- 1State Key Laboratory of Biocontrol and School of Life Sciences, Southern Marine Science and Engineering Guangdong Laboratory (Zhuhai), Guangdong Provincial Key Laboratory for Aquatic Economic Animals and Guangdong Provincial Engineering Technology Research Center for Healthy Breeding of Important Economic Fish, Sun Yat-Sen University, Guangzhou, China
- 2Laboratory for Marine Fisheries Science and Food Production Processes, Qingdao National Laboratory for Marine Science and Technology, Qingdao, China
- 3College of Ocean, Hainan University, Haikou, China
Coronavirus disease 2019 (COVID-19) raises the issue of how hypoxia destroys normal physiological function and host immunity against pathogens. However, there are few or no comprehensive omics studies on this effect. From an evolutionary perspective, animals living in complex and changeable marine environments might develop signaling pathways to address bacterial threats under hypoxia. In this study, the ancient genomic model animal Takifugu obscurus and widespread Vibrio parahaemolyticus were utilized to study the effect. T. obscurus was challenged by V. parahaemolyticus or (and) exposed to hypoxia. The effects of hypoxia and infection were identified, and a theoretical model of the host critical signaling pathway in response to hypoxia and infection was defined by methods of comparative metabolomics and proteomics on the entire liver. The changing trends of some differential metabolites and proteins under hypoxia, infection or double stressors were consistent. The model includes transforming growth factor-β1 (TGF-β1), hypoxia-inducible factor-1α (HIF-1α), and epidermal growth factor (EGF) signaling pathways, and the consistent changing trends indicated that the host liver tended toward cell proliferation. Hypoxia and infection caused tissue damage and fibrosis in the portal area of the liver, which may be related to TGF-β1 signal transduction. We propose that LRG (leucine-rich alpha-2-glycoprotein) is widely involved in the transition of the TGF-β1/Smad signaling pathway in response to hypoxia and pathogenic infection in vertebrates as a conserved molecule.
Introduction
Severe acute respiratory syndrome coronavirus 2 (SARS-CoV-2) causes coronavirus disease 2019 (COVID-19). As of October 22, 2021, more than 242.3 million SARS-CoV-2 infections and 4.9 million COVID-19-related deaths have been documented (1, 2). A previous proteomic study of COVID-19 autopsies indicated dysregulation of key factors involved in hypoxia, angiogenesis, blood coagulation, and fibrosis in multiple organs from COVID-19 patients, and these factors contributed to typical histopathological features of COVID-19, such as microthrombi, proliferation of fibroblasts/myofibroblasts and fibrosis in alveolar septa, and intussusceptive angiogenesis (3, 4). COVID-19 raises the issue of how hypoxia destroys the normal physiological function and immunity of the human body and therefore promotes the invasion of SARS-CoV-2 (5, 6).
Physiological hypoxia plays a role in shaping innate and adaptive immunity and maintaining physiological homeostasis, while pathological hypoxia drives tissue dysfunction and disease development through immune cell dysregulation (7). A prominent instance of pathological hypoxia is inflammation. Usually, inflammation demands the recruitment of a large number of myeloid cells, and the severe metabolic burden and huge demand for energy for the transport of cells contribute to an increase in oxygen consumption (8–10). Therefore, host cells have developed strategies to adapt to the antibacterial response under hypoxia. For instance, neutrophils, which play a major role in countering infection, principally utilize glycolysis as a way to obtain energy (11), and an oxygen concentration as low as 4.5% does not significantly affect their respiratory burst activity (12); therefore, neutrophils recognize and phagocytize pathogens under hypoxia (13).
From an evolutionary perspective, animals living in complex and changeable marine environments might develop signaling pathways to address bacterial threats under hypoxia (14). In this study, Takifugu obscurus (an ideal model to study the vertebrate genome) and Vibrio parahaemolyticus (a common opportunistic pathogen in the temperate zone and the tropics) were utilized to study the effects of hypoxia on immunity against pathogens (15–17). The metabolomic and proteomic characteristics of the entire liver of T. obscurus exposed to hypoxia and/or infection with V. parahaemolyticus were comparatively analyzed, and a theoretical model of the critical signaling pathway in response to hypoxia and infection was defined. We propose that LRG (leucine-rich alpha-2-glycoprotein) is widely involved in the transforming growth factor-β1 (TGF-β1) signaling pathway in response to hypoxia and pathogenic infection in vertebrates as a conserved molecule.
Results
Hypoxia-Accelerated Deaths Caused by Infection of V. parahaemolyticus and Fibrosis Caused by the Two Stressors
We established and observed a model of T. obscurus under hypoxia and infection with V. parahaemolyticus for 7 days, and then the mortality rate was counted (Figure 1A). Deaths first occurred on Day 4 in the normoxic infection (NI) (5 * 107 colony-forming unit, cfu) group, and the final survival rate after 7 days was 60%. Deaths occurred on Day 6 in the hypoxic control (HC) group, and the final survival rate was 90%. The number of deaths in the hypoxic infection (HI) (5 * 107 cfu) group increased from the first day, and the final survival rate was 50%. The final survival rate in the HI (5 * 109 cfu) group was 20%, which meant that the bacterial load affected the final mortality. Therefore, although hypoxia had no significant effect on the number of deaths caused by V. parahaemolyticus infection, it advanced the occurrence of deaths (Figure 1A). Pathological observation of the liver indicated that tissue damage and fibrosis occurred in the walls of interlobular veins, arteries or bile ducts in the portal area (Figures 1B, C). Deaths principally occurred on the first day (from 12 to 24 h); therefore, we anticipated that the host response in the early stage of infection would largely determine the final survival. Accordingly, metabolomic and proteomic analyses of the entire liver were performed at 12 h.
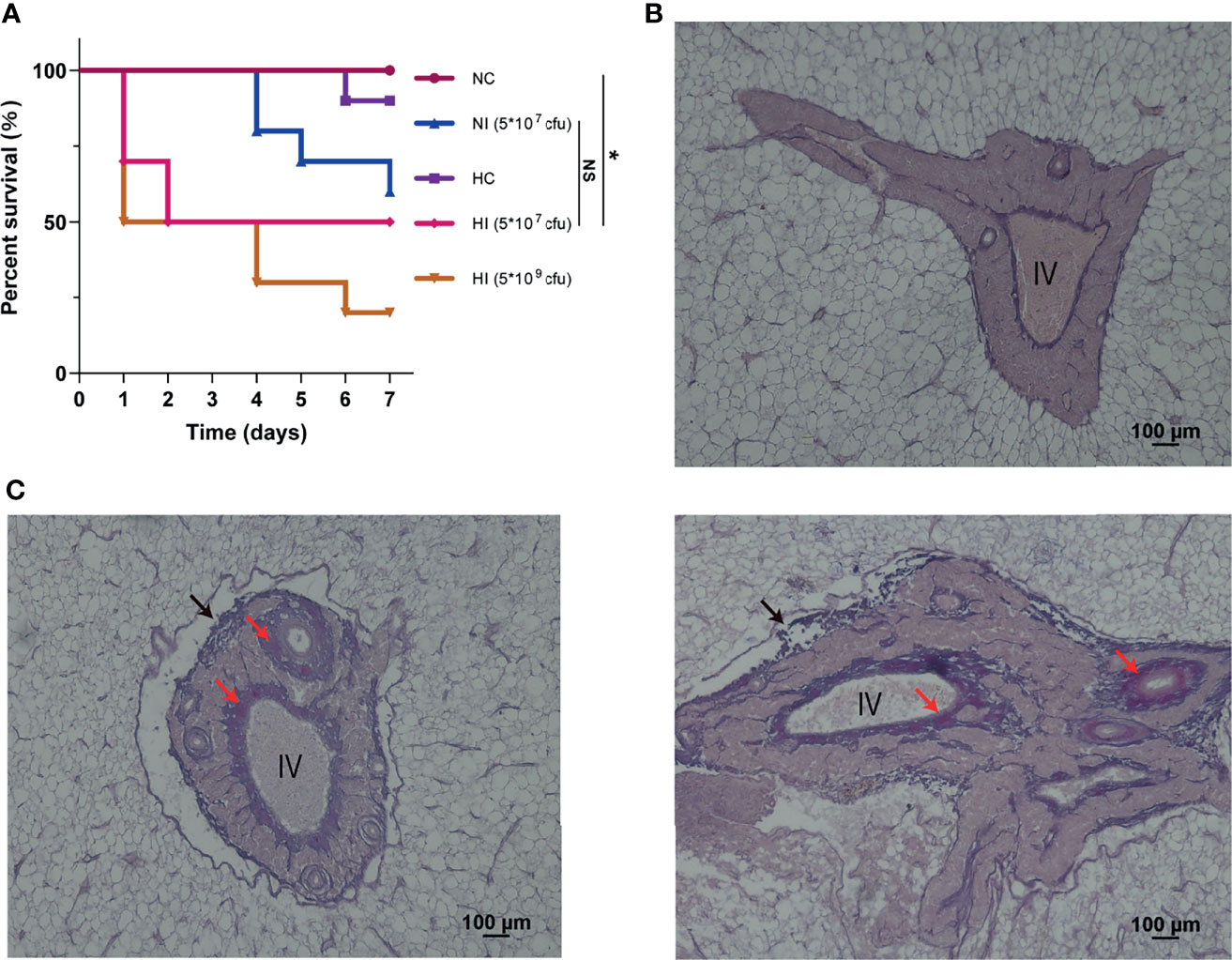
Figure 1 Survival curve and pathological observation of portal area. (A) The survival curve was measured in the independent preliminary experiment. For treatments of hypoxia and infection, T. obscurus were exposed to hypoxia for 12 h and then infected by V. parahaemolyticus. Finally, the survival rate of T. obscurus was monitored for 7 d. n = 10 biologically independent animals per group. P values were determined using two-sided log-rank (Mantel-Cox) tests. NS, not significant. *P value < 0.05. (B) Representative image from at least three biological replicates of the NC group. IV, interlobular vein. (C) Representative images of different stages of fibrosis from at least three biological replicates of the HI group. Black arrows, damaged walls; Red arrows, fibers.
Screening and Characterization of Differential Metabolites
Principal component analysis (PCA) compared the differences in metabolic characteristics between the normoxic control (NC) and HI groups and the degree of variation between parallel samples in the group. Partial least squares discriminant analysis (PLS-DA) established a model of the relationship between metabolite production and sample categories, and variable importance in the projection (VIP) was obtained to assist in the screening of differential metabolites (Figure 2). The model demonstrated good explanatory and predictive ability (Neg: R2Y = 0.99, Q2Y = 0.77. Pos: R2Y = 0.99, Q2Y = 0.63). The method described before was used to indicate that overfitting had not occurred (R2 > Q2, y-intercept of Q2 < 0) (18). As expected, minor differences in metabolic characteristics of hypoxia or infection were obtained (Figure S1).
