- 1Laboratory of Functional Genomics, HUN-REN Biological Research Centre, Szeged, Hungary
- 2PhD School in Biology, University of Szeged, Szeged, Hungary
- 3National Korányi Institute of Pulmonology, Budapest, Hungary
- 4Laboratory of Bioinformatics, HUN-REN Biological Research Centre, Szeged, Hungary
- 5Department of Biochemistry and Medical Chemistry, Medical School, University of Pécs, Pécs, Hungary
- 6Macrophage Polarization Group, Institute of Genetics, HUN-REN Biological Research Centre, Szeged, Hungary
- 7Synthetic and Systems Biology Unit, Institute of Biochemistry, HUN-REN Biological Research Centre, Szeged, Hungary
- 8Department of Dermatology and Allergology, University of Szeged, Szeged, Hungary
- 9National Institute of Oncology, Budapest, Hungary
- 10Avicor Ltd., Szeged, Hungary
- 11Avidin Ltd., Szeged, Hungary
- 12Department of Physiology, Anatomy and Neuroscience, Faculty of Science and Informatics, University of Szeged, Szeged, Hungary
- 13CS-Smartlab Devices Ltd., Kozármisleny, Hungary
Introduction: Tobacco smoking generates airway inflammation in chronic obstructive pulmonary disease (COPD), and its involvement in the development of lung cancer is still among the leading causes of early death. Therefore, we aimed to have a better understanding of the disbalance in immunoregulation in chronic inflammatory conditions in smoker subjects with stable COPD (stCOPD), exacerbating COPD (exCOPD), or non-small cell lung cancer (NSCLC).
Methods: Smoker controls without chronic illness were recruited as controls. Through extensive mapping of single cells, surface receptor quantification was achieved by single-cell mass cytometry (CyTOF) with 29 antibodies. The CyTOF characterized 14 main immune subsets such as CD4+, CD8+, CD4+/CD8+, CD4−/CD8−, and γ/δ T cells and other subsets such as CD4+ or CD8+ NKT cells, NK cells, B cells, plasmablasts, monocytes, CD11cdim, mDCs, and pDCs. The CD4+ central memory (CM) T cells (CD4+/CD45RA−/CD45RO+/CD197+) and CD4+ effector memory (EM) T cells (CD4+/CD45RA−/CD45RO+/CD197−) were FACS-sorted for RNA-Seq analysis. Plasma samples were assayed by Luminex MAGPIX® for the quantitative measurement of 17 soluble immuno-oncology mediators (BTLA, CD28, CD80, CD27, CD40, CD86, CTLA-4, GITR, GITRL, HVEM, ICOS, LAG-3, PD-1, PD-L1, PD-L2, TIM-3, TLR-2) in the four studied groups.
Results: Our focus was on T-cell-dependent differences in COPD and NSCLC, where peripheral CD4+ central memory and CD4+ effector memory cells showed a significant reduction in exCOPD and CD4+ CM showed elevation in NSCLC. The transcriptome analysis delineated a perfect correlation of differentially expressed genes between exacerbating COPD and NSCLC-derived peripheral CD4+ CM or CD4+ EM cells. The measurement of 17 immuno-oncology soluble mediators revealed a disease-associated phenotype in the peripheral blood of stCOPD, exCOPD, and NSCLC patients.
Discussion: The applied single-cell mass cytometry, the whole transcriptome profiling of peripheral CD4+ memory cells, and the quantification of 17 plasma mediators provided complex data that may contribute to the understanding of the disbalance in immune homeostasis generated or sustained by tobacco smoking in COPD and NSCLC.
1 Introduction
Tobacco smoking frequently causes airway inflammation and oxidative stress linking the pathogenesis of chronic obstructive pulmonary disease (COPD) and lung cancer. In 2019, 212.3 million COPD cases were reported with 3.3 million deaths (1). A WHO survey showed that COPD was the third leading cause of death worldwide in 2019 (2). COPD affects more than 50% of the smoker’s population, and patients with COPD have a two-fold risk of developing lung cancer (3, 4). Previously, our group and others reviewed the molecular players and chronic inflammatory conditions in COPD establishing a lung cancer-prone microenvironment (5, 6). Free radicals such as reactive nitrogen or reactive oxygen species damage the DNA; additionally, the tobacco smoke-induced pulmonary inflammatory milieu and the genetic and epigenetic predisposition increase the mutational burden leading to the development of lung cancer (4, 5). Neoantigens were identified in COPD using mass spectrometry supporting the epidemiology data that COPD has been considered as a prestage of lung cancer (7). Non-small cell lung cancer, the most frequent comorbidity, accounts for 40%–70% of COPD patients (8). It was reported that nearly 87% of lung cancer-related deaths were affected by tobacco smoking in the USA (9). Therefore, the updated US Preventive Services Task Force (USPSTF) guidelines suggest regular diagnostic screening of patients at a minimum age of 50 with a minimum of 20 packs per year of smoking history (10). Lung cancer accounts for almost 23% of all cancer-related mortality and is one of the leading causes of cancer-related deaths with nearly 1.6 million annual deaths worldwide (11, 12).
Our current study aims to dissect the effect of tobacco smoke on the immunophenotype of the peripheral immune system in stable COPD, exacerbating COPD, and NSCLC patients. The current (2023) guideline of the Global Initiative for Chronic Obstructive Lung Disease (GOLD) defines COPD as follows: “heterogeneous lung condition characterized by chronic respiratory symptoms (dyspnea, cough, expectoration and/or exacerbations) due to abnormalities of the airways (bronchitis, bronchiolitis) and/or alveoli (emphysema) that cause persistent, often progressive, airflow obstruction” (13). COPD is a heterogeneous disease that represents a spectrum of disease severity in the range from a stable state to severe exacerbations. Stable COPD is characterized “when symptoms are well managed and pulmonary decline is minimized” (14). The exacerbation of COPD is also redefined by the recent GOLD 2023 guideline as “an event characterized by dyspnea and/or cough and sputum that worsen over ≤14 days, which may be accompanied by tachypnea and/or tachycardia and is often associated with increased local and systemic inflammation caused by airway infection, pollution, or other insult to the airways” (13). The dissection of airway inflammation in COPD is beyond the scope of our current study, but it has been reviewed elsewhere (5, 15, 16).
It is widely known that airway inflammation in COPD is associated with systemic inflammation frequently causing, e.g., cardiovascular disease (17), but the systematic investigation of the peripheral immune system in COPD has not been resolved yet completely. The markers of COPD from the biological fluids such as profiling the peripheral immune system or measuring soluble markers have been the subject of intensive research in the last years (18). Xiong et al. published recently the immunophenotyping of stable COPD and exacerbating COPD patients using an eight-member antibody panel for flow cytometry (FACS) (19). Xiong et al. found that Th1 cells, Th17 cells, Treg cell ratio, Th1/Th2 cell ratio, and the levels of C-reactive protein, interleukin (IL)-6, and IL-10 were significantly increased in patients with exCOPD. Halper-Stromberg et al. found that subjects with COPD had significantly lower levels of aggregated lymphocytes, aggregated T cells, CD4+ resting memory cells, and naive B cells and increased levels of monocytes compared with smoker controls (20). Using single-cell mass cytometry, Vasudevan et al. studied the bronchoalveolar lavage in COPD patients lacking information on the peripheral immune composition (21). Kapellos et al. used single-cell mass cytometry for the immunophenotyping of COPD patient-derived blood, but their study focused on the role of neutrophils in early-stage COPD with limited data on other compartments (22). Therefore, our mass cytometry panel was designed for the dissection of the complexity of peripheral immunity in stCOPD, exCOPD, and NSCLC.
In our study, NSCLC patients were recruited to reveal disturbances of the peripheral immune homeostasis in the context of stable or exacerbating COPD, all cases being affected by tobacco smoking, including smoker healthy volunteers. Molecular profiling and integration of multi-omics data prospectively is a novel approach to stratify patients with clinically heterogeneous diseases. High-resolution measurement characterizing a large number of cellular features was our focus in studying COPD in stable conditions vs. exacerbations. Our question of interest was the understanding of the disbalance in immunoregulation in chronic inflammatory conditions of smoker COPD cases and smoking-associated NSCLC. In our recent work, with extensive mapping of single cells, surface receptor quantification was achieved by cytometry by time-of-flight (CyTOF) with a panel consisting of 29 antibodies. Our aim was the deep immunophenotyping of COPD and NSCLC patients with a special focus on the T-cell compartment in order to shed light on the stCOPD, exCOPD, and NSCLC-associated T-cell phenotypes and the subsets of T-cell diversity. Our antibody panel consisted of markers also for the identification of other subsets such as NK cells, CD4+ NKT, CD8+ NKT, B cells, plasmablasts, monocytes, CD11cdim cells, monocytoid dendritic cells (mDCs), and plasmacytoid dendritic cells (pDCs). The dissection of the complex immunophenotype may contribute to the understanding of the involvement of the immune system in the chronic inflammatory condition caused by tobacco smoking with a special focus on smoking-associated diseases such as COPD and NSCLC.
In our current study, 14 main peripheral immune cell populations were identified and characterized by single-cell mass cytometry. The CyTOF results shed light on the CD4 effector memory and CD4 central memory T cells; therefore, the whole transcriptome profiling was carried out for these FACS-sorted particular cell types in all smoking conditions, such as healthy controls, stable COPD, exacerbating COPD, and NSCLC patient-derived samples. The concentration of 17 soluble mediators was also measured by the Luminex MAGPIX® system from the plasma samples to dissect the pattern of relevant cytokines/chemokines and immune checkpoint modulators circulating in the peripheral blood.
2 Materials and methods
2.1 Study subjects
The following subjects were recruited: 1) smoker healthy controls (SmHC) without chronic illness and without regular medication. 2) Stable COPD patients (stCOPD) were selected according to the guideline of GOLD with a smoking history and without known lung cancer. 3) Acute exacerbating COPD patients (exCOPD) were also selected based on the GOLD guideline; the withdrawal of the blood was before the glucocorticoid therapy. ExCOPD patients were selected with smoking history and without known lung cancer. ExCOPD was defined as increased dyspnea, cough, or sputum expectoration (quality or quantity) that led the subject to seek medical attention, as specified in international guidelines (23). 4) Smoker, non-small cell lung cancer (NSCL) patients were involved before receiving therapy. Bacterial or viral infection during the exacerbation was not tested. Samples were collected from December 2018 to December 2019 before the outbreak of COVID-19. All subjects were over 18 and self-conscious. The subjects gave their informed consent before participating in the study. The study was conducted in accordance with the Declaration of Helsinki, and the protocol (“Immunophenotyping in COPD and lung cancer”) was approved by the Ethics Committee of the National Public Health Center under the 33815-7/2018/EÜIG Project identification code.
2.2 Study design
Subjects were recruited for CyTOF from the following groups: 1) smoker healthy control (without known disease and without regular medication, n = 9), 2) smoker-stable COPD (n = 8) patients, 3) smoker-exacerbating COPD patients before receiving corticosteroid treatment (n = 8), and 4) therapy-naive NSCLC patients without chemotherapy and/or immunotherapy (n = 8). Subjects were recruited for Luminex from the following groups: 1) smoker healthy control (n = 9), 2) smoker-stable COPD (n = 11) patients, 3) smoker-exacerbating COPD patients before receiving corticosteroid treatment (n = 13), and 4) therapy-naive NSCLC patients without chemotherapy and/or immunotherapy (n = 13), This is a cross-sectional study with the collection of 20 mL venous peripheral blood at one time point (before receiving medication where it was applicable). Demographic and clinical data of the patients are summarized in Supplementary Table 1.
2.3 PBMC isolation
After the collection of 20 mL blood into an EDTA vacutainer (Becton Dickinson, Franklin Lakes, USA), PBMCs were purified by Leucosep tubes (Greiner Bio-One, Kremsmünster, Austria) according to the manufacturer’s instructions. If the pellet was light red, 2 mL of ACK lysing buffer (ACK: 0.15 M of NH4Cl, 10 mM of KHCO3, 0.1 mM of Na2EDTA, pH 7.3; Merck, Darmstadt, Germany) was applied at room temperature (RT) for 2 min. Samples were washed twice with 10 mL of PBS (Merck) and subsequently cell counted, and viability check was performed with Trypan Blue exclusion. Cryopreservation of PBMCs was carried out in stocks of 4 × 106 cells of 1 mL of FBS (Capricorn Scientific, Ebsdorfergrund, Germany) supplemented with 1:10 of DMSO (Merk) [v/v] in cryotubes (Greiner Bio-One) in liquid nitrogen (Messer, Bad Soden, Germany).
