- Department of Neurology, Tongji Hospital, Tongji Medical College, Huazhong University of Science and Technology, Wuhan, China
Background: In patients with autoimmune encephalitis (AE), the prediction of progression to a critically ill status is challenging but essential. However, there is currently no standard prediction model that comprehensively integrates the disease severity and other clinical features. The clinical assessment scale in autoimmune encephalitis (CASE) and the modified Rankin Scale (mRS) have both been applied for evaluating the severity of AE. Here, by combining the two scales and other clinical characteristics, we aimed to investigate risk factors and construct prediction models for early critical care needs of AE patients.
Methods: Definite and probable AE patients who were admitted to the neurology department of Tongji Hospital between 2013 and 2021 were consecutively enrolled. The CASE and mRS scores were used to evaluate the overall symptom severity at the time of hospital admission. Using logistic regression analysis, we analyzed the association between the total scores of the two scales and critical illness individually and then we evaluated this association in combination with other clinical features to predict early intensive care unit (ICU) admission. Finally, we constructed four prediction models and compared their performances.
Results: Of 234 patients enrolled, forty developed critical illness and were early admitted to the ICU (within 14 days of hospitalization). Four prediction models were generated; the models were named CASE, CASE-plus (CASE + prodromal symptoms + elevated fasting blood glucose + elevated cerebrospinal fluid (CSF) white blood cell (WBC) count), mRS and mRS-plus (mRS + prodromal symptoms + abnormal EEG results + elevated fasting blood glucose + elevated CSF WBC count) and had areas under the ROC curve of 0.850, 0.897, 0.695 and 0.833, respectively. All four models had good calibrations. In general, the models containing “CASE” performed better than those including “mRS”, and the CASE-plus model demonstrated the best performance.
Conclusion: Overall, the symptom severity at hospital admission, as defined by CASE or mRS, could predict early ICU admission, especially when assessed by CASE. Adding other clinical findings, such as prodromal symptoms, an increased fasting blood glucose level and an increased CSF WBC count, could improve the predictive efficacy.
Introduction
The severity of autoimmune encephalitis (AE) is highly heterogeneous because it can range from mild impairments in working memory to the most severe, persistent disorders of consciousness that would require lasting care or could even cause death (1–3). During the acute stage of the disease, the rapid progression of an immune inflammatory response may cause severe neurological deficits, status epilepticus, coma, and respiratory failure (4). Moreover, with a high risk of suffering from multiple concurrent complications, such as lung infections and sepsis, the reported mortality of AE was as high as 40% in some studies (1, 4). Therefore, some patients require admission to intensive care units (ICUs) for the maximum standard of care. It is still unknown why some patients with AE survive the acute phase of the disease, while others are overwhelmed by the life-threatening acute phase. Previously, several variables, such as anemia, a definite diagnosis of AE (5), cerebrospinal fluid (CSF) WBC >20 cells/mm3 (6), failure of first-line immunotherapy (7) and a high CSF IL-17A concentration (8), were found to be associated with critical illness and subsequent ICU admission. However, to date, there is no standard prediction model that comprehensively includes both the clinical symptom severity and laboratory tests. For the subset of the AE patients who are critically ill, delayed admission to the ICU may be an independent risk factor for poor outcome (9). Therefore, identifying the risk factors for the deterioration to critical illness is crucial for early administration to the intensive care and for timely therapeutic implementation in order to improve prognosis.
Scales are ubiquitously used to assess the severity of symptoms in neurological diseases (10–12). Due to the lack of customized scales, the modified Rankin Scale (mRS) is usually applied for evaluating the neurological severity and outcomes in AE patients (2, 13, 14). However, mRS was originally designed to measure disability after stroke, and it was weighed toward motor deficits and functional independence and apparently with shortage in measuring the non-motor symptoms that frequently occur in AE (15). The clinical assessment scale in autoimmune encephalitis (CASE) is a novel tool that was developed in 2019 to specifically evaluate the clinical severity of a series of syndromes, including definite AE, definite autoimmune limbic encephalitis (ALE), autoantibody negative but probable AE, definite acute disseminated encephalomyelitis (ADEM), and definite and probable brainstem encephalitis (16). The CASE is composed of nine major clinical features of AE, with a total score ranging from 0 to 27 (16), and this makes it a fine quantification tool that has great potential in the assessment of AE. Two studies that evaluated Chinese patients with antibody-positive AE confirmed the accuracy of the clinical evaluation of CASE (17, 18). However, CASE has not been popularized in clinical practice, nor is there a study comparing the performance of the mRS and CASE in predicting ICU admission independently or in combination with other clinical factors.
Prolonged hospital length of stay may increase the risk of hospital-acquired infection (19), leading to an increased likelihood of ICU admission for reasons not directly related to AE. Therefore, to be representative of ICU admission for AE-related reasons, this study aimed to investigate the association between symptom severity at hospital admission, as assessed by the CASE or mRS score, and early deterioration, requiring ICU care, in patients diagnosed with definite and probable AE, and to construct scale-based risk prediction models and compare the performances of these models. Finally, we explored whether the addition of other clinical factors to the models that evaluated symptom severity could improve their predictive efficacy.
Materials and Methods
Patients
We retrospectively extracted data from the medical records of consecutive patients diagnosed with encephalitis who were treated from January 2013 to October 2021 in the Department of Neurology, Tongji Hospital, and we screened those patients who met the clinical diagnostic criteria for definite and probable AE proposed by Mittal and Graus et al. in 2016 (3). Specifically, the patients with definite AE, definite ALE and autoantibody-negative but probable AE were included in this study. The detailed diagnostic criteria for each AE are described in the Supplementary Table 1. Patients were excluded when 1) they had infectious encephalitis with laboratory evidence, including tuberculosis or bacterial, fungal, viral (IgM), or parasitic infections; format correction: 2) they did not fulfill the probable AE criteria (e.g, AE mimics such as Creutzfeldt–Jakob disease, metabolic encephalopathy, neoplastic disorders and cerebrovascular disease (Identification of these disorders was based on history, physical examination, laboratory tests, and auxiliary tests; auxiliary tests used are listed in Supplementary Table 2); other diseases screened from the electronic database such as meningitis); 3) they had received immunotherapy before hospital admission or this admission was not their index AE admission; 4) they were under the age of 18; 5) they were admitted to the ICU immediately at hospitalization; or 6) they had incomplete medical records. Early ICU admission was defined as admission to the ICU at any time point within two weeks of hospitalization and patients admitted to the ICU beyond 14 days of hospitalization were classified into “non-early ICU admission” group. The protocol was approved by the institutional ethics board of Tongji Hospital, Tongji Medical College of Huazhong University of Science and Technology (ID: TJ-IRB20211221).
