- 1School of Public Health, North China University of Science and Technology, Tangshan, China
- 2College of Life Science, North China University of Science and Technology, Tangshan, China
- 3Hebei Key Laboratory of Occupational Health and Safety for Coal Industry, North China University of Science and Technology, Tangshan, China
- 4Affliated Tangshan Gongren Hospital, North China University of Science and Technology, Tangshan, China
Background: Toll-like receptor 3 (TLR3) is a pattern recognition receptor known to play a crucial role in the immune response to cancer. However, its effect on the efficacy of immunotherapy in lung adenocarcinoma (LUAD) remains unclear. This study aims to investigate the role of TLR3 in LUAD by examining its expression levels, prognostic significance, and impact on immune signaling pathways.
Methods: We analyzed the impact of TLR3 expression on the prognosis of lung adenocarcinoma patients using data from the Cancer Genome Atlas (TCGA) database and four additional cohorts (GSE72094, GSE30219, GSE50081 and GSE31210). Functional enrichment analyses were performed to compare molecular features between low and high TLR3 expression groups using gene set variation analysis (GSVA). We also examined the correlation between TLR3 and tumor mutation burden (TMB), immune infiltration, and PD-L1 expression. Further experimental validation was conducted using co-culture systems of LUAD cells and peripheral blood mononuclear cells (PBMCs) with PD1 inhibitors, and Western blot analysis to investigate the involvement of NF-κB signaling.
Results: TLR3 expression was significantly lower in LUAD tissues compared to normal tissues, with high TLR3 expression correlating with better survival outcomes across multiple cohorts. High TLR3 expression was associated with increased TMB and enhanced immune activation. Patients with high TLR3 expression exhibited higher immune checkpoint expression and immune cell infiltration. Experimental results showed that TLR3 agonists increased the susceptibility of LUAD cells to activated PBMCs under PD1 inhibitor therapy, inhibiting cell proliferation, migration, and invasion. Additionally, TLR3 has a strong positive correlation with MHC molecules and upregulated PD-L1 expression. NF-κB was identified as a key regulator of PD-L1 expression, with TLR3 agonists enhancing NF-κB and PD-L1 activity.
Conclusion: TLR3 enhances the anti-tumor immune response in LUAD by modulating NF-κB signaling and PD-L1 expression, making it a promising prognostic biomarker and therapeutic target. This study highlights the potential of TLR3 to improve immunotherapy outcomes, providing a comprehensive analysis of its role in LUAD and paving the way for novel therapeutic strategies targeting TLR3-mediated pathways.
1 Introduction
Based on the 2022 Global Cancer Statistics Report, lung cancer has the highest rates of both incidence and mortality compared to all other cancer types worldwide (1). Despite advancements in early detection and treatment methods, the overall survival (OS) rate for most LUAD patients has not significantly improved (2). Recently, PD1/PD-L1 targeted immunotherapy has shown significant clinical efficacy in the treatment of non-small cell lung cancer (NSCLC) (3–6). However, studies have shown that some tumor patients have response rates of less than 40% to anti-PD-L1/PD1 monotherapy due to primary or secondary resistance to immunotherapy (7, 8). Hence, the identification of targets that can increase the sensitivity of immune checkpoint inhibitors (ICIs) therapies by activating the immune response within the tumor microenvironment is of paramount importance.
Toll-like receptors (TLRs) are a highly conserved group of type I transmembrane proteins that play a crucial role in innate immunity (9, 10). Studies have showed that TLR3 is under-expressed in various cancer tissues, and its activation can convert cancer cells from immune tolerance to anti-tumor immunity, enhancing their anti-tumor activity (11–13). Similar to other TLRs, TLR3 agonist poly(I:C) has been demonstrated to activate natural killer (NK) cells and dendritic cells (DCs) via NF-κB signaling, leading to robust antitumor responses (14). It can also enhance the recruitment and activity of immunosuppressive cell types, such as myeloid-derived suppressor cells (MDSCs), through the internal signaling pathways of tumor cells, thereby promoting the formation of an immunosuppressive environment (15, 16).