2.4 Cell preparation for CyTOF
Cells were processed for CyTOF as described previously by our group (24). Briefly, cryotubes were thawed in a 37°C water bath for 2 min, and cells were transferred into a 14-mL 37°C warm RPMI (Capricorn Scientific) and centrifuged at 350×g for 6 min at RT. PBMCs were washed again with 5 mL of Maxpar Staining Buffer (MCSB, Fluidigm, South San Francisco, CA, USA) and centrifuged at 350×g for 6 min at RT, cells were counted in 1 mL of PBS, and viability was determined with Trypan Blue exclusion.
2.5 Antibody staining
The antibody staining of cells for CyTOF was performed as described previously by our group with minor modifications (25, 26). Briefly, viability was determined by cisplatin (5 µM of 195Pt, Fluidigm) staining for 3 min on ice in 300 µL of RPMI. The sample was diluted by 2 mL of RPMI (MCSB, Fluidigm) and centrifuged at 350×g for 5 min. PBMCs were washed again with 4 mL of MCSB and centrifuged at 350×g for 6 min at RT. Cells were resuspended in 50 µL of MCSB supplemented with 1:20 v/v TrueStain FcX™ FC receptor blocking solution (BioLegend, San Diego, USA) and incubated at RT for 10 min. Cells were stained with 29 antibodies of the Human Immune Monitoring Panel (Fluidigm) and incubated at 4°C for 45 min. The antibodies used for CyTOF are listed in Supplementary Table 2. Cells were washed twice with 1 mL of MCSB and prefixed with 1 mL of Pierce™ formaldehyde (Thermo Fisher Scientific, Waltham, MA, USA) solution diluted in PBS to 1.6% and incubated at RT for 10 min. Stained and prefixed cells were centrifuged at 800×g for 6 min at RT and resuspended in 1 mL of Fix & Perm solution (Fluidigm) supplemented with 1:1,000 v/v Ir191/Ir193 DNA intercalator (Fluidigm) for overnight incubation.
2.6 CyTOF data acquisition and data preprocessing
The acquisition of the samples for CyTOF was executed as described previously by our group with minor modifications (27, 28). Briefly, samples were washed three times with MCSB before being filtered through a 30-μm Celltrics (Sysmex Hungaria Kft, Hungary) gravity filter, and the cell concentration was adjusted to 7 × 105/mL in Maxpar Cell Acquisition Solution (Fluidigm). Finally, EQ four-element calibration beads (Fluidigm) were added at a 1:10 ratio (v/v) and acquired on a properly tuned Helios mass cytometer (CyTOF, Fluidigm), and 1 × 106 events per individual PBMC were collected to be able to identify rare cell subsets. The generated flow cytometry standard (FCS) files were randomized and normalized with the default setting of the internal FCS processing unit of the CyTOF software (Fluidigm, version 7.0.8493).
2.7 CyTOF data processing
Randomized and normalized FCS files were uploaded to the Cytobank analysis platform (Beckman Coulter, Brea, USA). Exclusion of normalization beads, dead cells, debris, and doublets was performed. There were no significant differences in cell counts between the examined groups. The FCS files with the CD45-positive living singlets were exported and further analyzed in R. Compensation methodology, FlowSOM clustering, and reduction of dimensionality were adapted from Crowell HL et al. [BioConductor CATALYST, compensation (29): data analysis (30):]. Utilizing the CATALYST and flowCore R package, FCS files were integrated, compensated, and transformed. After signal spillover compensation, CyTOF marker intensities were inverse hyperbolic sine-transformed (arcsinh) with cofactor 5. For the main population definition, we carried out a Self-Organizing Maps-based method (FlowSOM) metaclustering on compensated and transformed files. We identified different metaclusters which were separately subclustered with another round of FlowSOM. High-dimensional reduction and visualization were performed using the t-distributed stochastic neighbor embedding (t-SNE) algorithm/method. A total of 300,000 cells and 29 markers were used to create the peripheral human immune system UMAP (Uniform Manifold Approximation and Projection for Dimension Reduction) (Figure 1).
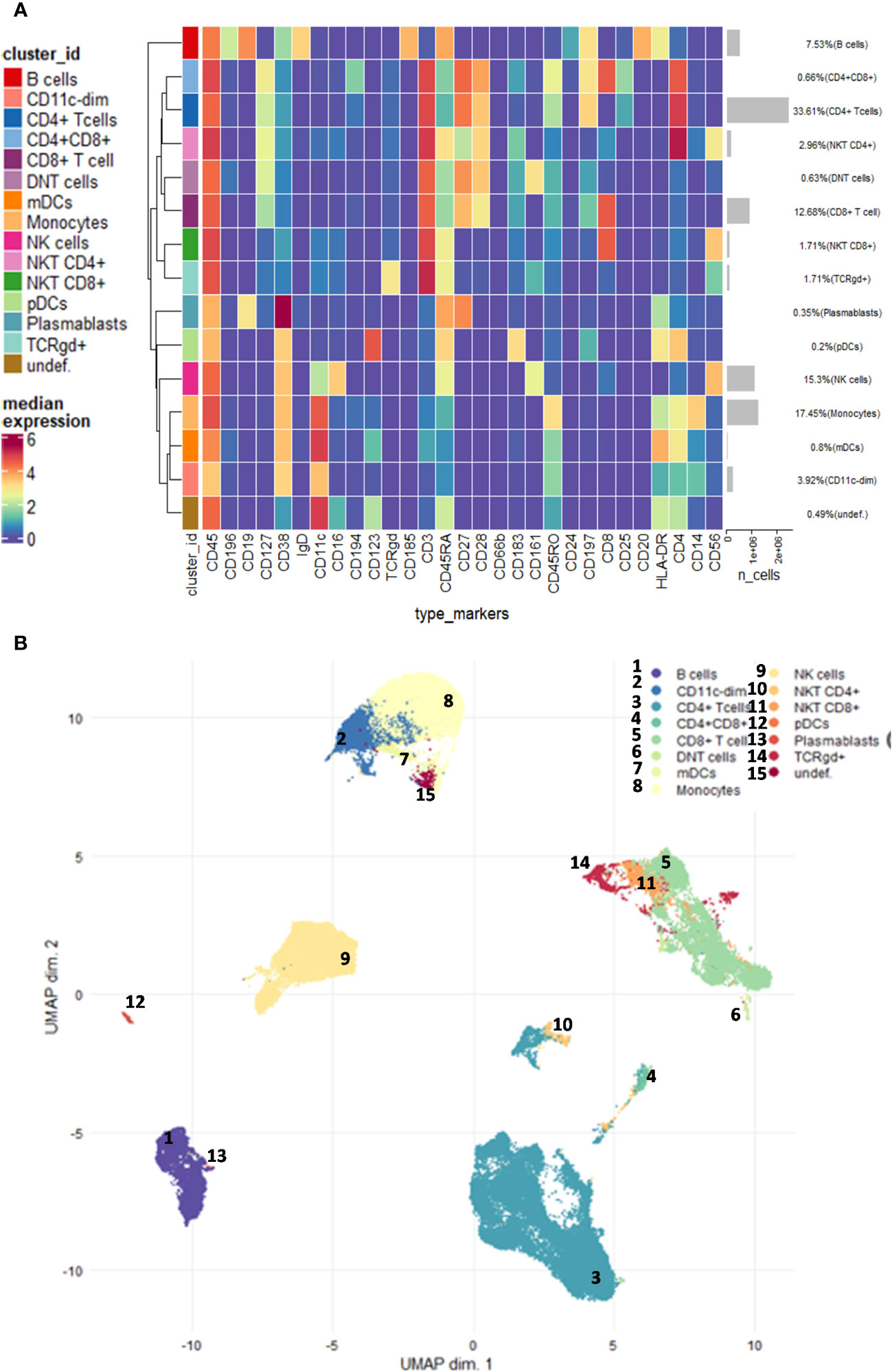
Figure 1 Demonstration of the studied main immune subsets. (A) Unsupervised clustering and the heatmap of expression intensities of the analysed immune cells. (B) The FlowSOM (Self-Organizing Maps for flow cytometry) and UMAP (Uniform Manifold Approximation and Projection for Dimension Reduction) analysis identified 14 main subsets as follows: CD4+ T cells, CD8+ T cells, CD4+CD8+ T cells, DN (double-negative) T cells (CD4−CD8−), γ/δ T cells, NK cells, CD4+ NKT cells, CD8+ NKT cells, plasmablasts, B cells, monocytes, CD11cdim cells, plasmacytoid dendritic cells (pDCs), and monocytoid dendritic cells (mDCs). The analysis was carried out on the entire dataset in the R software including 33 FCS files.
2.8 RNA sequencing
Cell sorting of CD4+ EM or CD4+ CM T cells was carried out. The PBMCs were thawed from liquid nitrogen. Cells were counted in PBS, and viability was determined with Trypan Blue exclusion. Cells were resuspended in 50 µL of PBS supplemented with 1:20 v/v TrueStain FcX™ FC receptor blocking solution (BioLegend) and incubated at RT for 10 min. The enrichment of CD4+ T cells was performed using the Dynabeads™ FlowComp™ Human CD4 Isolation Kit from PBMC following the instruction of the manufacturer (Thermo Fisher Scientific). After titrating the antibodies, the CD4+ T cells were incubated with CD45RA-FITC (Hl100 clone), CD45RO PERC/Cy5.5 (UCHL1 clone), and CD197(CCR7)-PB (G043H7 clone) (BioLegend) 25× diluted in FACS buffer (2% FBS in PBS) in 50 µL at RT for 45 min. After washing with 1 mL of FACS buffer and centrifugation at 350×g for 5 min, cells were resuspended in FACS buffer and 2 mM of EDTA for cell sorting using FACSJazz (Beckman Coulter). Manual gating was used to sort 1) CD4+ EM (CD45RA−/CD45RO+, CD197−) or 2) CD4+ CM (CD45RA−/CD45RO+, CD197+) cells. Cells were centrifuged at 350×g for 5 min and resuspended in 500 µL of TRIzol (Thermo Fisher Scientific) and stored at −80°C. The isolation of RNA was performed using the RNeasy Micro kit (Qiagen, Hilden, Germany). The RNAse inhibitor was added 20 U/µL diluted 100× (Thermo Fisher Scientific). The concentration and purity of RNA was measured using NanoDrop™ One (Thermo Fisher Scientific). Because of the relatively low number of memory cells and yielded RNA from one subject, the RNA samples of one study cohort were pooled in an equivalent manner (the same amount from three to five subjects within one group). The RNA sequencing (Massive Analysis of cDNA Ends = MACE = 3′ mRNA-Seq) was performed by GenXPro GMBH (Frankfurt Am Main, Germany) as a service. The normalized gene expression data of the RNA-Seq are listed in Supplementary Table 3.
2.9 Gene set enrichment analysis
Gene set enrichment analysis was performed using GSEA 4.1.0 (31). We ran the software in CLI mode and analyzed its outputs through custom R scripts, attached as a supplementary R Markdown file (Supplementary File 1).
2.10 Measurement of plasma proteins
The measurement of plasma proteins was performed as described previously by our group with minor modifications (32–34). Briefly, after the withdrawal of 20 mL of blood into an EDTA vacutainer (Becton Dickinson), human peripheral blood mononuclear cells and plasma samples were purified by Leucosep tubes (Greiner Bio-One, Austria). Plasma fractions were stored at −80°C in aliquots before running the assay. Luminex xMAP (MAGPIX®) technology was used to determine the protein concentrations of 17 distinct immuno-oncology checkpoint proteins (BTLA, CD28, CD80, CD27, CD40, CD86, CTLA-4, GITR, GITRL, HVEM, ICOS, LAG-3, PD-1, PD-L1, PD-L2, TIM-3, TLR-2) performing the Human Immuno-Oncology Checkpoint Protein Panel 1 - Immuno-Oncology Multiplex Assay (Cat. num.: HCKP1-11, Merck) according to the instructions of the manufacturer. Briefly, all samples were thawed and tested in a blind fashion. A 50-μl volume of each sample, 25 μl of standard, 25 μl of matrix solution, and 25 μl of universal assay buffer were added to a 96-well plate (provided with the kit) containing 25 μl of capture antibody-coated, fluorescent-coded beads. A biotinylated detection antibody mixture and streptavidin-PE were added to the plate after the appropriate incubation period. After the last washing step, 150 μl of drive fluid was added to the wells, and the plate was incubated for an additional 5 min and read on the Luminex MAGPIX® instrument. Luminex xPonent 4.2 software was used for data acquisition. Five-PL regression curves were generated to plot the standard curves for all analytes by the Analyst 5.1 (Merck) software calculating with bead median fluorescence intensity values. The panel of the investigated 17 plasma proteins and the range of detection (in pg/mL from the lower limit to the upper limit) are available in Supplementary Table 4. Data were pooled from two independent measurements and plotted in GraphPad Prism v8 (Dotmatics, Boston, USA).
