- 1Shanghai Institute of Hematology, State Key Laboratory of Medical Genomics, National Research Center for Translational Medicine at Shanghai, Ruijin Hospital, Shanghai Jiao Tong University School of Medicine, Shanghai, China
- 2Department of Pathology, Ruijin Hospital, Shanghai Jiao Tong University School of Medicine, Shanghai, China
- 3Department of Ultrasound, Ruijin Hospital, Shanghai Jiao Tong University School of Medicine, Shanghai, China
- 4State Key Laboratory of Microbial Metabolism, School of Life Sciences and Biotechnology, Shanghai Jiao Tong University, Shanghai, China
- 5Laboratory of Molecular Pathology, Pôle de Recherches Sino-Français en Science du Vivant et Génomique, Shanghai, China
The incidence of diffuse large B-cell lymphoma (DLBCL) increases by age and older DLBCL are commonly related to poor prognosis. However, the clinical and biological features of older DLBCL patients remain to be determined. A total of 2,445 patients with newly diagnosed DLBCL were enrolled for clinical data analysis according to age at diagnosis, with tumor samples of 1,150 patients assessed by DNA sequencing and 385 patients by RNA sequencing. Older DLBCL presented advanced disease stage, elevated serum lactate dehydrogenase, poor performance status, multiple extranodal involvement, high percentage of double expressor subtype, and adverse clinical outcome. According to molecular features, age was positively correlated with the oncogenic mutations of PIM1, MYD88, BTG2, CD79B, TET2, BTG1, CREBBP, TBL1XR1, and with the MYD88-like genetic subtype. These oncogenic mutations were involved in B-cell receptor/NF-κB signaling, B-cell differentiation, and histone acetylation based on biological functions. Older DLBCL also manifested reduction in CD4+ naïve T and CD8+ naïve T cells, and also increased recruitment of exhausted T cells and macrophages, leading to immunosuppressive tumor microenvironment. Our work thus contributes to the understanding of aging-related oncogenic mutations and tumor microenvironment alterations in lymphoma progression, and may provide new insights to mechanism-based targeted therapy in DLBCL.
Introduction
Diffuse large B-cell lymphoma (DLBCL) is the most common aggressive non-Hodgkin lymphoma, with the incidence increased by age. Age >60 at diagnosis is an important risk factor of the International Prognostic Index (IPI), indicating unfavorable clinical outcomes of patients treated by rituximab in combination with cyclophosphamide, doxorubicin, vincristine, and prednisone (R-CHOP) (1). More recently, the National Comprehensive Cancer Network database (NCCN)-IPI has further categorized DLBCL patients into 4 age groups (≤40 years, 41–60 years, 61–75 years, and >75 years) with increasing hazard ratio for inferior overall survival (2). As older DLBCL often present poor baseline health status and intolerance to immunochemotherapy, personalized therapy for older patients remain unmet clinical needs, which may rely on specific molecular features associated with age, especially oncogenic mutations and tumor microenvironment.
As reported, somatic mutations accumulate with age and may be related to tumor progression (3). In DLBCL, MYD88, PIM1, and CD79B mutations were more frequently observed in older patients (4, 5). Although less often occurred in lymphoid malignancies, TET2 mutations were reported as age-related in other hematological malignancies (6, 7). In addition, immunosenescence contributes to reduced functioning of the immune system with aging (8). As an important feature of immunosenescence, the output of naïve T cells decreases after thymic involution, thereby altering the distribution of CD4+ and CD8+ naïve T cells in peripheral blood and immune organ (9). Within the tumors, T-cell exhaustion is regulated by immunosuppressive cytokines such as IL-10, and immune cells like macrophages, manifested by loss of effector functions and overexpression of inhibitory receptors like programmed cell death protein-1 (PD-1) (10). Particularly, immunosuppressive M2 macrophages accumulate in older lymphoid tissues and regulate T-cell functions by secreting immunosuppressive cytokines and expressing ligands to inhibitory receptors, and promoting tumor growth and metastasis (11). However, the clinical and biological features of older DLBCL patients remain to be determined.
In this study, we investigated the clinical characteristics and prognostic significance linked to age at diagnosis in a large cohort of 2,445 patients with newly diagnosed DLBCL, and performed genomic and transcriptomic analyses to illustrate the oncogenic mutations and tumor microenvironment alterations associated with older patients.
Methods
Patients
A flow chart is outlined in Figure 1 to summarize the patient selection. From September 2002 to April 2021, a total of 2,445 patients with newly diagnosed DLBCL were included, with the last follow-up through August 31, 2021. Histological diagnosis was established based on the World Health Organization (WHO) classification reviewed by two experienced pathologists, LD and HY (12). Survival analysis was performed on 1,896 patients receiving R-CHOP-based immunochemotherapy. DNA and RNA sequencing were performed on 1,150 and 385 patients with available tumor and blood samples, respectively, for detection of genetic aberrations, tumor microenvironmental analysis, and gene set enrichment analysis (GSEA). This study was approved by the Shanghai Rui Jin Hospital Review Board with informed consent obtained in accordance with the Declaration of Helsinki.
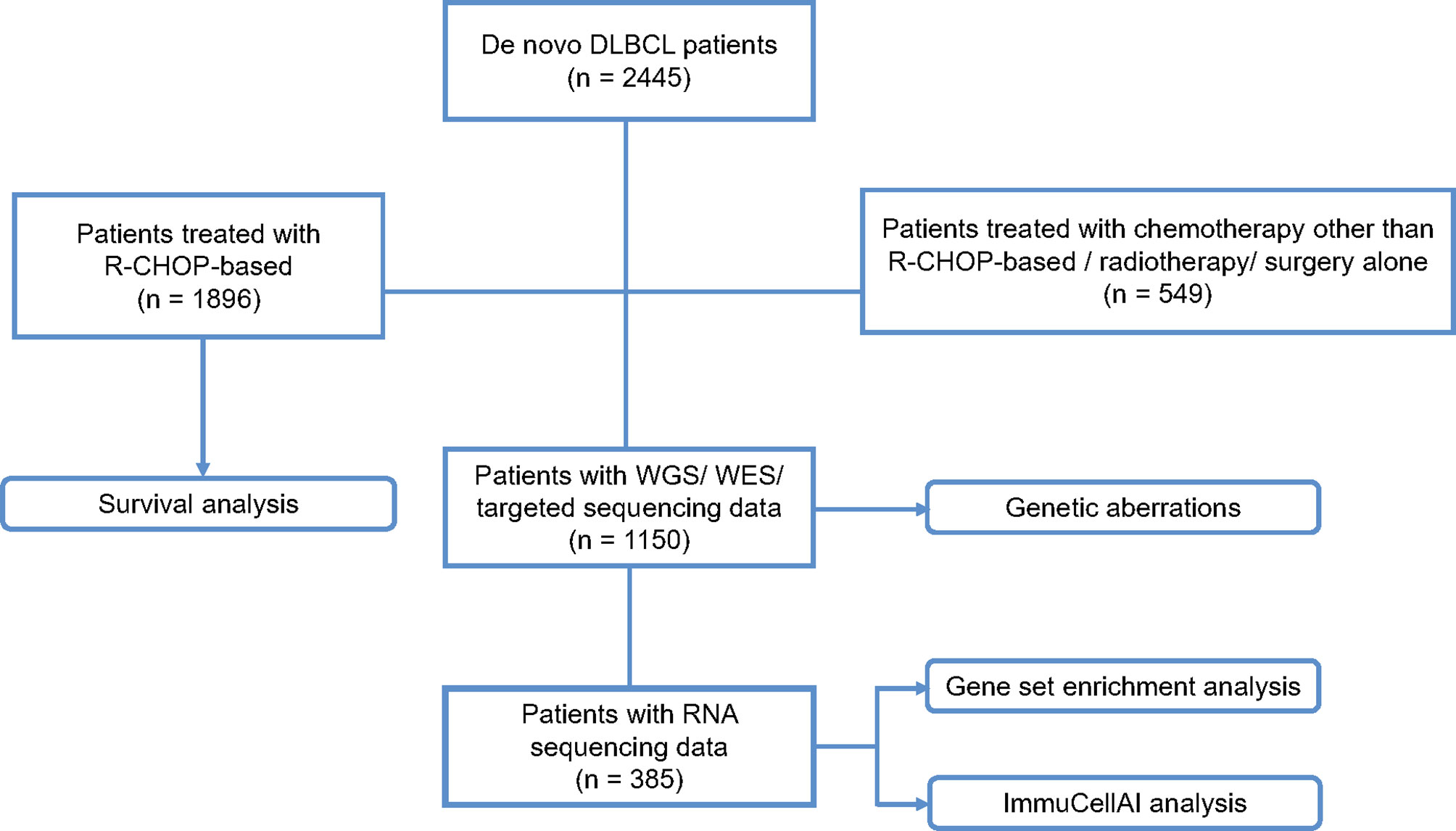
Figure 1 Flow chart of the patient selection and methods. DLBCL, diffuse large B-cell lymphoma; R-CHOP, rituximab, cyclophosphamide, doxorubicin, vincristine and prednisone; WGS, whole genome sequencing; WES, whole exome sequencing.
