- 1Department of Cardiology, Ningbo Hospital of Zhejiang University (Ningbo First Hospital), School of Medicine, Zhejiang University, Ningbo, China
- 2Department of Cardiology, Second Affiliated Hospital of Zhejiang University, School of Medicine, Zhejiang University, Hangzhou, China
- 3Cardiology Center, Ningbo First Hospital, Ningbo University, Ningbo, China
- 4School of Medicine, Ningbo University, Ningbo First Hospital, Ningbo, China
Background: Observational studies suggested that multiple sclerosis (MS) is associated with cardiovascular diseases (CVDs). However, the causal association has not been fully elucidated. Thus, we aim to assess the causality of the associations of MS with risk of CVDs.
Methods: A two-sample Mendelian randomization (MR) study was performed to explore the causality. Genetic instruments were identified for MS from a genome-wide association study (GWAS) involving 115,803 individuals. Summary-level data for CVDs were obtained from different GWAS meta-analysis studies. MR analysis was conducted mainly using the inverse-variance weighted (IVW) method. Sensitivity analyses were further performed to ensure the robustness of the results.
Results: This MR study found suggestive evidence that genetic liability to MS was associated with an increased risk of coronary artery disease (CAD) [odds ratio (OR), 1.02; 95% confidence interval (CI), 1.00–1.04; p = 0.03], myocardial infarction (MI) (OR, 1.03; 95% CI, 1.00–1.06; p = 0.01), heart failure (HF) (OR, 1.02; 95% CI, 1.00–1.04; p = 0.02), all-cause stroke (AS) (OR, 1.02; 95% CI, 1.00–1.05; p = 0.02), and any ischemic stroke (AIS) (OR, 1.02; 95% CI, 1.00–1.05; p = 0.04). The null-association was observed between MS and the other CVDs. Further analyses found little evidence of pleiotropy.
Conclusions: We provided suggestive genetic evidence for the causal associations of MS with increased risk of CAD, MI, HF, AS, and AIS, which highlighted the significance of active monitoring and prevention of cardiovascular risk to combat cardiovascular comorbidities in MS patients.
Introduction
Multiple sclerosis (MS) is one of the most common chronic neurological disorders targeting the central nervous system (1). Characterized most generally by impaired ambulation and slowed cognitive processing, MS is the principal non-traumatic cause of disability (2). Cardiovascular diseases (CVDs), comprising coronary artery disease (CAD), myocardial infarction (MI), heart failure (HF), atrial fibrillation (AF), and stroke, are the leading causes of mortality and morbidity worldwide (3). CVDs account for 30% of all global deaths and place a substantial economic burden on the society (4).
The associations between MS and CVDs drawn from epidemiological studies have attracted much attention in the recent years (5, 6). A population-based retrospective cohort study based on 12,251 patients with MS and 72,572 controls suggested that MS was associated with an increased risk of acute coronary syndrome [hazard ratio (HR), 1.28; 95% CI, 1.09–1.51] (7). MS was also associated with increased stroke risks (HR, 4.93; 95% CI, 2.85–8.55) in a cohort study followed 5 years (8). Similarly, another retrospective study involving 14,565 patients with MS and 72,825 matched controls found that MS contributed to a higher risk of MI (adjusted HR 1.63; 95% CI 1.43–1.87) (9). However, a Swedish cohort study of 7,767 cases and 76,045 controls indicated that MS was associated with a decreased risk of angina pectoris and AF (10). The higher incidence of cardiovascular comorbidity compared with age-matched healthy controls may influence low-, mid- and long-term clinical outcomes, namely, greater general disability status, worse physical outcomes, higher depression scores, cognitive aging, and low satisfaction with quality of life (11). Besides, cardiovascular comorbidity burden was reported to be associated with MRI-derived disease outcomes (such as white matter hyperintensities, gray matter volumes and hippocampal volumes) in MS patients (12, 13). Meanwhile, higher Framingham risk score was associated with higher risk of relapses, disability, and disease-modifying therapy escalation in patients with MS over a 5-year follow-up (14). Traditional observational studies are susceptible to potential confounders and reverse causality bias, making it difficult to infer the causality (15). Therefore, the potential causal relationship between MS and CVDs remains unclear.
The Mendelian randomization (MR) method uses genetic variants as the proxy of the exposure in order to evaluate the causal effect of that exposure on the outcome (16). Since the genetic variants are randomly passed to the offspring and kept constant after conception, they can circumvent confounding factors and diminish interference of reverse causality between exposures and outcomes (17).
Hence, to examine the potential causality of genetic liability to MS with the risk of CVDs, we performed a two-sample MR analysis, leveraging the most updated genome-wide association study (GWAS) data.
Methods
Study Design
The current study was a two-sample MR study based on the genetic data obtained from the worldwide genetic consortia. The conceptual schematic of the current MR study is shown in Figure 1. Briefly, genetic variants were used as instrumental variables to investigate the causal associations between MS and CVDs based on three core assumptions. First, the selected genetic variants should be significantly associated with MS (p <5 × 10−8). Second, the genetic variants should not be associated with any potential known confounders. Third, the genetic variants should influence the CVDs only via MS. All original studies included have obtained ethical review approval and informed consent from the participants.