Numerous research efforts indicate that individuals with high PD-L1 exhibit significantly improved survival rates after anti-PD1 antibody treatment (17–20). However, tumor cells can enhance PD-L1 transcription by activating transcriptional regulatory factors that modulate the JAK/STAT1/IRF1, NF-κB, and JAK/STAT3 signaling pathways in response to various cytokine stimuli (21, 22). As a novel form of immunotherapy, TLR3 agonists induce a significant upregulation of PD-L1 in cells by activating CD4+ and CD8+ T cells, as well as PD-L1 blockers (23). This phenomenon has been reported in a limited number of cancer types, including neuroblastoma. Therefore, this study investigates the role of TLR3 agonists in modulating the expression of PD-L1, an immune-related molecule present on the surface of lung adenocarcinoma cells, with the aim of enhancing the efficacy of sindelimab.
Given the critical role of TLRs in immune regulation and its potential impact on PD-L1 expression and NF-κB signaling, this study aims to provide valuable insights into TLR3 as an immunotherapy target, offering new avenues for enhancing the efficacy of immunotherapies in LUAD patients.
2 Materials and methods
2.1 Cell culture
A549 and PC9 cells were purchased from Procell (Hubei, China). These cells were cultivated in RPMI-1640 medium supplemented with 10% fetal bovine serum and antibiotics. The culturing environment was a humidified incubator set at 37°C and 5% CO2.
2.2 Chemicals and reagents
Sintilimab is an anti-PD-1 antibody supplied by Innovent Biologics (Jiangsu, China). TLR3 agonist Poly(I:C) was obtained from Invitrogen (CA, USA). NF-κB inhibitor BAY 11-7082 was purchased from MedChemExpress (NJ, USA). T Cell activator for activation of PBMCs were bought from StemCell Technologies(VAN, CAN). For cell culture, the fetal bovine serum (FBS) was obtained from Tianhang Biotechnology (Zhejiang, CHN). For western blot analysis, the antibody against β-actin was purchased from Proteintech (Chicago, IL,USA). The human NF-κB p65, NF-κB p65 (phospho S536), IKB alpha, IKB alpha (phospho S36), TLR3 and PD-L1 were purchased from Abcam(Cambridge, UK).
2.3 Migration and invasion assay
To conduct cell migration assays, 200 μL of FBS-free medium containing cells (3 × 104 cells) was placed into a 24-well Transwell culture insert. In the case of cell invasion assays, 1 × 105 cells were introduced into the upper chamber that was lined with 0.5% Matrigel (Corning Incorporated, Corning, USA). Subsequently, a total of 600μL of the complete medium was introduced into the lower chamber. Cells that moved to the bottom of the chamber or invaded it were preserved with 4% paraformaldehyde for 30 minutes and then dyed with 0.5% crystal violet (Solarbio, Beijing, China) for 15 minutes. At least five random fields of view were imaged under the microscope and cells was recorded.
2.4 Cell proliferation assays
For cell proliferation assays, cells from different treatment groups (5×103/well) were cultured in a 96-well plate and allowed to incubate for 24, 48, and 72 hours. The viability of the cells was assessed using the Cell Counting Kit-8 (Mei5 Biotechnology, Beijing, CHN). In the colony formation assay, cells from different treatment groups (3×105/well) were distributed into a six-well plate and cultured for 2 weeks in medium with 10% FBS. Colonies were subsequently treated with 4% paraformaldehyde for 20 minutes and then stained using 0.1% crystal violet (Solarbio, Beijing, China) for 15 minutes. Following this, the colonies were captured in photographs and subjected to analysis.