DNA Sequencing
DNA sequencing of 340 patients by whole-genome sequencing (WGS, n = 117) and whole-exome sequencing (WES, n = 223) were performed on available frozen or quality controlled FFPE tumor samples, as reported by our previous studies, along with detailed procedures for DNA sequencing (13–15). Targeted sequencing of 55 lymphoma associated genes were performed among 810 patients with available FFPE tumor samples based on the criteria as previously described (13–15). The mean depth of samples sequenced by WES and WGS was 120.25× (range 50–200×), with an average 97.65% (range 82.64–99.06%) of the target sequence being covered sufficiently deep for variant calling (≥10× coverage). The mean depth of samples sequenced by targeted sequencing was 1,351× (range 505–3,224×), with an average 88.57% (range 59.56–98.26%) of the target sequence being covered sufficiently deep for variant calling (200× coverage). Single nucleotide variations (SNVs) and indels were called by Genome Analysis Toolkit (GATK, v3.4) Haplotype Caller, and GATK Unified Genotyper and mapped to the genome location using the UCSC Genome Browser (http://genome.ucsc.edu) for annotation. The filtration of SNVs and indels was carried out by homemade pipeline with the software mentioned above. Variant allele frequency of mutations included should be over 5%. Mutations were filtered according to the rules listed below. Mutations were preserved if they met the following conditions: 1) sites reported as somatic mutations in our previous studies (13–15); 2) sites verified as somatic mutations by sequencing on paired blood samples; 3) sites commonly considered as hotspot mutations like MYD88L265P; 4) sites categorized into tier I and II variants according to the Guideline for Evidence-Based Categorization of Somatic Variants (16); 5) sites not included in the SNP database, or related to hematological malignancies (N >5) as reported in the COSMIC (the Catalogue of Somatic Mutations in Cancer). Mutations were excluded if they met the following conditions: 1) sites verified as germline mutations by sequencing on paired blood samples; 2) sites categorized into tier IV variants according to the Guideline for Evidence-Based Categorization of Somatic Variants; and 3) sites with extraordinarily high frequencies but never reported in previous studies. The mutation data among the 55 genes are listed in Supplementary Table 1. Clinical and pathological features of patients with WGS/WES/targeted sequencing data are shown in Supplementary Table 2. No statistically significant difference was found in the frequencies of mutations detected by WGS/WES/targeted sequencing called in the components of the cohort after balancing the baseline of clinical and pathological characteristics using propensity score matching, except DDX3X (Supplementary Tables 3, 4).
Based on the Gene Ontology database, 55 mutated genes were assigned to biological processes, namely, chromatin organization, immune response, cell-cycle/p53, and also oncogenic signaling pathways B-cell receptor (BCR)/NF-κB, JAK-STAT, PI3K-AKT, and Wnt. The list of genes involved in biological function is shown in Supplementary Table 5.
RNA Sequencing, ImmuCellAI Analysis and GSEA Analysis
RNA sequencing was performed on 385 patients with available frozen tumor samples. A total of 361 patients of them were from our previous studies (13–15) and 24 patients were newly analyzed, namely, 98 patients with WGS, 98 with WES, and 189 with targeted sequencing data, respectively. Clinical and pathological features of patients with RNA sequencing data are shown in Supplementary Tables 6–8. RNA was extracted by Trizol and an RNeasy Mini Kit (Qiagen) from available qualified frozen tumor samples of 385 patients. RNA was purified by Ribo-Zero rRNA Removal Kits (Illumina). RNA concentration was assessed on NanoDrop and integrity by an Agilent 2100 Bioanalyzer. RNA libraries were generated with a TruSeq RNA Library Preparation Kit (Illumina) based on instructions of the manufacturer. The concentration and the quality of RNA libraries were controlled by Qubit and BioAnalyzer 2100 system. Paired-end sequencing was performed on Illumina HiSeq sequencer following Illumina-provided protocols. The read pairs were aligned to Refseq hg19 through Burrows‐Wheeler Aligner version 0.7.13‐r1126. The transcript counts table files were generated by the HTSeq (17). Potential false positive results were excluded by visual inspection. R package “sva” was applied to remove batch effect by r 4.0.3. The expressional data related are shown in Supplementary Table 9. Cell of origin classification based on gene expression was performed using Lymph2Cx assay (18). Based on raw counts data of RNA sequencing, the infiltration of immune cells including 24 immune cell types were estimated by ImmuCellAI algorithm, a website tool (http://bioinfo.life.hust.edu.cn/web/ImmuCellAI/) (19). Pathway enrichment analysis was performed using GSEA v4.0.1 software as recommended by the GSEA team (http://www.broadinstitute.org/gsea). Pathways were considered of statistical significance with a P-value <0.05 and a false discovery rate <0.25.
Immunohistochemistry
Immunohistochemistry was performed on paraffin sections using antibodies against CD10, BCL6, MUM1, BCL2, and MYC by indirect immunoperoxidase method. Germinal center B-cell-like (GCB) and non-GCB phenotypes were determined with 30% cutoff values of CD10, BCL6, and MUM1, according to Han’s algorithm (20). BCL2/MYC double-expressors were defined by BCL-2 and MYC with cutoff values of 50 and 40%, respectively (12).
Fluorescence In-Situ Hybridization
Fluorescence in-situ hybridization of BCL2, BCL6, and MYC rearrangements was performed on paraffin sections with 10% cutoff values. The results in detail are listed in Supplementary Table 10.
Molecular Classification
DLBCL genotypes were identified as described by Lacy et al. using the 47 genes available among 1,150 patients with DNA sequencing data (R code version, https://github.com/ecsg-uoy/DLBCLGenomicSubtyping) (21).
Statistical Analysis
Pearson’s χ2 test or Fisher’s exact test was used to analyze baseline characteristics of patients. Progression-free survival (PFS) was calculated from the date of diagnosis to the date when disease progression or relapse was recognized or the date of last follow-up. Overall survival (OS) was measured from the date of diagnosis to the date of death or the date of last follow-up. Survival functions were analyzed using the Kaplan–Meier method and compared by the log-rank test. Univariate hazard was analyzed using the Cox regression method and the significant variables were then kept in multivariate set. Normalized gene expression in two groups was analyzed using Mann–Whitney U test. All statistical analysis was performed by Statistical Package for the Social Sciences (SPSS) 26.0 software. All tests were two-sided, with statistical significance defined as P <0.05.
Results
Clinical and Pathological Characteristics of DLBCL Patients Based on Age at Diagnosis
Among 2,445 patients with newly diagnosed DLBCL, 1,140 patients were >60 years old and 1,305 patients were ≤60 years old at diagnosis. The clinical characteristics of the patients are listed in Table 1. Patients diagnosed at age >60 years were associated with advanced Ann Arbor stage (P <0.001), elevated serum lactate dehydrogenase (LDH) (P <0.001), poor performance status (P <0.001), multiple extranodal involvement (P = 0.001), high percentage of double expressor subtype (P = 0.012), as compared to those at age ≤60 years. No significant difference was observed between the two age groups, according to gender, cell of origin (Hans), cell of origin (Lymph2Cx), or double/triple-hit.
Survival Analysis and Prognostic Significance of DLBCL Patients Based on Age at Diagnosis
Among 1,896 patients receiving R-CHOP-based immunochemotherapy, the median follow-up time was 55.0 months (0.2–224.2 months). The 3-year PFS and OS rates of patients diagnosed at age >60 years were 60.8 and 71.0%, significantly lower than those of patients at age ≤60 years (72.1%, P <0.001; 83.2%, P <0.001, respectively) (Figures 2A, B). Using univariate analysis, the 5 factors of IPI were of great significance and were included in the multivariate analysis. In the Cox proportional-hazards model, independent prognostic factors of inferior PFS and OS were age (P both <0.001), along with Ann Arbor stage (P both <0.001), serum LDH (P both <0.001), performance status (P both <0.001), and multiple extranodal involvement (P both <0.05) (Table 2). Similar results were observed based on age factor according to NCCN-IPI. The 3-year PFS and OS rates of patients >75 years were 51.2 and 57.6%, significantly lower than those of patients 61–75 years (62.0 and 72.8%), 41–60 years (72.5 and 83.4%), and ≤40 years (71.4 and 82.8%) (Figures 2C, D).