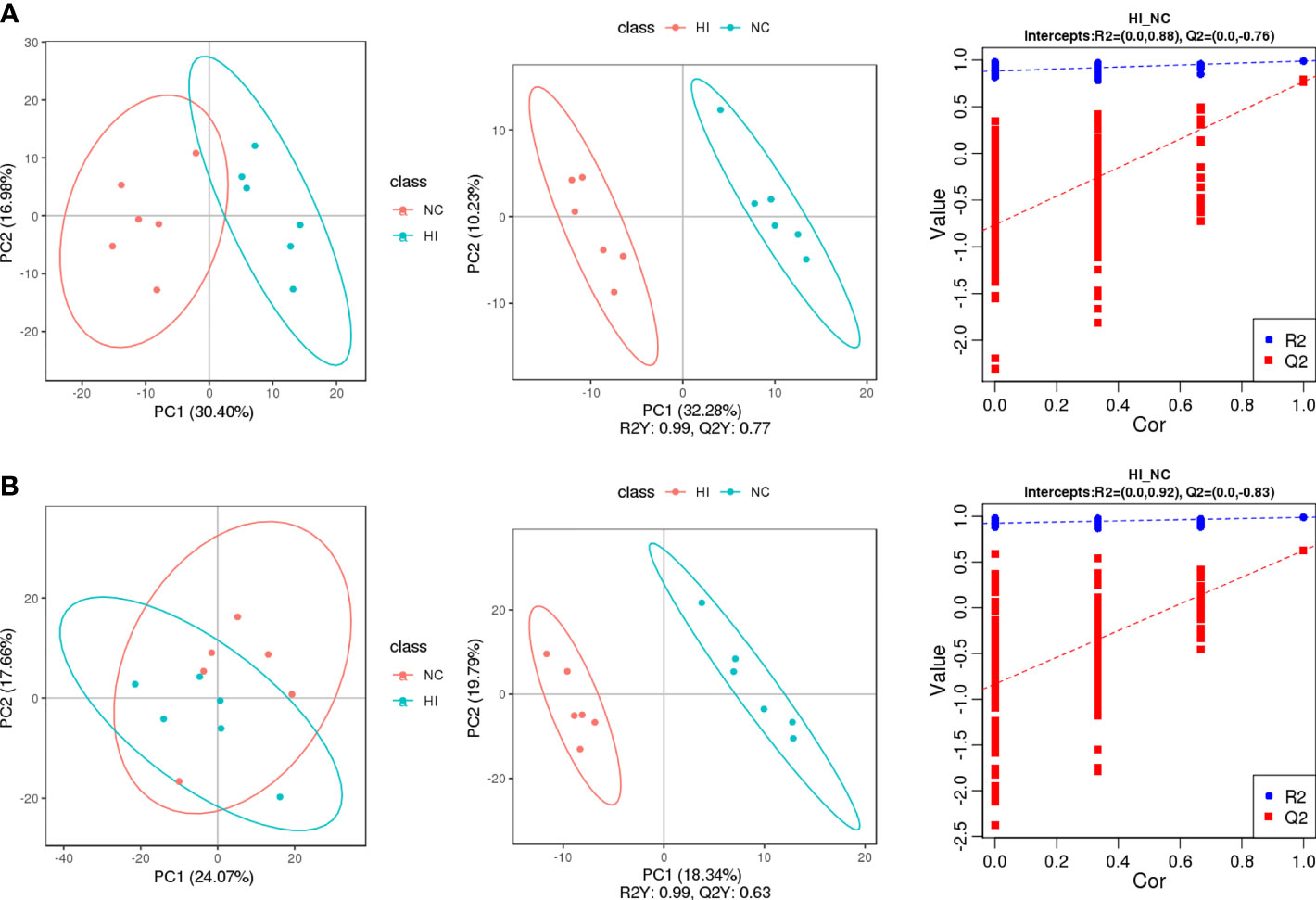
Figure 2 The screening of differential metabolites of the NC and HI groups was carried out for quality control and comparisons of differential metabolic characteristics. PC1 and PC2 represent the scores of the first and second principal components, respectively. (A) PCA, PLS-DA and function based on the sevenfold cross-validation method of the NC and HI groups in negative ion scanning mode. (B) PCA, PLS-DA and function based on the sevenfold cross-validation method of the NC and HI groups in positive ion scanning mode.
The differential metabolites of the 3 comparison groups were enriched in Kyoto Encyclopedia of Genes and Genomes (KEGG) pathways (Figure 3). Hypoxia affected the metabolisms of vitamins (nicotinamide/VB3, biotin/VB7, thiamine/VB1, folate/VB9 and VB6) and amino acids (histidine, lysine, arginine, phenylalanine, tyrosine, tryptophan, proline, valine, leucine, isoleucine and β-alanine) (Figure 3A). Under infection, the metabolism of serotonergic synapse was influenced. Moreover, taurine metabolism involved in bile acid synthesis was also modulated (Figure 3B). In addition to some of the above metabolites, sphingolipid was found to be regulated under hypoxia and infection (Figure 3C). These results indicate that the metabolisms of vitamins and amino acids are important for the host to resist hypoxia and infection.
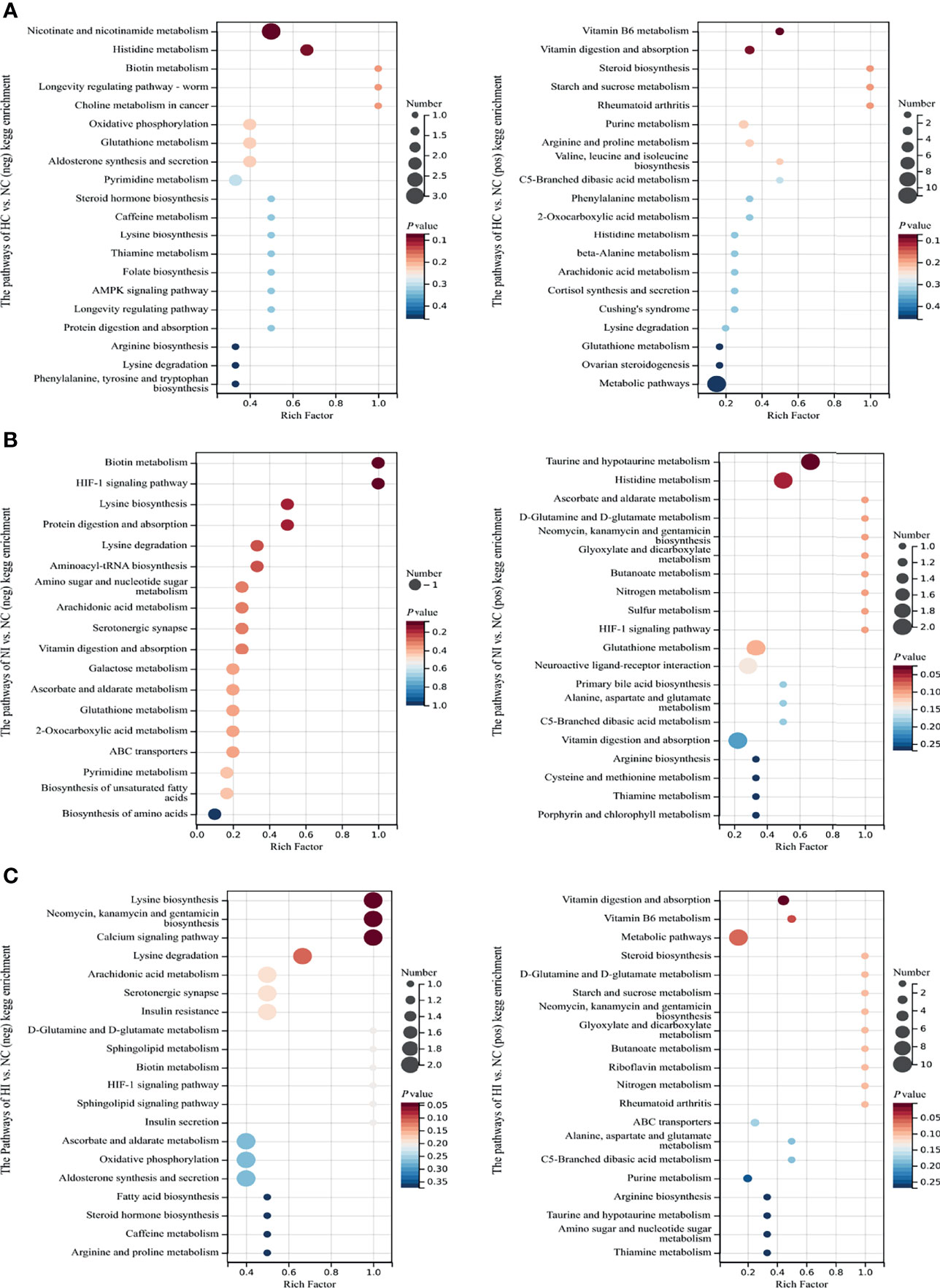
Figure 3 The enriched top 18 or 20 KEGG pathways sorted by P value based on the differential metabolites of the three compared groups. The size of the point indicates the number of enriched metabolites. Rich Factor, the ratio of x to M. (A) The enriched KEGG pathways of NC versus HC. (B) The enriched KEGG pathways of NC versus NI. (C) The enriched KEGG pathways of NC versus HI.
Characterization of Differential Proteins
The enriched Gene Ontology (GO) terms and KEGG pathways of differential proteins indicated cellular processes under hypoxia or (and) infection of V. parahaemolyticus (Figure 4). Under hypoxia, the main processes focused on RNA processing, lyase activity and ATPase activity, and the activities of many other enzymes were influenced. The enriched pathways focused on mitophagy, purine metabolism, galactose metabolism, drug metabolism-other enzymes and selenocompound metabolism. Interestingly, nucleotide-binding oligomerization domain (NOD)-like receptors (NLRs) signaling pathway was regulated (Figure 4A). Under infection, the main processes focused on the modification (deubiquitination, ADP-ribosylation and neddylation) and transport of proteins. The enriched KEGG pathways focused on sphingolipid metabolism, cell adhesion molecules, lysosomes, cytokine-cytokine receptor interactions and glycan degradation. Consistent with the metabolites, thiamine metabolism was influenced. Other vitamins like riboflavin/VB2 and pantothenic acid/VB5 were also modulated. In addition, fatty acids and galactose were also regulated (Figure 4B). Under hypoxia and infection, the main processes of transmembrane transport of substances and the regulation and activity of enzymes were seriously influenced. The enriched KEGG pathways focused on sphingolipid metabolism, glycosphingolipid biosynthesis, linoleic acid metabolism, ABC (ATP-binding cassette) transporters and mucin type O-glycan biosynthesis (Figure 4C), partially overlapped with the reactions under infection. Apparently, more biotic activities are influenced, which emphasizes the complexity of the effects of double stressors. According to association analysis between metabolome and proteome, five metabolites and 6 proteins were involved in purine metabolism under hypoxia, while 4 metabolites and 2 proteins were involved under hypoxia and infection (Tables S1–3). This indicates that purine metabolism is important for resisting infection under hypoxia.
