- 1Department of Respiratory Medicine, Graduate School of Medical Sciences, Kyushu University, Fukuoka, Japan
- 2Division of Immunogenetics, Department of Immunobiology and Neuroscience, Medical Institute of Bioregulation, Kyushu University, Fukuoka, Japan
- 3Division of Immunology and Genome Biology, Department of Molecular Genetics, Medical Institute of Bioregulation, Kyushu University, Fukuoka, Japan
Immune cells have been implicated in interstitial lung diseases (ILDs), although their phenotypes and effector mechanisms remain poorly understood. To better understand these cells, we conducted an exploratory mass cytometry analysis of immune cell subsets in bronchoalveolar lavage fluid (BALF) from patients with idiopathic pulmonary fibrosis (IPF), connective-tissue disease (CTD)-related ILD, and sarcoidosis, using two panels including 64 markers. Among myeloid cells, we observed the expansion of CD14+ CD36hi CD84hiCCR2– monocyte populations in IPF. These CD14+ CD36hi CD84hi CCR2– subsets were also increased in ILDs with a progressive phenotype, particularly in a case of acute exacerbation (AEx) of IPF. Analysis of B cells revealed the presence of cells at various stages of differentiation in BALF, with a higher percentage of IgG memory B cells in CTD-ILDs and a trend toward more FCRL5+ B cells. These FCRL5+ B cells were also present in the patient with AEx-IPF and sarcoidosis with advanced lung lesions. Among T cells, we found increased levels of IL-2R+ TIGIT+ LAG3+ CD4+ T cells in IPF, increased levels of CXCR3+ CD226+ CD4+ T cells in sarcoidosis, and increased levels of PD1+ TIGIT+ CD57+ CD8+ T cells in CTD-ILDs. Together, these findings underscore the diverse immunopathogenesis of ILDs.
Introduction
Interstitial lung disease (ILD) is a broad term for a group of disorders characterized by varying degrees of inflammation and scarring or fibrosis of the lung (1). In the majority of cases, ILD is a chronic disease with progressive scarring of lung tissue. Idiopathic pulmonary fibrosis (IPF) is the most common form of ILD and has a median survival rate of 3–5 years (2). Connective tissue disease (CTD), defined as systemic disorders characterized by autoimmune-mediated organ damage and circulating autoantibodies, is one of the common systemic diseases associated with ILD (3). Sarcoidosis is a granulomatous disorder of uncertain etiology, impacting various organ systems. Pulmonary involvement, including ILDs, represents the majority of morbidity and mortality associated with sarcoidosis (4).
It has been suggested that immune cells are involved in the pathogenesis of ILDs (4–7), with various subsets of immune cells potentially contributing to the development of ILDs, particularly macrophages and lymphoid cells. Macrophages, the most abundant immune cells in the lungs (8), have been shown to play a key role in the initiation and progression of fibrotic responses (9). The ability of macrophages to alter their functional traits in response to external stimuli allows them to exhibit a range of biological impacts. Recent studies have shown the existence of a unique population of macrophages in IPF lung explants (10–12) and murine models of pulmonary fibrosis (13, 14). T cells have also been implicated in the development of ILDs (6, 15). In addition, increasing evidence suggests that pathogenic B cells may contribute to the development of autoimmune diseases (16–18), although their role in the development of ILDs remains poorly understood. The inability to fully characterize immune cells in clinical specimens is partially due to a deficiency in available assays. While next-generation sequencing analysis has facilitated the thorough analysis of cells, there is a constraint on the number that can be examined. It can be challenging to accurately classify the type of immune cells solely based on transcripts. Mass cytometry, on the other hand, offers an in-depth, high-dimensional description of the immune cell population through the simultaneous utilization of multiple markers (19), which will significantly alter our understanding of ILDs.
The objective of this study is to utilize mass cytometry to phenotype macrophages, B cells, and T cells in bronchoalveolar lavage fluid (BALF) cells from patients with IPF, CTD-ILD, and sarcoidosis. By employing both unbiased and manually classified methods, we functionally characterize subpopulations of myeloid cells, B cells, and T cells that are overexpressed in each disease, thereby identifying cells that may potentially affect disease progression.
Materials and methods
Patients
Patients who underwent BALF collection and were newly diagnosed with IPF, CTD-ILD, and sarcoidosis between Jan 2017 and April 2022 at Kyushu University Hospital were eligible for enrollment in the study. The study was authorized by the Ethics Committee of Kyushu University Hospital (reference number 22117-00). The diagnostic criteria for IPF, CTD-ILD, and sarcoidosis were as described elsewhere (20–24). Interstitial pneumonia with autoimmune features (IPAF) was included in CTD-ILD in this study. Disease progression was defined as follows: a relative decline of at least 10% in the predicted value of forced vital capacity (FVC), a relative decline of 5-10% in the predicted value of FVC accompanied by worsening respiratory symptoms or increased lung involvement on high-resolution CT imaging, or worsening respiratory symptoms and increased lung involvement within 24 months, as modified by the inclusion criteria of the INBUILD trial (25). The experimental and analytical workflow is shown in Figure 1.
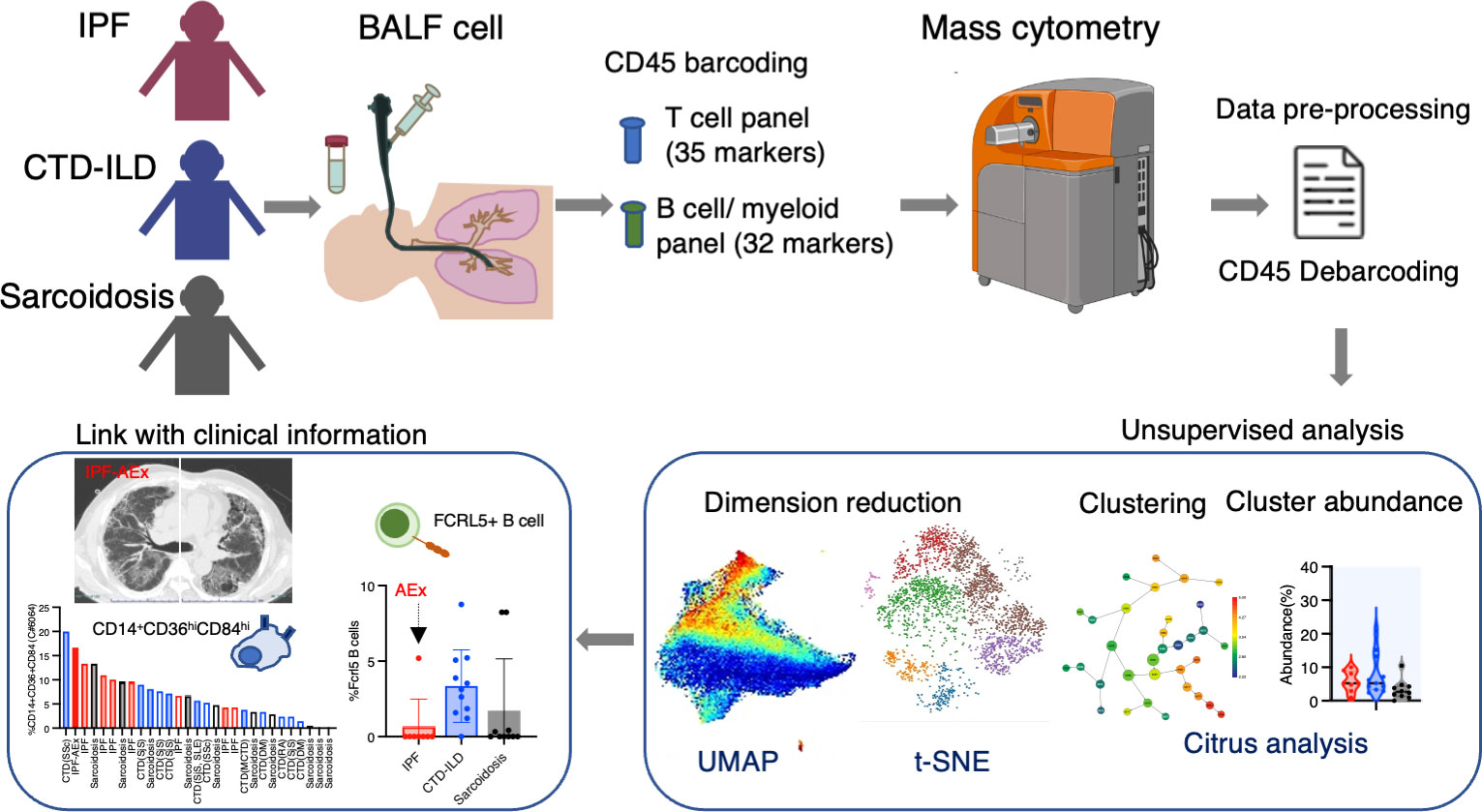
Figure 1 Graphical abstract of the study. Bronchoalveolar lavage fluid (BALF) samples were collected from patients with idiopathic pulmonary fibrosis (IPF), connective-tissue disease (CTD)-related ILD, and sarcoidosis. Following CD45 barcoding for individual sample identification, BALF cells were analyzed with a T cell panel (35 markers) and B cell/myeloid cell panel (32 markers) using mass cytometry. The expansion of CD14+ CD36hi CD84hi monocyte populations was found in IPF and ILDs with a progressive phenotype. FCRL5+ B cells were increased in CTD-ILDs, acute exacerbation (AEx) of IPF, and sarcoidosis with advanced lung lesions.
Mass cytometry
Antibodies were purchased either already metal-tagged (Standard Biotools) or in purified form (Supplementary Table 1). Purified antibodies were conjugated with metals using the Maxpar Antibody Labeling Kit (Standard Biotools) according to the manufacturer’s instructions and stored at 4°C. The cell labeling was performed as previously described (26). Briefly, cryopreserved BALF cells in Cellbanker1 (Takara #210409) were thawed in PBS and stained with Cell-ID™ Cisplatin-198Pt (Standard Biotools #201198, 1:2000 dilution) in PBS. Cells were incubated with FcR blocking reagent (Miltenyi, #130-059-901) and barcoded with each metal-labeled CD45 antibodies (Supplementary Table 1). After washing, CD45-labelled cells were mixed (maximum 6 samples together) and stained with APC-conjugated FCRL5 antibodies (BioLegend #340305)(for panel #2), followed by staining antibody cocktail (Panels #1 and #2, see Supplementary Table 1). The volume of antibodies was determined by preliminary experiments with metal minus one. After staining, cells were washed, fixed with 1.6% formaldehyde, and resuspended in Cell-ID Intercalator 103Rh (Standard Biotools #201103A) in Fix and Perm buffer (Standard Biotools) at 4°C overnight. For acquisition, cells were resuspended in MaxPar Cell Acquisition Solution (Standard Biotools #201240) containing one-fifth EQ Four Element Calibration Beads (Standard Biotools #201078) and were acquired at a rate of 200–300 events/second on a Helios mass cytometer (Standard Biotools). Files were converted to FCS, randomized, and normalized for EQ bead intensity using the Helios software. Concatenating fcs files in the same group into one file was conducted by FlowJo v10.8 (BD Biosciences). Manual gating, visualization of t-distributed stochastic neighbor embedding (tSNE), Uniform manifold approximation and projection (UMAP) analysis, and Citrus analysis (27) were performed using Cytobank Premium (Cytobank Inc.).