Data Collection
The following details about the acute phase of the disease were obtained by 3 neurologists (W-CM, W-HT and Z-YY): (1) demographic information (sex, age); (2) clinical features: comorbidities including hypertension, diabetes and autoimmune diseases; prodromal symptoms such as fever, headache, nonspecific respiratory or gastrointestinal symptoms and other nonspecific viral-like symptoms; the symptoms at onset and all of the symptoms that were present from the onset to hospital admission; the date of onset, the date of hospital admission and discharge; (3) laboratory results: the results of blood tests within 24 hours and the first laboratory CSF sample analysis after admission. For antibody detection, blood and CSF samples were sent to the same laboratory for detection of antibody types and titers using cell-based assay (CBA) in an indirect immunofluorescence (IIF) test and immunospot assay. Antibody titer was defined as low (+, 1:10 in blood or 1:1 in CSF), moderate (++, ≤1:100 in blood or ≤1:10 in CSF), or high (+++, ≥1:320 in blood or ≥1:32 in CSF), with initial dilution titers of CSF and serum of 1:1 vs. 1:10. Six basic types of antibodies were detected for every patient: anti-N-methyl-D-aspartate receptor (NMDAR) antibody, anti-α-amino-3-hydroxy-5-methyl-4-isoxazolepropionic acid 1 (AMPA1) receptor antibody, and anti-AMPA2 receptor antibody, anti-leucine-rich glioma-inactivated 1 (LGI1) antibody, anti-gamma-aminobutyric acid-B receptor (GABABR) antibody and anti-contactin-associated protein-like 2 (CASPR2) antibody. Other optional antibody types including anti-GABAAR antibody, anti-dipeptidyl-peptidase-like protein-6 (DPPX) antibody, anti-mGluR5 antibody, anti-glutamic acid decarboxylase 65 (GAD65) antibody, anti-myelin oligodendrocyte glycoprotein (MOG) antibody, anti-Ma2 antibody, anti-Dopamine 2 receptor (D2R) antibody, anti-Hu antibody and so on. (4) imaging and electroencephalography (EEG) data: the first results of magnetic resonance imaging (MRI) and EEG; (5) therapeutic data, including first-line immunotherapy (corticosteroids, intravenous immunoglobulin, plasma exchange) and second-line immunotherapy (cyclophosphamide, mycophenolate mofetil, and rituximab) (3, 20, 21); and (6) the scale data: the CASE and mRS scores at the time of hospital admission.
Scale Assessment
The CASE and mRS scores were assessed simultaneously upon hospital admission. The CASE contains nine items: seizures (current status), memory dysfunction, psychiatric symptoms (delusion, hallucination, disinhibition, aggression), consciousness, language problems, dyskinesia/dystonia, gait instability and ataxia, brainstem dysfunction, and weakness. Each item was based on a 3-point grading system, except for the item ‘‘brainstem dysfunction’’, which is rated by the number of symptoms (gaze paresis, tube feeding, and ventilator care due to hypoventilation), with one point given for each symptom and a maximum of three points (16). The mRS has six grades (0-5) as follows: 0 = no symptoms, 1 = no significant disability: able to carry out all usual activities despite the presence of symptoms, 2 = Slight disability: unable to perform all usual activities but able to look after their own affairs without assistance, 3 = Moderate disability: requiring some help but able to walk without assistance, 4 = Moderately severe disability: unable to walk or attend bodily needs without assistance, 5 = Severe disability: bedridden, incontinent and requiring constant nursing care and attention (15). Two neurologists (W-HT and Z-YY), who were blinded to the research purpose, evaluated the scales independently by reviewing the detailed medical records, and consensus was achieved after discussion in any discrepant cases. If no consensus was reached, a third senior neurologist (H-SS) made the final decision.
Statistical Analysis
Categorical variables are shown as counts and percentages and were analyzed by Pearson’s chi-squared test or Fisher’s exact test. Continuous variables are expressed as the mean ± SD or medians (quartiles), and Student’s t test or the Mann–Whitney U test was used to compare the group data accordingly. EEG and MRI data were missing in 75 (32.1%) and 8 (3.4%) patients because these examinations were either not completed or the results were not recorded in the medical records, and these patients were classified into the “unknown” group. Variables with P values < 0.05 in univariate analyses were subjected to multivariate logistic regression analysis with a stepwise elimination procedure to obtain an optimized model in terms of a minimal Akaike’s information criterion (AIC) value. The AE-related management decision based on the judgment of the attending clinicians was a reflection of the disease characteristics, and not all treatments were performed prior to ICU admission. Therefore, the treatment data were not included as risk factors in the multivariate logistic regression analysis. Variables with P values < 0.05 in the optimized multivariate regression model were used to build the final prediction model. Each model was calibrated by a calibration curve, which is actually a visualization of the Hosmer–Lemeshow test. The discriminatory ability of the models was assessed by calculating the area under the receiver operating characteristic (ROC) curves (AUC). ROC analysis was also used to calculate the optimal cutoff values, and these were determined by maximizing the Youden index. The accuracy of the optimal cutoff value was assessed by the sensitivity and specificity. Internal validation of the models was performed using bootstraps with 1000 replicates (22). To determine if any of the candidate models outperformed the others, we used the DeLong test (23) to explore each of the model pairs for a difference in the AUC values. Two-sided values of P<0.05 were considered statistically significant. All analyses were performed with IBM SPSS software, version 24 (SPSS Inc., Chicago, IL, USA), R software version 3.6.1 (The R Foundation for Statistical Computing, Vienna, Austria; http://www.r-project.org) and GraphPad Prism 8. We prepared this article using STROBE, which is the guideline for observational study reports.
