- 1Immunogenetics Laboratory, Institute of Biomedicine, University of Turku, Turku, Finland
- 2Institute of Biomedicine, Research Centre for Integrative Physiology and Pharmacology, and Centre for Population Health Research, University of Turku, Turku, Finland
- 3Department of Paediatrics, University of Turku and Turku University Hospital, Turku, Finland
- 4Pediatric Research Center, Children’s Hospital, University of Helsinki and Helsinki University Hospital, Helsinki, Finland
- 5Research Program for Clinical and Molecular Metabolism, Faculty of Medicine, University of Helsinki, Helsinki, Finland
- 6Tampere Center for Child Health Research, Tampere University Hospital, Tampere, Finland
- 7Department of Paediatrics, PEDEGO Research Unit, Medical Research Center, University of Oulu, Oulu, Finland
- 8Department of Children and Adolescents, Oulu University Hospital, Oulu, Finland
- 9Clinical Microbiology, Turku University Hospital, Turku, Finland
The non-HLA loci conferring susceptibility to type 1 diabetes determine approximately half of the genetic disease risk, and several of them have been shown to affect immune-cell or pancreatic β-cell functions. A number of these loci have shown associations with the appearance of autoantibodies or with progression from seroconversion to clinical type 1 diabetes. In the current study, we have re-analyzed 21 of our loci with prior association evidence using an expanded DIPP follow-up cohort of 976 autoantibody positive cases and 1,910 matched controls. Survival analysis using Cox regression was applied for time periods from birth to seroconversion and from seroconversion to type 1 diabetes. The appearance of autoantibodies was also analyzed in endotypes, which are defined by the first appearing autoantibody, either IAA or GADA. Analyzing the time period from birth to seroconversion, we were able to replicate our previous association findings at PTPN22, INS, and NRP1. Novel findings included associations with ERBB3, UBASH3A, PTPN2, and FUT2. In the time period from seroconversion to clinical type 1 diabetes, prior associations with PTPN2, CD226, and PTPN22 were replicated, and a novel association with STAT4 was observed. Analyzing the appearance of autoantibodies in endotypes, the PTPN22 association was specific for IAA-first. In the progression phase, STAT4 was specific for IAA-first and ERBB3 to GADA-first. In conclusion, our results further the knowledge of the function of non-HLA risk polymorphisms in detailing endotype specificity and timing of disease development.
Introduction
The HLA region on chromosome 6p21 is the major genetic risk factor for developing type 1 diabetes (T1D), and it confers around 50% of the overall genetic risk. The rest of the genetic risk is distributed among several loci throughout the genome. So far, at least 57 non-HLA T1D susceptibility regions have been confirmed (1), and the most recent study reported 78 genome-wide-significant regions (2). Many of the T1D risk associated genes affect immune cell or pancreatic β-cell functions (3).
Clinical T1D is preceded by a subclinical period during which β-cell destruction appears and impaired glucose tolerance emerges. The duration of this period varies strongly from weeks to years (4). The emergence of pre-diabetes and β-cell autoimmunity is marked by the emergence of the first islet-specific autoantibodies (AAB), of which those targeting insulin (IAA), glutamic acid decarboxylase 65 (GADA), and islet antigen-2 (IA-2A) are best characterized and can be used as markers of increased disease risk (5). IAA or GADA are most often found as the first appearing AAB and they at least partially seem to be associated with different mechanisms in the pathogenesis of T1D (known also as endotypes) (6). Advanced autoimmunity is indicated by the spreading of autoimmunity to cover at least two β-cell autoantigens. Many factors, including age, HLA class II genotypes, and certain non-HLA gene polymorphisms, affect the disease initiation, whereas other factors, including HLA class I genes, some non-HLA gene polymorphisms, and age at AAB seroconversion, are associated with enhanced progression to clinical T1D (3).
The Finnish Type 1 Diabetes Prediction and Prevention (DIPP) study is a birth cohort study, in which children are followed up from birth and monitored for the appearance of β-cell autoimmunity and the development of clinical T1D. We previously reported a survival analysis on 39 SNP markers from 37 T1D susceptibility loci in 521 persistently AAB-positive cases and 989 matched controls from the DIPP study (7). In this analysis, we set out to re-examine these prior findings by using the added power of the expanded cohort of 976 children with persistent AABs and 1910 matched controls. Altogether, 21 loci with prior evidence of association in the previous survival study (7) or in a family-based association study in the Finnish population (8) were chosen for analysis with the expanded cohort.
Materials and Methods
Subjects
All study subjects were participants in the Finnish Diabetes Prediction and Prevention Study (DIPP) (9), in which newborn children born in Turku, Oulu, and Tampere university hospitals are screened for HLA-conferred genetic risk for developing T1D. Both the original selection process, based on the typing of T1D-risk HLA-DQB1*03:02 and DQB1*02 and protection associated with DQB1*03:01 and DQB*06 02/3 alleles (10), and the development of the screening protocol during various phases of the study to higher sensitivity and specificity, including “full-house” HLA-DQB1 typing and selected HLA-DQA1 and HLA-DRB1 alleles (11), have been presented in detail before. Children with selected T1D-predisposing HLA genotypes are invited to a prospective follow-up study and subsequently screened for the appearance of AAB at regular clinic visits at 3–12-month intervals, depending on the age of the child and AAB positivity (10). In the original study protocol, children were first monitored for the appearance of islet cell antibodies (ICA) and, if testing positive, the biochemical AAB to insulin (IAA), glutamic acid decarboxylase 65 (GADA), and islet antigen‐2 (IA–2A) were analyzed from all available samples from such participants. Since 2003, all children have been tested for all four AAB at each clinical visit, as well as 1,006 children born between November 1994 and July 1997 (12). Progression to T1D was diagnosed according to the WHO criteria. The study was approved by the local ethics committee and written informed consent was obtained from the parents of the participating children.
