- 1Laboratory for Industrial and Applied Mathematics (LIAM), Department of Mathematics, York University, Toronto, ON, Canada
- 2Department of Health Sciences (DISSAL), Postgraduate School of Public Health, University of Genoa, Genoa, Italy
- 3Leeds Institute of Rheumatic and Musculoskeletal Medicine, University of Leeds, Leeds, United Kingdom
- 4Department of Medicine B, Rheumatology Unit and Zabludowicz Center for Autoimmune Diseases, Sheba Medical Center, Ramat-Gan, Israel
- 5Sackler Faculty of Medicine, Tel-Aviv University, Tel-Aviv, Israel
- 6Clinical Dermatology, Istituto di Ricovero e Cura a Carattere Scientifico (IRCCS) Galeazzi Orthopaedic Institute, Milan, Italy
- 7National Institute for Health Research Leeds Biomedical Research Centre, Leeds Teaching Hospitals, Leeds, United Kingdom
Background: Rheumatological and dermatological disorders contribute to a significant portion of the global burden of disease. Big Data are increasingly having a more and more relevant role, being highly ubiquitous and pervasive in contemporary society and paving the way for new, unprecedented perspectives in biomedicine, including dermatology and rheumatology. Rheumatology and dermatology can potentially benefit from Big Data.
Methods: A systematic review of the literature was conducted according to the “Preferred Reporting Items for Systematic Reviews and Meta-Analyses” (PRISMA) guidelines, mining “Uno per tutti”, a highly integrated and automated tool/meta-database developed at the University of Genoa, Genoa, Italy, and consisting of 20 major scholarly electronic databases, including PubMed/MEDLINE. Big Data- or artificial intelligence-based studies were judged based on the modified Qiao’s critical appraisal tool for critical methodological quality assessment of Big Data/machine learning-based studies. Other studies designed as cross-sectional, longitudinal, or randomized investigations, reviews/overviews or expert opinions/commentaries were evaluated by means of the relevant “Joanna Briggs Institute” (JBI)’s critical appraisal tool for the critical methodological quality assessment.
Results: Fourteen papers were included in the present systematic review of the literature. Most of the studies included concerned molecular applications of Big Data, especially in the fields of genomics and post-genomics. Other studies concerned epidemiological applications, with a practical dearth of studies assessing smart and digital applications for psoriatic arthritis patients.
Conclusions: Big Data can be a real paradigm shift that revolutionizes rheumatological and dermatological practice and clinical research, helping to early intercept psoriatic arthritis patients. However, there are some methodological issues that should be properly addressed (like recording and association biases) and some ethical issues that should be considered (such as privacy). Therefore, further research in the field is warranted.
Systematic Review Registration: Registration code 10.17605/OSF.IO/4KCU2.
Introduction
The global burden of disease (GBD) is the quantitative assessment of the health loss due to a given disorder, risk factor, or injury, over a span of time, worldwide. It is comprehensively, geo-spatially and temporally modeled and computed as the epidemiological, clinical, and societal burden imposed by a given disease, taking into account its economic-financial and humanistic effects, if inadequately managed and treated. Such a quantitative and broad approach enables practitioners and researchers as well as all relevant stakeholders, including public and global health decision- and policymakers, to consistently compare the burden of different diseases, risk factors, or injuries, over time and across countries.
Furthermore, these data can guide health policies, guiding them in a pure data-driven and evidence-based way, enabling them to prioritize and allocate resources, especially in developing countries and in other resource-limited settings (1). This framework allows to track the impact of a given health policy or medical intervention (pharmaceutical or non-pharmaceutical/surgical) and to verify if satisfactory progress has been attained towards the achievement of the Sustainable Development Goals (SDGs) set up by the United Nations (UN) General Assembly (2). In particular, SDG 3.4.1 has proposed the ambitious goal of achieving a 30% reduction in premature mortality due to non-communicable diseases by 2030 (2).
In order to monitor the achievement of such a target, the GBD initiative as well as other similar taskforces and groups, like the Global Health Estimates (GHE) initiative led by the World Health Organization (WHO), have designed and validated an array of reliable health-related metrics. These indicators include the number of years of life lost (YLLs), the number of years lived with disability (YLDs), and the number of disability-adjusted life years (DALYs), which enable scholars to compute life lost due to death (because of casualty or premature death) or disability, respectively, which do not allow to live life at maximum (100%) health (1).
GBD- and GHE-related measures are of crucial importance in providing stakeholders involved in the field of global and public health with data, especially in those settings and territories where there is a lack of data, or data are not updated and/or of good quality, in that data collection and analysis would be too much time- and resource-consuming (1).
Psoriasis is a chronic inflammatory skin disease, the complex, multi-factorial etiopathogenesis of which has yet to be elucidated in detail, and which significantly contributes to the GBD (3).
According to a recent study conducted according to the GBD 2019 methodology (4), there were more than 4.6 million incident cases of psoriasis worldwide in 2019, with an age-standardized incidence rate of 57.8 per 100,000 people. Compared to 1990, this corresponded to a reduction of 20.0%. By sex, the age-standardized incidence rate was comparable between men and women. Compared to 1990, this corresponded to a reduction by 19.5% and by 20.4%, respectively. The age-standardized incidence rate per 100,000 persons widely varied across geographic settings, with high-income countries and territories reporting the highest rate of psoriasis, followed by high-middle income countries. Similar patterns could be found for the other GBD-related metrics, including prevalence and YLDs.
Up to approximately 30% of psoriatic patients develop psoriatic arthritis, which can result in irreversible joint damage. According to a recently published systematic review of the literature and meta-analysis (5), the degree of psoriasis severity and nail pitting were found to be predictors of early-onset psoriatic arthritis insurgence and development, as well as higher body mass index and a family history of psoriatic arthritis. Psoriatic patients with arthralgia (overall relative risk or RR 2.15 [95% confidence interval or CI ranging from 1.16 to 3.99]) and/or with imaging-musculoskeletal inflammation (pooled RR 3.72 [95%CI 2.12 to 6.51]) were deemed at higher risk for developing psoriatic arthritis.
Early detection and interception of psoriatic arthritis in psoriatic patients would be of paramount importance for ensuring timely, effective treatment and management but is rather challenging for dermatologists to implement in their daily clinical practice (5).
The way healthcare provisions are delivered has profoundly changed in the last years, with the emergence of novel and innovative models and paths of managing and treating disorders. A new, holistic, and comprehensive biomedical framework known as “P4 medicine” (where the 4 Ps stay for preventative, predictive, personalized, and participatory) has been introduced by Doctor Leroy Hood, a pioneering and inspiring figure in the arena of systems biology and systems medicine, indicating the paradigm change from a “one-size-fits-all” approach to one in which the individual needs of each patient are taken into account (6–9).