Results
Clinical Characteristics of AE
From January 2013 to October 2021, a total of 769 patients with potential encephalitis were screened from the electronic database, of which 344 met the diagnosis criteria of probable or definite AE, and 234 patients were included in the final analysis after excluding the following patients: 43 patients received immunotherapy before hospitalization/non-index AE admission, 27 patients aged under 18 years old, and 40 patients admitted to the ICU immediately upon hospitalization. The flow chart of the study is shown in Figure 1. We did not formally calculate the sample size because the current number of patients was determined by the availability of existing data from the introduction of autoimmune encephalitis antibody testing. Of the enrolled patients, 54.7% (128/234) tested positive for neuronal antibodies, which included anti-NMDAR (n=69), LGI1 (n=14), CASPR2 (n=9), GABABR (n =14), AMPA (n=3), DPPX (n=7), GAD65 (n=1), mGluR 5 (n=1), MOG (n=3), NMDAR/AMPA (n=1), LGI1/GABABR (n=2), LGI1/CASPR2 (n=2), LGI1/AMPA (n=1) and GABABR/MOG (n=1). Details of antibodies are shown in Supplementary Table 3. Five patients were autoantibody negative but were clinically diagnosed with definite autoimmune limbic encephalitis, and the remaining 101 (43.2%) patients fulfilled the criteria for autoantibody-negative but probable AE. The characteristics of the patients are summarized in Table 1. The median age of the 234 patients (56.0% males) was 39.0 (IQR 26.0-54.3) years. All patients were in the acute phase of the index admission, and 73.5% (172/234) had an interval of less than 1 month from symptom onset to hospital admission. The timeline of patients from hospital admission to discharge is shown in Supplementary Figure 1. Epilepsy was the most common initial symptom (42.3%) and was the most common symptom from onset to hospital admission (52.6%), followed by psychiatric symptoms (50.4%). Half of the patients (52.6%) had prodromal symptoms. Two hundred and one (85.9%) patients received immunotherapy, while 14.1% of patients rejected any immunotherapies due to a mild disease severity, poor economic conditions, or an intolerance of side effects. Forty patients (17.1%) deteriorated for the early of their hospitalization and were admitted to the ICU. The common direct reasons for early ICU admission were status epilepticus (32.5%, 13/40), unstable vital sign (respiratory failure or blood pressure drop) (17.5%, 7/40), severe psychiatric symptoms (15.0%, 6/40) and decreased level of consciousness (7.5%, 3/40), details are shown in Supplementary Table 4.
Factors Associated With Early ICU Admission Among AE Patients
As shown in Table 1, the total CASE and mRS scores were both significantly associated with ICU admission (P<0.001). We found that the CASE and mRS scores were statistically correlated (r=0.642, P<0.001) (Figure 2), which was consistent with previous studies (7, 17, 18). Other variables that were significant associated with admission to the ICU included time from symptom onset to hospital admission of less than 1 month (P=0.024), prodromal symptoms (P=0.003); symptoms from onset to hospital admission: psychiatric symptoms (P=0.009), consciousness disorders (P<0.001); laboratory tests: elevated CSF pressure (P=0.001), elevated CSF WBC count (P<0.001), elevated fasting blood glucose (P<0.001), Na+ (P=0.006); abnormal or unknown EEG results (P=0.031), and anti-seizure medications (ASMs) therapy (P=0.009).
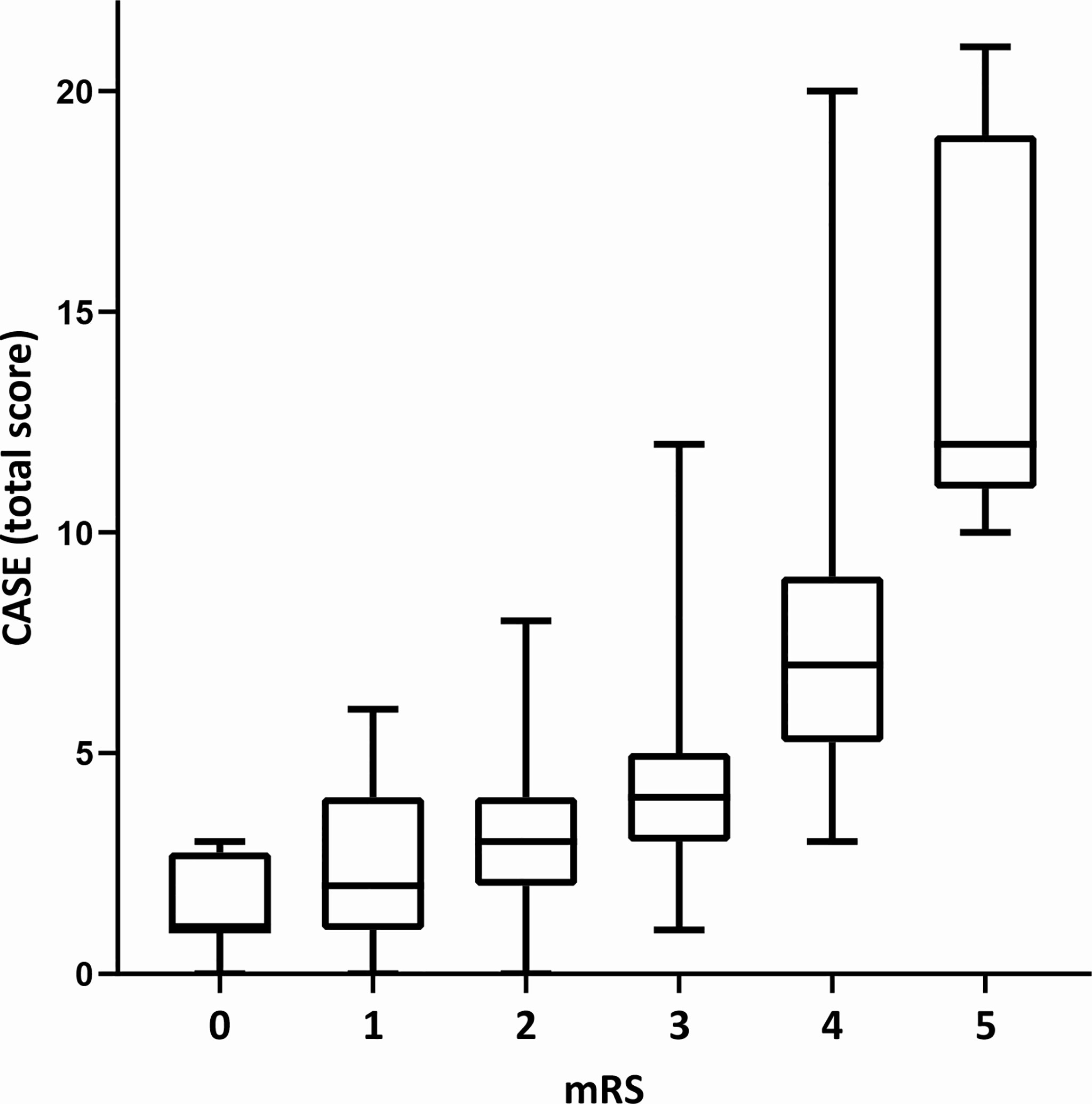
Figure 2 The total CASE score according to the mRS at the time of hospital admission. CASE, the Clinical Assessment Scale for Autoimmune Encephalitis. mRS, the modified Rankin scale. The CASE and mRS scores were statistically correlated (r = 0.642, P < 0.001).
