- 1Department of Medical Genetics, University of Oslo and Oslo University Hospital, Oslo, Norway
- 2K. G. Jebsen Inflammation Research Centre, University of Oslo, Oslo, Norway
- 3Department of Rheumatology, Diakonhjemmet Hospital, Oslo, Norway
- 4Department of Rheumatology, Martina Hansens Hospital, Bærum, Norway
- 5Department of Research, Cancer Registry of Norway, Institute for Population-Based Research, Oslo, Norway
- 6Institute of Clinical Molecular Biology, Christian-Albrechts-University of Kiel, Kiel, Germany
- 7Pharmacoepidemiology and Drug Safety Research Group, Department of Pharmacy, University of Oslo, Oslo, Norway
- 8PharmaTox Strategic Research Initiative, Faculty of Mathematics and Natural Sciences, University of Oslo, Oslo, Norway
- 9Division of Clinical Neuroscience, Department of Research and Innovation, Oslo University Hospital, Oslo, Norway
Background: Methotrexate (MTX) is the first line treatment of rheumatoid arthritis (RA), and methylation changes in bulk T cells have been reported after treatment with MTX. We have investigated cell-type specific DNA methylation changes across the genome in naïve and memory CD4+ T cells before and after MTX treatment of RA patients. DNA methylation profiles of newly diagnosed RA patients (N=9) were assessed by reduced representation bisulfite sequencing.
Results: We found that MTX treatment significantly influenced DNA methylation levels at multiple CpG sites in both cell populations. Interestingly, we identified differentially methylated sites annotated to two genes; TRIM15 and SORC2, previously reported to predict treatment outcome in RA patients when measured in bulk T cells. Furthermore, several of the genes, including STAT3, annotated to the significant CpG sites are relevant for RA susceptibility or the action of MTX.
Conclusion: We detected CpG sites that were associated with MTX treatment in CD4+ naïve and memory T cells isolated from RA patients. Several of these sites overlap genetic regions previously associated with RA risk and MTX treatment outcome.
Introduction
Rheumatoid arthritis (RA) is an autoimmune inflammatory disease leading to joint destruction, disability, systemic complications, shorter life expectancy and increased socioeconomic costs. RA can be divided into two major groups based on the presence or absence of antibodies to citrullinated peptide antigens (ACPA) (1). The RA incidence in Norway is 25 per 100 000 per year (2), and the majority of these patients will start treatment with one or more disease-modifying antirheumatic drugs (DMARDs), with the most extensively used being methotrexate (MTX) (2). The mechanisms of action of MTX are not fully understood, but are shown to involve reduced cell proliferation, increased apoptosis of T cells, increased endogenous adenosine release, altered expression of cellular adhesion molecules, changes in cytokine production, humoral responses and bone formation (3). Recently, the low-dose MTX modulation of inflammatory conditions was suggested to act through suppression of JAK/STAT signaling pathways (4). MTX shows good efficacy in a proportion of patients, but beyond two years after starting MTX, a study showed that only 53-71% of RA patients in close clinical follow-up remained on MTX monotherapy (5). A substantial number of patients do not respond adequately to MTX alone, and it is necessary to either discontinue or combine MTX with other drugs to achieve remission. The consequence of suboptimal treatment and delayed remission might be irreversible joint damage (6). Therefore, a treat-to-target approach, consisting of frequent clinical consultations and a quicker introduction of more potent drugs to reduce the disease activity, has been introduced (7). Additionally, biomarkers to predict treatment response is warranted, since the effect of first treatment is strongly associated with long-term outcome in RA patients (8).
The heterogeneity of the underlying biological mechanisms involved in RA is likely influencing the variable treatment outcome. RA is caused by a complex, and yet poorly understood, interplay between genetic, epigenetic and environmental factors. Smoking is, as of yet, the most important environmental risk factor (9, 10), and more than 100 different loci with single nucleotide polymorphisms (SNPs) alleles associated with increased risk of RA (11). Genetic variants have also been reported to be associated with MTX treatment (12) and MTX response in RA (13, 14) and juvenile idiopathic arthritis (JIA) (15).
Already in 1996, Kim et al. showed that inflammatory arthritis was associated with a significant degree of global DNA hypomethylation in peripheral blood mononuclear cells (PBMC) that was reversed with MTX treatment (16). In 2015, global DNA hypomethylation, which was reversed with MTX, was confirmed in bulk T cells from patients with RA (17). However, irrespective of disease, the overall degree of DNA methylation differs between T cell subsets, and a global loss of DNA methylation naturally occurs during differentiation of CD4+ memory cells from CD4+ naïve T cells (18). Furthermore, even though global DNA methylation changes have been reported during MTX treatment in RA patients, no specific genomic regions or loci have been identified yet. However, at diagnosis, DNA methylation levels at 21 CpGs, assessed in T cells using Infinium 450k array, have been reported to be associated with subsequent response to diverse DMARDs, and 1/3 of these patients received MTX monotherapy (19).
In the current study, we aimed to reveal genetic regions where DNA methylation is influenced by MTX treatment in T cell subsets from RA patients. To do this, we conducted an epigenome-wide association study using reduced representation bisulfite sequencing (mRRBS) in naïve and memory CD4+ T cells isolated from newly diagnosed RA patients before and after MTX treatment to identify differentially methylated positions (DMPs).
Material and methods
Subjects
The cohort consists of nine RA patients diagnosed according to the 2010 American College of Rheumatology/European League Against Rheumatism (ACR/EULAR) RA classification criteria (20). Eight patients were included at Diakonhjemmet hospital and one at Martina Hansen’s Hospital between 2014 and 2016 in a clinical cohort; The Norwegian Very Early Arthritis Clinic (NOR-VEAC) observational study (ISRCTN05526276) (21). All RA patients were recruited at the day of diagnosis (newly diagnosed), followed by blood sampling and CD4 T cells isolation on the same day. The patients had not been treated with any DMARDs nor steroids at baseline.
Clinical Data
All patients were clinically examined and gave a blood sample at two timepoints, baseline and a follow-up. The timepoint for the follow-up was three months for N=6 patients and six months for N=3 patients, and they were all clinically examined by a rheumatologist at the time of blood sample withdrawal. The patients’ disease activity was monitored with Disease activity score 28 DAS28 (22), a composite score which includes the clinical assessment of 28 joints, blood values of inflammatory parameters and a score (0–100) from both the clinician and the patient. Depending on the values, the patients were categorized; >5.1: high disease activity, >3.2, but ≤ 5.1: moderate disease activity and ≤ 3.2: low disease activity. If the patient had DAS28 ≤ 2.6, clinical remission was achieved. The EULAR response criteria were used to classify the patients as non-, moderate or good responders to MTX, depending on change and level of the patients’ DAS28 score (23). DAS28 and EULAR response criteria are used to monitor response to treatment and in this study, the disease progression between the two blood withdrawals.
Available clinical data included 28 and 32 swollen joint count, 28 and 32 tender joint count, patient global health, DAS28, rheumatoid factor-status, ACPA-status, c-reactive protein (CRP), erythrocyte sedimentation rate (ESR), gender, age, smoking status and medication (Table 1) at both time points. Radiographs of hands and feet were taken at baseline and follow-up.
Isolation of Immune Cells and DNA Extraction
The isolation of T cells from all samples was initiated within 30 minutes after blood sampling. 200 ml whole blood was collected using a blood bag (Fresenius Kabi, Oslo, Norway) pre-filled with 2 ml 0.5M EDTA (Thermo Fisher Scientific Inc, Massachusetts, USA) and directly diluted in 300 ml PBS (PBS without MgCl, Thermo Fisher Scientific Inc) with 1 ml 1mM EDTA (1 mM) and 10 ml 2% foetal bovine serum (BioNordika, Oslo, Norway). The blood-PBS solution was transferred to 50 ml SepMate™ tubes (STEMcell Technologies, Vancouver, British Columbia, Canada) pre-filled with 14 ml Lymfoprep (Alere, Massachusetts, USA) and centrifuged following the company’s recommendations. The PBMC enriched cell suspension was washed with PBS (0.4% 2 mM EDTA). The CD4+ cells were isolated using EasySep™ Human CD4+CD25+ T Cell Isolation Kit (STEMcell Technologies) with enrichment of CD4+ cells, followed by depletion of CD25+ cells. The CD4+ CD25low cells were separated into CD4+ memory (CD8-, CD14-, CD16-, CD19-, CD20-, CD36-, CD56-, CD123-, TCR gamma/delta, CD66b, glycophorinA, CD25-, CD45RO+) and CD4+ naïve (CD8-, CD14-, CD16-, CD19-, CD20-, CD36-, CD56-, CD123-, TCR gamma/delta, CD66b, glycophorinA, CD25-, CD45RO-) T cells (EasySep™ PE Selection Kit and CD45RO-PE (BioLegend, San Diego, USA). Isolated cells were tested for purity and viability by using BD Accuri™ C6 Cytometer (BD Biosciences, New Jersey, USA). We used Fluorescence Minus One to set the gates.