The study cohort comprised 976 case subjects with β-cell autoimmunity, defined by having at least one persistently positive AAB (IAA, GADA, or IA–2A), and 1,910 AAB negative, clinically healthy control subjects. Controls were matched according to cases for sex, study center, and birth date (a range of two months before or after birth was included for matching) (Table 1). During the follow-up period, 560 cases developed positivity for multiple AABs and altogether 426 subjects progressed to T1D. The median age (interquartile range) was 10.47 (5.98–14.97) years in all cases. The median ages at seroconversion and for progression to clinical T1D (interquartile ranges) were 3.30 (1.49–5.11) years and 6.75 (3.68–9.82) years, respectively. The median follow-up time (interquartile range) for signs of autoimmunity and for developing clinical T1D was 10.83 (6.65–15.01) years in the controls. More details on the study subjects are presented in Table S1.

Table 1 Number of AAB positive cases and their matched controls in complete cohort (A) and endotype specific subgroups (B).
Genotyping
DNA for all study subjects was extracted from EDTA blood using salt extraction. For the new data set, the bulk of single-nucleotide polymorphisms (SNP) were genotyped at the Finnish Functional Genomics Centre core facility (Turku, Finland) using the Taqman OpenArray® real-time PCR platform with QuantStudio 12K Flex Real-Time PCR System (Thermo Fisher Scientific, Waltham, Massachusetts, USA). Two SNPs (CD226 rs763361, NRP1 rs2666236) were genotyped using Taqman® SNP genotyping Assays in QuantStudio™ 3 Real-Time PCR System (Thermo Fisher Scientific, Waltham, Massachusetts, USA). Only original Thermo Fisher reagents (assay and mastermix) were used in both Taqman SNP genotyping methods used. SNP genotyping for the previous data set of 521 cases and 989 controls, which were included in the current data set, was performed using the Sequenom (San Diego, California, USA) platform in the Genome Centre of Eastern Finland, University of Eastern Finland, Kuopio (7). Alleles were called using either QuantStudio™ Design and Analysis Desktop Software (QuantStudio 3 SNP analyses) or ThermoFisher Connect™ cloud Genotyping app (OpenArray SNP analyses).
There were no genotyping quality control issues after the subjects with subpar qPCR amplification were removed from the analysis. Genotyping success rate for all SNPs was high (>0.98) throughout the sample collection and none of the SNPs exhibited significant deviation from Hardy–Weinberg equilibrium (HWE) (p <0.05) in the control population.
The HLA DR-DQ haplotypes were determined using sequence-specific oligonucleotide probes as described earlier (13). Based on their HLA genotypes, the study participants were divided into four risk groups: strongly increased risk, moderately increased risk, slightly increased risk and a combined group for neutral or protective genotypes (13).
Allele frequencies for the SNPs under study were derived from the gnomAD v.2.1.1 European (Finnish) (14).
Autoantibody Analysis
The protocols for determining ICA and biochemical AABs (IAA, GADA, and IA–2A) have been described earlier (15, 16).
Statistical Analysis
Statistical analyses were conducted using the IBM SPSS Statistics 25.0 software package (Armonk, NY, USA). A Cox proportional hazards model was used to assess the effect of SNPs from birth to the emergence of β-cell autoimmunity and progression to T1D and from the time of seroconversion to clinical T1D. In addition to the main analysis with all case subjects, the same analyses were conducted separately in two subgroups of cases with either IAA or GADA as the first solely observed AAB at the seroconversion.
Both recessive and dominant inheritance models were tested, and the model showing the higher significance was selected for presentation (in Table 2) in each analysis category. Testing additive model was omitted since it does not show the effect size or direction for the tested genotype. The models were adjusted for HLA-conferred genetic risk by including the risk groups in the model. Resulting P-values were corrected for multiple testing by the number of SNPs being studied in each category using the Benjamini and Hochberg false discovery rate (FDR) step-up procedure (α = 0.05) (17) incorporated in the R package multtest (Katherine S. Pollard, Houston N. Gilbert, Yongchao Ge, Sandra Taylor, and Sandrine Dudoit. multtest: resampling-based multiple hypothesis testing, r package version 2.46.0.). A corrected P-value of <0.05 was considered statistically significant in the survival analysis. Genotyping success rate analysis for all study subjects and HWE calculations in the control population were performed using the PLINK 1.07 software package (URL: http://pngu.mgh.harvard.edu/purcell/plink/) (18).