Moreover, thanks to its latest scientific achievements and technological improvements, medicine, including dermatology and rheumatology (9), is entering a new, unprecedented era, characterized by the production and release of an incredible wealth of data, known as Big Data. They are characterized by several key dimensions and features, which include velocity (Big Data or “Fast Data” can be generated, mined, retrieved, processed and analyzed in real-time and can be deployed for nowcasting/forecasting predictions), volume (related to the massive amount of data, the magnitude of which poses serious challenges to the classical ways of storing and processing data, requiring cutting-edge and powerful analytical capacities and infrastructures), variety (referring to the incredible diversity of data sources, ranging from administrative to patient-reported, healthcare-generated data, etc.), veracity (trustworthiness, credibility, reliability, and accuracy of data), and value (related to the transformation from raw “useless” data to smart, applicable, and actionable data) (10).
Different tools, channels, and sources can produce Big Data: from large-scale, nationwide surveys, databases, repositories, and registries (the so-called epidemiological/clinical Big Data) to wet-lab, next-generation sequencing and high-throughput technologies (molecular Big Data) and computational approaches (infodemiological or digital Big Data) (10).
Big Data, smart and digital technologies, and Artificial intelligence are dramatically transforming research and clinical practices into disruptive ones, being informed, and guided by purely data-driven approaches (10–12).
As outlined in a commentary by Scarpa (13), psoriatic arthritis presents a certain degree of clinical complexity, overlapping with other rheumatological diseases, on the one hand, but, on the other hand, having its own features (etiopathological, biological, clinical, epidemiological, diagnostic, prognostic, and therapeutic). To reflect such complexity, the expressions “psoriatic disease” (13) and “psoriatic syndrome” (14) have been introduced in the scholarly literature. A contemporary, rather sophisticated conceptual framework distinguishes between phenotypes (“cluster of visible features and properties”), genotypes, endotypes (“various disease expressions in patient groups depending on different disease mechanisms”), regiotypes (“regional differences among endotypes due to environmental exposures”), inflatypes (“different inflammatory mechanisms among endotypes”), and theratypes (“targeted therapeutics depending on the specific disease endotype”) (15). All such complexity and dynamic variability require a multidisciplinary approach for effective treatment and management of psoriatic disease, establishing clinical networks and models of close dermatology-rheumatology collaborations (16).
In the present review paper, we will show how dermatology and rheumatology can benefit from the use of the so-called “Big Data”, smart and digital technologies, and artificial intelligence, especially in the efforts of early intercepting and mitigating against the burden of psoriatic arthritis. More specifically, the present paper will provide a brief overview on the potential uses of Big Data in the field of “precision rheumatology” and “precision dermatology” (11, 12), focusing on psoriatic arthritis, broken down according to tool/source/channel, showing practical examples and applications of Big Data, as well as their major shortcomings and limitations. Big Data, smart and digital technologies, and artificial intelligence can be leveraged to dissect the clinical complexity underlying the conceptual framework of “psoriatic disease”, paving the way for personalized treatment of psoriatic arthritis (17, 18) (Figure 1).
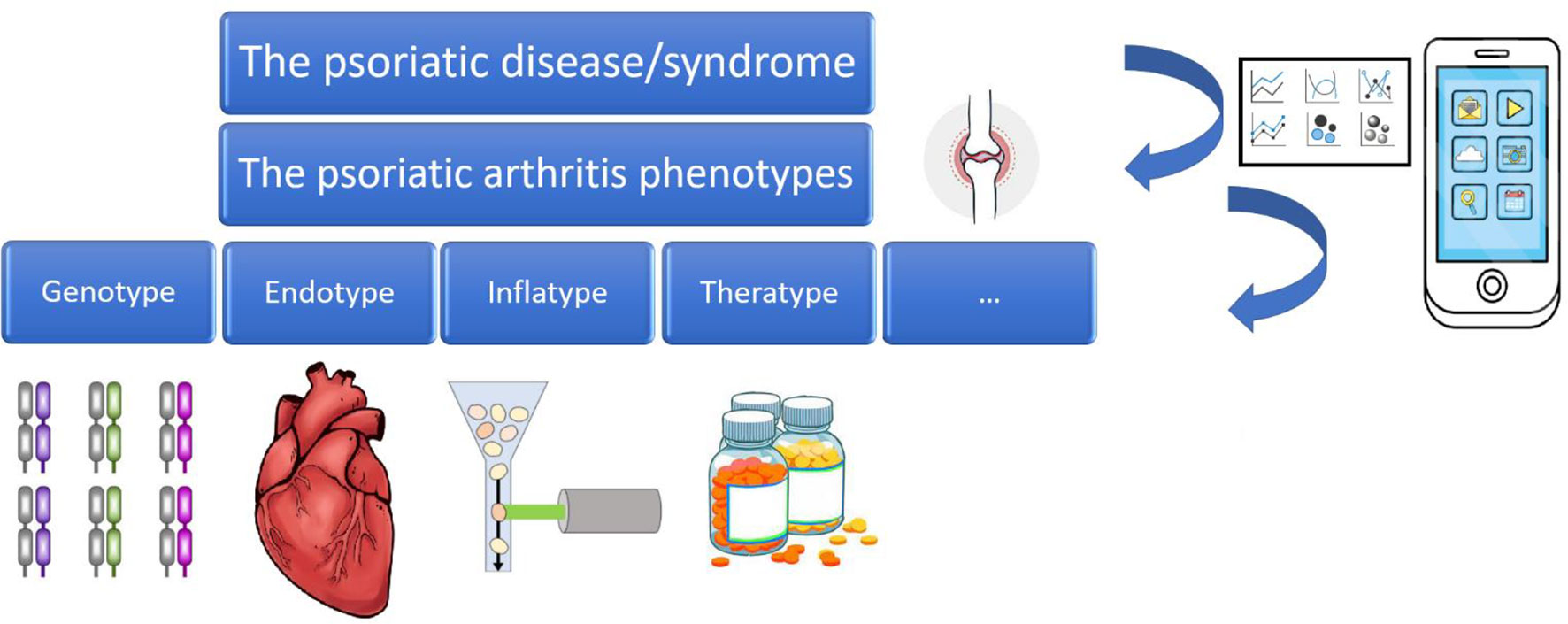
Figure 1 The psoriatic disease/syndrome and its various phenotypes and endotypes, leveraged by Big Data, smart and digital technologies, and Artificial Intelligence.