Data analysis
Live cells were selected by the exclusion of cisplatin-positive cells and doublets. CD45+ were selected and further analyzed. We first conducted principal component analysis (PCA) using median expression as a univariate summary for each marker on total cells, myeloid cells (CD11b+CD11c+), and T cells (CD2+CD3+) in the three diseases of interest (IPF, CTD-ILD, and sarcoidosis) using R package factoextra, ggplot2, ggplotly in RStudio (version 2022.12.0 + 353 with R version 4.2.2) to compare samples at a gross level (Supplementary Figure 1). For myeloid cells, CD11b+CD11c+ cells were gated, and the Citrus algorithm was conducted with clustering channels of CD11b, CD11c, CD64, CD14, CD16, CD32, CD36, CD38, CD84, CD86, CD163, CD206, CD209, CD223, HLA-DR, CCR2, CCR5, ST2 using following parameters: association models = nearest shrunken centroid (PAMR), cluster characterization = abundance, minimum cluster size = 5%, cross-validation folds = 5, false discovery rate = 1%. Two cases of CTD-ILDs (dermatomyositis-related, IgG4-related) were excluded due to low cell numbers to calculate clusters. A UMAP analysis was performed on myeloid cells, incorporating clustering channels of CD11b, CD11c, CD64, CD14, CD16, CD206, HLA-DR, and CCR2 with the following parameters: numbers of neighbors = 10, minimum distance = 0.01 (comparison between IPF, CTD-ILD, and sarcoidosis), or with clustering channels of CD11b, CD11c, CD64, CD14, CD16, CD32, CD36, CD38, CD84, CD86, CD163, CD206, CD209, CD223, TIM-1, HLA-DR, CCR2, CCR5, ST2 using following parameters: numbers of neighbors = 15, minimum distance = 0.01 (comparison among IPF cases).
For B cells, CD3–CD64– and CD19+ or CD138+ cells were gated, and viSNE was conducted with clustering channels of CD19, CD38, CD11c, IgA, IgG, CD138, CD21, ST2, CXCR5, CD24, CD27, TIM-1, IgM, HLA-DR, IgD, FCRL5 on individual files and concatenated files using following parameters: iterations = 1000, perplexity = 30, theta = 0.5. Two cases of CTD-ILDs (dermatomyositis-related, IgG4-related) were excluded due to low cell numbers to calculate clusters.
For T cells, the CD2+CD3+ cell population was selectively gated in concatenated files. A UMAP analysis was performed on T cells, incorporating clustering channels of CD4, CD8, CD45RA, CD45RO, CCR7, CD28, and Fas, with the following parameters: numbers of neighbors = 10, minimum distance = 0.01. The Citrus algorithm was conducted with clustering channels of CD4, CD5, CD7, CD8a, CD11a, CD16, CD27, CD28, CD44, CD45RA, CD45RO, CD49d, CD57, CD69, CD226, Fas, IL-2R, PD-L1, PD-L2, PD-1, OX40, TIGIT, TIM3, CTLA-4, CD223 (LAG-3), BTLA, ICOS, ST2, CCR7, CXCR3, HLA-DR using following parameters: association models = nearest shrunken centroid (PAMR), cluster characterization = abundance, minimum cluster size = 5%, cross-validation folds = 5, false discovery rate = 1%. viSNE for T cells was conducted with clustering channels of CD4, CD5, CD7, CD8a, CD11a, CD16, CD27, CD28, CD44, CD45RA, CD45RO, CD49d, CD57, CD69, CD226, Fas, IL-2R, PD-L1, PD-L2, PD-1, OX40, TIGIT, TIM3, CTLA-4, CD223 (LAG-3), BTLA, ICOS, ST2, CCR7, CXCR3, HLA-DR using following parameters: iterations = 1000, perplexity = 30, theta = 0.5. Choosing between UMAP and viSNE as the dimensionality reduction tool was based on their ability to preserve the connections between global structures and the distances between cell groups (UMAP was better than viSNE) and their ability to display a clear, non-overlapping representation of cell subgroups that makes it easier to see the differences between groups (viSNE was better than UMAP).
Heatmaps were generated using the R package ComplexHeatmap in RStudio by calculating the median expression levels of each channel within the Citrus-generated clusters, normalizing the values to a maximum of 100 to denote maximum expression. The numerical values of total cells, T cells, myeloid cells, and B cells in each case are shown in Supplementary Table 2.
Statistical analysis
For the Citrus algorism experiment, we employed a PAMR association model, using a stringent threshold of 1% FDR, as outlined in the Data Analysis section. The Student’s two-tailed unpaired t-test was utilized to perform a comparative analysis between the two groups. The statistical analysis was conducted through a one-way ANOVA, complemented by Tukey’s multiple comparison tests, to determine the significance among the three groups. A two-way ANOVA, accompanied by Tukey’s multiple comparison tests, was performed to evaluate the manually gated cell proportions using GraphPad Prism 9 software. A P-value less than 0.05 was considered to indicate statistical significance.
Results
Patient characteristics
We analyzed 8 cases of IPF, 13 of CTD-ILD, and 10 of sarcoidosis (Table 1). Among the IPF cases, one patient experienced acute exacerbation (AEx) of IPF on admission. Thirteen cases of CTD-ILDs were observed, comprising Sjogren’s syndrome (SjS) (n = 3), dermatomyositis (DM) (n = 3), systemic sclerosis (SSc) (n = 2), mixed connective tissue disease (MCTD) (n = 1), systemic lupus erythematosus (SLE) complicated with adult-onset Still’s disease (AOSD) (n = 1), IgG4-related disease (n = 1), rheumatoid arthritis (RA)(n = 1), and IPAF (n = 1). The presence of autoantibodies and radiological patterns in each of the CTD-ILD cases and the diagnosis of the IPAF case were described in Supplementary Table 3.
Differential cell counts for BALF revealed lymphocytosis in 1 out of 8 cases (12.5%) of IPF as well as in 7 out of 13 cases (53.8%) of CTD-ILD and 7 out of 11 cases (63.6%) of sarcoidosis when the cut-off for the percentage of lymphocytes was set to >20%.
Expansion of CD14+CD36hiCD84hiCCR2– monocytes in BALF from patients with IPF
To investigate myeloid cell populations (identified as CD45+CD11b+CD11c+) that could provide insight into ILD conditions, we first conducted UMAP to see the major myeloid populations. The UMAP plot categorized 4 major subtypes, monocytes, CCR2+ macrophages, alveolar macrophages, dendritic cells, with no significant difference in IPF, CTD-ILD, and sarcoidosis (Figures 2A, B). We next utilized the Citrus algorithm to further investigate differently abundant myeloid cell subpopulations through the analysis of 18 parameters. Our analysis identified 33 clusters of myeloid cells, of which 23 were significantly differentiated between the groups (Figure 2C and Supplementary Figure 2). Clusters #6074, #6025, #6066, and #6054 were prevalent in sarcoidosis and characterized by CD64+ CD11blo CD14– CD223(LAG3)+ HLA-DR+ CD163hi expression (Figures 2D, E). Cluster #6059, which was abundant in CTD-ILD, was marked by CD64+ CD11c+ CD11b+ CD38hi expression (Figures 2D, E). Clusters #6075, #6064, and #6056, which were prevalent in IPF, were comprised of CD64+ CD11bhi CD11chi CD14+ CD36hi CD84hi CCR2– monocyte subpopulations (Figures 2D, E), different from CD14+CCR2+ monocyte subpopulations (clusters #6078, #6082) (Figures 2D, 3A). A recent report indicated that CD36hi CD84hi macrophages were increased in IPF compared to control and COPD lungs (28), which is consistent with our findings. Notably, the CD14+ CD36hi CD84hi CCR2– monocyte subpopulation was also increased in ILDs with a progressive phenotype (Figures 3B, C), suggesting that these cells may have pathogenic properties.