Risk Models for Prediction Early ICU Admission
Model Construction
Next, we conducted two multivariate logistic regression analyses. One included the CASE score and the variables with P<0.05 in the univariate analysis, and the other included the mRS score and variables with P<0.05 in the univariate analysis. The results of the optimized multivariate regression model after the variable selection are shown as forest plots (Figure 3). As seen from the forest plots, there were four significant independent predictors (CASE, prodromal symptoms, elevated fasting blood glucose and elevated CSF WBC count) in the CASE model and five (mRS, prodromal symptoms, abnormal EEG results, elevated fasting blood glucose and elevated CSF WBC count) in the mRS model.
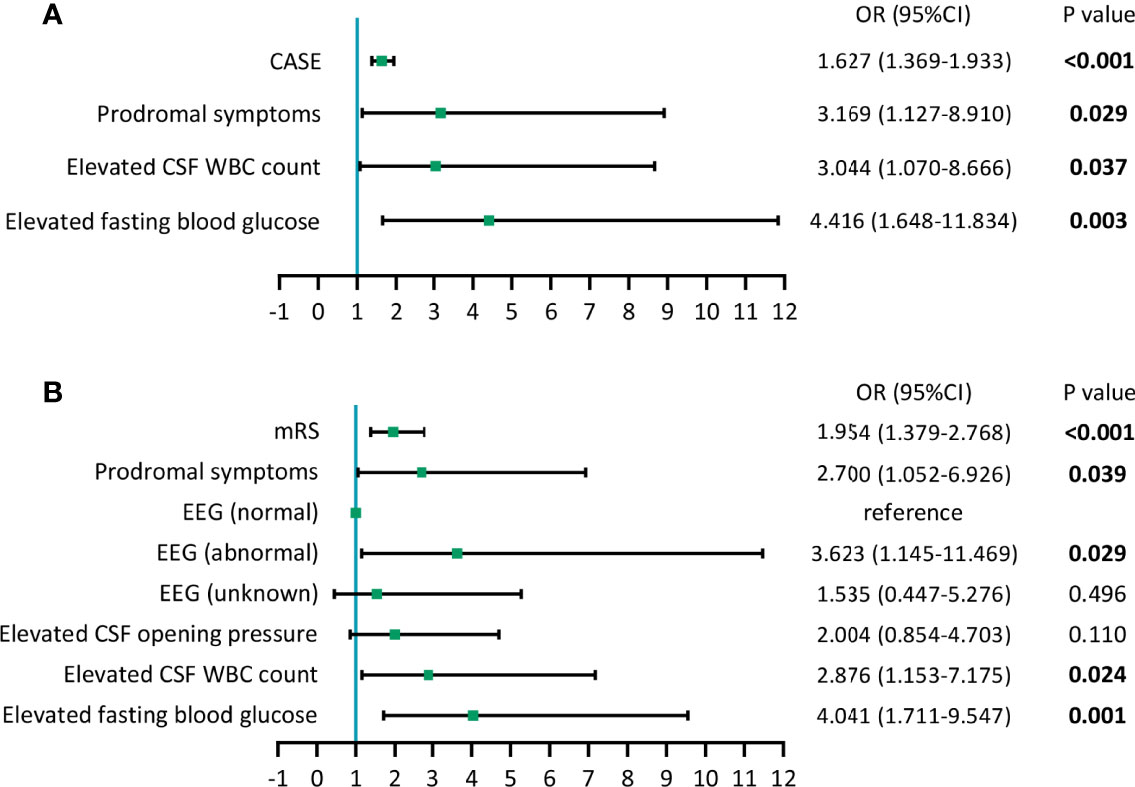
Figure 3 Forest plots of models of multivariate logistic regression analysis. (A) Results of multivariate logistic regression analysis containing CASE. (B) Results of multivariate logistic regression analysis containing mRS.
Based on the results of the multivariate analysis, we developed the following four candidate predictive models:
Model 1: CASE: CASE alone;
Model 2: CASE-plus: CASE + prodromal symptoms + elevated fasting blood glucose + elevated CSF WBC count;
Model 3: mRS: mRS alone;
Model 4: mRS-plus: mRS + prodromal symptoms + abnormal EEG results + elevated fasting blood glucose + elevated CSF WBC count
Model Evaluation
Discrimination
The ROC curves for each model are presented in Figure 4A1. Figure 4A2 shows the uncorrected AUC values and the bootstrapped optimism corrected AUC values for each model. The bootstrap-adjusted AUC values were similar to the uncorrected AUC values. The optimal cutoff scores, which were derived from the ROC analysis, of Model 1 and Model 3 were 4.5 and 2.5, respectively. We also summarized the sensitivity and specificity in estimating the risk of ICU admission using 4.5 and 2.5 as the cutoff values (Figure 4A2). To determine if any of the four candidate models outperformed the others, we used DeLong’s test (23) to test each of the four correlated possible model pairs for a difference in predicting the uncorrected AUC scores. We found a significant difference in the discriminant ability of each pair (P<0.05), except for Model 1 and Model 4 (P=0.671), and the P values are listed in Figure 4A3. By combining Figure 4A, we can conclude that Model 2 performed best, followed by Model 1, Model 4 and Model 3, with AUCs of 0.897 (95% CI 0.842-0.953, P<0.001), 0.850 (95% CI 0.773-0.927, P<0.001), 0.833 (95% CI 0.760-0.906, P<0.001) and 0.695 (95% CI 0.599-0.792, P<0.001), respectively (Figure 4A2).