DNA was isolated from CD4+ memory and CD4+ naïve T cells using RNA/DNA/Protein Purification Plus Kit (Norgen Biotek Corp, Ontario, Canada), and cleaned using QIAamp DNA Micro Kit (Qiagen, Hilden, Germany). The extracted DNA from the CD4+ naïve cells was treated with proteinase K and RNase A (Master-Pure Complete DNA & RNA Purification kit, Epicentre) and we performed clean-up using 1.8x Agencourt Ampure XP beads (Thermo Fisher Scientific). The extracted DNA from CD4+ memory cells was treated with 10 mg/ml proteinase K (Sigma-Aldrich, Missouri, USA) and cleaned with Genomic DNA Clean & Concentrator Kit (Zymo Research, California, USA). Extracted and Proteinase K-treated DNA from both cell types was quantified and qualified by Qubit 2.0 fluormeter dsDNA HS Assay Kit (Thermo Fisher Scientific Inc) and NanoDrop (Model ND1000, software v3.0.0, Thermo Fisher Scientific Inc).
Library Preparation for Multiplexed Reduced Representation Bisulfite Sequencing
The DNA extracted from the CD4+ naïve cells was prepped for multiplexed reduced representation bisulfite sequencing (mRRBS) on Illumina HiSeq3000 according to the Boyle et al. mRRBS protocol (24), using the Diagenode Premium RRBS kit (Diagenode, Seraing, Belgium) (25). The mRRBS libraries (N=18) were sequenced with indexes from the Diagenode Premium RRBS kit as 50 base pair, single end reads with 20% PhiX spike control and 6 samples per lane.
The CD4+ memory cells were prepped for mRRBS on Illumina HiSeq2500 at Institute of Clinical Molecular Biology, Christian-Albrechts-University of Kiel, using an in-house protocol based on Boyle et al. (24) and Gu et al. (26). The 18 libraries were sequenced as 50 base pair single end reads with 10% PhiX spike control and 6 samples per lane.
Achieved Phred quality score was >28 in all reads used from mRRBS sequencing, for both cell populations.
One sample (technical replicate) was sequenced on both platforms (HiSeq 2500 and HiSeq 3000) and was compared to determine how the different sequencing platforms would impact the results. The correlation was 0.9341 for this sample, and hence we found the results to be comparable.
DNA Methylation Analysis
Sequencing Alignment and Quality Control
DNA methylation analyses were carried out using a combination of the programming languages Unix, Python, Java and R (27) with Bioconductor (v2.10) (28). Fastq-files were pre-trimmed and aligned to the current Human Genome version 19 (hg19) with a maximum of two mismatches per read length using BSMAP (29). The methratio.py script was used to calculate DNA methylation percentage per loci. Quality control of sequencing reads was initially performed using FastQC (https://www.bioinformatics.babraham.ac.uk/projects/fastqc). After alignment, the alignment efficiency and specificity of the reads were assessed using the HsMetrics and RrbsSummaryMetrics (Piccard tools, http://broadinstitute.github.io/picard). To reduce the possibility of bias in the analyses, we removed both CpGs on the sex chromosomes and overlapping SNPs, and RnBeads were used for further QC analysis, including manual inspection of the diagnostic QC plots. The list of potentially polymorphic CpGs has been generated using the 2011051 release of the 1,000 Genomes project (30). Computational threshold for inclusion when analysing the mRRBS data were set to include CpGs with ≥ 10 reads to enable identification of minimum 10% difference in DNA methylation. Further, we set the minimum number of samples in each comparison to ≥ 5 samples in both baseline and follow-up samples. To minimize the number of false positives, the data was, in addition to the former filtering, we used false discovery rate (FDR) adjusted p-value ≤ 0.05 (Figure 1). Annotation was done using the R package AnnotatR (31) from Bioconductor. All genome positions are given according to Genome build 37/hg19. We investigated whether genes annotated to our DMPs were among genes carrying genetic variants involved in MTX treatment efficiency or toxicity (12–15).
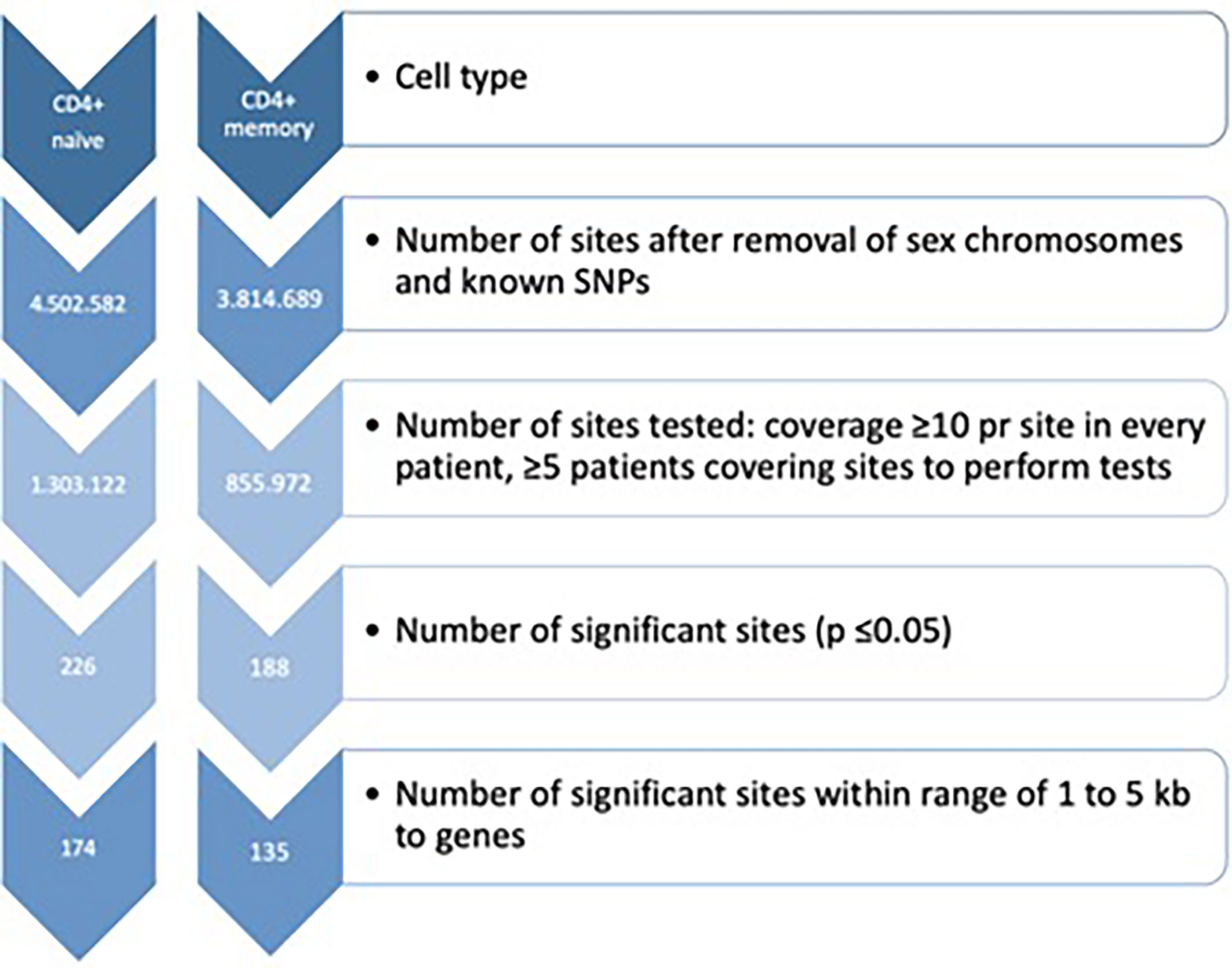
Figure 1 Filtration criteria for identification of differentially DNA methylated CpG sites in both CD4+ naïve and memory T cells. SNPs, Single nucleotide polymorphisms.
Differential DNA Methylation
To identify DMPs associated with disease activity and response to MTX, we performed a paired-samples analysis in RnBeads (32). DNA methylation was calculated individually within each patient. Then, baseline DNA methylation was tested against DNA methylation from the follow-up sample individually, and these results were then combined as a mean DNA methylation change across all patients. The regression model was adjusted for known covariates; gender, age, smoking status and ACPA. We performed analyses in naïve and memory CD4+ T cells separately. P-values for the DMP level were calculated using the limma method (33) and fitted using an empirical Bayes approach. FDR-adjusted P-values ≤0.05 were considered statistically significant. QQ (quantile-quantile) plots with lambda-values and standard errors were generated in the R packages ggplot2 and QQperm.