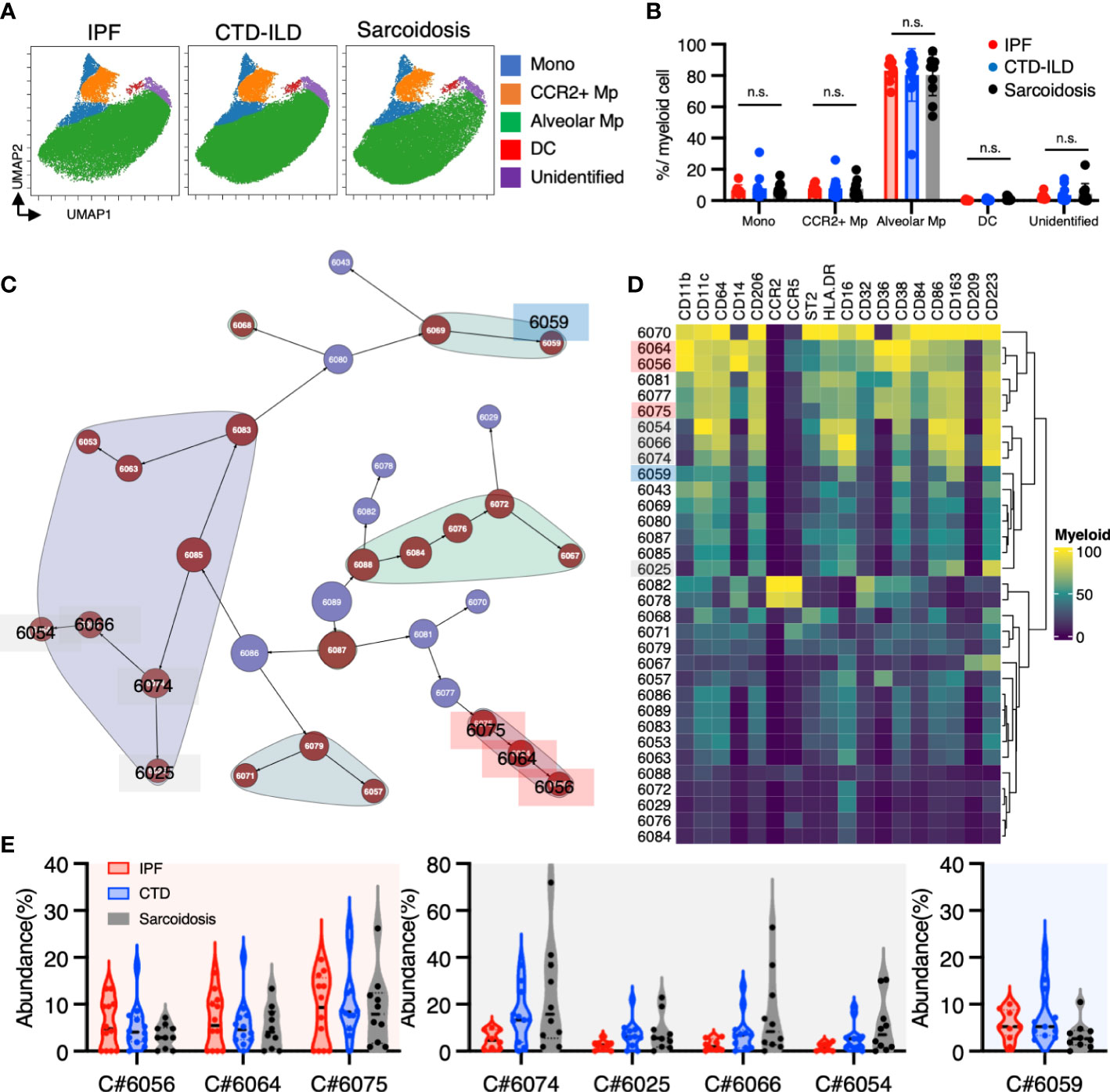
Figure 2 Characterization of myeloid cell subsets in BALF from patients with IPF, CTD-ILD, and sarcoidosis. (A) UMAP plots of concatenated samples visualizing the distribution of CD11b+CD11c+ myeloid cell subpopulations in BALF from patients with IPF, CTD-ILD, and sarcoidosis. Monocytes are defined by CD64+CD14+, CCR2+ macrophages (Mp) by CCR2+ CD64+ CD14–, Alveolar Mp by CD64+CD206+, dendritic cells (DC) by CD64– CD206– CD11c+ HLA-DR+, unidentified cells by CD64– CD206– CD11clo HLA-DR–. (B) The proportions of myeloid cell subpopulations in IPF, CTD-ILD, and sarcoidosis. Graphical plots represent individual samples. Statistical differences were analyzed by two-way ANOVA followed by Tukey’s multiple comparison test. n.s. not significant. (C) Citrus network tree visualizing the hierarchical relationship and intensity of each marker between identified myeloid cell populations gated by CD45+CD11b+ CD11c+ from IPF (n = 8), CTD-ILD (n = 11), and sarcoidosis (n = 10). Clusters with significant differences were represented in red, and those without significant differences in blue. Circle size reflects the number of cells within a given cluster. (D) Heatmap illustrating the expression markers across different clusters of myeloid cells as determined by the Citrus analysis. (E) Citrus-generated violin plots for eight representative and differentially regulated populations. Each cluster number (C#) corresponds to the number shown in panel (C). All differences in abundance were significant at a false discovery rate < 0.01.
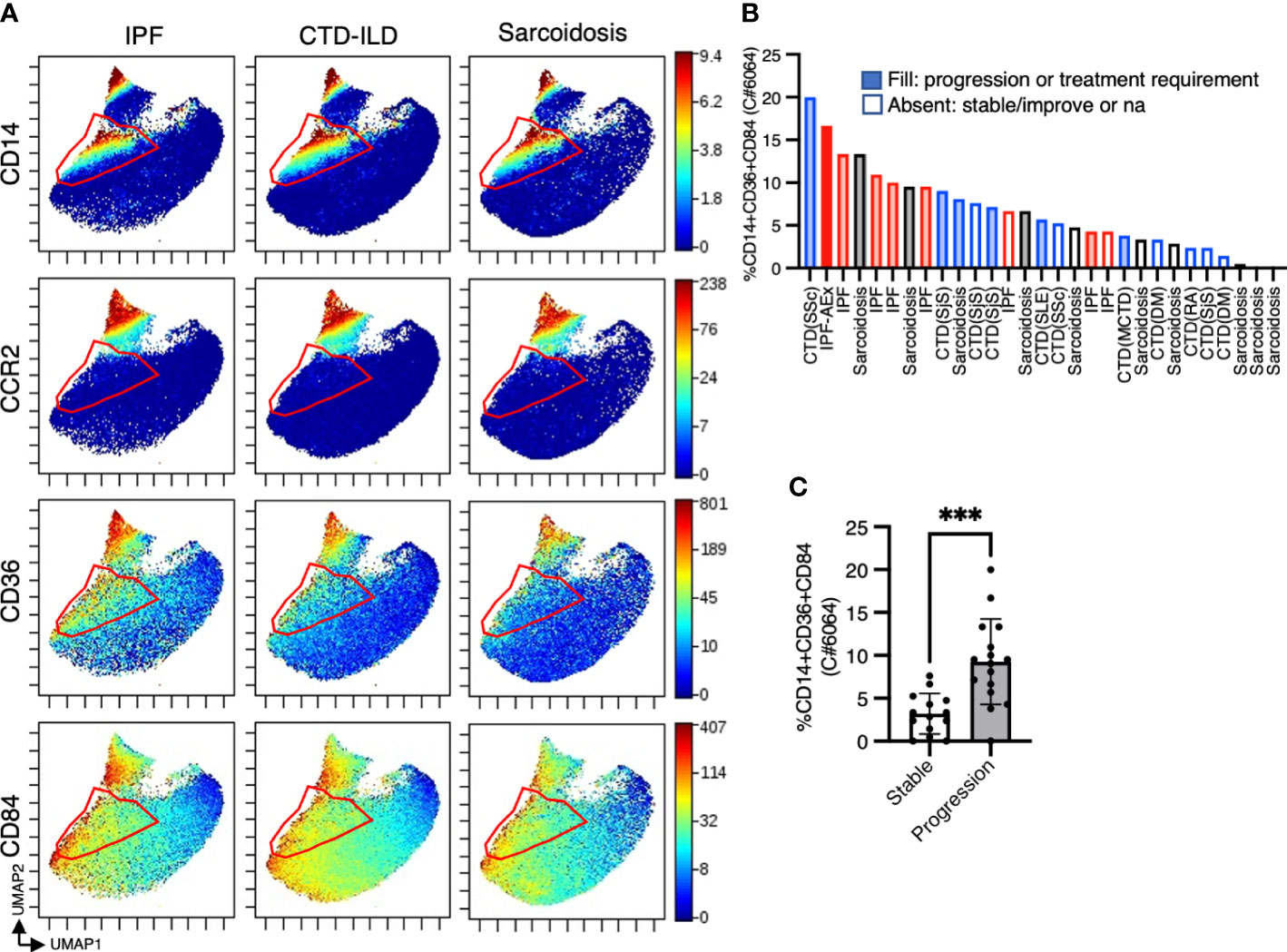
Figure 3 The proportion of CD14+CD36hiCD84hi monocytes was correlated with disease progression. (A) UMAP plots showing CD14, CCR2, CD36, and CD84 expression in myeloid cells. Red triangles indicate CD14+ CCR2– cell subpopulations. (B) The proportion of CD14+ CD36hi CD84hi monocytes (cluster #6064 defined by the Citrus analysis in Figure 2C) abundance in myeloid cell populations in individual samples and (C) the correlation with disease progression. *** p < 0.001. Information for disease with clinical progression is also shown. IPF, idiopathic pulmonary fibrosis; CTD-ILD, connective-tissue disease-related interstitial lung disease; AEx, acute exacerbation; SSc, systemic sclerosis; SjS, Sjogren syndrome; SLE, systemic lupus erythematosus; RA, rheumatoid arthritis; DM, dermatomyositis; MCTD, mixed connective tissue disease; na, not accessed.
B cell subpopulations in the lungs of ILDs
Next, we sought to determine whether there were differential representations of B cell subsets in BALF cells of individuals with ILDs. Utilizing CD45+CD3–CD64– and CD19+ or CD138+ as gating parameters, we were able to identify both B cells and plasma cells. It was observed that B cells tended to be more prevalent in individuals with CTD-ILDs and sarcoidosis compared to those with IPF, although the percentages of B cells/plasma cells remained low across all groups (Figure 4A). A t-SNE analysis of 17 parameters among B cells/plasma cells revealed the presence of various B cell subpopulations, including IgD+ naïve B cells, IgM+ memory B cells, IgG+ memory B cells, IgA+ memory B cells, IgD–CD27– double negative (DN) B cells, plasmablasts, and plasma cells (Figure 4B and Supplementary Figure 4). This marked the first time that these B cell subpopulations were identified. The abundance of these subpopulations varied between groups, with particularly high levels of IgG memory B cells observed in individuals with CTD-ILDs compared to the other groups (Figure 4C).