2.5 Quantitative real-time PCR
RNA from the cells was isolated with TRIZOL reagent (Invitrogen, CA, USA) and subsequently reverse-transcribed into cDNA using the RevertAid first-strand cDNA synthesis kit (Thermo Fisher Scientific, MA, USA). After this step, use SYBRGreen PCR Master Mix (Vazyme, China) for real-time quantitative PCR. The reverse transcription and qRT-PCR reaction setups were arranged following the guidelines provided by the manufacturer. To determine the relative levels of gene expression, the 2^(−ΔΔCT) method was employed, with normalization to GAPDH expression levels for every sample. The primers can be found in Supplementary Table 1.
2.6 Western blot and primary antibodies
Cells were disrupted using RIPA buffer (Thermo Fisher Scientific, MA, USA), and the total protein concentration was determined employing a BCA kit (Solarbio Science and Technology, Beijing, CHN). 10% SDS-PAGE was utilized to separate proteins, which were then transferred onto nitrocellulose membranes. The membranes underwent blocking with 5% milk at room temperature for a duration of 2 hours. After the blocking step, the membranes were exposed to the specified primary antibody, followed by a 1h incubation at 37˚C with a secondary antibody conjugated to horseradish peroxidase (HRP). Imaging was carried out using enhanced chemiluminescence luminescence reagents (Seven Biotech, Beijing, CHN). β-actin served as the reference control. All antibodies, including anti-NF-κB p65, anti-NF-κB p65 (phospho S536), anti-IKB alpha (phospho S36), Anti-TLR3, and Anti-PD-L1, were obtained from Abcam (Eugene, USA).
2.7 Construction of PD-L1 promoter plasmid and dual-luciferase reporter assay
We created reporter constructs that contained a 1424 bp fragment from the promoter region of PD-L1. The primers used were PD-L1-PF (5′-GG GGTACC TTT ATG CCC TGG GTC TTG-3′) and PD-L1-PR (5′-CCG CTCGAG TGA CCT TCG GTG AAA TCG-3′), these constructs featured cloning sites for Kpn I and Xho I (NEB, Ipswich, USA), as indicated by the underlined sequences. Subsequently, the PCR product was inserted into the pGL3-basic reporter vector (Promega, Madison, USA). Verification of all constructs was performed through direct sequencing. LUAD cells (2 × 105)were seeded in 24-well plates. Upon reaching 80% confluence, the cells were transfected using 200ng of pGL3-PD-L1pro and 1ng of pRL-SV40 plasmid with Lipofectamine 2000 reagent (Thermo Fisher Scientific, USA). Subsequently, the cells were then exposed to either 20 mM of the NF-κB inhibitor or a control for an additional 24 hours. After the treatment period, cells were harvested and the fluorescence signal was measured using a dual-luciferase reporter assay (Promega, USA).
2.8 Electrophoretic mobility shift assay
Isolation of nuclear proteins from A549 cells through the use of NE-PER™ Nuclear and Cytoplasmic Extraction Reagents (Thermo Fisher Scientific, Waltham, USA). The capacity for DNA binding was evaluated with a LightShiftTM Chemiluminescent EMSA kit (Thermo Fisher Scientific, Waltham, USA). The probes corresponding to the putative binding site of NF-κB on the promoter of PD-L1 were synthesized by Sangon Biotech (Shanghai, China). The sequences were PD-L1-PF (5′-AGC TTT CAA AAG GGC TTT CTT AAC CCT CAC C-3′) and PD-L1-PR (5′-GGT GAG GGT TAA GAA AGC CCT TTT GAA AGC T-3′). For regular EMSA, the binding reaction components were added in order according to the manufacturer’s instructions. During this phase, 20μg of nuclear extracts were treated with 20fmol of biotin-labeled probes for a duration of 15 minutes. To verify the specificity of the interactions between DNA and proteins, unlabeled competitive probes (cold probe and mutant probe) were employed. Additionally, the binding ability was verified using A549 nuclear protein treated with NF-κB inhibitor (BAY 11-7082). The reactions involving binding were carried out using electrophoresis in a 6.5% polyacrylamide gel. Following this, the samples were placed onto a nylon membrane with a positive charge and were cross-linked using UV light at a wavelength of 254 nm during the cross-linking process. Finally, chemiluminescence was used to visualize.
