expression levels of selected m6A genes were normalized and sixteen clusters were identified, including two for regulatory T cells, seven for conventional CD4+ T cells and seven for CD8+ T cells. We used the boxplot and t-SNE plot to evaluate the associations between selected m6A genes and T cells populations.
Statistical Analysis
We used R software (version 4.0.4) to conduct all of the statistical analyses. The analysis of the correlation was conducted via Spearman’s correlation method. We performed the Wilcoxon rank sum test (Mann-Whitney U-test) to compare the difference between the two groups. As for the difference among more than two groups, we used the Kruskal–Wallis H-test to evaluate the variance. Schoenfeld residuals was used to confirm the assumptions of the Cox proportional hazard models. P < 0.05 was considered as the statistically significant threshold. The tests used in each part of study were also presented in the figure.
Results
The Genetic Landscape of m6A Regulators in Early-Stage LUAD
The flowchart of our study is presented in Figure S1. The somatic mutations of 23 m6A regulators were infrequent in early-stage LUAD. 92 of the 355 (25.9%) samples had the somatic mutations of m6A regulators, which primarily included missense mutations and nonsense mutations. The results revealed that the top three genes with the highest mutation were ZC3H13 (5%), IGF2BP1 (3%) and LRPPRC (3%) (Figure 1A). Then, we explored the relationship between m6A regulators’ expression and DNA methylation (Table S3). All of the significant correlations between m6A regulators’ expression and DNA methylation were negative. IGF2BP1, IGF2BP2, and IGF2BP3 were the top three genes whose expression was closely related to the DNA methylation level (Figure 1B). The copy number alterations of the m6A regulators were prevalent, which included copy number gains and losses. VIRMA, IGF2BP3, YTHDF1 displayed prevalent copy number gains, while ZC3H13, ELAVL1 and WTAP showed widespread copy number losses (Figure 1C). Compared with normal samples, we found that VIRMA, METTL3, RBM15, ELAVL1, HNRNPC, HNRNPA2B1, IGF2BP1, IGF2BP3, YTHDF1, YTHDF2, LRPPRC were highly expressed in tumors, while METTL14, ZC3H13 and FTO were mainly downregulated (Figure 1E). We then evaluated the correlation of the expression levels among the m6A regulators. According to the Spearman correlation analysis, we found that most of m6A regulators revealed a significant inverse correlation with the other, such as HNRNPA2B1, YTHDF1 and HNRNPC (Figure 1D and Table S4). Additionally, we utilized univariate and multivariate Cox regression to evaluate m6A regulators’ prognostic value (overall survival). The forest plot suggested that RBM15 was highly associated with patients’ overall survival and could be recognized as a protective factor, while ALKBH5 was negatively correlated with the patient’s prognosis (Figures S2A, B).
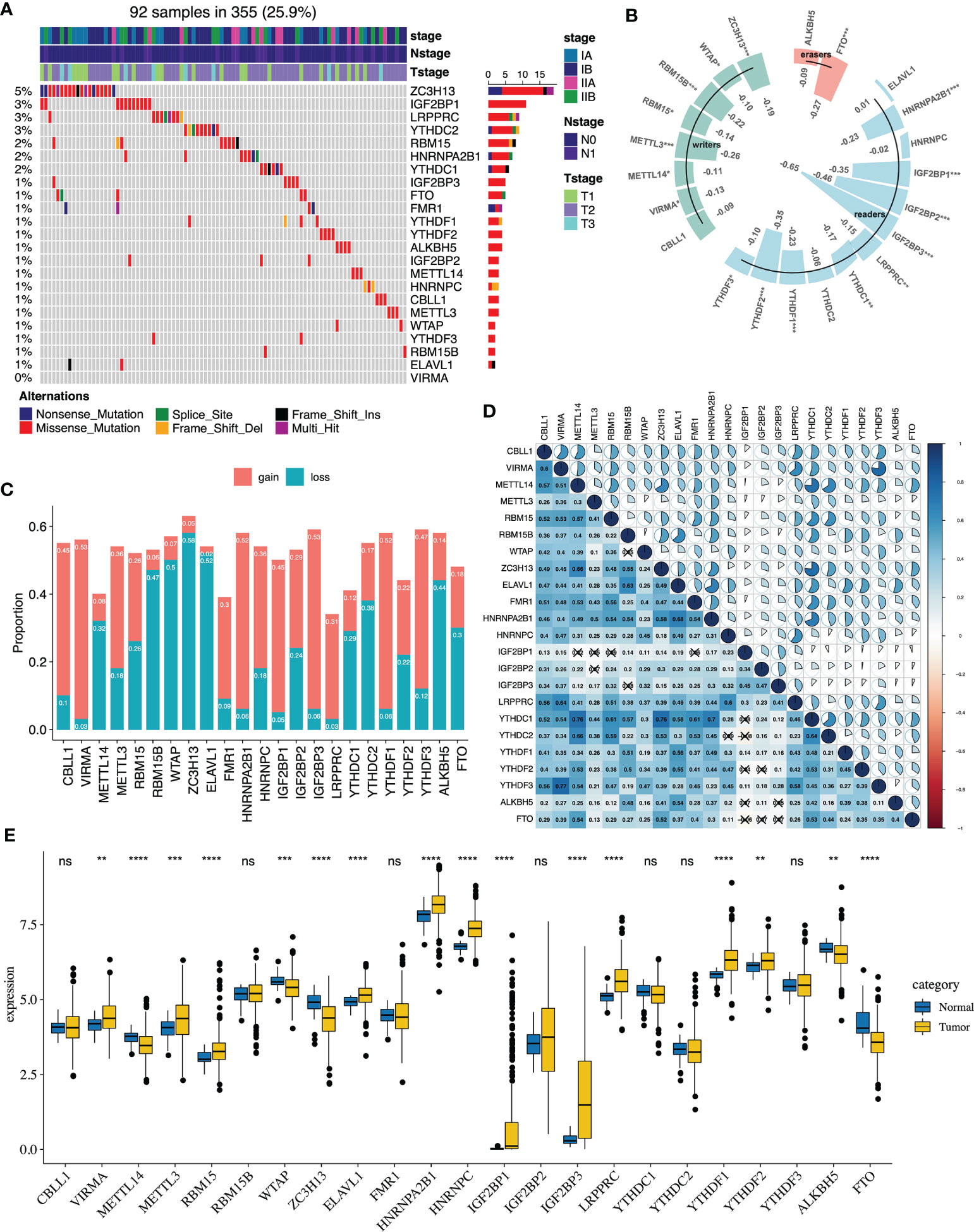
Figure 1 The genetic features of 23 m6A regulators in early-stage lung adenocarcinoma of the TCGA cohort. (A) The genetic mutations of 23 m6A regulators in 92 patients. (B) The correlation analysis between DNA methylation and 23 m6A regulators’ expression (*P < 0.05; **P < 0.01; ***P < 0.001). (C) The CNA landscape of 23 m6A regulators. Gain represents gain and high level amplification, loss represents homozygous deletion and hemizygous deletion. (D) The correlation analysis between the 23 m6A regulators via the Spearman correlation method. (E) The m6A regulators expression between normal and tumor groups (*P < 0.05; **P < 0.01; ***P < 0.001; ****P < 0.0001). ns, not significant.
Identification of Specific Phenotypes Based on m6A Regulators
To stratify patients into different m6A phenotypes, we performed the NMF algorithm according to 23 m6A regulators’ expression levels (Figure S3A, B). Three m6A phenotypes were identified in the meta-GEO cohort, including 120 patients in m6A-C1, 230 patients in m6A-C2 and 195 patients in m6A-C3. Then, we conducted a log-rank test and the Kaplan-Meier curves of the meta-GEO cohort revealed that m6A-C2 had the best prognosis, whereas m6A-C1 was related to unfavorable prognosis (Figure S4C). And similar results were shown in the Kaplan-Meier curves of the TCGA cohort (Figure S4D). The expression patterns of these three clusters were aberrantly different. The expression level of YTHDF3 was increased in patients of m6A-C3; The expression levels of IGF2BP1, IGF2BP3 were predominantly elevated in patients of m6A-C1; The expression level of IGF2BP2, LRPPRC, WATP, YTHDF3 were relatively increased in patients of m6A-C2 (Figures S3C, D and Table S6). We further conducted multivariate Cox regression analysis to determine the correlation of the m6A clusters and patients’ clinical outcomes. The results indicated that m6A clusters were correlated with patients’ OS especially in the TCGA cohort (m6A-C2 vs. m6A-C1, HR = 0.529 [95%CI = 0.308 - 0.908], P = 0.0209; Figure S4).
The Expression Levels of m6A Genes in Exhausted T Cells in LUAD
We have analyzed the expression levels of 23 m6A genes in different T cells of LUAD via a single-cell database. The results indicated that CBLL1 and WTAP served as two significant factors in T cells infiltration. The expression levels of WTAP were relatively higher in CD4-C2-ANXA1, CD4-C7-CXCL13, CD4-C9-CTLA4 and CD8-C6-LAYN populations than others, indicating CD8+ T cells and conventional CD4+ T cells (Figure S9A). The expression levels of CBLL1 were relatively higher in CD4-C2-ANXA1 and CD4-C8-FOXP3 populations than others, indicating conventional CD4+ T cells and regulatory T cells (Figure S9C). According to the t-SNE plot, the T cells enrichment regions with high CBLL1 and WTAP expression were also highly overlapped with the above clusters (Figures S9B, D, E).