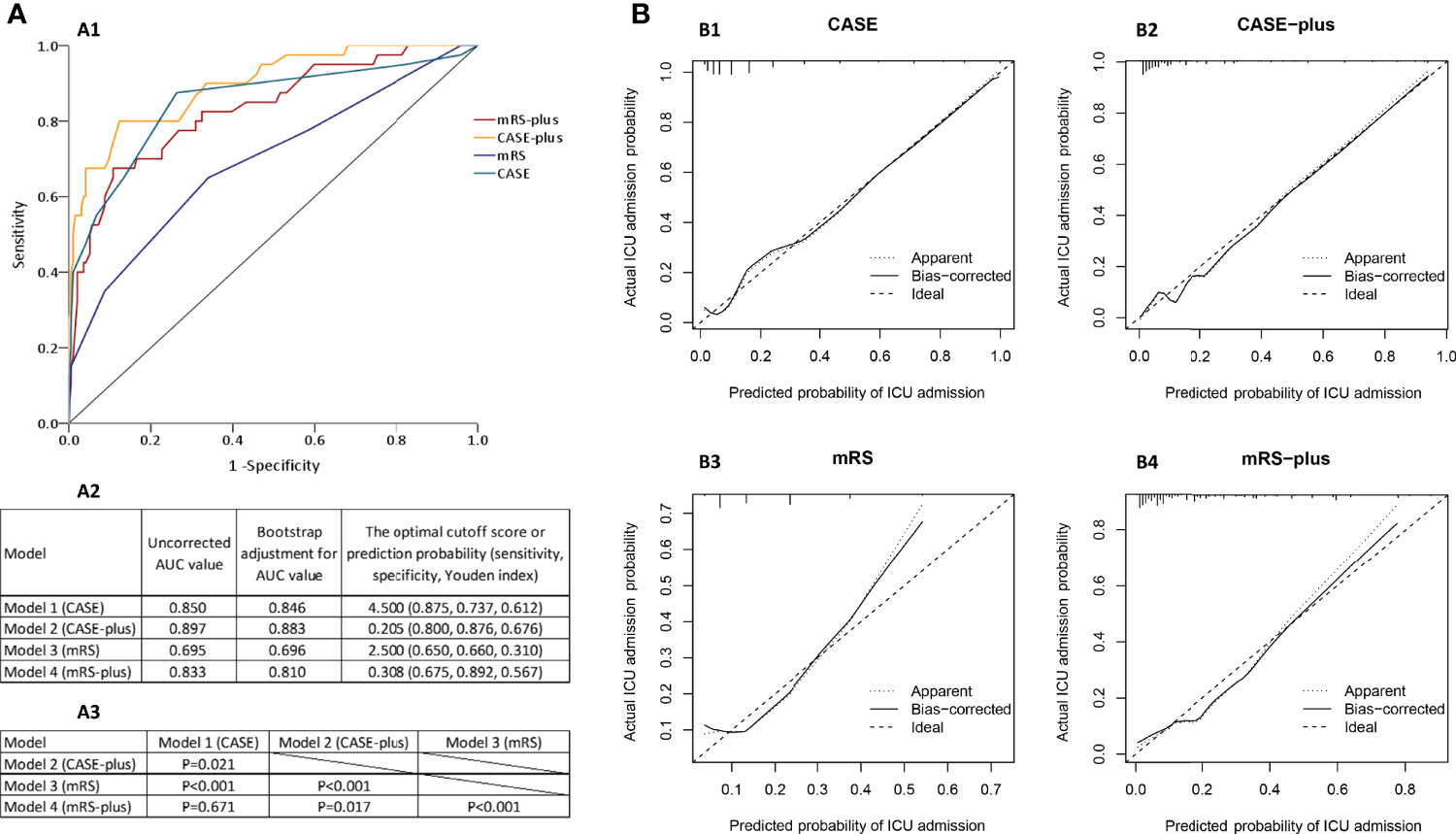
Figure 4 Risk prediction models for early ICU admission in patients with AE. (A1) Receiver operating characteristic (ROC) curves of the four models for predicting early ICU admission. (A2) Uncorrected and the bootstrap-adjusted AUC values and optimal cutoff values of the four models. The AUC values of CASE, CASE-plus, mRS, mRS-plus were 0.850 (95% CI 0.773-0.927, P<0.001), 0.897 (95% CI 0.842-0.953, P<0.001), 0.695 (95% CI 0.599-0.792, P<0.001), and 0.833 (95% CI 0.760-0.906, P<0.001), respectively. (A3) P values for the pairwise comparison of original AUC values of the four models. (B) The calibration curves of the four models in predicting ICU admission. The y-axis represents the actual probability of ICU admission, and the x-axis represents the predicted probability of ICU admission. A perfect model would fully match the 45° ideal line.
Calibration
The Hosmer–Lemeshow test of these four models showed (with P values of 0.14, 0.44, 0.35 and 0.40 for Models 1, 2, 3 and 4, respectively) that the calibrations of the four models were adequate and that the models were correctly specified. The apparent and bootstrapped calibration curves for each model (Figure 4B) showed the excellent agreement between the observed outcomes and the predictions, with predicted probabilities positioned on or around a 45° line of the plot.
Discussion
In this retrospective study of critically ill and noncritically ill patients with AE who were treated in a tertiary hospital, we investigated the association between the patient characteristics and early ICU admission. In the univariate analysis, both the CASE and mRS scores were significantly associated with ICU admission. Next, we developed four risk prediction models: CASE, CASE-plus (CASE plus = CASE + prodromal symptoms + elevated fasting blood glucose + elevated CSF WBC count), mRS, and mRS-plus (mRS plus = mRS + prodromal symptoms + abnormal EEG results + elevated fasting blood glucose + elevated CSF WBC count). Among the four models, the CASE-plus model demonstrated the best performance.
CASE is the first clinical severity scale that was specifically designed for the various syndromes of AE. Although lacking large-scale validation, Cai and Zhang et al. proved that CASE performed well and had a significant positive correlation with mRS in two groups of Chinese patients with antibody-positive AE (17, 18). As a comprehensive scale covering multiple domains of AE, CASE has inherent advantages when compared to mRS. First, the detailed assessment of various specific clinical manifestations allows CASE to represent the overall severity of the disease, especially in patients with nonmotor symptoms and who develop common intensive care signs, such as status epilepticus, coma and mechanical ventilation due to central hypoventilation, and these variables are not included in mRS. Second, the total score of CASE ranges from 0 to 27, and mRS is a 6-point scale (15, 16). This discrepancy makes CASE more precise and sensitive in differentiating the severity of disease within the same range of measurements that are also defined by mRS (16). In our study, total CASE scores of 4.5/27 and total mRS scores of 2.5/6 at the time of hospitalization were the optimal cutoff values in predicting ICU admission; to some extent, these values reflect the early predictive value and sensitivity of the CASE score compared with the mRS score. The cutoff value of the total CASE score also implied that patients with multiple moderate to severe symptoms at the time of admission were more likely to progress to critical conditions. In such cases, quantified symptoms can serve as an alert, which allows patients to receive advanced treatment in a timely manner. Several limitations of CASE may also exist. First, CASE is more complicated and time-consuming than mRS, and it is difficult to use CASE to evaluate some symptoms in specific situations. For example, in sedated patients, the assessment of symptoms such as language and memory can be challenging. Second, the score of each item is unweighted, and CASE is a three-point scale, which may be unfair in assessing some fatal symptoms, such as central hypoventilation. Overall, the total CASE score might be a better optimal predictor of early ICU admission than the mRS score because of its more comprehensive characteristics.