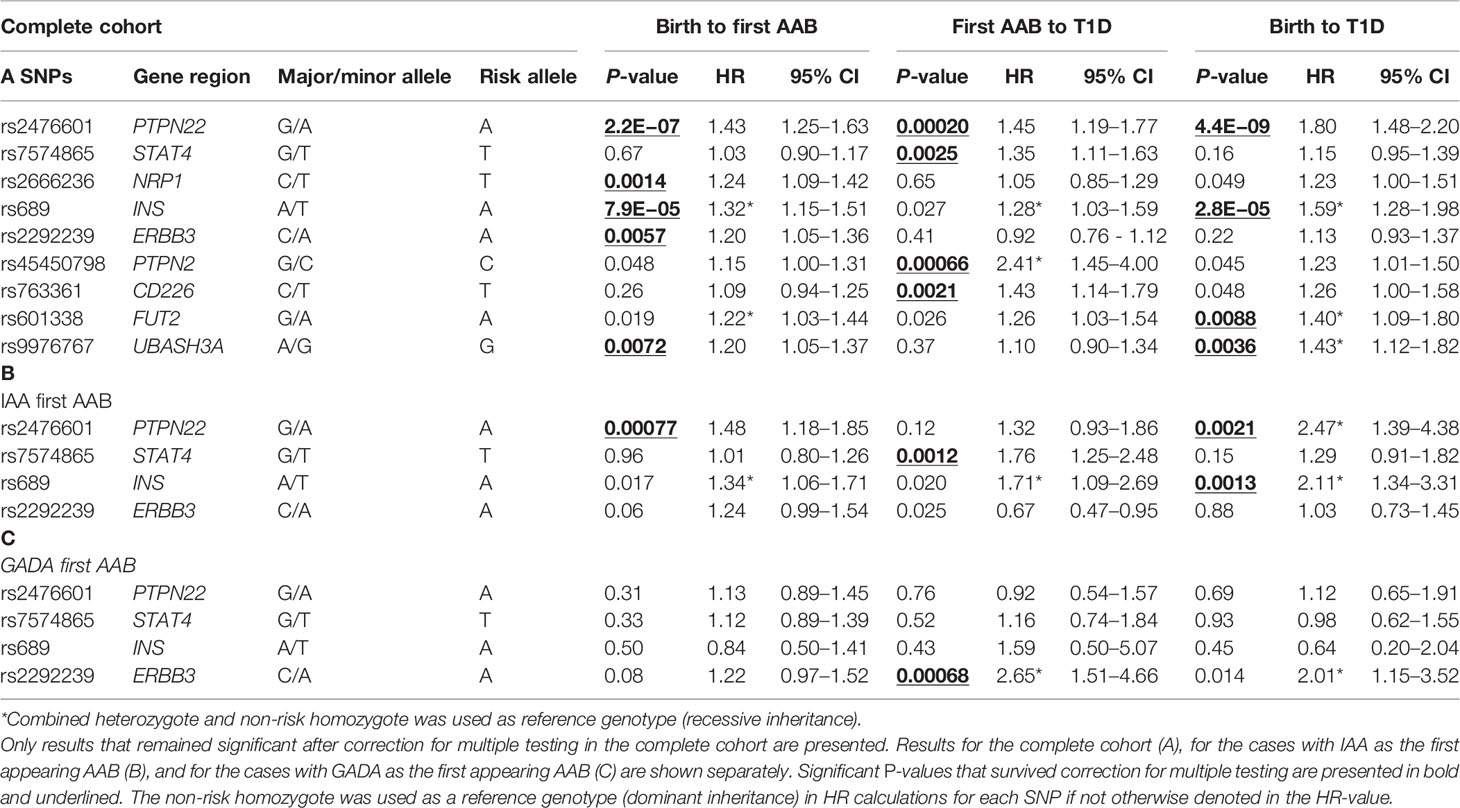
Table 2 Cox regression survival analysis hazard ratios and corresponding P-values are shown for the time periods from birth to the appearance of the first AAB, from the first AAB to a clinical T1D diagnosis, and from birth to clinical T1D diagnosis.
Results
All the SNPs being studied (Table 3) were established risk markers of T1D. The more particular aim of this follow-up study was to determine whether some markers were specifically associated with the early phase of autoimmunity development or its progression to a clinical diagnosis of T1D and whether they were specific for endotypes characterized by either IAA or GADA as the first autoantibody to appear.
Table 2 contains results for the loci that have shown a significant association at least in one tested time period within either the full data set (A) or the IAA-first (B) or GADA-first (C) subsets. Complete results for all tested loci are shown in Table S2. Survival analysis of the entire follow-up period from birth to clinical T1D using multivariate Cox regression demonstrated significant associations with PTPN22, INS, FUT2, and UBASH3A (p = 4.4E−9, 2.8E−5, 0.0088, and 0.0036, respectively) (Table 2A). The known risk allele was associated with increased disease development in all loci.
Effects of Non-HLA Gene Polymorphisms in Different Phases of Autoimmunity Development
PTPN22, INS, and UBASH3A also demonstrated a significant association (after correction for multiple testing) with the appearance of the first islet-specific AAB when followed from birth (p = 2.2E−07, 7.9E−05, and 0.0072, respectively), but FUT2 was not significant after correction for multiple testing (p = 0.019). Additionally, ERBB3 and NRP1, which did not show an association in the analysis from birth to T1D, presented significant associations with the appearance of the first islet-specific AAB (p = 0.0057 and 0.0014, respectively).
When the further period from seroconversion to clinical T1D was analyzed, four loci presented significant associations (PTPN22, PTPN2, STAT4, and CD226, p = 0.00020, 0.00066, 0.0025, and 0.0021, respectively). In all these loci, the known risk allele increased progression to diabetes. Of these four loci, PTPN22 was the only one detected in the analysis of the whole period from birth to T1D.
The Associations of Non-HLA SNPs With Disease Pathogenesis in Relation to Disease Endotypes
To explore the genetic heterogeneity of disease endotypes, the cases and respective controls were analyzed separately based on the initial appearing AAB (IAA or GADA). When the whole follow-up period from birth to clinical T1D was studied, significant associations were observed with PTPN22 and INS in the IAA-first group (p = 0.0021 and 0.0013, respectively) (Table 2B).
When the follow-up period from birth to AAB seroconversion was analyzed, PTPN22 was the only locus significantly associated with the IAA-first group (p = 0.00077). In the progression from AAB positivity to clinical T1D in the IAA subgroup, a significant association of STAT4 with disease risk was observed (p = 0.0012).
When follow-up periods from birth to clinical T1D or from birth to AAB seroconversion were studied in the subgroup with GADA as the first AAB, no significant association was detected. In the progression from AAB positivity to clinical diabetes, ERBB3 showed a significant association in this subgroup (p = 0.00068) (Table 2C).
An interesting, albeit non-significant observation, was the opposite direction of ERBB3 and FUT2 effects in the progression phase of the IAA-first vs. GADA-first subgroups. The risk alleles of both loci showed association with a protective effect in the IAA-first subgroup (HR = 0.67, HR = 0.60, respectively), and risk effect in the GADA-first subgroup (HR = 2.65, HR = 1.89, respectively), but these observations were not significant after correction for multiple testing except for the ERBB3 GADA-first association (p = 0.00068) (Table S2).
Discussion
The number of Finnish DIPP follow-up study subjects with persisting autoimmunity analyzed here has almost doubled since our previous report on non-HLA loci affecting initiation and development of T1D-specific autoimmunity (7). The increased statistical power of the expanded data set allowed new findings on the effect of non-HLA gene polymorphisms in various phases of the autoimmune process and in different endotypes of the autoimmune process leading to clinical T1D.