Material and Methods
Study Protocol and Reporting of the Findings
The findings of the present systematic review are reported according to the “Preferred Reporting Items for a Systematic Review and Meta-Analysis” (PRISMA) 2020 guidelines (19). The study protocol was registered within the “Open Science Framework” (OSF, registration code 10.17605/OSF.IO/4KCU2).
Literature Search
A string of ad hoc keywords was utilized, including terms such as “big data”, “artificial intelligence”, “machine learning”, “deep learning”, “random forests”, “support vector machine”, “artificial neural networks”, “natural language processing”, “k-nearest neighbors”, “Bayesian model”, “digital health”, mHealth, eHealth, uHealth, “psoriatic arthritis”, prevention, and “early interception”. This search string was designed based on a comprehensive systematic literature review conducted on the current status of the use of big data and artificial intelligence in rheumatic and musculoskeletal diseases (RMDs) to inform the “European Alliance of Associations for Rheumatology” (EULAR) recommendations (20). Moreover, extensive cross-referencing was applied in order to maximize the chance of getting all potentially eligible and relevant studies.
Databases Mining
A highly automated and integrated discovery tool/meta-database consisting of 20 scholarly electronic databases, including PubMed/MEDLINE, named as “Uno per tutti”, developed at the University of Genoa, Genoa, Italy, was mined from inception, without time or language filters and restrictions. Wild-card option and “medical subject headings” (MeSH) terms were used when necessary. An experienced librarian and research methodologist from the University of Genoa, Genoa, Italy, were involved in the design and implementation of the search strategy.
Inclusion and Exclusion Criteria
Inclusion and exclusion criteria were devised and formulated according to the PICOS components.
Inclusion criteria were the following: namely, i) studies written in any language, ii) original investigations applying sophisticated statistical or artificial intelligence-based techniques or relying on big data (C, comparators), and focusing on psoriatic arthritis (P, patients), or reviews on the topic under study (S, study design). Any kind of intervention (diagnostic test, pharmacological treatment, telehealthcare provision, etc.) was considered for eligibility. The feasibility and the potential effectiveness of exploiting Big Data, Artificial intelligence, and smart technologies were the major outcomes (O) of the present study. Articles that did not meet with these inclusion criteria were excluded.
Inclusion and exclusion criteria are further detailed in Table 1.
Data Abstraction
Two independent researchers (N.L.B. and C.B.) abstracted relevant data from included studies. These data included: the surname of the first author of the study, study design, study country, study period, sample size, and main findings. Any potential disagreement between the two authors was solved involving a third author (D.M-G), who acted as a final referee.
Data Synthesis
Data abstracted were collected and provided in a tabular fashion. A qualitative thematic synthesis was offered, based on the major topics addressed and covered by the retained studies.
Methodological Quality Appraisal
Studies retained in the present systematic literature review were critically appraised in terms of methodological quality according to relevant checklists and guidelines. Big Data- and artificial intelligence/machine learning-based studies were judged based on a modified version of the recently proposed Qiao’s checklist (21) (Supplementary Table 2). This checklist consists of nine categories/domains: namely, i) unmet needs, ii) reproducibility, iii) robustness, iv) stability of results, v) generalizability, vi) clinical significance, and vii) suggested clinical use. The first category/domain comprises of a single item (“limits in current non-Big Data/machine learning approaches”), including low diagnostic/predictive accuracy or particularly time- and resource-consuming diagnostic procedure. The second category/domain comprises of three items: namely, i) feature engineering methods/parameters choice (feature generated before model training/identification and choice of predictors/covariates), ii) platforms and/or packages utilized, including details of databases and the mining step performed, and iii) hyperparameters/meta-data needed for study replication and confirmation. The third category/domain comprises of a single item: validated, reliable methods (such as leave-one-out, k-fold cross-validation or bootstrapping) used to overcome the issue of over-fitting. The fourth category/domain consists of a single item: computed/estimated variation during the validation step. The fifth category/domain comprises of a single item: external data validation, that is to say, validation in settings/datasets/databases different from the original setting(s)/dataset(s)/database(s) of the research framework. The last category/domain consists of two items: namely, i) explanation of the importance of each predictor/covariate, and ii) suggested potential applications and uses during routine daily clinical practice. Studies that reported at least 4 “yes” out of 9 “yes” for the modified Qiao’s checklist were considered acceptable.
Other studies designed as cross-sectional, longitudinal, or randomized investigations, reviews/overviews or expert opinions/commentaries were evaluated by means of the relevant “Joanna Briggs Institute” (JBI)’s critical appraisal tool for the critical methodological quality assessment.
Two authors (N.L.B. and C.B.) were independently involved in this process. Any disagreement between the two authors was resolved through the involvement of a third author (D.M-G.), who acted as the final referee.
Results
The initial list of retrieved items consisted of 4,339 studies (Supplementary Table 1). After the automated removal of 3,521 duplicates, 818 items were analyzed. Based on titles/abstracts, 792 studies were excluded and 26 full-texts were in-depth reviewed. Based on the above-mentioned inclusion/exclusion criteria, fourteen studies were included in the present systematic review (22–35) (Figure 2). Their major characteristics are reported in Table 2. The quality appraisal is shown in Table 3 and Supplementary Tables 3-5.
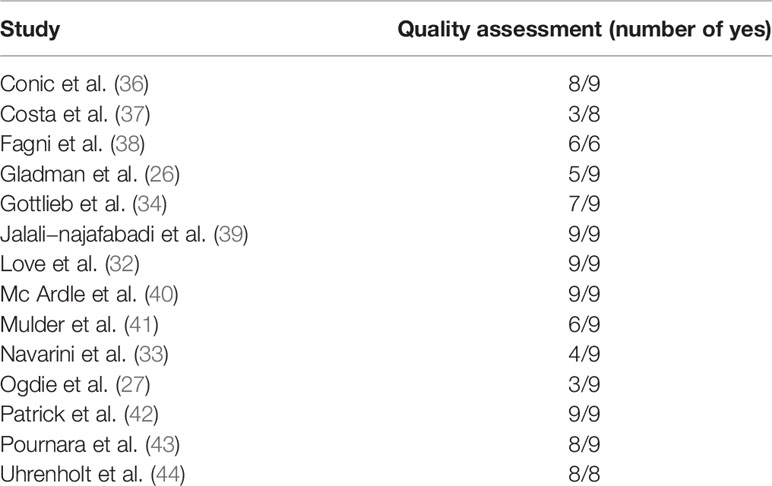
Table 3 Methodological quality assessment of the studies retained in the present systematic literature review.