Overlap between reported RA risk GWAS loci and the significant DMPs observed in our study was investigated using a window size of maximum +/- 500 kb surrounding the SNPs reported by Yarwood et al. (34).
Results
Characterization of Patients
All nine patients had active joint inflammation when the baseline blood sample was drawn, and they were all treatment naïve for DMARDs and steroids (Table 1), however four patients were taking regular medications for other chronic illnesses (Supplementary Table S1). The majority of patients were female (N=6) and ACPA positive (N=8). At baseline, the patients had a mean DAS28 of 5.4, and five patients had high disease activity according to DAS28 (>5.1). Three patients had erosions on radiographs of the hands and/or feet at baseline.
Two patients started treatment with MTX monotherapy, and seven with MTX and prednisolone combined. The MTX start dose was 15 mg/week for all patients, except one who started with 20 mg/week. At follow-up, four patients were in clinical remission (DAS28 ≤2.6) and the mean DAS28 was 2.8. At the time of the second blood sample, the patients either received MTX monotherapy (N=5) or MTX with prednisolone (N=4). Dosage of prednisolone and MTX varied slightly between patients at follow-up (Table 1 and Supplementary Table S1). At follow-up, seven patients had a good response, one patient had a moderate response and one patient had no improvement to MTX according to the EULAR response criteria (23) (Table 1).
Data Generation and Quality Assessment
Flow cytometry showed that the mean purity was 94% (range 85 to 99%) for CD4+ memory T cells (CD4+CD45RO+) and 82% (range 68 to 95%) for CD4+ naïve T cells (CD4+CD45RA+) (Supplementary Figure S1). The bisulfite conversion rate was ≥97.2% for CD4+ naïve T cells, and ≥99.5% for CD4+ memory T cells (Supplementary Table S2). The number of aligned mRRBS reads was >13.1 million for each subset of samples (divided by cell type), and the mean CpG coverage was >9.5 per sample (Supplementary Table S2). The on target base count was >331 million across the samples, and the mean 10x coverage ranged from 15.9 – 24.3%. Altogether, 1,303,122 and 855,972 CpG sites were investigated in CD4+ naïve and CD4+ memory T cells, respectively (Figure 1). The tested CpGs were generally not covered by the CpGs tested using Infinium 450k or EPIC microarray technologies.
MTX Treatment Influenced DNA Methylation Levels at Multiple CpG Sites in Both CD4+ Naïve and Memory T Cells
The mean DNA methylation across all CpGs throughout the genome was higher in CD4+ naïve T cells (0.639) than in CD4+ memory T cells (0.557) (Supplementary Table S3). The distribution of the significant (PFDR adj <0.05) DMPs across chromosomes is shown in Figure 2, and information about the sites is found in Supplementary Table S4. Q-Q plots showed a deviation of the observed from the expected, providing evidence of DNA methylation differences before and after MTX treatment (Supplementary Figure S2), but with low inflation (lambda=1.06 for CD4+ naïve and lambda=0.84 for CD4+ memory T cells).
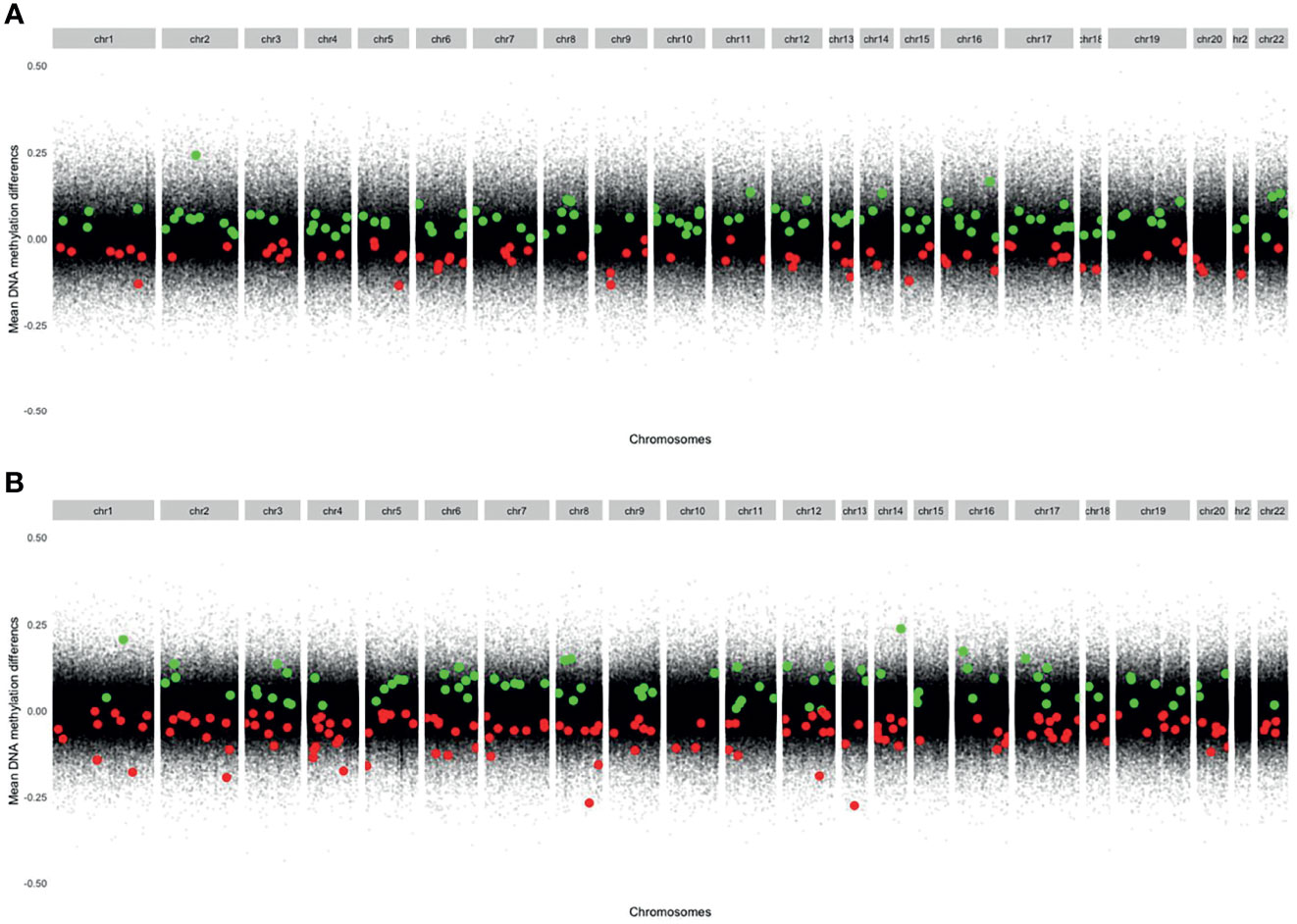
Figure 2 Modified Manhattan plots showing mean DNA methylation differences on the y-axis and genomic positions on the x-axis. Green dots represent significant DMPs with increased DNA methylation at follow-up. Red dots represent significant DMPs with decreased DNA methylation at follow-up. (A) CD4+ memory T cells (Increased DNA methylation: 111 DMPs. Decreased DNA methylation: 77 DMPs). (B) CD4+ naïve T cells (Increased DNA methylation: 84 DMPs. Decreased DNA methylation: 142 DMPs). DMPs, Differentially methylated positions.
In CD4+ naïve T cells, MTX treatment was associated with 226 significant DMPs, whereas 142 DMPs (62.8%) showed decreased DNA methylation. The 226 DMPs were annotated to 174 genes (Figure 1), and the genes for the most significant DMP (PFDR adj <5x10-5) were ZNF793, DNAAFS, TRIM15, TRIM10 and USP37 (Supplementary Table S4).
In CD4+ memory T cells, MTX treatment was significantly associated with DNA methylation differences at 188 DMPs with 111 DMPs (59.0%) displaying increased DNA methylation. The 188 DMPs represented 135 genes, with WDR81, NXNRD1, GRAMD2B, TRAF2, SPART, HDAC4 and NR2E1 annotated to the most significant DMPs (Supplementary Table S4).