As all gene polymorphisms being studied here are confirmed T1D risk genes, it is conceivable that they affect survival from birth to clinical T1D. Therefore, the lack of such evidence in this analysis could plausibly demonstrate insufficient power to detect small effects, and in fact, when the follow-up period from birth to clinical T1D was examined, most of the polymorphisms under study did not show an association. The effect locus conveys on disease susceptibility can be restricted to either the initiation of autoimmunity or the spreading of autoimmunity, which in part may decrease the power to detect significant association signals in the entire follow-up period. For that reason, the main objective of the survival analysis in the whole cohort was to examine whether the genes studied exert their effects in the phase of islet autoimmunity initiation or in the progression from AAB positivity to clinical disease. We defined the progression period from the first AAB seroconversion to clinical diabetes to involve both the spreading of autoimmunity and the β-cell destruction, and therefore the risk effect has the potential to be mediated by different mechanisms.
The analysis of the time period from birth to the appearance of the first AAB replicated associations in our earlier study (7) with PTPN22 and INS. The prior suggestive association (that did not survive the correction for multiple testing) observed for NRP1, was now confirmed as a significant association. On the other hand, the prior significant association evidence found with IFIH1 was now reduced to a suggestive association (Table S2). Novel findings in the current study were significant associations between the appearance of AABs and ERBB3, NRP1, and UBASH3A. Three of these loci have shown an association also in other studies: PTPN22 and INS in TEDDY (19), and PTPN22 and UBASH3A in DAISY (20). IFIH1 has been reported to be associated with progression from AAB positivity (20, 21), but we did not observe such an association in our cohort.
We have found association evidence earlier for IL2RA, CD226, PTPN2, LOC646538, and PTPN22 in the analysis of the time period from AAB positivity to clinical T1D (7, 22). The previous significant association with PTPN2 was replicated in this study and earlier suggestive evidence with PTPN22 and CD226 was now confirmed with significant associations. A significant association with progression from seroconversion to clinical T1D and STAT4 was a novel observation.
The discovery of specific gene effects in separate analyses of endotypes, characterized by autoimmunity initiated with either IAA or GADA as the first AAB, is expected to produce important information on the pathogenetic mechanisms (6). Novel, endotype-specific significant associations were also found in this study. As in the whole cohort, PTPN22 demonstrated the strongest association with the development of autoimmunity in the subgroup, with IAA as the first AAB. However, in the GADA-first group, no significant effect was seen, unlike in the TEDDY study (23). As was the case in our earlier study and the TEDDY study, INS was associated with the IAA-first endotype (7, 22). In the combined cohort for AAB appearance, we could not replicate the SH2B3 association observed in TEDDY (24). The other known T1D risk gene, TNFAIP3, which in TEDDY was associated with progression to clinical disease, (25) did not show any association in the current study.
The progression from AAB positivity to clinical T1D is connected with the decreasing ability of β-cells to produce insulin, and its rate is associated with alleles in class I HLA genes (26, 27) and with several genes outside the HLA region. A significantly steeper decrease in the first-phase insulin response was observed during follow-up in AAB-positive DIPP children in an earlier study with risk genotypes of CTSH, PTPN2, IKZF4, and FUT2 genes (28). PTPN2 and ERBB3 (which is in tight linkage disequilibrium with IKZF4) showed a significant association with progression from seroconversion to clinical disease in the current study, but the associations with ERBB3 (Table 2A) and FUT2 were seen only in the GADA-first group, and the FUT2 association was only suggestive (Table S2).
With a few exceptions, the mechanistic effects of T1D risk genes are largely unknown (3). PTPN22 encodes protein tyrosine phosphatase, which acts as a negative regulator of T-cell receptor signaling. Its variant rs2476601, which causes a missense R620W mutation, increases the risk of multiple autoimmune diseases and is associated with a multitude of cell population-specific effects, some of which are contradictory. For example, increased T-effector activation has been reported (29), but also a compensatory increased frequency of naive regulatory T cells, important, in suppression of autoimmunity (30, 31). This polymorphism also modulates multiple other pathways, including B-cell and Toll-like receptors, NLRP3, and integrin signaling (3). It therefore seems likely that these multiple effects may affect both the initiation and progression phases of the disease. Our data also support a model where rs2476601 has multiple effects in different cell populations throughout disease pathogenesis.
In the current data, STAT4 was significantly associated with the progression from seroconversion to clinical T1D, specifically among IAA-first subjects. This gene is involved in signal transduction and activates transcription in response to proinflammatory cytokines (32, 33). Additionally, it participates in T helper 1 cell regulation and is expressed in activated monocytes, dendritic cells, and macrophages, and its inhibition in the NOD mouse model prevents autoimmune diabetes (32). A meta-analysis of the significance of STAT4 rs7574865 T1D risk allele T has confirmed its association with T1D in multiple populations, in addition to the already earlier found association with other autoimmune diseases (34). A recent study also reported a higher STAT4 expression level in peripheral blood mononuclear cells in T1D cases compared to controls and an association of the rs7574865 T allele with younger age at the T1D diagnosis (35). The finding of a young age at diagnosis in risk allele positive children concurs with our finding of rs7574865 risk allele T increasing development of AABs and with the association of IAA as the first AAB because IAA dominates over GADA as the first AAB among the youngest seroconverted children, which is also reflected in a younger age at diagnosis (36).
Strong associations of NRP1 and UBASH3A with AAB induction were seen for the first time in the DIPP cohort in this study (7). The NRP1 gene encodes neuropilin 1, which is a co-receptor for vascular endothelial growth factor (VEGF) and is essential for its binding and activity in endothelial cells, including pancreatic islets as well. Various isoforms have been implicated in affecting β-cell regeneration (37). The effects of NRP1 polymorphism on the large spectrum of VEGF functions are, however, still largely unknown. T1D associated SNP alleles in UBASH3A increase its expression in primary human CD4+ T cells and inhibit NF-κB signaling, resulting in reduced IL-2 production, which might increase the probability of initiating autoimmunity (38).
