- 1Department of Oncology, The Second Affiliated Hospital of Xi’an Jiaotong University, Xi’an, China
- 2China-Australia Joint Research Center for Infectious Diseases, School of Public Health, Xi’an Jiaotong University Health Science Center, Xi’an, China
- 3National and Local Joint Engineering Research Center of Biodiagnosis and Biotherapy, The Second Affiliated Hospital of Xi’an Jiaotong University, Xi’an, China
- 4Melbourne Sexual Health Centre, Alfred Health, Melbourne, VIC, Australia
- 5Central Clinical School, Faculty of Medicine, Nursing and Health Sciences, Monash University, Melbourne, VIC, Australia
Background: The association between gut microbiome and coronavirus disease 2019 (COVID-19) has attracted much attention, but its causality remains unclear and requires more direct evidence.
Methods: In this study, we conducted the bidirectional Mendelian randomization (MR) analysis to assess the causal association between gut microbiome and COVID-19 based on the summary statistics data of genome-wide association studies (GWASs). Over 1.8 million individuals with three COVID-19 phenotypes (severity, hospitalization and infection) were included. And 196 bacterial taxa from phylum to genus were analyzed. The inverse-variance weighted (IVW) analysis was chosen as the primary method. Besides, false discovery rate (FDR) correction of p-value was used. To test the robustness of the causal relationships with p-FDR < 0.05, sensitivity analyses including the secondary MR analyses, horizontal pleiotropy test, outliers test, and “leave-one-out” analysis were conducted.
Results: In the forward MR, we found that 3, 8, and 10 bacterial taxa had suggestive effects on COVID-19 severity, hospitalization and infection, respectively. The genus Alloprevotella [odds ratio (OR) = 1.67; 95% confidence interval (95% CI), 1.32–2.11; p = 1.69×10−5, p-FDR = 2.01×10−3] was causally associated with a higher COVID-19 severity risk. In the reverse MR, COVID-19 severity, hospitalization and infection had suggestive effects on the abundance of 4, 8 and 10 bacterial taxa, respectively. COVID-19 hospitalization causally increased the abundance of the phylum Bacteroidetes (OR = 1.13; 95% CI, 1.04–1.22; p = 3.02×10−3; p-FDR = 2.72×10−2). However, secondary MR analyses indicated that the result of COVID-19 hospitalization on the phylum Bacteroidetes required careful consideration.
Conclusion: Our study revealed the causal association between gut microbiome and COVID-19 and highlighted the role of “gut-lung axis” in the progression of COVID-19.
1 Introduction
The COVID-19 pandemic, caused by the severe acute respiratory syndrome coronavirus 2 (SARS-CoV-2), is imposing significant economic and healthcare challenges on society and is expected to do so for the foreseeable future (1, 2). While SARS-CoV-2 is known to mainly infect respiratory tract, increasing evidence suggests its potential involvement in the pathogenesis of COVID-19 via the gastrointestinal tract (3, 4). In addition to its ability to infect and replicate in intestinal enterocytes (5), SARS-CoV-2 can induce the upregulation of angiotensin converting enzyme-2 receptor expression in intestinal epithelial cells (6, 7). Frequent occurrence of gastrointestinal symptoms has been observed in individuals with SARS-CoV-2 infection (8), and a meta-analysis indicated that those with gastrointestinal involvement have an increased risk of severe disease (9).
Emerging evidence has shed light on the connection between the gut microbiome and the pathogenesis of COVID-19, through a mechanism known as the “gut-lung axis” (3, 10, 11). The gastrointestinal tract is considered to be the largest organ of the human immune system (12). Epidemiological studies have indicated that SARS-CoV-2 infection could result in alterations of gut microbiome (13–16), and the prognosis of COVID-19 appeared to be closely linked to the composition of gut microbiome (17–19). Previous studies have suggested that the resident microbiota in the gastrointestinal tract played a critical role in regulating host immunity, thereby providing defense against SARS-CoV-2 infection (20–22). Probiotics, a beneficial group of microorganisms, are known for their effectiveness in restoring homeostasis of gut microbiota, enhancing immunity, and exhibiting antiviral potential (3, 23). Clinical trials have demonstrated that probiotic supplements could restore the homeostasis of gut microbiota, potentially leading to improved prognosis of COVID-19 (24–26). These evidences suggested that the gut microbiome might be a target for the prevention, diagnosis and treatment of COVID-19. However, the association between gut microbiome and COVID-19 was not well-established because it could be easily influenced by unmeasured confounders (27–29). Furthermore, the association was susceptible to unavoidable biases and reverse causation (30).
Randomized controlled trials are considered the benchmark for investigating the causal link between gut microbiome and COVID-19. Regrettably, the screening of gut microbiome for early diagnosis and prognosis of COVID-19 is currently limited owing to the impact of external factors, such as research methods and technology (30). Additionally, a substantial amount of human and material resources are required to conduct the randomized controlled trials, resulting in a burdensome workload. In these circumstances, MR analysis has been advocated as an emerging approach (31). MR analysis is an alternative approach to assess the causal link between exposure and outcome. This method utilizes genetic variants as instrumental variables (IVs) that are randomly distributed during meiosis as unconfounded surrogates for the exposure (32). MR is analogous to the random assignment of interventions in the randomized controlled trials and can thus address the issues of reverse causation and confounders that are commonly found in nonrandomized studies (30). Owing to these strengths, MR analysis has been extensively used to identify factors associated with COVID-19 (28, 33–36).
In the study, utilizing the bi-directional MR approach, we evaluated both the causal impacts of gut microbiome on COVID-19 phenotypes and the causal impacts of COVID-19 phenotypes on gut microbiome. Our objective was to clarify the involvement of gut microbiome in the diagnosis and prognosis of COVID-19, with the ultimate goal of promoting the development of novel strategies, including probiotic therapy, fecal microbiome transplantation, and antimicrobial stewardship.
2 Methods
2.1 Study design
The MR analysis is based on the following assumptions (Figure 1A) (30). (1) IVs are strongly linked to the exposure. (2) IVs are not linked to the confounders. (3) IVs can only influence the outcome through the exposure, without involving alternative pathways. Figure 1B depicts the study design for examining the causal link between gut microbiome and COVID-19 using the bi-directional MR analysis. We first selected gut microbiome as the exposure and COVID-19 as the outcome to detect whether the gut microbiome prevented or promoted the occurrence of COVID-19. To explore the changes in gut microbiome after the occurrence of COVID-19, we conducted a reverse MR analysis (COVID-19 as the exposure; gut microbiome as the outcome). This MR study followed the Strengthening the Reporting of Observational Studies in Epidemiology-Mendelian Randomization (STROBE-MR) guidelines (Table S1) (37).
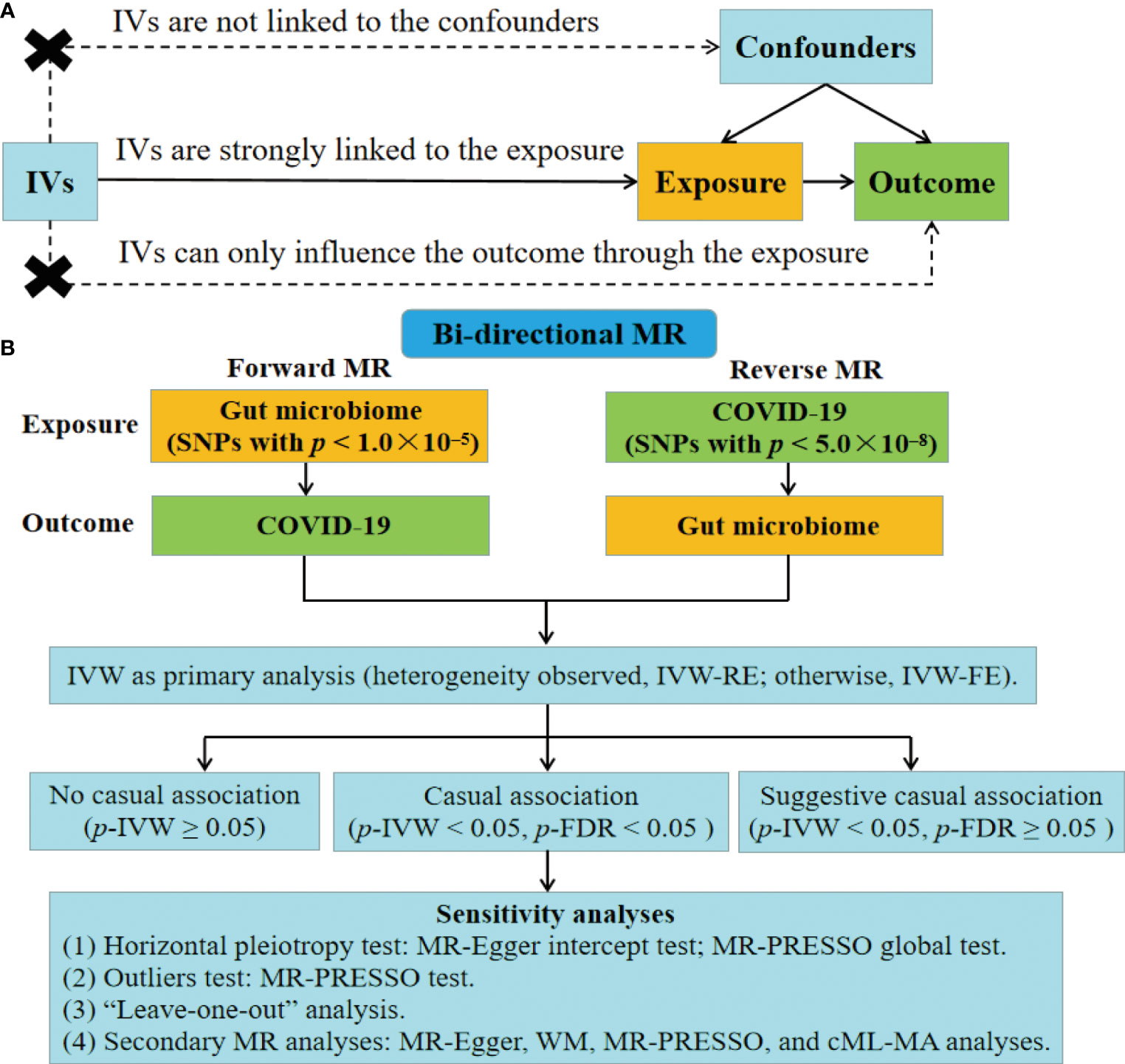
Figure 1 Fow diagram of the study. (A) Schematic of the MR design. (B) Overview of the bi-directional MR study. IVs, instrumental variables; FE, fixed-effect; RE, random-effect; FDR, false discovery rate; WM, weighted median; MR-PRESSO, MR pleiotropy residual sum and outlier; cML-MA, constrained maximum likelihood and model averaging-based.