2.9 Tumor cells and PBMCs co-culture system
Peripheral blood samples were diluted with an equal volume EasySepTM Buffer and slowly added to SepMate tubes containing Lymphoprep™ (StemCell Technologies, VAN, CAN). The samples underwent centrifugation at 2000 rpm for a duration of 30 minutes at room temperature, which ranged from 15 to 25°C, with the brake disabled. PBMCs were cultivated in RPMI-1640 medium supplemented with 10% fetal bovine serum and stimulated using the T Cell activator for a duration of 3 days. Activated PBMC were incubated with LUAD cells at a 5:1 effector to target ratio for 24 hours. The PBMCs/A549 cocultured cells were treated with either anti-PD1 antibody (50µg/ml sintilimab) or Poly(I:C).
2.10 Bioinformatic analysis
The mutation annotation format (MAF) was obtained from the TCGA database through the utilization of the “maftools” R package, allowing us to examine the mutational landscape of patients categorized into different TLR3 expression groups.
Cox regression analysis was performed using the “survival” package to assess the association of variables with OS. The “survminer” package was employed to create Kaplan-Meier curves and perform log-rank tests.
A set of 50 hallmark gene sets (h.all.v7.5.symbols.gmt) and Kyoto Encyclopedia of Genes and Genomes (KEGG) pathways (c2.cp.kegg.v7.2.symbols) retrieved from the MsigDB database was estimated using gene set variation analysis (GSVA). Significant pathways between different groups were visualized using “ComplexHeatmap” R package.
To estimate cellular abundance in the TME, we used microenvironmental gene profiles associated with specific immune cell subsets from the previous study. The enrichment of 24 types of immune cells within the TME was assessed using GSVA (24). The immune checkpoint distribution was analyzed, and determine immune/stromal scores of tumor tissues using the “ESTIMATE” R package (25). We also analyzed the correlation between the expression of immunomodulatory factors including chemokines, immunoinhibitor, immune stimulators, histocompatibility complex (MHC), and TLR3 mRNA level in LUAD.
The “pRRophetic” R package was employed to predict the chemotherapeutic sensitivity for each lung adenocarcinoma sample using default parameters (26, 27). For immunotherapy, we used published data set of anti-PD1 and anti-CTLA4 cohorts (GSE91061) with melanoma (28), anti-PD-L1 cohort of urothelial carcinoma (IMvigor210). Subclass mapping for predicting clinical response to ICIs (29).
2.11 Statistical analyses
Statistical evaluations and graphical representations were carried out utilizing GraphPad Prism 8.0 (GraphPad Software, CA, USA) and R version 3.6.1. Correlation analyses were conducted using Pearson correlation analysis. Student’s t-test was used for two-group comparisons, with results presented as mean ± SD. The estimation of overall survival was performed using the Kaplan–Meier technique in conjunction with Cox regression analysis. P < 0.05 is the threshold for statistically significant differences.
3 Results
3.1 TLR3 expression and its clinical significance in LUAD
The workflow of this research is shown in Figure 1. Based on data from TCGA, our analysis revealed that the expression levels of TLR3 in LUAD tissues were notably reduced compared to those in normal tissues (Figure 2A). Subsequent studies on the clinical outcomes of TLR3 and LUAD across six cohorts (TCGA, GSE72094, GSE13213, GSE14814, GSE11969, and GSE68465) showed that high TLR3 expression is associated with better survival (Figures 2B–F).