The ERBB3 risk allele is associated with the appearance of AABs in the whole cohort in our study. The hazard ratio (HR) for the whole cohort is very similar to the HR observed in both the IAA-first and GADA-first subgroups, although it does not quite reach significance in smaller subgroups. However, when the period from seroconversion to clinical T1D is observed, the ERBB3 risk allele A shows effects in opposite directions between the IAA-first and GADA-first subgroups, with a significant risk effect in the GADA-first group and a protective suggestive effect (non-corrected p <0.05) in the IAA-first subgroup. The whole cohort does not show an association with progression to T1D, probably because the contradictory effects in the two subgroups neutralize each other. It thus seems possible that ERBB3 rs2292239 or the region around it is associated with at least two different effector mechanisms. The 12q13 region includes a group of genes with very strong linkage disequilibrium and, e.g., IKZF4 and ERBB3 risk alleles both have a very similar effect on T1D susceptibility (8, 39), but independent SNP associations have also been detected in this region (39). A gene expression study of six genes in the region demonstrated a higher expression of ERBB3 on antigen-presenting cells after LPS, poly C, or CpG stimulation. The study also observed a higher proportion of ERBB3 positive monocytes and dendritic cells, and the proportion of these cells correlated with the ability of antigen-presenting cells to induce T-cell proliferation (40). However, the exact mechanism of how these differences would affect the risk of T1D remains unclear. Interestingly, a recent paper from the Belgian Diabetes Registry described that ERBB3/IKZF4 risk alleles increased the progression from single to multiple AABs, but this was seen only in female relatives (41). Alleged multiple independent effects emphasize the possibility of the importance of the IKZF4 gene, which encodes the Eos molecule, which in turn in mouse models seems to be important for T-reg cell functions and suppression of autoimmunity (42, 43).
CD226 was significantly associated with the progression from seroconversion to clinical T1D. Neither IAA- nor GADA-first subjects showed a significant association, suggesting that the result of the entire cohort contributes to both IAA and GADA-initiated disease pathways. The HR effect size was similar in both groups to that observed in the whole cohort. CD226 is a co-stimulatory molecule known to augment effector/memory T cell and NK cell activation, and the disease-associated variant may promote the MARK/ERK pathway and thus enhance the production of inflammatory and cytotoxic molecules (3). It might therefore play a major role in the phase of β-cell destruction by immune cells. Similar to CD226, also PTPN2 was strongly associated with progression from seroconversion to clinical T1D, but not with AAB induction or with IAA or GADA associated disease. This is in accordance with its role in sensitization of pancreatic β-cells to interferon-induced apoptosis (44).
We were able to validate most of our earlier observations in this expanded follow-up study and found new associations in some genes, suggesting that they affect either specific stages of the development of T1D and/or one of the two major endotypes characterized by either IAA or GADA as the first AAB. Many of these findings are in agreement with reports from other follow-up studies, whereas others await confirmation. Differences between participating populations and study protocols may explain differences in identified associations, e.g., between the international TEDDY study and the Finnish DIPP study. Children eligible for the TEDDY study from the general population represented a few high and moderate risk HLA genotypes, covering together around 42% of Finnish children with T1D, whereas the DIPP study recruited a larger follow-up group, covering 60–70% of the genotypes found in T1D children, including also lower HLA risk genotypes. A higher frequency of non-HLA gene risk genotypes like INS has actually been found in children with T1D, whose HLA genotypes are associated with a relatively low T1D risk (45, 46). There are also differences in the definitions of various stages of autoimmunity between studies. We analyzed the progression from the appearance of the first AAB to the diagnosis of clinical diabetes, whereas the TEDDY study analyzed the progression from the appearance of multiple AABs to the diagnosis, which is a shorter period (25).
In addition to sample size restrictions, there are other limitations to the study. The progress to autoantibody positivity and to the clinical diagnosis has been found to be associated with high genetic risk scores combining HLA and non-HLA markers (47) and it might be useful in the future to somehow adjust for the individual genetic risk. However, the recruitment criterion for the DIPP study is defined by the HLA class II genotype associated with high or moderate T1D risk. The appearance of islet autoantibodies, which is a prerequisite for the development of the disease, is mainly associated with high-risk class II HLA genotypes (48). In conclusion, our results contribute to a more specific analysis of the function of non-HLA T1D risk polymorphisms by detailing disease endotype specificity and timing of the disease process. The numbers of participants in follow-up cohorts who have developed AABs and especially T1D, are limited and one can expect only the strongest associations to turn out to be significant although the numbers of AAB-positive subjects and diagnosed T1D cases, are as far as we know, higher than ever published earlier in follow-up studies of this type. A deeper understanding of the mechanisms through which risk effects are mediated will increase our understanding of the development of T1D and potentially open new avenues to better treatments and even disease prevention.
Data Availability Statement
The datasets presented in this article are not readily available because of data privacy regulations. Requests to access the datasets should be directed to DIPP Steering Committee,am9oYW5uYS5sZW1wYWluZW5AdXR1LmZp.
Ethics Statement
The studies involving human participants were reviewed and approved by The Ethics Committees at the three participating University Hospitals, Turku, Oulu, and Tampere. Written informed consent to participate in this study was provided by the participants’ legal guardian/next of kin. Written informed consent was obtained from the individual(s), and minor(s)’ legal guardian/next of kin, for the publication of any potentially identifiable images or data included in this article.
Author Contributions
A-PL, MV, JI, and JL designed the experiments. The manuscript was written by A-PL, MV, JI, and JL. A-PL, MV, JI, JT, MK, JL, and RV contributed in reviewing and editing the article. A-PL, MV, JI, and JL designed the study and drafted the manuscript. A-PL and MV performed the experiments and analyzed the data. A-PL, JI, JT, MK, and RV acquired the data and critically reviewed the manuscript. A-PL is the guarantor of this work and, as such, had full access to all the data in the study and takes responsibility for the integrity of the data and the accuracy of the data analysis. All authors listed have made a substantial, direct, and intellectual contribution to the work and approved it for publication.
Funding
This work was funded by the Sigrid Jusélius Foundation, the Finnish Medical Foundation, the Juvenile Diabetes Research Foundation (JDRF), grant number 3-SRA-2020-955-S-B and the Academy of Finland grant number 286765.
Conflict of Interest
The authors declare that the research was conducted in the absence of any commercial or financial relationships that could be construed as a potential conflict of interest.