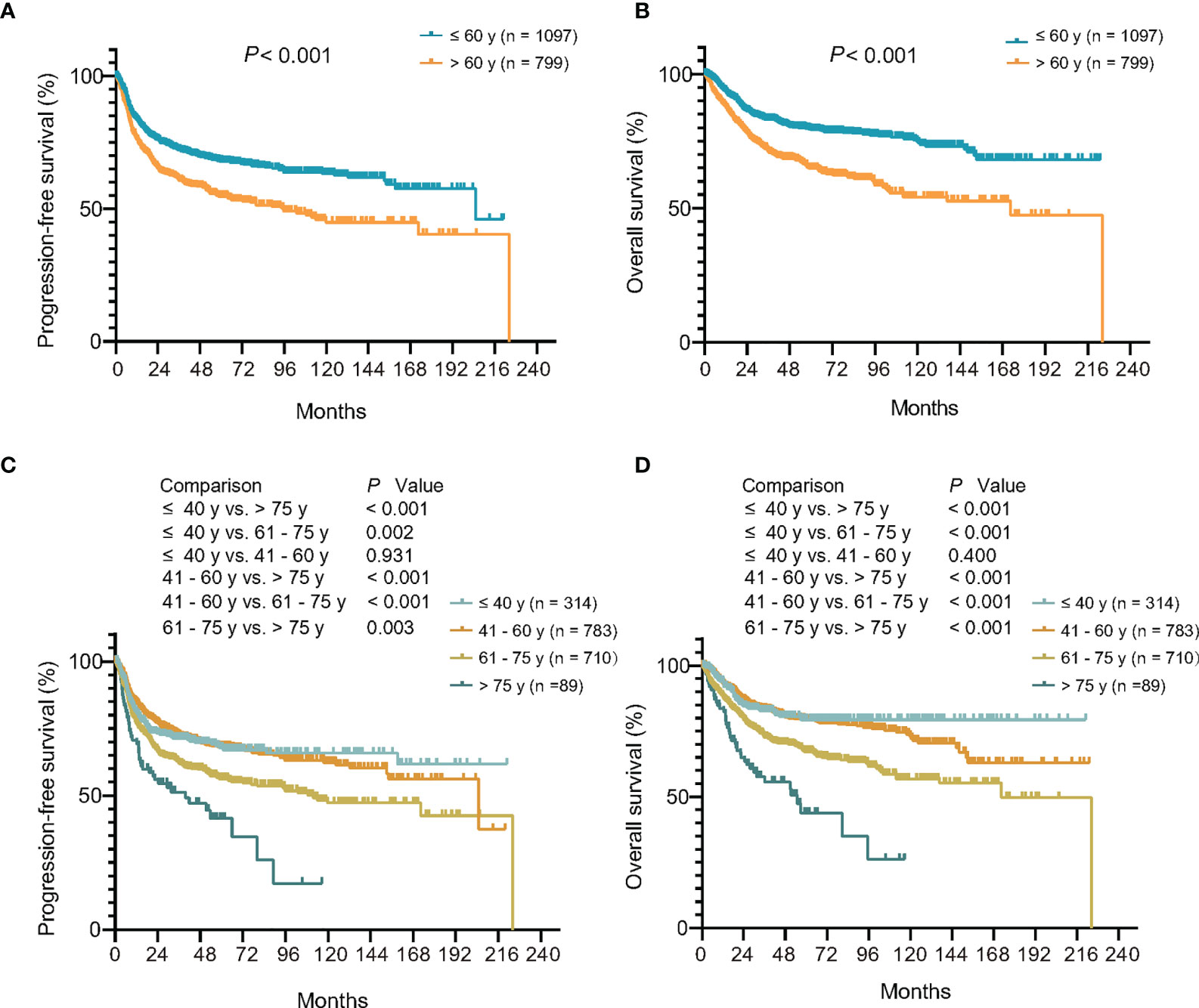
Figure 2 Relationship between age at diagnosis and clinical outcome upon R-CHOP-based immunochemotherapy. (A, B) Kaplan–Meier models of progression-free survival (A) and overall survival (B) according to age at diagnosis of IPI. (C, D) Kaplan–Meier models of progression-free survival (C) and overall survival (D) according to age at diagnosis of NCCN-IPI. R-CHOP, rituximab, cyclophosphamide, doxorubicin, vincristine, and prednisone; IPI, International Prognostic Index; NCCN, National Comprehensive Cancer Network database.
Oncogenic Mutation Alterations Related to Age at Diagnosis
Oncogenic mutations closely related to age at diagnosis were analyzed in 1,150 patients, namely, 117 cases by WGS, 223 cases by WES, and 810 cases by targeted sequencing. A total of 55 genes related to the tumorigenesis of DLBCL were analyzed (Figure 3A). The association of oncogenic mutations and age at diagnosis among the patients were assessed by univariate logistic regression. Eight genes were significantly correlated with age (Figure 3B), namely, PIM1 (OR = 1.015, 95% CI = 1.005–1.026, P = 0.002), MYD88 (OR = 1.022, 95% CI = 1.011–1.033, P <0.001), BTG2 (OR = 1.014, 95% CI = 1.003–1.025, P = 0.015), CD79B (OR = 1.030, 95% CI = 1.016–1.044, P <0.001), TET2 (OR = 1.016, 95% CI = 1.003-1.030, P = 0.014), BTG1 (OR = 1.014, 95% CI = 1.001–1.028, P = 0.037), CREBBP (OR = 1.016, 95% CI = 1.002–1.031, P = 0.025), and TBL1XR1 (OR = 1.019, 95% CI = 1.002–1.036, P = 0.025). Meanwhile, the same results were observed on MYD88L265P mutation alone (OR = 1.024, 95% CI = 1.011–1.037, P <0.001), or with CD79B mutation (OR = 1.038, 95% CI = 1.013–1.064, P = 0.002) (Figure 3C).
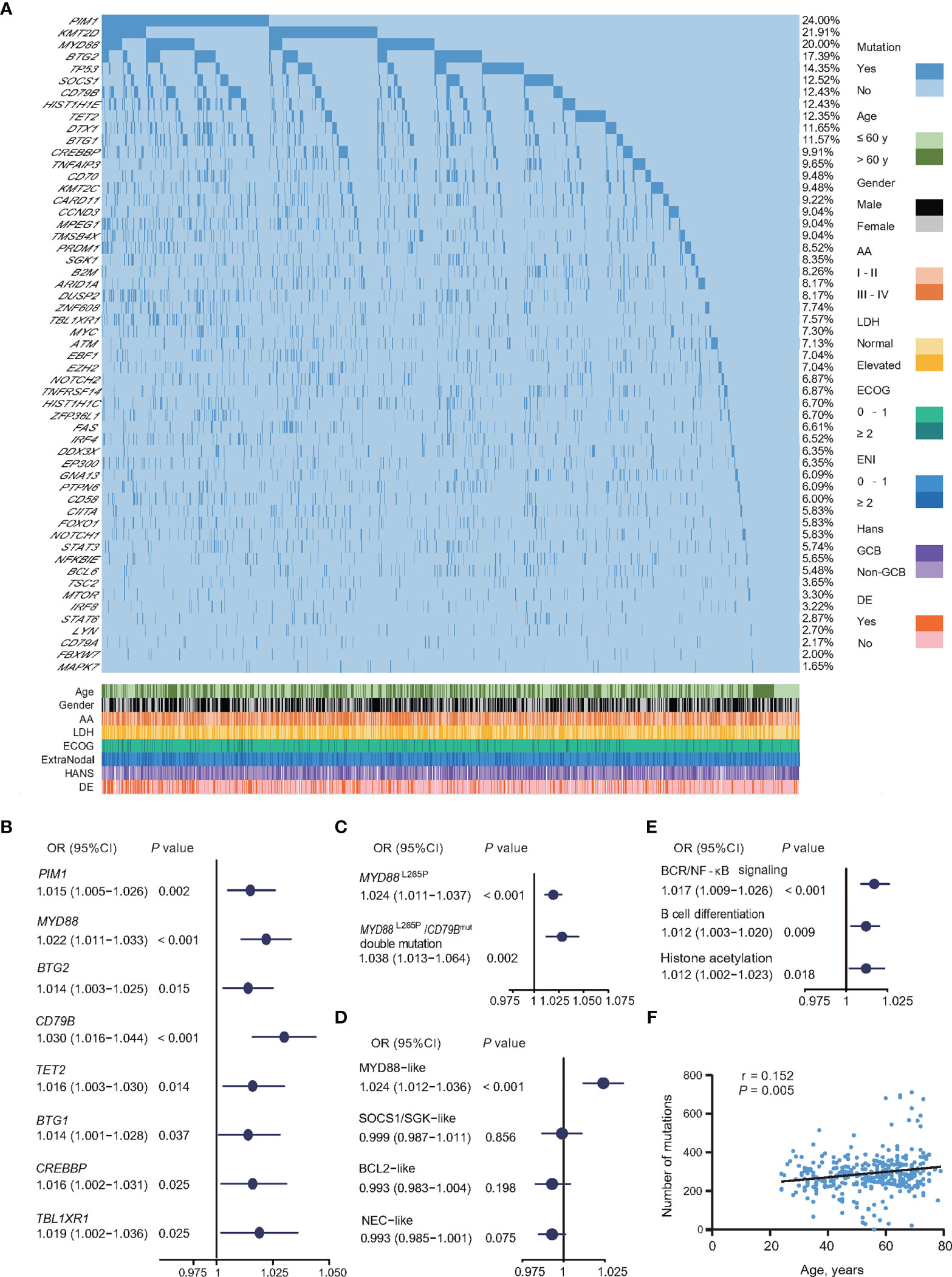
Figure 3 Relationship between oncogenic mutations and age at diagnosis in DLBCL. (A) Oncogenic mutations identified by WGS/WES/targeted sequencing in DLBCL patients. (B) Univariate logistic regression analysis of oncogenic mutations according to age at diagnosis. (C) Univariate logistic regression analysis of MYD88L265P mutations alone or with CD79B mutations according to age at diagnosis. (D) Univariate logistic regression analysis of genetic subtypes according to age at diagnosis. (E) Univariate logistic regression analysis of oncogenic pathways according to age at diagnosis. (F) Correlation between the number of oncogenic mutations sequenced by WGS/WES and age at diagnosis in DLBCL. (B–E) Odd ratios (OR), 95% confidence intervals (95% CI), and P-values are indicated on the left of each forest plot. DLBCL, diffuse large B-cell lymphoma; WGS, whole genome sequencing; WES, whole exome sequencing; AA, Ann Arbor; LDH, lactate dehydrogenase; ECOG, Eastern Cooperative Oncology Group; ENI, extranodal involvement; DE, double expressor; GCB, germinal center B-cell.