2.11 Clustering of samples based on cytokine levels
The concentration levels of analytes, measured in samples from four patient categories (smoker healthy control, stable COPD, exacerbated COPD, treatment-naive smoker NSCLC) were visualized on a heatmap using the pheatmap R package (35). Hierarchical clustering was carried out based on Euclidian distance and using the hclust method in R.
2.12 Statistical analysis
In CyTOF, median signal intensities, cell frequencies, and subpopulation frequencies were analyzed with GraphPad Prism 8.0.1. The normality of distributions was tested with D’Agostino & Pearson test and passed if all the group’s alpha values were under 0.05. Normally distributed datasets were compared with the ordinary one-way ANOVA or Brown-Forsythe ANOVA when the standard deviations were not equal. For non-parametric analysis, the Kruskal–Wallis test was applied. All types of significance tests were corrected for multiple comparisons by controlling the false discovery rate (FDR) with the two-stage Benjamini, Krieger, and Yekutieli approach with an FDR cutoff of 10%. Differences are considered significant at *p < 0.05, **p < 0.01, and ***p < 0.001 (error bars specify means ± SD). In Luminex, statistical significance was calculated using Student’s t-test. Statistical computations were done by dplyr v1.0.6 and rstatix v0.7.0, while graph generation was done by ggpubr v0.4.0 and ggprism v1.0.3 R packages.
3 Results
3.1 Single-cell immunophenotyping of peripheral mononuclear cells shed light on the disbalance of immune homeostasis in stable COPD, exacerbating COPD, and NSCLC
Using single-cell mass cytometry with the 29-membered antibody panel of the commercially available Human Immune Monitoring kit, we could identify the main immune subsets in the peripheral blood of the studied groups, such as smoker healthy controls (SmHCs), smoker-stable COPD (stCOPD) patients, smoker-exacerbating COPD (exCOPD) patients, and smoker NSCLC patients. During the data analysis, calibration beads were excluded, and singlets, live cells, and CD45+ cells were manually gated. Further unsupervised analysis was performed on CD45+ living singlets where the following populations were excluded by manual gating: CD3+CD14+, CD3+CD19+, CD19+CD14+, and CD19+CD56+ (Supplementary Figure 1) The FlowSOM (Self-Organizing Maps for flow cytometry) and UMAP analysis identified 14 main subsets, namely, CD4+ T cells, CD8+ T cells, CD4+CD8+ T cells, DN (double-negative) T cells (CD4−CD8−), γ/δ T cells, NK cells, CD4+ NKT cells, CD8+ NKT cells, plasmablasts, B cells, monocytes, CD11cdim cells, pDCs, and mDCs (Figure 1; Supplementary Figure 2). The frequency of the main subsets was quantified and expressed as the % of living CD45 singlets (Supplementary Figure 2). The CD4+ T cells were reduced in stCOPD and exCOPD to one-third and half of the SmHCs, respectively (Supplementary Figure 2A). The percentage of CD8+ T cells outperformed the SmHCs, but in the exCOPD, these cells were also half of the SmHCs (Supplementary Figure 2A). The DN T cells were also the lowest in exCOPD. Conversely, the percentage of monocytes was two times higher in exCOPD than in SmHCs; the CD11cdim cells were 10 times higher both in stCOPD and exCOPD compared with the SmHCs and five times higher than in the NSCLC group. Both the pDCs and mDCs were with the lowest frequency in the exCODP group (Supplementary Figure 2D).
The activation of adaptive immunity in smoker COPD patients and the dysfunction/exhaustion of T cells in lung cancer have long been known (36–38). Therefore, our focus was on the analysis of T-cell subsets. Fourteen metaclusters (MCs) were identified in the CD4+ T cells (Supplementary Figure 3). Significant differences were found in MC10 (CD4+/CD185+) with the decrease in exCOPD and NSCLC (Figure 2A); the merged MC13 and MC14 were the CD38bright CD4+ T cells (CD38++ MCs) with the highest frequency in exCOPD (Figure 2B); conversely, the CD183+ MCs (MC5+MC6+MC8+MC10) were the lowest in exCOPD (Figure 2C). Regarding marker expressions on all CD4+ T cells, CD27 decreased in NSCLC vs. SmHC (Figure 2D), and CD127 decreased in stCOPD vs. exCOPD (Figure 2E). Our interest turned toward CD4 memory cells, such as CD4+ CM (central memory) cells and CD4 EM (effector memory). The CD4+ CM cells were defined as CD4+/CD45RA−/CD45RO+/CD197+ merging 03, 10, 11, and 13 MCs. The CD4+ EM cells were defined as CD4+/CD45RA−/CD45RO+/CD197− merging 02, 04, 09, and 14 MCs. The percentage of CD4+ CM and CD4+ EM cells was the lowest in exCOPD (Figures 2F, G).
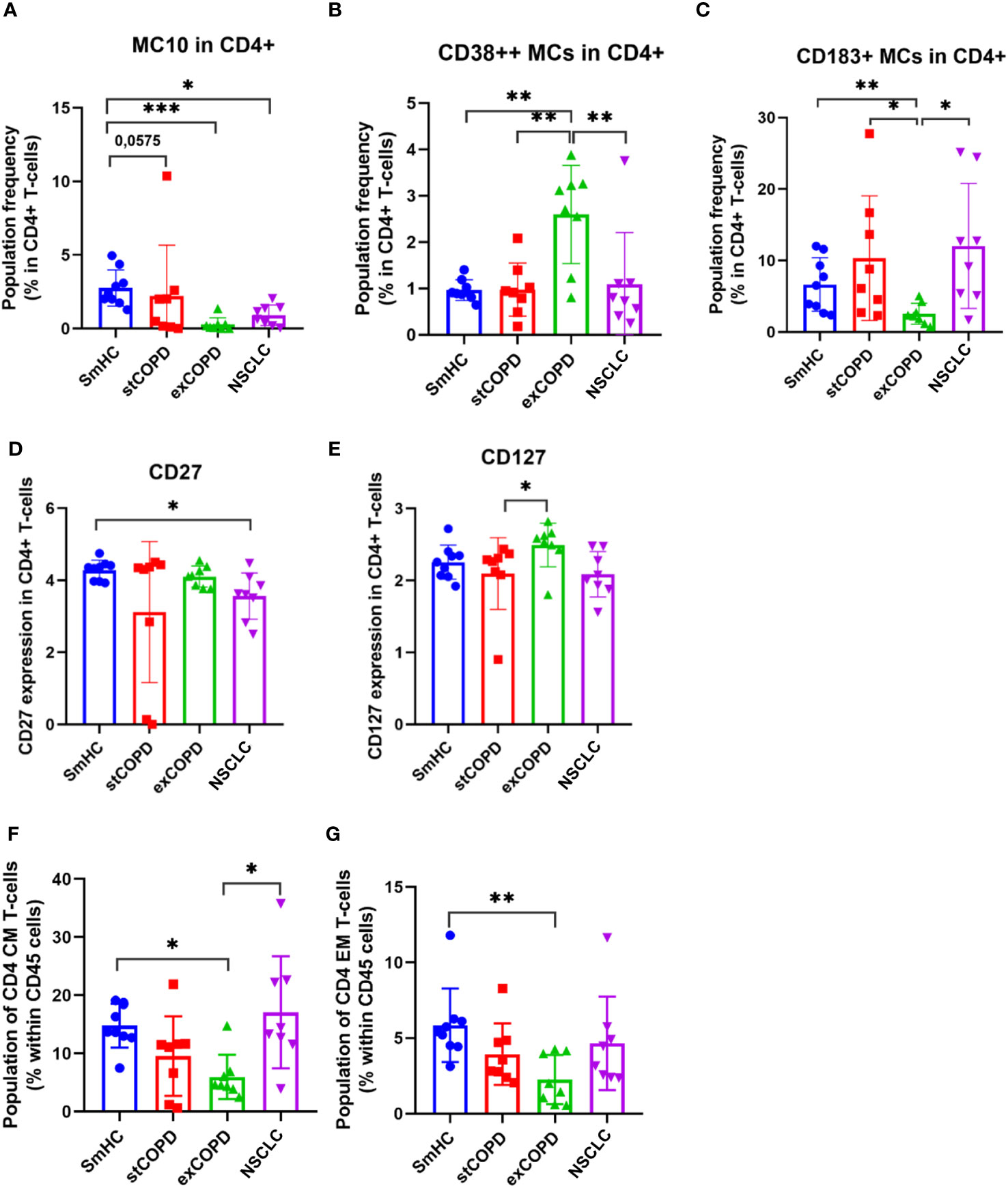
Figure 2 The population frequency (A–C) and marker expression profile of CD4+ T cells (D, E). The differences in the percentage of CD4+ CM (F) and CD4+ EM cells (G). *p < 0.05, **p < 0.01, ***p < 0.001.
Next, the CD8+ T cells were clustered, and 15 MCs were defined (Supplementary Figure 4). Significant differences were found in the following MCs as shown in Figure 3: MC01: CD45RAdim, MC06: CD28+/CD194+, MC09: CD27+/CD45RO+, MC13: CD27+/CD183+/CD194dim, and MC14: CD45RA+/CD197+ (Figure 3). The CD27+ MCs represent cells of the MC04, MC05, MC07, MC08, MC09, MC11, MC13, MC14, and MC15, which were the lowest in stCOPD (Figure 3F). The CD183+ MCs represent cells of the MC04, MC07, MC09, MC11, MC12, MC13, and MC14, which were also the lowest in stCOPD (Figure 3G). The expression of CD27 and CD28 showed a similar pattern with the lowest frequency in the stCOPD (Figures 3H, I). The CD127 was significantly reduced in stCOPD vs. SmHC (Figure 3J), and the CD183 and CD197 were reduced in stCOPD and NSCLC vs. SmHC (Figures 3K, L).