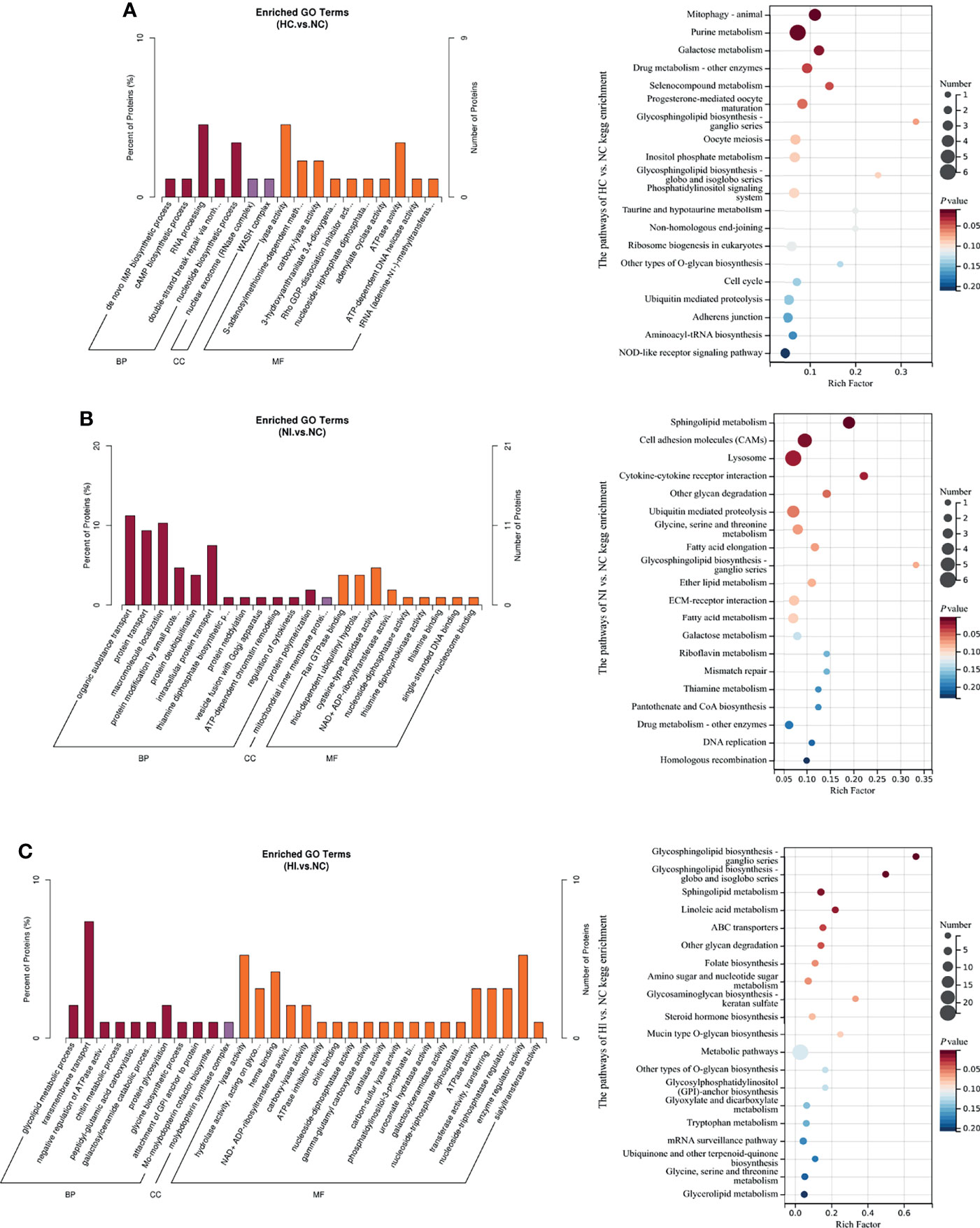
Figure 4 The enriched GO terms (P value < 0.05) and top 20 KEGG pathways sorted by P value based on the differentially expressed proteins of the three compared groups. Percent of proteins: the ratio of x to n. The size of the point indicates the number of enriched metabolites. Rich Factor, the ratio of x to M; BP, biological process; CC, cellular component; MF, molecular function. (A) The enriched GO terms and KEGG pathways of NC versus HC. (B) The enriched GO terms and KEGG pathways of NC versus NI. (C) The enriched GO terms and KEGG pathways of NC versus HI.
Theoretical Signaling Pathways in Response to Hypoxia or (and) Infection of V. parahaemolyticus
All identified differential metabolites and proteins were used to draw Venn diagrams, which revealed that there were 22 metabolites and 14 proteins that changed under hypoxia or infection of V. parahaemolyticus. Sixteen metabolites and 6 proteins also changed under simultaneous stressors (Figure 5A). Importantly, the changing trends of these 22 metabolites or proteins were consistent in the three pairs of groups, which indicated that hypoxia and bacterial infection may aggravate each other. We examined the specific functions of these 22 metabolites or proteins and found that seven of them were principally involved in TGF-β1, hypoxia-inducible factor-1α (HIF-1α), and epidermal growth factor (EGF) signaling pathways (Figures 5B, C). Finally, a theoretical model of the host in response to hypoxia and bacterial infection was drawn through mechanisms that had been or had not been determined (Figure 6).
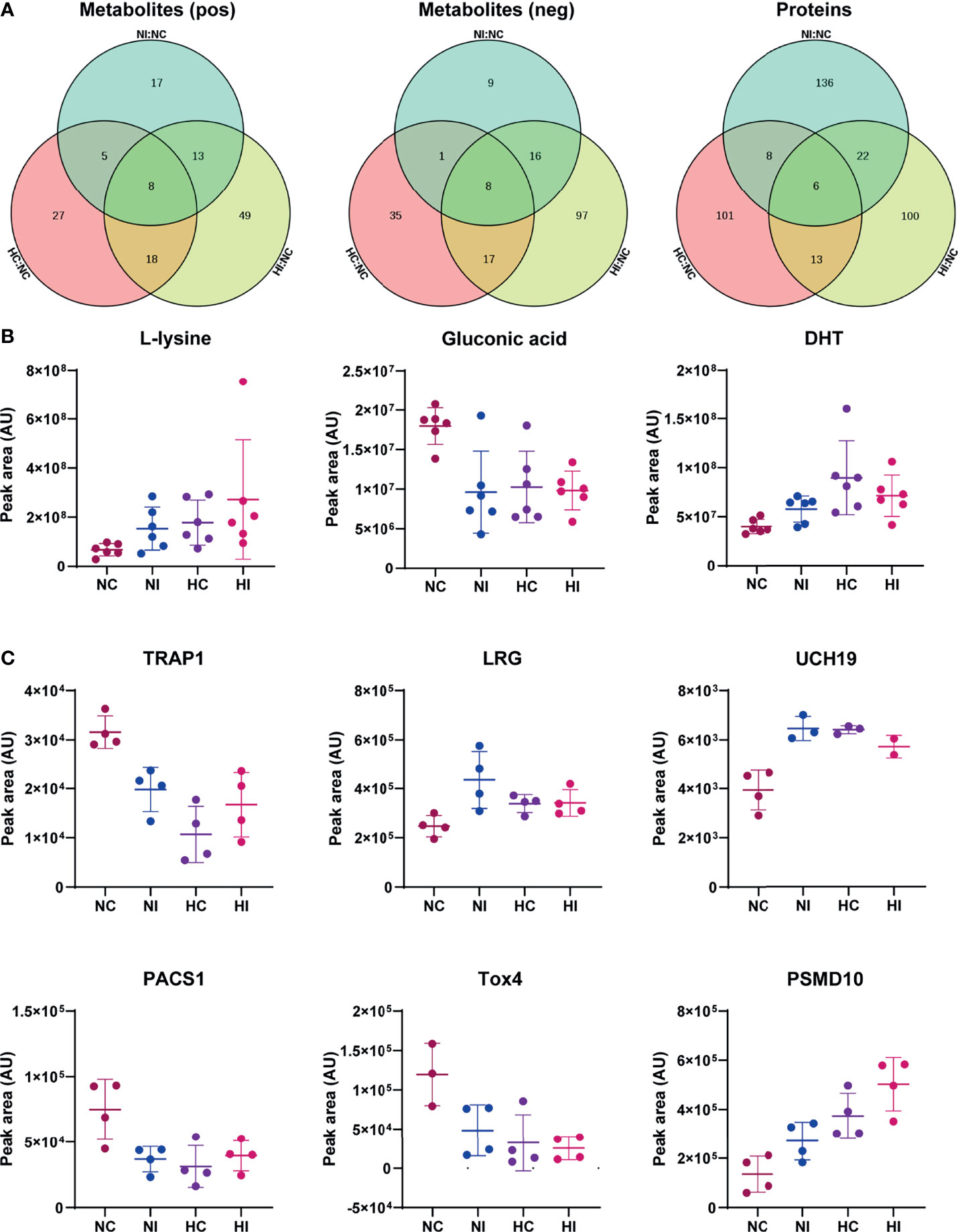
Figure 5 Common differential metabolites and proteins. The peak area was used for relative quantification. (A) Venn diagram of differential metabolites of the three compared groups (NC versus NI, NC versus HC, NC versus HI) in positive ion scanning mode; Venn diagram of differential metabolites of the three compared groups in negative ion scanning mode; Venn diagram of differential proteins of the three compared groups. (B) Three of the common differential metabolites. DHT: 5α-dihydrotestosterone. (C) The common differential proteins. TRAP1, TGF-β receptor-associated protein 1; LRG, leucine-rich alpha-2-glycoprotein; UCH19, ubiquitin C-terminal hydrolase 19; PACS1, phosphofurin acidic cluster sorting protein 1; Tox4, TOX high mobility group box family member 4; PSMD10, 26S protein non-ATPase regulatory subunit 10; AU, arbitrary unit. Error bars on graphs indicate mean ± SD.
