- 1Channing Division of Network Medicine, Brigham and Women’s Hospital, Boston, MA, United States
- 2Division of Pulmonary and Critical Care Medicine, Brigham and Women’s Hospital, Boston, MA, United States
- 3Division of Pulmonary, Critical Care, Sleep and Allergy, Veterans Affairs Boston Healthcare System, West Roxbury, MA, United States
- 4Harvard Medical School, Boston, MA, United States
- 5Division of Pulmonary, Critical Care, and Sleep Medicine. Yale School of Medicine, New Haven, CT, United States
- 6Department of Medicine, Baylor College of Medicine, Houston, TX, United States
- 7Department of Epidemiology, Colorado School of Public Health, Aurora, CO, United States
- 8Division of Pulmonary and Critical Care, University of Michigan, Ann Arbor, MI, United States
- 9Medical Service, Veterans Affairs Ann Arbor Healthcare System, Ann Arbor, MI, United States
- 10Pulmonary Critical Care Sleep and Physiology Division, Department of Medicine, University of California, San Diego, San Diego, CA, United States
- 11Pulmonary and Critical Care Section, Veterans Affairs San Diego Healthcare System, San Diego, CA, United States
- 12Department of Biomolecular Engineering, University of California, Santa Cruz, Santa Cruz, CA, United States
- 13Division of General Medicine and Primary Care, Brigham and Women’s Hospital, Boston, MA, United States
Rationale: Cigarette smoking (CS) impairs B-cell function and antibody production, increasing infection risk. The impact of e-cigarette use ('vaping') and combined CS and vaping ('dual-use') on B-cell activity is unclear.
Objective: To examine B-cell receptor sequencing (BCR-seq) profiles associated with CS, vaping, and dual-use.
Methods: BCR-seq was performed on blood RNA samples from 234 participants in the COPDGene study. We assessed multivariable associations of B-cell function measures (immunoglobulin heavy chain (IGH) subclass expression and usage, class-switching, V allele usage, and clonal expansion) with CS, vaping, and dual-use. We adjusted for multiple comparisons using the Benjamini-Hochberg method, identifying significant associations at 5% FDR and suggestive associations at 10% FDR.
Results: Among 234 non-Hispanic white (NHW) and African American (AA) participants, CS and dual-use were significantly positively associated with increased secretory IgA production, with dual-use showing the strongest associations. Dual-use was positively associated with class switching and B-cell clonal expansion, indicating increased B-cell activation, with similar trends in those only smoking or only vaping. The IGHV5-51*01 allele was increased in dual users.
Conclusions: CS and vaping additively enhance B-cell activation, most notably in dual-users. CS and vaping are significantly associated to multiple alterations in B-cell function including increased class switching, clonal expansion, and a shift towards IgA-producing cell populations. These changes could be relevant to response to infection and vaccinations and merit further study.
Introduction
Use of electronic cigarettes, i.e. vaping, has increased substantially since their introduction to the U.S. market in 2007 (1). Numerous studies have demonstrated that vaping induces inflammatory responses and has adverse health effects (2–6). More precise characterization of the inflammatory effects of vaping may better define its effects on health, both for vaping alone and vaping in conjunction with combustible cigarettes, i.e. dual-use.
B-cells participate in adaptive immunity largely by producing antibodies that protect mucosal surfaces and provide antigen-specific responses to infection. Combustible tobacco cigarette smoking (CS) has adverse effects on B-cells resulting in increased susceptibility to infections (7). Proper B-cell function depends on B-cell activation, a process in which naïve B-cells are activated by exposure to an antigen. This process triggers clonal expansion of B-cell populations with specifically rearranged B-cell receptor (BCR) genes, which encode the specific immunoglobulin (Ig) produced by each clone. In conjunction with T-cell help, these activated clones undergo somatic hypermutation, a prerequisite for affinity maturation, the process by which immunoglobulins are optimized to bind specific antigens. BCR sequencing (BCR-seq) allows for the identification and sequence-specific characterization of B-cells and expanded B-cell clones, providing rich characterization of the B-cell response (8).
We hypothesized that vaping and dual-use alter B-cell function and the ability of B-cells to respond appropriately to antigens through activation and antibody production. To address this question, we performed BCR-seq in 234 participants from the Genetic Epidemiology of COPD (COPDGene) (9) Study, a large study of individuals who currently or previously smoked that is enriched for participants with COPD.
Methods
Study population
Written informed consent was obtained from all study participants, and institutional review board approval was obtained at all study centers. The Genetic Epidemiology of COPD (COPDGene) (9) study enrolled 10,198 non-Hispanic white (NHW) and African American (AA) individuals who smoked 10 or more pack-years of cigarettes during their lifetime and who were aged 45–80 at study enrollment. COPDGene is an ongoing longitudinal study with completed enrollment, 5-year, and 10-year visits. At each visit, anthropometric measurements, spirometry, chest computed tomography (CT) imaging, and blood samples were collected. At the 5-year follow up visit, we collected questionnaire data on use of cigarette and e-cigarette products. All data in this paper comes from the 5-year study visit where both blood RNA-seq and vaping data are available.
Participant selection
Selection of participants for BCR-seq (8) was performed using a stratified random sampling approach as follows. First, all participants with available blood RNA and complete vaping and CS data from COPDGene Phase 2 at the time of participant selection were considered (n=3,601). They were stratified into five groups based on vaping and CS status – never smokers, former combustible cigarette smokers, current cigarette smokers (without current vaping), current vapers (without current cigarette use), and current dual-users (vaping and cigarette use). All participants in the current vaper (n=41) and dual-user group (n=57) with available samples were selected for BCR-seq, and the remaining 136 participants were randomly sampled from the set of available participants in the other CS groups. Participants taking oral corticosteroids were excluded from the analysis as these medications are known to modify B-cell function.
Definition of key study variables
CS and vaping behavior were ascertained by self-report. Vapers were participants who reported using at least one e-cigarette within the prior week and had a history of smoking tobacco cigarettes, but not within the last 30 days. Current cigarette users reported current smoking with an average of at least one cigarette per day without any e-cigarette use. Dual-users were vapers who also reported current CS, and former cigarette users were defined as those who reported a history of smoking but did not meet criteria for current CS or vaping. In most of the reported analyses, former cigarette users are used as the reference group.
Information on the age, sex, and race of participants was elicited through self-report. For sex, participants were asked if they were male or female. For race, participants were asked if they were NHW, Black or African American, Asian, Pacific Islander, American Indian or Alaska Native, or Other with the option to select multiple categories. By design, inclusion in COPDGene was limited to participants self-identifying as NHW or AA. A separate ethnicity question asked participants if they were Hispanic or Latino. For this study, participants were coded as NHW if they indicated “White” and AA if they indicated “Black or African American.”
In the U.S., COPD primarily develops in the setting of cigarette smoking exposure, and B-cell lymphoid follicles are associated with COPD severity (10–12). Therefore, we examined the association of BCR-seq measures with COPD and COPD-related traits. COPD status was determined by GOLD spirometry grades based on post-bronchodilator spirometry testing where participants were grouped into normal spirometry (FEV1/FVC > 0.7 and FEV1 % predicted > 80%) or GOLD spirometry grade 1, GOLD grade 2-4, or preserved ratio with impaired spirometry (PRISm) (13). For all analyses, the reference group included formerly smoking individuals with normal spirometry. Global Lung Initiative (GLI) race-neutral equations were used to calculate % predicted spirometry values. Computed tomography (CT) imaging measures of emphysema and airway wall thickness were generated by Thirona (https://thirona.eu/) and the following measures were analyzed: % low attenuation area less than -950 Hounsfield units for emphysema and airway wall thickness as % of overall airway volume [wall area percent (14)].
B-cell receptor sequencing library preparation
Details regarding generation of RNAseq data in COPDGene were previously published (15). Whole blood was collected and stored in PAXgene Blood RNA tubes, and total RNA was extracted using Qiagen PreAnalytiX PAXgene Blood miRNA Kit (Qiagen, Valencia, CA). Sequencing libraries were prepared using 200 ng of total RNA as input following a protocol modified from (8). Additional details regarding library preparation and data processing can be found in the Supplementary Methods.
We generated adaptive immune receptor repertoire sequencing data for B-cell receptors (hereafter, ‘BCR-seq’) data using a set of isotype-specific immunoglobulin heavy chain (IGH) constant region primers. Reads were aligned to International Immunogenetics Information System (IMGT) reference germline sequences, and clonal relationships between BCR sequences were inferred using the spectralClones function from the scoper R package contained within the Immcantation suite of software packages (https://immcantation.readthedocs.io/en/stable/about.html). Mutated sequences were defined as sequences that were aligned but differed from the IMGT reference by one or more bases.