2.2 Data sources
GWAS summary data on the human gut microbiome was obtained from the largest multi-ethnic GWAS meta-analysis conducted by the MiBioGen consortium (38). It included 18,340 participants from twenty-four cohorts. Data of gut microbiome was generated by targeting the 16S ribosomal RNA gene, specifically the V4, V3-V4, or V1-V2 regions, and primarily using the Illumina sequencing platform (39). Microbiota quantitative trait loci mapping analysis was conducted to investigate the interactions between host genetic variants and gut microbiome. In addition, the covariate-adjusted abundance of gut microbiome was analyzed, considering factors such as age, sex, technical variables, and principal components (38). Data of gut microbiome covered 211 taxa whose mean abundance more than 1%, encompassing 131 genera, 35 families, 20 orders, 16 classes, and 9 phyla. 196 bacterial taxa were ultimately included in the MR analysis after excluding 15 taxa belonging to unknown groups (12 genera and 3 families). More detailed information about the GWAS of gut microbiome can be found in the literatures (38, 39).
The GWAS summary data on COVID-19 was obtained from the global COVID-19 Host Genetics Initiative, with the exception of the “23andMe” dataset (40). The researchers had already adjusted the original data for covariates, including age, sex, age2, age × sex, and principal components. The MR study included 1,683,768 participants, with 38,984 infected cases and 1,644,784 un-infected individuals, for COVID-19 infection analysis. 1,887,658 participants, with 9,986 hospitalized cases and 1,877,672 un-infected individuals, for COVID-19 hospitalization analysis. 1,388,342 participants, with 5,101 severe cases and 1,383,241 un-infected individuals, for COVID-19 severity analysis. Individuals who died or required respiratory assistance as a result of COVID-19 infection were classified as severe cases (40).
2.3 Selection of IVs
Single nucleotide polymorphisms (SNPs) were used as IVs based on these criteria. (1) In the forward MR analysis, since few SNPs of gut microbiome met a p less than 5.0×10–8, we relaxed the p-value threshold (Figure 1B). SNPs with p lower than 1.0×10–5 were selected as IVs, following previous studies (41–47). In the reverse MR analysis, SNPs of COVID-19 with p < 5.0×10–8 were used (Figure 1B). (2) We applied clumping to restrict SNPs with low linkage disequilibrium (r2 less than 0.001; genetic distance = 10,000 kb) (32). (3) Palindromic SNPs were removed. (4) Only SNPs with minor allele frequency (MAF) more than 0.01 were included. (5) We also calculated F-statistics for the SNPs to assess their instrumental strengths. . SNPs with an F-statistic < 10 would be removed (48).
2.4 Statistical analysis
We conducted the bi-directional MR analysis to assess the causal impacts of gut microbiome on COVID-19 phenotypes and the causal impacts of COVID-19 phenotypes on gut microbiome (Figure 1B). IVW analysis was selected as the primary method complying with the STROBE-MR guidelines (37). It employs a meta-analysis method that combines the Wald ratio to provide the casual estimate. IVW analysis is considered precise and robust because it utilizes information from all IVs (32, 49). In addition, the heterogeneity was evaluated using Cochran’s Q test. If no heterogeneity was observed (Q_p-value < 0.05), the fixed-effect (FE) model of IVW was utilized. Alternatively, a random-effect (RE) model of IVW was applied (30, 32). The OR and corresponding 95% CI were reported as the results of the bi-directional MR analysis. P-value < 0.05 was considered was considered statistically significant. Besides, we conducted the FDR correction (p-FDR) with the threshold of 0.05. A causal association was considered significant when the IVW approach yielded a p-FDR < 0.05. In addition, we defined a suggestive association as having a p < 0.05 but a p-FDR ≥ 0.05 with the IVW approach (Figure 1B).
To assess the robustness of the findings related to causal relationships with a significance level of p-FDR < 0.05, several sensitivity analyses were conducted (Figure 1B). First, we employed the MR-Egger test (50) and MR pleiotropy residual sum and outlier (MR-PRESSO) global test (51) to identify horizontal pleiotropy. Second, the MR-PRESSO test was conducted to test for outliers of the SNPs. Third, we employed the “leave-one-out” analysis to assess the potential influence of individual SNP on the MR effect. Furthermore, we performed secondary MR analyses including the MR-Egger analysis (52), weighted median (WM) (53) analysis, MR-PRESSO analysis (51), and constrained maximum likelihood and model averaging-based (cML-MA) analysis (54). We considered a causal association to be authentic only when all MR methods indicated the same direction of effect. MR-Egger is capable of identifying certain deviations from the standard IV assumptions, and producing an effect estimate that is not affected by such deviations (52). WM can yield a causal estimate even when half of the information is derived from invalid IVs (53). cML-MA is a MR method that uses constrained maximum likelihood and model averaging, and has been shown to be resilient against both correlated and uncorrelated pleiotropy while maintaining a low type-I error rate (54). “TwoSampleMR” (55), “MRPRESSO” (51), and “MRcML” (54) were the primary R packages utilized in the study. All analyses were conducted using the R v4.1.2 (R Foundation, Vienna, Austria).
2.5 Ethical approval
This study analyzing publicly available summary-level data was exempt from ethical approval.
3 Results
3.1 Causal effects of gut microbiome on COVID-19 phenotypes
In the forward MR (gut microbiome as the exposure), we identified 2148, 2127, and 2137 SNPs associated with gut microbiome for COVID-19 severity, hospitalization, and infection, respectively. F-statistics greater than 10 for all SNPs related to gut microbiome indicated the absence of the weak instrument bias (Table S2). The adjusted IVW results after accounting for heterogeneity are presented in Table S3.
As shown in Figure 2A, IVW analysis revealed that genus Ruminococcus gnavus group (OR = 0.77; 95% CI, 0.62–0.95; p = 1.44×10−2), genus Oxalobacter (OR = 0.84; 95% CI, 0.71–1.00; p = 4.96×10−2), and genus Ruminiclostridium6 (OR = 0.78; 95% CI, 0.62–0.98; p = 3.55×10−2) showed suggestive associations with a reduced risk for COVID-19 severity. On the other hand, IVW analysis revealed a causal link between the genus Alloprevotella (OR = 1.67; 95% CI, 1.32–2.11; p = 1.69×10−5) and heightened COVID-19 severity risk after FDR correction (p-FDR = 2.01×10−3). In addition, the IVW, MR-Egger, WM, MR-PRESSO, and cML-MA methods yielded the similar direction for the causal effect of genus Alloprevotella on COVID-19 severity (Figure 3A).
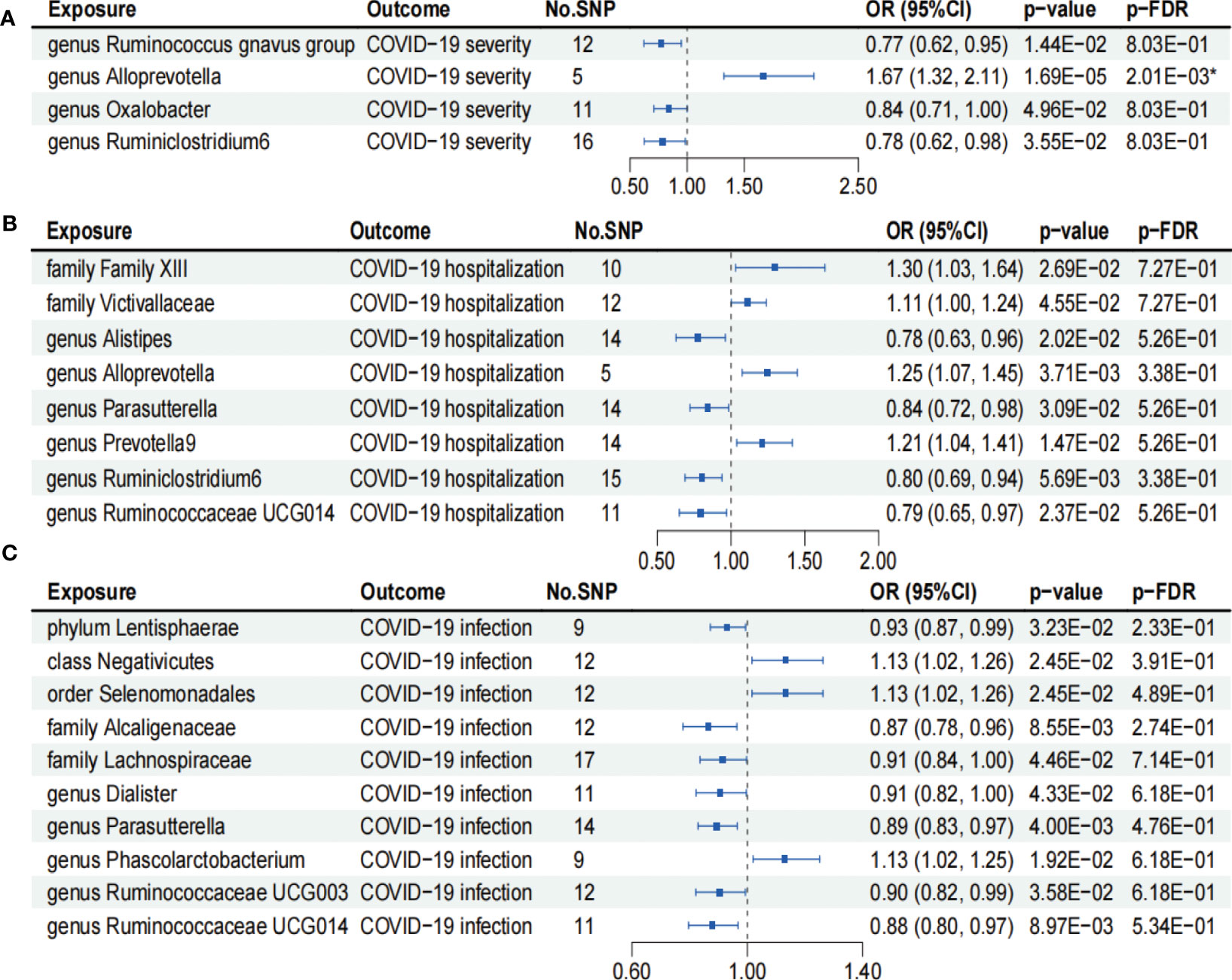
Figure 2 Forests plot of causal effects of gut microbiome on COVID-19 phenotypes (p-IVW > 0.05). (A) COVID-19 severity. (B) COVID-19 hospitalization. (C) COVID-19 infection. FDR, false discovery rate. * p-FDR < 0.05. E-01 represents 10−1.