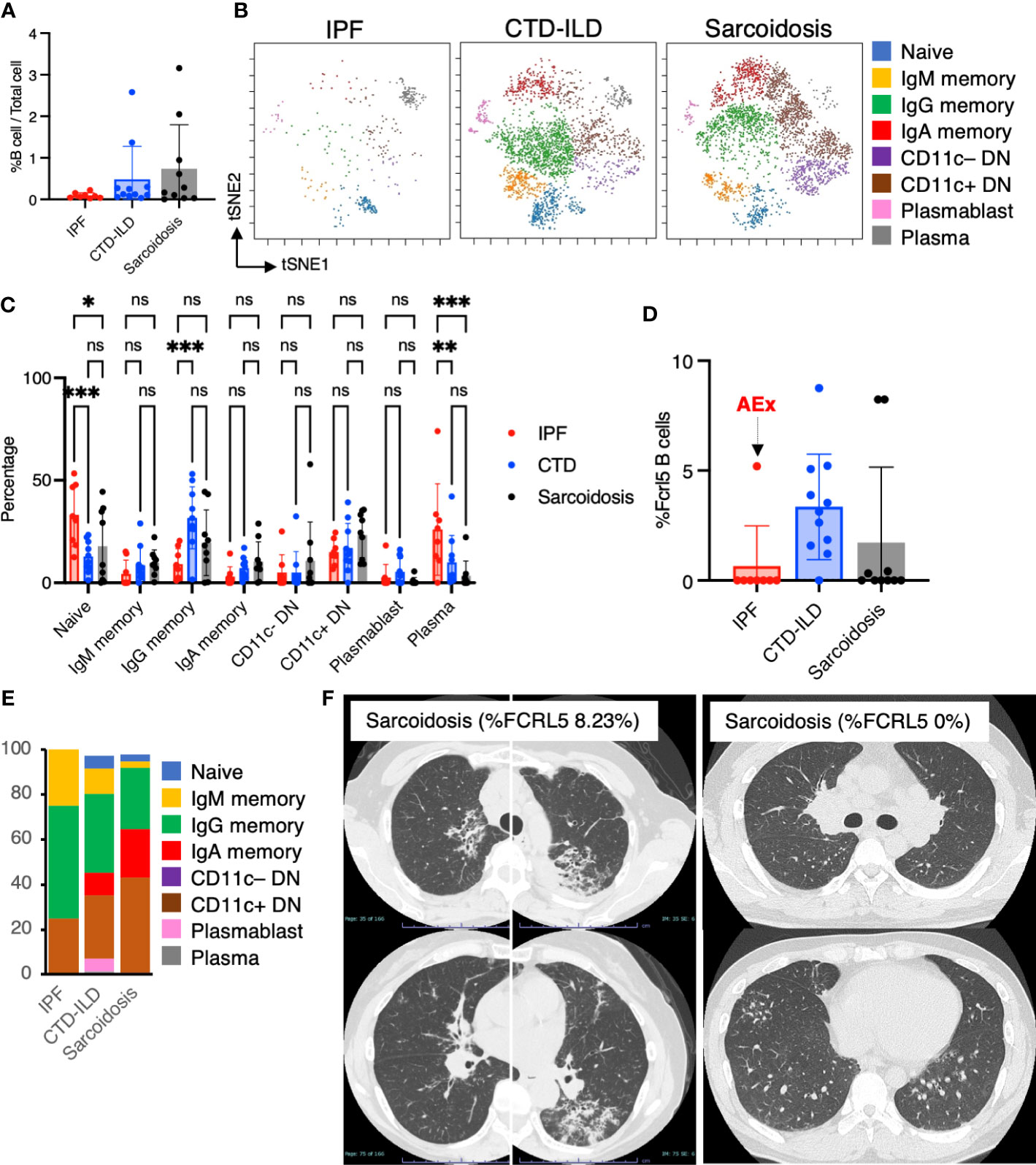
Figure 4 Characterization of B cell subsets in BALF from patients with IPF, CTD-ILD, and sarcoidosis. (A) Percentage of B cells and plasma cells in CD45+ BALF cells. (B) t-stochastic neighborhood embedding (t-SNE) plots of concatenated samples visualizing the distribution of B cell sub-populations in CD64–CD3– and CD19+ or CD138+ gated B cells in BALF from patients with IPF, CTD-ILD, sarcoidosis. Naive B cells are defined by CD19+IgD+, IgM memory B cells: CD19+ IgM+ CD27+, IgG memory B cells: CD19+ IgG+ CD27+, IgA memory B cells: CD19+ IgA+ CD27+, CD11c– double negative (DN) B cells: CD19+ CD11c– IgD– CD27–, CD11c+ DN B cells: CD19+ CD11c+ IgD–CD27–, plasmablasts: CD19+ CD27+ CD38+ CD138–, plasma cells: CD19– CD138+ and IgG+ or IgA+. (C) Percentage of B cell sub-populations in IPF, CTD-ILD, and sarcoidosis. Graphical plots represent individual samples. (D) Percentage of FCRL5 expressing B cells in total B cells. ns: not significant, * p < 0.05, ** p < 0.01, *** p < 0.001. (E) The proportion of each B cell subsets in FCRL5 expressing B cells. (F) Representative chest CT images of patients with sarcoidosis exhibiting a high percentage of FCRL5 B cells and a low percentage of FCRL5 B cells in BALF.
Recent evidence indicated that CD11c+ DN B cells and FCRL5+ B cells are involved in the development of autoimmune conditions (16–18, 29). In this study, we, therefore, sought to examine these B cell subsets. While we did not observe any differences in CD11c+ DN B cells between individuals with IPF, CTD-ILDs, and sarcoidosis (Figure 4C), there was a tendency for FCRL5+ B cells to increase in CTD-ILDs relative to the other groups (Figure 4D). FCRL5+ B cells were mostly IgG+ or IgA+ in each group (IPF: 75%, CTD-ILD: 80.3%, Sarcoidosis: 91.7%) (Figure 4E), suggesting that these FCRL5+ B cells were class-switched B cells. Interestingly, 0% of FCRL5+ B cells were in all IPF cases except for one, which was AEx of IPF. The higher FCRL5+ B cell percentage in sarcoidosis was associated with progressive lung involvement (Figure 4F). Conversely, a patient with sarcoidosis exhibiting a low percentage of FCRL5+ B cells, bilateral hilar lymphadenopathy, and small nodules on CT (Figure 4F) demonstrated marked improvement on follow-up. These findings suggest that FCRL5+ B cells may possess pathogenic properties in CTD-ILDs as well as in the context of AEx of IPF and sarcoidosis.
Characteristic T cell subpopulations in the lungs of ILDs
In addition to myeloid cell and B cell subsets, we investigated whether subsets of T cells differentially existed in ILDs. The prevalence of T cells appeared to be elevated in sarcoidosis and CTD-ILDs compared to IPF, with the CD4/CD8 ratio tending towards an increase in sarcoidosis, although not reaching statistical significance (Figure 5A). To further visualize T cell differentiation within diseased lungs, we constructed UMAP plots (Figure 5B). BALF T cells primarily exhibited memory or effector phenotypes, with a scarcity of naive T cells (Figures 5B, C). Specifically, we observed a higher abundance of transient memory CD4 T cells in sarcoidosis and a higher abundance of effector memory CD4 T cells in IPF (Figure 5C). We next utilized the Citrus algorithm to distinguish differently abundant T cell subpopulations in IPF, CTD-ILD, and sarcoidosis in an unsupervised manner through analysis of 31 parameters (Figure 5D and Supplementary Figure 5). Our analysis identified 31 clusters of T cells, of which 9 were significantly differentiated between the groups. Cluster #5508, prevalent in sarcoidosis, was characterized by CD4+ CD226+ CXCR3+ (Figures 5E, F). Cluster #5520, prevalent in IPF, was comprised of CD4+ IL-2R+ TIGIT+ LAG3+, which are considered to be CD4+ regulatory T cells (Tregs) (Figures 5E, F) (6). Cluster #5527, which was abundant in CTD-ILDs, was marked by CD8+ CD57+ PD-1+ TIGIT+ (Figures 5E, F).
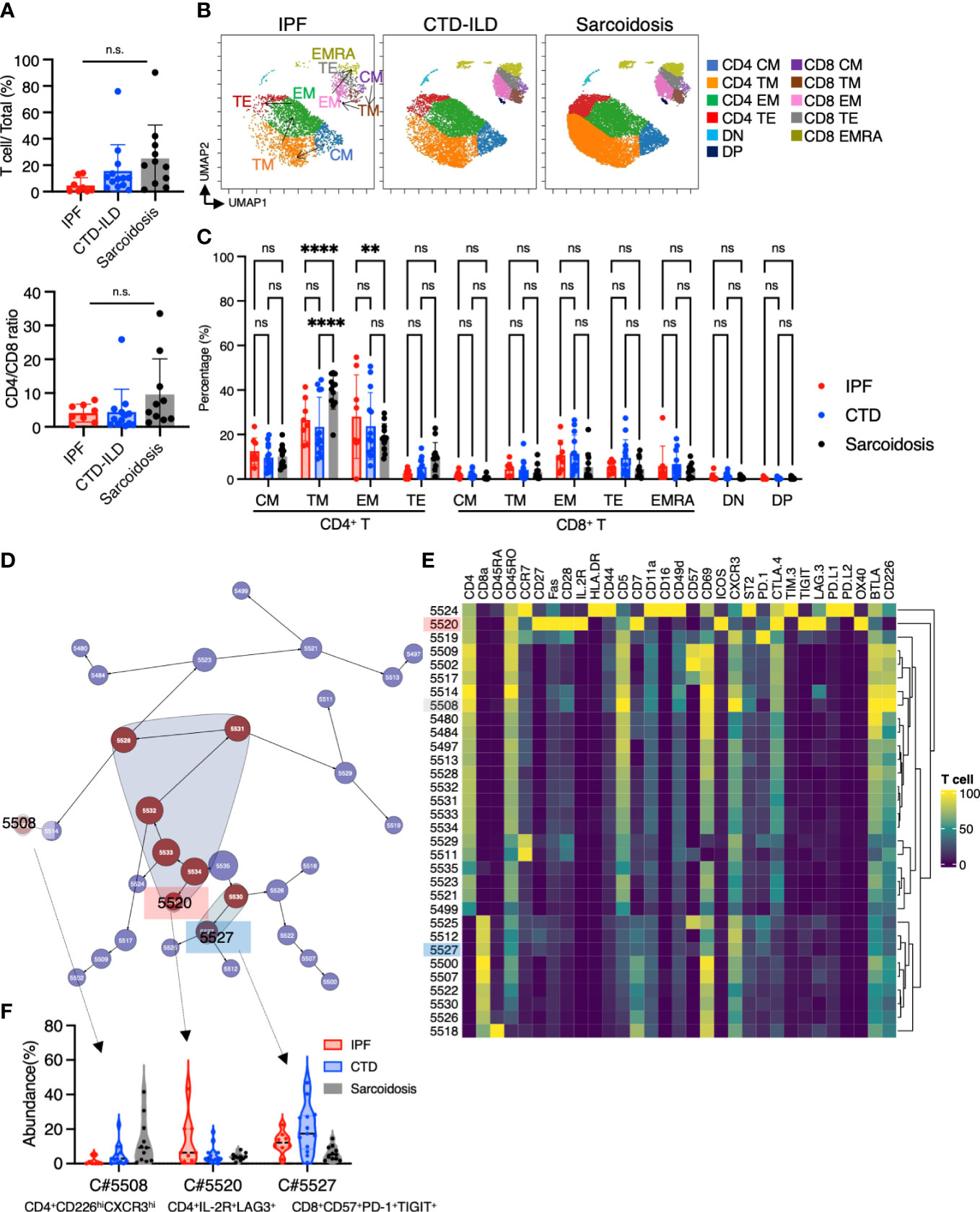
Figure 5 Characterization of T cell subsets in BALF from patients with IPF, CTD-ILD, and sarcoidosis. (A) The proportion of T cells (defined by CD2+CD3+) among CD45+ BALF cells and the CD4+ T cell/CD8+ T cell ratio from IPF (n = 8), CTD-ILD (n = 13), and sarcoidosis (n = 10). ns: not significant. (B) UMAP plots of concatenated samples visualizing the distribution of CD2+CD3+ T cell differentiation in BALF from patients with IPF, CTD-ILD, and sarcoidosis. Central memory (CM) T cells are defined by CCR7+ CD45RO+ CD28+ Fas+, Transitional memory (TM) by CCR7– CD45RO+ CD28+ Fas+, Effector memory (EM) by CCR7– CD45RO+ CD28– Fas+, Terminal effector (TE) by CCR7– CD45RO+/– Fas–, Effector memory RA (EMRA) by CCR7– CD45RO– CD45RA+ Fas+/–. Arrows indicate the trajectory of T-cell differentiation. DN: CD4– CD8– double negative, DP: CD4+ CD8+ double positive. (C) Percentage of T cell subpopulations in IPF, CTD-ILD, and sarcoidosis. Graphical plots represent individual samples. Statistical differences were analyzed by two-way ANOVA followed by Tukey’s multiple comparison test. ns. not significant, ** p < 0.01, **** p < 0.0001. (D) The Citrus network tree displays the hierarchical relationship and intensity of each marker among the T-cell populations in BALF from IPF (n = 8), CTD-ILD (n = 13), and sarcoidosis (n = 10). (E) Heatmap illustrating the expression markers across different clusters of T cells as determined by the Citrus analysis. (F) Citrus-generated violin plots for three representative and differentially regulated populations. Each cluster number (C#) corresponds to the number shown in panel (D). All differences in abundance are significant at a false discovery rate < 0.01.