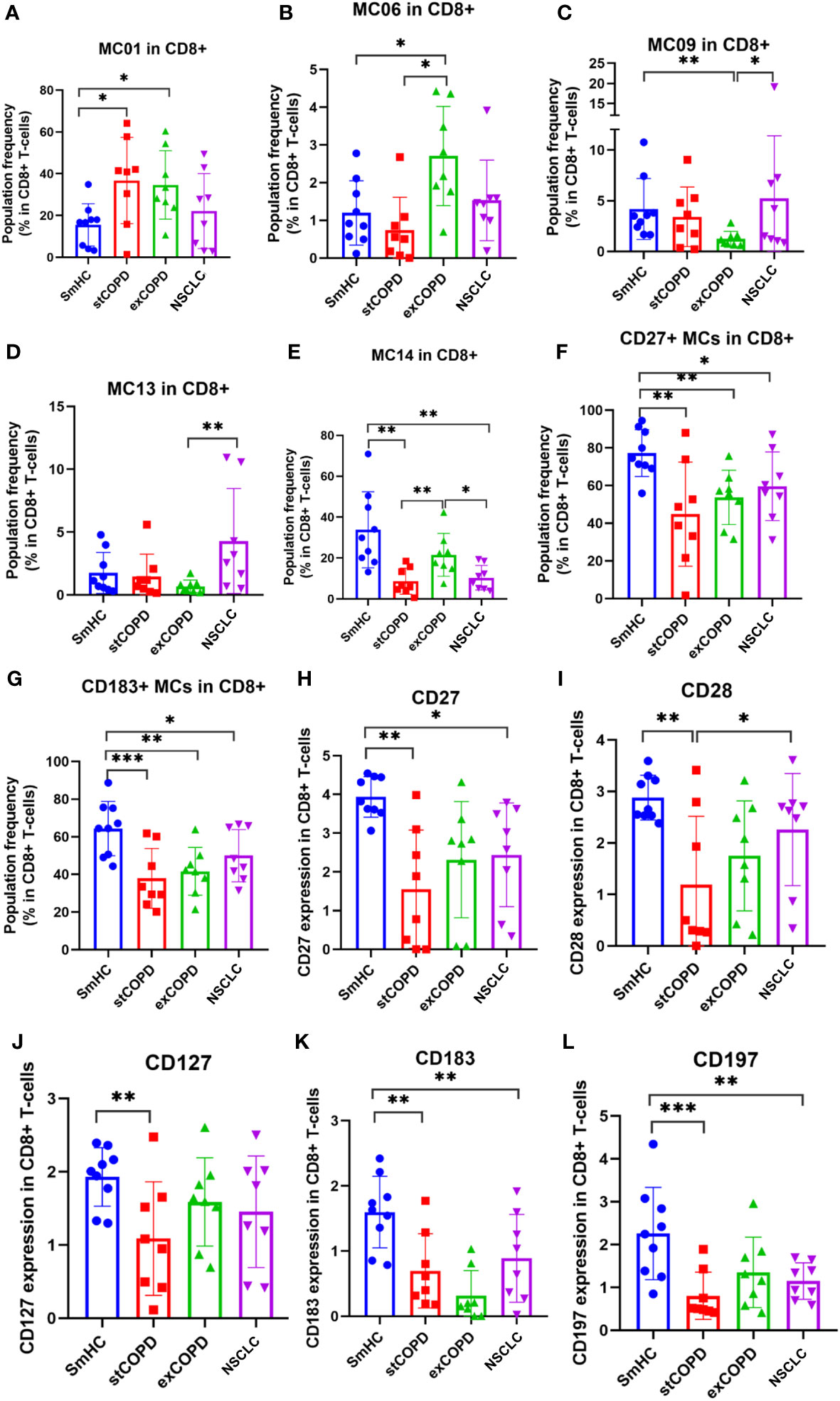
Figure 3 The population frequency (A–G) and marker expression profile of CD8+ T cells (H–L). *p < 0.05, **p < 0.01, ***p < 0.001.
Analyzing the CD4+CD8+ double-positive T cells, CD25 was elevated in exCOPD, CD27 was decreased in exCOPD and NSCLC, and CD8 was the lowest in NSCLC (Figures 4A–C).
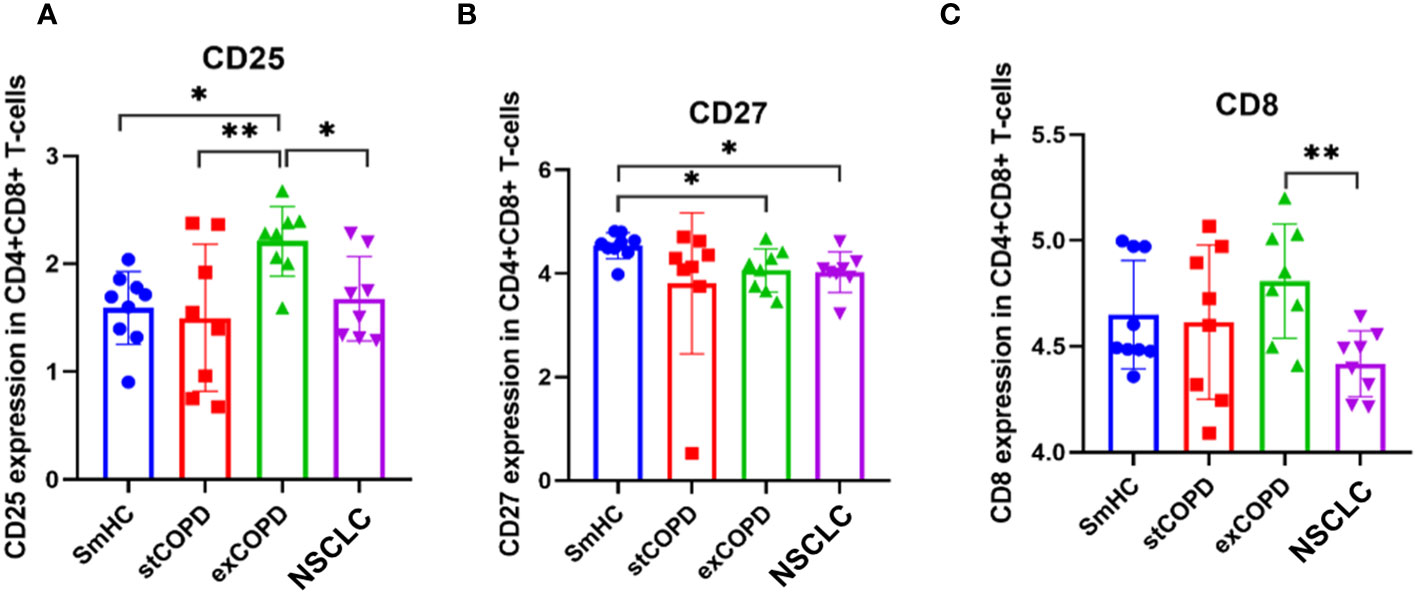
Figure 4 Marker expression (A) CD25, (B) CD27, (C) CD8 profile of CD4+CD8+ T-cells. p *<0.05, **<0.01.
The double-negative CD4−CD8− (DN) T cells delineated five MCs (Supplementary Figure 5). Significant differences in the frequency of the cells were recorded in the following MCs: MC02: CD27+/CD127+/CD161+ that was decreased in exCOPD and NSCLC, MC04: CD38++ that was in excess in exCOPD, and MC05: CD38++/CD183+/CD196+ that was gradually increased from stCOPD to exCOPD and further increased in NSCLC (Figures 5A–C). The surface expression of CD28 decreased in NSCLC vs. exCOPD, and CD183 expression was lower in exCOPD and in the NSCLC groups vs. SmHC (Figures 5D, E).
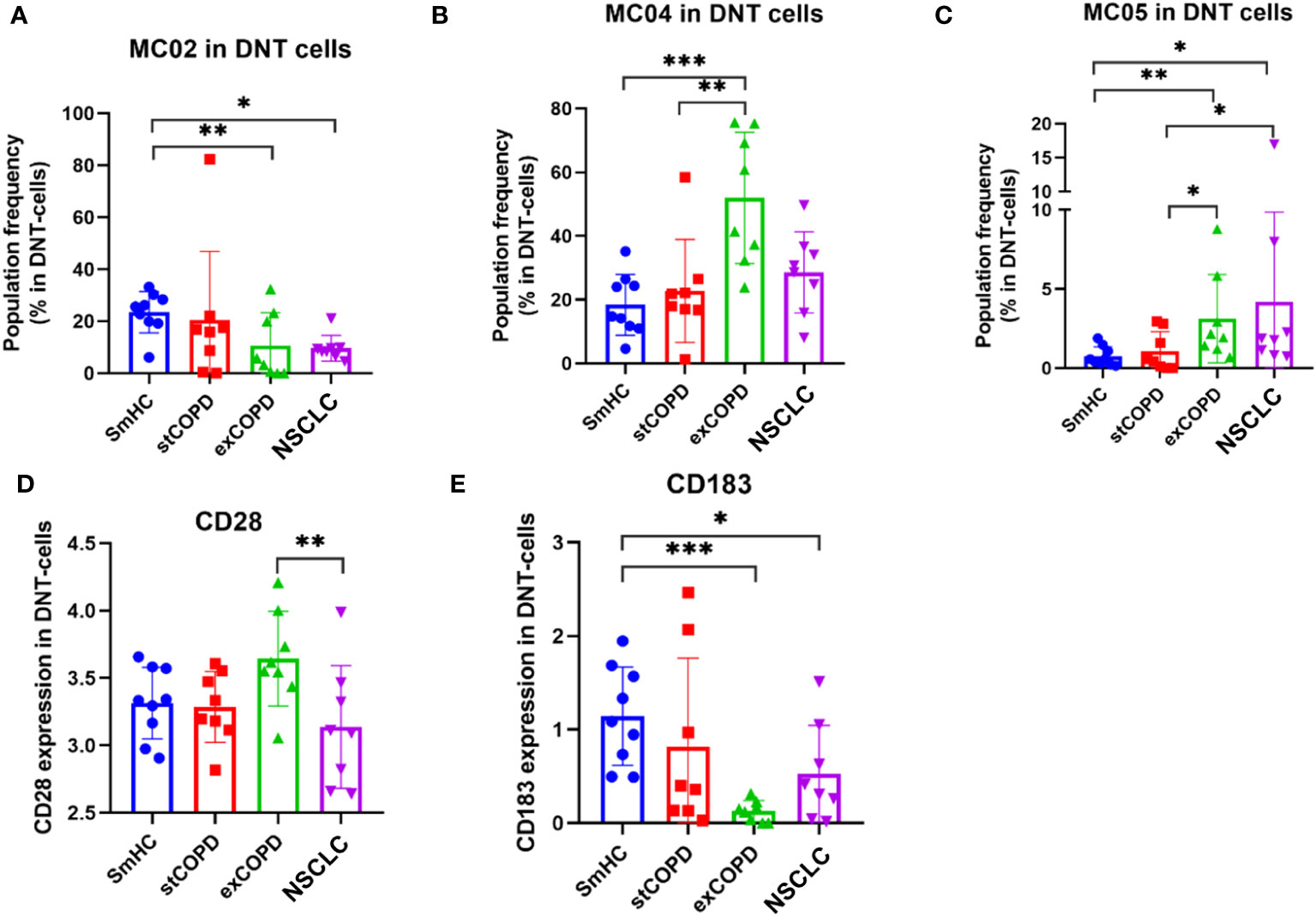
Figure 5 The population frequency of the metaclusters in CD4−CD8− (DN) T cells (A–C) and the marker expression profile of (D) CD28 and (E) CD183 on the surface of DN T cells. *p < 0.05, **p < 0.01, ***p < 0.001.
The γ/δ T cells were divided into 11 MCs (Supplementary Figure 6). The CD27+ γ/δ T cells were determined merging MC03, MC04, MC05, MC06, MC07, and MC09 MCs. The CD27 expression on the surface γ/δ T cells was decreased in exCOPD compared with SmHC and NSCLC (Figure 6A). The CD27+ γ/δ T cells were determined merging MC03, MC04, MC06, MC07, and MC11 MCs. The CD183 expression in the γ/δ T-cell subset decreased in stCOPD and exCOPD compared with SmHC (Figure 6B).
The deep immunophenotyping using CyTOF revealed significant differences in 46 specific markers/populations of other, non-T-cell compartments, but because of the focus of our current study on T cells, the other subtypes are listed in the Supplementary Material, such as CD4+ NKT cells and CD8+ NKT cells (Supplementary Figure 7), classic NK cells (Supplementary Figures 8, 9), B cells (Supplementary Figures 10, 11), plasmablasts (Supplementary Figure 12), monocytes (Supplementary Figures 13, 14), CD11cdim cells, and mDCs (Supplementary Figures 15, 16). However, it is worth emphasizing some of the significant differences which may be relevant in the context of exacerbation of inflammation in the impairment of the antitumor immune response, such as the CD16 (FcγRIII) decrease both in CD4+ NKT and CD8+ NKT cells in NSCLC compared with SmHC (Supplementary Figure 7). We could detect the increase of the G-protein-coupled receptors, such as the CD183 (CXCR3) or CD194 (CCR4) on the cell surface of NK cells in NSCLC compared with the other three groups (Supplementary Figure 9). The MC08 metacluster of B cells positive for CD19+/CD20+/CD45RA+/CD185+/CD27+ was dramatically decreased in stCOPD, exCOPD, and NSCLC compared with SmHC (Supplementary Figures 10, 11). The expression of CD27, CD19, CD185 (CXCR5), and CD196 (CCR6) was reduced on the surface of B cells in the NSCLC group (Supplementary Figure 11). CD19, CD27, and CD45RA were also decreased on the surface of plasmablasts (Supplementary Figure 12). The main significant changes in the myeloid compartment of the PBMCs are also highlighted. The cells of the MC03 (CD14+/CD11chigh+/CD38+/CD45RO+/HLA-DRlow+), the classical monocytes (CD14+/CD16−), and HLA-DRlow cells were significantly higher in exCOPD among monocytes (Supplementary Figures 13, 14). On the contrary, MC06 (CD14+/CD11chigh/CD38+low/CD123dim/CD45RO+/HLA-DRhigh+), MC07 (CD14+/CD11chigh/CD38+/CD45RO+/HLA-DR+), MC08 (CD14+/CD11chigh/CD38+/CD45ROhigh, HLA-DRlow+), and CD16+ cells were the lowest in exCOPD in the monocyte compartment (Supplementary Figures 13, 14). The MC01 (CD196high) of CD11cdim cells was the lowest in exCOPD, but the MC03 (CD196−) cells were of the highest frequency in exCOPD among CD11cdim cells (Supplementary Figures 15, 16).