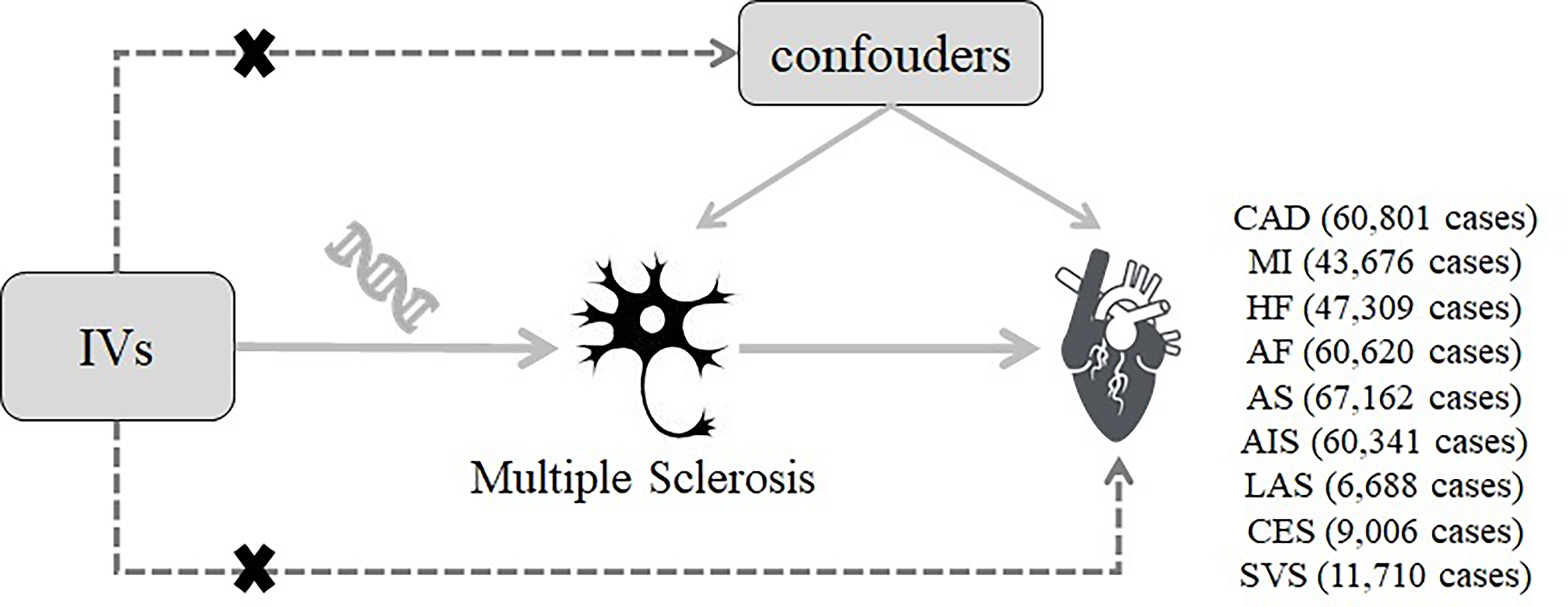
Figure 1 Diagram of the two-sample Mendelian randomization study for the association between multiple sclerosis and the risk of cardiovascular diseases. IVs, instrumental variables; CAD, coronary artery disease; MI, myocardial infarction; HF, heart failure; AF, atrial fibrillation; AS, all-cause stroke; AIS, any ischemic stroke; LAS, large artery stroke; CES, cardioembolic stroke; SVS, small vessel stroke.
Instrument Selection
Genetic instrumental variables for MS were extracted from the International Multiple Sclerosis Genetics Consortium, namely, 47,429 MS cases and 68,374 control, which explain ~48% of the estimated heritability for MS (2). The genetic associations with MS were adjusted for confounding factors, namely, use of immunomodulatory drugs, age, gender, batch effects, and the first 10 principal components (accounting for unmeasured confounders). In total, 233 single nucleotide polymorphisms (SNPs) were identified, in which 78 reached genome-wide significance (p <5 × 10−8). The F-statistics was calculated to assess the strength of each instrument. A threshold of F-statistics >10 indicated that the genetic variants had relatively strong estimated effects in the MR analyses (18). In addition, to ensure that the SNPs were valid and independent (r2 <0.01), the linkage disequilibrium (LD) in selected SNPs was calculated based on the 1,000 genome LD reference panel (EUR population). In the current MR study, 68 independent SNPs were identified as instrumental variables for MS after exclusion of ten SNPs in moderate LD (r2 >0.01).
Data Sources for Outcomes
Summary-level data for the associations of MS-associated SNPs with CAD and MI were derived from the Coronary Artery Disease Genome-Wide Replication and Meta-analysis plus The Coronary Artery Disease (CARDIoGRAMplusC4D) 1,000 genome-based GWAS meta-analysis, which involved 60,801 CAD cases (123,504 controls) and 43,676 MI cases (128,199 controls) from 48 cohorts (approximately 77% of participants with European ancestry) (19). Summary-level data for AF were obtained from meta-analyzed GWAS, namely, 1,030,836 participants (60,620 cases and 970,216 controls) from the Nord-Trøndelag Health Study (HUNT), the Collaborative Analysis of Diagnostic Criteria in Europe study (deCODE), the Michigan Genomics Initiative (MGI), the DiscovEHR, the UK Biobank, and the Atrial Fibrillation Consortium (AFGen) Consortium (20). Summary statistics for HF were acquired from the Heart Failure Molecular Epidemiology for Therapeutic Targets (HERMES) Consortium, which contained 47,309 cases and 930,014 control of European ancestry (21). For stroke and ischemic stroke subtypes, summary data were extracted from a multi-ancestry GWAS of 29 studies in the MEGASTROKE consortium involving 67,162 cases of all-cause stroke (AS), 60,341 cases of any ischemic stroke (AIS), 6,688 cases of large artery stroke (LAS), 9,006 cases of cardioembolic stroke (CES), and 11,710 cases of small vessel stroke (SVS) (22). The information of all the genetic datasets used in the current study is displayed in Table 1.