Exploiting Large Epidemiological Databases and Registries for Early Intercepting Psoriatic Arthritis Patients
Gladman et al. (22) have reviewed existing registries specifically focused on psoriatic patients, including registries based at the University of Toronto, in Toronto, Ontario, and at the Memorial University, in St John’s, Newfoundland in Canada, as well as in Leeds, Bradford and Bath from the UK. Other psoriatic arthritis-related registries are based in Sweden, in Cagliari, Sardinia, in Italy, and in New York, USA. The authors have also proposed a general structure of an international registry specifically dedicated to the collection of psoriatic arthritis cases, in such a way that the different national/local registries can communicate with each other and be easily combined, integrated and mined by researchers. These registries can contain also biological information about patients and provide details about the “molecular epidemiology” of psoriatic arthritis.
Ogdie et al. (23) conducted a retrospective cohort study and mined MarketScan claims data from 2006 to 2019, totaling a sample of 13,661 patients. Subjects suffering from psoriatic arthritis reported a history of complaints for arthritis and dermatological issues in a higher percentage with respect to those without psoriatic arthritis. Arthritis, axial symptoms, and tendonitis/enthesitis generally preceded the diagnosis of psoriatic arthritis. Generally, psoriasis was diagnosed six months before psoriatic arthritis, which was intercepted by rheumatologists, followed by general practitioners and dermatologists.
Deploying Artificial Intelligence Coupled With Epidemiological Big Data for Early Intercepting, Treating and Managing Psoriatic Arthritis Patients
Artificial intelligence can significantly complement and add to expert clinical knowledge. Love et al. (24) extracted a cohort of 2,318 psoriatic arthritis patients from a large academic electronic medical record database (comprising of more than 1,350,000 adult patients). Out of these 2,318 subjects with psoriatic arthritis, 550 were randomly selected for an in-depth chart review and for building, training and validating an artificial based-algorithm. The authors combined structured (i.e., codified) data with unstructured (i.e., textual/narrative, free-text) data and exploited natural language processing (NLP). By integrating these two forms of data, Love and colleagues were able to identify 31 psoriatic arthritis-related predictors. The positive predictive value (PPV) of a single psoriatic arthritis code was 57%, with a 95% confidence interval ranging from 55% to 58%. Integrating structured and unstructured data, by means of NLP, the predictive accuracy of the random forest algorithm significantly improved, reaching a PPV of 90-93% and a sensitivity of 87%, with a much increased area under the curve (AUC).
Navarini et al. (25) harnessed artificial intelligence for predicting cardiovascular risk in psoriatic arthritis patients. The use of already existing validated tools, such as the “Framingham Risk Score” calculator suffers from limitations. The authors explored the implementation of new ad hoc algorithms based on supervised machine learning techniques, such as support vector machine, random forest/feature analysis, and K-nearest neighbor. Machine learning approach proved to be feasible in psoriatic arthritis patients and outperformed with respect to conventional cardiovascular risk factor calculators. AUC ranged from 0.76 to 0.85.
Gottlieb et al. (26) coupled machine learning with evidence-based medicine and individual patient efficacy meta-analysis (IPEM). Authors deployed Bayesian elastic net to quantitatively evaluate baseline data from a cohort of 2,148 psoriatic arthritis patients, assessing a set of 275 predictors. Machine learning was able to identify predictors for an additional benefit of secukinumab.
Deploying Artificial Intelligence Coupled With Molecular Big Data for Early Intercepting Psoriatic Arthritis Patients
Jalali−najafabadi et al. (27) exploited genetic/genomics data from 1,462 psoriatic arthritis and 1,132 psoriatic patients and applied a set of seven supervised artificial intelligence-based algorithms. Besides training and internally validating the risk prediction model, this was externally validated on a UK Biobank dataset consisting of 1,187 participants. HLA_C_*06 and HLA_B_*27 were found to be the most important genetic features. AUC was moderate, ranging from 0.53 to 0.55, which was slightly improved when adding further HLA features (AUC from 0.57 to 0.61).
Patrick et al. (28) devised an automated computational pipeline for predicting the insurgence of psoriatic arthritis among psoriasis patients utilizing data from six cohorts with more than 7,000 genotyped psoriatic arthritis and psoriatic patients. The authors were able to identify nine novel loci for psoriasis and its clinical subtypes, achieving a satisfactory AUC of 0.82 when combining a molecular signature consisting of 200 genetic markers. Precision and specificity were excellent (more than 90% and 100%, respectively), deploying conditional inference forest or shrinkage discriminant analysis.
Conic et al. (29) investigated the feasibility of exploiting the red cell distribution width and mean platelet volume as predictors of major cardiovascular events in psoriatic arthritis patients. The authors coupled a Big Data-based database (Explorys) with a smaller observational trial, to validate their findings. Higher values of the parameters under study predicted a higher cardiovascular risk as well as a poor therapeutic response.
Mulder et al. (30) aimed to detect disease-specific immune profiles from the phenotype of peripheral blood immune cells, which were effectively able to differentiate between psoriasis and psoriatic arthritis patients. Authors utilized a random forest-based algorithm coupled with in-depth flow cytometry and with an excellent AUC of 0.95 and found that psoriatic arthritis patients exhibited upregulated differentiated CD4+CD196+CD183-CD194+ and CD4+CD196-CD183-CD194+ T-cells, whereas CD196+ and CD197+ monocytes, memory CD4+ and CD8+ T-cell subsets and CD4+ regulatory T-cells were downregulated. Joint scores were found to correlate with memory CD8+CD45RA-CD197- effector T-cells and CD197+ monocytes.
Mc Ardle et al. (31) coupled cutting-edge serum proteomics with multivariate machine learning analyses to differentiate between patients suffering from psoriatic arthritis and those with rheumatoid arthritis. Different proteomics techniques were utilized: namely, nano-liquid chromatography mass spectrometry, SomaScan, an aptamer-based assays, and Luminex, a multiplexed antibody assay. AUC was ranging from 0.69 to 0.94, being the lowest for the bead-based immunoassay and the highest for the nano-liquid chromatography mass spectrometry. Machine learning applied on a subset of identified proteins could distinguish between psoriatic arthritis and rheumatoid arthritis patients with an AUC in the range of 0.79-0.85.
Finally, Pournara et al. (32) applied finite mixture models methodology on a cohort of 1,894 psoriatic arthritis patients treated with secukinumab to identify clinically meaningful clusters and phenotypes. The authors were able to identify seven patient clusters (“endotypes” and “theratypes”) in terms of different articular, entheseal and cutaneous burdens and therapeutic responses to secukinumab.
Deploying Digital and Smart Technologies for Early Intercepting Psoriatic Arthritis Patients
In Denmark, Uhrenholt et al. (33) assessed the feasibility of collecting patient-reported outcome measures (PROMs) by means of smartphone apps. The authors find a high acceptability of touchscreen devices among rheumatological patients, including those suffering from psoriatic arthritis, with a high reliability of data collected and correlation with already validated measures of psoriatic arthritis.