All 1,150 patients were genetically classified (21), namely, MYD88-like (191, 16.6%), SOCS1/SGK1-like (148, 12.9%), BCL2-like (222, 19.3%), and NEC-like subtype (589, 51.2%). MYD88-like subtype was significantly associated with age (OR = 1.024, 95% CI = 1.012–1.036, P <0.001) (Figure 3D). Based on biological functions, mutations involving BCR/NF-κB signaling (OR = 1.017, 95% CI = 1.009–1.026, P <0.001), B-cell differentiation (OR = 1.012, 95% CI = 1.003–1.020, P = 0.009), and histone acetylation (OR = 1.012, 95% CI = 1.002–1.023, P = 0.018) were significantly associated with age (Figure 3E). The total mutation load in the coding genome also showed positive correlation with age in 340 patients with WGS or WES data (P = 0.005, r = 0.152) (Figure 3F).
Tumor Microenvironment Alterations Related to Age at Diagnosis
The abundance of immune cells in tumor microenvironment was evaluated using RNA sequencing data. The association between the abundance of immune cells and age was estimated using Spearman’s rank correlation. With abundance of CD4+ naïve T and CD8+ naïve T cells decreased at age (P = 0.006 and P = 0.006), and abundance of exhausted T cells and macrophages increased with age (P = 0.002 and P = 0.046) (Figure 4A).
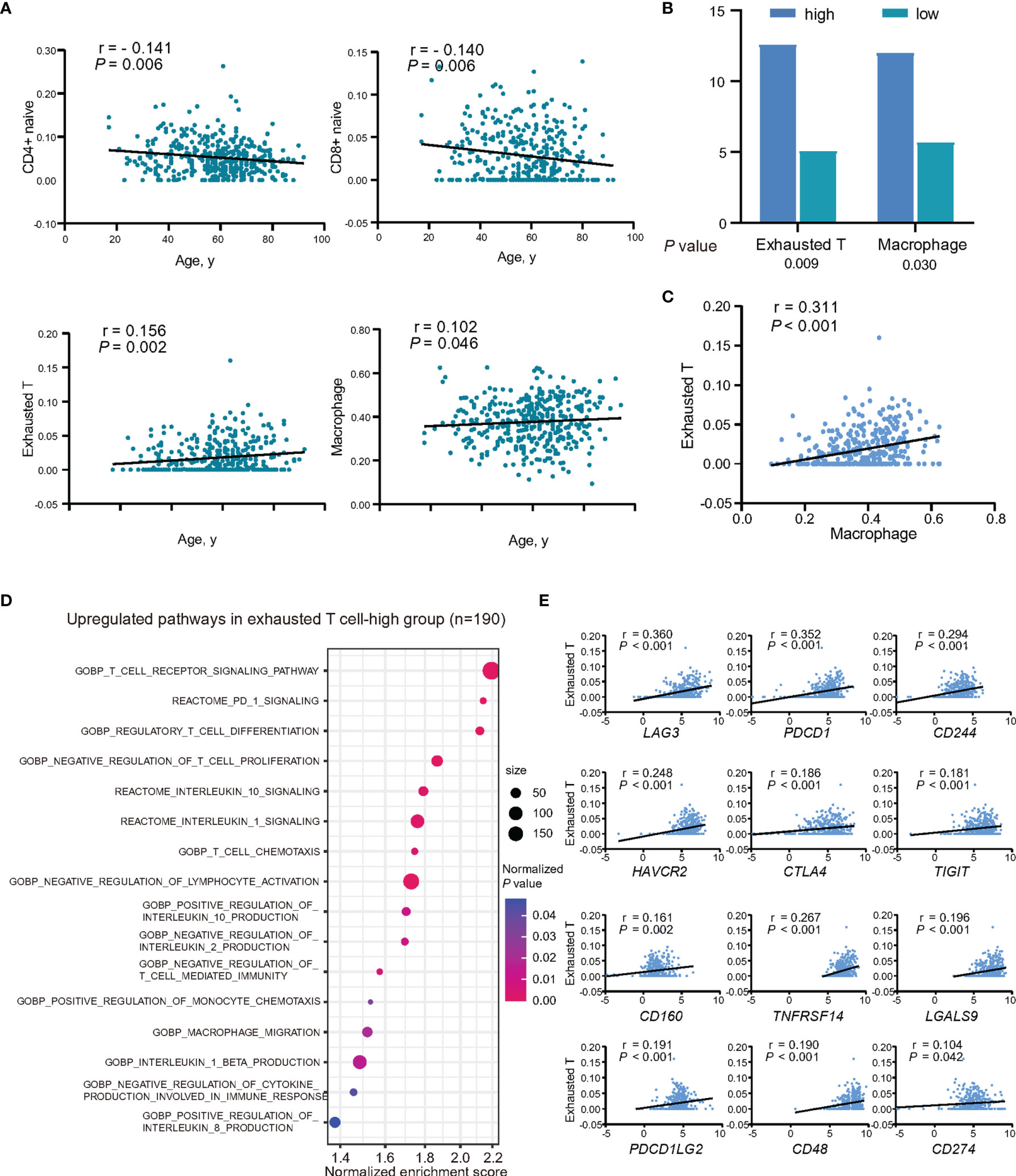
Figure 4 Relationship between intratumor immune cells and age at diagnosis in DLBCL. (A) Abundance of immune cell subtypes in patients showing decreased or increased trend with age at diagnosis. (B) Mutation rates of CD79B between exhausted T-high group and exhausted T-low group, and also between macrophage-high group and macrophage-low group. Lower graph indicates P-values. (C) Correlation between the abundance of exhausted T cells and macrophages. P-value and r-value were indicated in the plot. (D) Pathway enrichment analysis in exhausted T-high group (n = 190), as compared to exhausted T-low group (n = 195, P <0.05). Color of points indicates normalized P-value of upregulated pathways in two groups. Size of points indicates number of genes included in each gene set. (E) Correlations between the expression of inhibitory receptors, ligands, and the abundance of exhausted T cells. P-values and r-values were indicated in each plot.
As exhausted T cells and macrophages play essential roles in immune evasion, their relations with age-associated mutations and effects on tumor progression were further assessed. All the patients were subsequently divided into two groups according to the median of abundance of the two immune cells, respectively. CD79B mutations were found more frequently mutated in the exhausted T-high group and macrophage-high group (P = 0.009 and P = 0.030) (Figure 4B). As revealed by GSEA, BCR signaling pathway was upregulated according to CD79B mutations (Supplementary Figure 1A). IL10, one of the key cytokines associated with T-cell exhaustion and macrophage M2 polarization, was found significantly higher in CD79B mutation patients (P = 0.001) (Supplementary Figure 1B). The abundance of macrophages showed positive linear correlations with the abundance of exhausted T cells (P <0.001) (Figure 4C).