Statistical Analysis
We performed a two-sample MR analysis using the fixed-effects inverse-variance weighted (IVW) method as the main analysis to evaluate the causal associations between MS and CVDs. The genome-wide significant (p <5 × 10−8) and independent (r2 <0.01) SNPs were identified as the genetic instrumental variables of MS from the exposure dataset. Then the genetic associations of MS-related SNPs with CVDs were obtained from corresponding outcome datasets. The Wald ratio method was applied to derive MR estimates of the effect of MS on CVDs, which was the ratio of SNP-outcome genetic effect over SNP-exposure genetic effect (23). This method provided the most robust causal estimates, while was relatively sensitive to pleiotropy (24). Thus, the Weighted median method, the MR-Egger regression, and the MR Pleiotropy Residual Sum and Outlier (MR-PRESSO) method were further applied as supplementary analyses. Specifically, the Weighted median method can generate reliable causal estimates when at least 50% of the weight in the analysis comes from effective SNPs (25). The MR-Egger regression can detect potential pleiotropy and provide estimates after correction for pleiotropy (26). The MR-PRESSO method could detect possible outliers and calculate causal estimates after removing the identified outliers (27). The Cochrane’s Q-derived p-value was calculated to evaluate the degree of heterogeneity, where p <0.05 indicated horizontal pleiotropy (28). The p-value for the intercept in MR-Egger was used to detect the directional pleiotropic effect (26). Furthermore, scatter plots depicting the associations of genetic liability to MS with CVDs were provided. The Bonferroni correction was used to account for multiple testing and association with a p-value <0.006 (0.05/9 outcomes) was considered statistically significant. The p-values between 0.006 and 0.05 were deemed suggested associations. The specialized web application named mRnd (https://shiny.cnsgenomics.com/mRnd/) was used for the statistical power calculation of MR analyses (29). The main parameters contained the sample size, proportion of cases, odds ratio (OR) of outcome, and proportion of variance explained for the exposure variable (r2). All the data analyses were conducted using R Software (version 4.1.1.; R Foundation for Statistical Computing, Vienna, Austria), and R package TwoSampleMR (https://github.com/MRCIEU/TwoSampleMR), and MR-PRESSO (https://github.com/rondolab/MR-PRESSO) (17).
Results
The detailed characteristics of the SNPs associated with MS are displayed in Supplementary Table 1. The F-statistics of all 68 SNPs was above the threshold of 10, which indicated that they strongly predicted MS in the MR analysis.
The results of the MR analysis estimates for the effect of MS on risk of CVDs are shown in Figure 2. In the IVW analyses, one unit increase in log odds of MS was suggestively associated with higher risks of CAD (OR, 1. 02; 95% CI, 1.00–1.04; p = 0.03), MI (OR, 1.03; 95% CI, 1.00–1.06; p = 0.01), HF (OR, 1.02; 95% CI, 1.00–1.04; p = 0.02), AS (OR, 1.02; 95% CI, 1.00–1.05; p = 0.02), and AIS (OR, 1.02; 95% CI, 1.00–1.05; p = 0.04) (Figure 2). However, no evidence was found for the causal associations between MS and the risk of AF (OR, 1.00; 95% CI, 0.98–1.01; p = 0.92) or other ischemic stroke subtypes (LAS: OR, 1.00; 95% CI, 0.95–1.06; p = 0.87; CES: OR, 1.02; 95% CI, 0.98–1.07; p = 0.26; SVS: OR, 1.03; 95% CI, 0.98–1.09; p = 0.21) (Figure 2). In addition, scatter plots visually displayed the associations between MS and subtypes of CVDs (Supplementary Figures 1–9).