Uniquely identified BCR sequences were collapsed by clone and then quantified to represent antibody isotype expression (log 2 counts of the number of unique BCR sequences present within each isotype class) and usage (number of unique BCR sequences present within each isotype class divided by the total number of BCR sequences), B-cell activation measured through class switching (number of unique BCR sequences in the IgA, IgG, and IgE isotypes divided by the total number of BCR sequences), length of the CDR3 region in nucleotides, and the clonal diversity of the B-cell population in each individual as measured by Hill numbers (16). V allele usage was defined as the number of unique and mutated BCR sequences containing a specific V allele (as defined by IGHV genes from the IMGT reference) divided by total number of unique BCR sequences. For each BCR-seq measure, we analyzed only those measures where the isotype or V allele class in question was present at >1% of the total unique sequences for 25% of the participants or more, applying a multiple comparison testing FDR threshold of 0.05, resulting in 10 V segments for analysis. The one exception was measurement of the IgE isotype which was analyzed despite being below this threshold due to its established clinical importance.
B-cell receptor sequencing measures
BCR sequencing involves sequencing transcripts of the B-cell receptor using a set of primers targeting the Fc-region of the immunoglobin heavy chain (IGH). This provides comprehensive assessment of the BCR repertoire including antibody isotype (IgM, IgD, IgA, IgG, and IgE), V alleles corresponding to the variable region that determines antibody specificity, and clonal expansion and somatic hypermutation of specific B-cell populations. These sequence counts are first collapsed by clone (such that sequences arising from a single B-cell clone are counted as a single occurrence) and summarized into quantitative measures of 1) isotype usage (proportion of antibody transcripts for each isotype within each individual), 2) isotype expression (log2 transformed counts that represent the number of unique B-cells per isotype within each individual), 3) class switching (proportion of class-switched B-cells per individual), 4) V allele usage (proportion of antibody transcripts for each V allele within each individual), 5) CDR3 length by isotype, and 6) B-cell clonal diversity measured by Hill numbers.
Statistical analysis
We performed analyses in R version >4.0 (www.r-project.org). We assessed normality of continuous variables by visual inspection of histograms. Results are shown as mean ± standard deviation or median [interquartile range], as appropriate. Differences in continuous variables were assessed with Student t-tests or Wilcoxon tests. Categorical variables were compared by ANOVA or Kruskal-Wallis tests, as appropriate. We considered false discovery rate (FDR)-adjusted Benjamini-Hochberg (17) p-values less below 0.05 to be significant and between 0.05 and 0.1 to be suggestive.
For each of the BCR-seq measures, we used univariable analysis and multivariable regression to examine the association between each of these measures with the primary outcomes of CS, vaping, and dual-use. Further, we examined associations to secondary outcomes, including age, sex, and race as well as COPD affection status (GOLD 2-4), CT emphysema measures [ % low attenuation area (LAA) < -950 Hounsfeld units (HU) (14)], and CT airway wall thickness [wall area % (14)]. Age was calculated from the study date and the date of birth. Sex was determined by the concordance of self-report and XX or XY status. Race was based on self-report. Principal components of genetic similarity were calculated using Eigensoft 3.0, as previously published (18).
For analyses of smoking/vaping, demographic variables, and GOLD spirometry grade, multivariable models included the following covariates: age, sex, self-identified race, vaping/smoking behavior, GOLD grade, pack-years of smoking, and inhaled corticosteroid use. For CT imaging measures, models were additionally adjusted for CT scanner model. To visualize the results, we constructed violin plots and heatmaps.
Sensitivity analyses were performed for the significant associations observed with smoking/vaping status and self-reported race adjusting for self-reported income level and social deprivation index, a measure of area-level deprivation (19), and principal components of genetic similarity. We additionally performed interaction analyses between self-reported race and smoking/vaping variables by including the main effects and cross-product interaction terms in a regression model.
Results
Characteristics of study participants
A schematic of our study design is shown in Figure 1. We included 234 COPDGene NHW and AA participants with smoking/vaping and BCR-seq data, and a table of their characteristics is shown in Table 1. Compared to other groups, dual-users were more likely to be younger, NHW, have more pack-years of cigarette smoking (CS), lower FEV1 % predicted, and thicker airway walls. Compared to dual-users, individuals who only vaped were slightly older, were less likely to be male, had similar pack-years of smoking, but had higher FEV1 % predicted, and more quantitative emphysema (% LAA < -950 HU). To evaluate for bias with respect to which individuals had available RNA-seq data in the overall COPDGene cohort, we compared characteristics and found similar rates of smoking and vaping, though fewer African Americans in the group had available RNA-seq data (Table 2).
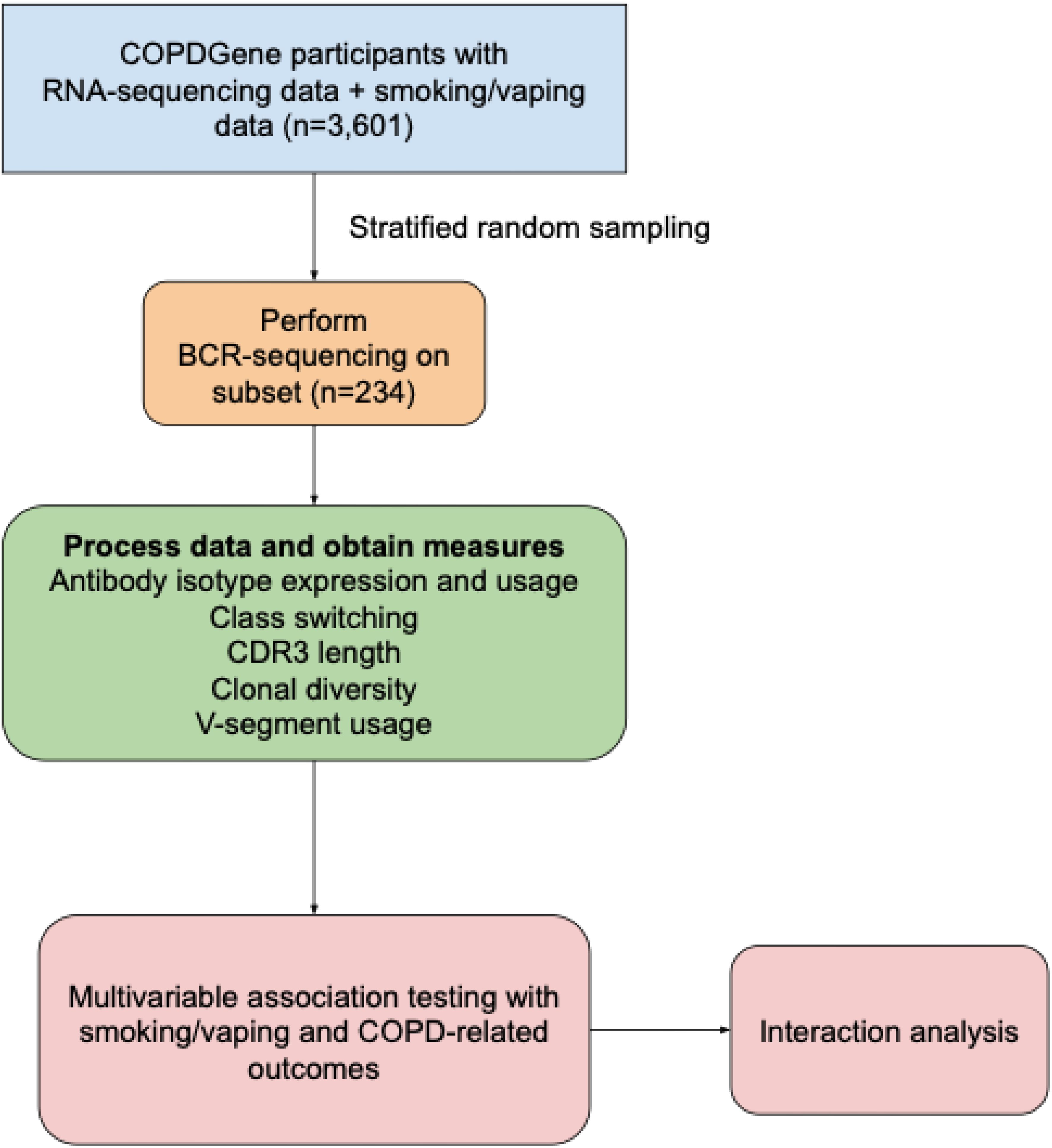
Figure 1. Schematic of study design. COPDGene, Genetic Epidemiology of COPD study; BCR, B-cell receptor; COPD, chronic obstructive pulmonary disease.