Correspondingly, GSEA analysis revealed negative regulation of T-cell immunity (T-cell differentiation, lymphocyte activation, and T-cell proliferation, and IL-2 production), upregulation of PD-1 signaling, inhibitory cytokines production and signaling, and also the recruitment of the inhibitory immune cells in exhausted T-high group, as compared to exhausted T-low patients (Figure 4D). IL10, but not TGFB1 (TGFβ), showed positive linear correlations with the abundance of exhausted T-cells (P <0.001) (Supplementary Figure 2A). When analyzing the expression levels of inhibitory receptors and their ligands and the abundance of exhausted T cells, inhibitory receptors, namely, LAG3, PDCD1 (PD-1), CD244 (2B4), HAVCR2 (TIM3), CTLA4, TIGIT, CD160, and BTLA, and ligands to inhibitory receptors, namely, TNFRSF14 (HVEM), LGALS9, PDCD1LG2 (PDL2), CD48, and CD274 (PDL1) showed positive linear correlations with the abundance of exhausted T cells (all P <0.05) (Figure 4E and Supplementary Figure 2B). Among them, the expression of CD244 and PDCD1 was increased with age (P = 0.016 and P = 0.031) (Supplementary Figure 2C).
According to the abundance of macrophages, GSEA analysis indicated that upregulation of macrophage recruitment and activation, immunoregulatory interactions of lymphoid and non-lymphoid cells, inflammation, collagen degradation, PD-1 signaling, angiogenesis, aging, and also inhibitory cytokine production and signaling in macrophage-high group, as compared to macrophage-low group (Figure 5A). Markers of macrophage M1 and M2 were further compared, showing that M2 markers, namely, CD163 (log2foldchange = 2.693, P <0.001), MSR1 (log2foldchange = 2.785, P <0.001), and MRC1 (log2foldchange = 1.807, P <0.001) were significantly upregulated in macrophage-high group (Figure 5B). Meanwhile, cytokines IL10, CSF1, IL1B, IL6, and TGFB1, which were involved in M2 polarization, showed positive linear correlations with the abundance of macrophages (all P <0.001) (Supplementary Figure 3A). Since macrophages may contribute to T-cell exhaustion through the expression of ligands to inhibitory receptors, the relationships between the expression levels of inhibitory receptors and their ligands with the abundance of macrophages were analyzed. Among them, ligands to inhibitory receptors, namely, PDCD1LG2, CD274, LGALS9, TNFRSF14, PVR, and CD48, and also inhibitory receptors, namely, HAVCR2, CD244, LAG3, VSIR (VISTA), PDCD1, CTLA4, CD160, and ENTPD1 (CD39), showed positive linear correlations with the abundance of macrophages (all P <0.05) (Figure 5C and Supplementary Figure 3B). When analyzing the expression levels of chemokines and the abundance of macrophage, chemokines related to macrophage recruitment CCL8, CXCL16, CCL2, CCL18, CCL3, CCL4, CCL5, CXCL12, CXCL8, CX3CL1, CCL14, and CCL26 showed positive linear correlations with the abundance of macrophages (all P <0.05) (Figure 5D). Among these chemokines, the expression of CCL3 and CCL5 was increased with age (P = 0.024 and P = 0.045) (Supplementary Figure 3C).
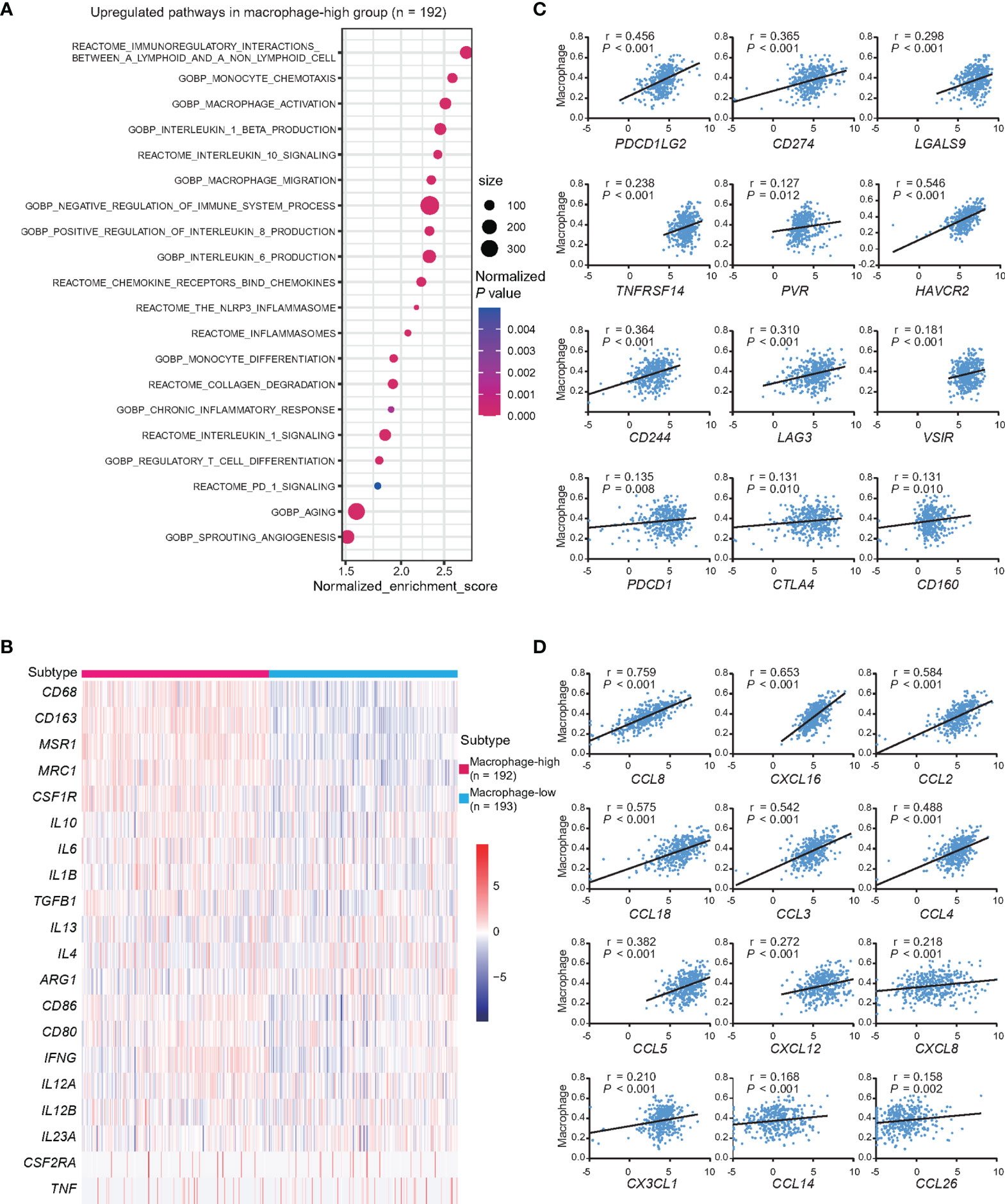
Figure 5 Dysfunctions of macrophages and age at diagnosis in DLBCL. (A) Pathway enrichment analysis in macrophage-high group (n = 192), as compared to macrophages-low group (n = 193, P <0.05). Color of points indicates normalized P-value of upregulated pathways in two groups. Size of points indicates number of genes included in each gene set. (B) Heatmap of genes associated with the markers of macrophage M2 and M1 in macrophage-high group (n = 192), as compared to macrophages-low group (n = 193). (C) Correlations between the expression of inhibitory receptors, ligands, and the abundance of macrophages. P-values and r-values were indicated in each plot. (D) Correlations between the expression of chemokines and the abundance of macrophages. P-values and r-values were indicated in each plot.
Discussion
Age at diagnosis is one of the major risk factors in DLBCL, resulting in poor response to R-CHOP-based immunochemotherapy and adverse clinical outcomes, particularly in patients diagnosed over 75 years. In our cohort, older DLBCL presented advanced disease stage, elevated serum LDH, poor performance status, multiple extranodal involvement, high percentage of double expressor subtype, and poor prognosis, which may be resulted from specific biological features.
Based on the genomic data of a large cohort, we observed overall enhanced mutagenesis in the coding region as the age at diagnosis increased in DLBCL, probably due to spontaneous deamination (22). Among oncogenic mutations, eight genes manifested positive association with age at diagnosis. PIM1, MYD88, and CD79B mutations are frequent mutations related to MYD88 genetic subtype and activated B cell-like DLBCL dependent on BCR signaling and constitutive activation of NF-κB pathway, which were found to be enriched in patients that experienced inferior survival and early progression upon R-CHOP treatment (23–26). BTG2 and BTG1 were considered as anti-proliferation genes and frequently deleted or mutated in hematological malignancies with aggressive cell behavior and treatment resistance (27). TET2 mutations impaired enhancer H3K27 acetylation and disrupted transit of B cells through germinal centers, leading to lymphomagenesis (28). CREBBP mutations resulted in reduced histone H3 acetylation, enhanced cell proliferation and low expression of MHC II, provoking immune evasion and disease progression (29, 30). TBL1XR1 mutations co-opted SMRT/HDAC3 repressor complexes toward binding the memory B-cell transcription factor BACH2 and disrupted the differentiation into plasma cells, leading to a striking extranodal immunoblastic lymphoma phenotype (31). Moreover, based on biological functions, mutations involving BCR/NF-κB signaling, B-cell differentiation, and histone acetylation were positively associated with age at diagnosis, which provides potential therapeutic targets, such as BTK inhibitors and epigenetic regulators (32, 33). Together, distinct oncogenic mutations function as important determinators for disease progression in older DLBCL patients and older DLBCL might be considered as a specific entity associated with genetic subtyping.