3.2 The transcriptome of CD4+ EM or CD4+ CM cells showed a correlation in NSCLC with exacerbating COPD samples
The CD183 (CXCR3) showed reduced expression in the aforementioned T-cell subsets, and its influence on the development of memory T cells is known (39). Additionally, alterations in memory T-cells subsets were reported in COPD previously (40–42). Therefore, the CD4+ EM and CD4+ CM cells were FACS-sorted for transcriptomic analysis (RNA sequencing) in our study. The purified RNA was pooled from three to five subjects within one study cohort in an RNA equivalent manner in order to avoid bias from differences in the amount of isolated RNA (Supplementary Table 3). The pooled RNA-Seq identified 203 differentially upregulated and 167 differentially downregulated genes in the CD4+ CM cells of exacerbating COPD and NSCLC samples showing 0.807 Pearson correlation (Supplementary Figure 17). The pooled RNA-Seq identified 234 differentially upregulated and 90 differentially downregulated genes in the CD4+ EM cells of exacerbating COPD and NSCLC samples showing 0.747 Pearson correlation (Supplementary Figure 18). The normalized expression data of the full list of the results of RNA-Seq are found in Supplementary Table 3. The gene set enrichment analyses (GSEA) showed a similar pattern between lung NSCLC and exacerbating COPD-derived peripheral CD4+ CM and CD4+ EM cells (Figure 7). The Hallmark and Kyoto Encyclopedia of Genes and Genomes (KEGG) gene sets were analyzed for the normalized expression values in relation to the healthy control samples. Pathways in angiogenesis were upregulated in CD4+ CM cells both in exCOPD and NSCLC, and pathways in cell division, protein secretion, transcription, response to androgens, or response to ultraviolet radiation were upregulated in CD4+ EM cells both in exCOPD and NSCLC. The 10 most repressed genes were in CD4+ CM cells both in exCOPD and NSCLC samples: MAP3K2, SUZ12, SYNE2, HNRNPF, RNF24, APLP2, HIPK3, FBXL5, SEPT2, and GLCCI1. The 10 most upregulated genes were in CD4+ CM cells both in exCOPD and NSCLC samples: AC008481.1, COMMD1, MRPL41, C12orf57, RPS3AP5, AL009174.1, AC026979.2, AC108161.1, CUTA, and AL450998.1. The 10 most repressed genes were in CD4+ EM cells both in exCOPD and NSCLC samples: FGFBP2, CERKL, RBL2, GNLY, SYNE1, HNRNPLL, PTPN4, GLS, ITGAM, and TGFBR3. The 10 most upregulated genes were in CD4+ EM cells both in exCOPD and NSCLC samples: AC008677.2, AC245033.4, AL450998.1, RPS18P9, RPS3AP5, EEF1A1P5, RPL27AP, RPL41P5, AC008481.1, and S100A9. The gene ID, long name of the genes, and normalized expression values are listed in Supplementary Table 3.
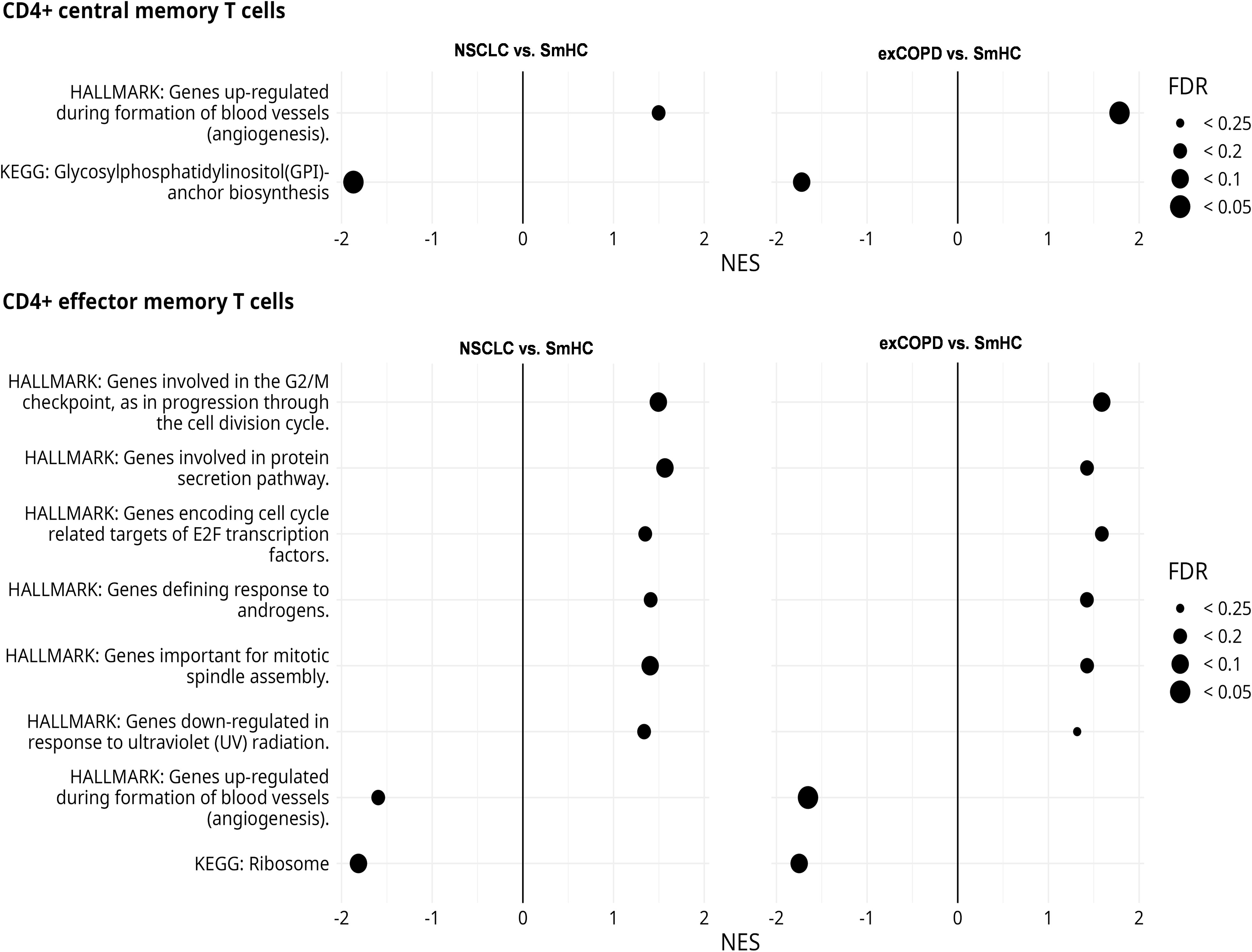
Figure 7 Results of gene set enrichment analyses of CD4+ central memory and effector memory T cells. The figure shows those Hallmark and KEGG gene sets that had similar trends in NSCLC vs. smoker healthy controls (SmHCs) and exacerbated COPD vs. SmHC comparisons. Positive normalized effect score (NES) values indicate enrichment in control. Point sizes indicate false discovery rate (FDR) value ranges.
3.3 The clustering based on the concentration of the immuno-oncology checkpoint soluble mediators in the plasma of the peripheral blood
After the withdrawal of peripheral blood, the PBMCs were purified for CyTOF and RNA-Seq analysis, but the plasma of the peripheral blood was used for the quantitative analysis of immuno-oncology mediators using the multiplex Luminex MAGPIX® technology. The list of the analyzed soluble proteins, the UniProt ID, and assay sensitivity are listed in Supplementary Table 4. The individual concentration values of the 17 measured proteins and multiple comparisons among the four studied groups are visualized on the scatter plots in Supplementary Figure 19. We highlighted only the significant differences in the text. The inhibitory CD366 (TIM-3) was elevated in stCOPD vs. SmHC, and it was lower in exCOPD vs. stCOPD. The inhibitory CD273 (PD-L2) was significantly the lowest in exCOPD compared with the other three groups. The T-cell stimulatory CD86 (B7-2) and GITRL were higher in exCOPD vs. SmHC (Supplementary Figure 19). For better clarity of the results, clustering was applied and is shown in Figure 8. The concentration values after the Z-score transformation resulted in three main clusters (Figure 8A). Cluster 1 with the highest concentration of inflammatory mediators lacks SmHCs and includes three exCOPD patients, two stCOPD patients, and one NSCLC patient. Cluster 2 with the lowest concentration of the measured soluble mediators includes five SmHCs, six exCOPD, four stCOPD, and four NSCLC patients. The intermediate cluster 3 in terms of the medium expression of immuno-oncology mediators represents four SmHCs, five stCOPD, four exCOPD, and eight NSCLC patients.
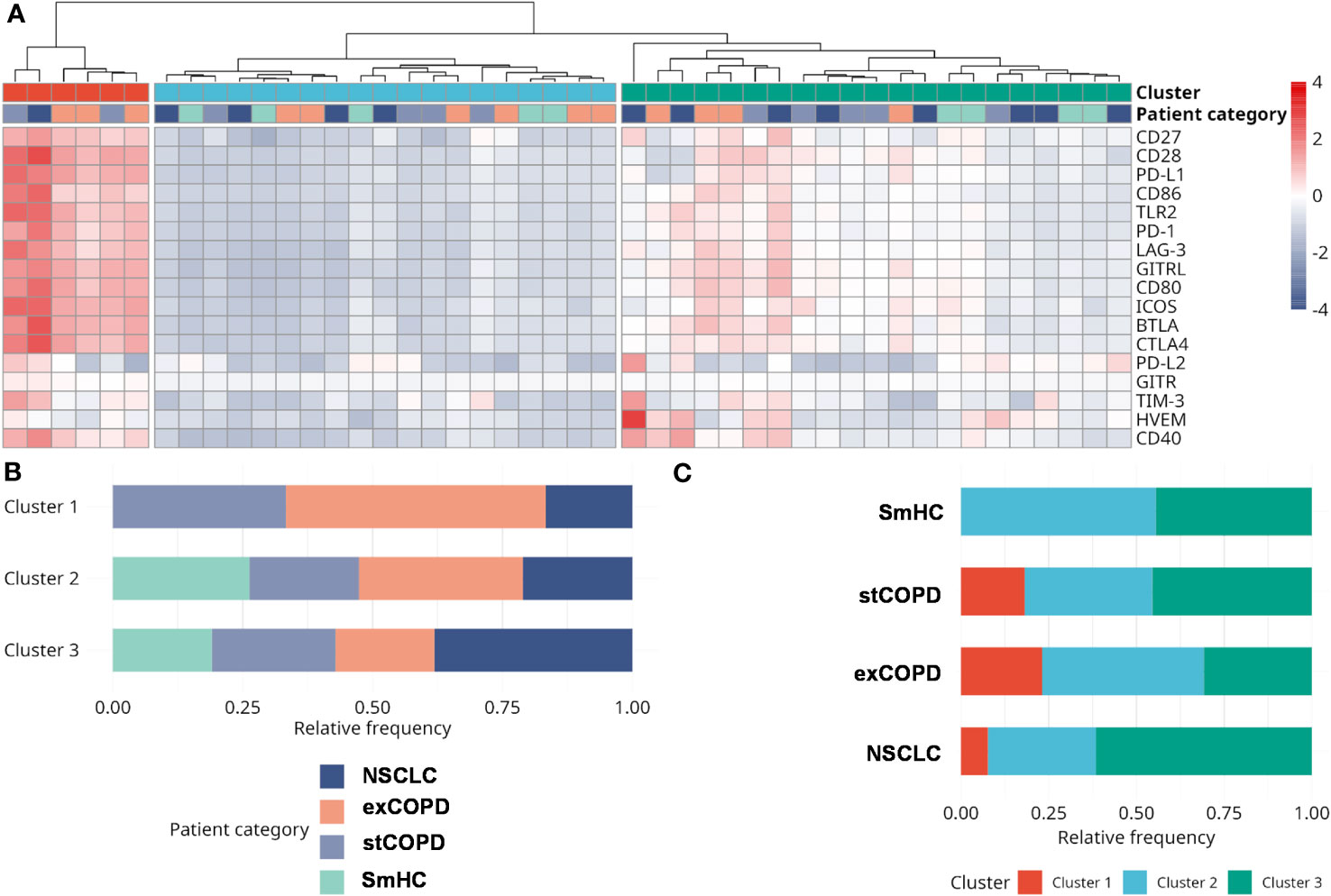
Figure 8 The analysis of the concentration of immuno-oncology mediators of the plasma of the patients. (A) Analyte concentrations in different samples are shown color-coded. Concentration levels have been normalized using the Z-score transformation. Three main sample clusters were identified using hierarchical clustering. (B) The distribution of patient categories in the three clusters. (C) The distribution of clusters among different patient categories. Clusters 1 and 2 can be characterized by the highest and lowest analyte levels, respectively. Cluster 3 shows intermediate analyte levels and contains the largest fraction of NSCLC samples.