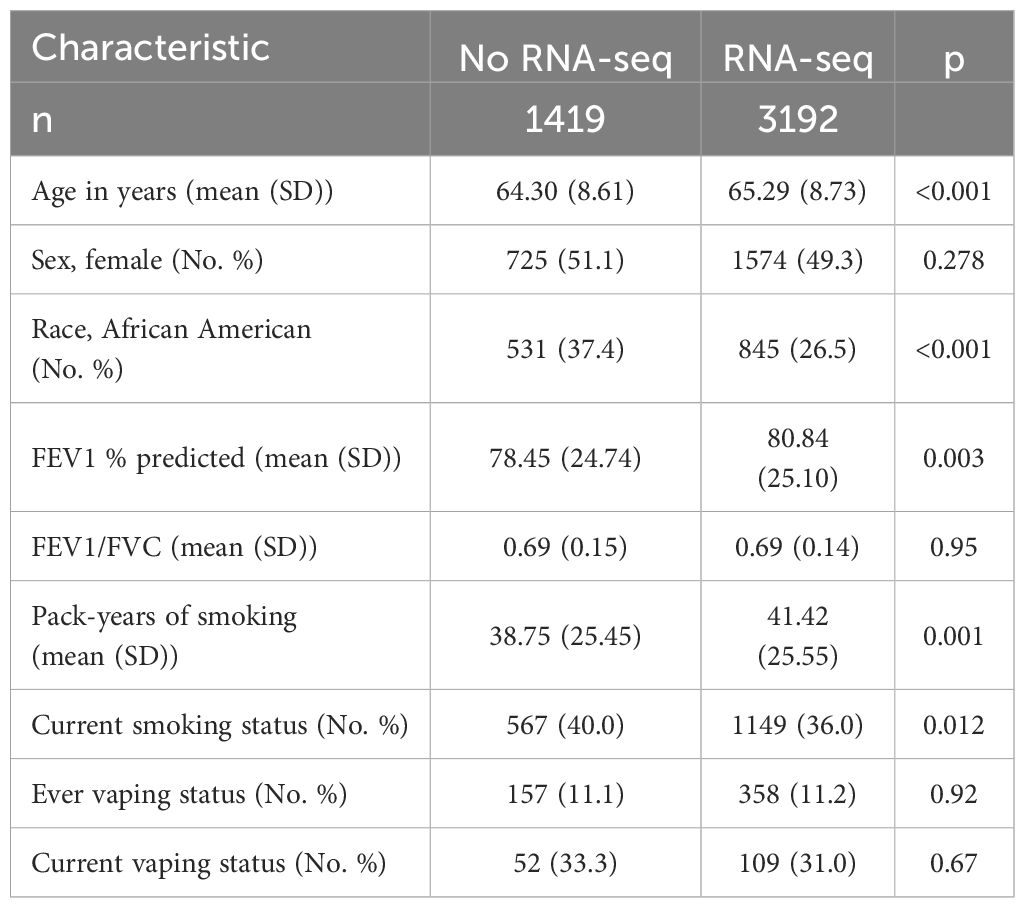
Table 2. Characteristics of COPDGene Visit 2 participants with vaping data comparing those with and without RNA-seq data.
Associations between BCR-seq measures and cigarette smoking, vaping, and dual-use
Significant (q-value < 0.05) and suggestive (q-value <0.1) associations for vaping, CS, and dual-use are shown in– Table 3, while complete model results are shown in Tables 4–8. Overall, we observed that the most pronounced changes in antibody production were associated with dual-use. Specifically, dual-use resulted in a shift in isotype usage towards IgA, IgG1 and IgG2, and away from IgM (Figure 2A). It was also associated with increased class switching suggestive of B-cell activation (Figure 2B) and increased usage of specific V alleles, most notably IGHV5-51*01, IGHV3-1*01, and IGHV1-18*01. Hill biodiversity analysis also demonstrated reduced antibody diversity for participants engaged in dual-use (Figure 3), suggesting that there is a greater amount of B-cell clonal expansion in this group. Since CS status is often associated with socioeconomic variables, we tested these associations after adjusting for income level and social deprivation index, a composite measure of area-level deprivation, which had minimal effect on the significance of these associations (Table 9).
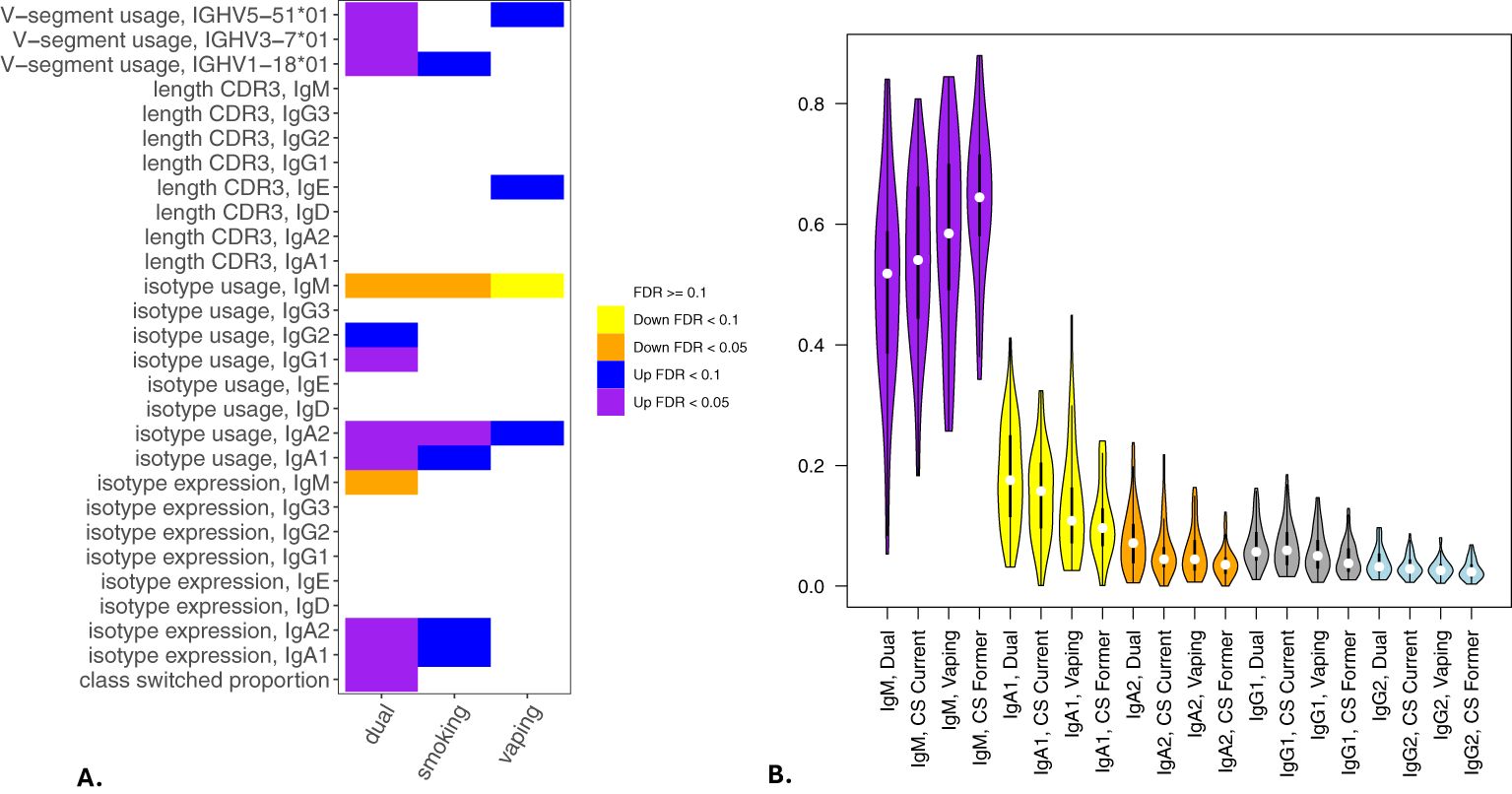
Figure 2. Associations of BCR measures with vaping and cigarette smoking. Significant associations between BCR measures and vaping, cigarette smoking, and dual-use from multivariable models analyzing class switching, isotype expression and usage, V allele usage, and CDR3 length (in nucleotides) are shown in (A). (B) shows IgM, IgA and IgG isotype usage among participants engaged in current smoking, vaping, or dual-use with former smokers included for comparison. Significance is assessed by t-tests. *p<=0.05, **p<=0.005, ns p>0.05.