The Correlation of m6A Phenotypes With Immune Infiltration
We utilized the Hallmarker gene set to perform the GSVA enrichment analysis, which revealed different biological processes. Some tumor-related targets (MYC, E2F and G2M) and tumor-related biological processes (angiogenesis, epithelial-mesenchymal transition (EMT) and hypoxia) were enriched in m6A-C1. On the contrary, m6A-C2 were relatively enriched pathways of cancer development and progression, including Wnt-β-catenin, Notch and TGF-β signaling pathways. And m6A-C3 exhibited the GSVA scores between m6A-C1 and m6A-C2 in the above gene sets (Figure 2D). To determine the relationship between different m6A phenotypes and immune infiltrating cells, we conducted the CIBERSORT algorithm among three m6A clusters. The fraction of immune infiltrating cells was presented in Figure 2A. We also compared the fraction of immune cells among these three m6A clusters. The results indicated that the proportion of macrophages M0, macrophages M1, plasma cells and activated memory CD4 T cells were the highest in m6A-C1, the proportion of dendritic cells resting, eosinophils, monocytes, mast cells resting, T cells CD4 memory resting, T cells follicular helper and NK cells activated were the highest in m6A-C2 and the proportion of mast cells activated were the highest in m6A-C3 (Figure 2B). To further evaluate the clinical significance of these three m6A phenotypes, we also analyzed the expression of different biomarkers (PD-1, PD-L1 and CTLA-4) in the immunotherapy (52, 53). The results revealed that PD-L1 and CTLA-4 expression were markedly elevated in m6A-C1, while PD-1 expression was relatively increased in m6A-C2 (Figure 2C).
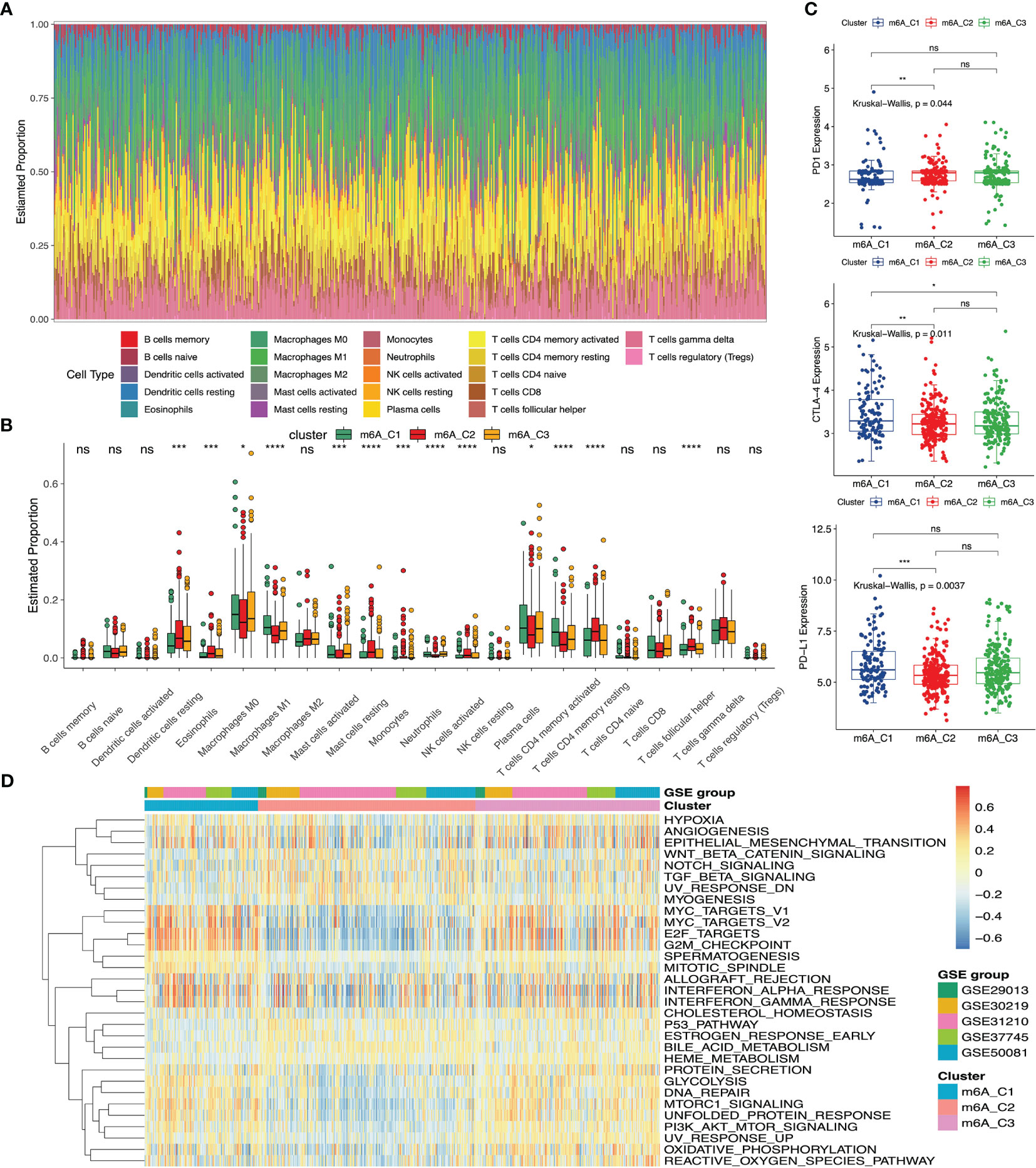
Figure 2 The immune and biological landscape of three different clusters in meta-GEO cohort. (A) The results of the CIBERSORT analysis reveals the estimated proportion of different immune infiltrating cells. (B) The results of the CIBERSORT analysis reveals the estimated proportion of multiple immune infiltrating cells in three m6A clusters. Kruskal-Wallis H test was applied to compare the difference among three clusters (*P < 0.05; **P < 0.01; ***P < 0.001, ****P < 0.0001; ns, not significant). The estimated proportion of each immune cell was symbolized by the scattered dots. The median proportion was symbolized by the thick line. The interquartile range was represented by the range between the top and bottom of each box. (C) The difference of PD1, CTLA-4 and PD-L1 expression levels among three m6A clusters. (D) GSVA results were presented in the meta-GEO cohort among three clusters. The sample annotations of the heatmap were the composition of different GEO cohorts.
We then utilized Spearman’s correlation analyses to investigate the relationship between different immune infiltrating cells and m6A regulators. Our results revealed that the expression of LRPPRC, VIRMA, YTHDF2, YTHDC1, HNRNPC, METTL14 and METTL3 were negatively correlated with immune infiltration, whereas WTAP expression was positively related to immune infiltration (Figure S5A). LRPPRC was negatively correlated with infiltrating levels of immune cells, including type 1 T helper cell, T follicular helper cell, macrophage, effector memory CD8 T cell and activated dendritic cell. Thus, we divided the patients into two subgroups according to the high- and low-expression level of LRPPRC to analyze its role in early-stage LUAD. We used the CIBERSORT algorithm to explore the difference of immune infiltration between low- and high-LRPPRC. Our results implied that infiltrating levels of mast cells resting, dendritic cells activated, T cells follicular helper and T cells CD4 memory activated were relatively elevated in the high-LRPPRC group, whereas T cells CD8, mast cells activated, and plasma cells were the opposite (Figure S5B). Furthermore, we analyzed the immune-related scores between the two groups, which indicated that ImmuneScore, ESTIMATEScore and StromalScore were higher in the high-LRPPRC group (Figure S5C). And we evaluated biomarkers’ expression in immunotherapy. We found LRPPRC expression was negatively associated with the expression of PD-1, PD-L1 and CTLA-4 (Figure S5D). Besides, we performed the GSEA analysis to evaluate related gene sets of the low- and high-LRPPRC subgroups. Our results implied that patients in the low-LRPPRC enriched genes of mRNA processing, RNA splicing and mRNA processing, and patients in the high-LRPPRC enriched genes of Staphylococcus aureus infection, hematopoietic cell lineage and complement and coagulation cascades (Figure S5E). In a word, we hypothesized that LRPPRC could impede the activation of immune cells (like CD8 T cells) or mRNA processing to regulate cancer development and progression.
The DEGs Among Three m6A Phenotypes
To determine the extensive role of these three m6A clusters stratified by 23 m6A regulators’ expression, we further analyzed the DEGs across m6A-C1, m6A-C2 and m6A-C3 in the meta-GEO cohort. 306 DEGs were regarded as m6A-correlated signatures and utilized for later analysis. The Venn diagram was used to reveal the overlapping DEGs among the three m6A clusters (Figure 3A). Next, we carried out the GO and KEGG enrichment analysis of these overlapping DEGs to evaluate relevant biological processes and pathways. The results indicated that this m6A-correlated signature was closely related to some biological processes, including chromosome segregation, nuclear division and regulation of cell cycle phase transition (Figure 3B). And m6A-correlated signature was closely related to the pathways, including p53 signaling, oocyte meiosis and cell cycle pathway (Figure 3C). According to the overlapping DEGs, we utilized the NMF clustering analysis and stratified patients in the meta-GEO cohort into three distinct clusters (Figures S6A, B). These three clusters were defined as m6A-R-C1, m6A-R-C2 and m6A-R-C3, which showed different clinical parameters. The heatmap revealed that patients with pathological stage IA were mainly classified by m6A-R-C2, whereas patients with pathological stage II were mostly represented by m6A-R-C3 (Figure S6C and Table S5). We then evaluated the overall survival of patients in the three cluster-Rs via multivariate Cox regression analysis and the log-rank test. Kaplan-Meier curves showed that patients in m6A-R-C2 had the best prognosis, whereas patients in m6A-R-C3 had the worst prognosis (Figure 3D). The results revealed that age, pathological stage and cluster-R were associated with patients’ OS (m6A-R-C2 vs. m6A-R-C3, HR = 0.444 [95%CI = 0.295 - 0.666], P < 0.001; m6A-R-C1 vs. m6A-R-C3, HR = 0.476 [95%CI = 0.343 - 0.661], P < 0.001, Figure 3E). We further evaluated m6A regulators’ expression among three cluster-Rs and determined that most of the m6A regulators’ expression varied in different cluster-Rs (Figure 3F). Moreover, we analyzed the immune landscape of the three cluster-Rs. We performed the ssGSEA analysis to evaluate the proportion of twenty-eight immune cells in these three m6A gene-related phenotypes. We found that activated CD4 T cells, memory B cells were relatively enriched in m6A-R-C3 (Figure S4E). We then utilized the ESTIMATE algorithm to figure out the differences among the three cluster-Rs. The results indicated that m6A-R-C2 exhibited the lowest ImmuneScore, ESTIMATEScore and StromalScore, suggesting that m6A-R-C2 was rarely related to immunity (Figure S6D). Also, we found that CTLA-4 and PD-L1 expression were lowest in m6A-R-C2 and highest in m6A-R-C3, which also suggested different immune features across three cluster-Rs (Figure 3G).