CASE and mRS reflect the pro tempore status of the patient; however, other symptoms can develop in the early course of AE. Also, laboratory and imaging abnormalities may contribute to disease deterioration. We then included more variables associated with AE to screen out other potential risk factors for ICU admission. We found that prodromal symptoms, abnormal EEG results, elevated fasting blood glucose and elevated CSF WBC count were independent predictors in the multivariable analysis. In our study, 52.6% of patients had prodromal symptoms, which is consistent with previous studies (34%-62.8%) (2, 24, 25). Prodromal symptoms are nonspecific, vary in presentation and, more importantly, indicate infection (26). In fact, infections have long been suspected to play a role in triggering or enhancing the autoimmune process (26, 27). Accumulating evidence suggests that viral infections may be associated with the development of AE (28, 29). In other autoimmune diseases, such as myasthenia gravis (MG) (30) and Guillain–Barre syndrome (GBS) (31, 32), patients who triggered by infection often have a higher ICU admission rate and a more unfavorable prognosis. Elevated CSF WBC count, an indicator of inflammation within the central nervous system (33), was also found to be associated with ICU admission in another study (6). Previously elevated fasting blood glucose might have been ignored as a variable in AE. However, in the setting of acute inflammation, stress hyperglycemia is often observed, which is common in critically ill patients and appears to be a marker of disease severity (34). We found that patients with elevated fasting glucose levels were four times more likely to be admitted to the ICU than patients without elevated fasting glucose levels. However, only 11 of the 49 patients with abnormal fasting glucose levels were diagnosed with diabetes or with an impaired fasting glucose tolerance, indicating that the majority of these patients had acute glucose instability. Therefore, it is feasible for an abnormal fasting blood glucose level at admission to serve as an indicator for the early identification of critical illness in AE cases. Thus, we postulate that infection-triggered and multisystem-involved patients with AE may suffer a more severe disease index.
To generate prediction models with a higher sensitivity, we integrated the above risk factors into CASE and mRS. We found that the predictability of each ICU admission model (except for mRS), as measured by the area under the ROC curve, was more than 0.80. Models containing the CASE score performed better than those containing the mRS score. The CASE-plus and mRS-plus model performed better than the CASE or mRS models, respectively. The best-performing model was the CASE-plus model. This result confirmed that considering both clinical phenotypes and biological disturbances would precisely predict disease progression.
Several limitations should be noted in our study. First, the retrospective nature of the design makes it difficult to control for confounding factors and may lead to possible information bias. AE is a disease that has gradually received attention with the development of antibody detection technology in recent years. Many of the large studies on AE are also retrospective (35, 36), and the results are repeatable. The assessment of CASE was performed retrospectively, and there would inevitably be a small number of patients with incomplete documentation of some items, such as the grading of dyskinesia/dystonia and memory dysfunction. For these patients, we carefully reviewed the medical records, and if no relevant symptoms were recorded throughout the course of the disease, it was considered not present. Nonetheless, CASE has been used in retrospective studies (17, 18, 37) and it is feasible to consider the results of our study are reliable. Second, this is a single-center study, and selection bias may exist. For example, the ICU admission rate was lower than that in previous studies in Western countries (13, 38). As a national tertiary hospital, Tongji Hospital receives a wide coverage and a great number of patients, which makes the patients representative of the general population. In fact, the overall severity and severity distribution of the patients in our study are comparable to those from several domestic studies (17, 18, 39, 40). Third, although the internal validation in our study showed good efficacy, this study was not externally validated. Generalizing the conclusions of this study requires validation in further external datasets, and a prospective, multicenter study with a larger sample size will be necessary in the future.
To the best of our knowledge, this is the first study to integrate the scale-based disease severities of patients with AE into predictive models for ICU admission. Both the CASE and mRS models could accurately predict the risk of ICU admission in AE patients, but the CASE model performed better. Patients with CASE scores ≥5 were more likely to be admitted to the ICU. Adding prodromal symptoms, elevated fasting blood glucose and CSF WBC count to the CASE model could improve the predictive ability of the existing grading scale.
Data Availability Statement
The data supporting the findings of this study are available from the corresponding authors, upon reasonable request.
Ethics Statement
The studies involving human participants were reviewed and approved by the Institutional Ethics Board of Tongji Hospital Tongji Medical College of Huazhong University of Science and Technology (ID: TJ-IRB20211221). Written informed consent from the participants’ legal guardian/next of kin was not required to participate in this study in accordance with the national legislation and the institutional requirements.
Author Contributions
CW designed the study, acquired data, performed the analysis and drafted the work; YF analyzed and interpreted of the data; YZ and HW acquired data; SH designed the study. SH and SZ led the study, critically reviewed and revised the manuscript. All authors contributed to the article and approved the submitted version.
Funding
This study was supported by the Hubei Technological Innovation Special Fund (CN) [Grant NO.2019ACA132] and Hubei Natural Science Foundation Grant NO. 2020CFB805.
Conflict of Interest
The authors declare that the research was conducted in the absence of any commercial or financial relationships that could be construed as a potential conflict of interest.
Publisher’s Note
All claims expressed in this article are solely those of the authors and do not necessarily represent those of their affiliated organizations, or those of the publisher, the editors and the reviewers. Any product that may be evaluated in this article, or claim that may be made by its manufacturer, is not guaranteed or endorsed by the publisher.
Acknowledgments
The authors would like to thank all the participants for their valuable data, cooperation, and participation.
Supplementary Material
The Supplementary Material for this article can be found online at: https://www.frontiersin.org/articles/10.3389/fimmu.2022.916111/full#supplementary-material
Abbreviations
ADEM, acute disseminated encephalomyelitis; AE, autoimmune encephalitis; AIC, Akaike’s information criterion; ALE, autoimmune limbic encephalitis; AMPA, α-amino-3-hydroxy-5-methyl-4-isoxazolepropionic acid; ASMs, anti-seizure medications; AUC, area under the receiver operating characteristic (ROC) curve; CASE, the clinical assessment scale in autoimmune encephalitis; CASPR, contactin associated protein; CSF, cerebrospinal fluid; D2R, Dopamine 2 receptor; DPPX, dipeptidyl-peptidase-like protein; EEG, electroencephalography; GABA, gamma-aminobutyric acid; GAD, glutamic acid decarboxylase; GBS, Guillain–Barre syndrome; ICU, intensive care unit; LGI1, leucine-rich glioma inactivated 1; MG, myasthenia gravis; mGluR, metabotropic glutamate receptor; MOG, myelin oligodendrocyte glycoprotein; MRI, magnetic resonance imaging; mRS, the modified Rankin Scale; NMDA, N-methyl-D-aspartate; WBC, white blood cell.