4 Discussion
The aim of our study was to have a deeper understanding of the disbalance in the immune homeostasis of smoker-stable COPD and smoker-exacerbating COPD. Since tobacco smoking and an established COPD frequently lead to lung cancer, human smoker NSCLC samples were also investigated. The self-perpetuating systemic inflammation caused by tobacco smoking has been intensively studied earlier by our group and others (5, 43, 44). Cigarette smoking caused alterations in the peripheral immune landscape in COPD that has been reviewed by Taucher et al. (45). Using single-cell mass cytometry, we have recently shown the peripheral immunophenotype of smoker advanced NSCLC patients who underwent chemotherapy or immune checkpoint inhibitor (ICI) therapy (34). However, a limited number of studies are available using CyTOF for the immunophenotyping of the PBMCs of smoker COPD and NSCLC samples. Previously, Vasudevan et al. showed a lower PD-L1 and PD-L2 expression of myeloid cells that supports chronic inflammation in the bronchoalveolar lavage (BAL) of smoker COPD patients using CyTOF (21). Freeman et al. used a 12-color FACS panel for the immunophenotyping of PBMC BAL and sputum samples of COPD cases (46). They published gating strategies to define seven populations (CD4+ and CD8+ T cells, B cells, monocytes, macrophages, neutrophils, and eosinophils) and proposed future analysis to reveal the correlation of immune subset phenotypes with smoking history, spirometry, and other physiologic parameters of the COPD patients. Using an 8-color FACS panel, Xiong et al. showed an increase of Th1, Th17, and Treg phenotypes in the peripheral blood of acute exacerbating COPD patients compared with stCOPD (19). In a murine model of cigarette smoke exposure, Kapellos et al. showed an expansion of CD11b+Ly6G+CD117−CD62L−CD172a− neutrophils in the bone marrow using CyTOF (22). According to our current knowledge, our study is the first to compare the peripheral immunophenotype of smoker healthy controls with stCOPD, exCOPD, and smoker NSCLC. The peripheral blood of the patients was extensively studied by a 29-member antibody panel for single-cell mass cytometry. We could determine and analyze 14 immune subsets, namely, CD4+ T cells, CD8+ T cells, CD4+CD8+ T cells, DN (double-negative) T cells (CD4−CD8−), γ/δ T cells, NK cells, CD4+ NKT cells, CD8+ NKT cells, plasmablasts, B cells, monocytes, CD11cdim cells, pDCs, and mDCs. The extensive immunophenotyping of T cells is shown in the main text, and additionally, 46 further significant differences are shown about NK, NKT, B, or myeloid cells in the Supplementary Material. The ratio of CD4+ T cells and CD8+ T cells was significantly elevated in NSCLC at the expense of the reduction of monocytes and CD11cdim cells. An increased expression of CD38, an activation marker functioning as a cyclic ADP ribose hydrolase, was shown on CD4+ T cells. The increased CD38-mediated signaling in COPD and the generation of adenosine diphosphate ribose and cyclic adenosine diphosphate ribose were described previously by Guedes et al. (47). The CD183 and the chemokine receptor CXCR3 may interact with CXCL9, CXCL10, and CXCL11 affecting T-cell polarization (48), and we found that CD183+ MCs were in the lowest frequency both in CD4+ T cells in exCOPD and in CD8+ T cells in stCOPD. The CD183 expression was also reduced in DN T cells in exCODP and in γ/δ T cells in stCOPD. The CD183 may affect memory T-cell development (39, 49). Indeed CD4+ CM and CD4+ EM cells were in the lowest frequency in exCOPD cases. Roberts et al. published earlier the lower percentage of CD4+ CM and CD4+ EM cells in COPD compared with smoker controls (40). Because of the central role of CD4+ T cells in the polarization of T-cell-mediated responses, the CD4+ CM and CD4+ EM cells were selected for FACS sorting and whole transcriptome analysis by RNA-Seq. This is the first study to show the peripheral immunophenotyping and transcriptome of human patient-derived CD4+ CM and CD4+ EM cells from smoker controls, stCOPD, exCOPD, and NSCLC subjects. The chronic inflammation persistent in exacerbating COPD and NSCLC showed 0.8 or 0.74 Pearson correlation of the co-expression of differentially expressed genes in CD4+ CM or CD4+ EM cells, respectively. Some of the markers such as S100A9 are induced by tobacco smoking and contribute to the development of COPD or decreased survival in NSCLC (50, 51). Neoantigens are generated during tobacco smoking both in COPD and lung cancer (7), and naive CD4+ T cells are exposed to HLA-demonstrated epitopes that may lead to the development of antigen-specific long-lived memory T cells. The CD4+ CM T cells favor trafficking in the primary or secondary lymphoid organs from the periphery, while CD4+ EM T cells migrate to the non-lymphoid sites of inflammation (52). The analysis of the tissue-resident immune composition of COPD and lung cancer has been published previously by other groups (41, 53, 54). Our focus was on the characterization of peripheral immunity, the dissection of single-cell heterogeneity of the subsets of PBMCs, and the quantitative measurement of peripheral immuno-oncology mediators. Sahin et al. published about the serum biomarkers in stCOPD and exCOPD measuring 11 parameters and found that leukocyte and neutrophil cell count, red cell distribution width, C-reactive protein, neutrophil-to-lymphocyte ratio, and platelet-to-lymphocyte ratio were higher in exCOPD than healthy controls (55). Barta et al. recently found in the sputum that IL-6 and growth-regulated oncogene-α were higher in stCOPD than in non-smoker controls, and the IL-1Rα, RANTES, MIG, BMP-4, BMP-6, GDNF, and Acrp30 were higher in exCOPD than stCOPD (56). Our panel for the Luminex MAGPIX® was a commercially available immuno-oncology panel that showed the mixed phenotype of COPD and lung cancer patients. The plasma of exCOPD patients showed an inflammatory phenotype with the lowest concentration of inhibitory PD-L2, a lower amount of TIM-3 vs. stCOPD, and an increased level of stimulatory CD86 and GITRL vs. smoker controls. The distribution of the defined clusters represents the heterogeneity of the human subjects belonging to either SmHC, stCOPD, exCOPD, or NSCLC categories. These disease-associated heterogenic phenotypes may harbor therapeutic resistance and contribute to the challenging scenarios for the treatment options.
Among others, the authors reviewed how chronic inflammation provides the soil for the development of cancer (57). The authors reviewed previously also how COPD may precondition for the development of lung cancer (5). One of the main messages of the current study is the smoking-related systemic inflammation linking exCOPD and NSCLC demonstrated here as a complex single-cell mass cytometric profile. The authors reported here a complex view of the peripheral immunophenotype with a special focus on the T-cell compartment (Figure 9). Freeman et al. published earlier the decrease of CD4+ and CD8+ T cells in the peripheral blood of acute exacerbation COPD patients in agreement with our results (58).
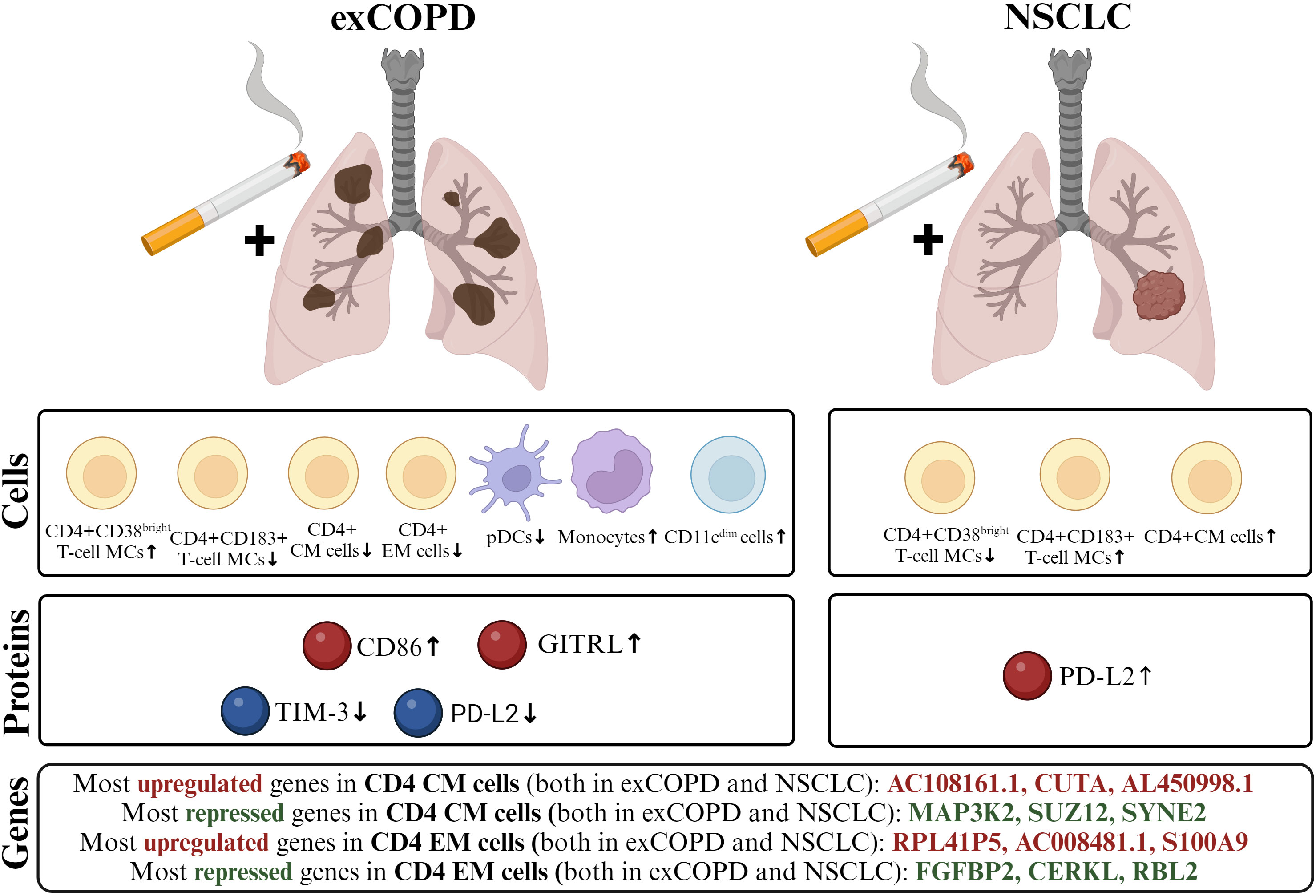
Figure 9 The schematic cartoon of our result focusing on three levels: genes, proteins, and cells. The transcriptome of CD4+ CM and CD4+ EM cells showed a correlation in exCOPD and NSCLC. The three most differentially expressed genes are highlighted. Next, soluble mediators were measured, where CD86 and GITRL were higher in the plasma of exCOPD patients, while TIM-3 and PD-L2 were lower in exCOPD patients. Lastly, single-cell immunophenotyping revealed alterations in the composition of peripheral compartments, where the frequency of the immune subsets was quantified and demonstrated characteristics for exCOPD and NSCLC.