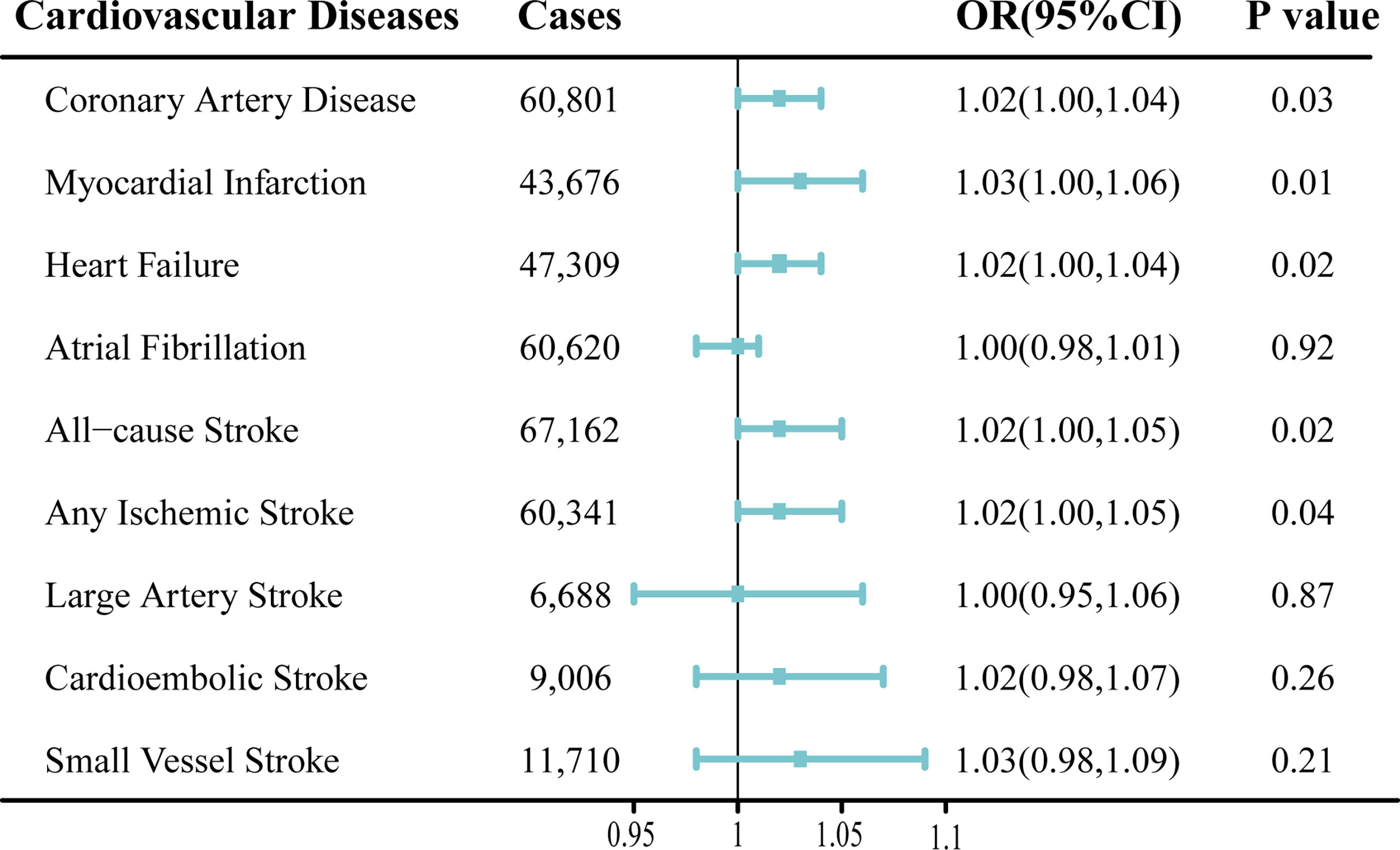
Figure 2 Mendelian randomization analysis estimates of multiple sclerosis and the risk of cardiovascular diseases. Odds ratios are scaled to per genetically predicted 1 log-odds unit increase in the liability to multiple sclerosis. OR, odds ratio; CI, confidence interval.
The Weighted median method, MR-Egger regression, and MR-PRESSO method were performed as sensitivity analyses to reanalyze the suggestive associations observed in primary IVW analyses (Table 2). In the sensitivity analyses, the association patterns remained directionally consistent in most statistical models, and stable association was found in the MR-PRESSO analysis of MI (OR, 1.03; 95% CI, 1.01–1.05; p =0.02) (Table 2). Weak evidence of directional pleiotropy was found in the MR-Egger intercept tests (p >0.05; Table 3). However, the Cochrane’s Q test suggested that the main MR analyses suffered from different degrees of heterogeneity (Table 3). Nonetheless, we had more than 80% statistical power to detect the ORs for associations of MS with CAD (89%), MI (96%), HF (88%), AS (98%), and AIS (96%) (Supplementary Table 2).
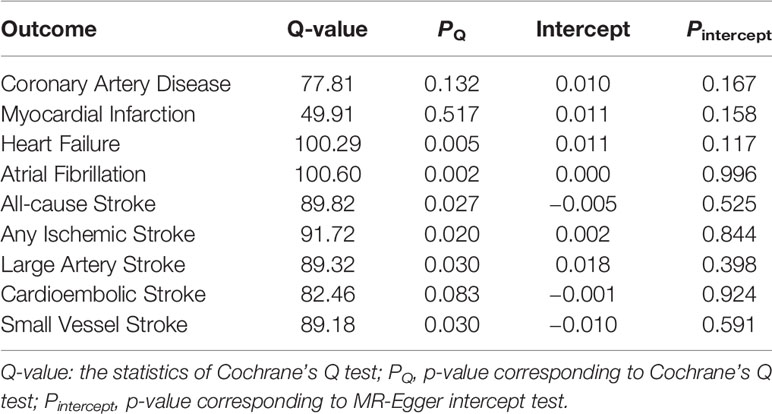
Table 3 Heterogeneity and pleiotropy tests for the associations of multiple sclerosis with cardiovascular diseases.
Discussion
In the current study, summary-level data from the large consortia and genetic studies were leveraged to investigate the causality of MS with CVDs. We found suggestive genetic evidence for the causal associations of genetic liability to MS with elevated risk of CAD, MI, HF, AS, and AIS, but not with AF or other stroke subtypes (LAS, CES, and SVS).