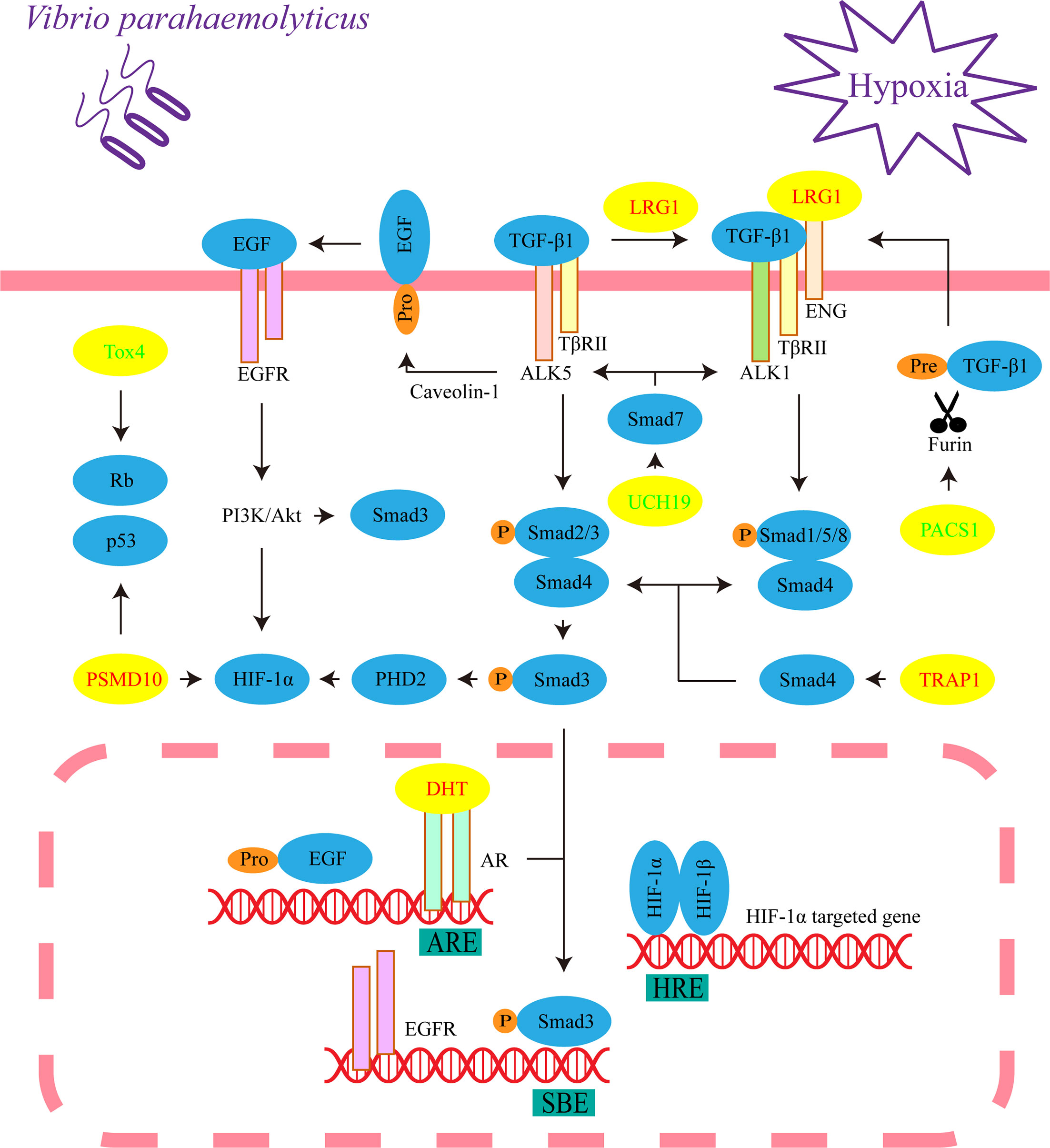
Figure 6 Critical signaling pathways in response to hypoxia and/or V. parahaemolyticus infection. This represents the cellular process of liver cells under three treatments (hypoxia, infection, and infection under hypoxia). The yellow ellipses indicate differential metabolites or proteins. The increased metabolites or proteins are shown in red font, while the decreased metabolites or proteins are shown in green font. ENG, endoglin.
Discussion
Enriched KEGG Pathways and GO Terms
According to the results of KEGG pathways and GO terms, B vitamins were found to play an important role in the effects of hypoxia and infection. VB1-derived molecules are important cofactors for numerous enzymes involved in energy production via the tricarboxylic acid (TCA) cycle (19). VB2 may promote the proliferation of neutrophils and monocytes and activate macrophages to enhance host resistance to infection (20). Sirtuin 1, an inhibitor of non-redox enzymes that helps cells survive under various stress conditions, converts nicotinamide adenine dinucleotide (NAD) to nicotinamide/VB3 (21). VB5 is necessary for coenzyme A (CoA) synthesis, and CoA is a key cofactor in TCA cycle and fatty acid metabolism (19). VB6 can be used as an inhibitor of cell proliferation (22), and VB7 deficiency is associated with increased inflammation (23), while VB9 deficiency has been shown to inhibit the proliferation of primary human CD8+ T lymphocytes (24). Taken together, B vitamins are the key factors that regulate immunity under hypoxia and bacterial infection. In addition, amino acid homeostasis was destroyed. A variety of amino acid metabolism was regulated, and lysine was significantly increased under hypoxia and infection (Figure 5B), however, little is known about its specific role.
Pattern recognition receptor NLRs signaling pathway was regulated under hypoxia, indicating that hypoxia may affect the host innate immunity against pathogens. Under infection, the metabolisms of serotonin and bile acid were modulated, and this might have regulated virulence of V. parahaemolyticus (25, 26). Sphingolipid, an important component of biofilm structure, plays an important role in cell signaling, and V. parahaemolyticus has been demonstrated to destroy the integrity of the host cell membrane (27). The other cellular processes enriched by KEGG pathways and GO terms may partly be attributed to the effectors secreted by V. parahaemolyticus because the identified effectors of the type III secretion system (T3SS) have been proven to control the cellular processes of the host (28). Under hypoxia and infection, mucin-type O-glycans was regulated, which was considered to have critical roles in defending mucus barrier integrity, and therefore regulated host–microbe interactions at mucosal sites (29). Taken together, these results represented the effects of hypoxia and infection on the host.
LRG Regulates TGF-β1 Signaling Pathway
We assigned TGF-β receptor-associated protein 1 (TRAP1), LRG, ubiquitin C-terminal hydrolase 19 (UCH19) and phosphofurin acidic cluster sorting protein 1 (PACS1) to TGF-β signal transduction, which is essential to maintain the homeostasis and normal immune function of the liver, pancreas and gastrointestinal system (30). The compound formed by TGF-β receptor I (TβR I, also known as activin receptor-like kinase, ALK) and TGF-β receptor II (TβR II) recognizes TGF-β and then phosphorylates the C-termini of the transcription factors Smad2 and Smad3. The common mediator Smad4 binds to phosphorylated Smad2 and Smad3 and translocated into the nucleus to regulate target gene expression (31). In the above processes, TRAP1, as a molecular chaperone of Smad4, assists it in moving to the compound and binding to Smad2 (32).
LRG1 is a highly conserved member of the leucine-rich repeat family of proteins and promotes the transition of TGF-β1 signaling from Smad2/3 to Smad1/5/8 signaling transduction, which leads to cell proliferation and pathogenic angiogenesis (33). Interestingly, LRG was significantly increased in the liver and significantly decreased in the heart in COVID-19 autopsies (3). Some recent studies indicated that LRG involved in regulations of EGF and HIF-1α signaling pathways (34, 35), and modulated kidney fibrosis (36). Therefore, we propose that LRG is widely involved in the transition of the TGF-β1/Smad signaling pathway in response to hypoxia and pathogenic infection in vertebrates as a conserved molecule.
Eukaryotes utilize the ubiquitin proteasome system (UPS) to degrade proteins, and TGF-β/Smad signal transduction is blocked by inhibitory Smad7, which recruits the ubiquitin ligase Smurf2 to form a complex that degrades the TβR compound through the UPS (37). However, ubiquitination is reversed by the deubiquitinating enzyme UCH37, which competitively binds Smad7 (38). In addition, UCH37 stabilizes Smad2/3 and promotes TGF-β1 signal transduction (39). Similarly, we propose that the reduction of UCH19 may promote the transition of TGF-β from Smad2/3 to Smad1/5/8 signaling transduction. A pathway is found to prevent the excessive activation of TGF-β signal transduction in the model. Furin, a member of the proprotein convertase family, is located in the trans-Golgi network (TGN), which catalyzes the maturation of TGF-β1 (40, 41). PACS1 is involved in controlling the correct subcellular localization of furin (42). Therefore, the reduction in PACS1 may inhibit the release of mature TGF-β1 by affecting the correct localization of furin to the TGN.
Fibrosis in the portal area of the liver may be related to the signal transduction of TGF-β1 (Figure 1C). It is believed that inflammatory monocytes, tissue-resident macrophages, activation of TGF-β1, cytokines and recognition of the microbiome by pattern recognition receptors all contribute to fibrosis (43). Repairs after injuries are related to the accumulation of collagen and fibronectin in the extracellular matrix. When the injuries are excessive, the accumulation of these components leads to excessive fibrosis (43). The accumulation is closely related to TGF-β1 (44). The activation of TGF-β1 mediated by integrin in the extracellular matrix results in activation of classical Smad signaling and Smad-independent signaling pathways, and they contribute to the expression of profibrotic genes and other transduction of signaling related to fibrosis (45).
EGF Signaling Pathway
Considering the correlation between protein kinase B (Akt) and Smad3, we assigned 5α-dihydrotestosterone (DHT) to the EGF signaling pathway, which plays an essential role in impairing apoptosis dominated by TGF-β1/Smad3 (46). The proapoptotic activity of the transcription factor Smad3 in hepatocytes requires nuclear translocation governed by C-terminal phosphorylation, and the activation of p38 mitogen-activated protein kinase is also required. In the nucleus, Smad3 enhances apoptosis by binding the promoter of Bcl-2 (an important inhibitor of apoptosis) to inhibit its transcription (47). However, Akt interacts directly with unphosphorylated Smad3 in the cytoplasm. At the same time, Akt phosphorylates and therefore inhibits the activities of some upstream kinases of p38 signaling (48–50). We propose that Akt may hinder the phosphorylation and nuclear translocation of Smad3.
Caveolin-1 (a structural protein of caveolae) and TGF-β1 mediate the correct localization and activation of metalloprotease TACE/ADAM17, which is necessary for the activation of EGF signaling (51, 52). After translocating to the nucleus, the complex formed by DHT and androgen receptors (ARs) may directly bind to the androgen response element (ARE) in the promoter region of the EGF gene, which simultaneously increases the expression of the EGFR gene (53–55). Interestingly, translocated Smad3 binds to the Smad-binding element (SBE) of the EGFR promoter and is rarely responsible for transcriptional activation (56). However, the DHT/AR complex inhibits TGF-β1/Smad3 transcriptional responses by repressing the binding of Smad3 to SBE (57). In conclusion, DHT may prevent the phosphorylation and nuclear translocation of Smad3, therefore inhibiting the apoptotic effect of TGF-β1/Smad3 signaling by activating EGF/phosphatidylinositol-3-kinase (PI3K)/Akt, this seems to be synergistic with LRG.