Altogether, 593,509 sites were tested in both cell types, but none of the significant DMPs overlapped. However, one gene, PCDH17, was found to harbor significant DMPs in both CD4+ naïve and memory T cells after MTX treatment. In CD4+ naïve T cells, the mean DNA methylation value for the PCDH17 CpG site was decreased from 57.5% to 32.0% after treatment with MTX, while the mean DNA methylation increased from 4.3% to 5.5% in CD4+ memory T cells.
Next, we investigated the DNA methylation at genes annotated to the 21 DMPs identified by Glossop et al. to be differentially methylated in T cells collected before treatment from responders vs non-responders (19). Interestingly, two of the genes, SORCS2 and TRIM15, were found to harbor CpGs that significantly changed their methylation levels after MTX treatment. The intronic CpG in SORCS2 showed variable mean DNA methylation before treatment (96.5%), but was completely methylated in CD4+ memory T cells after MTX treatment (Figure 3A). All patients with successful measurements of DNA methylation for SORCS2 at both time points (N=5) had a good response to MTX and had low disease activity (DAS28 ≤ 3.2) at follow-up, but three of the five patients were not in clinical remission (DAS28 ≤ 2.6) (Table 1). The CpG located in the promoter of TRIM15 showed a mean decrease in DNA methylation in CD4+ naïve T cells from 98.9% before treatment to 95.0% after MTX. Seven of the nine patients had measures at the CpG in TRIM15 at both time points (Figure 3B). All the seven patients had a good response to MTX and low disease activity (DAS28 ≤ 3.2) after treatment (Table 1). Two patients did not show individual decrease in DNA methylation before and after MTX. These two patients were among the three patients who achieved remission (DAS28 ≤ 2.6) at the follow-up time point. The remaining four patients with decrease in DNA methylation did not achieve clinical remission (Table 1).
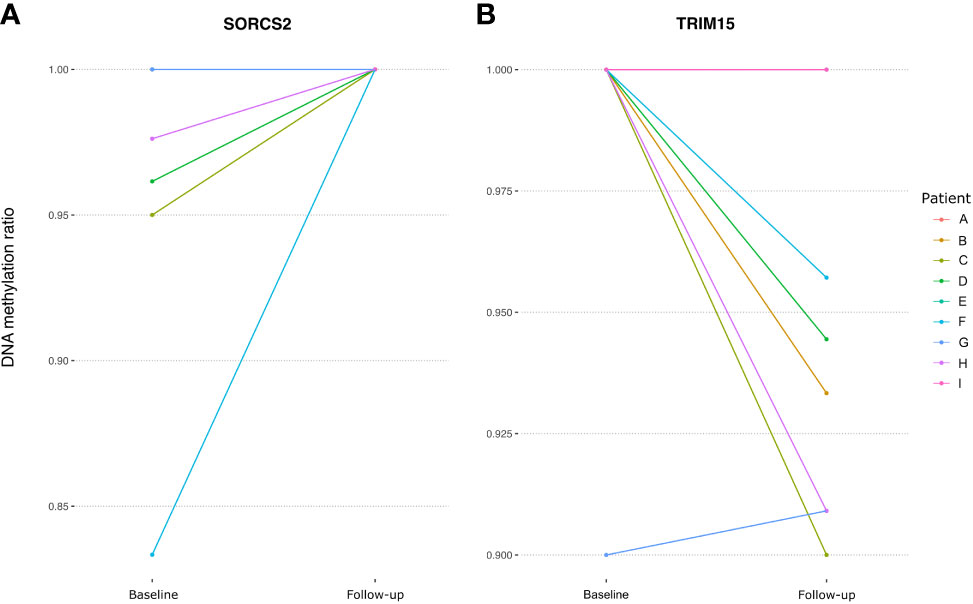
Figure 3 Spaghetti plots showing the change in DNA methylation in RA patients at a DMP located in (A) SORCS2 and (B) TRIM15 between baseline and follow-up. RA; Rheumatoid arthritis, DMP, Differentially methylated position; SORCS2, Sortilin Related VPS10 Domain Containing Receptor 2; TRIM15, Tripartite Motif Containing 15.
DMPs Associated With MTX Treatment That Overlap With Genetic RA Risk Loci
Next, we wanted to investigate if any of the DMPs associated with MTX treatment in RA patients were in regions overlapping genetic RA risk loci (34), since the genes annotated for SNP risk variants already have been implicated in RA susceptibility and pathogenesis. One could envisage that genes influencing disease development (either through methylation changes or as risk SNPs influencing gene expression levels) also could be involved in the MTX treatment process, especially when patients respond to the treatment. Given that few RA risk loci have been fine mapped, we searched for DMPs located +/- 500 kb from the lead RA risk SNP (Supplementary Table S5). In CD4+ naïve T cells, 11 DMPs were in the proximity of genetic RA risk loci, and for the SYNGR1 gene, two DMPs were detected. In CD4+ memory cells, 16 DMPs were found near RA risk SNPs, and CCR6 had two DMPs located within the gene. The only instance where the same gene was annotated for both the risk SNP and the DMP, was for the WDFY4 gene. WDFY4 has one significant DMP in CD4+ memory T cells before and after treatment, and a trend of increased overall mean methylation in the RA patients after treatment with MTX was seen across several sites (Figure 4).
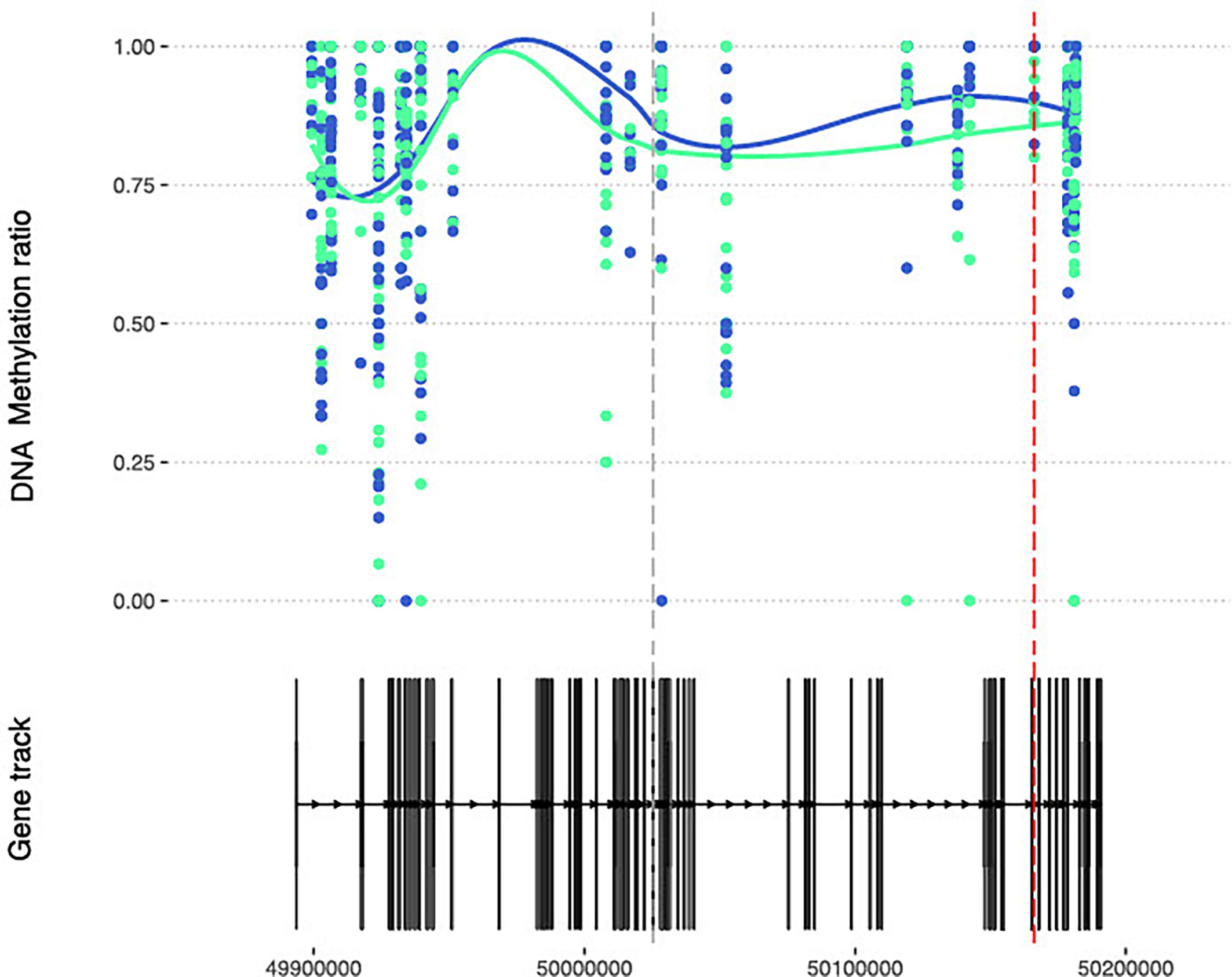
Figure 4 DNA methylation at CpGs overlapping the RA susceptible WDFY4 gene. DNA methylation at CpGs (N=41) overlapping the WDFY4 gene. Smoothed lines show mean DNA methylation values for the two patient sampling time points (baseline methylation values in green and follow-up values in blue). The red dotted vertical line marks the position of the DMP, and the grey dotted line marks the RA risk SNP. RA, Rheumatoid arthritis; WDFY4, WD Repeat and FYVE Domain-Containing Protein 4; SNP, Single nucleotide polymorphism.