Results reported by several previous observational epidemiological studies on the associations between MS and CVDs were inconsistent and mixed. A meta-analysis, namely, 12 studies of 471,874 individuals indicated that compared with the general population, the risk of CVDs in patients with MS increased 29% (OR, 1.29; 95% CI, 1.80–2.51) (30). Another recent meta-analysis comprising 19 studies (44 to 66,616 participants) reported that the pooled OR estimate for MI was 1.41 in MS patients compared to the healthy controls (31). Besides, a meta-analysis of 9 observational studies suggested that MS was associated with an increased risk of MI and HF, but not ischemic heart disease or bradycardia (32). A population-based retrospective cohort study involving 12,251 MS patients and 72,572 controls showed that MS was associated with both acute coronary syndrome (HR, 1.28; 95% CI, 1.09–1.51) and cerebrovascular disease (HR, 1.59; 95% CI, 1.32–1.92) risk (7). Similarly, several retrospective cohort studies indicated MS led to a higher risk among MI, stroke, and HF (5, 6), which was in line with our MR results. Meanwhile, several prior studies also suggested that MS was associated with an increased risk of stroke (8, 33, 34). However, some other studies drew the inconsistent conclusion that MS was not a risk factor for some subtypes of CVDs. In a prospective cohort study, after adjusting for year and sex, the incidence of heart disease between MS patients and controls was no longer different [incidence rate ratio (IRR) 1.00; 95% CI, 0.94–1.06] (35). In addition, a population case–cohort study found no association between MS and ischemic heart disease and a 43% lower risk of AF compared with non-MS patients (10). Therefore, the current MR study was timely to draw attention to the assessment of the causality of MS with CVDs. A very recent two-sample MR study reported that MS was causally associated with an increased risk of MI (OR = 1.03), which was in line with our findings (36). However, this study found weak evidence for the causal association between MS and stroke using data from the CARDIoGRAMplusC4D consortium (40,585 stroke cases), while the genetic associations in the current study were obtained from the MEGASTROKE consortium involving 67,162 cases of all-cause stroke. On the one hand, the calculation of risk was according to the log odds of MS, which may partly explain the low values of ORs. On the other hand, there were much more healthy populations included in the outcome datasets in the current study, especially in the HF and the AF datasets.
The current two-sample MR study indicated suggestive causal associations between MS and a range of CVDs. Several underlying mechanisms have been proposed to explain the increased risk of CVDs in patients with MS. The causality found in the MR analysis was most likely due to common risk factors or etiological pathways, such as inflammation (37–39). Meanwhile, chronic pressure was associated with worsening disease in MS patients, which was related to a higher risk of stroke and MI (40, 41). Another speculative possibility was that immobilization and lack of physical activity amongst MS patients also increased the risk of CVDs (42). Besides, the immune-modulating treatments such as interferon β (INF-β) for MS patients were found to be associated with the risk of CVDs (43, 44). Other MS drugs like fingolimod were also reported to contribute to the risk of CVDs (45, 46). Previous studies have found that homocysteine plasma levels may be higher in patients with MS, which was a risk factor for CVDs (47–49). Other observational studies suggested endothelial dysfunction was evident in patients with MS (50, 51), which was a well-established risk factor of CAD (52).
The present study included several notable advantages. First, for the first time, MR analysis was employed to explore the causality of MS with a range of CVDs, which could largely reduce the influence of the environmental confounders and reverse causality. Second, the selected SNPs explained a relatively high proportion of MS. Furthermore, the large sample size of each MR analysis and the robust estimated effects of each instrumental variable (all F-statistics >10) ensure the statistical power in our study. Finally, several sensitivity analyses, such as the Weighted median analysis, MR-Egger regression, MR-PRESSO, and leave-one-out analysis were conducted to confirm the consistency of causal relationships.
However, several limitations should be considered when interpreting our findings. First, the influence of potential pleiotropy still could not be completely ruled out, though weak evidence of horizontal pleiotropy was found in the MR-Egger intercept tests and MR-PRESSO analyses. Second, different degrees of heterogeneity were detected in the Cochrane’s Q tests, indicating our analyses may be affected by pleiotropy. Third, MS patients in the current study were all of European descent, which might limit the generalizability of our findings to other populations. Further studies are needed to verify our findings in the non-European descents. Finally, we reported the relatively modest effect size (low values of ORs), thus the results of the current MR study should be interpreted with caution.
Conclusion
In conclusion, this MR study provided suggestive genetic evidence for the causal associations of MS with increased risks of CAD, MI, HF, AS, and AIS. These findings highlight the significance of active monitoring and prevention of cardiovascular risk to combat cardiovascular comorbidities in MS patients. Further studies are required to illuminate the effectiveness of timely treating MS on reducing the risk of CVDs and investigate the potential mechanisms of these causal links. Considering the modest effect size, the results should be interpreted with caution.
Data Availability Statement
The datasets presented in this study can be found in online repositories. The names of the repository/repositories and accession number(s) can be found in the article/Supplementary Material.
Author Contributions
FY and TH designed the study and analyzed the data. FY drafted the manuscript. TH, JY, KH and HC interpreted the data and provided feedback on manuscript drafts. All authors listed have made a substantial, direct, and intellectual contribution to the work and approved it for publication.
Funding
This work was supported by grants from the Key Laboratory of Precision Medicine for Atherosclerotic Diseases of Zhejiang Province, China (Grant No. 2022E10026), the Major Project of Science and Technology Innovation 2025 in Ningbo, China (Grant No. 2021Z134), and the Key Research and Development Project of Zhejiang Province, China (Grant No. 2021C03096).
Conflict of Interest
The authors declare that the research was conducted in the absence of any commercial or financial relationships that could be construed as a potential conflict of interest.
Publisher’s Note
All claims expressed in this article are solely those of the authors and do not necessarily represent those of their affiliated organizations, or those of the publisher, the editors and the reviewers. Any product that may be evaluated in this article, or claim that may be made by its manufacturer, is not guaranteed or endorsed by the publisher.