Fagni et al. (34) reviewed the applications in the field of mHealth for early intercepting psoriatic arthritis patients. In particular, the authors identified the “Joint Pain Assessment Scoring Tool” (JPAST), a European Union-funded project on digital health, as a promising digital prognostic program that integrates PROMs (patient symptom checker inputs) with biological (serological and genetic) data.
Finally, Costa et al. (35) evaluated telemedicine services offered to a cohort of 105 psoriatic arthritis patients during the still ongoing “Coronavirus Disease 2019” (COVID-19) pandemic. Patients were willing to interact with their patients by means of live video-calls or telephone calls, could upload online photographs and pictures of their manifestations and could receive laboratory and/or instrumental exams and reports via email. During these services, pharmacological treatment could be added, switched or withdrawn/tapered.
Discussion
To the best of our knowledge, this review represents the first systematic review addressing the current state of the use of Big Data, Artificial intelligence, digital and smart technologies for psoriatic arthritis. Most of the studies included concerned molecular applications of Big Data, especially in the fields of genomics and post-genomics. Other studies concerned epidemiological applications, with a practical dearth of studies assessing smart and digital applications for psoriatic arthritis patients.
Epidemiological/clinical Big Data can come from large-scale, often nationwide surveys. These data can guide public and global health policies as well as inform evidence-based medicine and, more specifically, dermatology and rheumatology on a variety of diseases, including psoriatic arthritis and collecting several related measures (39).
Whilst randomized controlled clinical trials represent the gold standard for building and appraising a body of rigorous and clinically relevant evidence, they are often time- and resource-consuming. may not always reflect real-life clinical practice and heterogeneous patient populations, as such limiting the generalizability and external validity of their findings. Real-life or real-world evidence, collected during daily clinical practice and by means of “pragmatic trials”, provides a complementary, “more relaxed” perspective to rigorously and tightly randomized controlled clinical trials (36, 42). While the latter are based on sometimes intrusive data collection methods, with regular study visits, the former exploit digital technologies (like smartphone applications, logs, and electronic health/medical records, or EHR/EMR). In this respect, Big Data-based studies can add to well-designed “small data”-based investigations and randomized controlled clinical trials (41).
To paraphrase what Doctor Lukas Kappenberger, pioneering father of the so-called “computational medicine”, has stated in 2005, the science (i.e., randomized controlled clinical trials) tells scholars and practitioners what they can do, the guidelines and checklists implement what they should do, and clinical registries/databases tell them what they are actually doing, and observing (40, 43).
Currently, there are lots of sources generating epidemiological Big Data, such as surveys, medical insurance data, vital registration data, cohort data, inpatient and outpatient data, among others (40).
These data can be retrospectively or prospectively collected: prospective clinical registries can be defined as large/very large datasets of observational data which have been collected prospectively and systematically and in a structured fashion, to reflect real-world clinical practices and outcomes of a given procedure (treatment, or surgical intervention) across large patient populations, including specific clinical/demographic (sub-)populations (40).
Furthermore, besides being complementary, randomized controlled clinical trials can be embedded within clinical registries (40): this enables to save time and resources and strengthens the generalizability of the findings (40).
Summarizing, Big Data repositories, registries, and databases are increasingly common in the field of rheumatological and dermatological practice and clinical research: there are, however, significant considerable variations in socio-demographic characteristics, co-morbidities, and major complication rates between individual (single- or multi-center) and database-based studies, and even among registry-studies themselves (for example, clinical versus administrative database). This should be accounted for when critically appraising rheumatological and dermatological research and in risk adjustment modeling (40).
In particular, administrative databases (40) can provide researchers and scholars, as well as practitioners and policy- and decision-makers with a lot of information concerning disease epidemiology, co-morbidities, disparities and inequalities in access to healthcare and clinical outcomes. Furthermore, they can inform in a data-driven fashion the decision-making processes underlying dermatological and rheumatological pharmacological treatments or surgical procedures, in terms of pre-operative risk stratification parameters in order to significantly curb/minimize peri-operative morbidity and mortality rates. On the other hand, administrative databases (40) may suffer from clerical inaccuracies, recording bias (due to the very nature of the database and secondary to economic-financial incentives underlying the collection, and maintenance of the dataset), temporal changes in nosology and nomenclature systems as well as in billing codes, and, finally, a dearth of several clinically relevant parameters, including rheumatological and dermatological specific variables and outcomes.
A major issue seriously limiting the deployment of databases and registries is related to their inter-operability and sometimes inconsistent use of definitions. Moreover, not all databases meet regulatory standards (40) and are enough curated/validated. As such, data standardization and meta-data are urgently warranted (40). In this sense, the proposal by Gladman et al. (22) is aimed at overcoming these issues.
Conversely, clinical studies, especially those relying on “Small Data”, even though well-designed and well-conducted, are generally statistically underpowered and are plagued by several biases, including participants sampling and selection bias, which hinders the generalizability of the findings, with samples being not representative of the entire population. It is also difficult to stratify according to a given dermatological or rheumatological pharmacological treatment or surgical procedure if the sample is particularly heterogeneous and the sample size does not allow to make sufficiently statistically robust and reliable calculations. Confidence and certainty can increase with “Big Data”, paralleling, however, the growth of complexity and associated computational costs (45, 46). Also, Big Data-based databases can be affected by biases, as previously mentioned, such as confounding, prevalent user bias (“the depletion of susceptibles”), immortal time bias, measurement bias, recording or association biases and other statistical artifacts, like “reverse epidemiology” or “reverse causality” (47). For instance, Escalante et al. (47), analysing a cohort of 779 patients with rheumatoid arthritis, found that body mass exerted a paradoxical effect on mortality, with patients with high body mass index reporting lower mortality. The authors mentioned that this paradoxical effect could be, at least partly, mediated by comorbidity and by the level of systemic inflammation. However, it is more plausible to deem this effect as a mere statistical artifact.
Furthermore, there are other issues plaguing Big Data-based databases, including selection bias (44). The so-called “index event bias”, which belongs to the family of selection biases, could explain what is known as the “risk factor paradox”, an unexpected phenomenon characterized by discrepant impacts of modifiable risk factors on the progression towards rheumatic diseases, such as osteoarthritis or rheumatoid arthritis.
Wet-lab, bioinformatics, and high-throughput technologies, including microarray chips, next-generation DNA and RNA sequencing and whole-exome sequencing, chromatin-immunoprecipitation-coupled sequencing, and mass-spectrometry-based proteomics analysis can generate a wealth of molecular big data, paving the way for a personalized/individualized rather than “one-size-fits-it-all” rheumatology and dermatology (38).