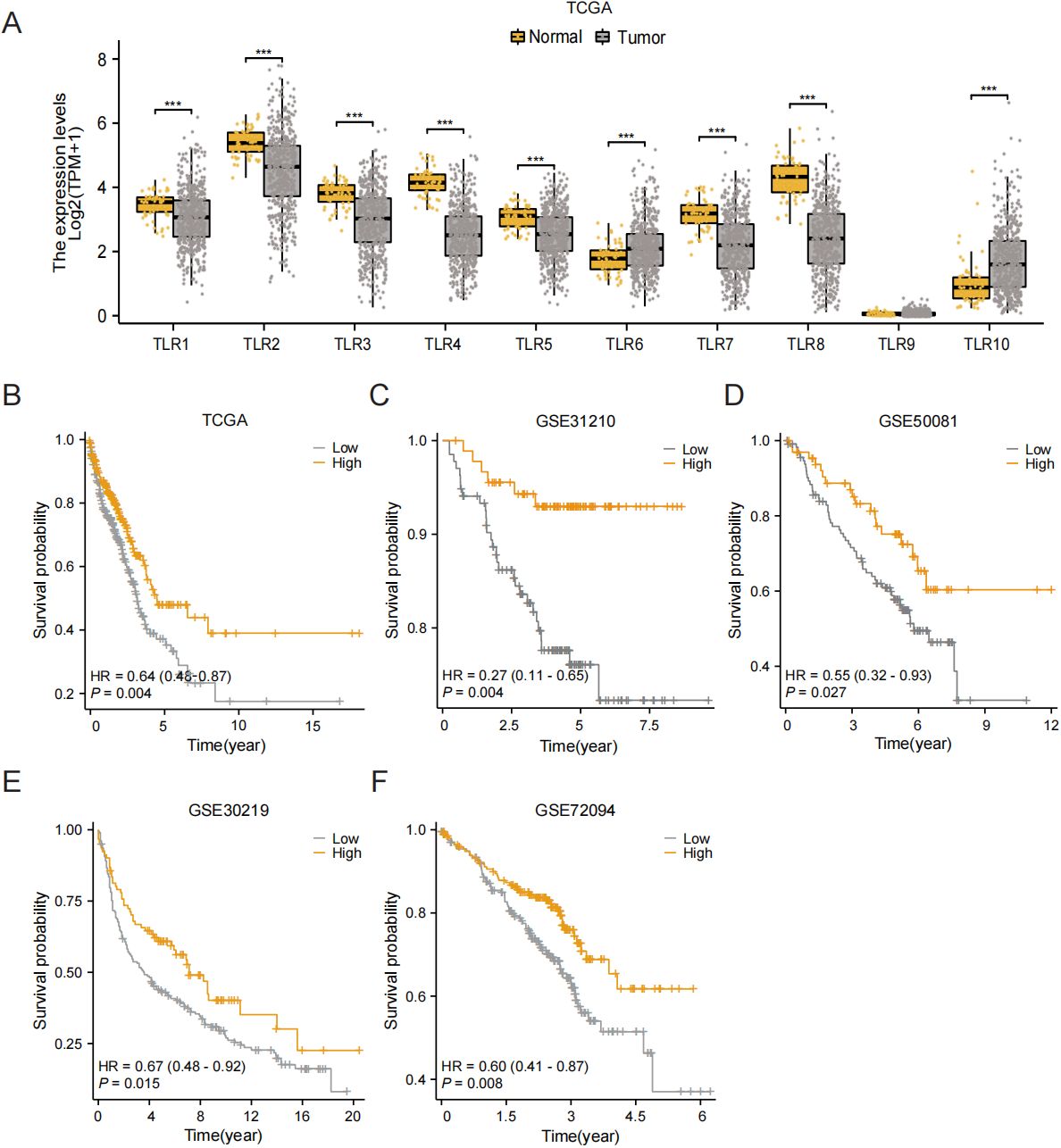
Figure 2. High TLR3 expression is associated with good prognosis in LUAD. (A) TLR3 expression in normal tissue and lung adenocarcinoma using TCGA dataset. (B-F) Kaplan-Meier plots for TLR3 expression based on datasets from TCGA, GSE31210, GSE50081, GSE30219 and GSE72094. ***P < 0.001.