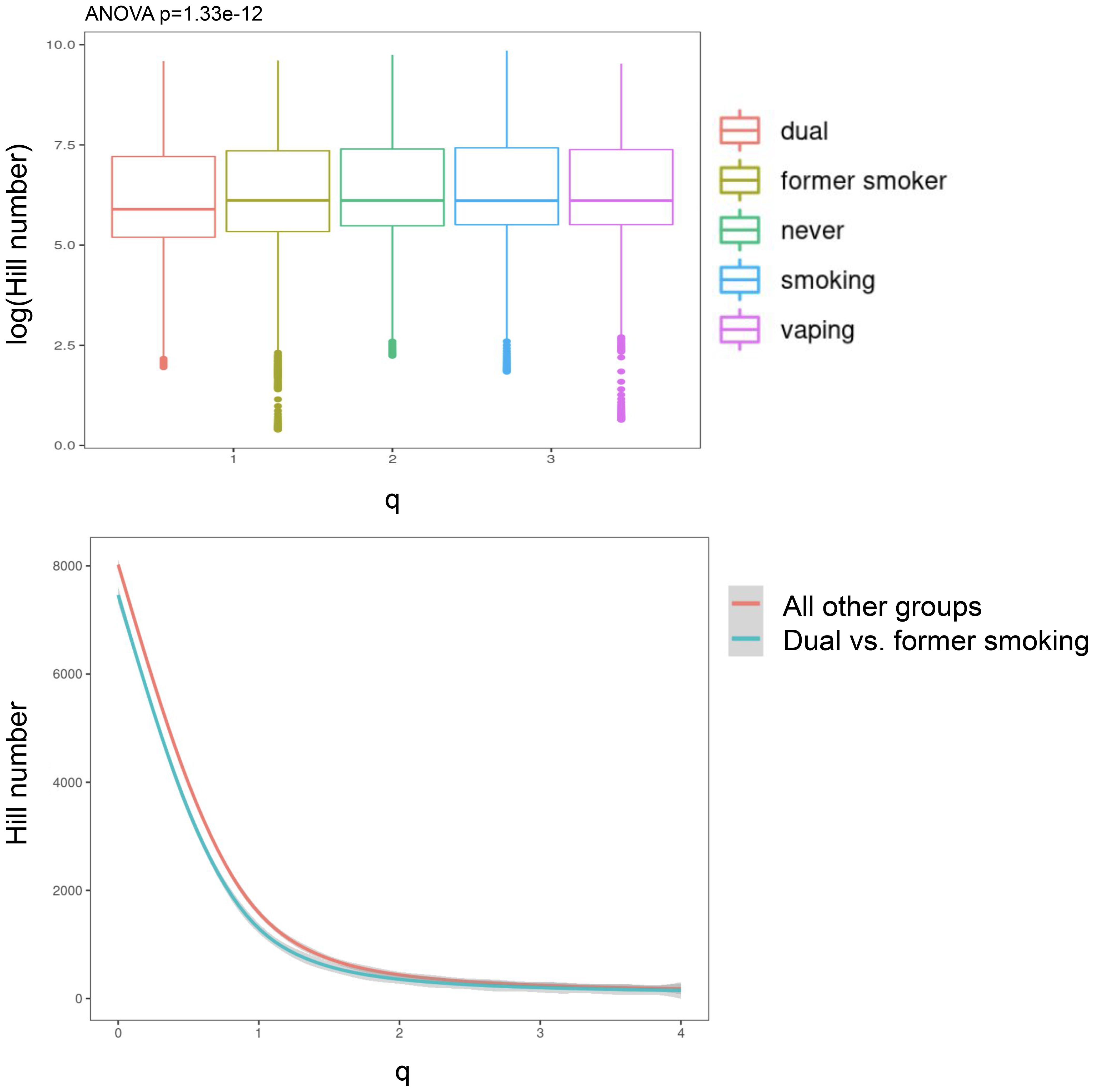
Figure 3. Hill biodiversity numbers show less antibody diversity (and more clonal expansion) in dual users relative to other smoking/vaping groups. The top panel is a boxplot showing that the dual users (i.e., vaping + smoking) have lower log-Hill values than other groups. In the bottom panel, we collapsed all other groups and compared the hill numbers in a continuous fashion over a range of q-values.
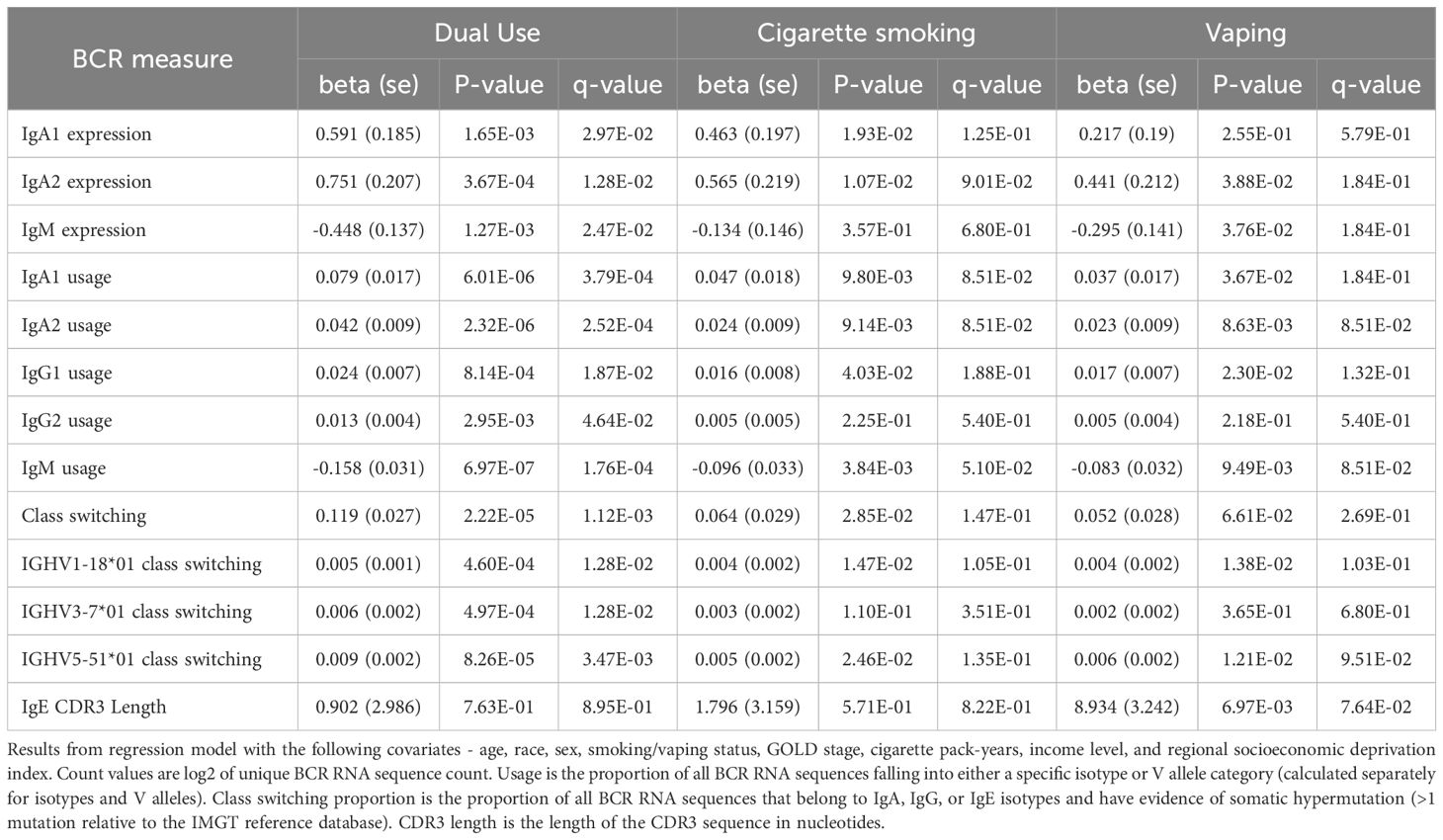
Table 9. Significant BCR associations to vaping, smoking, and dual use adjusting for socioeconomic status.
Current CS also was significantly associated with increased secretory IgA usage and decreased IgM usage, with several other suggestive associations. Vaping showed the same trend towards increased IgA usage and decreased IgM, though these associations were suggestive but not significant. There was also a suggestive association to decreased CDR3 length in IgE antibody transcripts (Figure 4). Overall, vaping and CS showed a similar trend in effect sizes compared to dual-use, suggesting that the effects were similar but less pronounced in current smokers and vapers relative to dual-users.
To examine the appropriateness of using former rather than never smokers as the reference group, we performed multivariable linear regressions comparing isotype usage in former versus never smoking individuals (Table 10), which demonstrated no significant differences between these groups after adjusting for multiple comparisons, suggesting that most of the smoking effects on B-cell function assessed in this study may resolve after cessation.
Associations between sex and self-reported race on antibody production
In multivariable models, we observed significant associations for 8 BCR-seq measures with self-reported race (Table 11). Comparing NHW versus AA participants, NHW-identifying participants had decreased usage of IgG1 and IgG3 isotypes and increased usage of IgM (Figure 5). Univariate associations are shown in Figure 6. To investigate the extent to which these associations may be driven by variables related to income or socioeconomic status, we repeated the analysis adjusting for self-reported income level and area deprivation index, and 4 of the 8 significant associations remained significant, and all 8 associations had a consistent effect direction (Table 12). After adjusting for principal components of genetic similarity, the isotype usage associations with race were attenuated, though notably, the principal component variables were also not associated with isotype usage. We observed no interaction between self-identified race and CS, vaping, or dual-use on isotype usage (all p > 0.05). In the sex analysis, we observed one significant association in which male compared to female sex was significantly associated with decreased CDR3 length in IgM isotype sequences (p < 0.05).