Molecular big data can elucidate the mechanisms underlying the etiopathogenesis of a given rheumatic/dermatological disease and identify new potential druggable targets for the development of ad hoc pharmacological therapies. Personalized rheumatology can benefit from genome-wide association and post-genomics studies (37), aimed at the identification of new transcription factors, genotypic and phenotypic validations of potential transcriptional regulators, and molecular/cellular mechanisms.
Summarizing, genomics and post-genomics (immunomics- and proteomics)-based assays coupled with machine learning could identify a set of biomarkers that could capture early psoriatic arthritis and differentiate it from other rheumatological conditions, providing individualized/personalized clinical subtype risk assessment. Once validated in larger cohorts, these panels could assist dermatologists and rheumatologists in their decision-making processes (37).
Latest technological achievements in the field of mobile health (mHealth) and ubiquitous health (uHealth), with smartphones, smart devices, smartwatches, and other wearable sensors (38) are revolutionizing the field of rheumatology and dermatology, directly involving, and engaging the patient, improving their therapeutical adherence and compliance, and also enabling remote patient monitoring. Wearable sensors of different types could enable the collection of different crucial parameters (37). Teledermatology and telerheumatology (48, 49) appear promising ways of early intercepting psoriatic arthritis patients.
To summarize, mHealth and digital health-based interventions, including telemonitoring or text messaging, can facilitate clinical data collection and can be customized, meeting the needs of “personalized rheumatology” and “personalized dermatology”.
Conclusions and Future Prospects
Big data analytical techniques can be used to tackle dense, multidimensional datasets concerning clinically heterogeneous and complex diseases, like psoriatic arthritis. Contemporary dermatology and rheumatology can harness digital and smart technologies and artificial intelligence for early intercepting, treating and managing psoriatic arthritis patients. Big Data are increasingly having a more and more relevant role, being highly ubiquitous and pervasive in contemporary society, permeating it and paving the way for new, unprecedented perspectives in biomedicine, including rheumatology and dermatology. Big Data can be a real paradigm shift that revolutionizes dermatological and rheumatological practice and clinical research. However, there are some methodological issues that should be properly addressed and some ethical issues, including ensuring and preserving privacy, that should be considered. Therefore, further research in the field is urgently warranted.
Data Availability Statement
The original contributions presented in the study are included in the article/Supplementary Material. Further inquiries can be directed to the corresponding author.
Author Contributions
NLB conceived the paper. All other authors critically revised it. All authors contributed to the article and approved the submitted version.
Funding
NLB was partially funded by the Celgene supported PARTNER fellowship programme.
Conflict of Interest
The authors declare that the research was conducted in the absence of any commercial or financial relationships that could be construed as a potential conflict of interest.
Publisher’s Note
All claims expressed in this article are solely those of the authors and do not necessarily represent those of their affiliated organizations, or those of the publisher, the editors and the reviewers. Any product that may be evaluated in this article, or claim that may be made by its manufacturer, is not guaranteed or endorsed by the publisher.
Supplementary Material
The Supplementary Material for this article can be found online at: https://www.frontiersin.org/articles/10.3389/fimmu.2022.847312/full#supplementary-material
Supplementary Table 1 | Initial search literature results broken down according to each database mined.
Supplementary Table 2 | Modified Qiao’s checklist for critically appraising Big Data/machine learning-based studies.
Supplementary Table 3 | Outcomes of the assessment of the trustworthiness, relevance, and results of the studies retained in the present systematic literature review, based on the modified Qiao’s critical appraisal tool for critical methodological quality assessment of Big Data/machine learning-based studies.
Supplementary Table 4 | Outcomes of the assessment of the trustworthiness, relevance, and results of the studies retained in the present systematic literature review, based on the Joanna Briggs Institute (JBI)’s critical appraisal tool for critical methodological quality assessment of cross-sectional investigations.
Supplementary Table 5 | Outcomes of the assessment of the trustworthiness, relevance, and results of the studies retained in the present systematic literature review, based on the relevant Joanna Briggs Institute (JBI)’s critical appraisal tool for the critical methodological quality assessment (namely, the “Explanation of text and expert opinion critical appraisal tool”).
References
1. Mathers CD. History of Global Burden of Disease Assessment at the World Health Organization. Arch Public Health (2020) 78:77. doi: 10.1186/s13690-020-00458-3
2. GBD 2017 SDG Collaborators. Measuring Progress From 1990 to 2017 and Projecting Attainment to 2030 of the Health-Related Sustainable Development Goals for 195 Countries and Territories: A Systematic Analysis for the Global Burden of Disease Study 2017. Lancet (2018) 392(10159):2091–138. doi: 10.1016/S0140-6736(18)32281-5
3. Rendon A, Schäkel K. Psoriasis Pathogenesis and Treatment. Int J Mol Sci (2019) 20(6):1475. doi: 10.3390/ijms20061475
4. Damiani G, Bragazzi NL, Karimkhani Aksut C, Wu D, Alicandro G, McGonagle D, et al. The Global, Regional, and National Burden of Psoriasis: Results and Insights From the Global Burden of Disease 2019 Study. Front Med (Lausanne) (2021) 8:743180. doi: 10.3389/fmed.2021.743180
5. Zabotti A, De Lucia O, Sakellariou G, Batticciotto A, Cincinelli G, Giovannini I, et al. Predictors, Risk Factors, and Incidence Rates of Psoriatic Arthritis Development in Psoriasis Patients: A Systematic Literature Review and Meta-Analysis. Rheumatol Ther (2021) 8(4):1519–34. doi: 10.1007/s40744-021-00378-w
6. Auffray C, Charron D, Hood L. Predictive, Preventive, Personalized and Participatory Medicine: Back to the Future. Genome Med (2010) 2(8):57. doi: 10.1186/gm178
7. Sagner M, McNeil A, Puska P, Auffray C, Price ND, Hood L, et al. The P4 Health Spectrum - A Predictive, Preventive, Personalized and Participatory Continuum for Promoting Healthspan. Prog Cardiovasc Dis (2017) 59(5):506–21. doi: 10.1016/j.pcad.2016.08.002
8. Bragazzi NL. From P0 to P6 Medicine, a Model of Highly Participatory, Narrative, Interactive, and "Augmented" Medicine: Some Considerations on Salvatore Iaconesi's Clinical Story. Patient Prefer Adherence (2013) 7:353–9. doi: 10.2147/PPA.S38578
9. Bragazzi NL, Damiani G, Martini M. From Rheumatology 1.0 to Rheumatology 4.0 and Beyond: The Contributions of Big Data to the Field of Rheumatology. Mediterr J Rheumatol (2019) 30(1):3–6. doi: 10.31138/mjr.30.1.3
10. Bragazzi NL, Gianfredi V, Villarini M, Rosselli R, Nasr A, Hussein A, et al. Vaccines Meet Big Data: State-Of-the-Art and Future Prospects. From the Classical 3Is ("Isolate-Inactivate-Inject") Vaccinology 1.0 to Vaccinology 3.0, Vaccinomics, and Beyond: A Historical Overview. Front Public Health (2018) 6:62. doi: 10.3389/fpubh.2018.00062
11. Poddubnyy D. Precision Medicine in Rheumatology: Are We Getting Closer? Lancet (2021) 397(10271):258–9. doi: 10.1016/S0140-6736(20)32652-0
12. Fredman G, Skov L, Mann M, Dyring-Andersen B. Towards Precision Dermatology: Emerging Role of Proteomic Analysis of the Skin. Dermatology (2021) 1:1–10. doi: 10.1159/000516764
13. Scarpa R. Psoriatic Syndrome or Psoriatic Disease? J Rheumatol (2020) 47(6):941. doi: 10.3899/jrheum.200051
14. Lubrano E, Scriffignano S, Perrotta FM. Psoriatic Arthritis, Psoriatic Disease, or Psoriatic Syndrome? J Rheumatol (2019) 46(11):1428–30. doi: 10.3899/jrheum.190054
15. Agache I, Akdis CA. Precision Medicine and Phenotypes, Endotypes, Genotypes, Regiotypes, and Theratypes of Allergic Diseases. J Clin Invest (2019) 129(4):1493–503. doi: 10.1172/JCI124611
16. Mendes-Bastos P, Nero P, Ferreira P, Bernardo A, Barcelos A, Pimentel Dos Santos FM, et al. A Multidisciplinary Approach in Psoriatic Disease: The Different Models of Dermatology-Rheumatology Collaborations in Portugal. Acta Reumatol Port (2021) 46(4):333–41.