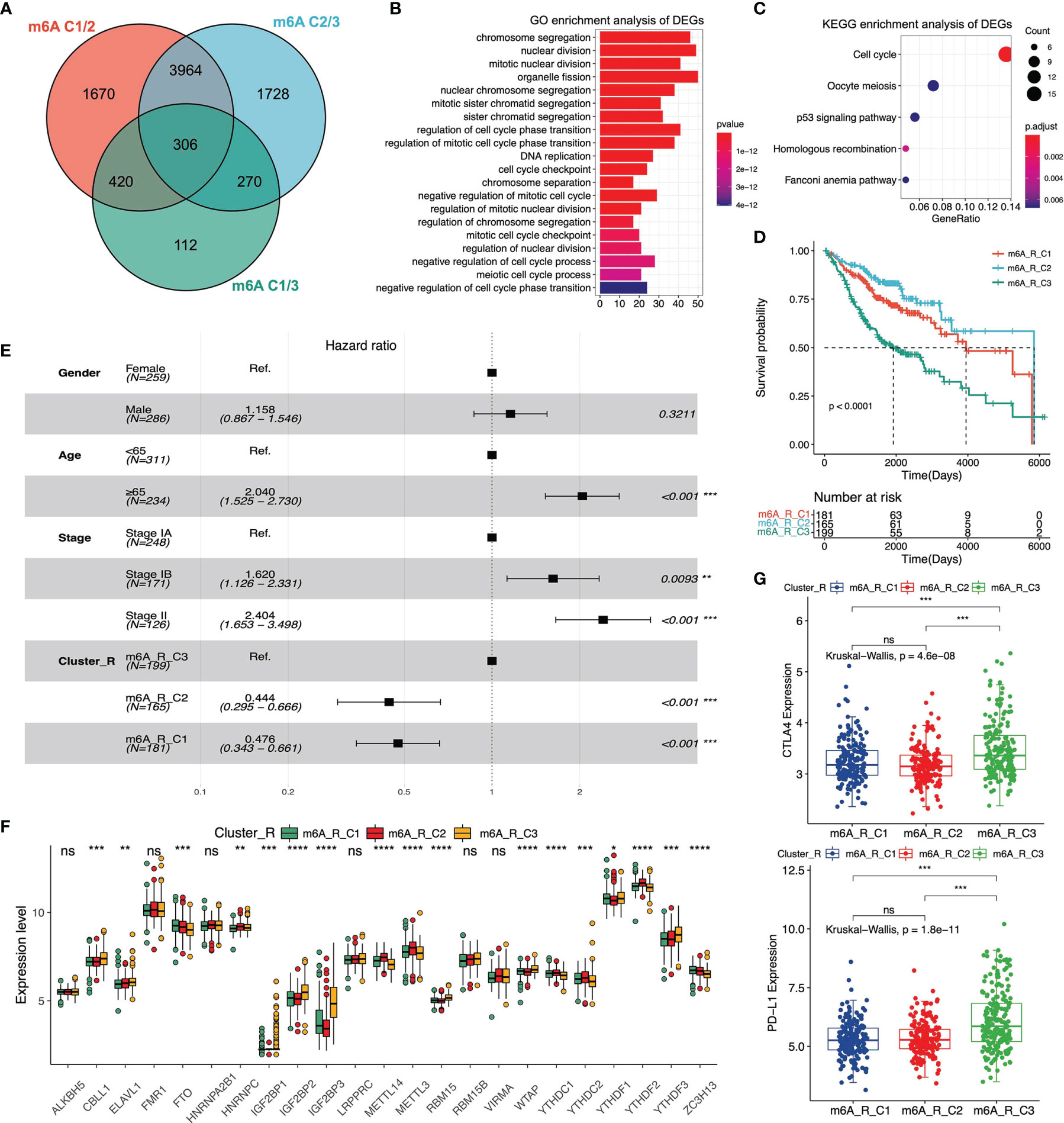
Figure 3 Analysis of differentially expressed genes (DEGs) among three m6A clusters and enrichment analysis in meta-GEO cohort. (A) The Venn diagram reveals the m6A correlated differentially expressed genes. (B) GO enrichment analysis of the DEGs among three clusters. (C) KEGG enrichment analysis of the DEGs among three clusters. (D) The Kaplan-Meier curves of three m6A gene-related clusters via Log-rank test. (E) Multivariate Cox regression analysis of clinical parameters among three m6A gene-related clusters. 95% confidence interval was symbolized by the horizontal line. Hazard ratio (HR) was symbolized by the vertical dotted line. (F) The 23 m6A regulators’ expression among three m6A gene-related clusters. (G) The expression levels of CTLA-4 and PD-L1 among three m6A gene-related clusters. *P < 0.05; **P < 0.01; ***P < 0.001; ****P < 0.0001; ns, not significant.
Establishment of the m6A-Predictive Score and Nomogram
Although m6A-related clusters and cluster-Rs can stratify early-stage LUAD patients into distinct groups correlated with different prognoses, this predictive method was not efficient to predict patients in the individual cohort. Thus, a more accurate and consistent predictive model based on m6A-related clusters and cluster-Rs was needed. And we established the m6A-predictive score to classify early-stage LUAD patients, which was of great importance. The m6A-predictive score was established based on five key genes selected via univariate Cox regression analysis (Table S7), random forest analysis and multivariate Cox regression analysis (Table S8) (m6A-predictive score = -3.2370611 * ExprLRIG1 + 0.3936202 * ExprCTSV + 0.5548459 * ExprKIF20A + 0.7905488 * ExprATP13A3 - 0.4148747 * ExprTMPRSS2). We used the alluvial diagram to illustrate the connection among GSE groups, m6A-related clusters, m6A-related cluster-Rs and m6A-predictive score (Figure 4A). We identified patients in m6A-C1 were more likely to have a higher m6A-predictive score, while patients in m6A-C2 were more likely to have a lower m6A-predictive score. The above findings were also illustrated by a violin diagram (Figure 4E). Also, we found that patients in the m6A-R-C3 had the highest m6A-predictive score among these three cluster-Rs (Figure 4B). We then divided the patients into low- and high-score subgroups and determined the efficacy of the m6A-predictive score (Figure S7B). In the meta-GEO cohort, Kaplan-Meier curves implied that a higher m6A-predictive score was related to a worse prognosis of early-stage LUAD patients (Figure 4D, left panel). And we utilized the time-dependent ROC curves to evaluate the predictive efficacy of the m6A-predictive score in the meta-GEO cohort, which revealed that the 1-, 3- and 5-year AUCs were 0.67, 0.71 and 0.75 (Figure S7A). Besides, we also analyzed the relationship between PD-L1 expression and m6A-predictive score in the meta-GEO cohort. We observed that PD-L1 expression was remarkedly elevated in the high m6A-score group (Figure 4F, left panel).
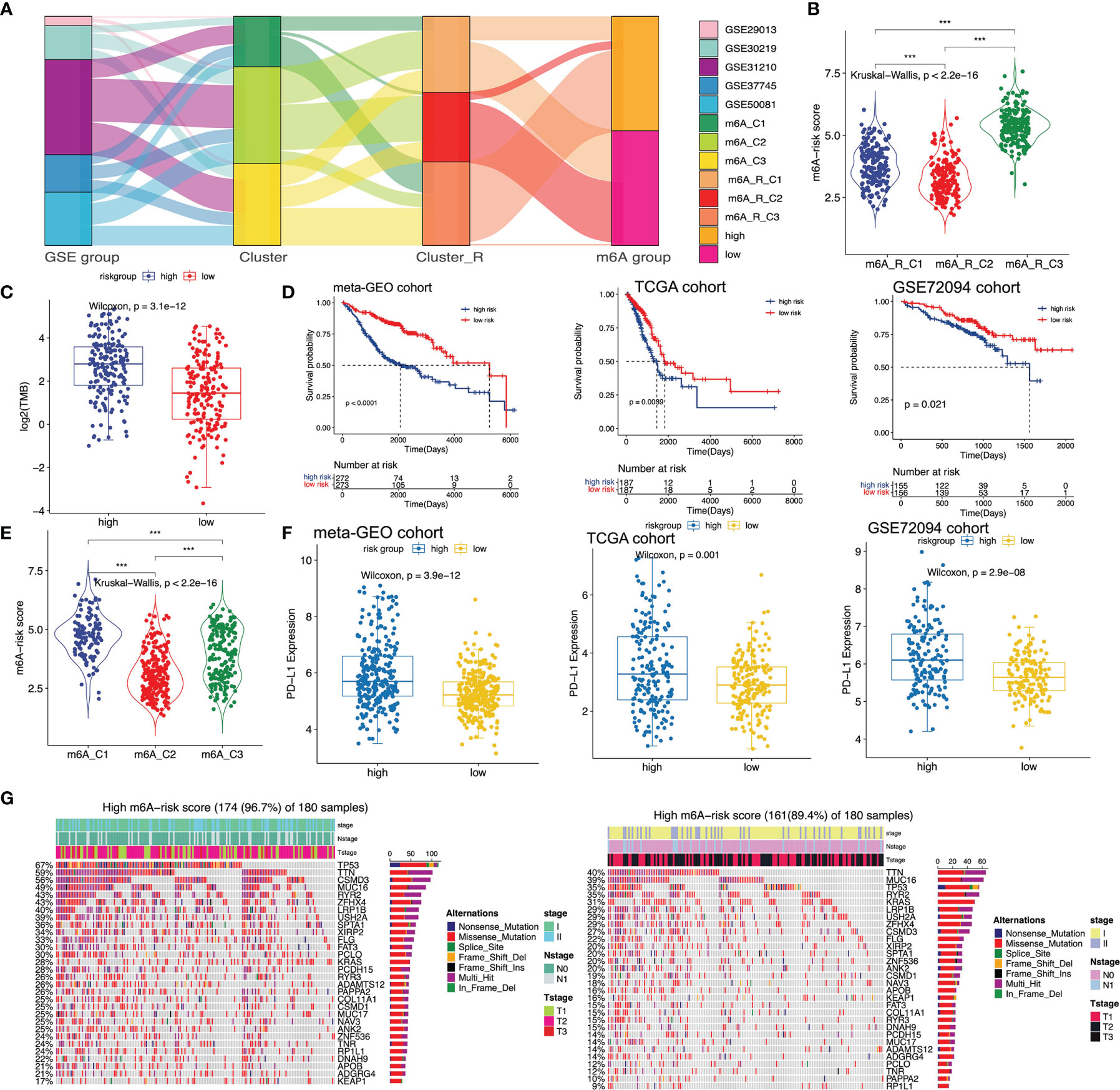
Figure 4 Establishment of m6A-predictive score and evaluation its correlated clinical landscapes. (A) Sankey diagram of different GSE groups with various m6A clusters, m6A cluster-Rs and m6A-predictive score. (B) Comparison of m6A-predictive score among three clusters in the meta-GEO cohort (*P < 0.05; **P < 0.01; ***P < 0.001). (C) Comparison of tumor mutation burden (TMB) between low and high m6A-score groups in TCGA cohort. (D) Kaplan-Meier curves of low and high m6A-score groups in meta-GEO, TCGA and GSE72094 cohorts via Log-rank test. (E) Comparison of m6A-predictive score among three clusters in the meta-GEO cohort (*P < 0.05; ** P< 0.01; ***P < 0.001). (F) Distribution of PD-L1 expression levels in different m6A-score groups of meta-GEO, TCGA and GSE72094 cohorts. (G) Mutational features of the top mutated genes in low and high m6A-score groups in the TCGA cohort. The sample annotations were shown in the upper part of the diagram, including stage, Tstage and Nstage.