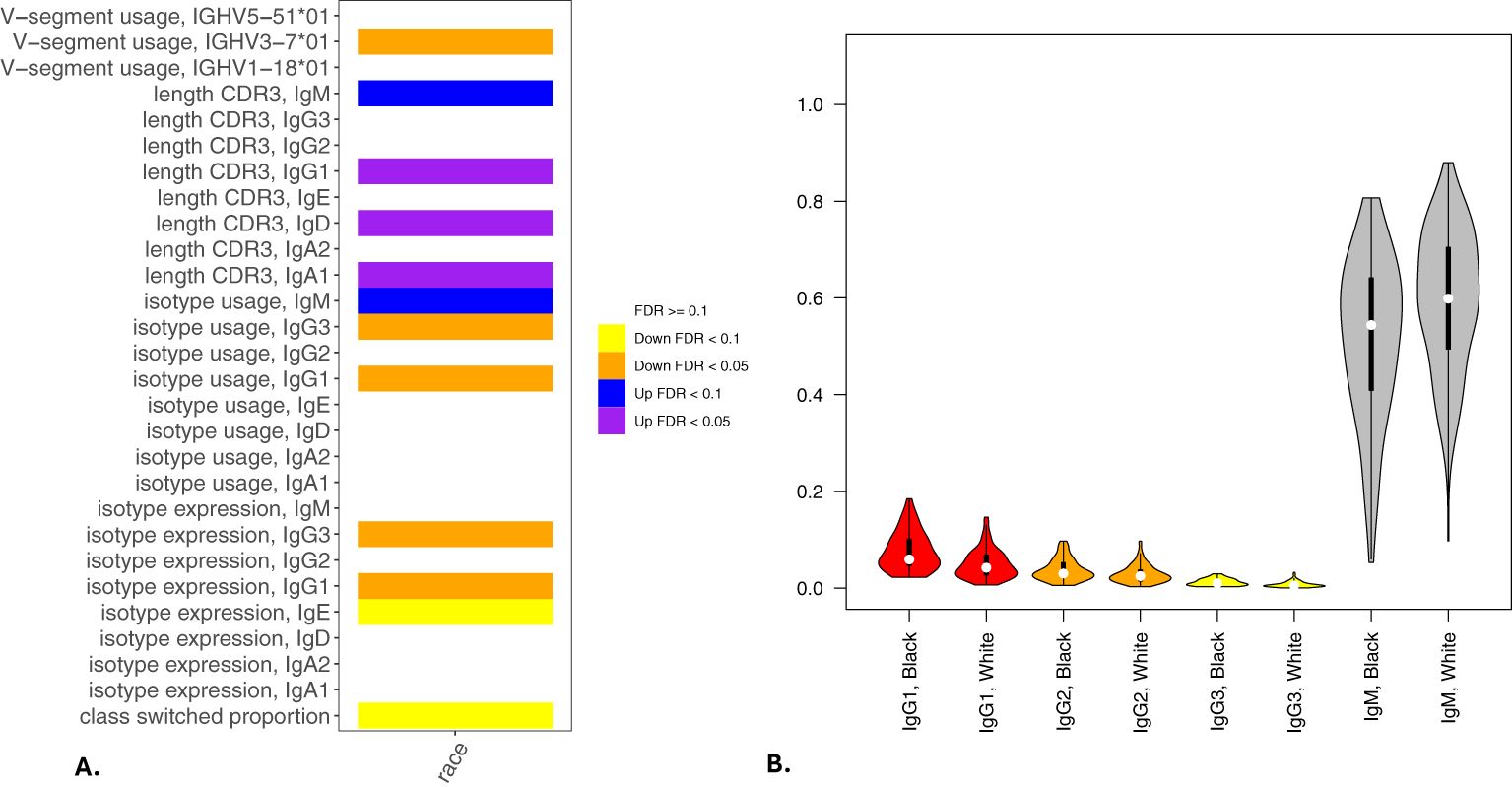
Figure 5. Associations of BCR measures with self-reported race. Significant associations between BCR measures and self-reported AA or NHW race from multivariable models analyzing class switching, isotype expression and usage, V allele usage, and CDR3 length (in nucleotides) are shown in (A). (B) shows IgG and IgM isotype usage by self-reported race. Significance is assessed by t-tests. *p<=0.05, **p<=0.005, ns p>0.05.
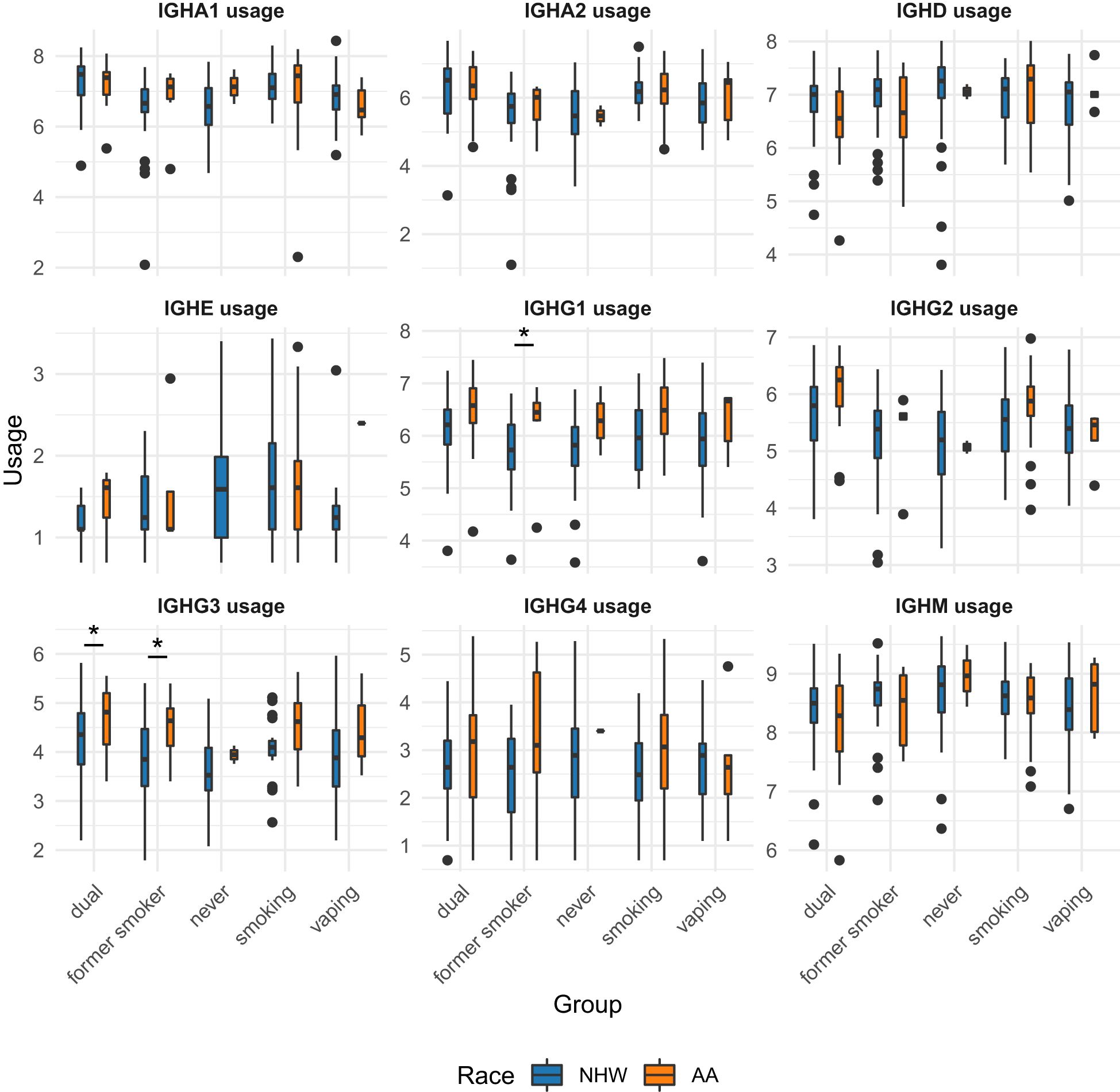
Figure 6. Univariate associations of immunoglobulin subtype usage across smoking/vaping group, separated by self-identified race. NHW, non-Hispanic white; AA, African American. Student t-tests were performed and p-values < 0.05 are indicate by with an asterisk (*).