Publisher’s Note
All claims expressed in this article are solely those of the authors and do not necessarily represent those of their affiliated organizations, or those of the publisher, the editors and the reviewers. Any product that may be evaluated in this article, or claim that may be made by its manufacturer, is not guaranteed or endorsed by the publisher.
Acknowledgments
The authors thank all the DIPP project staff and families for participating in the study. We would also like to thank statistician Bernd Pape for his help in designing the statistical methods used in this study. We also give special thanks to Ritva Suominen, Mia-Maria Karlsson, Piia Nurmi, and Terhi Laakso for their skillful technical assistance.
Supplementary Material
The Supplementary Material for this article can be found online at: https://www.frontiersin.org/articles/10.3389/fimmu.2022.909020/full#supplementary-material
References
1. Onengut-Gumuscu S, Chen W, Burren O, Cooper NJ, Quinlan AR, Mychaleckyj JC, et al. Fine Mapping of Type 1 Diabetes Susceptibility Loci and Evidence for Colocalization of Causal Variants With Lymphoid Gene Enhancers. Nat Genet (2015) 47:381–6. doi: 10.1038/ng.3245
2. Robertson CC, Inshaw JRJ, Onengut-Gumuscu S, Chen W, Santa Cruz DF, Yang H, et al. Fine-Mapping, Trans-Ancestral and Genomic Analyses Identify Causal Variants, Cells, Genes and Drug Targets for Type 1 Diabetes. Nat Genet (2021) 53:962–71. doi: 10.1038/s41588-021-00880-5
3. Shapiro MR, Thirawatananond P, Peters L, Sharp RC, Ogundare S, Posgai AL, et al. De-Coding Genetic Risk Variants in Type 1 Diabetes. Immunol Cell Biol (2021) 99:496–508. doi: 10.1111/imcb.12438
4. Steck AK, Johnson K, Barriga KJ, Miao D, Yu L, Hutton JC, et al. Age of Islet Autoantibody Appearance and Mean Levels of Insulin, But Not GAD or IA-2 Autoantibodies, Predict Age of Diagnosis of Type 1 Diabetes. Diabetes Care (2011) 34:1397–9. doi: 10.2337/dc10-2088
5. Ilonen J, Lempainen J, Veijola R. The Heterogeneous Pathogenesis of Type 1 Diabetes Mellitus. Nat Rev Endocrinol (2019) 15:635–50. doi: 10.1038/s41574-019-0254-y
6. Battaglia M, Ahmed S, Anderson MS, Atkinson MA, Becker D, Bingley PJ, et al. Introducing the Endotype Concept to Address the Challenge of Disease Heterogeneity in Type 1 Diabetes. Diabetes Care (2020) 43:5–12. doi: 10.2337/dc19-0880
7. Lempainen J, Laine AP, Hammais A, Toppari J, Simell O, Veijola R, et al. Non-HLA Gene Effects on the Disease Process of Type 1 Diabetes: From HLA Susceptibility to Overt Disease. J Autoimmun (2015) 61:45–53. doi: 10.1016/j.jaut.2015.05.005
8. Laine AP, Knip M, Ilonen J. Finnish Pediatric Diabetes Register. Transmission Disequilibrium Analysis of 31 Type 1 Diabetes Susceptibility Loci in Finnish Families. Tissue Antigens (2013) 82:35–42. doi: 10.1111/tan.12143
9. Ilonen J, Hammais A, Laine A, Lempainen J, Vaarala O, Veijola R, et al. Patterns of β-Cell Autoantibody Appearance and Genetic Associations During the First Years of Life. Diabetes (2013) 62:3636–40. doi: 10.2337/db13-0300
10. Kupila A, Muona P, Simell T, Arvilommi P, Savolainen H, Hämäläinen AM, et al. Feasibility of Genetic and Immunological Prediction of Type I Diabetes in a Population-Based Birth Cohort. Diabetologia (2001) 44:290–7. doi: 10.1007/s001250051616
11. Ilonen J, Kiviniemi M, Lempainen J, Simell O, Toppari J, Veijola R, et al. Genetic Susceptibility to Type 1 Diabetes in Childhood - Estimation of HLA Class II Associated Disease Risk and Class II Effect in Various Phases of Islet Autoimmunity. Pediatr Diabetes (2016) 17 Suppl 22:8–16. doi: 10.1111/pedi.12327
12. Kukko M, Kimpimäki T, Korhonen S, Kupila A, Simell S, Veijola R, et al. Dynamics of Diabetes-Associated Autoantibodies in Young Children With Human Leukocyte Antigen-Conferred Risk of Type 1 Diabetes Recruited From the General Population. J Clin Endocrinol Metab (2005) 90:2712–7. doi: 10.1210/jc.2004-1371
13. Ilonen J, Kiviniemi M, Lempainen J, Simell O, Toppari J, Veijola R, et al. Genetic Susceptibility to Type 1 Diabetes in Childhood - Estimation of HLA Class II Associated Disease Risk and Class II Effect in Various Phases of Islet Autoimmunity. Pediatr Diabetes (2016) 17 Suppl 22:8–16. doi: 10.1111/pedi.12327
14. Karczewski KJ, Francioli LC, Tiao G, Cummings BB, Alföldi J, Wang Q, et al. The Mutational Constraint Spectrum Quantified From Variation in 141,456 Humans. Nature (2020) 581:434–43. doi: 10.1038/s41586-020-2308-7
15. Siljander HTA, Simell S, Hekkala A, Lähde J, Simell T, Vähäsalo P, et al. Predictive Characteristics of Diabetes-Associated Autoantibodies Among Children With HLA-Conferred Disease Susceptibility in the General Population. Diabetes (2009) 58:2835–42. doi: 10.2337/db08-1305