Next, we validated the m6A-predictive score with two independent cohorts, the TCGA cohort and the GSE72094 cohort. We first calculated the m6A-predictive score and divided each cohort into low- and high-score subgroups in TCGA (Figure S7D) and GSE72094 cohorts (Figure S7F). Log-rank test was then utilized to determine the correlation of m6A-predictive score and the clinical outcomes in both cohorts. We found that in the TCGA and GSE72094 cohorts, the increase of predictive score was related to poor clinical outcomes (Figure 4D, middle and right panel). Also, we evaluated the efficacy of the m6A-predictive score and the results revealed that 1-, 3- and 5-year AUCs were 0.6, 0.61 and 0.63 in the TCGA cohort (Figure S7C), 0.69, 0.63 and 0.84 in the GSE72094 cohort, indicating this scoring system’s 5-year predictive efficacy was the highest (Figure S7E). We also found that PD-L1 expression was mainly increased in the high-score group (Figure 4F, middle and right panel). Recent studies have found that TMB can play an essential role in predicting the response of immunotherapy (54). Thus, we further explored the correlation of m6A-predictive score and TMB in the TCGA cohort. Our results revealed that patients of the high-score group had higher TMB (Figure 4C). Besides, we analyzed the top mutational genes in these two subgroups respectively. The mutational feature indicated that genes in the high-score group had higher mutational rates than the other (Figure 4G). The top three genes in the high-score group were TP53 (67%), TTN (59%) and CSMD3 (56%), while those in the other group were TTN (40%), MUC16 (39%) and TP53 (35%). In a word, we comprehensively evaluated the interaction between m6A-predictive score and somatic mutations.
We then integrated clinical parameters and m6A-predictive score to build a nomogram, which aimed to elevate the accuracy and reliability of the predictive model in early-stage LUAD. Univariate and multivariate Cox regression models were utilized in the meta-GEO cohort, and the results revealed that age, stage and m6A-predictive score were remarkedly related to patients’ OS (Figure S8A). Then we built the nomogram according to the above three clinical characteristics (Figure S8C). And we compared the C-index among all of the selected clinical parameters, which indicated that the nomogram had the highest C-index (Figure S8B). Additionally, the calibration plots revealed the great concordance between the prognosis and our nomogram, indicating our nomogram served as an essential factor to predict clinical outcomes of early-stage LUAD patients (Figure S8E). Furthermore, the time-dependent ROC curves were utilized to evaluate the accuracy of our nomogram, which showed that 1-, 3- and 5-year AUCs were 0.68, 0.74 and 0.75, respectively (Figure S8D).
Application of m6A-Predictive Score in Predicting the Immunotherapeutic Effect
To explore the detailed relationship between m6A-predictive score and tumor immunity, we compared the immune scores between low- and high-score subgroups in the meta-GEO cohort. The results indicated that ESTIMATEScore and ImmuneScore of the high-score group were predominantly higher, while there was no difference of StromalScore between the two groups (Figure 5A). We then calculated the fraction of immune infiltrating cells between these two subgroups in the meta-GEO cohort via the different algorithms. Using the CIBERSORT algorithms, the results implied that the fraction of macrophages M0, macrophages M1, mast cells activated, neutrophils, plasma cells, T cells CD8 and T cells CD4 memory activated were relatively higher in the high-score group, whereas dendritic cells resting, eosinophils, monocytes, mast cells resting, T cells gamma delta, T cells follicular helper, T cells CD4 memory resting, NK cells resting, and NK cells activated were lower (Figure 5E). And the results of the MCPcounter algorithms implied that the fraction of B lineage, CD8 T cells, cytotoxic lymphocytes, fibroblasts, monocytic lineage and NK cells were higher in the high-score group, whereas the fraction of endothelial cells, neutrophils, myeloid dendritic cells were lower (Figure 5G). Besides, we performed the GSEA enrichment analysis of the high-score subgroup, which revealed that mitotic cell cycle, regulation of cell cycle and cell cycle process were the top three biological processes enriched (Figure 5F).
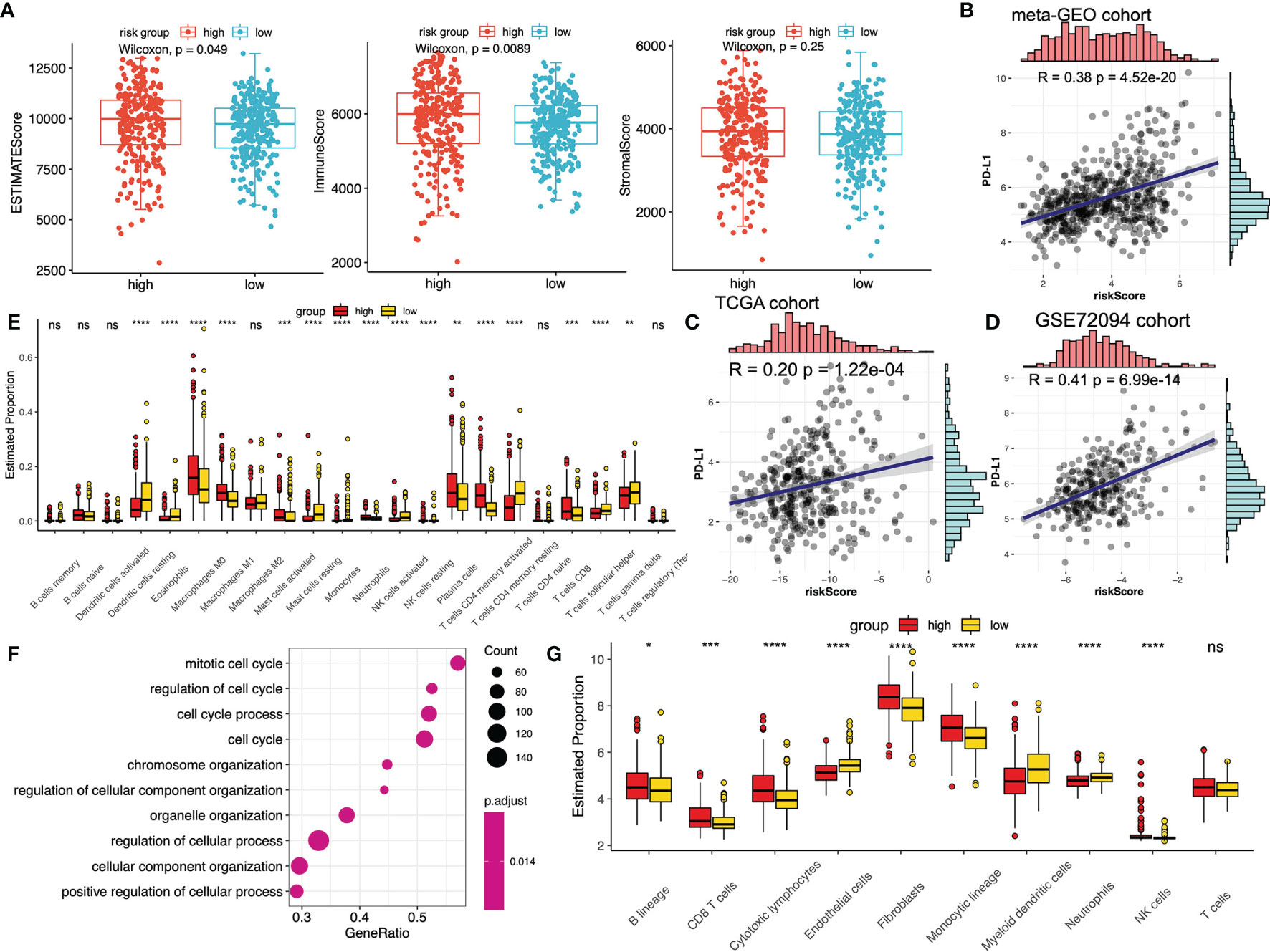
Figure 5 The relationship between m6A-predictive score and immune infiltration. (A) Distribution of different immune scores (ESTIMATEScore, ImmuneScore and StromalScore) in low and high m6A-score groups. (B–D) The correlation analysis of PD-L1 expression levels and m6A-predictive score via Spearman correlation method in meta-GEO cohort, TCGA cohort and GSE72094 cohort. (E) The estimated fraction of different immune infiltrating cells calculated by CIBERSORT algorithm in different m6A-score groups (*P < 0.05; **P < 0.01; ***P < 0.001; ****P < 0.0001). (F) GSEA enrichment analysis (GO) of the top differentially expressed genes in the group with a high m6A-predictive score. (G) The estimated fraction of different immune infiltrating cells calculated by MCPcounter algorithm in different m6A-score groups. ns, not significant.