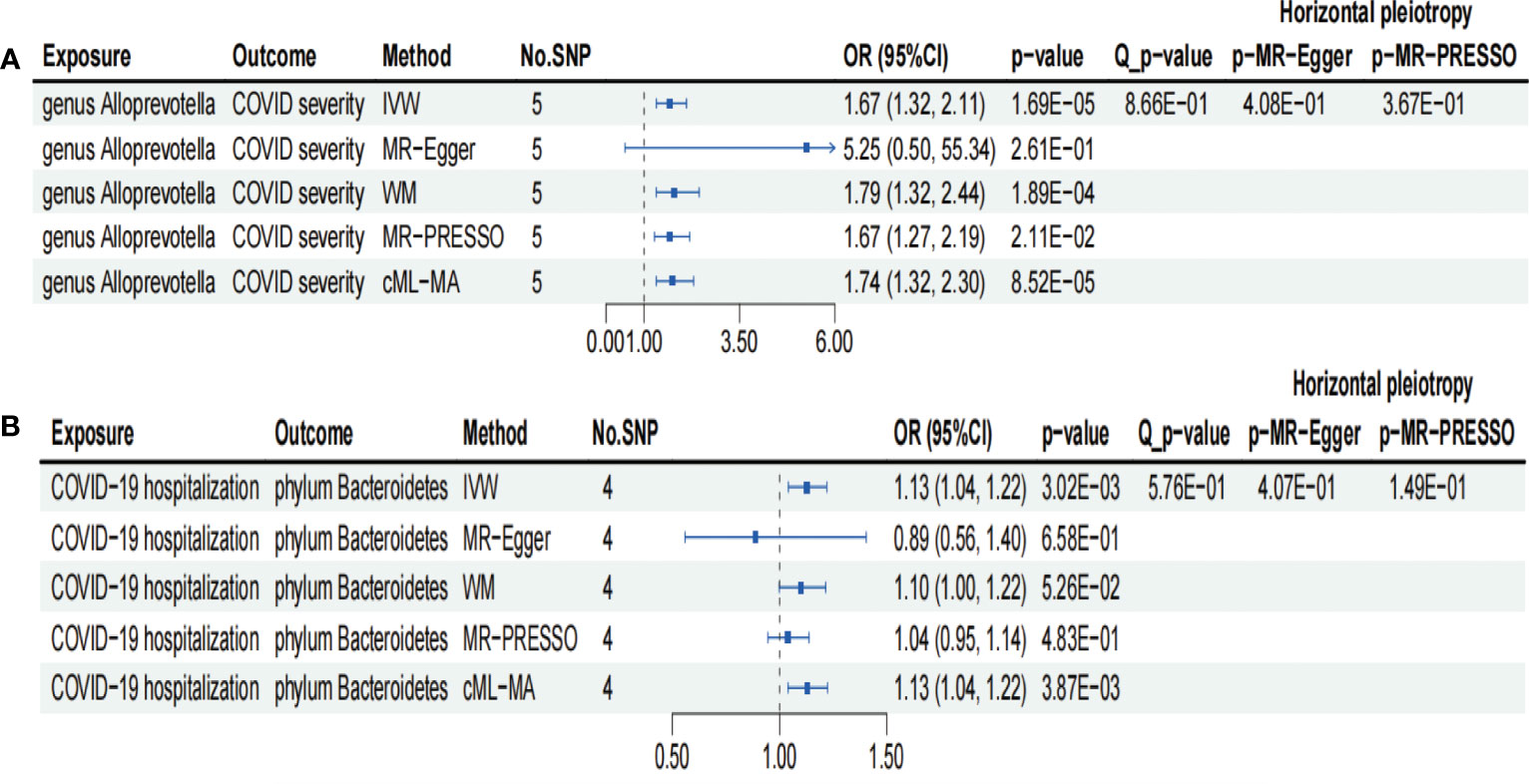
Figure 3 Forests plot of sensitivity analyses for two associations. (A) Genus Alloprevotella with COVID-19 severity. (B) COVID-19 hospitalization with phylum Bacteroidetes. IVs, instrumental variables; WM, weighted median; MR-PRESSO, MR pleiotropy residual sum and outlier; cML-MA, constrained maximum likelihood and model averaging-based. E-01 represents 10−1.
According to the results of the IVW analysis presented in Figure 2B, genus Alistipes (OR = 0.78; 95% CI, 0.63–0.96; p = 2.02×10−2), genus Parasutterella (OR = 0.84; 95% CI, 0.72–0.98; p = 3.09×10−2), genus Ruminiclostridium6 (OR = 0.80; 95% CI, 0.69–0.94; p = 5.69×10−3), and genus Ruminococcaceae UCG014 (OR = 0.79; 95% CI, 0.65–0.97; p = 2.37×10−2) showed suggestive associations with a reduced risk for COVID-19 hospitalization. Conversely, family Family XIII (OR = 1.30; 95% CI, 1.03–1.64; p = 2.69×10−2), family Victivallaceae (OR = 1.11; 95% CI, 1.00–1.24; p = 4.55×10−2), genus Alloprevotella (OR = 1.25; 95% CI, 1.07–1.45; p = 3.71×10−3), and genus Prevotella9 (OR = 1.21; 95% CI, 1.04–1.41; p = 1.47×10−2) exhibited suggestive associations with an increased risk for COVID-19 hospitalization. However, the aforementioned associations ceased to be statistically significant once they underwent FDR correction (p-FDR > 0.05).
We found that phylum Lentisphaerae (OR = 0.93; 95% CI, 0.87–0.99; p = 3.23×10−2), family Alcaligenaceae (OR = 0.87; 95% CI, 0.78–0.96; p = 8.55×10−3), family Lachnospiraceae (OR = 0.91; 95% CI, 0.84–1.00; p = 4.46×10−2), genus Dialister (OR = 0.91; 95% CI, 0.82–1.00; p = 4.33×10−2), genus Parasutterellaon (OR = 0.89; 95% CI, 0.83–0.97; p = 4.00×10−3), genus Ruminococcaceae UCG003 (OR = 0.90; 95% CI, 0.82–0.99; p = 3.58×10−2), and genus Ruminococcaceae UCG014 (OR = 0.88; 95% CI, 0.80–0.97; p = 8.97×10−3) showed suggestive associations with a reduced risk for COVID-19 infection using the IVW method (Figure 2C). On the other hand, class Negativicutes (OR = 1.13, 95% CI, 1.02–1.26; p = 2.45×10−2), order Selenomonadales (OR = 1.13; 95% CI, 1.02–1.26; p = 2.45×10−2), and genus Phascolarctobacterium (OR = 1.13; 95% CI, 1.02–1.25; p = 1.92×10−2) exhibited suggestive associations with a higher risk for COVID-19 infection (Figure 2C). After FDR correction, the aforementioned associations lost their statistical significance (p-FDR > 0.05).
3.2 Causal effects of COVID-19 phenotypes on gut microbiome
In the reverse MR (COVID-19 as the exposure), we identified 1233, 827 and 1033 SNPs associated with COVID-19 severity, hospitalization, and infection respectively. All SNPs of gut microbiome included in the analysis had an F-statistic greater than 10 (Table S4), suggesting the absence of weak bias of IVs. Additionally, the adjusted IVW results, which considered heterogeneity, are presented in Table S5.
As shown in Figure 4A, IVW analysis suggested that COVID-19 severity showed a suggestive association with a decreased abundance of the genus Ruminococcus1 (OR = 0.94; 95% CI, 0.90–0.99; p = 1.83×10−2). In contrast, COVID-19 severity exhibited suggestive associations with an increased abundance of the genus Candidatus Soleaferrea (OR = 1.09; 95% CI, 1.00–1.18; p = 4.22×10−2), genus Olsenella (OR = 1.15; 95% CI, 1.04–1.28; p = 6.26×10−3), and genus Parasutterella (OR = 1.08; 95% CI, 1.02–1.14; p = 1.35×10−2). Nevertheless, the aforementioned associations between COVID-19 severity and gut microbiome lost their statistical significance after undergoing FDR correction (p-FDR > 0.05).