16. Karjalainen JK. Islet Cell Antibodies as Predictive Markers for IDDM in Children With High Background Incidence of Disease. Diabetes (1990) 39:1144–50. doi: 10.2337/diab.39.9.1144
17. Benjamini Y, Hochberg Y. Controlling the False Discovery Rate: A Practical and Powerful Approach to Multiple Testing. J R Stat Society: Ser B (Methodological) (1995) 57:289–300. doi: 10.1111/j.2517-6161.1995.tb02031.x
18. Purcell S, Neale B, Todd-Brown K, Thomas L, Ferreira MAR, Bender D, et al. PLINK: A Tool Set for Whole-Genome Association and Population-Based Linkage Analyses. Am J Hum Genet (2007) 81:559–75. doi: 10.1086/519795
19. Sharma A, Liu X, Hadley D, Hagopian W, Chen WM, Onengut-Gumuscu S, et al. Identification of non-HLA Genes Associated With Development of Islet Autoimmunity and Type 1 Diabetes in the Prospective TEDDY Cohort. J Autoimmun (2018) 89:90–100. doi: 10.1016/j.jaut.2017.12.008
20. Steck AK, Dong F, Wong R, Fouts A, Liu E, Romanos J, et al. Improving Prediction of Type 1 Diabetes by Testing Non-HLA Genetic Variants in Addition to HLA Markers. Pediatr Diabetes (2014) 15:355–62. doi: 10.1111/pedi.12092
21. Winkler C, Lauber C, Adler K, Grallert H, Illig T, Ziegler A, et al. An Interferon-Induced Helicase (IFIH1) Gene Polymorphism Associates With Different Rates of Progression From Autoimmunity to Type 1 Diabetes. Diabetes (2011) 60:685–90. doi: 10.2337/db10-1269
22. Lempainen J, Hermann R, Veijola R, Simell O, Knip M, Ilonen J. Effect of the PTPN22 and INS Risk Genotypes on the Progression to Clinical Type 1 Diabetes After the Initiation of Beta-Cell Autoimmunity. Diabetes (2012) 61:963–6. doi: 10.2337/db11-0386
23. Krischer JP, Lynch KF, Lernmark Å, Hagopian WA, Rewers MJ, She J, et al. Genetic and Environmental Interactions Modify the Risk of Diabetes-Related Autoimmunity by 6 Years of Age: The TEDDY Study. Diabetes Care (2017) 40:1194–202. doi: 10.2337/dc17-0238
24. Krischer JP, Liu X, Vehik K, Akolkar B, Hagopian WA, Rewers MJ, et al. Predicting Islet Cell Autoimmunity and Type 1 Diabetes: An 8-Year TEDDY Study Progress Report. Diabetes Care (2019) 42:1051–60. doi: 10.2337/dc18-2282
25. Krischer JP, Liu X, Lernmark Å, Hagopian WA, Rewers MJ, She J, et al. The Influence of Type 1 Diabetes Genetic Susceptibility Regions, Age, Sex, and Family History on the Progression From Multiple Autoantibodies to Type 1 Diabetes: A TEDDY Study Report. Diabetes (2017) 66:3122–9. doi: 10.2337/db17-0261
26. Mikk ML, Heikkinen T, El-Amir MI, Kiviniemi M, Laine AP, Harkonen T, et al. The Association of the HLA-A*24:02, B*39:01 and B*39:06 Alleles With Type 1 Diabetes is Restricted to Specific HLA-DR/DQ Haplotypes in Finns. HLA (2017) 89:215–24. doi: 10.1111/tan.12967
27. Balke EM, Balti EV, van der Auwera B, Weets I, Costa O, Demeester S, et al. Accelerated Progression to Type 1 Diabetes in the Presence of HLA-A*24 and -B*18 Is Restricted to Multiple Islet Autoantibody-Positive Individuals With Distinct HLA-DQ and Autoantibody Risk Profiles. Diabetes Care (2018) 41:1076–83. doi: 10.2337/dc17-2462
28. Koskinen MK, Mikk ML, Laine AP, Lempainen J, Loyttyniemi E, Vahasalo P, et al. Longitudinal Pattern of First-Phase Insulin Response Is Associated With Genetic Variants Outside the Class II HLA Region in Children With Multiple Autoantibodies. Diabetes (2020) 69:12–9. doi: 10.2337/db19-0329
29. Burn GL, Svensson L, Sanchez-Blanco C, Saini M, Cope AP. Why is PTPN22 a Good Candidate Susceptibility Gene for Autoimmune Disease? FEBS Lett (2011) 585:3689–98. doi: 10.1016/j.febslet.2011.04.032
30. Valta M, Gazali AM, Viisanen T, Ihantola E, Ekman I, Toppari J, et al. Type 1 Diabetes Linked PTPN22 Gene Polymorphism Is Associated With the Frequency of Circulating Regulatory T Cells. Eur J Immunol (2020) 50:581–8. doi: 10.1002/eji.201948378
31. Ferreira RC, Castro Dopico X, Oliveira JJ, Rainbow DB, Yang JH, Trzupek D, et al. Chronic Immune Activation in Systemic Lupus Erythematosus and the Autoimmune PTPN22 Trp620 Risk Allele Drive the Expansion of FOXP3+ Regulatory T Cells and PD-1 Expression. Front Immunol (2019) 0:2606. doi: 10.3389/fimmu.2019.02606
32. Newby BN, Brusko TM, Zou B, Atkinson MA, Clare-Salzler M, Mathews CE. Type 1 Interferons Potentiate Human CD8+ T-Cell Cytotoxicity Through a STAT4- and Granzyme B-Dependent Pathway. Diabetes (2017) 66:3061–71. doi: 10.2337/db17-0106
33. Holz A, Bot A, Coon B, Wolfe T, Grusby MJ, von Herrath MG. Disruption of the STAT4 Signaling Pathway Protects From Autoimmune Diabetes While Retaining Antiviral Immune Competence. J Immunol (1999) 163:5374–82.