Immunological phenotypes in a patient with acute exacerbation of IPF
One of the patients suffering from IPF experienced an acute exacerbation. The patient complained of worsening dyspnea upon exertion and a dry cough. Upon admission, chest CT images revealed the emergence of bilateral diffuse ground glass opacities superimposed on a honeycomb pattern, accompanied by peripheral traction bronchiectasis primarily in the basal lungs (Figure 6A). The patient’s blood procalcitonin, beta-D-glucan, and cytomegalovirus antigenemia were negative. The results of a PCR test for SARS-CoV-2 were also negative. BAL was performed for differential diagnosis, and a culture of the BAL fluid showed no presence of bacteria/mycobacteria. BALF cell differentiation showed a preponderance of macrophages (Figure 6B). The patient was then diagnosed with an acute exacerbation of IPF and treated with pulse methylprednisolone, tacrolimus, antibiotics, and recombinant thrombomodulin. Upon admission, he required 5 L/min of oxygenation at rest. He showed improvement, requiring only 0.5 L/min of oxygenation, and was discharged three weeks after admission. We compared mass cytometry analysis of cell subpopulations between AEx (n = 1) and other cases (n = 7) of IPF. The proportion of CD14+ CD36hi CD84hi CCR2– monocyte populations (clusters #6056, #6064, #6075) in myeloid cells was highest in AEx compared to stable conditions in IPF (Figure 6C). A UMAP of myeloid cells showed increased CD36 and CD84 expression in AEx of IPF (Figure 6D). On the contrary, the proportions of myeloid clusters #6025 and #6054, characterized by CD64+ CD11blo CD14– CD223 (LAG3)+ HLA-DR+ CD163hi expression, were lowest in AEx of IPF (Figure 6C). t-SNE analysis of T cells showed a decreased proportion of CD8+ T cells and an increased proportion of CD4+ CD57– CD7+ CD44+ PD1– subsets in AEx of IPF (Figure 6E).
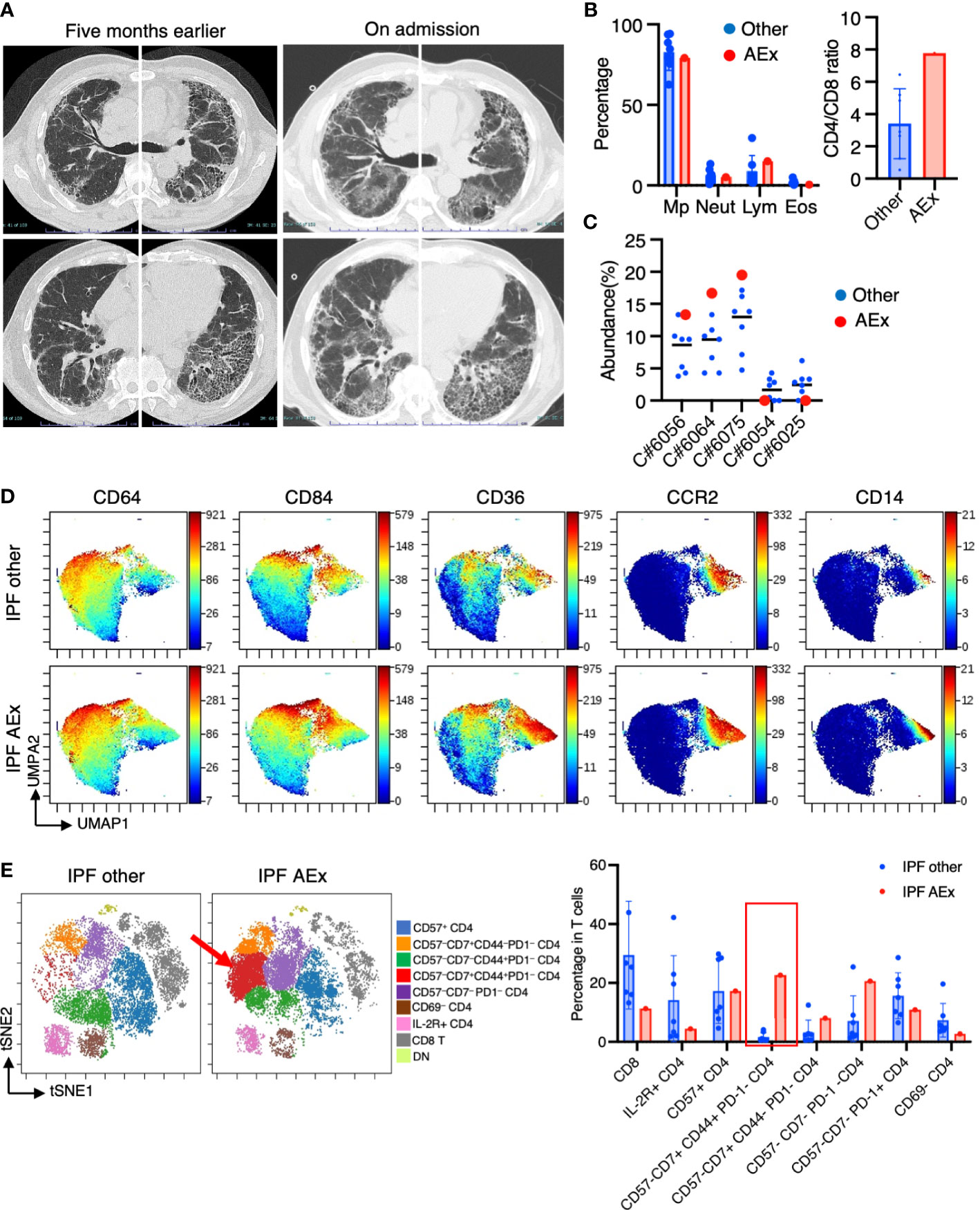
Figure 6 Immunological phenotypes in a patient with acute exacerbation of IPF. (A) Chest computed tomography images of the patient upon admission reveal the emergence of bilateral diffuse ground glass opacities superimposed on a honeycomb pattern, accompanied by peripheral traction bronchiectasis primarily in the basal lungs. (B) Comparison of BALF cell differentiation and CD4/CD8 ratio between patients with a patient experiencing an acute exacerbation (AEx) of the condition and the other cases of IPF. (C) Citrus-generated plots for myeloid sub-populations in IPF patients with stable condition and AEx. Each cluster number (C#) corresponds to the number shown in Figure 2C. (D) A uniform manifold approximation and projection (UMAP) of myeloid cells (CD45+CD11b+CD11c+ gated) showing cell distributions and each marker expression in BALF cells from concatenated samples with AEx and other cases of IPF. (E) t-SNE plots visualizing the distribution of T cell subpopulations in BALF T cells (gated as CD45+CD2+CD3+) from patients with AEx and other cases of IPF. Double negative (DN) T cells were defined as CD4–CD8– T cells. A red arrow indicates expansion of the CD57-CD7+CD44+PD-1-CD4 T cell subpopulation in AEx-IPF.
Immunological phenotypes in BALF cells from patients with CTD-ILD
CTD-ILD encompasses diverse diseases that may exhibit divergent immune cell abnormalities. Hence, we have compared each CTD-ILD, that is, SjS, DM, SSc, RA, SLE, MCTD, IgG4-related, and IPAF. Figures 7A, B show that the CD28–CD4/CD28+CD4 ratio was significantly divergent between the diseases, with the highest ratio from SLE-related ILDs. Previous research indicated that CD28– CD4+ T cells were prevalent in the blood of SLE patients with nephritis (30). These CD28– CD4+ T cells were infiltrated in the renal tissue and may have contributed to renal injury (30). Given this prior report, we speculated that these CD28– CD4+ T cells might also play a pathological role in SLE-related ILDs. Subsequently, we performed a CITRUS analysis on T cell subsets between SjS and DM (the CITRUS analysis can be conducted if the sample size is three or more.) (Figures 7C–E). The CITRUS analysis revealed that TIM-3hi CD8+ T cells were more abundant in patients with DM compared to those with SjS, which is concordant with the findings from prior publications (31, 32).