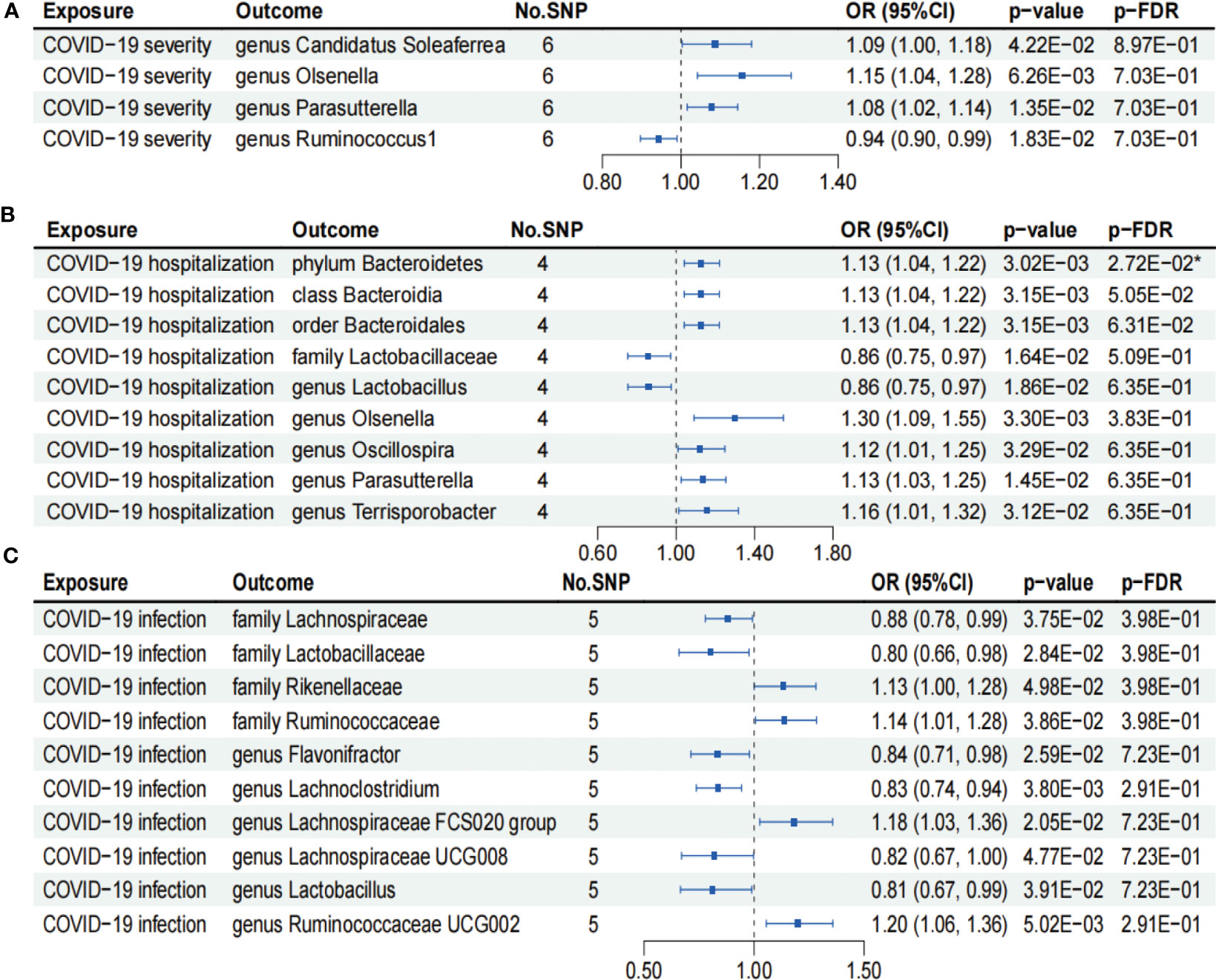
Figure 4 Forests plot of causal effects of COVID-19 phenotypes on gut microbiome (p-IVW > 0.05). (A) COVID-19 severity. (B) COVID-19 hospitalization. (C) COVID-19 infection. FDR, false discovery rate. * p-FDR < 0.05. E-01 represents 10−1.
As presented in Figure 4B, IVW analysis suggested that COVID-19 hospitalization showed suggestive associations with a decreased abundance of the family Lactobacillaceae (OR = 0.86; 95% CI, 0.75–0.97; p = 1.64×10−2) and genus Lactobacillus (OR = 0.86; 95% CI, 0.75–0.97; p = 1.86×10−2). On the other hand, COVID-19 hospitalization exhibited suggestive associations with a higher abundance of the class Bacteroidia (OR = 1.13; 95% CI, 1.04–1.22; p = 3.15×10−3), order Bacteroidales (OR = 1.13; 95% CI, 1.04–1.22; p = 3.15×10−3), genus Oscillospira (OR = 1.12; 95% CI, 1.01–1.25; p = 3.29×10−2), genus Parasutterella (OR = 1.13; 95% CI, 1.03–1.25; p = 1.45×10−2), and genus Terrisporobacter (OR = 1.16; 95% CI, 1.01–1.32; p = 3.12×10−2). Notably, COVID-19 hospitalization was causally associated with an increased abundance of phylum Bacteroidetes (OR = 1.13; 95% CI, 1.04–1.22; p = 3.02×10−3), even after FDR correction (p-FDR = 2.72×10−2).
We found that COVID-19 infection showed suggestive associations with a decreased abundance of the family Lachnospiraceae (OR = 0.88; 95% CI, 0.78–0.99; p = 3.75×10−2), family Lactobacillaceae (OR = 0.80; 95% CI, 0.66–0.98; p = 2.84×10−2), genus Flavonifractor (OR = 0.84; 95% CI, 0.71–0.98; p = 2.59×10−2), genus Lachnoclostridium (OR = 0.83; 95% CI, 0.74–0.94; p = 3.80×10−3), genus Lachnospiraceae UCG008 (OR = 0.82; 95% CI, 0.67–1.00; p = 4.77×10−2), and genus Lactobacillus (OR = 0.81; 95% CI, 0.67–0.99; p = 3.91×10−2) using the IVW analysis (Figure 4C). In contrast, COVID-19 infection exhibited suggestive associations with an increased abundance of the family Rikenellaceae (OR = 1.13; 95% CI, 1.00–1.28; p = 4.98×10−2), family Ruminococcaceae (OR = 1.14; 95% CI, 1.01–1.28; p = 3.86×10−2), genus Lachnospiraceae FCS020 group (OR = 1.18; 95% CI, 1.03–1.36; p = 2.05×10−2), and genus Ruminococcaceae UCG002 (OR = 1.20; 95% CI, 1.06–1.36; p = 5.02×10−3) (Figure 4C). However, the aforementioned associations between COVID-19 infection and gut microbiome failed to pass the FDR correction test (p-FDR > 0.05).
3.3 Sensitivity analyses
We conducted several sensitivity analyses to evaluate the robustness of the MR estimates of the two associations which passed the FDR correction test (genus Alloprevotella with COVID-19 severity; COVID-19 hospitalization with phylum Bacteroidetes; Figure 3). Results from the MR-Egger test and MR-PRESSO global test indicated the absence of horizontal pleiotropy (p-MR-Egger > 0.05 and p-MR-PRESSO > 0.05) in the two associations. The MR-PRESSO analysis revealed that there were no outlier SNPs in the MR results. However, MR-Egger analysis suggested a different direction for the causal estimate of COVID-19 hospitalization on the phylum Bacteroidetes compared to IVW, WM, MR-PRESSO, and cML-MA analyses (Figure 3B). Therefore, the result of causal estimate of COVID-19 hospitalization on phylum Bacteroidetes requires careful consideration. Additionally, the “leave-one-out analysis” indicated that excluding any individual SNP did not significantly alter the overall results of the two associations (Figure 5).
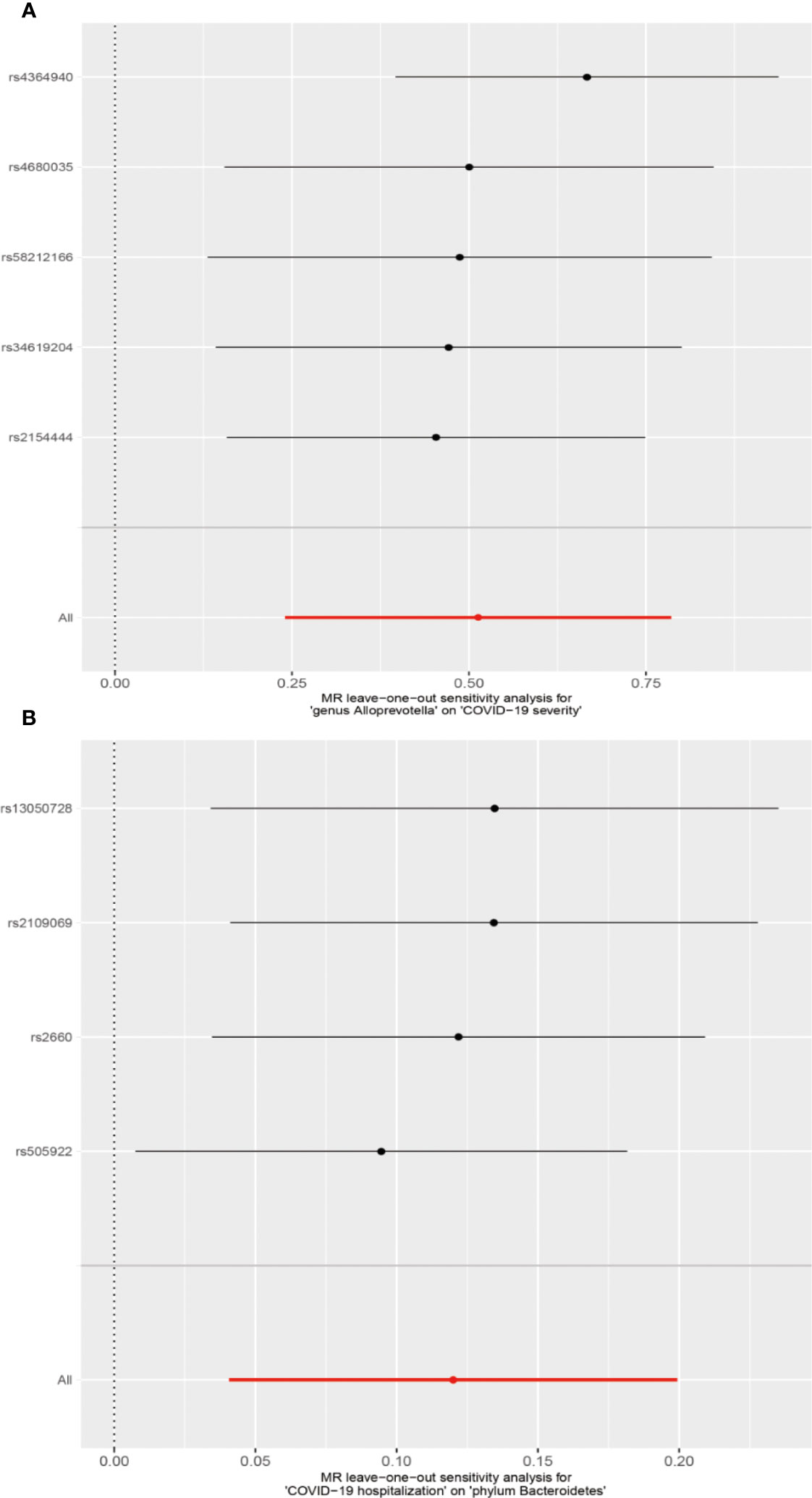
Figure 5 MR leave-one-out sensitivity analysis for two associations. (A) Genus Alloprevotella with COVID-19 severity. (B) COVID-19 hospitalization with phylum Bacteroidetes.