Focusing on T-cell biology, activation markers such as CD38 on CD4 T cells, CD25 on CD4+CD8+ T cells, and CD28 on DN T cells were shown upregulated in exCOPD in conjunction with the drop of the Th1 marker CD183 and chemokine receptor CXCR3 on CD4, CD8, DN, and γ/δ T cells in exCOPD. Similarly, the decrease of CD183 was also shown on cytotoxic CD8+ T cells and DN T cells in NSCLC. The decrease of the co-stimulatory CD27 was also shown on CD4 T cells, CD8 T cells, and CD4+CD8+ T cells in NSCLC. Although the frequency of CD4+ CM and CD4+ EM cells was not the same in exCOPD and NSCLC, their transcriptomic profile showed 0.8 and 0.74 Pearson correlation, respectively. Since CD4+ helper T cells orchestrate the polarization of the adaptive immunity, the high coverage of mRNAs in these memory cells may play a role in the antigen-dependent inflammatory pathways in exCOPD and NSCLC. The authors hypothesize that tobacco smoking-generated inflammation polarizes CD4+ CM and CD4+ EM cells that may contribute to the exacerbation of COPD and impairment of tumor-protective immunity in NSCLC. Taken together, it is hard to estimate which difference in the immunophenotype has the most meaningful clinical importance. Our data may serve as a resource for future studies that will lead to a deeper understanding of the disbalance in immune homeostasis in smoker COPD or NSCLC patients.
Our study has limitations which are as follows: 1) that PBMCs lack a granulocyte compartment which has a significant effect on the chronic inflammation in the studied lung pathologies. 2) Our CyTOF panel consisted of cell surface markers instead of the implementation of subset-specific cytokine/chemokine measurement. 3) We could not investigate tissue-resident immune cells of the affected lung in COPD or NSCLC cases. 4) We could enroll SmHCs with 10 years younger age than the patients. The access to the healthy but aging population is limited with a special focus on the inclusion criteria of the smoking habit. Therefore, we could enroll smoker healthy subjects for the withdrawal of blood from this group. 5) Bacterial or viral infections were not excluded and may influence the immunophenotype of exCOPD or NSCLC. However, according to our knowledge, this is the first study on the deep immunophenotyping of the single-cell heterogeneity in smoker controls, stCOPD, exCOPD, and NSCLC patients with an extension of the transcriptome of CD4+ CM and CD4+ EM cells in the four studied groups. Future studies are warranted to measure the soluble mediators of the identified disease-associated subsets to link serum concentrations with the producing immune subsets.
Data availability statement
The original contributions presented in the study are publicly available. This data can be found here: https://www.ncbi.nlm.nih.gov/geo/; GSE250254.
Ethics statement
The study was conducted in accordance with the Declaration of Helsinki, and the protocol (‘Immunophenotyping in COPD and lung cancer’) was approved by the Ethics Committee of the National Public Health Center under the 33815-7/2018/EÜIG Project identification code. The studies were conducted in accordance with the local legislation and institutional requirements. The participants provided their written informed consent to participate in this study.
Author contributions
NG: Formal analysis, Investigation, Methodology, Visualization, Writing – original draft. JB: Data curation, Methodology, Writing – original draft. PN: Data curation, Investigation, Methodology, Writing – original draft. ES: Investigation, Methodology, Writing – original draft. IB: Methodology, Writing – original draft. JF: Methodology, Writing – original draft. BA: Data curation, Methodology, Visualization, Writing – original draft. ÁZ: Data curation, Formal analysis, Writing – original draft. ZH: Data curation, Formal analysis, Methodology, Writing – original draft. ZC: Data curation, Formal analysis, Writing – original draft. MM: Formal analysis, Methodology, Writing – original draft. GB: Methodology, Writing – original draft. JT: Data curation, Formal analysis, Writing – original draft. LP: Funding acquisition, Resources, Supervision, Writing – original draft. GS: Conceptualization, Data curation, Funding acquisition, Resources, Supervision, Visualization, Writing – original draft.
Funding
The author(s) declare financial support was received for the research, authorship, and/or publication of this article. This research was funded by the 2017-1.3.1-VKE-2017-00028, and 2020‐1.1.6‐JÖVŐ−2021‐00003, FK22-142877 (GS), FK-142312 (MM), and KFI_16-1-2017-0105, and GINOP_PLUSZ-1.1.2-21-2021-00003 grant from the National Research, Development, and Innovation Office. This work was supported by the János Bolyai Research Scholarship of the Hungarian Academy of Sciences BO/00582/22/8 (GS), BO/00193/23/5 (MM) and BO/00594/22/8 (ZC). This work was supported by the by the ÚNKP-23-5 -SZTE-694 (GS), ÚNKP-22-4 -SZTE-291 (GB), ÚNKP-23-4 -SZTE-334 (GB), ÚNKP-23-5 -SZTE-688 (MM), and ÚNKP-23-5 -SZTE-712 (ZC) New National Excellence Program of the Ministry for Innovation and Technology. This manuscript was prepared with the professional support of the doctoral student scholarship program of the Ministry of Innovation and Technology, financed by the National Research, Development, and Innovation Fund for NG (C1764415).
Conflict of interest
Author LP was the CEO of the company Avicor Ltd and Avidin Ltd. Author GS was employed by the company CS-Smartlab Devices Ltd.
The remaining authors declare that the research was conducted in the absence of any commercial or financial relationships that could be construed as a potential conflict of interest
Publisher’s note
All claims expressed in this article are solely those of the authors and do not necessarily represent those of their affiliated organizations, or those of the publisher, the editors and the reviewers. Any product that may be evaluated in this article, or claim that may be made by its manufacturer, is not guaranteed or endorsed by the publisher.
Supplementary material
The Supplementary Material for this article can be found online at: https://www.frontiersin.org/articles/10.3389/fimmu.2023.1297577/full#supplementary-material
References
1. Safiri S, Carson-Chahhoud K, Noori M, Nejadghaderi SA, Sullman MJM, Ahmadian Heris J, et al. Burden of chronic obstructive pulmonary disease and its attributable risk factors in 204 countries and territories, 1990-2019: results from the Global Burden of Disease Study 2019. BMJ (2022) 378:e069679. doi: 10.1136/bmj-2021-069679
2. Lindberg A, Lindberg L, Sawalha S, Nilsson U, Stridsman C, Lundback B, et al. Large underreporting of COPD as cause of death-results from a population-based cohort study. Respir Med (2021) 186:106518. doi: 10.1016/j.rmed.2021.106518
3. Zhao G, Li X, Lei S, Zhao H, Zhang H, Li J. Prevalence of lung cancer in chronic obstructive pulmonary disease: A systematic review and meta-analysis. Front Oncol (2022) 12:947981. doi: 10.3389/fonc.2022.947981
4. Durham AL, Adcock IM. The relationship between COPD and lung cancer. Lung Cancer (2015) 90(2):121–7. doi: 10.1016/j.lungcan.2015.08.017
5. Szalontai K, Gemes N, Furak J, Varga T, Neuperger P, Balog JA, et al. Chronic obstructive pulmonary disease: epidemiology, biomarkers, and paving the way to lung cancer. J Clin Med (2021) 10(13):2889. doi: 10.3390/jcm10132889
6. Qi C, Sun SW, Xiong XZ. From COPD to lung cancer: mechanisms linking, diagnosis, treatment, and prognosis. Int J Chron Obstruct Pulmon Dis (2022) 17:2603–21. doi: 10.2147/COPD.S380732
7. Zeneyedpour L, Dekker LJM, van Sten-van THJJM, Burgers PC, Ten Hacken NHT, Luider TM. Neoantigens in chronic obstructive pulmonary disease and lung cancer: A point of view. Proteomics Clin Appl (2019) 13(2):e1800093. doi: 10.1002/prca.201800093
8. Forder A, Zhuang R, Souza VGP, Brockley LJ, Pewarchuk ME, Telkar N, et al. Mechanisms contributing to the comorbidity of COPD and lung cancer. Int J Mol Sci (2023) 24(3):2859. doi: 10.3390/ijms24032859
9. Peters EN, Warren GW, Sloan JA, Marshall JR. Tobacco assessment in completed lung cancer treatment trials. Cancer (2016) 122(21):3260–2. doi: 10.1002/cncr.30223
10. Colson YL, Shepard JO, Lennes IT. New USPSTF guidelines for lung cancer screening: better but not enough. JAMA Surg (2021) 156(6):513–4. doi: 10.1001/jamasurg.2021.0242
11. Ramaswamy A. Lung cancer screening: review and 2021 update. Curr Pulmonol Rep (2022) 11(1):15–28. doi: 10.1007/s13665-021-00283-1
12. Hirsch FR, Scagliotti GV, Mulshine JL, Kwon R, Curran WJ Jr., Wu YL, et al. Lung cancer: current therapies and new targeted treatments. Lancet (2017) 389(10066):299–311. doi: 10.1016/S0140-6736(16)30958-8
13. Agusti A, Celli BR, Criner GJ, Halpin D, Anzueto A, Barnes P, et al. Global initiative for chronic obstructive lung disease 2023 report: GOLD executive summary. Eur Respir J (2023) 61(4):2300239. doi: 10.1183/13993003.00239-2023
14. Bollmeier SG, Hartmann AP. Management of chronic obstructive pulmonary disease: A review focusing on exacerbations. Am J Health Syst Pharm (2020) 77(4):259–68. doi: 10.1093/ajhp/zxz306
15. Wang Y, Xu J, Meng Y, Adcock IM, Yao X. Role of inflammatory cells in airway remodeling in COPD. Int J Chron Obstruct Pulmon Dis (2018) 13:3341–8. doi: 10.2147/COPD.S176122
16. Contoli M, Baraldo S, Conti V, Gnesini G, Marku B, Casolari P, et al. Airway inflammatory profile is correlated with symptoms in stable COPD: A longitudinal proof-of-concept cohort study. Respirology (2020) 25(1):80–8. doi: 10.1111/resp.13607
17. King PT. Inflammation in chronic obstructive pulmonary disease and its role in cardiovascular disease and lung cancer. Clin Transl Med (2015) 4(1):68. doi: 10.1186/s40169-015-0068-z
18. Angelis N, Porpodis K, Zarogoulidis P, Spyratos D, Kioumis I, Papaiwannou A, et al. Airway inflammation in chronic obstructive pulmonary disease. J Thorac Dis (2014) 6 Suppl 1(Suppl 1):S167–72. doi: 10.3978/j.issn.2072-1439.2014.03.07
19. Xiong XF, Zhu M, Wu HX, Fan LL, Cheng DY. Immunophenotype in acute exacerbation of chronic obstructive pulmonary disease: a cross-sectional study. Respir Res (2022) 23(1):137. doi: 10.1186/s12931-022-02058-x
20. Halper-Stromberg E, Yun JH, Parker MM, Singer RT, Gaggar A, Silverman EK, et al. Systemic markers of adaptive and innate immunity are associated with chronic obstructive pulmonary disease severity and spirometric disease progression. Am J Respir Cell Mol Biol (2018) 58(4):500–9. doi: 10.1165/rcmb.2017-0373OC
21. Vasudevan S, Vasquez JJ, Chen W, Aguilar-Rodriguez B, Niemi EC, Zeng S, et al. Lower PDL1, PDL2, and AXL expression on lung myeloid cells suggests inflammatory bias in smoking and chronic obstructive pulmonary disease. Am J Respir Cell Mol Biol (2020) 63(6):780–93. doi: 10.1165/rcmb.2020-0085OC