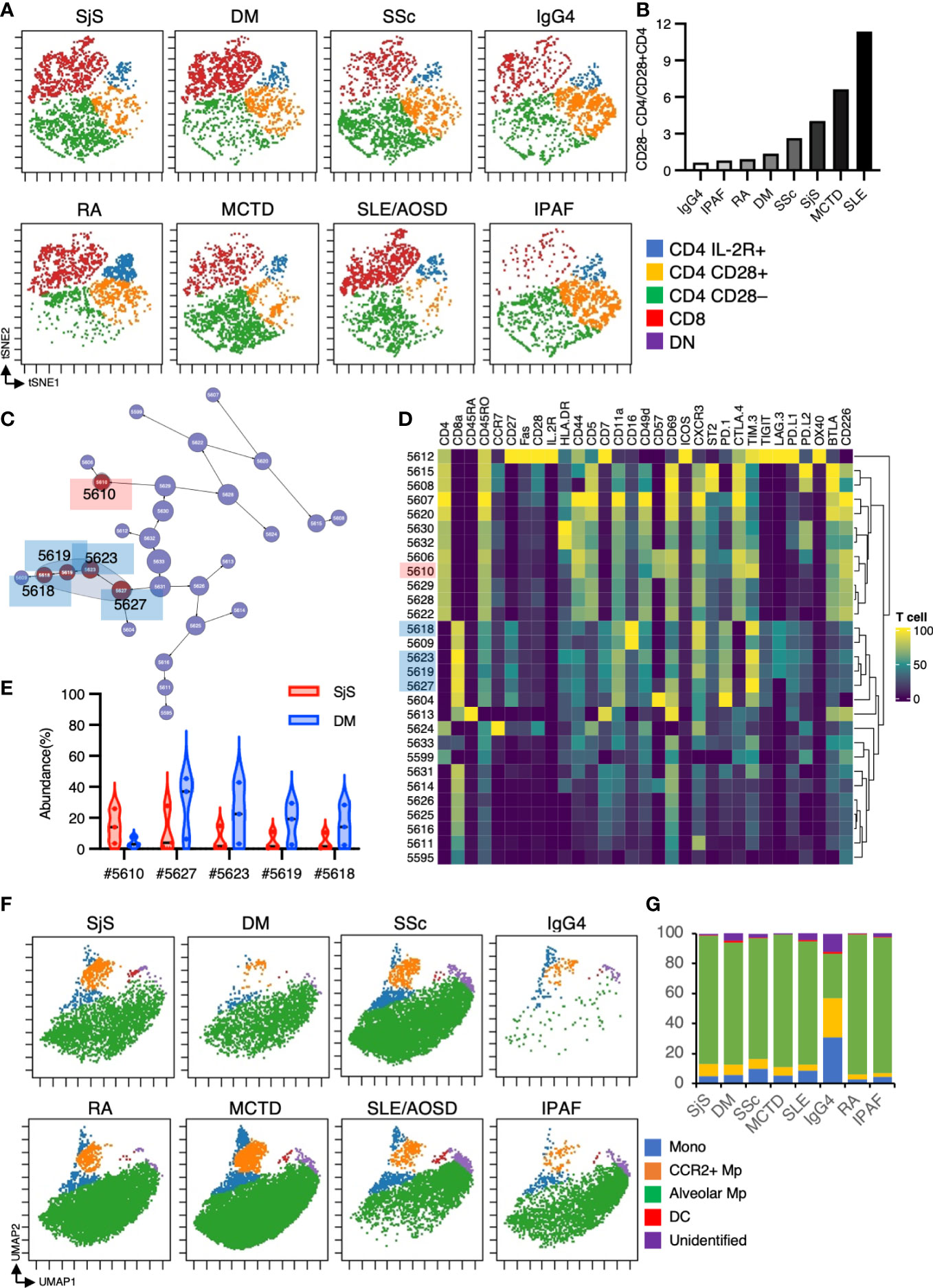
Figure 7 Immunological phenotypes in BALF cells from patients with CTD-ILD. (A) t-SNE plots visualizing the distribution of T cell subpopulations in BALF T cells (gated as CD45+CD2+CD3+) from patients with CTD-ILD. DN: CD4–CD8– double negative T cells. (B)The ratio of CD28– CD4 T cells/CD28+ CD4 T cells defined in t-SNE plots in each disease. (C) The Citrus network tree displays the hierarchical relationship and intensity of each marker among the T-cell populations in BALF from Sjogren syndrome-related ILD (n = 3) and dermatomyositis associated-ILD (n = 3). (D) Heatmap illustrating the expression markers across different clusters of T cells as determined by the Citrus analysis. (E) Citrus-generated violin plots for five representative and differentially regulated populations. Each cluster number (C#) corresponds to the number shown in panel (C). All differences in abundance are significant at a false discovery rate < 0.01. (F) UMAP plots visualizing the distribution of CD11b+CD11c+ myeloid cell subpopulations in BALF from patients with CTD-ILD. Monocytes are defined by CD64+CD14+, CCR2+ macrophages (Mp) by CCR2+ CD64+ CD14–, Alveolar Mp by CD64+CD206+, dendritic cells (DC) by CD64– CD206– CD11c+ HLA-DR+, unidentified cells by CD64– CD206– CD11clo HLA-DR–. (G) The proportions of myeloid cell subpopulations in CTD-ILD.
In the myeloid subset, a lower proportion of alveolar macrophages with higher monocytes and CCR2+ macrophages were observed in IgG4-related ILDs (Figures 7F, G). Nevertheless, due to the very limited number of cases in each group, it is challenging to conclude whether the disparities in cell populations are intrinsic differences or not.
Discussion
We have here demonstrated the characteristic immune cell subpopulations present in BALF from patients with IPF, CTD-ILDs, and sarcoidosis. Our analysis revealed an expansion of CD14+CD36hiCD84hi CCR2– monocytes in patients with IPF, an increase in FCRL5+ B cell in patients with CTD-ILDs and AEx of IPF, increased levels of IL-2R+ TIGIT+ LAG3+ CD4+ T cells in IPF, increased levels of CXCR3+ CD226+ CD4+ T cells in sarcoidosis, and increased levels of PD1+ TIGIT+ CD57+ CD8+ T cells in CTD-ILDs.
CD36 is a scavenger receptor expressed on the surface of immune and non-immune cells that acts as a signaling receptor for damage-associated molecular patterns (DAMPs) and pathogen-associated molecular patterns (PAMPs) and also serves as a transporter for long-chain free fatty acids (33). CD84 is an immunoreceptor expressed on the surface of various immune cells that regulates a range of immunological processes, including T cell cytokine secretion, natural killer cell cytotoxicity, monocyte activation, autophagy, T–B interactions, and B cell tolerance at the germinal center checkpoint (34). Ayaub et al. recently reported that CD36hi CD84hi macrophages were expanded as a specific subpopulation of macrophages in the lungs of patients with IPF compared to healthy or chronic obstructive pulmonary disease lungs using single-cell RNA-sequencing, mass cytometry, and flow cytometry (28). Ayaub et al. demonstrated that these CD36hi CD84hi macrophages expressed both alveolar and interstitial lung macrophage markers (HLA-DR+, CD11b+, CD206+), which is consistent with our results. Importantly, our study demonstrated these macrophage populations could be detected from BALF samples, which is less invasive compared to a lung biopsy and more suitable for clinical applications. We further determined that these CD36hi CD84hi macrophages were CD14 positive, indicating that these cells originated from monocytes.
We discovered these CD36hi CD84hi monocyte subpopulations were highest in AEx of IPF among all IPF cases. Nakashima et al. demonstrated that pulmonary fibrosis led to significant alterations in the bone marrow, including the expansion and activation of monocytic cells, which enhanced fibrosis upon subsequent lung injury (35). The pathobiology of IPF AEx is likely triggered by an acute event that leads to widespread acute lung injury, along with the acceleration of underlying chronic factors contributing to the fibrotic process (23). From this evidence, we speculate that these CD36hi CD84hi monocyte subpopulations may be involved in accelerating the AEx of IPF. Interestingly, these CD36hiCD84hi CD14+CCR2– monocyte populations were ST2+ and CCR5+ but distinct from CCR2+ monocyte populations (Figures 2D, 3A). Liang et al. demonstrated that the expression of CCL2, a ligand for CCR2, suppressed bleomycin-induced pulmonary fibrosis in mice (36). In addition, it has been shown that CCR2 deficiency in a mouse model of silica-induced pulmonary fibrosis resulted in an expansion of the fibrotic area (37), suggesting that CCR2hi monocyte-derived macrophages may have a suppressive role in fibrosis. Increased monocyte count in blood samples has been identified as a cellular biomarker for poor outcomes in fibrotic diseases, including IPF (38). A more detailed analysis of subsets of monocytes in the blood may be more useful for predicting the prognosis of ILDs.
FCRL5, encoded by the immunoglobulin superfamily receptor translocation-associated 2 (IRTA2) gene, is a member of the Fc receptor-like family, and its expression is mainly restricted to B cells. It has nine extracellular immunoglobulin domains, two immunoreceptor tyrosine-based inhibitory motifs, and one presumed immunoreceptor tyrosine-based activation motif in its cytoplasmic tail (39). FCRL5 signaling, in conjunction with B cell receptor activation and TLR9 engagement, can lead to B-cell proliferation, activation, isotype switching, and the production of IgG- and IgA-positive B cells (29). Higher FCRL5 expression predicted response to rituximab in rheumatoid arthritis (40) and granulomatosis with polyangiitis and microscopic polyangiitis (16). There are reports that single nucleotide variants in the FCRL5 gene increase an individual’s predisposition to multiple sclerosis (41) or SLE (42). This evidence indicates the pathogenic role of FCRL5 on autoimmunity. This study demonstrated increased FCRL5+ B cells in CTD-ILDs and a case of AEx of IPF. A recent study has demonstrated that rituximab was not inferior to cyclophosphamide in treating patients with CTD-ILDs with fewer adverse events (43). Collectively, higher FCRL5+ B cells in BALF could be used as a biomarker as a rationale for using rituximab.
We observed higher levels of IL-2R+ CD4+ T cells in patients with IPF compared to those with CTD-ILDs and sarcoidosis. These IL-2R+ CD4+ T cells likely represent Tregs, given that IL-2R+ expressing CD4+ T cells are a sole, distinct T cell subpopulation characterized by the highest FOXP3 expression between T cell subsets (6). In the study by Serezani et al. (6), the proportions of CD4 Tregs were a higher tendency in IPF compared to controls. Increased activated Treg proportion was correlated with the severity of IPF (44). However, the precise roles of Tregs in the development of pulmonary fibrosis have not been fully elucidated, and the existing literature on Tregs in pulmonary fibrosis is inconsistent (45). On the one hand, Tregs may contribute to the progression of pulmonary fibrosis by secreting platelet-derived growth factor (PDGF), transforming growth factor-β (TGF-β), and other factors that promote epithelial-mesenchymal transition and alter the Th1/Th2 balance. On the other hand, Tregs may inhibit fibrosis by promoting the repair of epithelial cell damage, inhibiting the accumulation of fibroblasts, and strongly suppressing the production and function of pro-inflammatory factors and cells. The roles of Treg would, thus, be dependent on the time and microenvironment in regard to pulmonary fibrosis. We would like to note that these Tregs express PD-L1. We previously reported the PD-L1 expression in T cells from BALF and the emergence of PD-1 and PD-L1 co-expressing T cells in particular situations, such as a severe immune-checkpoint inhibitor-related ILD (46) and adult T cell leukemia-affected lungs (47). These PD-L1 expressing T cells, including CD4 T cells, may have an immune-suppressing function on neighboring effector T cells or a cis-regulatory fashion on itself (48) via PD-1/PD-L1 interaction.