4 Discussion
The association between the gut microbiome and COVID-19 has been of great interest. In the study, we conducted the bi-directional MR analysis to assess the causal effects and casual directions between gut microbiome and COVID-19 phenotypes. We identified 45 links between gut microbiome and COVID-19 phenotypes, of which 43 were suggestive links and two were strong links. Following the FDR correction, we found that the genus Alloprevotella was causally related with a higher risk of COVID-19 severity, while COVID-19 hospitalization was causally linked to an increase in the abundance of the phylum Bacteroidetes. As far as we know, this is the first bidirectional MR study to comprehensively investigate the causal association between gut microbiome and COVID-19.
It has suggested that the gut microbiome could modulate the host’s immune system, potentially affecting the disease process of COVID-19 (3, 10, 11). In this study, we found that several bacterial taxa, including the phylum Lentisphaerae (infection), family Alcaligenaceae (infection), family Lachnospiraceae (infection), genus Ruminococcus gnavus group (severity), genus Oxalobacter (severity), genus Ruminiclostridium6 (severity, hospitalization), genus Alistipes (hospitalization), genus Parasutterella (hospitalization, infection), genus Ruminococcaceae UCG003 (infection), genus Ruminococcaceae UCG014 (hospitalization, infection), and genus Dialister (infection), had suggestive protective effects against COVID-19 phenotypes. Short-chain fatty acids (SCFAs), which belonged to immunomodulatory metabolites, played a vital role in alleviating pulmonary diseases (56). SCFAs might regulate lung immunity through the following mechanisms (10). First, SCFAs directly migrated to lung tissues through the circulation and exerted regulatory effects on pulmonary immunity (10). Second, SCFAs stimulated the differentiation and activation of B cells, leading to the production of immunoglobulin A. In the lung, immunoglobulin A facilitated the clearance of viruses (57). Third, SCFAs enhanced the differentiation and activation of Treg cells, which produced IL-10 and TGF-β, thereby reducing lung inflammation and injury (58, 59). Several studies revealed that the above bacteria, including the family Lachnospiraceae (14), genus Ruminococcus gnavus group (60), genus Alistipes (14), and genus Parasutterella (61), had the capability to produce short-chain fatty acids and exerted an anti-inflammatory effect, thereby potentially alleviating COVID-19 symptoms (3, 14). Interestingly, clinical trials have demonstrated that supplementing with the family Lachnospiraceae could be an effective way to enhance recovery from COVID-19 and alleviate associated symptoms (26, 62, 63). On the other hand, the family XIII (hospitalization), family Victivallaceae (hospitalization), class Negativicutes (infection), order Selenomonadales (infection), genus Alloprevotella (hospitalization), genus Prevotella9 (hospitalization), and genus Phascolarctobacterium (infection) were found to have suggestive contributory effects on COVID-19 phenotypes. Specifically, the IVW analysis suggested that the genus Alloprevotella was casually associated with a higher risk of COVID-19 severity after FDR correction. In addition, it was found that the genus Alloprevotella was enriched in COVID-19 hospitalized patients at the nasopharynx (64, 65). Previous studies have suggested that genus Prevotella9 (66), genus Alloprevotella (64, 67), and genus Phascolarctobacterium (68) exhibited increased inflammatory properties and were thought to be clinically important pathobionts involved in promoting chronic inflammation. This might explain why these bacteria pose a risk for COVID-19. Taken together, these findings highlight the significance of gut microbiome as a modifiable factor in enhancing the outlook of COVID-19.
Previous research has revealed that COVID-19 patients often suffer from various gastrointestinal reactions (8, 9). In this study, IVW analysis revealed that COVID-19 phenotypes could potentially reduce the abundance of the family Lactobacillaceae (hospitalization, infection), family Lachnospiraceae (infection), genus Ruminococcus1 (severity), genus Lactobacillus (hospitalization, infection), genus Flavonifractor (infection), genus Lachnoclostridium (infection), and genus Lachnospiraceae UCG008 (infection). In addition, COVID-19 phenotypes potentially increased the abundance of the class Bacteroidia (hospitalization), order Bacteroidales (hospitalization), family Rikenellaceae (infection), family Ruminococcaceae (infection), genus Candidatus Soleaferrea (severity), genus Olsenella (severity, hospitalization), genus Parasutterella (severity, hospitalization), genus Oscillospira (hospitalization), genus Terrisporobacter (hospitalization), genus Lachnospiraceae FCS020 group (infection), and genus Ruminococcaceae UCG002 (infection). Notably, COVID-19 hospitalization was found to be casually associated with an increased abundance of the phylum Bacteroidetes after FDR correction by the IVW analysis, although MR-Egger suggested a different causal direction. Recent studies have also observed a decrease in the abundance of the family Lachnospiraceae (69) and an increase in the abundance of the phylum Bacteroidetes (15, 70–72), family Ruminococcaceae (69, 73), and genus Oscillospira (16) subsequent to SARS-CoV-2 infection. We therefore deem that COVID-19 may exacerbate disease symptoms by disrupting the gut microbiota homeostasis. Generally speaking, these findings support the notion that COVID‐19 has impacts on gut microbiome dysbiosis through the “gut-lung axis”.
In the study, we observed a notable correlation between the gut microbiome residing in the gastrointestinal tract and the clinical outcomes of SARS-CoV-2 infection. Additionally, we discovered that SARS-CoV-2 infection had the potential to induce modifications in the gut microbiome. These findings provided support for the bidirectional interaction between the gut and the lung known as the “gut-lung axis” (74). The gut microbiome reportedly played a crucial role in modulating immune responses in the lung (3, 10). An underlying mechanism by which the gut microbiome contributed to influencing the outcomes of COVID-19 is via the activities of its metabolites (e.g., SCFAs) (11, 29). The potential exists for the gut microbiome, along with its metabolites, to impact the gene expression of type I interferon (IFN-I) receptors in respiratory epithelial cells. This, in turn, could restrict the proliferation of influenza viruses by stimulating the production of IFN-α and IFN-β (75, 76). Furthermore, the metabolites originating from the gut microbiome had the capacity to stimulate the migration of dendritic cells from the lung to the draining lymph node and promote T-cell priming through the activation of inflammasomes (77). On the other hand, the gut microbiome stimulated the release of inflammatory factors that disseminated throughout the body, exerting their effects on various mucosal tissues and exacerbating the cytokine storm, thereby exacerbating the severity of the condition (23). In this study, we observed a causal association between the genus Alloprevotella and an elevated risk of severe COVID-19. A study has indicated a positive correlation between the abundance of genus Alloprevotella and the level of C-reactive protein, a well-known marker of inflammation (78). We hypothesized that the genus Alloprevotella could promote inflammation and, thus, exacerbate the disease. However, research focused on the direct influence of the gut microbiome in COVID-19 remains limited.
SARS-CoV-2 has been suggested to potentially spread from the lung through transportation via immune cells within the circulatory and lymphatic systems (3, 23). Direct infection of gut epithelial cells by SARS-CoV-2 compromised the integrity of the gut barrier and facilitated microbial translocation. This cascade set off a cytokine storm, exacerbating dysregulation in the gut microbiome, metabolites, electrolytes, and gut barrier functions (3, 79). The invasion of SARS-CoV-2 could trigger the activation of pattern-recognition receptors, which were recognized by innate immune cells. This activation led to the release of diverse pro-inflammatory cytokines (80). These immune responses, once activated, could potentially impair gut permeability, disturb the equilibrium of gut microbiome, and lead to an increase in opportunistic pathogens (e.g., Bacteroidetes) and a decrease in commensal symbionts (e.g., Lactobacillus). On the other hand, ACE2 receptors in the gut appeared to be a critical factor in mediating the interaction between SARS-CoV-2 and the gut microbiome. SARS-CoV-2 bound to the ACE2 receptor, leading to a decrease in ACE2 receptor concentration and consequently in the diversity of the gut microbiome (3, 74, 81). COVID-19 has been shown to impact the gut microbiome in this study, specifically phylum Bacteroidetes, potentially through these mechanisms.