Variations in immune infiltration and expression patterns of immune-related genes across LUAD clusters suggest that the response to immunotherapy may differ among these groups. TMB is predictive biomarkers of therapeutic response to immune checkpoint inhibitors (ICIs). In our investigation of the relationship between TMB and TLR3, we observed that levels of TMB were markedly elevated in the group with high TLR3 expression (P < 0.05) (Figure 3A). Patients with higher TMB scores had better survival outcomes compared to those with lower TMB scores (Figure 3B). Additionally, the influences of TP53, KRAS, EGFR and STK11 mutations on TLR3 were also investigated using GSE72094 dataset. LUAD patients with wild type of TP53 and STK11 had higher TLR3 expression when compared to those with mutations, while the EGFR mutation showed the opposite trend (Figures 3C–F). To explore the synergistic or antagonistic potential of TMB and TLR3 in predicting survival, patients were categorized based on these factors. Patients with both high TMB and TLR3 had the most favorable prognoses, while those with low TMB and TLR3 had the worst outcomes (Figure 3G).
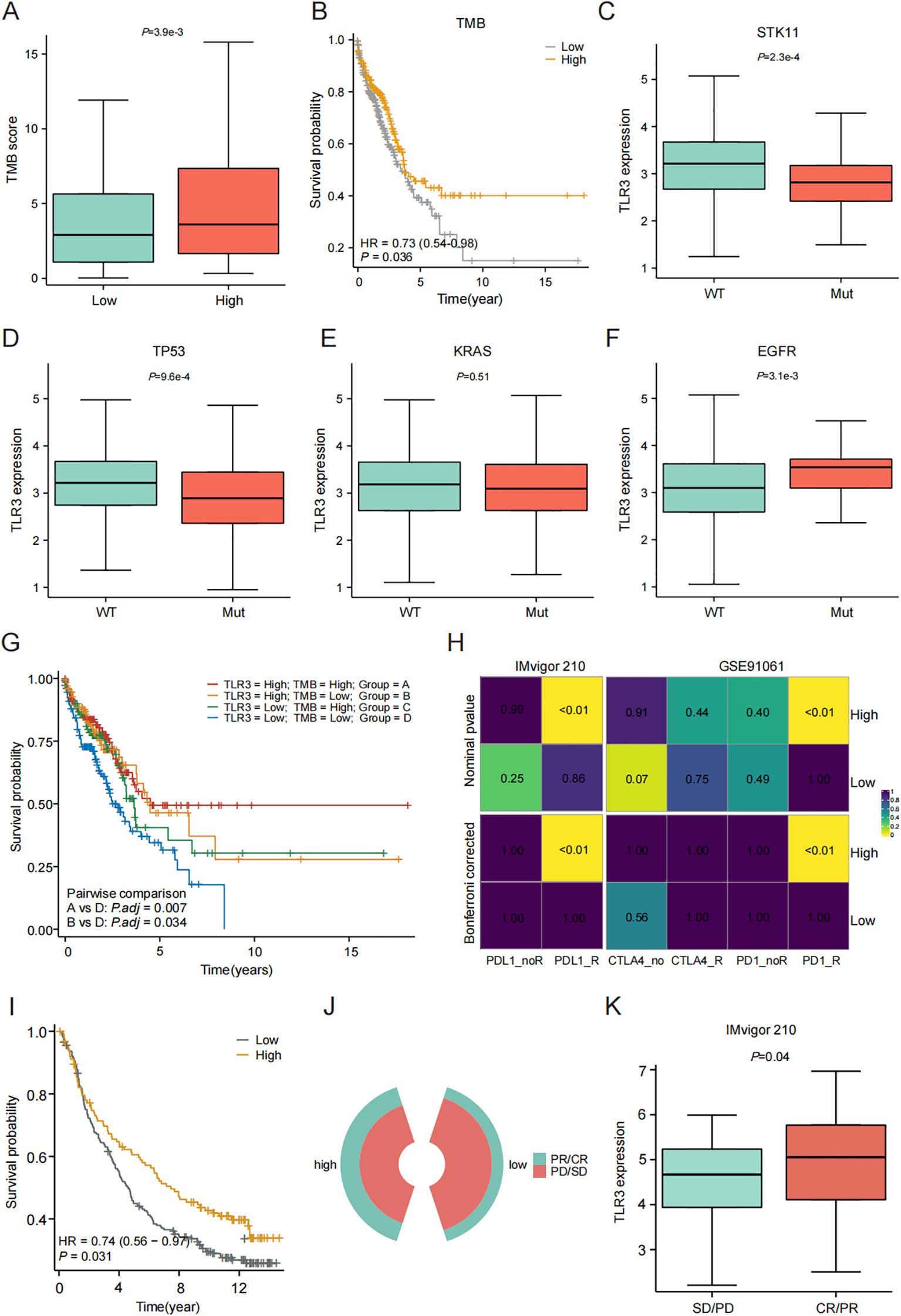
Figure 3. Evaluation of TLR3 expression on immunotherapy response. (A) Comparison of TMB between high- and low-TLR3 expression groups. (B) Kaplan-Meier curve of OS for patients classified by TMB. (C-F) The TLR3 expression in relation to mutation of STK11, TP53, KRAS and EGFR. (G) TMB combined with TLR3 expression to predict the prognosis of LUAD patients. (H) The subclass mapping algorithm predicts response to immunotherapy between high- and low-TLR3 expression groups. (I) Survival analyses for low and high TLR3 expression groups in Imvigor210 cohort. (J, K) Comparison of TLR3 expression between SD/PD and CR/PR groups of in IMvigor210 cohort. CR, complete response; PD, progressive disease; PR, partial response; SD, stable disease. Data shown as mean ± SD.