Bidirectional Regulation of HIF-1α Signaling Pathway
In this model, TGF-β1 and EGF signaling indicate a response of bidirectional regulation to HIF-1α. Under normoxic conditions, the prolyl hydroxylase PHD2 hydroxylates two proline residues of HIF-1α, and HIF-1α is degraded through the UPS (58, 59). However, hypoxia hinders this process, causing HIF-1α to translocate to the nucleus and form a dimer by interacting with HIF-1β. Then, the dimer binds to the hypoxia response element (HRE) to regulate gene transcription (60). TGF-β1 inhibits the gene transcription of PHD2 by activating Smad2/3 (61); therefore, the transition of TGF-β1 signaling from Smad2/3 to Smad1/5/8 signaling transduction may relieve this inhibition and ultimately promote the degradation of HIF-1α. In contrast, DHT induces PI3K/Akt activation through EGF signaling and promotes HIF-1α gene transcription and protein synthesis (53). In the proteomic results, HIF-1α is not a significantly differential protein, and this supports the bidirectional regulation.
Cell Proliferation, V. parahaemolyticus and Cancers
At present, cell proliferation seems to be a significant event under hypoxia and infection. Interestingly, the last two common differential proteins, TOX high mobility group box family member 4 (Tox4) and 26S protein non-ATPase regulatory subunit 10 (PSMD10, also known as gankyrin), reflect an association with Rb and p53. Rb and p53 are two key tumor suppressors that inhibit abnormal cell proliferation, and PSMD10 is their negative regulator (62, 63). Therefore, PSMD10 is overexpressed in numerous types of cancers, including hepatocellular carcinoma, breast cancer and pancreatic cancer (62, 64, 65). PSMD10 has been demonstrated to promote the proliferation, invasion, metastasis and angiogenesis of cancer cells through PI3K/Akt/HIF-1α signaling, which may depend on promoting the degradation of HIF-1α (66, 67). Phosphatase nuclear targeting subunit (PNUTS) is one of the target genes of HIF-1α, and it regulates the phosphorylation of Rb and p53 by binding to protein phosphatase-1 (PP1). Under hypoxia, the separation of PNUTS and PP1 leads to the dephosphorylation and activation of Rb, and the activity of p53 is enhanced (68, 69). Some evidence proposes that Tox4 is capable of binding to PNUTS and PP1 to form complexes; however, it is unclear how Tox4 is involved in regulating the activities of Rb and p53 (70, 71). Another report provides direct evidence that Tox4 inhibits cell proliferation (72). Accordingly, Tox4 may be a positive regulator of Rb and p53. In this model, the decrease in Tox4 and the increase in PSMD10 synergistically promoted cell proliferation. Gluconic acid is associated with cancers. Glucose oxidase reacts with intracellular glucose and O2 to produce hydrogen peroxide and gluconic acid (73); therefore, the decrease in gluconic acid means that glucose may be principally utilized for cell proliferation (Figure 5B).
Eleven biological agents are clearly designated carcinogens by the International Agency for Research on Cancer. Epstein Barr virus (EBV), Kaposi’s sarcoma herpes virus (KSHV) and Opisthorchis viverrini control cell proliferation as one of the carcinogenic mechanisms (74). The reason why V. parahaemolyticus stimulates the proliferation of host cells partially overlaps with EBV (75). A previous report principally attributed this activity to the effector VgpA of the T3SS of V. parahaemolyticus. VgpA translocates into host cells and binds to EBV nuclear antigen 1-binding protein 2 (EBP2) in the nucleus (76). c-Myc, the key transcription factors controlling cell growth, metabolism and angiogenesis, form a positive feedback loop to promote cancer cell proliferation with EBP2 (77). We provide evidence to support the purpose of focusing on the role of microbiota in changing the balance between host cell proliferation and death in cancer progression (78), especially those bacteria that deploy additional virulence factors such as V. parahaemolyticus.
Conclusion
The main finding of this study is that both of hypoxia and bacterial infection will lead to differences in some metabolites and proteins involved in TGF-β1, EGF and HIF-1α pathways, which may be related to pathological hypoxia caused by inflammation and represent conserved vertebrate signaling pathways. The specific effects of the pathways should be widely studied. In particular, it is necessary to study whether cell proliferation aggravates the metabolic burden. This study provides a basis for understanding how hypoxia destroys the immunity of animals and promotes disease progression.
Materials and Methods
Animals and Treatments of Stressors
Five hundred T. obscurus (length: from 7.5 to 8.0 cm, 10 months old) were purchased from Guangzhou Jinyang Aquaculture Company Limited, China. All fish were allowed to adapt laboratory conditions for 2 weeks. Then 400 T. obscurus were randomly divided into the NC (100 fish, normal dissolved oxygen concentrations without infection), NI (100 fish, normal dissolved oxygen concentrations with infection), HC (100 fish, hypoxic condition without infection) and HI (100 fish, hypoxic condition with infection) groups. Each group was treated in two repeated water tanks, and each 100 liters of water contained 50 fish. Before formal experiment, the remaining fish were used to determine the survival curve in preliminary experiment to determine the bacterial dose of the formal experiment.
Dissolved oxygen concentrations were controlled by adding nitrogen or O2 to the tanks. The dissolved oxygen concentrations of the normoxic and hypoxic groups were 7.60 ± 0.20 mg/L and 2.50 ± 0.20 mg/L, respectively (25.0 ± 0.5°C). V. parahaemolyticus was grown in Luria-Bertani medium, supplemented with NaCl to a final concentration of 3% (w/v), at 37°C. After reaching the logarithmic growth phase, V. parahaemolyticus was centrifuged at 5000 x g for 5 min and prepared the suspension with phosphate-buffered saline (PBS, Sangon Biotech, cat. no. E607008, China). After 12 h of hypoxic treatment, each fish in the infection group was injected with 5 * 107 cfu V. parahaemolyticus RIMD 2210633 as described before (the survival curve was measured in the independent preliminary experiment, and 10 T. obscurus in each infection group were injected with 5 * 107 or 5 * 109 cfu V. parahaemolyticus according to the same steps) (79, 80), and PBS was used as the control.
Sample Collection
After infection in the formal experiment, the oxygen concentration was maintained for 12 h. Then, six fish were taken from each group and anesthetized with 200 mg/L tricaine methanesulfonate (BIDE, cat. no. BD234866, China). For metabolomic and proteomic analyses, the entire liver of each fish (2.60 ± 0.32 g) was rapidly cut into small pieces of about 0.5 cm3 and transferred to a 15 ml centrifuge tube, then was stored in liquid nitrogen until omics analysis. For pathological observation, another 4 fish from each group were taken and anesthetized, the liver of each fish was rapidly cut into small pieces of about 0.5 cm3 and fixed in 4% paraformaldehyde overnight at 4°C before dehydration.
Pathological Observation
The livers from four fish in each group were treated with Advanced Smart Processor Vacuum Tissue Processor ASP300 S (Leica, Germany) before paraffin embedding. The clearing reagent was turpentine oil. The tissue was then embedded in paraffin and sliced to a thickness of 5 μm using the microtome RM2235 (Leica, Germany). After rehydration, the sections were stained with hematoxylin and eosin (81). And then the sections were mounted and visualized with versatile stereo microscope SMZ 800N (Nikon, Japan).
Metabolomic Analysis Based on Liquid Chromatography Coupled to Mass Spectrometry (LC–MS)
Considering the heterogeneity of liver tissue, the entire liver was pulverized in a mortar containing liquid nitrogen using a cryogenically cooled pestle for preparing homogenate (liver powder), and 100 mg of homogenate was resuspended in 500 μL of prechilled aqueous solution (80% methanol, 0.1% formic acid, Thermo Fisher Chemical, USA) by vortexing (82). After incubation on ice for 5 min, the mixture was centrifuged at 15,000 x g and 4°C for 20 min. Some of the supernatant was diluted to a final concentration containing 53% methanol by adding LC–MS grade water (Merck, cat. no. 1153331000). The samples were then transferred to a fresh centrifuge tube and centrifuged at 15,000 x g and 4°C for 20 min. The untargeted LC–MS system included a Vanquish UHPLC system (Thermo Fisher Scientific, USA) and an Orbitrap Q Exactive™ HF-X mass spectrometer (Thermo Fisher Scientific). Samples were injected into a C18 column (Hypesil Gold, 100 * 2.1 mm, 1.9 μm) using a 17-min linear gradient at a flow rate of 0.2 mL/min. Eluent A (0.1% formic acid in water) and eluent B (methanol) were used for positive polarity mode, while eluent A (5 mM ammonium acetate/Thermo Fisher Chemical, pH 9.0) and eluent B (methanol) were used for negative polarity mode. A gradient run was set up as 0-1.5 min at 2% B, 1.5-12.0 min from 2% to 100% B, 12.0-14.0 min at 100% B, 14.0-14.1 min from 100% to 2% B, 14.1-17 min at 2% B. The mass spectrometer was operated with a spray voltage of 3.2 kV, a capillary temperature of 320°C, a sheath gas flow rate of 40 arb and an aux gas flow rate of 10 arb (82).
Proteomic Analysis Based on Data-Independent Acquisition (DIA) Mode
The homogenate was lysed with PASP lysis buffer (100 mM NH4HCO3/Merck, cat. no. 5330050050, 8 M urea, pH 8) and ultrasonicated on ice for 5 min. After centrifugation, the supernatant was reduced with 10 mM dithiothreitol (Merck, cat. no. D9163) for 1 h at 56°C. The product was alkylated with moderate iodoacetamide (Merck, cat. no. I6125) for 1 h and then mixed with a fourfold volume of precooled acetone (Beijing Chemical Works, cat. no. 11241203810051, China) at -20°C for 2 h. After centrifugation, the precipitate was obtained and washed with 1 mL of precooled acetone. The final precipitate was collected and dissolved in dissolution buffer (8 M urea, 100 mM TEAB/Merck-cat. no. T7408, pH 8.5). For trypsin treatment, each protein sample was mixed with DB lysis buffer (8 M urea, 100 mM TEAB/Merck, cat. no. T7408, pH 8.5), and the volume was made up to 100 μL, while trypsin (Promega, cat. no. V5280) and 100 mM TEAB buffer were added for digestion (37°C, 4 h). Then, trypsin and CaCl2 were added for digestion overnight. Samples were acidified by formic acid and desalted in a C18 column. Peptides were resuspended in loading buffer containing 70% acetonitrile (Thermo Fisher Chemical, cat. no. W6-4) and 0.1% acetic acid. Finally, the eluents of each sample were collected and lyophilized.