Tumor microenvironment is also implicated in DLBCL progression. Decreased CD4+ naïve T and CD8+ naïve T cells were age-related, due to declined production of new T cells from involuted and nonfunctional thymus and results in reduced richness of the TCR repertoire in aging populations (34). Exhausted T cells and macrophages were increased in older patients and related to age-related mutations, particularly CD79B. As mechanism of action, CD79B mutations contributed to activation of BCR signaling and resulted in upregulated expression of IL10, a key immunosuppressive cytokine linked to T-cell exhaustion and macrophage M2 polarization (35, 36). Higher abundance of exhausted T cells presented the suppression of T-cell immunity and upregulation of PD-1 signaling, related to recruitment of immunosuppressive cells and production of immunosuppressive cytokines. The positive correlation between the expression of IL10 and the abundance of exhausted T cells pointed out that IL10 might be involved in T-cell exhaustion in DLBCL (37). Meanwhile, several inhibitory receptors and ligands were positively correlated to the abundance of exhausted T cells, among which CD244 and PDCD1 showed correlation to age (10, 38). Indeed, the inhibitory receptors co-expressed at high levels on T cells and synergistically mediated T-cell exhaustion and dysfunction with the ligands on tumor cells and antigen presenting cells, which implies combinations of agents blocking inhibitory receptors may function in older DLBCL patients to overcome T-cell exhaustion and to restore anti-tumor immunity.
Macrophages constitute a major component of tumor microenvironment and are involved in cancer progression, metastasis and immune evasion, creating an immunosuppressive microenvironment through secretion of anti-inflammatory cytokines, expression of PD-L1, and recruitment of Th2 and Treg cells (39, 40). This was in keeping with the results by pathway analysis that higher abundance of macrophages was associated with the enrichment pathways of negative regulation of immune, chronic inflammatory response, collagen degradation, angiogenesis, and PD-1 signaling in macrophage-high DLBCL. Importantly, higher abundance of macrophages also manifested increased expression of markers of immunosuppressive macrophage M2, namely, CD163, MSR1, and MRC1 (41–43). The positive correlation between the expression of cytokines of M2 polarization and the abundance of macrophages suggested that IL10, CSF1, IL1B, IL6, and TGFB1 could involve in M2 polarization in DLBCL (44–48). As macrophages was a major source of immunosuppressive cytokines and showed positive correlation to inhibitory receptors and ligands, the abundance of macrophages was in positive correlation with exhausted T cells and could contribute to age-related immunosuppression in DLBCL (40, 49). Several chemokines related to macrophage chemotaxis and immunosuppressive function increased with macrophage, and among them, CCL3 and CCL5 are secreted factors of senescence-associated secretory phenotype and increased with age and age-associated macrophage recruitment (50). Macrophage infiltrations were linked to poor prognosis in DLBCL, suggesting inhibition of macrophages may also be alternative immunomodulatory strategy in DLBCL (14).
However, there was a difference in distribution of clinical features between western and Chinese DLBCL populations according to previous reports and our cohort, namely, age at diagnosis (median age: 62–66 years vs. 53–59 years), Ann Arbor stage (III–IV: 54.1–62.8% vs. 35.2–57.4%), and performance status (Eastern Cooperative Oncology Group score ≥2: 16.7–37.3% vs. 13.0–19.9%) (21, 51–60). Future epidemiology studies are necessary to elucidate the different characteristics between western and eastern countries, which may provide better understanding of targeted therapeutic approaches in DLBCL.
In conclusion, older DLBCL could be a specific entity with unfavorable clinical and molecular features, represented by accumulation of oncogenic mutations and immunosuppressive tumor microenvironment alterations. Thus, clinical studies on immunotherapies warrant further investigation on future mechanism-based treatment in older DLBCL.
Data Availability Statement
The datasets including WGS, WES and RNA sequencing data were deposited on The National Omics Data Encyclopedia (https://www.biosino.org/node) in project OEP001143 and OEP001040.
Ethics Statement
The studies involving human participants were reviewed and approved by the Shanghai Rui Jin Hospital Review Board. Written informed consent to participate in this study was provided by the participants.
Author Contributions
WLZ, PPX, QT, LW, and SC designed and supervised the study. YZ collected and analyzed the data, and wrote the manuscript. DF, QS, and ZYS recruited patients, collected study data, and prepared biological samples. LD and HMY reviewed the histopathologic diagnoses. ZHL acquired the biological samples. YF, QL and HF provided technical support for bioinformatic analysis. All authors listed have made a substantial, direct, and intellectual contribution to the work and approved it for publication.
Conflict of Interest
The authors declare that the research was conducted in the absence of any commercial or financial relationships that could be construed as a potential conflict of interest.
Publisher’s Note
All claims expressed in this article are solely those of the authors and do not necessarily represent those of their affiliated organizations, or those of the publisher, the editors and the reviewers. Any product that may be evaluated in this article, or claim that may be made by its manufacturer, is not guaranteed or endorsed by the publisher.
Acknowledgments
We sincerely appreciate the efforts of the doctors for enrolling patients and all the patients enrolled for allowing us to analyze their clinical data. Meanwhile, we thank the fundings supported by the National Natural Science Foundation of China (82130004, 81830007 and 82070204), Shanghai Municipal Education Commission Gaofeng Clinical Medicine (20152206 and 20152208), Multicenter Clinical Research Project by Shanghai Jiao Tong University School of Medicine (DLY201601), Clinical Research Plan of Shanghai Hospital Development Center (SHDC2020CR1032B), Chang Jiang Scholars Program, Samuel Waxman Cancer Research Foundation, and the Foundation of National Facility for Translational Medicine (Shanghai, TMSK-2020-115).
Supplementary Material
The Supplementary Material for this article can be found online at: https://www.frontiersin.org/articles/10.3389/fimmu.2022.842439/full#supplementary-material
References
1. Project INHsLPF. A Predictive Model for Aggressive non-Hodgkin's Lymphoma. N Engl J Med (1993) 329(14):987–94. doi: 10.1056/NEJM199309303291402
2. Zhou Z, Sehn LH, Rademaker AW, Gordon LI, Lacasce AS, Crosby-Thompson A, et al. An Enhanced International Prognostic Index (NCCN-IPI) for Patients With Diffuse Large B-Cell Lymphoma Treated in the Rituximab Era. Blood (2014) 123(6):837–42. doi: 10.1182/blood-2013-09-524108
3. Vijg J, Dong X. Pathogenic Mechanisms of Somatic Mutation and Genome Mosaicism in Aging. Cell (2020) 182(1):12–23. doi: 10.1016/j.cell.2020.06.024