There are several interventions based on the gut microbiome that show potential for addressing COVID-19. First, the gut microbiome can serve as biomarkers for predicting the prognosis of COVID-19. Previous studies have suggested an association between the gut microbiome and the prognosis of COVID-19 (17–19). The microbiota associated with COVID-19, identified in this study, can also be considered as markers for predicting disease progression in COVID-19 (82). Second, a meta-analysis of 1198 patients indicated that probiotics could alleviate symptoms and immune responses and reduce the duration of symptoms in patients with COVID-19 (83). These findings demonstrate the ability of probiotics to effectively reshape gut microbiome homeostasis and reduce inflammatory responses, ultimately acting as adjuvants against SARS-CoV-2. Third, fecal transplants might represent a safe intervention to alleviate gastrointestinal symptoms and modulate immune responses (84, 85). Fourth, meta-analysis indicated that bacterial co-infections were infrequent and that widespread antibiotic usage did not improve the clinical outcome of COVID-19 (86). Therefore, it is crucial to implement antimicrobial stewardship to prevent antibiotic-induced dysbiosis of the gut microbiota and mitigate the risks of disease severity and antimicrobial resistance (87, 88).
This study exhibited several strengths. First, this study utilized the largest publicly available GWAS data of gut microbiome and COVID-19 from over 1.8 million individuals with different ethnicities, providing reliable evidence to elucidate the association between gut microbiome and COVID-19. Second, previous epidemiological studies might be prone to biases due to confounders or reverse causation, but the MR design could effectively minimize these biases. Third, stringent quality control procedures and multiple sensitivity analysis approaches were employed in this study to assess the robustness of the MR estimates (30). Fourth, the potential links identified in this study could assist in further investigations into the mechanisms underlying the links between gut microbiome and COVID-19 phenotypes.
The study’s limitations, nevertheless, must be acknowledged. Due to a lack of sufficient SNPs (less than three) after linkage disequilibrium, we relaxed the p-value threshold (p lower than 1.0×10–5) of SNPs of gut microbiome (gut microbiome as the exposure) in accordance with previous studies (41–47), which might result in weak instrumental variables. To address this issue, we calculated the F-statistics to measure the power of each SNP. All SNPs used in the study having an F-statistic greater than 10 indicated the absence of weak instrument bias. Additionally, bacterial taxa at the species level were not available. Further research is required to elucidate the causal links between the species of gut microbiome and COVID-19 phenotypes.
By conducting the bi-directional MR analysis using the publicly available GWAS summary data, we comprehensively explored the causal link between gut microbiome and COVID-19. This study revealed the interaction between gut microbiome and COVID-19 through the “gut-lung axis”. These findings support the notion that the gut microbiome can serve as an intervention target and may offer new insights into preventing, diagnosing and treating COVID-19.
Data availability statement
The original contributions presented in the study are included in the article/Supplementary Materials. Further inquiries can be directed to the corresponding authors.
Ethics statement
Ethical review and approval was not required for the study on human participants in accordance with the local legislation and institutional requirements. The patients/participants provided their written informed consent to participate in this study.
Author contributions
ZL, GZ, LZ, and LS conceived and designed the study. ZL and GZ wrote the original manuscript, analyzed the data, and drew the figures. LZ and LS supervised the study with funding support. LZ and LS took responsibility for the contents of the article. All authors critically reviewed and revised the manuscript. All authors contributed to the article and approved the submitted version.
Funding
LS was supported by the Free Exploration Research Project of the Second Affiliated Hospital of Xi’an Jiaotong University (Grant number: 2020YJ(ZYTS)282), Hospital Research Fund of the Second Affiliated Hospital of Xi’an Jiaotong University (Grant number: YJ(ZD)201706), and Wu Jieping Medical Foundation (320.6750.2021-16-32). LZ was supported by the Bill and Melinda Gates Foundation (INV-006104). The study design, data collection, analysis, interpretation, and decision to submit the article for publication were performed independently of the sponsors.
Acknowledgments
The authors gratefully thank the COVID-19 Host Genetics Initiative and the MiBioGen consortium for providing GWAS summary data.
Conflict of interest
The authors declare that the research was conducted in the absence of any commercial or financial relationships that could be construed as a potential conflict of interest.
Publisher’s note
All claims expressed in this article are solely those of the authors and do not necessarily represent those of their affiliated organizations, or those of the publisher, the editors and the reviewers. Any product that may be evaluated in this article, or claim that may be made by its manufacturer, is not guaranteed or endorsed by the publisher.
Supplementary material
The Supplementary Material for this article can be found online at: https://www.frontiersin.org/articles/10.3389/fimmu.2023.1217615/full#supplementary-material
References
1. Sachs JD, Karim SSA, Aknin L, Allen J, Brosbol K, Colombo F, et al. The Lancet commission on lessons for the future from the COVID-19 pandemic. Lancet (2022) 400(10359):1224–80. doi: 10.1016/S0140-6736(22)01585-9
2. Munblit D, Nicholson TR, Needham DM, Seylanova N, Parr C, Chen J, et al. Studying the post-COVID-19 condition: research challenges, strategies, and importance of core outcome set development. BMC Med (2022) 20(1):50. doi: 10.1186/s12916-021-02222-y
3. Zhang F, Lau RI, Liu Q, Su Q, Chan FKL, Ng SC. Gut microbiota in COVID-19: key microbial changes, potential mechanisms and clinical applications. Nat Rev Gastroenterol Hepatol (2022) 20:1–15. doi: 10.1038/s41575-022-00698-4
4. Eleftheriotis G, Tsounis EP, Aggeletopoulou I, Dousdampanis P, Triantos C, Mouzaki A, et al. Alterations in gut immunological barrier in SARS-CoV-2 infection and their prognostic potential. Front Immunol (2023) 14:1129190. doi: 10.3389/fimmu.2023.1129190
5. Lamers MM, Beumer J, van der Vaart J, Knoops K, Puschhof J, Breugem TI, et al. SARS-CoV-2 productively infects human gut enterocytes. Science (2020) 369(6499):50–4. doi: 10.1126/science.abc1669
6. Zang R, Gomez Castro MF, McCune BT, Zeng Q, Rothlauf PW, Sonnek NM, et al. TMPRSS2 and TMPRSS4 promote SARS-CoV-2 infection of human small intestinal enterocytes. Sci Immunol (2020) 5(47). doi: 10.1126/sciimmunol.abc3582
7. Zhang H, Shao B, Dang Q, Chen Z, Zhou Q, Luo H, et al. Pathogenesis and mechanism of gastrointestinal infection with COVID-19. Front Immunol (2021) 12:674074. doi: 10.3389/fimmu.2021.674074
8. Elshazli RM, Kline A, Elgaml A, Aboutaleb MH, Salim MM, Omar M, et al. Gastroenterology manifestations and COVID-19 outcomes: a meta-analysis of 25,252 cohorts among the first and second waves. J Med Virol (2021) 93(5):2740–68. doi: 10.1002/jmv.26836
9. Mao R, Qiu Y, He JS, Tan JY, Li XH, Liang J, et al. Manifestations and prognosis of gastrointestinal and liver involvement in patients with COVID-19: a systematic review and meta-analysis. Lancet Gastroenterol Hepatol (2020) 5(7):667–78. doi: 10.1016/S2468-1253(20)30126-6
10. Rastogi S, Mohanty S, Sharma S, Tripathi P. Possible role of gut microbes and host's immune response in gut-lung homeostasis. Front Immunol (2022) 13:954339. doi: 10.3389/fimmu.2022.954339
11. de Oliveira GLV, Oliveira CNS, Pinzan CF, de Salis LVV, Cardoso CRB. Microbiota modulation of the gut-lung axis in COVID-19. Front Immunol (2021) 12:635471. doi: 10.3389/fimmu.2021.635471
12. Gilbert JA, Blaser MJ, Caporaso JG, Jansson JK, Lynch SV, Knight R. Current understanding of the human microbiome. Nat Med (2018) 24(4):392–400. doi: 10.1038/nm.4517
13. Seong H, Kim JH, Han YH, Seo HS, Hyun HJ, Yoon JG, et al. Clinical implications of gut microbiota and cytokine responses in coronavirus disease prognosis. Front Immunol (2023) 14:1079277. doi: 10.3389/fimmu.2023.1079277
14. Zuo T, Liu Q, Zhang F, Lui GC, Tso EY, Yeoh YK, et al. Depicting SARS-CoV-2 faecal viral activity in association with gut microbiota composition in patients with COVID-19. Gut (2021) 70(2):276–84. doi: 10.1136/gutjnl-2020-322294
15. Sun Z, Song ZG, Liu C, Tan S, Lin S, Zhu J, et al. Gut microbiome alterations and gut barrier dysfunction are associated with host immune homeostasis in COVID-19 patients. BMC Med (2022) 20(1):24. doi: 10.1186/s12916-021-02212-0