Mobile phases A (2% acetonitrile, pH 10.0) and B (98% acetonitrile, pH 10.0) were used to develop a gradient elution. The lyophilized powder was dissolved in solution A and centrifuged at 12,000 x g for 10 min at room temperature. The sample was fractionated using a C18 column (Waters BEH C18, 4.6 * 250 mm, 5 μm) on an L3000 HPLC system (RIGOL, China), and the column oven was set as 45°C. The eluates were monitored at UV 214 nm, collected in one tube per minute and finally combined into four fractions. All fractions were dried under vacuum and then reconstituted in 0.1% (v/v) formic acid in water.
For gradient elution, mobile phases A (0.1% formic acid in H2O) and B (0.1% formic acid in 80% acetonitrile) were used. A half mixture containing 4 μg of fraction supernatant and 0.8 μL of iRT reagent (Biognosys, Switzerland) was injected into the EASY-nLC 1200 UHPLC system (Thermo Fisher Scientific), and peptides were separated using a 100-min linear gradient at a flow rate of 600 nL/min. MS data were acquired on a Q Exactive™ HF-X mass spectrometer (Thermo Fisher Scientific) operating with a spray voltage of 2.1 kV (Nanospray Flex™ electron spray ionization) and a capillary temperature of 320°C. For data-dependent acquisition (DDA) mode, the m/z range covered 350 to 1500 with a resolution of 120,000 (at m/z 200). The automatic gain control target value was 3 * 106, and the maximum ion injection time was 80 ms. The top 40 precursors of the highest abundance in the full scan were selected and fragmented by higher energy collisional dissociation and analyzed by MS/MS, where the resolution was 15,000 (at m/z 200). The automatic gain control target value was 5 * 104. The maximum ion injection time was 45 ms with a normalized collision energy of 27%, an intensity threshold of 1.1 * 104, and a dynamic exclusion parameter of 20 s. The raw MS detection data were used to construct a DDA spectrum library. For DIA mode, the m/z range covered from 350 to 1500. MS1 resolution was set to 60,000 (at 200 m/z). The full scan AGC target value was 5 * 105, and the maximum ion injection time was 20 ms. Peptides were fragmented by high-energy collision dissociation in MS2, in which the resolution was set to 30,000 (at 200 m/z). The AGC target value was 1 * 106, with a normalized collision energy of 27%.
Data Processing and Analysis
The raw metabolite data were processed using Compound Discoverer 3.1 (Thermo Fisher Scientific) to perform peak alignment, peak picking, and quantitation. The peaks were matched with the mzCloud (https://www.mzcloud.org/), mzVault and MassList databases. The metabolites were annotated using the KEGG PATHWAY Database (https://www.genome.jp/kegg/pathway.htmL), the Human Metabolome Database (https://hmdb.ca/metabolites) and the LIPID MAPS® Database (http://www.lipidmaps.org/). PCA and PLS-DA were performed at metaX (83). The screening criteria for differential metabolites were VIP > 1.0, fold change (FC) > 1.2 or FC < 0.833 and P value < 0.05 (Student’s t test). The raw data from the DDA mode were analyzed using Proteome Discoverer 2.2 (Thermo Fisher Scientific) on the basis of the genome of T. rubripes (https://www.ncbi.nlm.nih.gov/genome/63) to construct the DDA spectrum library, and the raw data from the DIA mode were imported to the DDA spectrum library to obtain chromatographic peaks. The protein quantitation results obtained from the calculation of peak area were statistically analyzed by Student’s t test. The screening criteria for differential proteins were FC > 1.2 or FC < 0.833 and P value < 0.05 (Student’s t test). GO analysis was conducted using InterProScan (European Bioinformatics Institute). KEGG pathway analysis was performed as described above. The specific information on all differential metabolites and proteins can be found in supplementary materials (Tables S4–12). Visualizations of Venn diagrams were performed using the OmicShare tools (https://www.omicshare.com/tools). The P values of KEGG and GO were obtained by hypergeometric testing, and the formula is as follows:
N: The number of proteins with GO annotation information in all proteins. n: the number of differential proteins in N. M: the number of proteins annotated to a GO entry in all proteins. x: The number of differential proteins annotated to a GO entry. The hypergeometric test for KEGG followed the same principle.
Data Availability Statement
The datasets presented in this study can be found in online repositories. The names of the repository/repositories and accession number(s) can be found below: http://www.proteomexchange.org/, PXD029027, https://db.cngb.org/, METM0000025.
Ethics Statement
The animal study was reviewed and approved by Institutional Animal Care and Use Committee (IACUC), Sun Yat-Sen University (Approval No. SYSU-IACUC-2021-B1920).
Author Contributions
DL and JX conceived the study. JX, HY, XY, SG, ND, and YL carried out the experiment. JX performed the data analysis and wrote the first draft of the manuscript. HL, YZ, and DL acquired funding, and all authors contributed substantially to revisions and approved the final manuscript.
Funding
This work was supported by the National Key R&D Program of China (2018YFD0900301), Research and Development Projects in Key Areas of Guangdong Province (2021B0202070002), the Science and Technology Planning Project of Guangzhou, China (201904020043), the Guangdong Provincial Special Fund for Modern Agriculture Industry Technology Innovation Teams (2019KJ143), and an Innovation Group Project of the Southern Marine Science and Engineering Guangdong Laboratory (Zhuhai) (311021006).
Conflict of Interest
The authors declare that the research was conducted in the absence of any commercial or financial relationships that could be construed as a potential conflict of interest.
Publisher’s Note
All claims expressed in this article are solely those of the authors and do not necessarily represent those of their affiliated organizations, or those of the publisher, the editors and the reviewers. Any product that may be evaluated in this article, or claim that may be made by its manufacturer, is not guaranteed or endorsed by the publisher.
Acknowledgments
We would like to thank Prof. Jianyi Pan of Zhejiang Sci-Tech University for presenting the strain as a gift and Prof. Rong Chen of Lingnan Normal University for insightful discussions. Novogene provided technical assistance for metabolomic and proteomic analyses. The ProteomeXchange Consortium (84) and the iProX partner repository (85) provide storage for the raw proteomic data. The China National GeneBank DataBase (86, 87) provides storage for the metabolomic raw data.
Supplementary Material
The Supplementary Material for this article can be found online at: https://www.frontiersin.org/articles/10.3389/fimmu.2021.825358/full#supplementary-material
References
1. Dong E, Du H, Gardner L. An Interactive Web-Based Dashboard to Track COVID-19 in Real Time. Lancet Infect Dis (2020) 20(5):533–4. doi: 10.1016/S1473-3099(20)30120-1
2. Singh S, McNab C, Olson RM, Bristol N, Nolan C, Bergstrøm E, et al. How an Outbreak Became a Pandemic: A Chronological Analysis of Crucial Junctures and International Obligations in the Early Months of the COVID-19 Pandemic. Lancet (2021) 398(10316):2109–24. doi: 10.1016/S0140-6736(21)01897-3
3. Nie X, Qian L, Sun R, Huang B, Dong X, Xiao Q, et al. Multi-Organ Proteomic Landscape of COVID-19 Autopsies. Cell (2021) 184(3):775–91. doi: 10.1016/j.cell.2021.01.004
4. Ackermann M, Verleden SE, Kuehnel M, Haverich A, Welte T, Laenger F, et al. Pulmonary Vascular Endothelialitis, Thrombosis, and Angiogenesis in Covid-19. N Engl J Med (2020) 383(2):120–8. doi: 10.1056/NEJMoa2015432
5. Bhattacharya S, Agarwal S, Shrimali NM, Guchhait P. Interplay Between Hypoxia and Inflammation Contributes to the Progression and Severity of Respiratory Viral Diseases. Mol Aspects Med (2021) 81:101000. doi: 10.1016/j.mam.2021.101000
6. Huang R, Huestis M, Gan ES, Ooi EE, Ohh M. Hypoxia and Viral Infectious Diseases. JCI Insight (2021) 6(7):e147190. doi: 10.1172/jci.insight.147190
7. Taylor CT, Colgan SP. Regulation of Immunity and Inflammation by Hypoxia in Immunological Niches. Nat Rev Immunol (2017) 17(12):774–85. doi: 10.1038/nri.2017.103
8. Campbell EL, Bruyninckx WJ, Kelly CJ, Glover LE, McNamee EN, Bowers BE, et al. Transmigrating Neutrophils Shape the Mucosal Microenvironment Through Localized Oxygen Depletion to Influence Resolution of Inflammation. Immunity (2014) 40(1):66–77. doi: 10.1016/j.immuni.2013.11.020
9. Colgan SP, Campbell EL, Kominsky DJ. Hypoxia and Mucosal Inflammation. Annu Rev Pathol (2016) 11:77–100. doi: 10.1146/annurev-pathol-012615-044231
10. Campbell EL, Kao DJ, Colgan SP. Neutrophils and the Inflammatory Tissue Microenvironment in the Mucosa. Immunol Rev (2016) 273(1):112–20. doi: 10.1111/imr.12456
11. Borregaard N, Herlin T. Energy Metabolism of Human Neutrophils During Phagocytosis. J Clin Invest (1982) 70(3):550–7. doi: 10.1172/jci110647
12. Gabig TG, Bearman SI, Babior BM. Effects of Oxygen Tension and pH on the Respiratory Burst of Human Neutrophils. Blood (1979) 53(6):1133–9. doi: 10.1182/blood.V53.6.1133.1133