17. Menter MA, Griffiths CE. Psoriasis: The Future. Dermatol Clin (2015) 33(1):161–6. doi: 10.1016/j.det.2014.09.012
18. Winthrop KL, Weinblatt ME, Bathon J, Burmester GR, Mease PJ, Crofford L, et al. Unmet Need in Rheumatology: Reports From the Targeted Therapies Meeting 2019. Ann Rheum Dis (2020) 79(1):88–93. doi: 10.1136/annrheumdis-2019-216151
19. Page MJ, McKenzie JE, Bossuyt PM, Boutron I, Hoffmann TC, Mulrow CD, et al. The PRISMA 2020 Statement: An Updated Guideline for Reporting Systematic Reviews. BMJ (2021) 372:n71. doi: 10.1136/bmj.n71
20. Kedra J, Radstake T, Pandit A, Baraliakos X, Berenbaum F, Finckh A, et al. Current Status of Use of Big Data and Artificial Intelligence in RMDs: A Systematic Literature Review Informing EULAR Recommendations. RMD Open (2019) 5(2):e001004. doi: 10.1136/rmdopen-2019-001004
21. Qiao N. A Systematic Review on Machine Learning in Sellar Region Diseases: Quality and Reporting Items. Endocr Connect (2019) 8(7):952–60. doi: 10.1530/EC-19-0156
22. Gladman DD, Ritchlin C, Helliwell PS. Psoriatic Arthritis Clinical Registries and Genomics. Ann Rheum Dis (2005) 64 Suppl 2(Suppl 2):ii103–5. doi: 10.1136/ard.2004.030973
23. Ogdie A, Rozycki M, Arndt T, Shi C, Kim N, Hur P. Longitudinal Analysis of the Patient Pathways to Diagnosis of Psoriatic Arthritis. Arthritis Res Ther (2021) 23(1):252. doi: 10.1186/s13075-021-02628-2
24. Love TJ, Cai T, Karlson EW. Validation of Psoriatic Arthritis Diagnoses in Electronic Medical Records Using Natural Language Processing. Semin Arthritis Rheumatol (2011) 40(5):413–20. doi: 10.1016/j.semarthrit.2010.05.002
25. Navarini L, Sperti M, Currado D, Costa L, Deriu MA, Margiotta DPE, et al. A Machine-Learning Approach to Cardiovascular Risk Prediction in Psoriatic Arthritis. Rheumatology (Oxford) (2020) 59(7):1767–9. doi: 10.1093/rheumatology/kez677
26. Gottlieb AB, Mease PJ, Kirkham B, Nash P, Balsa AC, Combe B, et al. Secukinumab Efficacy in Psoriatic Arthritis: Machine Learning and Meta-Analysis of Four Phase 3 Trials. J Clin Rheumatol (2021) 27(6):239–47. doi: 10.1097/RHU.0000000000001302
27. Jalali-Najafabadi F, Stadler M, Dand N, Jadon D, Soomro M, Ho P, et al. Application of Information Theoretic Feature Selection and Machine Learning Methods for the Development of Genetic Risk Prediction Models. Sci Rep (2021) 11(1):23335. doi: 10.1038/s41598-021-00854-x
28. Patrick MT, Stuart PE, Raja K, Gudjonsson JE, Tejasvi T, Yang J, et al. Genetic Signature to Provide Robust Risk Assessment of Psoriatic Arthritis Development in Psoriasis Patients. Nat Commun (2018) 9(1):4178. doi: 10.1038/s41467-018-06672-6
29. Conic RR, Damiani G, Schrom KP, Ramser AE, Zheng C, Xu R, et al. Psoriasis and Psoriatic Arthritis Cardiovascular Disease Endotypes Identified by Red Blood Cell Distribution Width and Mean Platelet Volume. J Clin Med (2020) 9(1):186. doi: 10.3390/jcm9010186
30. Mulder MLM, He X, van den Reek JMPA, Urbano PCM, Kaffa C, Wang X, et al. Blood-Based Immune Profiling Combined With Machine Learning Discriminates Psoriatic Arthritis From Psoriasis Patients. Int J Mol Sci (2021) 22(20):10990. doi: 10.3390/ijms222010990
31. Mc Ardle A, Kwasnik A, Szentpetery A, Hernandez B, Parnell A, de Jager W, et al. Identification and Evaluation of Serum Protein Biomarkers That Differentiate Psoriatic Arthritis From Rheumatoid Arthritis. Arthritis Rheumatol (2022) 74(1):81–91. doi: 10.1002/art.41899
32. Pournara E, Kormaksson M, Nash P, Ritchlin CT, Kirkham BW, Ligozio G, et al. Clinically Relevant Patient Clusters Identified by Machine Learning From the Clinical Development Programme of Secukinumab in Psoriatic Arthritis. RMD Open (2021) 7(3):e001845. doi: 10.1136/rmdopen-2021-001845
33. Uhrenholt L, Christensen R, Dreyer L, Schlemmer A, Hauge EM, Krogh NS, et al. Using a Novel Smartphone Application for Capturing of Patient-Reported Outcome Measures Among Patients With Inflammatory Arthritis:A Randomized, Crossover, Agreement Study. Scand J Rheumatol (2022) 51(1):25–33. doi: 10.1080/03009742.2021.1907925
34. Fagni F, Knitza J, Krusche M, Kleyer A, Tascilar K, Simon D. Digital Approaches for a Reliable Early Diagnosis of Psoriatic Arthritis. Front Med (Lausanne) (2021) 8:718922. doi: 10.3389/fmed.2021.718922
35. Costa L, Tasso M, Scotti N, Mostacciuolo E, Girolimetto N, Foglia F, et al. Telerheumatology in COVID-19 Era: A Study From a Psoriatic Arthritis Cohort. Ann Rheum Dis (2020) 80:E46. doi: 10.1136/annrheumdis-2020-217806