Acknowledgments
Summary-level data for genetic associations with the MS and CVDs have been contributed by the International Multiple Sclerosis Genetics Consortium, the CARDIoGRAMplusC4D, the MEGASTROKE, the HERMES, the AFGen, the HUNT, the MGI, the deCODE, and the UK Biobank. The authors thank all investigators for providing the data publicly.
Supplementary Material
The Supplementary Material for this article can be found online at: https://www.frontiersin.org/articles/10.3389/fimmu.2022.861885/full#supplementary-material
References
1. Dobson R, Giovannoni G. Multiple Sclerosis - A Review. Eur J Neurol (2019) 26:27–40. doi: 10.1111/ene.13819
2. International Multiple Sclerosis Genetics Consortium. Multiple Sclerosis Genomic Map Implicates Peripheral Immune Cells and Microglia in Susceptibility. Sci (New York NY) (2019) 365(6460):eaav7188. doi: 10.1126/science.aav7188
3. Roth GA, Forouzanfar MH, Moran AE, Barber R, Nguyen G, Feigin VL, et al. Demographic and Epidemiologic Drivers of Global Cardiovascular Mortality. N Engl J Med (2015) 372:1333–41. doi: 10.1056/NEJMoa1406656
4. Lennon RP, Claussen KA, Kuersteiner KA. State of the Heart: An Overview of the Disease Burden of Cardiovascular Disease From an Epidemiologic Perspective. Primary Care (2018) 45:1–15. doi: 10.1016/j.pop.2017.11.001
5. Jadidi E, Mohammadi M, Moradi T. High Risk of Cardiovascular Diseases After Diagnosis of Multiple Sclerosis. Multiple Sclerosis (Houndmills Basingstoke England) (2013) 19:1336–40. doi: 10.1177/1352458513475833
6. Christiansen CF, Christensen S, Farkas DK, Miret M, Sørensen HT, Pedersen L. Risk of Arterial Cardiovascular Diseases in Patients With Multiple Sclerosis: A Population-Based Cohort Study. Neuroepidemiology (2010) 35:267–74. doi: 10.1159/000320245
7. Palladino R, Marrie RA, Majeed A, Chataway J. Evaluating the Risk of Macrovascular Events and Mortality Among People With Multiple Sclerosis in England. JAMA Neurol (2020) 77:820–8. doi: 10.1001/jamaneurol.2020.0664
8. Tseng CH, Huang WS, Lin CL, Chang YJ. Increased Risk of Ischaemic Stroke Among Patients With Multiple Sclerosis. Eur J Neurol (2015) 22:500–6. doi: 10.1111/ene.12598
9. Marrie RA, Garland A, Schaffer SA, Fransoo R, Leung S, Yogendran M, et al. Traditional Risk Factors may Not Explain Increased Incidence of Myocardial Infarction in MS. Neurology (2019) 92:e1624–33. doi: 10.1212/WNL.0000000000007251
10. Roshanisefat H, Bahmanyar S, Hillert J, Olsson T, Montgomery S. Multiple Sclerosis Clinical Course and Cardiovascular Disease Risk - Swedish Cohort Study. Eur J Neurol (2014) 21:1353–e88. doi: 10.1111/ene.12518
11. Jakimovski D, Topolski M, Genovese AV, Weinstock-Guttman B, Zivadinov R. Vascular Aspects of Multiple Sclerosis: Emphasis on Perfusion and Cardiovascular Comorbidities. Expert Rev Neurotherapeut (2019) 19:445–58. doi: 10.1080/14737175.2019.1610394
12. Maillard P, Seshadri S, Beiser A, Himali JJ, Au R, Fletcher E, et al. Effects of Systolic Blood Pressure on White-Matter Integrity in Young Adults in the Framingham Heart Study: A Cross-Sectional Study. Lancet Neurol (2012) 11:1039–47. doi: 10.1016/S1474-4422(12)70241-7
13. Srinivasa RN, Rossetti HC, Gupta MK, Rosenberg RN, Weiner MF, Peshock RM, et al. Cardiovascular Risk Factors Associated With Smaller Brain Volumes in Regions Identified as Early Predictors of Cognitive Decline. Radiology (2016) 278:198–204. doi: 10.1148/radiol.2015142488
14. Petruzzo M, Reia A, Maniscalco GT, Luiso F, Lanzillo R, Russo CV, et al. The Framingham Cardiovascular Risk Score and 5-Year Progression of Multiple Sclerosis. Eur J Neurol (2021) 28:893–900. doi: 10.1111/ene.14608
15. Lawlor DA, Harbord RM, Sterne JA, Timpson N, Davey Smith G. Mendelian Randomization: Using Genes as Instruments for Making Causal Inferences in Epidemiology. Stat Med (2008) 27:1133–63. doi: 10.1002/sim.3034
16. Davey Smith G, Hemani G. Mendelian Randomization: Genetic Anchors for Causal Inference in Epidemiological Studies. Hum Mol Genet (2014) 23:R89–98. doi: 10.1093/hmg/ddu328
17. Hemani G, Zheng J, Elsworth B, Wade KH, Haberland V, Baird D, et al. The MR-Base Platform Supports Systematic Causal Inference Across the Human Phenome. eLife (2018) 7:e34408. doi: 10.7554/eLife.34408
18. Pierce BL, Ahsan H, Vanderweele TJ. Power and Instrument Strength Requirements for Mendelian Randomization Studies Using Multiple Genetic Variants. Int J Epidemiol (2011) 40:740–52. doi: 10.1093/ije/dyq151