Immune checkpoint inhibitors (ICIs) treatment was one of the emerging immunotherapies, which was widely used in clinical practice (55). Some prevalent ICIs have been already utilized to treat cancer patients, including PD-1/L1 and CTLA-4 (21, 56). In addition, some recent studies have indicated that IPS could be regarded as a novel predictor to predict immunotherapeutic responses. Thus, we first performed the correlation analysis to evaluate the correlation of m6A-predictive score and PD-L1 expression. Our study found that PD-L1 expression was strongly related to m6A-predictive score in meta-GEO cohort (Figure 5B, R = 0.38, P = 4.52e-20), TCGA cohort (Figure 5C, R = 0.20, P =1.22e-04) and GSE72094 cohort (Figure 5D, R = 0.41, P = 6.99e-14). Also, we analyzed the correlation of other two ICIs (PD-1 and CTLA-4) and the m6A-predictive score. Our results implied that CTLA-4 expression was increased in the high-score group of the meta-GEO cohort and PD-1 expression was relatively higher in the GSE72094 cohort (Figure 6B). And m6A-predictive score was correlated with CTLA-4 (R = 0.32, P = 1.24e-14) and PD-1 expression (R = 0.16, P = 0.005) in meta-GEO and GSE72094 respectively (Figure 6C). We then evaluated the distribution of the IPS score between these two subgroups, which revealed that the IPS score was predominantly higher in the low-score group in the meta-GEO and TCGA cohorts (Figure 6A). Furthermore, due to the correlation of immune response and m6A-predictive score, we applied our m6A-predictive score to evaluate its predictive value in an anti-PD-1 cohort (49) (study of Gide et al.) and an adoptive T cell therapy cohort (50) (study of Lauss et al.). Based on the log-rank test, our results revealed that patients with low m6A-predictive scores exhibited prolonged OS and PFS in the anti-PD-1 cohort (Figure 6D). On the contrary, the prognosis of patients with the low m6A-predictive score was poor in the adoptive T cell therapy cohort (Figure 6E). In conclusion, the above findings indicated that our m6A-predictive score was significantly related to immunotherapies and could serve as a crucial factor in predicting patients’ prognoses.
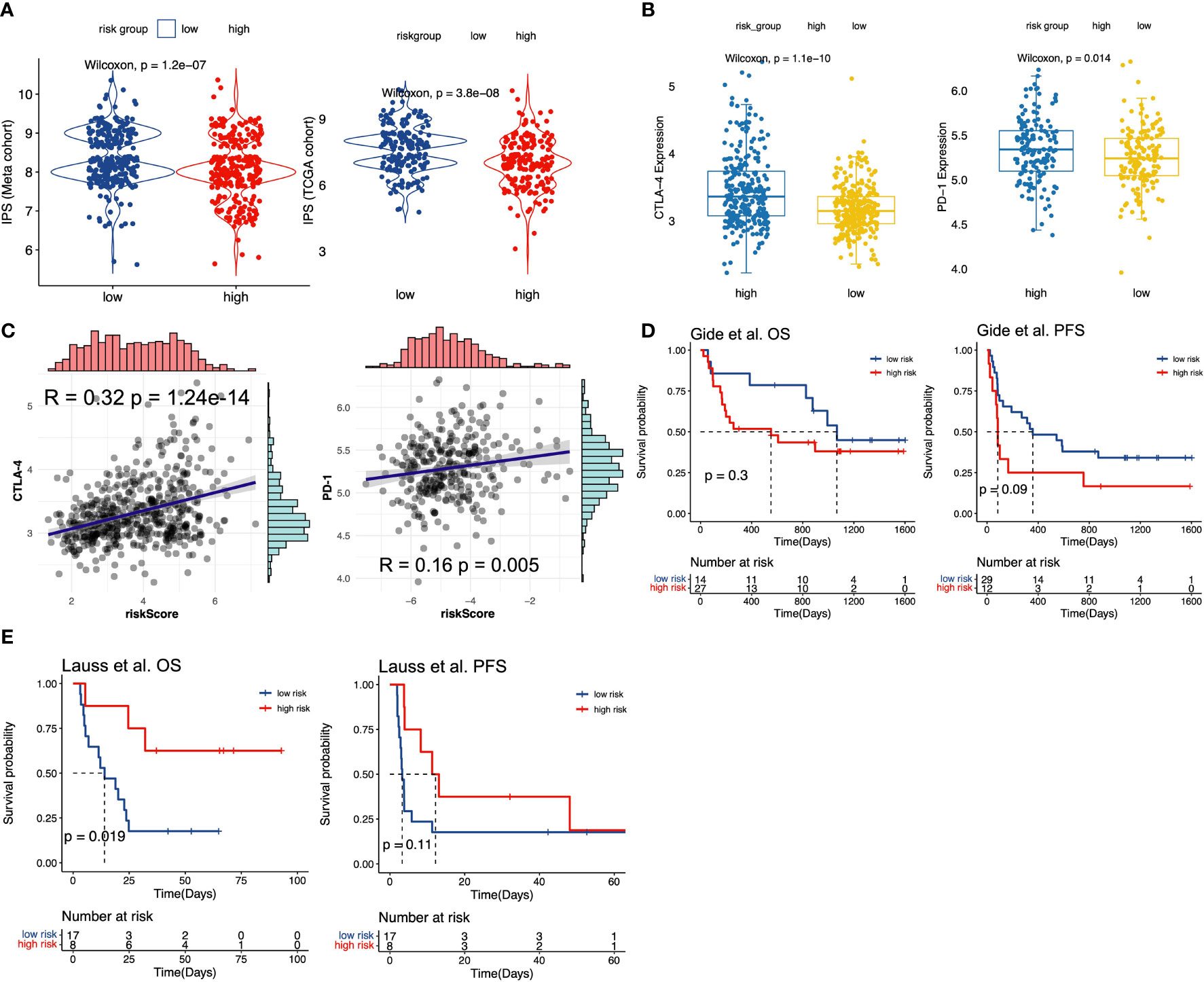
Figure 6 The value of m6A-predictive score to predict patients’ clinical outcomes with immunotherapy. (A) Comparison of IPS scores between low and high m6A-score groups in meta-GEO and TCGA cohorts. (B) The distribution of CTLA-4 expression levels between low and high m6A-score groups in meta-GEO cohort. The distribution of PD-1 expression levels between low and high m6A-score groups in GSE72094 cohort. (C) The Spearman correlation analysis of CTLA-4 and predictive score in meta-GEO cohort. The Spearman correlation analysis of PD-1 and predictive score in GSE72094 cohort. (D) Kaplan-Meier curves for low and high m6A-score groups in Gide et al. (49) cohort. (E) Kaplan-Meier curves for low and high m6A-score groups in Lauss et al. (50) cohort.
Discussion
Abundant evidence revealed that m6A modification served as a critically essential factor in tumor immunity and could regulate malignant behaviors via the complex interaction among different m6A regulatory proteins (57, 58). Previous publications have investigated the role of several m6A regulators in the TME, but no study has systematically explored the whole TME characteristics recognized by diverse m6A regulators in early-stage LUAD. Thus, classifying different m6A modification clusters in the TME could expand our knowledge of the more comprehensive association between anti-tumor immunotherapy and m6A modification, which may promote the development of more efficient and reliable immunotherapy strategies.
In the present study, we have identified three distinct m6A clusters according to 23 m6A regulators’ expression levels, which were classified by different biological processes. Our results implied that the m6A-C1 was classified through multiple tumor-related biological processes (angiogenesis, EMT and hypoxia) and biomarkers (MYC and E2F); m6A-C2 was classified through several pathways correlated with tumorigenesis and progression, such as Wnt, TGF-beta and Notch pathways. Emerging evidence indicated that TME could play an essential role in the occurrence and development of tumors, which can also affect the immunotherapeutic responses (18, 59). Moreover, studies have shown that patients with more abundance of dendritic cells (60), NK cells (61) and T follicular helper cells (62) were more likely to respond to immunotherapy with ICIs. The m6A-C2 was mostly identified with its high infiltrating levels of the above immune cells, suggesting its potential strengths in predicting immunotherapeutic responses. And the m6A-C2 was also proven to have the best prognosis among three m6A modification clusters. Previous publications have demonstrated that the activation of some targets (MYC and E2F) was closely related to tumorigenesis and metastasis (63, 64). In addition, studies have revealed that EMT and hypoxia were related to poor prognosis and may be highly correlated with TME (65, 66). These biological processes and some specific immune infiltrating cells were enriched in m6A-C1, and the survival analysis showed that patients of m6A-C1 had unfavorable prognoses. Integrating the biological processes and TME characteristics of different m6A clusters, our stratification was proven to be reliable and may foster the research of immunotherapy in early-stage LUAD.