36. Hernandez-Boussard T, Monda KL, Crespo BC, Riskin D. Real World Evidence in Cardiovascular Medicine: Ensuring Data Validity in Electronic Health Record-Based Studies. J Am Med Inform Assoc (2019) 26(11):1189–94. doi: 10.1093/jamia/ocz119
37. Elliott A, McGonagle D, Rooney M. Integrating Imaging and Biomarker Assessment to Better Define Psoriatic Arthritis and Predict Response to Biologic Therapy. Rheumatology (Oxford) (2021) 60(Supplement_6):vi38–52. doi: 10.1093/rheumatology/keab504
38. Parad JE, Liao W. Bioinformatic Applications in Psoriasis: Genetics, Transcriptomics, and Microbiomics. Semin Cutan Med Surg (2019) 38(1):E3–11. doi: 10.12788/j.sder.2019.003
39. Mease PJ. Measures of Psoriatic Arthritis: Tender and Swollen Joint Assessment, Psoriasis Area and Severity Index (PASI), Nail Psoriasis Severity Index (NAPSI), Modified Nail Psoriasis Severity Index (mNAPSI), Mander/Newcastle Enthesitis Index (MEI), Leeds Enthesitis Index (LEI), Spondyloarthritis Research Consortium of Canada (SPARCC), Maastricht Ankylosing Spondylitis Enthesis Score (MASES), Leeds Dactylitis Index (LDI), Patient Global for Psoriatic Arthritis, Dermatology Life Quality Index (DLQI), Psoriatic Arthritis Quality of Life (PsAQOL), Functional Assessment of Chronic Illness Therapy-Fatigue (FACIT-F), Psoriatic Arthritis Response Criteria (PsARC), Psoriatic Arthritis Joint Activity Index (PsAJAI), Disease Activity in Psoriatic Arthritis (DAPSA), and Composite Psoriatic Disease Activity Index (CPDAI). Arthritis Care Res (Hoboken) (2011) 63 Suppl 11:S64–85. doi: 10.1002/acr.20577
40. Meltzer SN, Weintraub WS. The Role of National Registries in Improving Quality of Care and Outcomes for Cardiovascular Disease. Methodist Debakey Cardiovasc J (2020) 16(3):205–11. doi: 10.14797/mdcj-16-3-205
41. Ogdie A, Coates L. The Changing Face of Clinical Trials in Psoriatic Arthritis. Curr Rheumatol Rep (2017) 19(4):21. doi: 10.1007/s11926-017-0642-z
42. Hemkens LG. How Routinely Collected Data for Randomized Trials Provide Long-Term Randomized Real-World Evidence. JAMA Netw Open (2018) 1(8):e186014. doi: 10.1001/jamanetworkopen.2018.6014
43. Heart Rhythm Society, Food and Drug Administration. in: Proceedings Document From the Policy Conference on Pacemaker and ICD Performance, Washington, DC, 2005 Sep 16, Kappenberger L, panelist.
44. Choi HK, Nguyen US, Niu J, Danaei G, Zhang Y. Selection Bias in Rheumatic Disease Research. Nat Rev Rheumatol (2014) 10(7):403–12. doi: 10.1038/nrrheum.2014.36
45. Gossec L, Kedra J, Servy H, Pandit A, Stones S, Berenbaum F, et al. EULAR Points to Consider for the Use of Big Data in Rheumatic and Musculoskeletal Diseases. Ann Rheum Dis (2020) 79(1):69–76. doi: 10.1136/annrheumdis-2019-215694
46. Huizinga TWJ, Knevel R. Interpreting Big-Data Analysis of Retrospective Observational Data. Lancet Rheumatol (2020) 2(11):e652–3. doi: 10.1016/S2665-9913(20)30289-7
47. Escalante A, Haas RW, del Rincón I. Paradoxical Effect of Body Mass Index on Survival in Rheumatoid Arthritis: Role of Comorbidity and Systemic Inflammation. Arch Intern Med (2005) 165(14):1624–9. doi: 10.1001/archinte.165.14.1624
48. Muehlensiepen F, Knitza J, Marquardt W, May S, Krusche M, Hueber A, et al. Opportunities and Barriers of Telemedicine in Rheumatology: A Participatory, Mixed-Methods Study. Int J Environ Res Public Health (2021) 18(24):13127. doi: 10.3390/ijerph182413127
Keywords: psoriatic arthritis, big data, artificial intelligence, digital technologies, early interception, prevention
Citation: Bragazzi NL, Bridgewood C, Watad A, Damiani G, Kong JD and McGonagle D (2022) Harnessing Big Data, Smart and Digital Technologies and Artificial Intelligence for Preventing, Early Intercepting, Managing, and Treating Psoriatic Arthritis: Insights From a Systematic Review of the Literature. Front. Immunol. 13:847312. doi: 10.3389/fimmu.2022.847312
Received: 02 January 2022; Accepted: 19 January 2022;
Published: 10 March 2022.
Edited by:
Michele Maria Luchetti Gentiloni, Marche Polytechnic University, ItalyCopyright © 2022 Bragazzi, Bridgewood, Watad, Damiani, Kong and McGonagle. This is an open-access article distributed under the terms of the Creative Commons Attribution License (CC BY). The use, distribution or reproduction in other forums is permitted, provided the original author(s) and the copyright owner(s) are credited and that the original publication in this journal is cited, in accordance with accepted academic practice. No use, distribution or reproduction is permitted which does not comply with these terms.
*Correspondence: Nicola Luigi Bragazzi , bragazzi@yorku.ca