DNA Methylation Changes in Genes Involved in MTX Response
Interestingly, among the genes annotated to significant DMPs were several solute carrier (SLC) transporters (Supplementary Table S4). These transporters play a role in transport of substrates across biological membranes, which is important in the absorption of drugs. Changes in DNA methylation after MTX treatment at CpGs annotated to SLC6A5, SLC9B1, SLC25A3, and SLC29A1 in CD4+ naïve T cells, and SLC30A5 and SLC38A1 in CD4+ memory T cells (Table 2). Mainly increased (SLC6A5, SLC25A3, SLC30A5 and SLC38A1), but also decreased DNA methylation (SLC9B1 and SLC29A1) was observed at these sites after MTX treatment.
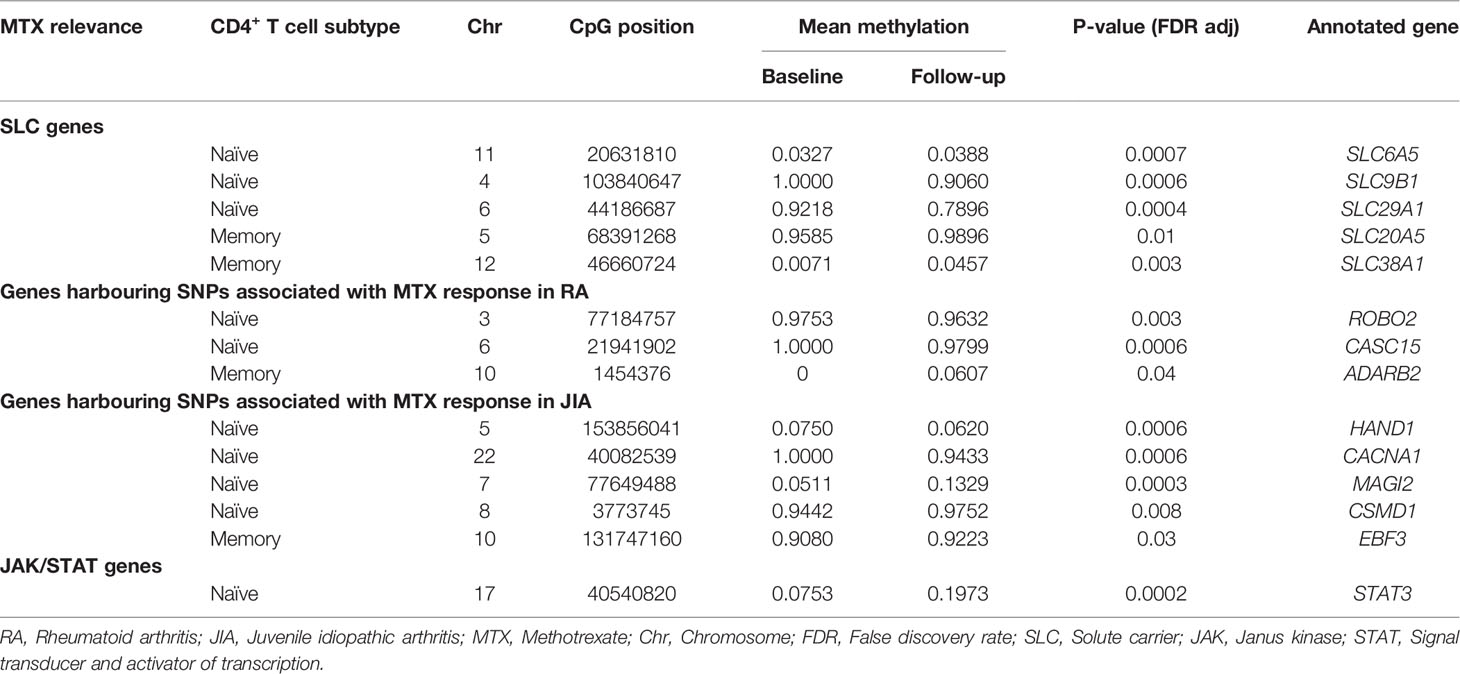
Table 2 DMPs annotated to genes previously reported to be relevant to MTX function or treatment response in RA or JIA.
We also investigated whether genes implicated through SNPs found to be associated with MTX response in either RA (13, 14) or JIA (15) were among the genes annotated to CpGs showing differential DNA methylation after MTX treatment in the RA patients (Table 2). Indeed, DMPs identified in naïve CD4+ T cells were annotated to ROBO2, CASC15, HAND1, CACNA1, MAGI2 and CSMD1 genes. The DMPs in the two latter genes showed increased DNA methylation, while the first four showed decreased DNA methylation between the two sample points. For the significant DMPs in CD4+ memory T cells, only ADARB2 (PFDR adj=0.04) and EBF3 (PFDR adj=0.03) harbored genetic MTX response variants, and they both showed increased DNA methylation.
Given the suggested role of JAK/STAT signaling pathway involved in MTX treatment, as well as a central role in RA (35), we checked whether genes encoding any of the four JAK proteins (JAK1, JAK2, JAK3 and TYK2) or the seven STAT proteins (STAT1, STAT2, STAT3, STAT4, STAT5A, STAT5B and STAT6) were among the genes annotated to the significant DMPs (Supplementary Table S4). One DMP was found in the promoter region of STAT3 (Table 2 and Figure 5A) and showed an overall mean increase in DNA methylation after treatment with MTX in CD4+ naïve T cells (Figure 5B). Interestingly, in contrast, the two patients with moderate or no improvement in DAS28 after treatment with MTX showed decrease in DNA methylation of STAT3 after treatment (Figure 5B).
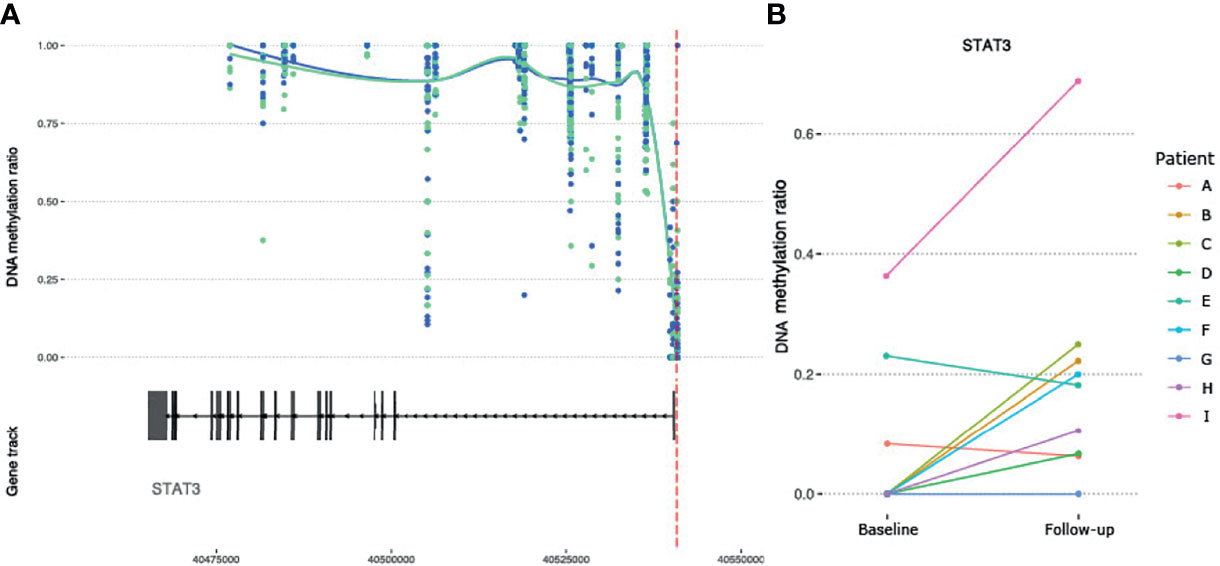
Figure 5 DNA methylation at CpGs overlapping the RA susceptible STAT3 gene. (A) DNA methylation at CpGs (N= 73) overlapping the STAT3 gene. Smoothed lines show mean DNA methylation values for the two patient sampling time points (baseline methylation values in green and follow-up values in blue). The red dotted vertical line marks the position of the significant DMP. (B) Intra-individual changes in DNA methylation between baseline and follow-up for the significant DMP in STAT3. RA, Rheumatoid arthritis; STAT3, Signal transducer and activator of transcription 3; DMP, Differentially methylated position.