Next, we analyzed the biological processes of the overlapping DEGs among the three m6A modification clusters, and the results revealed that RNA segregation and nuclear division were enriched, indicating the overlapping DEGs could be considered as an m6A-correlated signature. Three m6A cluster-Rs were then identified based on the overlapping DEGs, which also exhibited a strong correlation with prognosis and TME characteristics. The results revealed that m6A modification could serve as a significant factor in classifying patients with different TME features. The m6A-R-C2 was rarely related to immunity and had the best clinical outcomes, whereas m6A-R-C3 was the opposite. However, due to the heterogeneity of each patient, using m6A clusters or cluster-Rs to stratify patients into independent groups was not convenient and effective. Therefore, we constructed a scoring system (m6A-predictive score) to accurately quantify m6A modified subgroups. The m6A-C1 with relatively low immune infiltrating levels had a higher m6A-predictive score, while the m6A-C1 with abundant immune infiltrating cells had a lower m6A-predictive score. We further evaluated the exact role of the m6A-predictive score, which showed that this score could be an effective prognostic predictor in the training and validation cohorts of early-stage LUAD. In addition, our results implied a strong relationship between this score and TMB, somatic mutational rate, immune-related score and immune response predictors (IPS, PD-1, PD-L1 and CTLA-4). The findings suggested m6A-predictive score was a robust and reliable scoring system, which can be used to define the m6A modification subgroups in independent cohorts, and the scoring system was also associated with specific immune features. Moreover, we used this scoring system to evaluate its predictive efficacy in two immunotherapy cohorts. Interestingly, our study found that patients’ clinical outcomes with low m6A-predictive scores were favorable in the anti-PD-1 cohort, while the prognosis was poor in the adoptive T cell therapy cohort. The results implied the different performance of our m6A-predictive score in different kinds of immunotherapy cohorts, suggesting its precision of predicting immunotherapeutic responses.
Due to LRPPRC being negatively correlated with most of the immune infiltrating cells, we then analyzed its role in modulating the TME. Previous publications have revealed that LRPPRC was involved in multiple physiological and pathological processes, such as energy metabolism (67). As one of the m6A regulatory proteins, LRPPRC expression increased in diverse cancer tissues but decrease in normal tissues (67). To be specific, Tian et al. revealed that upregulation of LRPPRC was associated with growth and invasion and was related to poor prognosis in LUAD (68). In our study, we divided patients into two subgroups according to LRPPRC expression and compared the immune landscapes between the low- and high-expression subgroups. We found that immune-related scores and immune checkpoints expression were relatively lower in the high-expression group, which could offer strong evidence that LRPPRC expression was negatively associated with immune infiltration in the TME. Due to the lack of studies of the relationship between LRPPRC and the TME, our work may shed new light on the research of this LRPPRC and provide a new understanding of m6A regulators’ role in the TME.
Somatic mutational genes play a crucial role in tumorigenesis and progression, which are highly related to the diagnosis, treatment and prognosis in various cancers (69, 70). Here, we evaluated the difference of somatic mutation genes between the low- and high-score subgroups. Our results implied that the mutational rates of TP53, TNN and CSMD3 were the top three in the high-score group, whereas TNN, MUC16 and TP53 were the top three mutational genes in the low-score group. Li et al. have reported that MUC16 mutations were strongly correlated with immune-related pathways in gastric cancer (71). Specifically, MUC16 mutations could increase the infiltrating levels of cytotoxic T lymphocytes in the TME (72). And TTN was also proven to be an essential factor in predicting responses of immunotherapy with ICIs (73). TP53 mutations were ubiquitous in various cancers, which could predict responses of anti-PD-1 immunotherapy in LUAD (74, 75). The above gene mutations related to the m6A-predictive score were significantly correlated with tumor immunity, indicating the complex relationship between TME characteristics and m6A modification.
This research provided a novel insight into the interaction of m6A modification and TME characteristics in the early-stage LUAD. However, some limitations still existed in the work. First of all, the results of our bioinformatical research needed to be verified by using clinical trials with comprehensive clinical information. Secondly, to maintain the efficacy and reliability of our scoring system, newly identified m6A regulatory proteins were supposed to be integrated into our model in the future. Moreover, different kinds of appropriate immunotherapy cohorts of early-stage LUAD were needed to further validate the accuracy of the m6A-predictive score. In addition, our study was a retrospective study, and we need a prospective clinical trial of early-stage LUAD with immunotherapy to further strengthen our results.
In the present study, we systematically revealed the extensive role of 23 m6A regulators in TME by integrating 1230 early-stage LUAD patients. We found that different phenotypes classified by the m6A modification have distinct immune characteristics, indicating the strong interaction between tumor immunity and m6A modification in early-stage LUAD. Furthermore, a scoring system was established to evaluate the m6A modification phenotypes and the immune infiltration in individual cohorts. The investigation of m6A modification in early-stage LUAD could contribute to a better understanding of TME features and facilitate the development of more promising and potent immunotherapy in the future.
Data Availability Statement
All datasets presented in this study are included in the article/Supplementary Material. GEO data was downloaded from the GEO database (https://www.ncbi.nlm.nih.gov/gds/) under the accession number(s) GSE29013, GSE30219, GSE31210, GSE37745, GSE50081 and GSE72094. TCGA RNA-seq data were downloaded from the TCGA database via the UCSC Xena (https://xena.ucsc.edu/) under the accession number(s) LUAD-FPKM. The copy number alteration data and the DNA methylation data of the TCGA cohort were downloaded from the cBioportal database (https://www.cbioportal.org/). The immunotherapy data were downloaded from the TIDE database (http://tide.dfci.harvard.edu/) under the accession name “Gide et al., Cancer Cell 2019” and “Lauss et al., Nat Commun 2017”. All of the data can also be accessed by contacting with the corresponding authors.
Author Contributions
BZ and SG designed and supervised the study. BZ analyzed the data and wrote the original draft. SG edited the draft. All authors contributed to the article and approved the submitted version.
Funding
The study was funded by Institutional Fundamental Research Funds (2018PT32033), the Ministry of Education Innovation Team Development Project (IRT-17R10) and ETHICON·Excellent in surgery grant (2018-011-ZZ).
Conflict of Interest
The authors declare that the research was conducted in the absence of any commercial or financial relationships that could be construed as a potential conflict of interest.
Publisher’s Note
All claims expressed in this article are solely those of the authors and do not necessarily represent those of their affiliated organizations, or those of the publisher, the editors and the reviewers. Any product that may be evaluated in this article, or claim that may be made by its manufacturer, is not guaranteed or endorsed by the publisher.
Supplementary Material
The Supplementary Material for this article can be found online at: https://www.frontiersin.org/articles/10.3389/fimmu.2021.698236/full#supplementary-material
Abbreviations
m6A, N6-methyladenosine; LUAD, lung adenocarcinoma; NMF, non-negative matrix factorization; NSCLC, non-small cell lung cancer; TME, tumor microenvironment; ICIs, immune checkpoint inhibitors; IFN-γ, interferon-gamma; FPKM, fragments per kilobase million; TPM, transcripts per kilobase million; DEGs, differentially expressed genes; GSVA, Gene set variation analysis; GSEA, Gene Set Enrichment Analysis; GO, Gene Ontology; KEGG, Kyoto Encyclopedia of Genes and Genomes; ssGSEA, Single sample gene set enrichment analysis; IPS, Immunophenoscore; RF, random forest; ROC, receiver operating characteristic.