13. Colgan SP, Taylor CT. Hypoxia: An Alarm Signal During Intestinal Inflammation. Nat Rev Gastroenterol Hepatol (2010) 7(5):281–7. doi: 10.1038/nrgastro.2010.39
14. Cossins AR, Crawford DL. Fish as Models for Environmental Genomics. Nat Rev Genet (2005) 6(4):324–33. doi: 10.1038/nrg1590
15. Brenner S, Elgar G, Sandford R, Macrae A, Venkatesh B, Aparicio S. Characterization of the Pufferfish (Fugu) Genome as a Compact Model Vertebrate Genome. Nature (1993) 366(6452):265–8. doi: 10.1038/366265a0
16. Roest Crollius H, Weissenbach J. Fish Genomics and Biology. Genome Res (2005) 15(12):1675–82. doi: 10.1101/gr.3735805
17. Baker-Austin C, Oliver JD, Alam M, Ali A, Waldor MK, Qadri F, et al. Vibrio Spp. Infections. Nat Rev Dis Primers (2018) 4(1):8. doi: 10.1038/s41572-018-0005-8
18. Wang J-B, Pu S-B, Sun Y, Li Z-F, Niu M, Yan X-Z, et al. Metabolomic Profiling of Autoimmune Hepatitis: The Diagnostic Utility of Nuclear Magnetic Resonance Spectroscopy. J Proteome Res (2014) 13(8):3792–801. doi: 10.1021/pr500462f
19. Peterson CT, Rodionov DA, Osterman AL, Peterson SN. B Vitamins and Their Role in Immune Regulation and Cancer. Nutrients (2020) 12(11):3380. doi: 10.3390/nu12113380
20. Araki S, Suzuki M, Fujimoto M, Kimura M. Enhancement of Resistance to Bacterial Infection in Mice by Vitamin B2. J Vet Med Sci (1995) 57(4):599–602. doi: 10.1292/jvms.57.599
21. Hwang ES, Song SB. Nicotinamide is an Inhibitor of SIRT1 In Vitro, But can be a Stimulator in Cells. Cell Mol Life Sci (2017) 74(18):3347–62. doi: 10.1007/s00018-017-2527-8
22. DiSorbo DM, Wagner R, Nathanson L. In Vivo and In Vitro Inhibition of B16 Melanoma Growth by Vitamin B6. Nutr Cancer (1985) 7(1-2):43–52. doi: 10.1080/01635588509513838
23. Agrawal S, Agrawal A, Said HM. Biotin Deficiency Enhances the Inflammatory Response of Human Dendritic Cells. Am J Physiol Cell Physiol (2016) 311(3):C386–C91. doi: 10.1152/ajpcell.00141.2016
24. Courtemanche C, Elson-Schwab I, Mashiyama ST, Kerry N, Ames BN. Folate Deficiency Inhibits the Proliferation of Primary Human CD8+ T Lymphocytes In Vitro. J Immunol (2004) 173(5):3186–92. doi: 10.4049/jimmunol.173.5.3186
25. Kumar A, Russell RM, Pifer R, Menezes-Garcia Z, Cuesta S, Narayanan S, et al. The Serotonin Neurotransmitter Modulates Virulence of Enteric Pathogens. Cell Host Microbe (2020) 28(1):41–53.e8. doi: 10.1016/j.chom.2020.05.004
26. Gotoh K, Kodama T, Hiyoshi H, Izutsu K, Park K-S, Dryselius R, et al. Bile Acid-Induced Virulence Gene Expression of Vibrio Parahaemolyticus Reveals a Novel Therapeutic Potential for Bile Acid Sequestrants. PloS One (2010) 5(10):e13365. doi: 10.1371/journal.pone.0013365
27. Broberg CA, Zhang L, Gonzalez H, Laskowski-Arce MA, Orth K. A Vibrio Effector Protein is an Inositol Phosphatase and Disrupts Host Cell Membrane Integrity. Science (2010) 329(5999):1660–2. doi: 10.1126/science.1192850
28. De Nisco NJ, Casey AK, Kanchwala M, Lafrance AE, Coskun FS, Kinch LN, et al. Manipulation of IRE1-Dependent MAPK Signaling by a Vibrio Agonist-Antagonist Effector Pair. mSystems (2021) 6(1):e00872–20. doi: 10.1128/mSystems.00872-20
29. Bergstrom KSB, Xia L. Mucin-Type O-Glycans and Their Roles in Intestinal Homeostasis. Glycobiology (2013) 23(9):1026–37. doi: 10.1093/glycob/cwt045
30. Gough NR, Xiang X, Mishra L. TGF-β Signaling in Liver, Pancreas, and Gastrointestinal Diseases and Cancer. Gastroenterology (2021) 161(2):434–52.e15. doi: 10.1053/j.gastro.2021.04.064
31. Pardali E, Goumans M-J, ten Dijke P. Signaling by Members of the TGF-Beta Family in Vascular Morphogenesis and Disease. Trends Cell Biol (2010) 20(9):556–67. doi: 10.1016/j.tcb.2010.06.006
32. Wurthner JU, Frank DB, Felici A, Green HM, Cao Z, Schneider MD, et al. Transforming Growth Factor-Beta Receptor-Associated Protein 1 is a Smad4 Chaperone. J Biol Chem (2001) 276(22):19495–502. doi: 10.1074/jbc.M006473200
33. Wang X, Abraham S, McKenzie JAG, Jeffs N, Swire M, Tripathi VB, et al. LRG1 Promotes Angiogenesis by Modulating Endothelial TGF-β Signalling. Nature (2013) 499(7458):306–11. doi: 10.1038/nature12345
34. Xie Z-B, Zhang Y-F, Jin C, Mao Y-S, Fu D-L. LRG-1 Promotes Pancreatic Cancer Growth and Metastasis via Modulation of the EGFR/p38 Signaling. J Exp Clin Cancer Res (2019) 38(1):75. doi: 10.1186/s13046-019-1088-0
35. Zhang J, Zhu L, Fang J, Ge Z, Li X. LRG1 Modulates Epithelial-Mesenchymal Transition and Angiogenesis in Colorectal Cancer via HIF-1α Activation. J Exp Clin Cancer Res (2016) 35:29. doi: 10.1186/s13046-016-0306-2
36. Hong Q, Cai H, Zhang L, Li Z, Zhong F, Ni Z, et al. Modulation of Transforming Growth Factor-β-Induced Kidney Fibrosis by Leucine-Rich ⍺-2 Glycoprotein-1. Kidney Int (2021) in press. doi: 10.1016/j.kint.2021.10.023
37. Kavsak P, Rasmussen RK, Causing CG, Bonni S, Zhu H, Thomsen GH, et al. Smad7 Binds to Smurf2 to Form an E3 Ubiquitin Ligase That Targets the TGF Beta Receptor for Degradation. Mol Cell (2000) 6(6):1365–75. doi: 10.1016/s1097-2765(00)00134-9
38. Wicks SJ, Haros K, Maillard M, Song L, Cohen RE, Dijke PT, et al. The Deubiquitinating Enzyme UCH37 Interacts With Smads and Regulates TGF-Beta Signalling. Oncogene (2005) 24(54):8080–4. doi: 10.1038/sj.onc.1208944
39. Nan L, Jacko AM, Tan J, Wang D, Zhao J, Kass DJ, et al. Ubiquitin Carboxyl-Terminal Hydrolase-L5 Promotes Tgfβ-1 Signaling by De-Ubiquitinating and Stabilizing Smad2/Smad3 in Pulmonary Fibrosis. Sci Rep (2016) 6:33116. doi: 10.1038/srep33116
40. Thomas G. Furin at the Cutting Edge: From Protein Traffic to Embryogenesis and Disease. Nat Rev Mol Cell Biol (2002) 3(10):753–66. doi: 10.1038/nrm934
41. Dubois CM, Blanchette F, Laprise MH, Leduc R, Grondin F, Seidah NG. Evidence That Furin is an Authentic Transforming Growth Factor-Beta1-Converting Enzyme. Am J Pathol (2001) 158(1):305–16. doi: 10.1016/s0002-9440(10)63970-3
42. Wan L, Molloy SS, Thomas L, Liu G, Xiang Y, Rybak SL, et al. PACS-1 Defines a Novel Gene Family of Cytosolic Sorting Proteins Required for Trans-Golgi Network Localization. Cell (1998) 94(2):205–16. doi: 10.1016/s0092-8674(00)81420-8
43. Henderson NC, Rieder F, Wynn TA. Fibrosis: From Mechanisms to Medicines. Nature (2020) 587(7835):555–66. doi: 10.1038/s41586-020-2938-9
44. Ignotz RA, Massagué J. Transforming Growth Factor-Beta Stimulates the Expression of Fibronectin and Collagen and Their Incorporation Into the Extracellular Matrix. J Biol Chem (1986) 261(9):4337–45. doi: 10.1016/S0021-9258(17)35666-1
45. Kim KK, Sheppard D, Chapman HA. TGF-β1 Signaling and Tissue Fibrosis. Cold Spring Harb Perspect Biol (2018) 10(4):a022293. doi: 10.1101/cshperspect.a022293
46. Murillo MM, del Castillo G, Sánchez A, Fernández M, Fabregat I. Involvement of EGF Receptor and C-Src in the Survival Signals Induced by TGF-Beta1 in Hepatocytes. Oncogene (2005) 24(28):4580–7. doi: 10.1038/sj.onc.1208664
47. Yang Y-A, Zhang G-M, Feigenbaum L, Zhang YE. Smad3 Reduces Susceptibility to Hepatocarcinoma by Sensitizing Hepatocytes to Apoptosis Through Downregulation of Bcl-2. Cancer Cell (2006) 9(6):445–57. doi: 10.1016/j.ccr.2006.04.025
48. Conery AR, Cao Y, Thompson EA, Townsend CM, Ko TC, Luo K. Akt Interacts Directly With Smad3 to Regulate the Sensitivity to TGF-Beta Induced Apoptosis. Nat Cell Biol (2004) 6(4):366–72. doi: 10.1038/ncb1117
49. Kim AH, Khursigara G, Sun X, Franke TF, Chao MV. Akt Phosphorylates and Negatively Regulates Apoptosis Signal-Regulating Kinase 1. Mol Cell Biol (2001) 21(3):893–901. doi: 10.1128/MCB.21.3.893-901.2001
50. Liao Y, Hung M-C. Regulation of the Activity of P38 Mitogen-Activated Protein Kinase by Akt in Cancer and Adenoviral Protein E1A-Mediated Sensitization to Apoptosis. Mol Cell Biol (2003) 23(19):6836–48. doi: 10.1128/MCB.23.19.6836-6848.2003
51. Moreno-Càceres J, Caja L, Mainez J, Mayoral R, Martín-Sanz P, Moreno-Vicente R, et al. Caveolin-1 is Required for TGF-β-Induced Transactivation of the EGF Receptor Pathway in Hepatocytes Through the Activation of the Metalloprotease TACE/Adam17. Cell Death Dis (2014) 5:e1326. doi: 10.1038/cddis.2014.294
52. Sunnarborg SW, Hinkle CL, Stevenson M, Russell WE, Raska CS, Peschon JJ, et al. Tumor Necrosis Factor-Alpha Converting Enzyme (TACE) Regulates Epidermal Growth Factor Receptor Ligand Availability. J Biol Chem (2002) 277(15):12838–45. doi: 10.1074/jbc.M112050200
53. Mabjeesh NJ, Willard MT, Frederickson CE, Zhong H, Simons JW. Androgens Stimulate Hypoxia-Inducible Factor 1 Activation via Autocrine Loop of Tyrosine Kinase Receptor/Phosphatidylinositol 3'-Kinase/Protein Kinase B in Prostate Cancer Cells. Clin Cancer Res (2003) 9(7):2416–25.
54. Zheng Y, Izumi K, Yao JL, Miyamoto H. Dihydrotestosterone Upregulates the Expression of Epidermal Growth Factor Receptor and ERBB2 in Androgen Receptor-Positive Bladder Cancer Cells. Endocr Relat Cancer (2011) 18(4):451–64. doi: 10.1530/ERC-11-0010
55. Brass AL, Barnard J, Patai BL, Salvi D, Rukstalis DB. Androgen Up-Regulates Epidermal Growth Factor Receptor Expression and Binding Affinity in PC3 Cell Lines Expressing the Human Androgen Receptor. Cancer Res (1995) 55(14):3197–203.