16. Romani L, Del Chierico F, Macari G, Pane S, Ristori MV, Guarrasi V, et al. The relationship between pediatric gut microbiota and SARS-CoV-2 infection. Front Cell Infect Microbiol (2022) 12:908492. doi: 10.3389/fcimb.2022.908492
17. Troseid M, Holter JC, Holm K, Vestad B, Sazonova T, Granerud BK, et al. Gut microbiota composition during hospitalization is associated with 60-day mortality after severe COVID-19. Crit Care (2023) 27(1):69. doi: 10.1186/s13054-023-04356-2
18. Schult D, Reitmeier S, Koyumdzhieva P, Lahmer T, Middelhoff M, Erber J, et al. Gut bacterial dysbiosis and instability is associated with the onset of complications and mortality in COVID-19. Gut Microbes (2022) 14(1):2031840. doi: 10.1080/19490976.2022.2031840
19. Guo M, Wu G, Tan Y, Li Y, Jin X, Qi W, et al. Guild-level microbiome signature associated with COVID-19 severity and prognosis. mBio (2023) 14(1):e0351922. doi: 10.1128/mbio.03519-22
20. Salaris C, Scarpa M, Elli M, Bertolini A, Guglielmetti S, Pregliasco F, et al. Lacticaseibacillus paracasei DG enhances the lactoferrin anti-SARS-CoV-2 response in Caco-2 cells. Gut Microbes (2021) 13(1):1961970. doi: 10.1080/19490976.2021.1961970
21. Rossini V, Tolosa-Enguis V, Frances-Cuesta C, Sanz Y. Gut microbiome and anti-viral immunity in COVID-19. Crit Rev Food Sci Nutr (2022), 1–16. doi: 10.1080/10408398.2022.2143476
22. Hussain I, Cher GLY, Abid MA, Abid MB. Role of gut microbiome in COVID-19: an insight into pathogenesis and therapeutic potential. Front Immunol (2021) 12:765965. doi: 10.3389/fimmu.2021.765965
23. Xiang H, Liu QP. Alterations of the gut microbiota in coronavirus disease 2019 and its therapeutic potential. World J Gastroenterol (2022) 28(47):6689–701. doi: 10.3748/wjg.v28.i47.6689
24. Gutierrez-Castrellon P, Gandara-Marti T, Abreu YAAT, Nieto-Rufino CD, Lopez-Orduna E, Jimenez-Escobar I, et al. Probiotic improves symptomatic and viral clearance in Covid19 outpatients: a randomized, quadruple-blinded, placebo-controlled trial. Gut Microbes (2022) 14(1):2018899. doi: 10.1080/19490976.2021.2018899
25. Neris Almeida Viana S, do Reis Santos Pereira T, de Carvalho Alves J, Tianeze de Castro C, Santana C, Henrique Sousa Pinheiro L, et al. Benefits of probiotic use on COVID-19: a systematic review and meta-analysis. Crit Rev Food Sci Nutr (2022), 1–13. doi: 10.1080/10408398.2022.2128713
26. Vaezi M, Ravanshad S, Akbari Rad M, Zarrinfar H, Kabiri M. The effect of synbiotic adjunct therapy on clinical and paraclinical outcomes in hospitalized COVID-19 patients: a randomized placebo-controlled trial. J Med Virol (2023) 95(2):e28463. doi: 10.1002/jmv.28463
27. Nagata N, Takeuchi T, Masuoka H, Aoki R, Ishikane M, Iwamoto N, et al. Human gut microbiota and its metabolites impact immune responses in COVID-19 and its complications. Gastroenterology (2023) 164(2):272–88. doi: 10.1053/j.gastro.2022.09.024
28. Li Z, Wei Y, Zhu G, Wang M, Zhang L. Cancers and COVID-19 risk: a mendelian randomization study. Cancers (Basel) (2022) 14(9). doi: 10.3390/cancers14092086
29. Ancona G, Alagna L, Alteri C, Palomba E, Tonizzo A, Pastena A, et al. Gut and airway microbiota dysbiosis and their role in COVID-19 and long-COVID. Front Immunol (2023) 14:1080043. doi: 10.3389/fimmu.2023.1080043
30. Emdin CA, Khera AV, Kathiresan S. Mendelian randomization. JAMA (2017) 318(19):1925–6. doi: 10.1001/jama.2017.17219
31. Boef AG, Dekkers OM, le Cessie S. Mendelian randomization studies: a review of the approaches used and the quality of reporting. Int J Epidemiol (2015) 44(2):496–511. doi: 10.1093/ije/dyv071
32. Burgess S, Butterworth A, Thompson SG. Mendelian randomization analysis with multiple genetic variants using summarized data. Genet Epidemiol (2013) 37(7):658–65. doi: 10.1002/gepi.21758
33. Au Yeung SL, Zhao JV, Schooling CM. Evaluation of glycemic traits in susceptibility to COVID-19 risk: a mendelian randomization study. BMC Med (2021) 19(1):72. doi: 10.1186/s12916-021-01944-3
34. Baranova A, Cao H, Teng S, Zhang F. A phenome-wide investigation of risk factors for severe COVID-19. J Med Virol (2023) 95(1):e28264. doi: 10.1002/jmv.28264
35. Yao Y, Song H, Zhang F, Liu J, Wang D, Feng Q, et al. Genetic predisposition to blood cell indices in relation to severe COVID-19. J Med Virol (2023) 95(1):e28104. doi: 10.1002/jmv.28104
36. Luo S, Liang Y, Wong THT, Schooling CM, Au Yeung SL. Identifying factors contributing to increased susceptibility to COVID-19 risk: a systematic review of mendelian randomization studies. Int J Epidemiol (2022) 51(4):1088–105. doi: 10.1093/ije/dyac076
37. Skrivankova VW, Richmond RC, Woolf BAR, Davies NM, Swanson SA, VanderWeele TJ, et al. Strengthening the reporting of observational studies in epidemiology using mendelian randomisation (STROBE-MR): explanation and elaboration. BMJ (2021) 375:n2233. doi: 10.1136/bmj.n2233
38. Kurilshikov A, Medina-Gomez C, Bacigalupe R, Radjabzadeh D, Wang J, Demirkan A, et al. Large-Scale association analyses identify host factors influencing human gut microbiome composition. Nat Genet (2021) 53(2):156–65. doi: 10.1038/s41588-020-00763-1
39. Wang J, Kurilshikov A, Radjabzadeh D, Turpin W, Croitoru K, Bonder MJ, et al. Meta-analysis of human genome-microbiome association studies: the MiBioGen consortium initiative. Microbiome (2018) 6(1):101. doi: 10.1186/s40168-018-0479-3
40. Initiative C-HG. The COVID-19 host genetics initiative, a global initiative to elucidate the role of host genetic factors in susceptibility and severity of the SARS-CoV-2 virus pandemic. Eur J Hum Genet (2020) 28(6):715–8. doi: 10.1038/s41431-020-0636-6
41. Li P, Wang H, Guo L, Gou X, Chen G, Lin D, et al. Association between gut microbiota and preeclampsia-eclampsia: a two-sample mendelian randomization study. BMC Med (2022) 20(1):443. doi: 10.1186/s12916-022-02657-x
42. Liu K, Zou J, Fan H, Hu H, You Z. Causal effects of gut microbiota on diabetic retinopathy: a mendelian randomization study. Front Immunol (2022) 13:930318. doi: 10.3389/fimmu.2022.930318
43. Hou T, Dai H, Wang Q, Hou Y, Zhang X, Lin H, et al. Dissecting the causal effect between gut microbiota, DHA, and urate metabolism: a large-scale bidirectional mendelian randomization. Front Immunol (2023) 14:1148591. doi: 10.3389/fimmu.2023.1148591
44. Jin Q, Ren F, Dai D, Sun N, Qian Y, Song P. The causality between intestinal flora and allergic diseases: insights from a bi-directional two-sample mendelian randomization analysis. Front Immunol (2023) 14:1121273. doi: 10.3389/fimmu.2023.1121273
45. Cao J, Wang N, Luo Y, Ma C, Chen Z, Chenzhao C, et al. A cause-effect relationship between graves' disease and the gut microbiome contributes to the thyroid-gut axis: a bidirectional two-sample mendelian randomization study. Front Immunol (2023) 14:977587. doi: 10.3389/fimmu.2023.977587
46. Liu B, Ye D, Yang H, Song J, Sun X, Mao Y, et al. Two-sample mendelian randomization analysis investigates causal associations between gut microbial genera and inflammatory bowel disease, and specificity causal associations in ulcerative colitis or crohn's disease. Front Immunol (2022) 13:921546. doi: 10.3389/fimmu.2022.921546
47. Xiang K, Wang P, Xu Z, Hu YQ, He YS, Chen Y, et al. Causal effects of gut microbiome on systemic lupus erythematosus: a two-sample mendelian randomization study. Front Immunol (2021) 12:667097. doi: 10.3389/fimmu.2021.667097
48. Pierce BL, Ahsan H, Vanderweele TJ. Power and instrument strength requirements for mendelian randomization studies using multiple genetic variants. Int J Epidemiol (2011) 40(3):740–52. doi: 10.1093/ije/dyq151
49. Didelez V, Sheehan N. Mendelian randomization as an instrumental variable approach to causal inference. Stat Methods Med Res (2007) 16(4):309–30. doi: 10.1177/0962280206077743
50. Rees JMB, Wood AM, Burgess S. Extending the MR-egger method for multivariable mendelian randomization to correct for both measured and unmeasured pleiotropy. Stat Med (2017) 36(29):4705–18. doi: 10.1002/sim.7492
51. Verbanck M, Chen CY, Neale B, Do R. Detection of widespread horizontal pleiotropy in causal relationships inferred from mendelian randomization between complex traits and diseases. Nat Genet (2018) 50(5):693–8. doi: 10.1038/s41588-018-0099-7
52. Burgess S, Thompson SG. Interpreting findings from mendelian randomization using the MR-egger method. Eur J Epidemiol (2017) 32(5):377–89. doi: 10.1007/s10654-017-0255-x
53. Bowden J, Davey Smith G, Haycock PC, Burgess S. Consistent estimation in mendelian randomization with some invalid instruments using a weighted median estimator. Genet Epidemiol (2016) 40(4):304–14. doi: 10.1002/gepi.21965
54. Xue H, Shen X, Pan W. Constrained maximum likelihood-based mendelian randomization robust to both correlated and uncorrelated pleiotropic effects. Am J Hum Genet (2021) 108(7):1251–69. doi: 10.1016/j.ajhg.2021.05.014