We showed increased levels of CXCR3+ CD226+ CD4+ T cells in sarcoidosis. CXCR3 ligands, CXCL9 and CXCL11, are augmented in BALF from pulmonary sarcoidosis (49). These CXCL9 and CXCL11 are interferon-inducible chemokines and localized to epithelioid histiocytes and multinucleated giant cells forming non-necrotizing granulomas (49). Our observation matches the results of the previous study and suggests that CXCR3 ligands may recruit CXCR3-expressing CD4 T cells, which propagate a type 1 immune response and cause granuloma formation. CD226, also known as DNAM-1, is an adhesion molecule that plays a role in activating T cells through the interaction with CD115 and CD112 as ligands (50), competing for TIGIT as reciprocal functions. This is the first report to mention CD226 expression in T cells from sarcoidosis. We speculate that CD4 T cells migrating to the lungs through CXCR3 may be activated through CD226, potentially contributing to the pathogenesis of sarcoidosis.
CD57 expression divided T cell subpopulations in viSNE. CD57 expression is typically associated with NK cells, but it has also been found in T cells that have a more advanced differentiation state, reduced replicative capacity, and increased production of cytokines such as IFNγ (51–53). There is also some evidence that CD57+ CD8+ T cells may have cytotoxic functions and be correlated with autoimmune activity in type 1 diabetes (54). We found increased levels of CD57+CD8+PD-1+TIGIT+ subsets in CTD-ILDs, and these CD8+ subsets may be related to an autoimmune condition.
Our study has several limitations, such as the lack of data from healthy controls, the relatively small sample size, and the retrospective design, which resulted in missing clinical data for some cases. Selection biases may be present, as only patients who underwent the BAL procedure could enroll in this study. In summary, our study demonstrates different immune cell phenotypes in IPF, CTD-ILDs, and sarcoidosis. We discovered that CD14+CD36+CD84+ monocytes and FCRL5+ B cells, important immune subsets, are differentially expressed and may be pathogenic. Further studies based on these findings may result in the development of new cell-targeted strategies to inhibit ILD progression.
Data availability statement
The original contributions presented in the study are included in the article/Supplementary Material. Further inquiries can be directed to the corresponding author.
Ethics statement
The studies involving human participants were reviewed and approved by the Ethics Committee of Kyushu University Hospital. Written informed consent for participation was not required for this study in accordance with the national legislation and the institutional requirements.
Author contributions
Conceptualization: TY; Methodology: KH, TY, KM, KK, and YB; Investigation: KH, TY, KM, KK, KS, KT, DE, HA, and MU; Visualization: KH, TY, KM, KK, KS, KT, DE, HA, MU, SI, and YB; Funding acquisition: TY and YF; Supervision: SI, YB, YF, and IO; Writing-original draft: KH and TY; Writing-review and editing: SI, YB, YF, and IO. All authors contributed to the article and approved the submitted version.
Funding
This research was supported by the Kakihara Foundation and Boehringer Ingelheim (TY), and the Japan Agency for Medical Research and Development (YF). The funder was not involved in the study design, collection, analysis, interpretation of data, the writing of this article, or the decision to submit it for publication.
Acknowledgments
We thank Ms. Sanae Sekihara, PhD. from Standard Biotools, for her technical assistance. We also extend our appreciation to the Medical Research Center Initiative for High Depth Omics in Kyushu University. This manuscript has been submitted to BioRxiv (https://biorxiv.org/cgi/content/short/2023.01.12.523734v1).
Conflict of interest
The authors declare that the research was conducted in the absence of any commercial or financial relationships that could be construed as a potential conflict of interest.
Publisher’s note
All claims expressed in this article are solely those of the authors and do not necessarily represent those of their affiliated organizations, or those of the publisher, the editors and the reviewers. Any product that may be evaluated in this article, or claim that may be made by its manufacturer, is not guaranteed or endorsed by the publisher.
Supplementary material
The Supplementary Material for this article can be found online at: https://www.frontiersin.org/articles/10.3389/fimmu.2023.1145814/full#supplementary-material
References
1. Wijsenbeek M, Suzuki A, Maher TM. Interstitial lung diseases. Lancet (2022) 400(10354):769–86. doi: 10.1016/S0140-6736(22)01052-2
2. Hopkins RB, Burke N, Fell C, Dion G, Kolb M. Epidemiology and survival of idiopathic pulmonary fibrosis from national data in Canada. Eur Respir J (2016) 48(1):187–95. doi: 10.1183/13993003.01504-2015
3. Wells AU, Denton CP. Interstitial lung disease in connective tissue disease–mechanisms and management. Nat Rev Rheumatol (2014) 10(12):728–39. doi: 10.1038/nrrheum.2014.149
4. Spagnolo P, Rossi G, Trisolini R, Sverzellati N, Baughman RP, Wells AU. Pulmonary sarcoidosis. Lancet Respir Med (2018) 6(5):389–402. doi: 10.1016/S2213-2600(18)30064-X
5. Yanagihara T, Sato S, Upagupta C, Kolb M. What have we learned from basic science studies on idiopathic pulmonary fibrosis? Eur Respir Rev (2019) 28(153):190029. doi: 10.1183/16000617.0029-2019
6. Serezani AP, Pascoalino BD, Bazzano J, Vowell KN, Tanjore H, Taylor CJ, et al. Multi-platform single-cell analysis identifies immune cell types enhanced in pulmonary fibrosis. Am J Respir Cell Mol Biol (2022) 67(1):50–60. doi: 10.1165/rcmb.2021-0418oc
7. Yanagihara T, Inoue Y. Insights into pathogenesis and clinical implications in myositis-associated interstitial lung diseases. Curr Opin Pulm Med (2020) 26(5):507–17. doi: 10.1097/MCP.0000000000000698
8. Cai Y, Sugimoto C, Arainga M, Alvarez X, Didier ES, Kuroda MJ. In vivo characterization of alveolar and interstitial lung macrophages in rhesus macaques: Implications for understanding lung disease in humans. J Immunol (2014) 192(6):2821–9. doi: 10.4049/jimmunol.1302269
9. Wynn TA, Vannella KM. Macrophages in tissue repair, regeneration, and fibrosis. Immunity (2016) 44(3):450–62. doi: 10.1016/j.immuni.2016.02.015
10. Reyfman PA, Walter JM, Joshi N, Anekalla KR, McQuattie-Pimentel AC, Chiu S, et al. Single-cell transcriptomic analysis of human lung provides insights into the pathobiology of pulmonary fibrosis. Am J Respir Crit Care Med (2019) 199(12):1517–36. doi: 10.1164/rccm.201712-2410OC
11. Morse C, Tabib T, Sembrat J, Buschur KL, Bittar HT, Valenzi E, et al. Proliferating SPP1/MERTK-expressing macrophages in idiopathic pulmonary fibrosis. Eur Respir J (2019) 54(2):1802441. doi: 10.1183/13993003.02441-2018
12. Adams TS, Schupp JC, Poli S, Ayaub EA, Neumark N, Ahangari F, et al. Single-cell RNA-seq reveals ectopic and aberrant lung-resident cell populations in idiopathic pulmonary fibrosis. Sci Adv (2020) 6(28):eaba1983. doi: 10.1126/sciadv.aba1983
13. Satoh T, Nakagawa K, Sugihara F, Kuwahara R, Ashihara M, Yamane F, et al. Identification of an atypical monocyte and committed progenitor involved in fibrosis. Nature (2017) 541(7635):96–101. doi: 10.1038/nature20611
14. Joshi N, Watanabe S, Verma R, Jablonski RP, Chen CI, Cheresh P, et al. A spatially restricted fibrotic niche in pulmonary fibrosis is sustained by m-CSF/M-CSFR signalling in monocyte-derived alveolar macrophages. Eur Respir J (2020) 55(1):1900646. doi: 10.1183/13993003.00646-2019
15. Celada LJ, Kropski JA, Herazo-Maya JD, Luo W, Creecy A, Abad AT, et al. PD-1 up-regulation on CD4+ T cells promotes pulmonary fibrosis through STAT3-mediated IL-17A and TGF-β1 production. Sci Transl Med (2018) 10(460):1–15. doi: 10.1126/scitranslmed.aar8356
16. Owczarczyk K, Cascino MD, Holweg C, Tew GW, Ortmann W, Behrens T, et al. Fc receptor-like 5 and anti-CD20 treatment response in granulomatosis with polyangiitis and microscopic polyangiitis. JCI Insight (2020) 5(18):e136180. doi: 10.1172/jci.insight.136180
17. Jenks SA, Cashman KS, Zumaquero E, Marigorta UM, Patel AV, Wang X, et al. Distinct effector b cells induced by unregulated toll-like receptor 7 contribute to pathogenic responses in systemic lupus erythematosus. Immunity (2018) 49(4):725–739.e6. doi: 10.1016/j.immuni.2018.08.015
18. Wang S, Wang J, Kumar V, Karnell JL, Naiman B, Gross PS, et al. IL-21 drives expansion and plasma cell differentiation of autoreactive CD11chiT-bet+ b cells in SLE. Nat Commun (2018) 9(1):1–14. doi: 10.1038/s41467-018-03750-7
19. Korin B, Dubovik T, Rolls A. Mass cytometry analysis of immune cells in the brain. Nat Protoc (2018) 13(2):377–91. doi: 10.1038/nprot.2017.155
20. Raghu G, Remy-Jardin M, Myers JL, Richeldi L, Ryerson CJ, Lederer DJ, et al. Diagnosis of idiopathic pulmonary fibrosis an official ATS/ERS/JRS/ALAT clinical practice guideline. Am J Respir Crit Care Med (2018) 198(5):e44–68. doi: 10.1164/rccm.201807-1255ST
21. Hunninghake GW, Costabel U, Ando M, Baughman R, Cordier JF, Du Bois R, et al. Statement on sarcoidosis. joint statement of the American thoracic society (ATS), the European respiratory society (ERS) and the world association of sarcoidosis and other granulomatous disorders (WASOG) adopted by the ATS board of directors and by the ER. Am J Respir Crit Care Med (1999) 160(2):736–55. doi: 10.1164/ajrccm.160.2.ats4-99