Discussion
By using mRRBS, we identified significant DNA methylation changes associated with MTX treatment, and these changes were distinct in naïve and memory CD4+ T cells from RA patients. After MTX treatment, the majority of significant sites showed increased DNA methylation in CD4+ memory T cells and decreased DNA methylation in CD4+ naïve T cells, compared to the samples drawn from the same patients at the time of diagnosis before DMARD treatment. Some of the DMPs were located in genes earlier described to be differentially methylated in responders and non-responders to MTX in bulk T cells from RA patients before treatment (19), i.e. TRIM15 and SORCS2. In our study, we found that MTX treatment influenced the CpG for TRIM15 in CD4+ naïve T cells, while for SORCS2 in CD4+ memory T cells.
The DNA methylation levels varied largely at the same CpGs between CD4+ naïve and memory T cells. Globally, we observed higher methylation in CD4+ naïve T cells than in CD4+ memory T cells, which is in line with the previously described global loss of DNA methylation in heterochromatic parts of the genome during differentiation from CD4+ naïve to memory T cells (18). These findings highlight the importance of investigating DNA methylation profiles for CD4+ naïve and memory T cells, separately.
DNA methylation is known to be cell type specific and important for regulation of genes, but the exact mechanism is still unknown. In general, DNA methylation at gene promoter regions is negatively correlated with gene expression, but this is not always the case. Naïve and memory CD4+ T cells have different immune functions and disruption of cell type specific cellular processes as a result of altered DNA methylation may lead to phenotypic alterations. DNA methylation is, in addition and in tight interaction with histone modifications, believed to influence DNA accessibility and binding of e.g. the transcriptional complex including transcription factors and cofactors. Unfortunately, gene expression data is not available for these samples, which makes it difficult to draw any conclusions about the functional consequences of decreased DNA methylation on gene expression and thereby the biological implications for RA.
The design of our study aimed to reduce potential noise in the compared methylation profiles by 1) using a clinically well-described cohort of treatment naïve, newly diagnosed patients, 2) sampling the same patients before and after treatment with MTX, 3) methylation profiling of CD4+ naïve and memory T cells, separately, 4) using mRRBS to generate high resolution and genome-wide DNA methylation, and finally, 5) adjusting for gender, age, smoking status and ACPA in the statistical analyses to reduce influence of known covariates on DNA methylation.
This approach, however, reduced the number of patients included. Due to limited cohort size, we could not systematically assess CpGs that could be markers for response to MTX treatment. Future studies regarding treatment response are warranted, and such studies are further motivated by the observation that two of the genes (TRIM15 and SORCS2) with CpGs being influenced by MTX treatment were also reported by Glossop et al. to harbor epigenetic markers for treatment response to several DMARDs (19). Encouragingly in this respect, molecular signatures have been reported to accurately predict anti-TNF treatment response, but with distinct DNA methylation and transcriptomic profiles for RA patients treated with adalimumab and etanercept (36).
A recent study of DNA methylation and gene expression, associated with RA, in bulk CD4+ T cells found that 22% of the differentially methylated regions and the differentially expressed genes overlapped the same topologically associated domains (37). A number of these were also linked to genetic RA risk variants through expression or methylation quantitative traits.
The functional relevance of the genes annotated for our significant DMPs is supported by the notion that these genes have been reported to either influence RA risk (34) or in MTX treatment response or pathways (4, 13). Among the genes annotated for DMPs were several SLC transporters, which facilitate the transport of a wide array of substrates across biological membranes, including the absorption of drugs (38).
The most robust methylation differences, i.e. with more than one significant DMP detected, were for SYNGR1 in CD4+ naïve T cells and for CCR6 in CD4+ memory T cells. SYNGR1 and CCR6 have been identified as RA susceptibility loci in large GWAS studies (39, 40). Inhibition of ccr6, by monoclonal antibodies in mice, has been reported to supress arthritis (41). We also detected increased DNA methylation after MTX treatment in CD4+ memory T cells at a CpG located in the WDFY4 gene, which is a gene reported to harbour SNPs associated with several rheumatic diseases, i.e. systemic lupus erythematosus, JIA and RA (34, 42–44).
We found an overlap between our DMPs and eight regions harboring SNPs found to predict response to MTX treatment in RA (13, 14) and JIA (15). Our finding of MTX also influencing the DNA methylation at such loci suggest a role for genes in these regions, both genetically and epigenetically, during MTX treatment. Our DMPs draw attention to CD4+ naïve and memory T cells being important.
Furthermore, we detected a significant DMP in STAT3 in CD4+ naïve T cells showing increased DNA methylation in RA patients after treatment with MTX, which is of particular interest given the described role of the JAK/STAT pathway in MTX treatment (4). Recently, a study analyzing differential DNA methylation levels in naïve and memory CD4+ T cells in RA patients, also detected a central role for JAK1/STAT3/IL6 in these cell subtypes (45). This points to the importance of using anti-IL6 and JAK-inhibitors early in the disease development in RA. Suppression of the JAK/STAT pathway has been suggested to represent one of the principal anti-inflammatory and immunosuppressive mechanism of action of low-dose MTX (46). Expression of the STAT3 gene has been found to correlate with synovitis and modulation of Th17 differentiation in RA patients (47), while STAT3 inhibition has been reported to mediate chemokine expression in RA synoviocytes (48). A link between STAT3, HIF1α and Notch-1 signalling in regulation of pro-inflammatory mechanisms in RA has also been described (49). Interestingly, when we looked at individual DNA methylation values for STAT3 in our RA patients, we found that the two patients who obtained moderate or no clinical improvement according to their DAS28 score, showed decreased DNA methylation after treatment with MTX. Obviously, our study is too small to draw any conclusion, but given STAT3 role as a mediator of inflammatory mechanisms and that methylation changes can alter STAT3 expression, this is an interesting observation that should be explored further in larger patient cohorts.
Conclusion
We detected CpG sites with DNA methylation levels associated with MTX treatment in CD4+ naïve and memory T cells isolated from RA patients. Several of these CpGs were annotated to genes previously implicated in RA or MTX treatment, including TRIM15, SORCS2, CCR6, SYNGR1, WDFY4, and in particular STAT3 given its role of the JAK/STAT pathway in MTX treatment.
Data Availability Statement
The data presented in the study are deposited in the GEO repository, accession number GSE188509.
Ethics Statement
The studies involving human participants were reviewed and approved by The National Health Authorities and Ethics Committee (REK #2015/1546 and #2010/719), which was performed in accordance with the Declaration of Helsinki. The patients/participants provided their written informed consent to participate in this study.
Author Contributions
KGu has contributed with development and during laboratory procedures, developed bioinformatic pipeline, result interpretation and writing. LS has contributed during laboratory procedures, result interpretation and writing of the first draft. SFl has contributed to development and during laboratory procedures. MMæ, MMj, and EN have contributed in recruitment and clinical examination of the patients. IE, SFr, and AF have contributed to the library preparation. BA has contributed to the study design. SR and KGe have contributed to the bioinformatic pipeline and data analyses. BL has contributed to the study supervision, study design, results interpretation and writing. All authors have read, commented and approved the final manuscript.
Funding
This project was financed by the South-Eastern Norway Regional Health Authority (Helse Sør-Øst).
Conflict of Interest
The authors declare that the research was conducted in the absence of any commercial or financial relationships that could be construed as a potential conflict of interest.
Publisher’s Note
All claims expressed in this article are solely those of the authors and do not necessarily represent those of their affiliated organizations, or those of the publisher, the editors and the reviewers. Any product that may be evaluated in this article, or claim that may be made by its manufacturer, is not guaranteed or endorsed by the publisher.
Acknowledgments
We would like to thank all patients who participated in the study. We are grateful to Ellen Moholt and rheumatologists at Diakonhjemmet Hospital for patient recruitment, and to Kai Guttulsrød and personnel at the Department for Medical Biochemistry at Diakonhjemmet Hospital for helping us with the blood sampling. From the Department of Medical Genetics at Oslo University Hospital, we want to thank Helle Akselsen for assistance with the samples collection, and Fatima Heinicke and Ingvild Gabrielsen for assistance with cell separation and DNA extraction, and Hans Christian Dalsbotten Aass and the core facility for flow cytometry guidance.