19. Nikpay M, Goel A, Won HH, Hall LM, Willenborg C, Kanoni S, et al. A Comprehensive 1,000 Genomes-Based Genome-Wide Association Meta-Analysis of Coronary Artery Disease. Nat Genet (2015) 47:1121–30. doi: 10.1038/ng.3396
20. Nielsen JB, Thorolfsdottir RB, Fritsche LG, Zhou W, Skov MW, Graham SE, et al. Biobank-Driven Genomic Discovery Yields New Insight Into Atrial Fibrillation Biology. Nat Genet (2018) 50:1234–9. doi: 10.1038/s41588-018-0171-3
21. Shah S, Henry A, Roselli C, Lin H, Sveinbjörnsson G, Fatemifar G, et al. Genome-Wide Association and Mendelian Randomisation Analysis Provide Insights Into the Pathogenesis of Heart Failure. Nat Commun (2020) 11:163. doi: 10.1038/s41467-019-13690-5
22. Malik R, Chauhan G, Traylor M, Sargurupremraj M, Okada Y, Mishra A, et al. Multiancestry Genome-Wide Association Study of 520,000 Subjects Identifies 32 Loci Associated With Stroke and Stroke Subtypes. Nat Genet (2018) 50:524–37. doi: 10.1038/s41588-018-0058-3
23. Thompson JR, Minelli C, Del Greco MF. Mendelian Randomization Using Public Data From Genetic Consortia. Int J Biostatistics (2016) 12(2):/j/ijb.2016.12.issue-2/ijb-2015-0074/ijb-2015-0074.xml. doi: 10.1515/ijb-2015-0074
24. Burgess S, Bowden J, Fall T, Ingelsson E, Thompson SG. Sensitivity Analyses for Robust Causal Inference From Mendelian Randomization Analyses With Multiple Genetic Variants. Epidemiol (Cambridge Mass) (2017) 28:30–42. doi: 10.1097/EDE.0000000000000559
25. Bowden J, Davey Smith G, Haycock PC, Burgess S. Consistent Estimation in Mendelian Randomization With Some Invalid Instruments Using a Weighted Median Estimator. Genet Epidemiol (2016) 40:304–14. doi: 10.1002/gepi.21965
26. Bowden J. Davey Smith G and Burgess S. Mendelian Randomization With Invalid Instruments: Effect Estimation and Bias Detection Through Egger Regression. Int J Epidemiol (2015) 44:512–25. doi: 10.1093/ije/dyv080
27. Verbanck M, Chen CY, Neale B, Do R. Detection of Widespread Horizontal Pleiotropy in Causal Relationships Inferred From Mendelian Randomization Between Complex Traits and Diseases. Nat Genet (2018) 50:693–8. doi: 10.1038/s41588-018-0099-7
28. Greco MF, Minelli C, Sheehan NA, Thompson JR. Detecting Pleiotropy in Mendelian Randomisation Studies With Summary Data and a Continuous Outcome. Stat Med (2015) 34:2926–40. doi: 10.1002/sim.6522
29. Brion MJ, Shakhbazov K, Visscher PM. Calculating Statistical Power in Mendelian Randomization Studies. Int J Epidemiol (2013) 42:1497–501. doi: 10.1093/ije/dyt179
30. Manouchehrinia A, Tanasescu R, Tench CR, Constantinescu CS. Mortality in Multiple Sclerosis: Meta-Analysis of Standardised Mortality Ratios. J Neurol Neurosurg Psychiatry (2016) 87:324–31. doi: 10.1136/jnnp-2015-310361
31. Tavallaei MJ, Tavallaei AH, Ebrahimi N, Ghoshouni H, Afshari-Safavi A, Badihian S, et al. The Prevalence of Myocardial Infarction Among Multiple Sclerosis Patients: A Systematic Review and Meta-Analysis. Multiple Sclerosis Related Disord (2021) 56:103292. doi: 10.1016/j.msard.2021.103292
32. Rapp D, Michels S, Schöpe J, Schwingshackl L, Tumani H, Senel M, et al. Associations Between Multiple Sclerosis and Incidence of Heart Diseases: Systematic Review and Meta-Analysis of Observational Studies. Multiple Sclerosis Related Disord (2021) 56:103279. doi: 10.1016/j.msard.2021.103279
33. Lavela SL, Prohaska TR, Furner S, Weaver FM. Chronic Diseases in Male Veterans With Multiple Sclerosis. Preventing Chronic Dis (2012) 9:E55. doi: 10.5888/pcd9.110121
34. Thormann A, Magyari M, Koch-Henriksen N, Laursen B, Sørensen PS. Vascular Comorbidities in Multiple Sclerosis: A Nationwide Study From Denmark. J Neurol (2016) 263:2484–93. doi: 10.1007/s00415-016-8295-9
35. Marrie RA, Fisk J, Tremlett H, Wolfson C, Warren S, Blanchard J. Differing Trends in the Incidence of Vascular Comorbidity in MS and the General Population. Neurol Clin Practice (2016) 6:120–8. doi: 10.1212/CPJ.0000000000000230
36. Peng H, Wu X, Wen Y, Lin J, Guan W. Myocardial Infarction and Stroke Risks in Multiple Sclerosis Patients: A Two-Sample Mendelian Randomization Study. Multiple Sclerosis Related Disord (2022) 58:103501. doi: 10.1016/j.msard.2022.103501
37. Ross R. Atherosclerosis–An Inflammatory Disease. New Engl J Med (1999) 340:115–26. doi: 10.1056/NEJM199901143400207