56. Zhao Y, Ma J, Fan Y, Wang Z, Tian R, Ji W, et al. TGF-β Transactivates EGFR and Facilitates Breast Cancer Migration and Invasion Through Canonical Smad3 and ERK/Sp1 Signaling Pathways. Mol Oncol (2018) 12(3):305–21. doi: 10.1002/1878-0261.12162
57. Chipuk JE, Cornelius SC, Pultz NJ, Jorgensen JS, Bonham MJ, Kim S-J, et al. The Androgen Receptor Represses Transforming Growth Factor-Beta Signaling Through Interaction With Smad3. J Biol Chem (2002) 277(2):1240–8. doi: 10.1074/jbc.M108855200
58. Ivan M, Kondo K, Yang H, Kim W, Valiando J, Ohh M, et al. HIFalpha Targeted for VHL-Mediated Destruction by Proline Hydroxylation: Implications for O2 Sensing. Science (2001) 292(5516):464–8. doi: 10.1126/science.1059817
59. Epstein AC, Gleadle JM, McNeill LA, Hewitson KS, O'Rourke J, Mole DR, et al. C. Elegans EGL-9 and Mammalian Homologs Define a Family of Dioxygenases That Regulate HIF by Prolyl Hydroxylation. Cell (2001) 107(1):43–54. doi: 10.1016/s0092-8674(01)00507-4
60. Lisy K, Peet DJ. Turn Me on: Regulating HIF Transcriptional Activity. Cell Death Differ (2008) 15(4):642–9. doi: 10.1038/sj.cdd.4402315
61. McMahon S, Charbonneau M, Grandmont S, Richard DE, Dubois CM. Transforming Growth Factor Beta1 Induces Hypoxia-Inducible Factor-1 Stabilization Through Selective Inhibition of PHD2 Expression. J Biol Chem (2006) 281(34):24171–81. doi: 10.1074/jbc.M604507200
62. Higashitsuji H, Itoh K, Nagao T, Dawson S, Nonoguchi K, Kido T, et al. Reduced Stability of Retinoblastoma Protein by Gankyrin, an Oncogenic Ankyrin-Repeat Protein Overexpressed in Hepatomas. Nat Med (2000) 6(1):96–9. doi: 10.1038/71600
63. Higashitsuji H, Higashitsuji H, Itoh K, Sakurai T, Nagao T, Sumitomo Y, et al. The Oncoprotein Gankyrin Binds to MDM2/HDM2, Enhancing Ubiquitylation and Degradation of P53. Cancer Cell (2005) 8(1):75–87. doi: 10.1016/j.ccr.2005.06.006
64. Zhen C, Chen L, Zhao Q, Liang B, Gu YX, Bai ZF, et al. Gankyrin Promotes Breast Cancer Cell Metastasis by Regulating Rac1 Activity. Oncogene (2013) 32(29):3452–60. doi: 10.1038/onc.2012.356
65. Meng Y, He L, Guo X, Tang S, Zhao X, Du R, et al. Gankyrin Promotes the Proliferation of Human Pancreatic Cancer. Cancer Lett (2010) 297(1):9–17. doi: 10.1016/j.canlet.2010.04.019
66. Fu J, Chen Y, Cao J, Luo T, Qian Y-W, Yang W, et al. P28gank Overexpression Accelerates Hepatocellular Carcinoma Invasiveness and Metastasis via Phosphoinositol 3-Kinase/AKT/hypoxia-Inducible Factor-1α Pathways. Hepatology (2011) 53(1):181–92. doi: 10.1002/hep.24015
67. Chen J, Bai M, Ning C, Xie B, Zhang J, Liao H, et al. Gankyrin Facilitates Follicle-Stimulating Hormone-Driven Ovarian Cancer Cell Proliferation Through the PI3K/AKT/HIF-1α/Cyclin D1 Pathway. Oncogene (2016) 35(19):2506–17. doi: 10.1038/onc.2015.316
68. Lee SJ, Lim CJ, Min JK, Lee JK, Kim YM, Lee JY, et al. Protein Phosphatase 1 Nuclear Targeting Subunit is a Hypoxia Inducible Gene: Its Role in Post-Translational Modification of P53 and MDM2. Cell Death Differ (2007) 14(6):1106–16. doi: 10.1038/sj.cdd.4402111
69. Udho E, Tedesco VC, Zygmunt A, Krucher NA. PNUTS (Phosphatase Nuclear Targeting Subunit) Inhibits Retinoblastoma-Directed PP1 Activity. Biochem Biophys Res Commun (2002) 297(3):463–7. doi: 10.1016/s0006-291x(02)02236-2
70. Lee SJ, Lee JK, Maeng YS, Kim YM, Kwon YG. Langerhans Cell Protein 1 (LCP1) Binds to PNUTS in the Nucleus: Implications for This Complex in Transcriptional Regulation. Exp Mol Med (2009) 41(3):189–200. doi: 10.3858/emm.2009.41.3.022
71. Lee J-H, You J, Dobrota E, Skalnik DG. Identification and Characterization of a Novel Human PP1 Phosphatase Complex. J Biol Chem (2010) 285(32):24466–76. doi: 10.1074/jbc.M110.109801
72. Vanheer L, Song J, De Geest N, Janiszewski A, Talon I, Provenzano C, et al. Tox4 Modulates Cell Fate Reprogramming. J Cell Sci (2019) 132(20):jcs232223. doi: 10.1242/jcs.232223
73. Fu L-H, Qi C, Lin J, Huang P. Catalytic Chemistry of Glucose Oxidase in Cancer Diagnosis and Treatment. Chem Soc Rev (2018) 47(17):6454–72. doi: 10.1039/c7cs00891k
74. Bouvard V, Baan R, Straif K, Grosse Y, Secretan B, El Ghissassi F, et al. A Review of Human Carcinogens–Part B: Biological Agents. Lancet Oncol (2009) 10(4):321–2. doi: 10.1016/s1470-2045(09)70096-8
75. Shire K, Ceccarelli DF, Avolio-Hunter TM, Frappier L. EBP2, a Human Protein That Interacts With Sequences of the Epstein-Barr Virus Nuclear Antigen 1 Important for Plasmid Maintenance. J Virol (1999) 73(4):2587–95. doi: 10.1128/JVI.73.4.2587-2595.1999
76. Hu M, Zhang Y, Gu D, Chen X, Waldor MK, Zhou X. Nucleolar C-Myc Recruitment by a Vibrio T3SS Effector Promotes Host Cell Proliferation and Bacterial Virulence. EMBO J (2021) 40(2):e105699. doi: 10.15252/embj.2020105699
77. Liao P, Wang W, Shen M, Pan W, Zhang K, Wang R, et al. A Positive Feedback Loop Between EBP2 and C-Myc Regulates rDNA Transcription, Cell Proliferation, and Tumorigenesis. Cell Death Dis (2014) 5:e1032. doi: 10.1038/cddis.2013.536
78. Garrett WS. Cancer and the Microbiota. Science (2015) 348(6230):80–6. doi: 10.1126/science.aaa4972
79. Peng W, Shi Y, Li G-F, He L-G, Liang Y-S, Zhang Y, et al. Tetraodon Nigroviridis: A Model of Vibrio Parahaemolyticus Infection. Fish Shellfish Immunol (2016) 56:388–96. doi: 10.1016/j.fsi.2016.07.017
80. Chimalapati S, Lafrance AE, Chen L, Orth K. Vibrio Parahaemolyticus: Basic Techniques for Growth, Genetic Manipulation, and Analysis of Virulence Factors. Curr Protoc Microbiol (2020) 59(1):e131. doi: 10.1002/cpmc.131
81. Zheng B, Li S, Liu Y, Li Y, Chen H, Tang H, et al. Spexin Suppress Food Intake in Zebrafish: Evidence From Gene Knockout Study. Sci Rep (2017) 7(1):14643. doi: 10.1038/s41598-017-15138-6
82. Want EJ, Masson P, Michopoulos F, Wilson ID, Theodoridis G, Plumb RS, et al. Global Metabolic Profiling of Animal and Human Tissues via UPLC-Ms. Nat Protoc (2013) 8(1):17–32. doi: 10.1038/nprot.2012.135
83. Wen B, Mei Z, Zeng C, Liu S. Metax: A Flexible and Comprehensive Software for Processing Metabolomics Data. BMC Bioinf (2017) 18(1):183. doi: 10.1186/s12859-017-1579-y
84. Deutsch EW, Csordas A, Sun Z, Jarnuczak A, Perez-Riverol Y, Ternent T, et al. The ProteomeXchange Consortium in 2017: Supporting the Cultural Change in Proteomics Public Data Deposition. Nucleic Acids Res (2017) 45(D1):D1100–D6. doi: 10.1093/nar/gkw936
85. Ma J, Chen T, Wu S, Yang C, Bai M, Shu K, et al. Iprox: An Integrated Proteome Resource. Nucleic Acids Res (2019) 47(D1):D1211–D7. doi: 10.1093/nar/gky869
86. Chen FZ, You LJ, Yang F, Wang LN, Guo XQ, Gao F, et al. CNGBdb: China National GeneBank DataBase. Yi Chuan (2020) 42(8):799–809. doi: 10.16288/j.yczz.20-080
Keywords: fibrosis, cell proliferation, LRG, TGF-β1, HIF-1α, EGF
Citation: Xu J, Yu X, Ye H, Gao S, Deng N, Lu Y, Lin H, Zhang Y and Lu D (2022) Comparative Metabolomics and Proteomics Reveal Vibrio parahaemolyticus Targets Hypoxia-Related Signaling Pathways of Takifugu obscurus. Front. Immunol. 12:825358. doi: 10.3389/fimmu.2021.825358
Received: 30 November 2021; Accepted: 23 December 2021;
Published: 13 January 2022.
Edited by:
Qing Wang, Chinese Academy of Fishery Sciences, ChinaReviewed by:
Mohsin Ahmad Ghauri, Xi’an Jiaotong University, ChinaNan Wu, Institute of Hydrobiology (CAS), China
Copyright © 2022 Xu, Yu, Ye, Gao, Deng, Lu, Lin, Zhang and Lu. This is an open-access article distributed under the terms of the Creative Commons Attribution License (CC BY). The use, distribution or reproduction in other forums is permitted, provided the original author(s) and the copyright owner(s) are credited and that the original publication in this journal is cited, in accordance with accepted academic practice. No use, distribution or reproduction is permitted which does not comply with these terms.
*Correspondence: Yong Zhang, bHNzenlAbWFpbC5zeXN1LmVkdS5jbg==; Danqi Lu, bHVkYW5xaUBtYWlsLnN5c3UuZWR1LmNu