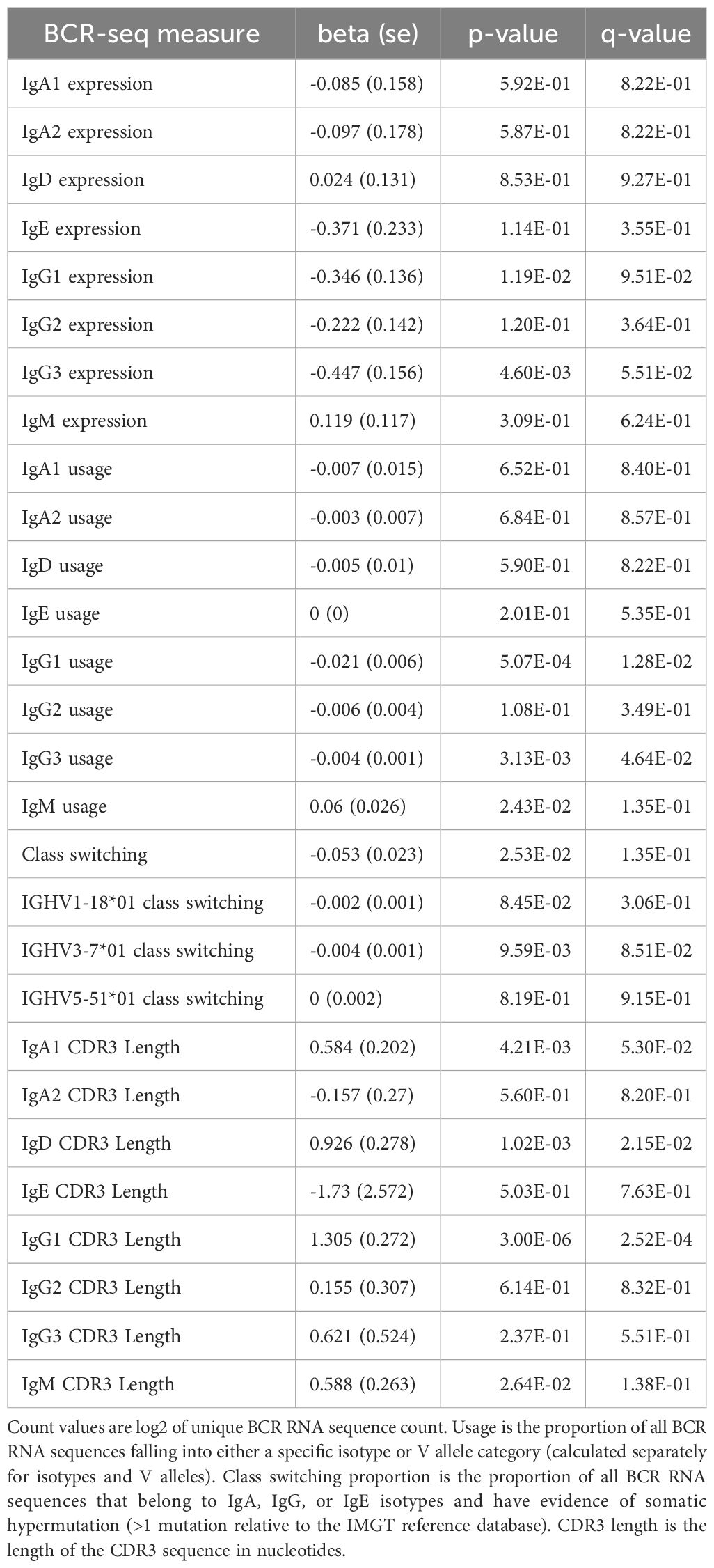
Table 12. BCR-seq associations to self-reported race adjusting for income level and national area deprivation index.
Associations to COPD and related phenotypes
We also examined associations between BCR-seq measures and age, COPD affection status, and CT-related measures of emphysema and airway wall thickness. We observed a significant univariate association between COPD and increased usage of the IGHV5-51*01 V allele and suggestive associations with increased class switching and a shift from IgM to IgA (Table 13). Similar but non-significant trends were associated with the CT-quantified airway wall thickness (Table 14). However, these associations were not significant in models adjusting for CS/vaping behavior, age, sex, and race. No significant associations were observed with CT emphysema measures.
Discussion
In this study of 234 individuals with B-cell receptor sequencing (BCR-seq) and cigarette smoking (CS), vaping, and COPD-related outcome data, we observed significant effects of CS and vaping leading to increased IgA expression and usage, increased class switching, and lower antibody diversity indicating greater clonal activation of specific B-cell populations. Taken together, these results demonstrate the potential dangers of dual-use compared to single product use, an under-recognized but important public health concern.
Our study demonstrates that dual use and CS are associated increased B-cell activation and increased production of secretory IgA (i.e. IgA2), consistent with mucosal exposure to compounds from vaping devices and combustible cigarettes. We note that vaping also showed a similar but nonsignificant trend with a consistent effect direction. It is possible that in a larger study these associations would reach statistical significance. IgA is secreted by the airway mucosa and is important in lung immune defense against pathogens (20). Our data suggest that CS and vaping trigger similar host immune responses with a greater effect observed in participants engaged in dual-use. Indeed, many patients who use e-cigarettes for smoking cessation will smoke tobacco cigarettes and vape, and our results underscore the importance of understanding the health effects of dual-use specifically, as well as vaping and CS alone.
Our findings are consistent with previous research demonstrating that CS increases IgA production in blood and lung (21, 22). Higher levels of class-switched memory B-cells have been observed in individuals who smoke compared to former and never smokers, irrespective of COPD status (23). Vaping is associated with increased circulating club cell protein and decreased transcutaneous oxygen tension (3), increased IL-10 and TNF-α (24), and methylation changes that may cause long-term alterations in cytokine levels (25, 26). Vaping has also been associated with increased expression of 191 inflammatory proteins from bronchoalveolar lavage fluid, including MUC5AC, which is important in mucin production (27). Clinically, vaping is associated with acute lung injury (2, 28), decreased FEV1/FVC and peak expiratory flow in asthmatics (24), and chronic bronchitis (27). However, the implications of dual-use on adaptive immunity are an important contribution of our study.
There are several mechanistic questions raised by our finding that dual-use leads to greater B-cell activation and class switching. The toxicology of cigarette smoking is highly complex and heterogeneous, involving thousands of compounds with variable delivery and dosage influenced by numerous factors such as solvents, filters, tobacco content, burn time, and individual inhalation patterns. The toxicology of vaping is less understood but shares many of the same intricate considerations. Nonetheless, a wealth of literature on the effects of smoking and vaping allow us to hypothesize on how smoking and vaping might be altering B-cell function. CS contains benzo-a-pyrene, a potent activator of aryl-hydrocarbon receptor. AHR is expressed in B-cell developmental subsets upon activation and is associated with a suppressed humoral immune response (29). Whether this mechanism can explain our findings for CS and/or vaping remains to be seen but is worth further investigation. Nitrosamines are metabolic products of nicotine and present in the saliva of both individuals who smoke cigarettes or vape. Nitrosamines can cause DNA adducts and induce formation of reactive oxygen species and NFkB-driven inflammation (30), the latter of which is an important regulator of B-cell differentiation and activation (31). Both smoking and vaping can lead to the release of damage-associated molecular patterns (DAMPs) and pathogen-associated molecular patterns (PAMPs) that can activate antigen-presenting cells that stimulate B-cell activation. Several other potential explanations and mechanistic pathways can be explored through further studies based on our findings.
The role of adaptive immunity and B-cells in COPD and emphysema pathogenesis is well-recognized (10, 20, 32, 33). Although we observed several univariable associations of blood B-cell transcriptomics to COPD and related phenotypes, none of these associations remained significant in multivariable models after adjustment for multiple comparisons. While seemingly in contrast to the well-known increase in lung lymphoid follicles and B-cell infiltration in severe COPD (10–12, 32, 33), this lack of significant associations is not surprising, as by definition the presence of germinal centers in lung lymphoid follicles indicates local B-cell division and maturation. Indeed, antigen exposure within the lung leads to local recruitment of memory (and perhaps naïve) B-cells, local expansion of B-cells and plasma cells within the airway tissue, and subsequent production of antigen-specific antibodies that tend to stay in the lung before getting into the bloodstream (10, 34). Since our analysis is limited to the transcriptome of circulating B-cells, we cannot address whether there is protein-level spillover of antibody produced by lung-resident B-cells into the blood, though other COPD-specific inflammatory changes in blood such as neutrophilia and the increase in several RNA and protein biomarkers are well-documented (35–37).
An intriguing aspect of BCR-seq is the ability to characterize the B-cell response at the level of specific V alleles. IGHV5-51*01 usage was increased in dual users, and as this V allele is increased in response to parainfluenza infections, it might suggest that these individuals are more susceptible to infections or are experiencing immunological reactions similar to those invoked by this viral infection. There was a univariate association of IGHV5-51*01 with COPD status, which might suggest that this V segment could link immune responses of smoking and vaping with COPD pathogenesis. IGHV1-18*01 has been associated with chronic lymphocytic leukemia (38). There is no known immunologic link between dual use and chronic lymphocytic leukemia, though such a connection would be of public health importance. The relevance of IGHV3-1*01 is less clear though this association is interesting and can be investigated in future studies.
We selected former smokers as the reference group because the vast majority of participants in our cohort were either current or former smokers, and vaping in this population typically occurs in the context of prior tobacco use. However, we acknowledge that the persistence of memory B-cell responses from prior smoking may confound the interpretation of our findings. This limitation highlights the importance of future studies that include a larger never-smoking comparator group to better isolate the effects of vaping and dual use.
Our sequencing strategy, which relied on forward primers targeting V genes in the variable region, carries a risk of primer binding bias due to mutations in the primer binding sites, particularly in groups with higher levels of B-cell activation. While this bias is somewhat mitigated because all participant groups were subjected to the same methodology, the unequal levels of B-cell activation between groups may have introduced skewed results, and future studies using template-switching approaches could help address this limitation.