38. Ramaglia V, Rojas O, Naouar I, Gommerman JL. The Ins and Outs of Central Nervous System Inflammation-Lessons Learned From Multiple Sclerosis. Annu Rev Immunol (2021) 39:199–226. doi: 10.1146/annurev-immunol-093019-124155
39. Yang R, Dunn JF. Multiple Sclerosis Disease Progression: Contributions From a Hypoxia-Inflammation Cycle. Multiple Sclerosis (Houndmills Basingstoke England) (2019) 25:1715–8. doi: 10.1177/1352458518791683
40. Bergh C, Udumyan R, Fall K, Nilsagård Y, Appelros P, Montgomery S. Stress Resilience in Male Adolescents and Subsequent Stroke Risk: Cohort Study. J Neurol Neurosurg Psychiatry (2014) 85:1331–6. doi: 10.1136/jnnp-2013-307485
41. Mostofsky E, Penner EA, Mittleman MA. Outbursts of Anger as a Trigger of Acute Cardiovascular Events: A Systematic Review and Meta-Analysis. Eur Heart J (2014) 35:1404–10. doi: 10.1093/eurheartj/ehu033
42. Ewanchuk BW, Gharagozloo M, Peelen E, Pilutti LA. Exploring the Role of Physical Activity and Exercise for Managing Vascular Comorbidities in People With Multiple Sclerosis: A Scoping Review. Multiple Sclerosis Related Disord (2018) 26:19–32. doi: 10.1016/j.msard.2018.08.022
43. Frantz S, Ertl G, Bauersachs J. Mechanisms of Disease: Toll-Like Receptors in Cardiovascular Disease. Nat Clin Pract Cardiovasc Med (2007) 4:444–54. doi: 10.1038/ncpcardio0938
44. McLaren JE, Michael DR, Ashlin TG, Ramji DP. Cytokines, Macrophage Lipid Metabolism and Foam Cells: Implications for Cardiovascular Disease Therapy. Prog Lipid Res (2011) 50:331–47. doi: 10.1016/j.plipres.2011.04.002
45. Kovarik JM, Lu M, Riviere GJ, Barbet I, Maton S, Goldwater DR, et al. The Effect on Heart Rate of Combining Single-Dose Fingolimod With Steady-State Atenolol or Diltiazem in Healthy Subjects. Eur J Clin Pharmacol (2008) 64:457–63. doi: 10.1007/s00228-007-0448-4
46. Feige J, Schernthaner C, Wipfler P, Sellner J. Delayed High-Grade Atrioventricular Block Requiring Pacemaker Implantation in a Multiple Sclerosis Patient Treated With Fingolimod. Multiple Sclerosis Related Disord (2020) 38:101515. doi: 10.1016/j.msard.2019.101515
47. Aksungar FB, Topkaya AE, Yildiz Z, Sahin S, Turk U. Coagulation Status and Biochemical and Inflammatory Markers in Multiple Sclerosis. J Clin Neurosci Off J Neurosurg Soc Australasia (2008) 15:393–7. doi: 10.1016/j.jocn.2007.02.090
48. Ramsaransing GS, Fokkema MR, Teelken A, Arutjunyan AV, Koch M, De Keyser J, et al. Plasma Homocysteine Levels in Multiple Sclerosis. J Neurol Neurosurg Psychiatry (2006) 77:189–92. doi: 10.1136/jnnp.2005.072199
49. Russo C, Morabito F, Luise F, Piromalli A, Battaglia L, Vinci A, et al. Hyperhomocysteinemia is Associated With Cognitive Impairment in Multiple Sclerosis. J Neurol (2008) 255:64–9. doi: 10.1007/s00415-007-0668-7
50. Minagar A, Jy W, Jimenez JJ, Sheremata WA, Mauro LM, Mao WW, et al. Elevated Plasma Endothelial Microparticles in Multiple Sclerosis. Neurology (2001) 56:1319–24. doi: 10.1212/WNL.56.10.1319
51. Senzaki K, Okada Y, Ochi H, Ochi M, Takei SI, Miura S, et al. Vascular Endothelial Dysfunction Associated With Severity in Multiple Sclerosis. Multiple Sclerosis Related Disord (2021) 54:103135. doi: 10.1016/j.msard.2021.103135
Keywords: multiple sclerosis, cardiovascular disease, Mendelian randomization, genome-wide association studies, causal association
Citation: Yang F, Hu T, He K, Ying J and Cui H (2022) Multiple Sclerosis and the Risk of Cardiovascular Diseases: A Mendelian Randomization Study. Front. Immunol. 13:861885. doi: 10.3389/fimmu.2022.861885
Received: 25 January 2022; Accepted: 21 February 2022;
Published: 15 March 2022.
Edited by:
Marcello Moccia, University of Naples Federico II, ItalyReviewed by:
Gorica Maric, University of Belgrade, SerbiaRaffaele Palladino, University of Naples Federico II, Italy
Omid Mirmosayyeb, Isfahan University of Medical Sciences, Iran
Copyright © 2022 Yang, Hu, He, Ying and Cui. This is an open-access article distributed under the terms of the Creative Commons Attribution License (CC BY). The use, distribution or reproduction in other forums is permitted, provided the original author(s) and the copyright owner(s) are credited and that the original publication in this journal is cited, in accordance with accepted academic practice. No use, distribution or reproduction is permitted which does not comply with these terms.
*Correspondence: Hanbin Cui, aGJjdWlfbmJkeXl5QG91dGxvb2suY29t
†These authors have contributed equally to this work