References
1. Shi J, Hua X, Zhu B, Ravichandran S, Wang M, Nguyen C, et al. Somatic Genomics and Clinical Features of Lung Adenocarcinoma: A Retrospective Study. PloS Med (2016) 13(12):e1002162. doi: 10.1371/journal.pmed.1002162
2. National Lung Screening Trial Research Team. Lung Cancer Incidence and Mortality With Extended Follow-Up in the National Lung Screening Trial. J Thorac Oncol (2019) 14(10):1732–42. doi: 10.1016/j.jtho.2019.05.044
3. Siegel RL, Miller KD, Jemal A. Cancer Statistics, 2020. CA Cancer J Clin (2020) 70(1):7–30. doi: 10.3322/caac.21590
4. Ettinger DS, Wood DE, Aggarwal C, Aisner DL, Akerley W, Bauman JR, et al. NCCN Guidelines Insights: Non-Small Cell Lung Cancer, Version 1.2020. J Natl Compr Canc Netw (2019) 17(12):1464–72. doi: 10.6004/jnccn.2019.0059
5. Li F, Huang Q, Luster TA, Hu H, Zhang H, Ng WL, et al. In Vivo Epigenetic CRISPR Screen Identifies Asf1a as an Immunotherapeutic Target in Kras-Mutant Lung Adenocarcinoma. Cancer Discov (2020) 10(2):270–87. doi: 10.1158/2159-8290.Cd-19-0780
6. Xu JY, Zhang C, Wang X, Zhai L, Ma Y, Mao Y, et al. Integrative Proteomic Characterization of Human Lung Adenocarcinoma. Cell (2020) 182(1):245–61.e17. doi: 10.1016/j.cell.2020.05.043
7. Field JK, Devaraj A, Duffy SW, Baldwin DR. CT Screening for Lung Cancer: Is the Evidence Strong Enough? Lung Cancer (2016) 91:29–35. doi: 10.1016/j.lungcan.2015.11.003
8. Aberle DR, Adams AM, Berg CD, Black WC, Clapp JD, Fagerstrom RM, et al. Reduced Lung-Cancer Mortality With Low-Dose Computed Tomographic Screening. N Engl J Med (2011) 365(5):395–409. doi: 10.1056/NEJMoa1102873
9. Bai R, Li L, Chen X, Chen N, Song W, Cui J. Neoadjuvant and Adjuvant Immunotherapy: Opening New Horizons for Patients With Early-Stage Non-Small Cell Lung Cancer. Front Oncol (2020) 10:575472. doi: 10.3389/fonc.2020.575472
10. Rosner S, Reuss JE, Forde PM. PD-1 Blockade in Early-Stage Lung Cancer. Annu Rev Med (2019) 70:425–35. doi: 10.1146/annurev-med-050217-025205
11. Dominissini D, Moshitch-Moshkovitz S, Schwartz S, Salmon-Divon M, Ungar L, Osenberg S, et al. Topology of the Human and Mouse M6a RNA Methylomes Revealed by M6a-Seq. Nature (2012) 485(7397):201–6. doi: 10.1038/nature11112
12. Zhang H, Shi X, Huang T, Zhao X, Chen W, Gu N, et al. Dynamic Landscape and Evolution of M6a Methylation in Human. Nucleic Acids Res (2020) 48(11):6251–64. doi: 10.1093/nar/gkaa347
13. Zaccara S, Ries RJ, Jaffrey SR. Reading, Writing and Erasing mRNA Methylation. Nat Rev Mol Cell Biol (2019) 20(10):608–24. doi: 10.1038/s41580-019-0168-5
14. Wang T, Kong S, Tao M, Ju S. The Potential Role of RNA N6-Methyladenosine in Cancer Progression. Mol Cancer (2020) 19(1):88. doi: 10.1186/s12943-020-01204-7
15. He L, Li H, Wu A, Peng Y, Shu G, Yin G. Functions of N6-Methyladenosine and its Role in Cancer. Mol Cancer (2019) 18(1):176. doi: 10.1186/s12943-019-1109-9
16. Ma S, Chen C, Ji X, Liu J, Zhou Q, Wang G, et al. The Interplay Between M6a RNA Methylation and Noncoding RNA in Cancer. J Hematol Oncol (2019) 12(1):121. doi: 10.1186/s13045-019-0805-7
17. Jin D, Guo J, Wu Y, Du J, Yang L, Wang X, et al. M6A mRNA Methylation Initiated by METTL3 Directly Promotes YAP Translation and Increases YAP Activity by Regulating the MALAT1-miR-1914-3p-YAP Axis to Induce NSCLC Drug Resistance and Metastasis. J Hematol Oncol (2019) 12(1):135. doi: 10.1186/s13045-019-0830-6
18. Quail DF, Joyce JA. Microenvironmental Regulation of Tumor Progression and Metastasis. Nat Med (2013) 19(11):1423–37. doi: 10.1038/nm.3394
19. Brahmer JR, Drake CG, Wollner I, Powderly JD, Picus J, Sharfman WH, et al. Phase I Study of Single-Agent Anti-Programmed Death-1 (MDX-1106) in Refractory Solid Tumors: Safety, Clinical Activity, Pharmacodynamics, and Immunologic Correlates. J Clin Oncol (2010) 28(19):3167–75. doi: 10.1200/jco.2009.26.7609
20. Hanna NH, Schneider BJ, Temin S, Baker S Jr, Brahmer J, Ellis PM, et al. Therapy for Stage IV Non-Small-Cell Lung Cancer Without Driver Alterations: ASCO and OH (CCO) Joint Guideline Update. J Clin Oncol (2020) 38(14):1608–32. doi: 10.1200/jco.19.03022
21. Chae YK, Arya A, Iams W, Cruz MR, Chandra S, Choi J, et al. Current Landscape and Future of Dual Anti-CTLA4 and PD-1/PD-L1 Blockade Immunotherapy in Cancer; Lessons Learned From Clinical Trials With Melanoma and non-Small Cell Lung Cancer (NSCLC). J Immunother Cancer (2018) 6(1):39. doi: 10.1186/s40425-018-0349-3
22. Zhang Y, Yang M, Ng DM, Haleem M, Yi T, Hu S, et al. Multi-Omics Data Analyses Construct TME and Identify the Immune-Related Prognosis Signatures in Human LUAD. Mol Ther Nucleic Acids (2020) 21:860–73. doi: 10.1016/j.omtn.2020.07.024
23. Kadara H, Choi M, Zhang J, Parra ER, Rodriguez-Canales J, Gaffney SG, et al. Whole-Exome Sequencing and Immune Profiling of Early-Stage Lung Adenocarcinoma With Fully Annotated Clinical Follow-Up. Ann Oncol (2017) 28(1):75–82. doi: 10.1093/annonc/mdw436
24. Su R, Dong L, Li Y, Gao M, Han L, Wunderlich M, et al. Targeting FTO Suppresses Cancer Stem Cell Maintenance and Immune Evasion. Cancer Cell (2020) 38(1):79–96.e11. doi: 10.1016/j.ccell.2020.04.017
25. Han D, Liu J, Chen C, Dong L, Liu Y, Chang R, et al. Anti-Tumour Immunity Controlled Through mRNA M(6)A Methylation and YTHDF1 in Dendritic Cells. Nature (2019) 566(7743):270–4. doi: 10.1038/s41586-019-0916-x
26. Xie Y, Xiao G, Coombes KR, Behrens C, Solis LM, Raso G, et al. Robust Gene Expression Signature From Formalin-Fixed Paraffin-Embedded Samples Predicts Prognosis of non-Small-Cell Lung Cancer Patients. Clin Cancer Res (2011) 17(17):5705–14. doi: 10.1158/1078-0432.Ccr-11-0196
27. Rousseaux S, Debernardi A, Jacquiau B, Vitte AL, Vesin A, Nagy-Mignotte H, et al. Ectopic Activation of Germline and Placental Genes Identifies Aggressive Metastasis-Prone Lung Cancers. Sci Transl Med (2013) 5(186):186ra66. doi: 10.1126/scitranslmed.3005723
28. Okayama H, Kohno T, Ishii Y, Shimada Y, Shiraishi K, Iwakawa R, et al. Identification of Genes Upregulated in ALK-Positive and EGFR/KRAS/ALK-Negative Lung Adenocarcinomas. Cancer Res (2012) 72(1):100–11. doi: 10.1158/0008-5472.Can-11-1403
29. Botling J, Edlund K, Lohr M, Hellwig B, Holmberg L, Lambe M, et al. Biomarker Discovery in Non-Small Cell Lung Cancer: Integrating Gene Expression Profiling, Meta-Analysis, and Tissue Microarray Validation. Clin Cancer Res (2013) 19(1):194–204. doi: 10.1158/1078-0432.Ccr-12-1139
30. Der SD, Sykes J, Pintilie M, Zhu CQ, Strumpf D, Liu N, et al. Validation of a Histology-Independent Prognostic Gene Signature for Early-Stage, Non-Small-Cell Lung Cancer Including Stage IA Patients. J Thorac Oncol (2014) 9(1):59–64. doi: 10.1097/jto.0000000000000042
31. Schabath MB, Welsh EA, Fulp WJ, Chen L, Teer JK, Thompson ZJ, et al. Differential Association of STK11 and TP53 With KRAS Mutation-Associated Gene Expression, Proliferation and Immune Surveillance in Lung Adenocarcinoma. Oncogene (2016) 35(24):3209–16. doi: 10.1038/onc.2015.375
32. Mayakonda A, Lin DC, Assenov Y, Plass C, Koeffler HP. Maftools: Efficient and Comprehensive Analysis of Somatic Variants in Cancer. Genome Res (2018) 28(11):1747–56. doi: 10.1101/gr.239244.118
33. Leek JT, Johnson WE, Parker HS, Jaffe AE, Storey JD. The Sva Package for Removing Batch Effects and Other Unwanted Variation in High-Throughput Experiments. Bioinformatics (2012) 28(6):882–3. doi: 10.1093/bioinformatics/bts034
34. Chen XY, Zhang J, Zhu JS. The Role of M6A RNA Methylation in Human Cancer. Mol Cancer (2019) 18(1):103. doi: 10.1186/s12943-019-1033-z
35. Zhao BS, Roundtree IA, He C. Post-Transcriptional Gene Regulation by mRNA Modifications. Nat Rev Mol Cell Biol (2017) 18(1):31–42. doi: 10.1038/nrm.2016.132
36. Li Y, Xiao J, Bai J, Tian Y, Qu Y, Chen X, et al. Molecular Characterization and Clinical Relevance of M(6)A Regulators Across 33 Cancer Types. Mol Cancer (2019) 18(1):137. doi: 10.1186/s12943-019-1066-3
37. Niu Y, Zhao X, Wu YS, Li MM, Wang XJ, Yang YG. N6-Methyl-Adenosine (M6A) in RNA: An Old Modification With a Novel Epigenetic Function. Genomics Proteomics Bioinf (2013) 11(1):8–17. doi: 10.1016/j.gpb.2012.12.002
38. Gaujoux R, Seoighe C. A Flexible R Package for Nonnegative Matrix Factorization. BMC Bioinf (2010) 11:367. doi: 10.1186/1471-2105-11-367
39. Hänzelmann S, Castelo R, Guinney J. GSVA: Gene Set Variation Analysis for Microarray and RNA-Seq Data. BMC Bioinf (2013) 14:7. doi: 10.1186/1471-2105-14-7