Furthermore, we analyzed the cohort of patients receiving immunotherapy using the subclass mapping algorithm to assess patient response to immunotherapy. The results showed that patients within the high TLR3 expression group were more likely to respond to anti-PD1 and PD-L1 therapy (Figure 3H). Additionally, we predicted the treatment response of TLR3 expression to immune checkpoint blockade using the anti-PD-L1 cohort of urothelial carcinoma (IMvigor210). High TLR3 expression was found to be a prognostic protective factor for patients receiving immunotherapy (Figure 3I). Also, TLR3 expression was higher in responders compared to non-responders (Figures 3J–K), indicating that patients with high TLR3 expression have increased immune sensitivity to PD-L1 blockade.
3.2 Molecular characteristics of high and low TLR3 expression in LUAD
Currently, in the majority of LUAD instances, classification occurs according to levels of molecular expression, potentially linking them to distinct biological functions. The hallmark gene sets, which encompass eight categories of processes, effectively summarize most of the relevant information derived from the original sets. Therefore, we explored the different molecular features of low and high TLR3 expression group.
It was observed that low-TLR3 expression exhibited the most activation in proliferation-related pathways (G2M checkpoint, E2F targets and Mitotic spindle), whereas those with high TLR3 expression had the lowest activation in these pathways. Conversely, patients with high TLR3 expression demonstrated the most immune activation (Complement, interferon alpha response, interferon gamma response and IL6-JAK-STAT3 signaling) and signaling-related processes (IL2-STAT5 signaling, TNFα signaling via NF-κB, KRAS signaling up, and PI3K-AKT-mTOR signaling) relevant processes (Figure 4A). Further enrichment analysis using the KEGG also confirmed these results (Figure 4B).