To our knowledge, this is the largest study to date of BCR-seq in humans or model systems. The strengths of this study include the novel use of BCR-seq in a large, deeply phenotyped cohort of participants engaged in current CS, vaping, or both. One limitation is that we did not have a suitable replication cohort, but our current findings highlight the need to obtain BCR-seq data longitudinally and in additional cohorts. We were not able to compare B-cell activation in lung versus blood, which is important for understanding the role of adaptive immunity in CS, vaping, and COPD pathogenesis. Single-cell and spatial transcriptomic or proteomic data would provide greater resolution of the adaptive immune responses to CS and vaping. T cell receptor sequencing that coincides with BCR-seq would provide a more comprehensive view of adaptive immune responses in this context as well. Ideally, we would have measured immunoglobulin protein levels concomitantly with BCR sequencing. However, BCR-seq has been used to analyze B-cell activation independently of circulating protein levels, as demonstrated in a study of meningococcal vaccine responses, where sequencing revealed distinct plasma cell signatures and vaccine-induced repertoire shifts that would not be captured by traditional immunogenicity measures (39). A larger sample size would be desirable to examine COPD-related outcomes, particularly longitudinal outcomes such as FEV1 decline, mortality, and exacerbations. Self-identified race was a secondary outcome, and this cohort recruited only non-Hispanic white and African American individuals, which is not ideal for this type of analysis. Ideally, a more diverse sample representative of additional racial and ethnic groups would be included, and this limitation suggests caution in interpreting the race associations reported in this study.
In conclusion, we observed that CS and vaping each enhance B-cell activation, and that dual-users show a trend towards greater effects than either alone. Self-identified race was strongly associated with IgG isotype usage. These findings highlight associations between B-cell activation and antibody transcription, suggesting potential differences in immune and vaccine responses linked to CS, vaping, and self-identified race.
Data availability statement
The datasets presented in this study can be found in online repositories. The names of the repository/repositories and accession number(s) can be found below: https://www.ncbi.nlm.nih.gov/gap/, phs000951.v6.p5.
Ethics statement
The studies involving humans were approved by Mass General Brigham Institutional Review Board. The studies were conducted in accordance with the local legislation and institutional requirements. The participants provided their written informed consent to participate in this study.
Author contributions
MM: Conceptualization, Data curation, Formal Analysis, Investigation, Methodology, Project administration, Software, Validation, Visualization, Writing – original draft, Writing – review & editing. ZX: Data curation, Formal Analysis, Writing – original draft, Writing – review & editing. AB: Writing – original draft, Writing – review & editing. MR: Writing – original draft, Writing – review & editing. MC: Writing – original draft, Writing – review & editing. CH: Writing – original draft, Writing – review & editing. ES: Writing – original draft, Writing – review & editing. MS: Writing – original draft, Writing – review & editing. FP: Writing – original draft, Writing – review & editing. GK: Writing – original draft, Writing – review & editing. JC: Writing – original draft, Writing – review & editing. LC-A: Writing – original draft, Writing – review & editing. CV: Conceptualization, Data curation, Funding acquisition, Resources, Writing – original draft, Writing – review & editing. PC: Conceptualization, Data curation, Formal Analysis, Funding acquisition, Investigation, Methodology, Project administration, Resources, Software, Supervision, Validation, Visualization, Writing – original draft, Writing – review & editing.
Funding
The author(s) declare that financial support was received for the research and/or publication of this article. MM is supported by MM is supported by K08HL159318 and grant funding from Genentech. MR was supported by the Canadian Institutes of Health Research (FRN:194027). PC is supported by NIHR01HL124233, R01HL147326, R01HL166992, and R01171213HL. MS is supported by R01HL155948. LC-A is supported by R01HL137052 and R01HL147326. The COPDGene project was supported by NHLBI grants U01 HL089897 and U01 HL089856 and by NIH contract 75N92023D00011. COPDGene is alsosupported by the COPD Foundation through contributions made to an Industry Advisory Board that has included AstraZeneca, Bayer Pharmaceuticals, Boehringer Ingelheim, Genentech, GlaxoSmithKline, Novartis, Pfizer, and Sunovion.
Conflict of interest
MM received consulting fees from TheaHealth, 2ndMD, TriNetX, Axon Advisors, Sano". CH reports grant support from Boehringer-Ingelheim, Novartis, Bayer and Vertex, outside of this study. PC has received grant support from Bayer and Sano" consulting fees from Verona Pharma. ES has received institutional grant support from Bayer and Northpond Laboratories. MS has received funding/support from AstraZeneca, Regeneron, and Sano", and has a financial interest in Crosswalk Health.
The remaining authors declare that the research was conducted in the absence of any commercial or financial relationships that could be construed as a potential conflict of interest.
The authors declare that this study received funding from the COPD Foundationt hrough contributions made to an Industry Advisory Board that has included AstraZeneca, Bayer Pharmaceuticals, Boehringer Ingelheim, Genentech, GlaxoSmithKline, Novartis, Pfizer, and Sunovion. The funder was not involved in the study design, collection, analysis, interpretation of data, the writing of this article or the decision to submit it for publication.
Generative AI statement
The author(s) declare that no Generative AI was used in the creation of this manuscript.
Publisher’s note
All claims expressed in this article are solely those of the authors and do not necessarily represent those of their affiliated organizations, or those of the publisher, the editors and the reviewers. Any product that may be evaluated in this article, or claim that may be made by its manufacturer, is not guaranteed or endorsed by the publisher.
Author disclaimer
The content is solely the responsibility of the authors and does not necessarily represent the official views of the National Heart, Lung, and Blood Institute or the National Institutes of Health.
Supplementary material
The Supplementary Material for this article can be found online at: https://www.frontiersin.org/articles/10.3389/fimmu.2025.1508786/full#supplementary-material
References
1. Erhabor J, Boakye E, Obisesan O, Osei AD, Tasdighi E, Mirbolouk H, et al. E-cigarette use among US adults in the 2021 behavioral risk factor surveillance system survey. JAMA Netw Open. (2023) 6:e2340859. doi: 10.1001/jamanetworkopen.2023.40859
2. Park J-A, Crotty Alexander LE, Christiani DC. Vaping and lung inflammation and injury. Annu Rev Physiol. (2022) 84:611–29. doi: 10.1146/annurev-physiol-061121-040014
3. Chaumont M, van de Borne P, Bernard A, Van Muylem A, Deprez G, Ullmo J, et al. Fourth generation e-cigarette vaping induces transient lung inflammation and gas exchange disturbances: results from two randomized clinical trials. Am J Physiol Lung Cell Mol Physiol. (2019) 316:L705–19. doi: 10.1152/ajplung.00492.2018
4. Moshensky A, Brand CS, Alhaddad H, Shin J, Masso-Silva JA, Advani I, et al. Effects of mango and mint pod-based e-cigarette aerosol inhalation on inflammatory states of the brain, lung, heart, and colon in mice. Elife. (2022) 11:e67621. doi: 10.7554/eLife.67621
5. Sayed IM, Masso-Silva JA, Mittal A, Patel A, Lin E, Moshensky A, et al. Inflammatory phenotype modulation in the respiratory tract and systemic circulation of e-cigarette users: a pilot study. Am J Physiol Lung Cell Mol Physiol. (2021) 321:L1134–46. doi: 10.1152/ajplung.00363.2021
6. Sharma A, Lee J, Fonseca AG, Moshensky A, Kothari T, Sayed IM, et al. E-cigarettes compromise the gut barrier and trigger inflammation. iScience. (2021) 24:102035. doi: 10.1016/j.isci.2021.102035
7. Qiu F, Liang C.-L, Liu H, Zeng Y.-Q, Hou S, Huang S, et al. Impacts of cigarette smoking on immune responsiveness: Up and down or upside down? Oncotarget. (2016) 8:268–84. doi: 10.1111/j.1475-6773.2012.01449.x