Supplementary Material
The Supplementary Material for this article can be found online at: https://www.frontiersin.org/articles/10.3389/fimmu.2021.713611/full#supplementary-material
Abbreviations
ACPA, Anti-citrullinated protein antibody; ACR, American College of Rheumatology; CpGs, CpG sites; CRP, C-reactive protein; DAS28, Disease activity score 28; DMARD, Disease modifying antirheumatic drugs; DMPs, Differentially methylated positions; ESR, Erythrocyte sedimentation rate; EULAR, European League Against Rheumatism; FDR, False discovery rate; GWAS, Genome-wide association studies; JIA, Juvenile idiopathic arthritis; mRRBS, Multiplexed reduced representation bisulfite sequencing; MTX, Methotrexate; NOR-VEAC, The Norwegian Very Early Arthritis Clinic; PBMCs, Peripheral blood mononuclear cells; RA, Rheumatoid arthritis; RF, Rheumatoid factor; SNPs, Single nucleotide polymorphisms.
References
1. Padyukov L, Seielstad M, Ong RT, Ding B, Ronnelid J, Seddighzadeh M, et al. A Genome-Wide Association Study Suggests Contrasting Associations in ACPA-Positive Versus ACPA-Negative Rheumatoid Arthritis. Ann Rheum Dis (2011) 70(2):259–65. doi: 10.1136/ard.2009.126821
2. Kvien TK, Heiberg LE, Kaufmann C, Mikkelsen K, Nordvag BY, Rødevand E, et al. A Norwegian DMARD Register: Prescriptions of DMARDs and Biological Agents to Patients With Inflammatory Rheumatic Diseases. Clin Exp Rheumatol (2005) 23(5 Suppl 39):S188–94.
3. Wessels JA, Huizinga TW, Guchelaar HJ. Recent Insights in the Pharmacological Actions of Methotrexate in the Treatment of Rheumatoid Arthritis. Rheumatol (Oxford) (2008) 47(3):249–55. doi: 10.1093/rheumatology/kem279
4. Thomas S, Fisher KH, Snowden JA, Danson SJ, Brown S, Zeidler MP. Methotrexate Is a JAK/STAT Pathway Inhibitor. PloS One (2015) 10(7):e0130078. doi: 10.1371/journal.pone.0130078
5. Haavardsholm EA, Aga AB, Olsen IC, Lillegraven S, Hammer HB, Uhlig T, et al. Ultrasound in Management of Rheumatoid Arthritis: ARCTIC Randomised Controlled Strategy Trial. BMJ (2016) 354:i4205. doi: 10.1136/bmj.i4205
6. Smolen JS, Aletaha D, Bijlsma JW, Breedveld FC, Boumpas D, Burmester G, et al. Treating Rheumatoid Arthritis to Target: Recommendations of an International Task Force. Ann Rheum Dis (2010) 69(4):631–7. doi: 10.1136/ard.2009.123919
7. Stoffer MA, Schoels MM, Smolen JS, Aletaha D, Breedveld FC, Burmester G, et al. Evidence for Treating Rheumatoid Arthritis to Target: Results of a Systematic Literature Search Update. Ann Rheum Dis (2016) 75(1):16–22. doi: 10.1136/annrheumdis-2015-207526
8. Farragher TM, Lunt M, Fu B, Bunn D, Symmons DP. Early Treatment With, and Time Receiving, First Disease-Modifying Antirheumatic Drug Predicts Long-Term Function in Patients With Inflammatory Polyarthritis. Ann Rheum Dis (2010) 69(4):689–95. doi: 10.1136/ard.2009.108639
9. Kallberg H, Ding B, Padyukov L, Bengtsson C, Ronnelid J, Klareskog L, et al. Smoking is a Major Preventable Risk Factor for Rheumatoid Arthritis: Estimations of Risks After Various Exposures to Cigarette Smoke. Ann Rheum Dis (2011) 70(3):508–11. doi: 10.1136/ard.2009.120899
10. Klareskog L, Malmstrom V, Lundberg K, Padyukov L, Alfredsson L. Smoking, Citrullination and Genetic Variability in the Immunopathogenesis of Rheumatoid Arthritis. Semin Immunol (2011) 23(2):92–8. doi: 10.1016/j.smim.2011.01.014
11. Okada Y, Wu D, Trynka G, Raj T, Terao C, Ikari K, et al. Genetics of Rheumatoid Arthritis Contributes to Biology and Drug Discovery. Nature (2014) 506(7488):376–81. doi: 10.1038/nature12873
12. Giletti A, Esperon P. Genetic Markers in Methotrexate Treatments. Pharmacogenom J (2018) 18(6):689–703. doi: 10.1038/s41397-018-0047-z
13. Taylor JC, Bongartz T, Massey J, Mifsud B, Spiliopoulou A, Scott IC, et al. Genome-Wide Association Study of Response to Methotrexate in Early Rheumatoid Arthritis Patients. Pharmacogenom J (2018) 18(4):528–38. doi: 10.1038/s41397-018-0025-5
14. Senapati S, Singh S, Das M, Kumar A, Gupta R, Kumar U, et al. Genome-Wide Analysis of Methotrexate Pharmacogenomics in Rheumatoid Arthritis Shows Multiple Novel Risk Variants and Leads for TYMS Regulation. Pharmacogenet Genomics (2014) 24(4):211–9. doi: 10.1097/FPC.0000000000000036
15. Cobb J, Cule E, Moncrieffe H, Hinks A, Ursu S, Patrick F, et al. Genome-Wide Data Reveal Novel Genes for Methotrexate Response in a Large Cohort of Juvenile Idiopathic Arthritis Cases. Pharmacogenom J (2014) 14(4):356–64. doi: 10.1038/tpj.2014.3
16. Kim YI, Logan JW, Mason JB, Roubenoff R. DNA Hypomethylation in Inflammatory Arthritis: Reversal With Methotrexate. J Lab Clin Med (1996) 128(2):165–72. doi: 10.1016/S0022-2143(96)90008-6
17. de Andres MC, Perez-Pampin E, Calaza M, Santaclara FJ, Ortea I, Gomez-Reino JJ, et al. Assessment of Global DNA Methylation in Peripheral Blood Cell Subpopulations of Early Rheumatoid Arthritis Before and After Methotrexate. Arthritis Res Ther (2015) 17:233. doi: 10.1186/s13075-015-0748-5
18. Durek P, Nordstrom K, Gasparoni G, Salhab A, Kressler C, de Almeida M, et al. Epigenomic Profiling of Human CD4(+) T Cells Supports a Linear Differentiation Model and Highlights Molecular Regulators of Memory Development. Immunity (2016) 45(5):1148–61. doi: 10.1016/j.immuni.2016.10.022
19. Glossop JR, Nixon NB, Emes RD, Sim J, Packham JC, Mattey DL, et al. DNA Methylation at Diagnosis is Associated With Response to Disease-Modifying Drugs in Early Rheumatoid Arthritis. Epigenomics (2017) 9(4):419–28. doi: 10.2217/epi-2016-0042
20. Aletaha D, Neogi T, Silman AJ, Funovits J, Felson DT, Bingham CO 3rd, et al. 2010 Rheumatoid Arthritis Classification Criteria: An American College of Rheumatology/European League Against Rheumatism Collaborative Initiative. Arthritis Rheum (2010) 62(9):2569–81. doi: 10.1002/art.27584
21. Brinkmann GH, Norvang V, Norli ES, Grovle L, Haugen AJ, Lexberg AS, et al. Treat to Target Strategy in Early Rheumatoid Arthritis Versus Routine Care - A Comparative Clinical Practice Study. Semin Arthritis Rheum (2019) 48(5):808–14. doi: 10.1016/j.semarthrit.2018.07.004
22. Prevoo ML, van ’t Hof MA, Kuper HH, van Leeuwen MA, van de Putte LB, van Riel PL. Modified Disease Activity Scores That Include Twenty-Eight-Joint Counts. Development and Validation in a Prospective Longitudinal Study of Patients With Rheumatoid Arthritis. Arthritis Rheum (1995) 38(1):44–8. doi: 10.1002/art.1780380107
23. van Riel PL, Renskers L. The Disease Activity Score (DAS) and the Disease Activity Score Using 28 Joint Counts (DAS28) in the Management of Rheumatoid Arthritis. Clin Exp Rheumatol (2016) 34(5 Suppl 101):S40–4.