34. de Azevêdo Silva J, Tavares N, Santos MMS, Moura R, Guimarães RL, Araújo J, et al. Meta-Analysis of STAT4 and IFIH1 Polymorphisms in Type 1 Diabetes Mellitus Patients With Autoimmune Polyglandular Syndrome Type III. Genet Mol Res (2015) 14:17730–8. doi: 10.4238/2015.December.21.46
35. Fichna M, Żurawek M, Bogusz-Górna K, Małecki PP, Niechciał E, Sidoruk A, et al. STAT4 Sequence Variant and Elevated Gene Expression are Associated With Type 1 Diabetes in Polish Children. Cent Eur J Immunol (2020) 45:22–8. doi: 10.5114/ceji.2019.92492
36. Ilonen J, Hammais A, Laine AP, Lempainen J, Vaarala O, Veijola R, et al. Patterns of Beta-Cell Autoantibody Appearance and Genetic Associations During the First Years of Life. Diabetes (2013) 62:3636–40. doi: 10.2337/db13-0300
37. Hasan NM, Kendrick MA, Druckenbrod NR, Huelsmeyer MK, Warner TF, MacDonald MJ. Genetic Association of the Neuropilin-1 Gene With Type 1 Diabetes in Children: Neuropilin-1 Expression in Pancreatic Islets. Diabetes Res Clin Pract (2010) 87:29. doi: 10.1016/j.diabres.2009.12.016
38. Ge Y, Paisie TK, Newman JRB, McIntyre LM, Concannon P. UBASH3A Mediates Risk for Type 1 Diabetes Through Inhibition of T-Cell Receptor-Induced NF-κb Signaling. Diabetes (2017) 66:2033–43. doi: 10.2337/db16-1023
39. Keene KL, Quinlan AR, Hou X, Hall IM, Mychaleckyj JC, Onengut-Gumuscu S, et al. Evidence for Two Independent Associations With Type 1 Diabetes at the 12q13 Locus. Genes Immun (2012) 13:66–70. doi: 10.1038/gene.2011.56
40. Wang H, Jin Y, Reddy MV, Prasad L, Podolsky R, Liu S, et al. Genetically Dependent ERBB3 Expression Modulates Antigen Presenting Cell Function and Type 1 Diabetes Risk. PLoS One (2010) 5:e11789. doi: 10.1371/journal.pone.0011789
41. Vandewalle J, van der Auwera BJ, Amin H, Quartier E, Desouter AK, Tenoutasse S, et al. Genetic Variation at ERBB3/IKZF4 and Sexual Dimorphism in Epitope Spreading in Single Autoantibody-Positive Relatives. Diabetologia (2021) 64:2511–6. doi: 10.1007/s00125-021-05546-9
42. Pan F, Yu H, Dang EV, Barbi J, Pan X, Grosso JF, et al. Eos Mediates Foxp3-Dependent Gene Silencing in CD4+ Regulatory T Cells. Science (2009) 325:1142–6. doi: 10.1126/science.1176077
43. Gokhale AS, Gangaplara A, Lopez-Occasio M, Thornton AM, Shevach EM. Selective Deletion of Eos (Ikzf4) in T-Regulatory Cells Leads to Loss of Suppressive Function and Development of Systemic Autoimmunity. J Autoimmun (2019) 105:102300. doi: 10.1016/j.jaut.2019.06.011
44. Santin I, Moore F, Colli ML, Gurzov EN, Marselli L, Marchetti P, et al. PTPN2, A Candidate Gene for Type 1 Diabetes, Modulates Pancreatic β-Cell Apoptosis via Regulation of the BH3-Only Protein Bim. Diabetes (2011) 60:3279–88. doi: 10.2337/db11-0758
45. Laine AP, Hermann R, Knip M, Simell O, Akerblom HK, Ilonen J. The Human Leukocyte Antigen Genotype has a Modest Effect on the Insulin Gene Polymorphism-Associated Susceptibility to Type 1 Diabetes in the Finnish Population. Tissue Antigens (2004) 63:72–4. doi: 10.1111/j.1399-0039.2004.00153.x
46. Bjørnvold M, Undlien DE, Joner G, Dahl-Jørgensen K, Njølstad PR, Akselsen HE, et al. Joint Effects of HLA, INS, PTPN22 and CTLA4 Genes on the Risk of Type 1 Diabetes. Diabetologia (2008) 51:589–96. doi: 10.1007/s00125-008-0932-0
47. Bonifacio E, Beyerlein A, Hippich M, Winkler C, Vehik K, Weedon MN, et al. Genetic Scores to Stratify Risk of Developing Multiple Islet Autoantibodies and Type 1 Diabetes: A Prospective Study in Children. PLoS Med (2018) 15(4):e1002548. doi: 10.1371/journal.pmed.1002548
48. Ilonen J, Kiviniemi M, Lempainen J, Simell O, Toppari J, Veijola R, et al. Genetic Susceptibility to Type 1 Diabetes in Childhood - Estimation of HLA Class II Associated Disease Risk and Class II Effect in Various Phases of Islet Autoimmunity. Pediatr Diabetes (2016) 17 Suppl 22:8–16. doi: 10.1111/pedi.12327
Keywords: type 1 diabetes, autoantibodies, seroconversion, follow-up-cohort, survival analysis
Citation: Laine AP, Valta M, Toppari J, Knip M, Veijola R, Ilonen J and Lempainen J (2022) Non-HLA Gene Polymorphisms in the Pathogenesis of Type 1 Diabetes: Phase and Endotype Specific Effects. Front. Immunol. 13:909020. doi: 10.3389/fimmu.2022.909020
Received: 31 March 2022; Accepted: 20 May 2022;
Published: 21 June 2022.
Edited by:
Arnaud Zaldumbide, Leiden University Medical Center, NetherlandsReviewed by:
Georgia Fousteri, San Raffaele Hospital (IRCCS), ItalyKaren Cerosaletti, Benaroya Research Institute, United States
Copyright © 2022 Laine, Valta, Toppari, Knip, Veijola, Ilonen and Lempainen. This is an open-access article distributed under the terms of the Creative Commons Attribution License (CC BY). The use, distribution or reproduction in other forums is permitted, provided the original author(s) and the copyright owner(s) are credited and that the original publication in this journal is cited, in accordance with accepted academic practice. No use, distribution or reproduction is permitted which does not comply with these terms.
*Correspondence: Antti-Pekka Laine, YXBsYWluZUB1dHUuZmk=; Mikael Knip, bWlrYWVsLmtuaXBAaGVsc2lua2kuZmk=