8. Vollmers C, Sit RV, Weinstein JA, Dekker CL, Quake SR. Genetic measurement of memory B-cell recall using antibody repertoire sequencing. Proc Natl Acad Sci U S A. (2013) 110:13463–8. doi: 10.1073/pnas.1312146110
9. Regan EA, Hokanson JE, Murphy JR, Make B, Lynch DA, Beaty TH, et al. Genetic epidemiology of COPD (COPDGene) study design. COPD. (2010) 7:32–43. doi: 10.3109/15412550903499522
10. Rojas-Quintero J, Ochsner SA, New F, Divakar P, Yang CX, Wu TD, et al. Spatial transcriptomics resolve an emphysema-specific lymphoid follicle B cell signature in chronic obstructive pulmonary disease. Am J Respir Crit Care Med. (2024) 209:48–58. doi: 10.1164/rccm.202303-0507LE
11. Hogg JC, Chu F, Utokaparch S, Woods R, Elliott WM, Buzatu L, et al. The nature of small airway obstruction in chronic obstructive pulmonary disease. N Engl J Med. (2004) 350:2645–53. doi: 10.1056/NEJMoa032158
12. Faner R, Cruz T, Casserras T, López-Giraldo A, Noell G, Coca I, et al. Network analysis of lung transcriptomics reveals a distinct B-cell signature in emphysema. Am J respiratory Crit Care Med. (2016) 193:1242–53. doi: 10.1164/rccm.201507-1311OC
13. Wan ES, Castaldi PJ, Cho MH, Hokanson JE, Regan EA, Make BJ, et al. Epidemiology, genetics, and subtyping of preserved ratio impaired spirometry (PRISm) in COPDGene. Respiratory Res. (2014) 15:89. doi: 10.1186/s12931-014-0089-y
14. Han MK, Kazerooni EA, Lynch DA, Liu LX, Murray S, Curtis JL, et al. Chronic obstructive pulmonary disease exacerbations in the COPDGene study: associated radiologic phenotypes. Radiology. (2011) 261:274–82. doi: 10.1148/radiol.11110173
15. Parker MM, Chase RP, Lamb A, Reyes A, Saferali A, Yun JH, et al. Correction to: RNA sequencing identifies novel non-coding RNA and exon-specific effects associated with cigarette smoking. BMC Med Genomics. (2019) 12:166. doi: 10.1186/s12920-019-0617-1
16. Alberdi A, Gilbert MTP. A guide to the application of Hill numbers to DNA-based diversity analyses. Mol Ecol Resour. (2019) 19:804–17. doi: 10.1111/men.2019.19.issue-4
17. Benjamini Y, Hochberg Y. Controlling the false discovery rate: A practical and powerful approach to multiple testing. J R Stat Society Ser B (Methodological). (1995) 57:289–300. doi: 10.1111/j.2517-6161.1995.tb02031.x
18. Sakornsakolpat P, Prokopenko D, Lamontagne M, Reeve NF, Guyatt AL, Jackson VE, et al. Genetic landscape of chronic obstructive pulmonary disease identifies heterogeneous cell-type and phenotype associations. Nat Genet. (2019) 51:494–505. doi: 10.1038/s41588-018-0342-2
19. Butler DC, Petterson S, Phillips RL, Bazemore AW. Measures of social deprivation that predict health care access and need within a rational area of primary care service delivery. Health Serv Res. (2013) 48:539–59. doi: 10.1111/j.1475-6773.2012.01449.x
20. Tesfaigzi Y, Curtis JL, Petrache I, Polverino F, Kheradmand F, Adcock IM, et al. Does chronic obstructive pulmonary disease originate from different cell types? Am J Respir Cell Mol Biol. (2023) 69:500–7. doi: 10.7554/eLife.67621
21. Tarbiah N, Todd I, Tighe PJ, Fairclough LC. Cigarette smoking differentially affects immunoglobulin class levels in serum and saliva: An investigation and review. Basic Clin Pharmacol Toxicol. (2019) 125:474–83. doi: 10.1111/bcpt.v125.5
22. Brusselle GG, Joos GF, Bracke KR. New insights into the immunology of chronic obstructive pulmonary disease. Lancet. (2011) 378:1015–26. doi: 10.1016/S0140-6736(11)60988-4
23. Brandsma C-A, Hylkema MN, Geerlings M, van Geffen WH, Postma DS, Timens W, Kerstjens HAM, et al. Increased levels of (class switched) memory B cells in peripheral blood of current smokers. Respir Res. (2009) 10:108. doi: 10.1186/1465-9921-10-108
24. Kotoulas S-C, Pataka A, Domvri K, Spyratos D, Katsaounou P, Porpodis K, et al. Acute effects of e-cigarette vaping on pulmonary function and airway inflammation in healthy individuals and in patients with asthma. Respirology. (2020) 25:1037–45. doi: 10.1111/resp.v25.10
25. Luo Y, Stent S. Smoking’s lasting effect on the immune system. Nature. (2024) 626:724–5. doi: 10.1038/d41586-024-00232-3
26. Peng G, Xi Y, Bellini C, Pham K, Zhuang ZW, Yan Q, et al. Nicotine dose-dependent epigenomic-wide DNA methylation changes in the mice with long-term electronic cigarette exposure. Am J Cancer Res. (2022) 12:3679–92. doi: 10.1165/rcmb.2018-0245PS
27. McAlinden KD, Eapen MS, Lu W, Sharma P, Sohal SS. The rise of electronic nicotine delivery systems and the emergence of electronic-cigarette-driven disease. Am J Physiol Lung Cell Mol Physiol. (2020) 319:L585–95. doi: 10.1152/ajplung.00160.2020
28. Crotty Alexander LE, Ware LB, Calfee CS, Callahan SJ, Eissenberg T, Farver C, et al. E-cigarette or vaping product use-associated lung injury: developing a research agenda. An NIH workshop report. Am J Respir Crit Care Med. (2020) 202:795–802. doi: 10.1164/rccm.201912-2332WS
29. Villa M, Gialitakis M, Tolaini M, Ahlfors H, Henderson CJ, Wolf CR, et al. Aryl hydrocarbon receptor is required for optimal B-cell proliferation. EMBO J. (2017) 36:116–28. doi: 10.15252/embj.201695027
30. Auschwitz E, Almeda J, Andl CD. Mechanisms of E-cigarette vape-induced epithelial cell damage. Cells. (2023) 12:2552. doi: 10.3390/cells12212552
31. Roy K, Chakraborty M, Kumar A, Manna AK, Roy NS. The NFκB signaling system in the generation of B-cell subsets: from germinal center B cells to memory B cells and plasma cells. Front Immunol. (2023) 14:1185597. doi: 10.3389/fimmu.2023.1185597
32. Polverino F, Seys LJM, Bracke KR, Owen CA. B cells in chronic obstructive pulmonary disease: moving to center stage. Am J Physiol Lung Cell Mol Physiol. (2016) 311:L687–95. doi: 10.1152/ajplung.00304.2016
33. Sullivan J-L, Bagevalu B, Glass C, Sholl L, Kraft M, Martinez FD, et al. B cell-adaptive immune profile in emphysema-predominant chronic obstructive pulmonary disease. Am J Respir Crit Care Med. (2019) 200:1434–9. doi: 10.1164/rccm.201903-0632LE
34. Syeda MZ, Hong T, Huang C, Huang W, Mu Q. B cell memory: from generation to reactivation: a multipronged defense wall against pathogens. Cell Death Discov. (2024) 10:117. doi: 10.1038/s41420-024-01889-5
35. Halper-Stromberg E, Yun JH, Parker MM, Singer RT, Gaggar A, Silverman EK, et al. Systemic markers of adaptive and innate immunity are associated with chronic obstructive pulmonary disease severity and spirometric disease progression. Am J Respir Cell Mol Biol. (2018) 58:500–9. doi: 10.1165/rcmb.2017-0373OC
36. Agustí A, Edwards LD, Rennard SI, MacNee W, Tal-Singer R, Miller BE, et al. Persistent systemic inflammation is associated with poor clinical outcomes in COPD: a novel phenotype. PloS One. (2012) 7:e37483. doi: 10.1371/journal.pone.0037483
37. Regan EA, Hersh CP, Castaldi PJ, DeMeo DL, Silverman EK, Crapo JD, et al. Omics and the search for blood biomarkers in chronic obstructive pulmonary disease. Insights from COPDGene. Am J Respir Cell Mol Biol. (2019) 61:143–9. doi: 10.1165/rcmb.2018-0245PS
38. Kostareli E, Gounari M, Agathangelidis A, Stamatopoulos K. Immunoglobulin gene repertoire in chronic lymphocytic leukemia: insight into antigen selection and microenvironmental interactions. Mediterr J Hematol Infect Dis. (2012) 4:e2012052. doi: 10.4084/mjhid.2012.052
Keywords: COPD, B cell, immune repertoire, vaping, smoking
Citation: Moll M, Xu Z, Boueiz A, Ryu MH, Silverman EK, Cho MH, Hersh CP, Sauler M, Polverino F, Kinney GL, Curtis JL, Crotty-Alexander LE, Vollmers C and Castaldi PJ (2025) B-cell immune repertoire sequencing in tobacco cigarette smoking, vaping, and chronic obstructive pulmonary disease in the COPDGene cohort. Front. Immunol. 16:1508786. doi: 10.3389/fimmu.2025.1508786
Received: 09 October 2024; Accepted: 07 April 2025;
Published: 02 May 2025.
Edited by:
Wenxia Song, University of Maryland, College Park, United StatesReviewed by:
Lance Kendall Blevins, Michigan State University, United StatesMarvyn T. Koning, Leiden University Medical Center (LUMC), Netherlands
Copyright © 2025 Moll, Xu, Boueiz, Ryu, Silverman, Cho, Hersh, Sauler, Polverino, Kinney, Curtis, Crotty-Alexander, Vollmers and Castaldi. This is an open-access article distributed under the terms of the Creative Commons Attribution License (CC BY). The use, distribution or reproduction in other forums is permitted, provided the original author(s) and the copyright owner(s) are credited and that the original publication in this journal is cited, in accordance with accepted academic practice. No use, distribution or reproduction is permitted which does not comply with these terms.
*Correspondence: Peter J. Castaldi, cmVwamNAY2hhbm5pbmcuaGFydmFyZC5lZHU=