24. Boyle P, Clement K, Gu H, Smith ZD, Ziller M, Fostel JL, et al. Gel-Free Multiplexed Reduced Representation Bisulfite Sequencing for Large-Scale DNA Methylation Profiling. Genome Biol (2012) 13(10):R92. doi: 10.1186/gb-2012-13-10-r92
25. Veillard A-C, Datlinger P, Laczik M, Squazzo S, Bock C. Diagenode[reg] Premium RRBS Technology: Cost-Effective DNA Methylation Mapping With Superior Coverage. Nat Meth (2016) 13(2):i-ii. doi: 10.1038/nmeth.f.391
26. Gu H, Smith ZD, Bock C, Boyle P, Gnirke A, Meissner A. Preparation of Reduced Representation Bisulfite Sequencing Libraries for Genome-Scale DNA Methylation Profiling. Nat Protoc (2011) 6(4):468–81. doi: 10.1038/nprot.2010.190
27. R Core Team. R: A Language and Environment for Statistical Computing. Vienna, Austria: R Foundation for Statistical Computing (2016).
28. Gentleman RC, Carey VJ, Bates DM, Bolstad B, Dettling M, Dudoit S, et al. Bioconductor: Open Software Development for Computational Biology and Bioinformatics. Genome Biol (2004) 5(10):R80. doi: 10.1186/gb-2004-5-10-r80
29. Xi Y, Li W. BSMAP: Whole Genome Bisulfite Sequence MAPping Program. BMC Bioinf (2009) 10:232. doi: 10.1186/1471-2105-10-232
30. Chen YA, Lemire M, Choufani S, Butcher DT, Grafodatskaya D, Zanke BW, et al. Discovery of Cross-Reactive Probes and Polymorphic CpGs in the Illumina Infinium HumanMethylation450 Microarray. Epigenetics (2013) 8(2):203–9. doi: 10.4161/epi.23470
31. Cavalcante RG, Sartor MA. Annotatr: Genomic Regions in Context. Bioinformatics (2017) 33(15):2381–3. doi: 10.1093/bioinformatics/btx183
32. Assenov Y, Muller F, Lutsik P, Walter J, Lengauer T, Bock C. Comprehensive Analysis of DNA Methylation Data With RnBeads. Nat Methods (2014) 11(11):1138–40. doi: 10.1038/nmeth.3115
33. Ritchie ME, Phipson B, Wu D, Hu Y, Law CW, Shi W, et al. Limma Powers Differential Expression Analyses for RNA-Sequencing and Microarray Studies. Nucleic Acids Res (2015) 43(7):e47. doi: 10.1093/nar/gkv007
34. Yarwood A, Huizinga TW, Worthington J. The Genetics of Rheumatoid Arthritis: Risk and Protection in Different Stages of the Evolution of RA. Rheumatol (Oxford) (2016) 55(2):199–209. doi: 10.1093/rheumatology/keu323
35. Malemud CJ. The Role of the JAK/STAT Signal Pathway in Rheumatoid Arthritis. Ther Adv Musculoskelet Dis (2018) 10(5-6):117–27. doi: 10.1177/1759720X18776224
36. Tao W, Concepcion AN, Vianen M, Marijnissen ACA, Lafeber F, Radstake T, et al. Multiomics and Machine Learning Accurately Predict Clinical Response to Adalimumab and Etanercept Therapy in Patients With Rheumatoid Arthritis. Arthritis Rheumatol (2021) 73(2):212–22. doi: 10.1002/art.41516
37. Ha E, Bang SY, Lim J, Yun JH, Kim JM, Bae JB, et al. Genetic Variants Shape Rheumatoid Arthritis-Specific Transcriptomic Features in CD4(+) T Cells Through Differential DNA Methylation, Explaining a Substantial Proportion of Heritability. Ann Rheum Dis (2021) 80(7):876–83. doi: 10.1136/annrheumdis-2020-219152
38. Lin L, Yee SW, Kim RB, Giacomini KM. SLC Transporters as Therapeutic Targets: Emerging Opportunities. Nat Rev Drug Discov (2015) 14(8):543–60. doi: 10.1038/nrd4626
39. Kim K, Bang SY, Lee HS, Cho SK, Choi CB, Sung YK, et al. High-Density Genotyping of Immune Loci in Koreans and Europeans Identifies Eight New Rheumatoid Arthritis Risk Loci. Ann Rheum Dis (2015) 74(3):e13. doi: 10.1136/annrheumdis-2013-204749
40. Cheng P, Zhang Y, Huang H, Zhang W, Yang Q, Guo F, et al. Association Between CCR6 and Rheumatoid Arthritis: A Meta-Analysis. Int J Clin Exp Med (2015) 8(4):5388–96.
41. Haas JD, Gonzalez FH, Schmitz S, Chennupati V, Fohse L, Kremmer E, et al. CCR6 and NK1.1 Distinguish Between IL-17A and IFN-Gamma-Producing Gammadelta Effector T Cells. Eur J Immunol (2009) 39(12):3488–97. doi: 10.1002/eji.200939922
42. Yang W, Shen N, Ye DQ, Liu Q, Zhang Y, Qian XX, et al. Genome-Wide Association Study in Asian Populations Identifies Variants in ETS1 and WDFY4 Associated With Systemic Lupus Erythematosus. PloS Genet (2010) 6(2):e1000841. doi: 10.1371/journal.pgen.1000841
43. McIntosh LA, Marion MC, Sudman M, Comeau ME, Becker ML, Bohnsack JF, et al. Genome-Wide Association Meta-Analysis Reveals Novel Juvenile Idiopathic Arthritis Susceptibility Loci. Arthritis Rheumatol (2017) 69(11):2222–32. doi: 10.1002/art.40216
44. Zhang Y, Bo L, Zhang H, Zhuang C, Liu R. E26 Transformation-Specific-1 (ETS1) and WDFY Family Member 4 (WDFY4) Polymorphisms in Chinese Patients With Rheumatoid Arthritis. Int J Mol Sci (2014) 15(2):2712–21. doi: 10.3390/ijms15022712
45. Pitaksalee R, Burska AN, Ajaib S, Rogers J, Parmar R, Mydlova K, et al. Differential CpG DNA Methylation in Peripheral Naive CD4(+) T-Cells in Early Rheumatoid Arthritis Patients. Clin Epigenet (2020) 12(1):54. doi: 10.1186/s13148-020-00837-1
46. Cronstein BN, Aune TM. Methotrexate and its Mechanisms of Action in Inflammatory Arthritis. Nat Rev Rheumatol (2020) 16(3):145–54. doi: 10.1038/s41584-020-0373-9
47. Krause A, Scaletta N, Ji JD, Ivashkiv LB. Rheumatoid Arthritis Synoviocyte Survival is Dependent on Stat3. J Immunol (2002) 169(11):6610–6. doi: 10.4049/jimmunol.169.11.6610
48. Rosengren S, Corr M, Firestein GS, Boyle DL. The JAK Inhibitor CP-690,550 (Tofacitinib) Inhibits TNF-Induced Chemokine Expression in Fibroblast-Like Synoviocytes: Autocrine Role of Type I Interferon. Ann Rheum Dis (2012) 71(3):440–7. doi: 10.1136/ard.2011.150284
Keywords: Rheumatoid arthritis, T cells, CD4 memory, CD4 naïve, DNA methylation, RRBS, epigenetics, methotrexate
Citation: Guderud K, Sunde LH, Flåm ST, Mæhlen MT, Mjaavatten MD, Norli ES, Evenrød IM, Andreassen BK, Franzenburg S, Franke A, Rayner S, Gervin K and Lie BA (2021) Methotrexate Treatment of Newly Diagnosed RA Patients Is Associated With DNA Methylation Differences at Genes Relevant for Disease Pathogenesis and Pharmacological Action. Front. Immunol. 12:713611. doi: 10.3389/fimmu.2021.713611
Received: 23 May 2021; Accepted: 27 October 2021;
Published: 18 November 2021.
Edited by:
Trine N. Jorgensen, Case Western Reserve University, United StatesReviewed by:
Åsa Andersson, Halmstad University, SwedenNisha Nair, The University of Manchester, United Kingdom
Copyright © 2021 Guderud, Sunde, Flåm, Mæhlen, Mjaavatten, Norli, Evenrød, Andreassen, Franzenburg, Franke, Rayner, Gervin and Lie. This is an open-access article distributed under the terms of the Creative Commons Attribution License (CC BY). The use, distribution or reproduction in other forums is permitted, provided the original author(s) and the copyright owner(s) are credited and that the original publication in this journal is cited, in accordance with accepted academic practice. No use, distribution or reproduction is permitted which does not comply with these terms.
*Correspondence: Line H. Sunde, bGluZWpvQG91cy1oZi5ubw==
†These authors have contributed equally to this work and share first authorship