55. Hemani G, Zheng J, Elsworth B, Wade KH, Haberland V, Baird D, et al. The MR-base platform supports systematic causal inference across the human phenome. Elife (2018) 7. doi: 10.7554/eLife.34408
56. Cait A, Hughes MR, Antignano F, Cait J, Dimitriu PA, Maas KR, et al. Microbiome-driven allergic lung inflammation is ameliorated by short-chain fatty acids. Mucosal Immunol (2018) 11(3):785–95. doi: 10.1038/mi.2017.75
57. Wu W, Sun M, Chen F, Cao AT, Liu H, Zhao Y, et al. Microbiota metabolite short-chain fatty acid acetate promotes intestinal IgA response to microbiota which is mediated by GPR43. Mucosal Immunol (2017) 10(4):946–56. doi: 10.1038/mi.2016.114
58. Sun M, Wu W, Chen L, Yang W, Huang X, Ma C, et al. Microbiota-derived short-chain fatty acids promote Th1 cell IL-10 production to maintain intestinal homeostasis. Nat Commun (2018) 9(1):3555. doi: 10.1038/s41467-018-05901-2
59. Arpaia N, Campbell C, Fan X, Dikiy S, van der Veeken J, deRoos P, et al. Metabolites produced by commensal bacteria promote peripheral regulatory T-cell generation. Nature (2013) 504(7480):451–5. doi: 10.1038/nature12726
60. Ahn JR, Lee SH, Kim B, Nam MH, Ahn YK, Park YM, et al. Ruminococcus gnavus ameliorates atopic dermatitis by enhancing treg cell and metabolites in BALB/c mice. Pediatr Allergy Immunol (2022) 33(1):e13678. doi: 10.1111/pai.13678
61. Ju T, Kong JY, Stothard P, Willing BP. Defining the role of parasutterella, a previously uncharacterized member of the core gut microbiota. ISME J (2019) 13(6):1520–34. doi: 10.1038/s41396-019-0364-5
62. Zrelli S, Amairia S, Zrelli M. Respiratory syndrome coronavirus-2 response: microbiota as lactobacilli could make the difference. J Med Virol (2021) 93(6):3288–93. doi: 10.1002/jmv.26746
63. De Boeck I, Cauwenberghs E, Spacova I, Gehrmann T, Eilers T, Delanghe L, et al. Randomized, double-blind, placebo-controlled trial of a throat spray with selected lactobacilli in COVID-19 outpatients. Microbiol Spectr (2022) 10(5):e0168222. doi: 10.1128/spectrum.01682-22
64. Lai CKC, Cheung MK, Lui GCY, Ling L, Chan JYK, Ng RWY, et al. Limited impact of SARS-CoV-2 on the human naso-oropharyngeal microbiota in hospitalized patients. Microbiol Spectr (2022) 10(6):e0219622. doi: 10.1128/spectrum.02196-22
65. Xu R, Lu R, Zhang T, Wu Q, Cai W, Han X, et al. Temporal association between human upper respiratory and gut bacterial microbiomes during the course of COVID-19 in adults. Commun Biol (2021) 4(1):240. doi: 10.1038/s42003-021-01796-w
66. Haran JP, Bradley E, Zeamer AL, Cincotta L, Salive MC, Dutta P, et al. Inflammation-type dysbiosis of the oral microbiome associates with the duration of COVID-19 symptoms and long COVID. JCI Insight (2021) 6(20). doi: 10.1172/jci.insight.152346
67. Zuo T, Zhang F, Lui GCY, Yeoh YK, Li AYL, Zhan H, et al. Alterations in gut microbiota of patients with COVID-19 during time of hospitalization. Gastroenterology (2020) 159(3):944–55.e8. doi: 10.1053/j.gastro.2020.05.048
68. Nagao-Kitamoto H, Leslie JL, Kitamoto S, Jin C, Thomsson KA, Gillilland MG 3rd, et al. Interleukin-22-mediated host glycosylation prevents clostridioides difficile infection by modulating the metabolic activity of the gut microbiota. Nat Med (2020) 26(4):608–17. doi: 10.1038/s41591-020-0764-0
69. Sencio V, Machelart A, Robil C, Benech N, Hoffmann E, Galbert C, et al. Alteration of the gut microbiota following SARS-CoV-2 infection correlates with disease severity in hamsters. Gut Microbes (2022) 14(1):2018900. doi: 10.1080/19490976.2021.2018900
70. Reinold J, Farahpour F, Fehring C, Dolff S, Konik M, Korth J, et al. A pro-inflammatory gut microbiome characterizes SARS-CoV-2 infected patients and a reduction in the connectivity of an anti-inflammatory bacterial network associates with severe COVID-19. Front Cell Infect Microbiol (2021) 11:747816. doi: 10.3389/fcimb.2021.747816
71. Li S, Yang S, Zhou Y, Disoma C, Dong Z, Du A, et al. Microbiome profiling using shotgun metagenomic sequencing identified unique microorganisms in COVID-19 patients with altered gut microbiota. Front Microbiol (2021) 12:712081. doi: 10.3389/fmicb.2021.712081
72. Al Bataineh MT, Henschel A, Mousa M, Daou M, Waasia F, Kannout H, et al. Gut microbiota interplay with COVID-19 reveals links to host lipid metabolism among middle Eastern populations. Front Microbiol (2021) 12:761067. doi: 10.3389/fmicb.2021.761067
73. Sokol H, Contreras V, Maisonnasse P, Desmons A, Delache B, Sencio V, et al. SARS-CoV-2 infection in nonhuman primates alters the composition and functional activity of the gut microbiota. Gut Microbes (2021) 13(1):1–19. doi: 10.1080/19490976.2021.1893113
74. Ralli T, Saifi Z, Rathee A, Aeri V, Kohli K. Decoding the bidirectional relationship between gut microbiota and COVID-19. Heliyon (2023) 9(3):e13801. doi: 10.1016/j.heliyon.2023.e13801
75. Bradley KC, Finsterbusch K, Schnepf D, Crotta S, Llorian M, Davidson S, et al. Microbiota-driven tonic interferon signals in lung stromal cells protect from influenza virus infection. Cell Rep (2019) 28(1):245–56.e4. doi: 10.1016/j.celrep.2019.05.105
76. Clarke TB, Davis KM, Lysenko ES, Zhou AY, Yu Y, Weiser JN. Recognition of peptidoglycan from the microbiota by Nod1 enhances systemic innate immunity. Nat Med (2010) 16(2):228–31. doi: 10.1038/nm.2087
77. Ichinohe T, Pang IK, Kumamoto Y, Peaper DR, Ho JH, Murray TS, et al. Microbiota regulates immune defense against respiratory tract influenza a virus infection. Proc Natl Acad Sci U S A (2011) 108(13):5354–9. doi: 10.1073/pnas.1019378108
78. Sun Y, Chen Q, Lin P, Xu R, He D, Ji W, et al. Characteristics of gut microbiota in patients with rheumatoid arthritis in shanghai, China. Front Cell Infect Microbiol (2019) 9:369. doi: 10.3389/fcimb.2019.00369
79. Shen S, Gong M, Wang G, Dua K, Xu J, Xu X, et al. COVID-19 and gut injury. Nutrients (2022) 14(20). doi: 10.3390/nu14204409
80. Merad M, Martin JC. Pathological inflammation in patients with COVID-19: a key role for monocytes and macrophages. Nat Rev Immunol (2020) 20(6):355–62. doi: 10.1038/s41577-020-0331-4
81. Stevens BR, Ellory JC, Preston RL. B(0)AT1 amino acid transporter complexed with SARS-CoV-2 receptor ACE2 forms a heterodimer functional unit: In situ conformation using radiation inactivation analysis. Funct (Oxf) (2021) 2(4):zqab027. doi: 10.1093/function/zqab027
82. Neag MA, Vulturar DM, Gherman D, Burlacu CC, Todea DA, Buzoianu AD. Gastrointestinal microbiota: a predictor of COVID-19 severity? World J Gastroenterol (2022) 28(45):6328–44. doi: 10.3748/wjg.v28.i45.6328
83. Tian Y, Ran H, Wen X, Fu G, Zhou X, Liu R, et al. Probiotics improve symptoms of patients with COVID-19 through gut-lung axis: a systematic review and meta-analysis. Front Nutr (2023) 10:1179432. doi: 10.3389/fnut.2023.1179432
84. Liu F, Ye S, Zhu X, He X, Wang S, Li Y, et al. Gastrointestinal disturbance and effect of fecal microbiota transplantation in discharged COVID-19 patients. J Med Case Rep (2021) 15(1):60. doi: 10.1186/s13256-020-02583-7
85. Bilinski J, Winter K, Jasinski M, Szczes A, Bilinska N, Mullish BH, et al. Rapid resolution of COVID-19 after faecal microbiota transplantation. Gut (2022) 71(1):230–2. doi: 10.1136/gutjnl-2021-325010
86. Malik SS, Mundra S. Increasing consumption of antibiotics during the COVID-19 pandemic: implications for patient health and emerging anti-microbial resistance. Antibiotics (Basel) (2022) 12(1). doi: 10.3390/antibiotics12010045
87. Langford BJ, So M, Simeonova M, Leung V, Lo J, Kan T, et al. Antimicrobial resistance in patients with COVID-19: a systematic review and meta-analysis. Lancet Microbe (2023) 4(3):e179–91. doi: 10.1016/S2666-5247(22)00355-X
Keywords: gut microbiota, SARS-CoV-2, COVID-19, Mendelian randomization, causality, rct, randomized controlled trial
Citation: Li Z, Zhu G, Lei X, Tang L, Kong G, Shen M, Zhang L and Song L (2023) Genetic support of the causal association between gut microbiome and COVID-19: a bidirectional Mendelian randomization study. Front. Immunol. 14:1217615. doi: 10.3389/fimmu.2023.1217615
Received: 05 May 2023; Accepted: 20 June 2023;
Published: 07 July 2023.
Edited by:
Linda L. D. Zhong, Nanyang Technological University, SingaporeReviewed by:
Simona Rolla, University of Turin, ItalyDiego Fernández Lázaro, University of Valladolid, Spain
Copyright © 2023 Li, Zhu, Lei, Tang, Kong, Shen, Zhang and Song. This is an open-access article distributed under the terms of the Creative Commons Attribution License (CC BY). The use, distribution or reproduction in other forums is permitted, provided the original author(s) and the copyright owner(s) are credited and that the original publication in this journal is cited, in accordance with accepted academic practice. No use, distribution or reproduction is permitted which does not comply with these terms.
*Correspondence: Lei Zhang, bGVpLnpoYW5nMUBtb25hc2guZWR1; Lingqin Song, c29uZ2xpbmdxaW5AbWFpbC54anR1LmVkdS5jbg==
†These authors have contributed equally to this work and share first authorship
‡ORCID: Zengbin Li, orcid.org/0000-0002-0237-4458