4. Di M, Huntington SF, Olszewski AJ. Challenges and Opportunities in the Management of Diffuse Large B-Cell Lymphoma in Older Patients. Oncologist (2021) 26(2):120–32. doi: 10.1002/onco.13610
5. Kim Y, Ju H, Kim DH, Yoo HY, Kim SJ, Kim WS, et al. CD79B and MYD88 Mutations in Diffuse Large B-Cell Lymphoma. Hum Pathol (2014) 45(3):556–64. doi: 10.1016/j.humpath.2013.10.023
6. Xie M, Lu C, Wang J, McLellan MD, Johnson KJ, Wendl MC, et al. Age-Related Mutations Associated With Clonal Hematopoietic Expansion and Malignancies. Nat Med (2014) 20(12):1472–8. doi: 10.1038/nm.3733
7. Niroula A, Sekar A, Murakami MA, Trinder M, Agrawal M, Wong WJ, et al. Distinction of Lymphoid and Myeloid Clonal Hematopoiesis. Nat Med (2021) 27(11):1921–7. doi: 10.1038/s41591-021-01521-4
8. Lian J, Yue Y, Yu W, Zhang Y. Immunosenescence: A Key Player in Cancer Development. J Hematol Oncol (2020) 13(1):151. doi: 10.1186/s13045-020-00986-z
9. Kasakovski D, Xu L, Li Y. T Cell Senescence and CAR-T Cell Exhaustion in Hematological Malignancies. J Hematol Oncol (2018) 11(1):91. doi: 10.1186/s13045-018-0629-x
10. Wherry EJ, Kurachi M. Molecular and Cellular Insights Into T Cell Exhaustion. Nat Rev Immunol (2015) 15(8):486–99. doi: 10.1038/nri3862
11. Jackaman C, Nelson DJ. Are Macrophages, Myeloid Derived Suppressor Cells and Neutrophils Mediators of Local Suppression in Healthy and Cancerous Tissues in Aging Hosts? Exp Gerontol (2014) 54:53–7. doi: 10.1016/j.exger.2013.11.009
12. Swerdlow SH, Campo E, Pileri SA, Harris NL, Stein H, Siebert R, et al. The 2016 Revision of the World Health Organization Classification of Lymphoid Neoplasms. Blood (2016) 127(20):2375–90. doi: 10.1182/blood-2016-01-643569
13. Shen R, Xu PP, Wang N, Yi HM, Dong L, Fu D, et al. Influence of Oncogenic Mutations and Tumor Microenvironment Alterations on Extranodal Invasion in Diffuse Large B-Cell Lymphoma. Clin Transl Med (2020) 10(7):e221. doi: 10.1002/ctm2.221
14. Huang YH, Cai K, Xu PP, Wang L, Huang CX, Fang Y, et al. CREBBP/EP300 Mutations Promoted Tumor Progression in Diffuse Large B-Cell Lymphoma Through Altering Tumor-Associated Macrophage Polarization via FBXW7-NOTCH-CCL2/CSF1 Axis. Signal Transduct Target Ther (2021) 6(1):10. doi: 10.1038/s41392-020-00437-8
15. Qin W, Fu D, Shi Q, Dong L, Yi H, Huang H, et al. Molecular Heterogeneity in Localized Diffuse Large B-Cell Lymphoma. Front Oncol (2021) 11:638757. doi: 10.3389/fonc.2021.638757
16. Li MM, Datto M, Duncavage EJ, Kulkarni S, Lindeman NI, Roy S, et al. Standards and Guidelines for the Interpretation and Reporting of Sequence Variants in Cancer: A Joint Consensus Recommendation of the Association for Molecular Pathology, American Society of Clinical Oncology, and College of American Pathologists. J Mol Diagn (2017) 19(1):4–23. doi: 10.1016/j.jmoldx.2016.10.002
17. Anders S, Pyl PT, Huber W. HTSeq–a Python Framework to Work With High-Throughput Sequencing Data. Bioinformatics (2015) 31(2):166–9. doi: 10.1093/bioinformatics/btu638
18. Scott DW, Wright GW, Williams PM, Lih CJ, Walsh W, Jaffe ES, et al. Determining Cell-of-Origin Subtypes of Diffuse Large B-Cell Lymphoma Using Gene Expression in Formalin-Fixed Paraffin-Embedded Tissue. Blood (2014) 123(8):1214–7. doi: 10.1182/blood-2013-11-536433
19. Miao YR, Zhang Q, Lei Q, Luo M, Xie GY, Wang H, et al. ImmuCellAI: A Unique Method for Comprehensive T-Cell Subsets Abundance Prediction and its Application in Cancer Immunotherapy. Adv Sci (Weinh) (2020) 7(7):1902880. doi: 10.1002/advs.201902880
20. Hans CP, Weisenburger DD, Greiner TC, Gascoyne RD, Delabie J, Ott G, et al. Confirmation of the Molecular Classification of Diffuse Large B-Cell Lymphoma by Immunohistochemistry Using a Tissue Microarray. Blood (2004) 103(1):275–82. doi: 10.1182/blood-2003-05-1545
21. Lacy SE, Barrans SL, Beer PA, Painter D, Smith AG, Roman E, et al. Targeted Sequencing in DLBCL, Molecular Subtypes, and Outcomes: A Haematological Malignancy Research Network Report. Blood (2020) 135(20):1759–71. doi: 10.1182/blood.2019003535
22. Chapuy B, Stewart C, Dunford AJ, Kim J, Kamburov A, Redd RA, et al. Molecular Subtypes of Diffuse Large B Cell Lymphoma are Associated With Distinct Pathogenic Mechanisms and Outcomes. Nat Med (2018) 24(5):679–90. doi: 10.1038/s41591-018-0016-8
23. Hartert KT, Wenzl K, Krull JE, Manske M, Sarangi V, Asmann Y, et al. Targeting of Inflammatory Pathways With R2CHOP in High-Risk DLBCL. Leukemia (2021) 35(2):522–33. doi: 10.1038/s41375-020-0766-4
24. Wilson WH, Young RM, Schmitz R, Yang Y, Pittaluga S, Wright G, et al. Targeting B Cell Receptor Signaling With Ibrutinib in Diffuse Large B Cell Lymphoma. Nat Med (2015) 21(8):922–6. doi: 10.1038/nm.3884
25. Ma J, Yan Z, Zhang J, Zhou W, Yao Z, Wang H, et al. A Genetic Predictive Model for Precision Treatment of Diffuse Large B-Cell Lymphoma With Early Progression. Biomark Res (2020) 8:33. doi: 10.1186/s40364-020-00214-3
26. Pedrosa L, Fernandez-Miranda I, Perez-Callejo D, Quero C, Rodriguez M, Martin-Acosta P, et al. Proposal and Validation of a Method to Classify Genetic Subtypes of Diffuse Large B Cell Lymphoma. Sci Rep (2021) 11(1):1886. doi: 10.1038/s41598-020-80376-0
27. Yuniati L, Scheijen B, van der Meer LT, van Leeuwen FN. Tumor Suppressors BTG1 and BTG2: Beyond Growth Control. J Cell Physiol (2019) 234(5):5379–89. doi: 10.1002/jcp.27407
28. Dominguez PM, Ghamlouch H, Rosikiewicz W, Kumar P, Beguelin W, Fontan L, et al. TET2 Deficiency Causes Germinal Center Hyperplasia, Impairs Plasma Cell Differentiation, and Promotes B-Cell Lymphomagenesis. Cancer Discov (2018) 8(12):1632–53. doi: 10.1158/2159-8290.CD-18-0657
29. Hashwah H, Schmid CA, Kasser S, Bertram K, Stelling A, Manz MG, et al. Inactivation of CREBBP Expands the Germinal Center B Cell Compartment, Down-Regulates MHCII Expression and Promotes DLBCL Growth. Proc Natl Acad Sci USA (2017) 114(36):9701–6. doi: 10.1073/pnas.1619555114
30. Mondello P, Tadros S, Teater M, Fontan L, Chang AY, Jain N, et al. Selective Inhibition of HDAC3 Targets Synthetic Vulnerabilities and Activates Immune Surveillance in Lymphoma. Cancer Discov (2020) 10(3):440–59. doi: 10.1158/2159-8290.CD-19-0116
31. Venturutti L, Teater M, Zhai A, Chadburn A, Babiker L, Kim D, et al. TBL1XR1 Mutations Drive Extranodal Lymphoma by Inducing a Pro-Tumorigenic Memory Fate. Cell (2020) 182(2):297–316.e27. doi: 10.1016/j.cell.2020.05.049
32. Dunleavy K, Erdmann T, Lenz G. Targeting the B-Cell Receptor Pathway in Diffuse Large B-Cell Lymphoma. Cancer Treat Rev (2018) 65:41–6. doi: 10.1016/j.ctrv.2018.01.002
33. Hogg SJ, Beavis PA, Dawson MA, Johnstone RW. Targeting the Epigenetic Regulation of Antitumour Immunity. Nat Rev Drug Discov (2020) 19(11):776–800. doi: 10.1038/s41573-020-0077-5
34. Cunha LL, Perazzio SF, Azzi J, Cravedi P, Riella LV. Remodeling of the Immune Response With Aging: Immunosenescence and Its Potential Impact on COVID-19 Immune Response. Front Immunol (2020) 11:1748. doi: 10.3389/fimmu.2020.01748
35. Li L, Zhang J, Chen J, Xu-Monette ZY, Miao Y, Xiao M, et al. B-Cell Receptor-Mediated NFATc1 Activation Induces IL-10/STAT3/PD-L1 Signaling in Diffuse Large B-Cell Lymphoma. Blood (2018) 132(17):1805–17. doi: 10.1182/blood-2018-03-841015
36. Davis RE, Ngo VN, Lenz G, Tolar P, Young RM, Romesser PB, et al. Chronic Active B-Cell-Receptor Signalling in Diffuse Large B-Cell Lymphoma. Nature (2010) 463(7277):88–92. doi: 10.1038/nature08638