22. Kapellos TS, Bassler K, Fujii W, Nalkurthi C, Schaar AC, Bonaguro L, et al. Systemic alterations in neutrophils and their precursors in early-stage chronic obstructive pulmonary disease. Cell Rep (2023) 42(6):112525. doi: 10.1016/j.celrep.2023.112525
23. Rabe KF, Hurd S, Anzueto A, Barnes PJ, Buist SA, Calverley P, et al. Global strategy for the diagnosis, management, and prevention of chronic obstructive pulmonary disease: GOLD executive summary. Am J Respir Crit Care Med (2007) 176(6):532–55. doi: 10.1164/rccm.200703-456SO
24. Balog JA, Hackler L Jr., Kovacs AK, Neuperger P, Alfoldi R, Nagy LI, et al. Single cell mass cytometry revealed the immunomodulatory effect of cisplatin via downregulation of splenic CD44+, IL-17A+ MDSCs and promotion of circulating IFN-gamma+ Myeloid cells in the 4T1 metastatic breast cancer model. Int J Mol Sci (2019) 21(1):170. doi: 10.3390/ijms21010170
25. Alfoldi R, Balog JA, Farago N, Halmai M, Kotogany E, Neuperger P, et al. Single Cell Mass Cytometry of Non-Small Cell Lung Cancer Cells Reveals Complexity of In vivo And Three-Dimensional Models over the Petri-dish. Cells (2019) 8(9):1093. doi: 10.3390/cells8091093
26. Kulmany AE, Frank E, Kovacs D, Kirisits K, Krupitza G, Neuperger P, et al. Antiproliferative and antimetastatic characterization of an exo-heterocyclic androstane derivative against human breast cancer cell lines. BioMed Pharmacother (2021) 140:111728. doi: 10.1016/j.biopha.2021.111728
27. Neuperger P, Balog JA, Tiszlavicz L, Furak J, Gemes N, Kotogany E, et al. Analysis of the single-cell heterogeneity of adenocarcinoma cell lines and the investigation of intratumor heterogeneity reveals the expression of transmembrane protein 45A (TMEM45A) in lung adenocarcinoma cancer patients. Cancers (Basel) (2021) 14(1):144. doi: 10.3390/cancers14010144
28. Balog JA, Honti V, Kurucz E, Kari B, Puskas LG, Ando I, et al. Immunoprofiling of drosophila hemocytes by single-cell mass cytometry. Genomics Proteomics Bioinf (2021) 19(2):243–52. doi: 10.1016/j.gpb.2020.06.022
29. Crowell HL, Chevrier S, Jacobs A, Sivapatham S, Tumor Profiler C, Bodenmiller B, et al. An R-based reproducible and user-friendly preprocessing pipeline for CyTOF data. F1000Res (2020) 9:1263. doi: 10.12688/f1000research.26073.1
30. Fish M, Ellis R, Bishop C, Todd K, Petrov N, Singer M, et al. Utilising mass cytometry with CD45 barcoding and standardised leucocyte phenotyping for immune trajectory assessment in critically ill patients. Br J Anaesth (2021) 126(4):e149–e52. doi: 10.1016/j.bja.2021.01.006
31. Subramanian A, Tamayo P, Mootha VK, Mukherjee S, Ebert BL, Gillette MA, et al. Gene set enrichment analysis: a knowledge-based approach for interpreting genome-wide expression profiles. Proc Natl Acad Sci USA (2005) 102(43):15545–50. doi: 10.1073/pnas.0506580102
32. Balog JA, Kemeny A, Puskas LG, Burcsar S, Balog A, Szebeni GJ. Investigation of newly diagnosed drug-naive patients with systemic autoimmune diseases revealed the cleaved peptide tyrosine tyrosine (PYY 3-36) as a specific plasma biomarker of rheumatoid arthritis. Mediators Inflamm (2021) 2021:5523582. doi: 10.1155/2021/5523582
33. Toth ME, Dukay B, Peter M, Balogh G, Szucs G, Zvara A, et al. Male and female animals respond differently to high-fat diet and regular exercise training in a mouse model of hyperlipidemia. Int J Mol Sci (2021) 22(8):4198. doi: 10.3390/ijms22084198
34. Neuperger P, Szalontai K, Gemes N, Balog JA, Tiszlavicz L, Furak J, et al. Single-cell mass cytometric analysis of peripheral immunity and multiplex plasma marker profiling of non-small cell lung cancer patients receiving PD-1 targeting immune checkpoint inhibitors in comparison with platinum-based chemotherapy. Front Immunol (2023) 14:1243233. doi: 10.3389/fimmu.2023.1243233
35. Aghdam MS, Ebrahimi A, Sheikh-Assadi M. Phytosulfokine alpha (PSKalpha) delays senescence and reinforces SUMO1/SUMO E3 ligase SIZ1 signaling pathway in cut rose flowers (Rosa hybrida cv. Angelina) Sci Rep (2021) 11(1):23227. doi: 10.1038/s41598-021-02712-2
36. Barnes PJ, Cosio MG. Characterization of T lymphocytes in chronic obstructive pulmonary disease. PloS Med (2004) 1(1):e20. doi: 10.1371/journal.pmed.0010020
37. Gadgil A, Duncan SR. Role of T-lymphocytes and pro-inflammatory mediators in the pathogenesis of chronic obstructive pulmonary disease. Int J Chron Obstruct Pulmon Dis (2008) 3(4):531–41. doi: 10.2147/copd.s1759
38. Thommen DS, Schreiner J, Muller P, Herzig P, Roller A, Belousov A, et al. Progression of lung cancer is associated with increased dysfunction of T cells defined by coexpression of multiple inhibitory receptors. Cancer Immunol Res (2015) 3(12):1344–55. doi: 10.1158/2326-6066.CIR-15-0097
39. Rabin RL, Alston MA, Sircus JC, Knollmann-Ritschel B, Moratz C, Ngo D, et al. CXCR3 is induced early on the pathway of CD4+ T cell differentiation and bridges central and peripheral functions. J Immunol (2003) 171(6):2812–24. doi: 10.4049/jimmunol.171.6.2812
40. Roberts MEP, Higgs BW, Brohawn P, Pilataxi F, Guo X, Kuziora M, et al. CD4+ T-cell profiles and peripheral blood ex-vivo responses to T-cell directed stimulation delineate COPD phenotypes. Chronic Obstr Pulm Dis (2015) 2(4):268–80. doi: 10.15326/jcopdf.2.4.2015.0131
41. Yuan R, Yu J, Jiao Z, Li J, Wu F, Yan R, et al. The roles of tissue-resident memory T cells in lung diseases. Front Immunol (2021) 12:710375. doi: 10.3389/fimmu.2021.710375
42. Fernandes JR, Pinto TNC, Arruda LB, da Silva C, de Carvalho CRF, Pinto RMC, et al. Age-associated phenotypic imbalance in TCD4 and TCD8 cell subsets: comparison between healthy aged, smokers, COPD patients and young adults. Immun Ageing (2022) 19(1):9. doi: 10.1186/s12979-022-00267-y
43. Elisia I, Lam V, Cho B, Hay M, Li MY, Yeung M, et al. The effect of smoking on chronic inflammation, immune function and blood cell composition. Sci Rep (2020) 10(1):19480. doi: 10.1038/s41598-020-76556-7
44. Hosseinzadeh A, Thompson PR, Segal BH, Urban CF. Nicotine induces neutrophil extracellular traps. J Leukoc Biol (2016) 100(5):1105–12. doi: 10.1189/jlb.3AB0815-379RR
45. Taucher E, Mykoliuk I, Lindenmann J, Smolle-Juettner FM. Implications of the immune landscape in COPD and lung cancer: smoking versus other causes. Front Immunol (2022) 13:846605. doi: 10.3389/fimmu.2022.846605
46. Freeman CM, Crudgington S, Stolberg VR, Brown JP, Sonstein J, Alexis NE, et al. Design of a multi-center immunophenotyping analysis of peripheral blood, sputum and bronchoalveolar lavage fluid in the Subpopulations and Intermediate Outcome Measures in COPD Study (SPIROMICS). J Transl Med (2015) 13:19. doi: 10.1186/s12967-014-0374-z
47. Guedes AG, Dileepan M, Jude JA, Deshpande DA, Walseth TF, Kannan MS. Role of CD38/cADPR signaling in obstructive pulmonary diseases. Curr Opin Pharmacol (2020) 51:29–33. doi: 10.1016/j.coph.2020.04.007
48. Karin N. CXCR3 ligands in cancer and autoimmunity, chemoattraction of effector T cells, and beyond. Front Immunol (2020) 11:976. doi: 10.3389/fimmu.2020.00976
49. Hu JK, Kagari T, Clingan JM, Matloubian M. Expression of chemokine receptor CXCR3 on T cells affects the balance between effector and memory CD8 T-cell generation. Proc Natl Acad Sci USA (2011) 108(21):E118–27. doi: 10.1073/pnas.1101881108
50. Railwah C, Lora A, Zahid K, Goldenberg H, Campos M, Wyman A, et al. Cigarette smoke induction of S100A9 contributes to chronic obstructive pulmonary disease. Am J Physiol Lung Cell Mol Physiol (2020) 319(6):L1021–L35. doi: 10.1152/ajplung.00207.2020
51. Koh HM, An HJ, Ko GH, Lee JH, Lee JS, Kim DC, et al. Prognostic role of S100A8 and S100A9 protein expressions in non-small cell carcinoma of the lung. J Pathol Transl Med (2019) 53(1):13–22. doi: 10.4132/jptm.2018.11.12
52. Pepper M, Jenkins MK. Origins of CD4(+) effector and central memory T cells. Nat Immunol (2011) 12(6):467–71. doi: 10.1038/ni.2038
53. Cheon IS, Son YM, Sun J. Tissue-resident memory T cells and lung immunopathology. Immunol Rev (2023) 316(1):63–83. doi: 10.1111/imr.13201
54. Lavin Y, Kobayashi S, Leader A, Amir ED, Elefant N, Bigenwald C, et al. Innate immune landscape in early lung adenocarcinoma by paired single-cell analyses. Cell (2017) 169(4):750–65.e17. doi: 10.1016/j.cell.2017.04.014
55. Sahin F, Kosar AF, Aslan AF, Yigitbas B, Uslu B. Serum biomarkers in patients with stable and acute exacerbation of chronic obstructive pulmonary disease: A comparative study. J Med Biochem (2019) 38(4):503–11. doi: 10.2478/jomb-2018-0050
56. Barta I, Paska C, Antus B. Sputum cytokine profiling in COPD: comparison between stable disease and exacerbation. Int J Chron Obstruct Pulmon Dis (2022) 17:1897–908. doi: 10.2147/COPD.S364982
57. Szebeni GJ, Vizler C, Kitajka K, Puskas LG. Inflammation and cancer: extra- and intracellular determinants of tumor-associated macrophages as tumor promoters. Mediators Inflamm (2017) 2017:9294018. doi: 10.1155/2017/9294018
58. Freeman CM, Martinez CH, Todt JC, Martinez FJ, Han MK, Thompson DL, et al. Acute exacerbations of chronic obstructive pulmonary disease are associated with decreased CD4+ & CD8+ T cells and increased growth & differentiation factor-15 (GDF-15) in peripheral blood. Respir Res (2015) 16(1):94. doi: 10.1186/s12931-015-0251-1
Keywords: tobacco smoking, stable COPD, exacerbating COPD, non-small cell lung cancer, single-cell mass cytometry, CD4 central memory T cells, CD4 effector memory T cells
Citation: Gémes N, Balog JÁ, Neuperger P, Schlegl E, Barta I, Fillinger J, Antus B, Zvara Á, Hegedűs Z, Czimmerer Z, Manczinger M, Balogh GM, Tóvári J, Puskás LG and Szebeni GJ (2023) Single-cell immunophenotyping revealed the association of CD4+ central and CD4+ effector memory T cells linking exacerbating chronic obstructive pulmonary disease and NSCLC. Front. Immunol. 14:1297577. doi: 10.3389/fimmu.2023.1297577
Received: 20 September 2023; Accepted: 27 November 2023;
Published: 20 December 2023.
Edited by:
Tiezheng Hou, University College London, United KingdomReviewed by:
Leslie Chavez-Galan, National Institute of Respiratory Diseases-Mexico (INER), MexicoWataru Fujii, Kyoto Prefectural University of Medicine, Japan
Krishna Prahlad Maremanda, Texas A and M University, United States
Copyright © 2023 Gémes, Balog, Neuperger, Schlegl, Barta, Fillinger, Antus, Zvara, Hegedűs, Czimmerer, Manczinger, Balogh, Tóvári, Puskás and Szebeni. This is an open-access article distributed under the terms of the Creative Commons Attribution License (CC BY). The use, distribution or reproduction in other forums is permitted, provided the original author(s) and the copyright owner(s) are credited and that the original publication in this journal is cited, in accordance with accepted academic practice. No use, distribution or reproduction is permitted which does not comply with these terms.
*Correspondence: László G. Puskás, laszlo@avidinbiotech.com; Gábor J. Szebeni, szebeni.gabor@brc.hu
†These authors have contributed equally to this work and share senior authorship