40. Subramanian A, Tamayo P, Mootha VK, Mukherjee S, Ebert BL, Gillette MA, et al. Gene Set Enrichment Analysis: A Knowledge-Based Approach for Interpreting Genome-Wide Expression Profiles. Proc Natl Acad Sci USA (2005) 102(43):15545–50. doi: 10.1073/pnas.0506580102
41. Yu G, Wang LG, Han Y, He QY. Clusterprofiler: An R Package for Comparing Biological Themes Among Gene Clusters. Omics (2012) 16(5):284–7. doi: 10.1089/omi.2011.0118
42. Newman AM, Liu CL, Green MR, Gentles AJ, Feng W, Xu Y, et al. Robust Enumeration of Cell Subsets From Tissue Expression Profiles. Nat Methods (2015) 12(5):453–7. doi: 10.1038/nmeth.3337
43. Becht E, Giraldo NA, Lacroix L, Buttard B, Elarouci N, Petitprez F, et al. Estimating the Population Abundance of Tissue-Infiltrating Immune and Stromal Cell Populations Using Gene Expression. Genome Biol (2016) 17(1):218. doi: 10.1186/s13059-016-1070-5
44. Charoentong P, Finotello F, Angelova M, Mayer C, Efremova M, Rieder D, et al. Pan-Cancer Immunogenomic Analyses Reveal Genotype-Immunophenotype Relationships and Predictors of Response to Checkpoint Blockade. Cell Rep (2017) 18(1):248–62. doi: 10.1016/j.celrep.2016.12.019
45. Yoshihara K, Shahmoradgoli M, Martínez E, Vegesna R, Kim H, Torres-Garcia W, et al. Inferring Tumour Purity and Stromal and Immune Cell Admixture From Expression Data. Nat Commun (2013) 4:2612. doi: 10.1038/ncomms3612
46. Ritchie ME, Phipson B, Wu D, Hu Y, Law CW, Shi W, et al. Limma Powers Differential Expression Analyses for RNA-Sequencing and Microarray Studies. Nucleic Acids Res (2015) 43(7):e47. doi: 10.1093/nar/gkv007
47. Camp RL, Dolled-Filhart M, Rimm DL. X-Tile: A New Bio-Informatics Tool for Biomarker Assessment and Outcome-Based Cut-Point Optimization. Clin Cancer Res (2004) 10(21):7252–9. doi: 10.1158/1078-0432.Ccr-04-0713
48. Fu J, Li K, Zhang W, Wan C, Zhang J, Jiang P, et al. Large-Scale Public Data Reuse to Model Immunotherapy Response and Resistance. Genome Med (2020) 12(1):21. doi: 10.1186/s13073-020-0721-z
49. Gide TN, Quek C, Menzies AM, Tasker AT, Shang P, Holst J, et al. Distinct Immune Cell Populations Define Response to Anti-PD-1 Monotherapy and Anti-PD-1/Anti-CTLA-4 Combined Therapy. Cancer Cell (2019) 35(2):238–55.e6. doi: 10.1016/j.ccell.2019.01.003
50. Lauss M, Donia M, Harbst K, Andersen R, Mitra S, Rosengren F, et al. Mutational and Putative Neoantigen Load Predict Clinical Benefit of Adoptive T Cell Therapy in Melanoma. Nat Commun (2017) 8(1):1738. doi: 10.1038/s41467-017-01460-0
51. Guo X, Zhang Y, Zheng L, Zheng C, Song J, Zhang Q, et al. Global Characterization of T Cells in Non-Small-Cell Lung Cancer by Single-Cell Sequencing. Nat Med (2018) 24(7):978–85. doi: 10.1038/s41591-018-0045-3
52. Shen X, Zhao B. Efficacy of PD-1 or PD-L1 Inhibitors and PD-L1 Expression Status in Cancer: Meta-Analysis. BMJ (2018) 362:k3529. doi: 10.1136/bmj.k3529
53. Rowshanravan B, Halliday N, Sansom DM. CTLA-4: A Moving Target in Immunotherapy. Blood (2018) 131(1):58–67. doi: 10.1182/blood-2017-06-741033
54. Samstein RM, Lee CH, Shoushtari AN, Hellmann MD, Shen R, Janjigian YY, et al. Tumor Mutational Load Predicts Survival After Immunotherapy Across Multiple Cancer Types. Nat Genet (2019) 51(2):202–6. doi: 10.1038/s41588-018-0312-8
55. Galluzzi L, Humeau J, Buqué A, Zitvogel L, Kroemer G. Immunostimulation With Chemotherapy in the Era of Immune Checkpoint Inhibitors. Nat Rev Clin Oncol (2020) 17(12):725–41. doi: 10.1038/s41571-020-0413-z
56. Santarpia M, Aguilar A, Chaib I, Cardona AF, Fancelli S, Laguia F, et al. Non-Small-Cell Lung Cancer Signaling Pathways, Metabolism, and PD-1/PD-L1 Antibodies. Cancers (Basel) (2020) 12(6):1475. doi: 10.3390/cancers12061475
57. Shulman Z, Stern-Ginossar N. The RNA Modification N(6)-Methyladenosine as a Novel Regulator of the Immune System. Nat Immunol (2020) 21(5):501–12. doi: 10.1038/s41590-020-0650-4
58. Winkler R, Gillis E, Lasman L, Safra M, Geula S, Soyris C, et al. M6A Modification Controls the Innate Immune Response to Infection by Targeting Type I Interferons. Nat Immunol (2019) 20(2):173–82. doi: 10.1038/s41590-018-0275-z
59. Ngambenjawong C, Gustafson HH, Pun SH. Progress in Tumor-Associated Macrophage (TAM)-Targeted Therapeutics. Adv Drug Deliv Rev (2017) 114:206–21. doi: 10.1016/j.addr.2017.04.010
60. Wculek SK, Cueto FJ, Mujal AM, Melero I, Krummel MF, Sancho D. Dendritic Cells in Cancer Immunology and Immunotherapy. Nat Rev Immunol (2020) 20(1):7–24. doi: 10.1038/s41577-019-0210-z
61. Shimasaki N, Jain A, Campana D. NK Cells for Cancer Immunotherapy. Nat Rev Drug Discov (2020) 19(3):200–18. doi: 10.1038/s41573-019-0052-1
62. Hollern DP, Xu N, Thennavan A, Glodowski C, Garcia-Recio S, Mott KR, et al. B Cells and T Follicular Helper Cells Mediate Response to Checkpoint Inhibitors in High Mutation Burden Mouse Models of Breast Cancer. Cell (2019) 179(5):1191–206.e21. doi: 10.1016/j.cell.2019.10.028
63. Kent LN, Leone G. The Broken Cycle: E2F Dysfunction in Cancer. Nat Rev Cancer (2019) 19(6):326–38. doi: 10.1038/s41568-019-0143-7
64. Gabay M, Li Y, Felsher DW. MYC Activation is a Hallmark of Cancer Initiation and Maintenance. Cold Spring Harb Perspect Med (2014) 4(6):a014241. doi: 10.1101/cshperspect.a014241
65. Jing X, Yang F, Shao C, Wei K, Xie M, Shen H, et al. Role of Hypoxia in Cancer Therapy by Regulating the Tumor Microenvironment. Mol Cancer (2019) 18(1):157. doi: 10.1186/s12943-019-1089-9
66. Erin N, Grahovac J, Brozovic A, Efferth T. Tumor Microenvironment and Epithelial Mesenchymal Transition as Targets to Overcome Tumor Multidrug Resistance. Drug Resist Update (2020) 53:100715. doi: 10.1016/j.drup.2020.100715
67. Cui J, Wang L, Ren X, Zhang Y, Zhang H. LRPPRC: A Multifunctional Protein Involved in Energy Metabolism and Human Disease. Front Physiol (2019) 10:595. doi: 10.3389/fphys.2019.00595
68. Tian T, Ikeda J, Wang Y, Mamat S, Luo W, Aozasa K, et al. Role of Leucine-Rich Pentatricopeptide Repeat Motif-Containing Protein (LRPPRC) for Anti-Apoptosis and Tumourigenesis in Cancers. Eur J Cancer (2012) 48(15):2462–73. doi: 10.1016/j.ejca.2012.01.018
69. Ding L, Getz G, Wheeler DA, Mardis ER, McLellan MD, Cibulskis K, et al. Somatic Mutations Affect Key Pathways in Lung Adenocarcinoma. Nature (2008) 455(7216):1069–75. doi: 10.1038/nature07423
70. Pleasance ED, Cheetham RK, Stephens PJ, McBride DJ, Humphray SJ, Greenman CD, et al. A Comprehensive Catalogue of Somatic Mutations From a Human Cancer Genome. Nature (2010) 463(7278):191–6. doi: 10.1038/nature08658
71. Li X, Pasche B, Zhang W, Chen K. Association of MUC16 Mutation With Tumor Mutation Load and Outcomes in Patients With Gastric Cancer. JAMA Oncol (2018) 4(12):1691–8. doi: 10.1001/jamaoncol.2018.2805
72. Hu J, Sun J. MUC16 Mutations Improve Patients' Prognosis by Enhancing the Infiltration and Antitumor Immunity of Cytotoxic T Lymphocytes in the Endometrial Cancer Microenvironment. Oncoimmunology (2018) 7(10):e1487914. doi: 10.1080/2162402x.2018.1487914
73. Jia Q, Wang J, He N, He J, Zhu B. Titin Mutation Associated With Responsiveness to Checkpoint Blockades in Solid Tumors. JCI Insight (2019) 4(10):e127901. doi: 10.1172/jci.insight.127901
74. Donehower LA, Soussi T, Korkut A, Liu Y, Schultz A, Cardenas M, et al. Integrated Analysis of TP53 Gene and Pathway Alterations in The Cancer Genome Atlas. Cell Rep (2019) 28(5):1370–84.e5. doi: 10.1016/j.celrep.2019.07.001
Keywords: m6A (N6-methyladenosine), lung adenocarcinoma, tumor microenvironment, immune infiltration, immunotherapy
Citation: Zhou B and Gao S (2021) Comprehensive Analysis of Clinical Significance, Immune Infiltration and Biological Role of m6A Regulators in Early-Stage Lung Adenocarcinoma. Front. Immunol. 12:698236. doi: 10.3389/fimmu.2021.698236
Received: 21 April 2021; Accepted: 13 September 2021;
Published: 28 September 2021.
Edited by:
Rifat Hamoudi, University of Sharjah, United Arab EmiratesReviewed by:
Zongqiang Huang, First Affiliated Hospital of Zhengzhou University, ChinaJohannes Fahrmann, University of Texas MD Anderson Cancer Center, United States
Copyright © 2021 Zhou and Gao. This is an open-access article distributed under the terms of the Creative Commons Attribution License (CC BY). The use, distribution or reproduction in other forums is permitted, provided the original author(s) and the copyright owner(s) are credited and that the original publication in this journal is cited, in accordance with accepted academic practice. No use, distribution or reproduction is permitted which does not comply with these terms.
*Correspondence: Shugeng Gao, Z2Fvc2h1Z2VuZ0BjaWNhbXMuYWMuY24=