37. Brooks DG, Trifilo MJ, Edelmann KH, Teyton L, McGavern DB, Oldstone MB. Interleukin-10 Determines Viral Clearance or Persistence In Vivo. Nat Med (2006) 12(11):1301–9. doi: 10.1038/nm1492
38. Blackburn SD, Shin H, Haining WN, Zou T, Workman CJ, Polley A, et al. Coregulation of CD8+ T Cell Exhaustion by Multiple Inhibitory Receptors During Chronic Viral Infection. Nat Immunol (2009) 10(1):29–37. doi: 10.1038/ni.1679
39. DeNardo DG, Ruffell B. Macrophages as Regulators of Tumour Immunity and Immunotherapy. Nat Rev Immunol (2019) 19(6):369–82. doi: 10.1038/s41577-019-0127-6
40. Jackaman C, Tomay F, Duong L, Abdol Razak NB, Pixley FJ, Metharom P, et al. Aging and Cancer: The Role of Macrophages and Neutrophils. Ageing Res Rev (2017) 36:105–16. doi: 10.1016/j.arr.2017.03.008
41. Gundra UM, Girgis NM, Ruckerl D, Jenkins S, Ward LN, Kurtz ZD, et al. Alternatively Activated Macrophages Derived From Monocytes and Tissue Macrophages are Phenotypically and Functionally Distinct. Blood (2014) 123(20):e110-22. doi: 10.1182/blood-2013-08-520619
42. Mantovani A, Sozzani S, Locati M, Allavena P, Sica A. Macrophage Polarization: Tumor-Associated Macrophages as a Paradigm for Polarized M2 Mononuclear Phagocytes. Trends Immunol (2002) 23(11):549–55. doi: 10.1016/S1471-4906(02)02302-5
43. Song Q, Hawkins GA, Wudel L, Chou PC, Forbes E, Pullikuth AK, et al. Dissecting Intratumoral Myeloid Cell Plasticity by Single Cell RNA-Seq. Cancer Med (2019) 8(6):3072–85. doi: 10.1002/cam4.2113
44. Jung M, Ma Y, Iyer RP, DeLeon-Pennell KY, Yabluchanskiy A, Garrett MR, et al. IL-10 Improves Cardiac Remodeling After Myocardial Infarction by Stimulating M2 Macrophage Polarization and Fibroblast Activation. Basic Res Cardiol (2017) 112(3):33. doi: 10.1007/s00395-017-0622-5
45. Pyonteck SM, Akkari L, Schuhmacher AJ, Bowman RL, Sevenich L, Quail DF, et al. CSF-1R Inhibition Alters Macrophage Polarization and Blocks Glioma Progression. Nat Med (2013) 19(10):1264–72. doi: 10.1038/nm.3337
46. Das S, Shapiro B, Vucic EA, Vogt S, Bar-Sagi D. Tumor Cell-Derived IL1beta Promotes Desmoplasia and Immune Suppression in Pancreatic Cancer. Cancer Res (2020) 80(5):1088–101. doi: 10.1158/0008-5472.CAN-19-2080
47. Weng YS, Tseng HY, Chen YA, Shen PC, Al Haq AT, Chen LM, et al. MCT-1/miR-34a/IL-6/IL-6R Signaling Axis Promotes EMT Progression, Cancer Stemness and M2 Macrophage Polarization in Triple-Negative Breast Cancer. Mol Cancer (2019) 18(1):42. doi: 10.1186/s12943-019-0988-0
48. Liu F, Qiu H, Xue M, Zhang S, Zhang X, Xu J, et al. MSC-Secreted TGF-Beta Regulates Lipopolysaccharide-Stimulated Macrophage M2-Like Polarization via the Akt/FoxO1 Pathway. Stem Cell Res Ther (2019) 10(1):345. doi: 10.1186/s13287-019-1447-y
49. Farhood B, Najafi M, Mortezaee K. CD8(+) Cytotoxic T Lymphocytes in Cancer Immunotherapy: A Review. J Cell Physiol (2019) 234(6):8509–21. doi: 10.1002/jcp.27782
50. Schafer MJ, Zhang X, Kumar A, Atkinson EJ, Zhu Y, Jachim S, et al. The Senescence-Associated Secretome as an Indicator of Age and Medical Risk. JCI Insight (2020) 5(12):e133668. doi: 10.1172/jci.insight.133668
51. El-Galaly TC, Villa D, Alzahrani M, Hansen JW, Sehn LH, Wilson D, et al. Outcome Prediction by Extranodal Involvement, IPI, R-IPI, and NCCN-IPI in the PET/CT and Rituximab Era: A Danish-Canadian Study of 443 Patients With Diffuse-Large B-Cell Lymphoma. Am J Hematol (2015) 90(11):1041–6. doi: 10.1002/ajh.24169
52. Ennishi D, Mottok A, Ben-Neriah S, Shulha HP, Farinha P, Chan FC, et al. Genetic Profiling of MYC and BCL2 in Diffuse Large B-Cell Lymphoma Determines Cell-of-Origin-Specific Clinical Impact. Blood (2017) 129(20):2760–70. doi: 10.1182/blood-2016-11-747022
53. Keane C, Gill D, Vari F, Cross D, Griffiths L, Gandhi M. CD4(+) Tumor Infiltrating Lymphocytes are Prognostic and Independent of R-IPI in Patients With DLBCL Receiving R-CHOP Chemo-Immunotherapy. Am J Hematol (2013) 88(4):273–6. doi: 10.1002/ajh.23398
54. Montalban C, Diaz-Lopez A, Dlouhy I, Rovira J, Lopez-Guillermo A, Alonso S, et al. Validation of the NCCN-IPI for Diffuse Large B-Cell Lymphoma (DLBCL): The Addition of Beta2 -Microglobulin Yields a More Accurate GELTAMO-IPI. Br J Haematol (2017) 176(6):918–28. doi: 10.1111/bjh.14489
55. Scott DW, Mottok A, Ennishi D, Wright GW, Farinha P, Ben-Neriah S, et al. Prognostic Significance of Diffuse Large B-Cell Lymphoma Cell of Origin Determined by Digital Gene Expression in Formalin-Fixed Paraffin-Embedded Tissue Biopsies. J Clin Oncol (2015) 33(26):2848–56. doi: 10.1200/JCO.2014.60.2383
56. Han Y, Yang J, Liu P, He X, Zhang C, Zhou S, et al. Prognostic Nomogram for Overall Survival in Patients With Diffuse Large B-Cell Lymphoma. Oncologist (2019) 24(11):e1251–e61. doi: 10.1634/theoncologist.2018-0361
57. Li M, Liu CL, Yin WJ, He YX, Xue XM, Duan ZJ, et al. The Clinical Significance of a New Classification Algorithm in Chinese DLBCL Cases. Zhonghua Xue Ye Xue Za Zhi (2012) 33(10):801–4. doi: 10.3760/cma.j.issn.0253-2727.2012.10.003
58. Liu Y, Wang X, Ding N, Mi L, Ping L, Jin X, et al. TP53 Arg72 as a Favorable Prognostic Factor for Chinese Diffuse Large B-Cell Lymphoma Patients Treated With CHOP. BMC Cancer (2017) 17(1):743. doi: 10.1186/s12885-017-3760-0
59. Ren W, Ye X, Su H, Li W, Liu D, Pirmoradian M, et al. Genetic Landscape of Hepatitis B Virus-Associated Diffuse Large B-Cell Lymphoma. Blood (2018) 131(24):2670–81. doi: 10.1182/blood-2017-11-817601
60. Shi Y, Han Y, Yang J, Liu P, He X, Zhang C, et al. Clinical Features and Outcomes of Diffuse Large B-Cell Lymphoma Based on Nodal or Extranodal Primary Sites of Origin: Analysis of 1,085 WHO Classified Cases in a Single Institution in China. Chin J Cancer Res (2019) 31(1):152–61. doi: 10.21147/j.issn.1000-9604.2019.01.10
Keywords: diffuse large B-cell lymphoma, aging, oncogenic mutations, tumor microenvironment, B-cell receptor, histone acetylation
Citation: Zhu Y, Fu D, Shi Q, Shi Z, Dong L, Yi H, Liu Z, Feng Y, Liu Q, Fang H, Cheng S, Wang L, Tian Q, Xu P and Zhao W (2022) Oncogenic Mutations and Tumor Microenvironment Alterations of Older Patients With Diffuse Large B-Cell Lymphoma. Front. Immunol. 13:842439. doi: 10.3389/fimmu.2022.842439
Received: 23 December 2021; Accepted: 24 February 2022;
Published: 25 March 2022.
Edited by:
Rachel Maurie Gerstein, University of Massachusetts Medical School, United StatesReviewed by:
Reuben Matthew Tooze, University of Leeds, United KingdomMichael Green, University of Texas MD Anderson Cancer Center, United States
Copyright © 2022 Zhu, Fu, Shi, Shi, Dong, Yi, Liu, Feng, Liu, Fang, Cheng, Wang, Tian, Xu and Zhao. This is an open-access article distributed under the terms of the Creative Commons Attribution License (CC BY). The use, distribution or reproduction in other forums is permitted, provided the original author(s) and the copyright owner(s) are credited and that the original publication in this journal is cited, in accordance with accepted academic practice. No use, distribution or reproduction is permitted which does not comply with these terms.
*Correspondence: Weili Zhao, zhao.weili@yahoo.com; Pengpeng Xu, pengpeng_xu@126.com
†These authors have contributed equally to this work