References
1. Diaz-Arias LA, Pardo CA, Probasco JC. Autoimmune Encephalitis in the Intensive Care Unit. Neurointensive Care Unit (2020) 249–63. doi: 10.1007/978-3-030-36548-6_17
2. Schubert J, Brämer D, Huttner HB, Gerner ST, Fuhrer H, Melzer N, et al. Management and Prognostic Markers in Patients With Autoimmune Encephalitis Requiring ICU Treatment. Neurol Neuroimmunol Neuroinflamm (2019) 6(1):e514. doi: 10.1212/nxi.0000000000000514
3. Graus F, Titulaer MJ, Balu R, Benseler S, Bien CG, Cellucci T, et al. A Clinical Approach to Diagnosis of Autoimmune Encephalitis. Lancet Neurol (2016) 15(4):391–404. doi: 10.1016/s1474-4422(15)00401-9
4. Mittal MK, Rabinstein AA, Hocker SE, Pittock SJ, Wijdicks EF, McKeon A. Autoimmune Encephalitis in the ICU: Analysis of Phenotypes, Serologic Findings, and Outcomes. Neurocrit Care (2016) 24(2):240–50. doi: 10.1007/s12028-015-0196-8
5. Harutyunyan G, Hauer L, Dünser MW, Moser T, Pikija S, Leitinger M, et al. Risk Factors for Intensive Care Unit Admission in Patients With Autoimmune Encephalitis. Front Immunol (2017) 8:835. doi: 10.3389/fimmu.2017.00835
6. Broadley J, Wesselingh R, Seneviratne U, Kyndt C, Beech P, Buzzard K, et al. Prognostic Value of Acute Cerebrospinal Fluid Abnormalities in Antibody-Positive Autoimmune Encephalitis. J Neuroimmunol (2021) 353:577508. doi: 10.1016/j.jneuroim.2021.577508
7. Broadley J, Wesselingh R, Seneviratne U, Kyndt C, Beech P, Buzzard K, et al. Peripheral Immune Cell Ratios and Clinical Outcomes in Seropositive Autoimmune Encephalitis: A Study by the Australian Autoimmune Encephalitis Consortium. Front Immunol (2020) 11:597858. doi: 10.3389/fimmu.2020.597858
8. Levraut M, Bourg V, Capet N, Delourme A, Honnorat J, Thomas P, et al. Cerebrospinal Fluid IL-17a Could Predict Acute Disease Severity in Non-NMDA-Receptor Autoimmune Encephalitis. Front Immunol (2021) 12:673021. doi: 10.3389/fimmu.2021.673021
9. Sonneville R, Gault N, de Montmollin E, Klein IF, Mariotte E, Chemam S, et al. Clinical Spectrum and Outcomes of Patients With Encephalitis Requiring Intensive Care. Eur J Neurol (2015) 22(1):6–16.e1. doi: 10.1111/ene.12541
10. Krishnan S, Sarma G, Sarma S, Kishore A. Do Nonmotor Symptoms in Parkinson's Disease Differ From Normal Aging? Mov Disord (2011) 26(11):2110–3. doi: 10.1002/mds.23826
11. Lee SA, Choi EJ, Jeon JY, Han SH, Kim HW, Lee GH, et al. Insomnia Moderates the Association Between Recurrent Seizures and Emotional Instability in Persons With Epilepsy. Epilepsy Behav (2021) 125:108414. doi: 10.1016/j.yebeh.2021.108414
12. Ryu WS, Hong KS, Jeong SW, Park JE, Kim BJ, Kim JT, et al. Association of Ischemic Stroke Onset Time With Presenting Severity, Acute Progression, and Long-Term Outcome: A Cohort Study. PLoS Med (2022) 19(2):e1003910. doi: 10.1371/journal.pmed.1003910
13. Titulaer MJ, McCracken L, Gabilondo I, Armangué T, Glaser C, Iizuka T, et al. Treatment and Prognostic Factors for Long-Term Outcome in Patients With Anti-NMDA Receptor Encephalitis: An Observational Cohort Study. Lancet Neurol (2013) 12(2):157–65. doi: 10.1016/s1474-4422(12)70310-1
14. Pruetarat N, Netbaramee W, Pattharathitikul S, Veeravigrom M. Clinical Manifestations, Treatment Outcomes, and Prognostic Factors of Pediatric Anti-NMDAR Encephalitis in Tertiary Care Hospitals: A Multicenter Retrospective/Prospective Cohort Study. Brain Dev (2019) 41(5):436–42. doi: 10.1016/j.braindev.2018.12.009
15. van Swieten JC, Koudstaal PJ, Visser MC, Schouten HJ, van Gijn J. Interobserver Agreement for the Assessment of Handicap in Stroke Patients. Stroke (1988) 19(5):604–7. doi: 10.1161/01.str.19.5.604
16. Lim JA, Lee ST, Moon J, Jun JS, Kim TJ, Shin YW, et al. Development of the Clinical Assessment Scale in Autoimmune Encephalitis. Ann Neurol (2019) 85(3):352–8. doi: 10.1002/ana.25421
17. Cai MT, Lai QL, Zheng Y, Fang GL, Qiao S, Shen CH, et al. Validation of the Clinical Assessment Scale for Autoimmune Encephalitis: A Multicenter Study. Neurol Ther (2021) 10(2):985–1000. doi: 10.1007/s40120-021-00278-9
18. Zhang Y, Tu E, Yao C, Liu J, Lei Q, Lu W. Validation of the Clinical Assessment Scale in Autoimmune Encephalitis in Chinese Patients. Front Immunol (2021) 12:796965. doi: 10.3389/fimmu.2021.796965
19. Jeon CY, Neidell M, Jia H, Sinisi M, Larson E. On the Role of Length of Stay in Healthcare-Associated Bloodstream Infection. Infect Control Hosp Epidemiol (2012) 33(12):1213–8. doi: 10.1086/668422
20. Abboud H, Probasco J, Irani SR, Ances B, Benavides DR, Bradshaw M, et al. Autoimmune Encephalitis: Proposed Recommendations for Symptomatic and Long-Term Management. J Neurol Neurosurg Psychiatry (2021) 92(8):897–907. doi: 10.1136/jnnp-2020-325302
21. Tan C, Jiang Y, Zhong M, Hu Y, Hong S, Li X, et al. Clinical Features and Outcomes in Pediatric Autoimmune Encephalitis Associated With CASPR2 Antibody. Front Pediatr (2021) 9:736035. doi: 10.3389/fped.2021.736035
22. Choi H, Detyniecki K, Bazil C, Thornton S, Crosta P, Tolba H, et al. Development and Validation of a Predictive Model of Drug-Resistant Genetic Generalized Epilepsy. Neurology (2020) 95(15):e2150–e60. doi: 10.1212/wnl.0000000000010597