22. Fischer A, du Bois R. Interstitial lung disease in connective tissue disorders. Lancet (2012) 380(9842):689–98. doi: 10.1016/S0140-6736(12)61079-4
23. Collard HR, Ryerson CJ, Corte TJ, Jenkins G, Kondoh Y, Lederer DJ, et al. Acute exacerbation of idiopathic pulmonary fibrosis an international working group report. Am J Respir Crit Care Med (2016) 194(3):265–75. doi: 10.1164/rccm.201604-0801CI
24. Fischer A, Antoniou KM, Brown KK, Cadranel J, Corte TJ, Du Bois RM, et al. An official European respiratory Society/American thoracic society research statement: Interstitial pneumonia with autoimmune features. Eur Respir J (2015) 46(4):976–87. doi: 10.1183/13993003.00150-2015
25. Flaherty KR, Wells AU, Cottin V, Devaraj A, Walsh SLF, Inoue Y, et al. Nintedanib in progressive fibrosing interstitial lung diseases. N Engl J Med (2019) 54(3):1900161. doi: 10.1056/NEJMoa1908681
26. Matsubara K, Kunimura K, Yamane N, Aihara R, Sakurai T, Sakata D, et al. DOCK8 deficiency causes a skewing to type 2 immunity in the gut with expansion of group 2 innate lymphoid cells. Biochem Biophys Res Commun (2021) 559(June):135–40. doi: 10.1016/j.bbrc.2021.04.094
27. Bruggner RV, Bodenmiller B, Dill DL, Tibshirani RJ, Nolan GP. Automated identification of stratifying signatures in cellular subpopulations. Proc Natl Acad Sci USA (2014) 111(26):E2770–7. doi: 10.1073/pnas.1408792111
28. Ayaub EA, Poli S, Ng J, Adams T, Schupp J, Quesada-Arias L, et al. Single cell RNA-seq and mass cytometry reveals a novel and a targetable population of macrophages in idiopathic pulmonary fibrosis. bioRxiv (2021) 2021:1. doi: 10.1101/2021.01.04.425268
29. Dement-Brown J, Newton CS, Ise T, Damdinsuren B, Nagata S, Tolnay M. Fc receptor-like 5 promotes b cell proliferation and drives the development of cells displaying switched isotypes. J Leukoc Biol (2012) 91(1):59–67. doi: 10.1189/jlb.0211096
30. Zhang T, Liu X, Zhao Y, Xu X, Liu Y, Wu X. Excessive IL-15 promotes cytotoxic CD4 + CD28– T cell-mediated renal injury in lupus nephritis. Immun Ageing (2022) 19(1):1–12. doi: 10.1186/s12979-022-00305-9
31. Nakazawa M, Suzuki K, Takeshita M, Inamo J, Kamata H, Ishii M, et al. Distinct expression of coinhibitory molecules on alveolar T cells in patients with rheumatoid arthritis–associated and idiopathic inflammatory myopathy–associated interstitial lung disease. Arthritis Rheumatol (2021) 73(4):576–86. doi: 10.1002/art.41554
32. Suzuki K, Yanagihara T, Matsumoto K, Chong SG, Ando H, Ide M, et al. Immune-checkpoint kinetics for T cells in anti-MDA5 positive interstitial lung disease. Rheumatology (2021) 60(1):e14–6. doi: 10.1093/rheumatology/keaa412
33. Chen Y, Zhang J, Cui W, Silverstein RL. CD36, a signaling receptor and fatty acid transporter that regulates immune cell metabolism and fate. J Exp Med (2022) 219(6):1–15. doi: 10.1084/jem.20211314
34. Cuenca M, Sintes J, Lányi Á, Engel P. CD84 cell surface signaling molecule: An emerging biomarker and target for cancer and autoimmune disorders. Clin Immunol (2019) 204(October 2018):43–9. doi: 10.1016/j.clim.2018.10.017
35. Nakashima T, Liu T, Hu B, Wu Z, Ullenbruch M, Omori K, et al. Role of B7H3/IL-33 signaling in pulmonary fibrosis–induced profibrogenic alterations in bone marrow. Am J Respir Crit Care Med (2019) 200(8):1032–44. doi: 10.1164/rccm.201808-1560OC
36. Liang J, Jung Y, Tighe RM, Xie T, Liu N, Leonard M, et al. A macrophage subpopulation recruited by CC chemokine ligand-2 clears apoptotic cells in noninfectious lung injury. Am J Physiol - Lung Cell Mol Physiol (2012) 302(9):933–40. doi: 10.1152/ajplung.00256.2011
37. Shichino S, Abe J, Ueha S, Otsuji M, Tsukui T, Kosugi-Kanaya M, et al. Reduced supply of monocyte-derived macrophages leads to a transition from nodular to diffuse lesions and tissue cell activation in silica-induced pulmonary fibrosis in mice. Am J Pathol (2015) 185(11):2923–38. doi: 10.1016/j.ajpath.2015.07.013
38. Scott MKD, Quinn K, Li Q, Carroll R, Warsinske H, Vallania F, et al. Increased monocyte count as a cellular biomarker for poor outcomes in fibrotic diseases: A retrospective, multicentre cohort study. Lancet Respir Med (2019) 7(6):497–508. doi: 10.1016/S2213-2600(18)30508-3
39. Rostamzadeh D, Kazemi T, Amirghofran Z, Shabani M. Update on fc receptor-like (FCRL) family: New immunoregulatory players in health and diseases. Expert Opin Ther Targets (2018) 22(6):487–502. doi: 10.1080/14728222.2018.1472768
40. Owczarczyk K, Lal P, Abbas AR, Wolslegel K, Holweg CTJ, Dummer W, et al. A plasmablast biomarker for nonresponse to antibody therapy to CD20 in rheumatoid arthritis. Sci Transl Med (2011) 3(101):1–10. doi: 10.1126/scitranslmed.3002432
41. Chorazy M, Wawrusiewicz-Kurylonek N, Adamska-Patruno E, Czarnowska A, Zajkowska O, Kapica-Topczewska K, et al. Variants of novel immunomodulatory fc receptor like 5 gene are associated with multiple sclerosis susceptibility in the polish population. Front Neurol (2021) 12(April):631134. doi: 10.3389/fneur.2021.631134
42. Wang YF, Zhang Y, Lin Z, Zhang H, Wang TY, Cao Y, et al. Identification of 38 novel loci for systemic lupus erythematosus and genetic heterogeneity between ancestral groups. Nat Commun (2021) 12(1):1–13. doi: 10.1038/s41467-021-21049-y
43. Maher TM, Tudor VA, Saunders P, Gibbons MA, Fletcher SV, Denton CP, et al. Rituximab versus intravenous cyclophosphamide in patients with connective tissue disease-associated interstitial lung disease in the UK (RECITAL): A double-blind, double-dummy, randomised, controlled, phase 2b trial. Lancet Respir Med (2023) 11(1):45–54. doi: 10.1016/S2213-2600(22)00359-9
44. Hou Z, Ye Q, Qiu M, Hao Y, Han J, Zeng H. Increased activated regulatory T cells proportion correlate with the severity of idiopathic pulmonary fibrosis. Respir Res (2017) 18:1–9. doi: 10.1186/s12931-017-0653-3
45. Wang F, Xia H, Yao S. Regulatory T cells are a double-edged sword in pulmonary fibrosis. Int Immunopharmacol (2020) 84(1277):106443. doi: 10.1016/j.intimp.2020.106443
46. Suzuki K, Yanagihara T, Matsumoto K, Kusaba H, Yamauchi T, Ikematsu Y, et al. Immune-checkpoint profiles for T cells in bronchoalveolar lavage fluid of patients with immune-checkpoint inhibitor-related interstitial lung disease. Int Immunol (2020) 32(8):547–57. doi: 10.1093/intimm/dxaa022
47. Yanagihara T, Ikematsu Y, Kato K, Yonekawa A, Ideishi S, Tochigi T, et al. Expression of PD-1 and PD-L1 on cytotoxic T lymphocytes and immune deficiency in a patient with adult T cell leukemia/lymphoma. Ann Hematol (2018) 97(2):359–60. doi: 10.1007/s00277-017-3146-z
48. Sugiura D, Maruhashi T, Okazaki I-M, Shimizu K, Maeda TK, Takemoto T, et al. Restriction of PD-1 function by cis-PD-L1/CD80 interactions is required for optimal T cell responses. Science (2019) 364(6440):558–66. doi: 10.1126/science.aav7062
49. Busuttil A, Weigt SS, Keane MP, Xue YY, Palchevskiy V, Burdick MD, et al. CXCR3 ligands are augmented during the pathogenesis of pulmonary sarcoidosis. Eur Respir J (2009) 34(3):676–86. doi: 10.1183/09031936.00157508
50. Shibuya A, Shibuya K. DNAM-1 versus TIGIT: Competitive roles in tumor immunity and inflammatory responses. Int Immunol (2021) 33(12):687–92. doi: 10.1093/intimm/dxab085
51. Batista MD, Tincati C, Milush JM, Ho EL, Ndhlovu LC, York VA, et al. CD57 expression and cytokine production by T cells in lesional and unaffected skin from patients with psoriasis. PloS One (2013) 8(2):3–8. doi: 10.1371/journal.pone.0052144
52. Fernandez S, French MA, Price P. Immunosenescent CD57+CD4+ T-cells accumulate and contribute to interferon-γ responses in HIV patients responding stably to ART. Dis Markers (2011) 31(6):337–42. doi: 10.3233/DMA-2011-0847
53. Palmer BE, Blyveis N, Fontenot AP, Wilson CC. Functional and phenotypic characterization of CD57 + CD4 + T cells and their association with hiv-1-induced t cell dysfunction. J Immunol (2005) 175(12):8415–23. doi: 10.4049/jimmunol.175.12.8415
Keywords: mass cytometry (CyTOF), idiopathic pulmonary fibrosis, connective-tissue disease-related interstitial lung disease, sarcoidosis, monocyte, FCRL5
Citation: Hata K, Yanagihara T, Matsubara K, Kunimura K, Suzuki K, Tsubouchi K, Eto D, Ando H, Uehara M, Ikegame S, Baba Y, Fukui Y and Okamoto I (2023) Mass cytometry identifies characteristic immune cell subsets in bronchoalveolar lavage fluid from interstitial lung diseases. Front. Immunol. 14:1145814. doi: 10.3389/fimmu.2023.1145814
Received: 16 January 2023; Accepted: 13 February 2023;
Published: 06 March 2023.
Edited by:
Shengjun Wang, Jiangsu University Affiliated People’s Hospital, ChinaReviewed by:
Wataru Fujii, Kyoto Prefectural University of Medicine, JapanBrian Vestal, National Jewish Health, United States
Copyright © 2023 Hata, Yanagihara, Matsubara, Kunimura, Suzuki, Tsubouchi, Eto, Ando, Uehara, Ikegame, Baba, Fukui and Okamoto. This is an open-access article distributed under the terms of the Creative Commons Attribution License (CC BY). The use, distribution or reproduction in other forums is permitted, provided the original author(s) and the copyright owner(s) are credited and that the original publication in this journal is cited, in accordance with accepted academic practice. No use, distribution or reproduction is permitted which does not comply with these terms.
*Correspondence: Toyoshi Yanagihara, eWFuYWdpaGFyYS50b3lvc2hpLjc1OUBtLmt5dXNodS11LmFjLmpw
†These authors have contributed equally to this work and share first authorship