23. DeLong ER, DeLong DM, Clarke-Pearson DL. Comparing the Areas Under Two or More Correlated Receiver Operating Characteristic Curves: A Nonparametric Approach. Biometrics (1988) 44(3):837–45. doi: 10.2307/2531595
24. Yu Y, Wu Y, Cao X, Li J, Liao X, Wei J, et al. The Clinical Features and Prognosis of Anti-NMDAR Encephalitis Depends on Blood Brain Barrier Integrity. Mult Scler Relat Disord (2021) 47:102604. doi: 10.1016/j.msard.2020.102604
25. Yang RN, Ge FF, Jiang JW, Wang Y, Zhang W. [Disease Characteristics, Treatment, and Prognosis of Chinese Patients With Autoimmune Encephalitis: A Retrospective Study]. Sichuan Da Xue Xue Bao Yi Xue Ban (2022) 53(1):142–8. doi: 10.12182/20220160206
26. Joubert B, Dalmau J. The Role of Infections in Autoimmune Encephalitides. Rev Neurol (Paris) (2019) 175(7-8):420–6. doi: 10.1016/j.neurol.2019.07.004
27. Venkatesan A, Benavides DR. Autoimmune Encephalitis and its Relation to Infection. Curr Neurol Neurosci Rep (2015) 15(3):3. doi: 10.1007/s11910-015-0529-1
28. Armangue T, Spatola M, Vlagea A, Mattozzi S, Cárceles-Cordon M, Martinez-Heras E, et al. Frequency, Symptoms, Risk Factors, and Outcomes of Autoimmune Encephalitis After Herpes Simplex Encephalitis: A Prospective Observational Study and Retrospective Analysis. Lancet Neurol (2018) 17(9):760–72. doi: 10.1016/s1474-4422(18)30244-8
29. Linnoila J, Pulli B, Armangué T, Planagumà J, Narsimhan R, Schob S, et al. Mouse Model of Anti-NMDA Receptor Post-Herpes Simplex Encephalitis. Neurol Neuroimmunol Neuroinflamm (2019) 6(2):e529. doi: 10.1212/nxi.0000000000000529
30. Sakaguchi H, Yamashita S, Hirano T, Nakajima M, Kimura E, Maeda Y, et al. Myasthenic Crisis Patients Who Require Intensive Care Unit Management. Muscle Nerve (2012) 46(3):440–2. doi: 10.1002/mus.23445
31. Nithyashree N, Dhanaraj M, Kumar S, Saraswathi MB. Factors Predicting Poor Outcome in Patients With Fulminant Guillaine-Barré Syndrome. Ann Indian Acad Neurol (2014) 17(4):463–5. doi: 10.4103/0972-2327.144040
32. Palace JA, Hughes RA. Guillain-Barré Syndrome With Severe Persistent Disability: Relationship to Hyperacute Guillain-Barré Syndrome. Eur J Neurol (1994) 1(1):21–7. doi: 10.1111/j.1468-1331.1994.tb00046.x
33. Baunbæk Egelund G, Ertner G, Langholz Kristensen K, Vestergaard Jensen A, Benfield TL, Brandt CT. Cerebrospinal Fluid Pleocytosis in Infectious and Noninfectious Central Nervous System Disease: A Retrospective Cohort Study. Medicine (2017) 96(18):e6686. doi: 10.3389/fimmu.2021.774664
34. Marik PE, Bellomo R. Stress Hyperglycemia: An Essential Survival Response! Crit Care (2013) 17(2):305. doi: 10.1186/cc12514
35. Shan W, Yang H, Wang Q. Neuronal Surface Antibody-Medicated Autoimmune Encephalitis (Limbic Encephalitis) in China: A Multiple-Center, Retrospective Study. Front Immunol (2021) 12:621599. doi: 10.3389/fimmu.2021.621599
36. Balu R, McCracken L, Lancaster E, Graus F, Dalmau J, Titulaer MJ. A Score That Predicts 1-Year Functional Status in Patients With Anti-NMDA Receptor Encephalitis. Neurology (2019) 92(3):e244–e52. doi: 10.1212/wnl.0000000000006783
37. Shim Y, Kim SY, Kim H, Hwang H, Chae JH, Choi J, et al. Clinical Outcomes of Pediatric Anti-NMDA Receptor Encephalitis. Eur J Paediatr Neurol (2020) 29:87–91. doi: 10.1016/j.ejpn.2020.10.001
38. Cohen J, Sotoca J, Gandhi S, Yeshokumar AK, Gordon-Lipkin E, Geocadin RG, et al. Autoimmune Encephalitis: A Costly Condition. Neurology (2019) 92(9):e964–e72. doi: 10.1212/wnl.0000000000006990
39. Li A, Gong X, Guo K, Lin J, Zhou D, Hong Z. Direct Economic Burden of Patients With Autoimmune Encephalitis in Western China. Neurol Neuroimmunol Neuroinflamm (2020) 7(6):e891. doi: 10.1212/nxi.0000000000000891
Keywords: autoimmune encephalitis, intensive care unit, risk factor, prediction, model
Citation: Wu C, Fang Y, Zhou Y, Wu H, Huang S and Zhu S (2022) Risk Prediction Models for Early ICU Admission in Patients With Autoimmune Encephalitis: Integrating Scale-Based Assessments of the Disease Severity. Front. Immunol. 13:916111. doi: 10.3389/fimmu.2022.916111
Received: 08 April 2022; Accepted: 16 May 2022;
Published: 10 June 2022.
Edited by:
Barbara M. P. Willekens, Antwerp University Hospital, BelgiumReviewed by:
Jeroen Kerstens, Antwerp University Hospital, BelgiumJustin R. Abbatemarco, Cleveland Clinic, United States
Copyright © 2022 Wu, Fang, Zhou, Wu, Huang and Zhu. This is an open-access article distributed under the terms of the Creative Commons Attribution License (CC BY). The use, distribution or reproduction in other forums is permitted, provided the original author(s) and the copyright owner(s) are credited and that the original publication in this journal is cited, in accordance with accepted academic practice. No use, distribution or reproduction is permitted which does not comply with these terms.
*Correspondence: Shanshan Huang, c2hhbmFodWFuZzNAZ21haWwuY29t; Suiqiang Zhu, emh1c3VpcWlhbmdAMTYzLmNvbQ==