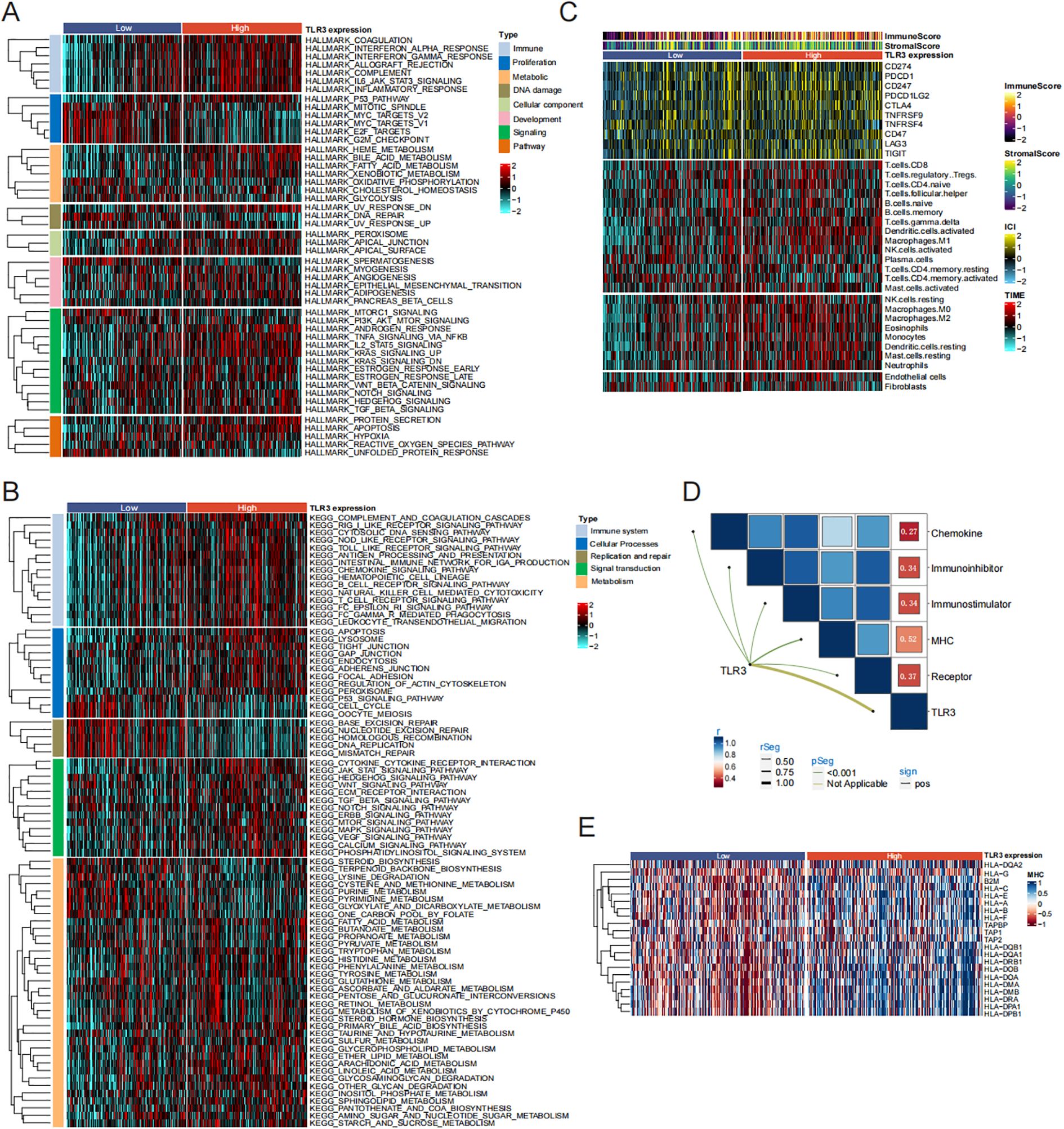
Figure 4. TLR3-related Pathway and immune infiltration analysis in LUAD. (A, B) Signal pathway enrichment analysis of 50 hallmark gene sets and KEGG analysis. (C) Immune checkpoint expression and immune cell infiltration enrichment in the TCGA cohort. (D, E) Correlation analysis between TLR3 expression and chemokine, receptor, MHC, immunoinhibitor and immunostimulator.
Due to the notable variations in immune processes across different clusters, we quantified the infiltration levels of microenvironmental immune cells and immune checkpoint expression in high and low TLR3 expression groups. As shown in Figure 4C, the estimation of various immune cell type abundances through the use of TIMER algorithms. Compared to the low TLR3 expression group, the high TLR3 expression group presented higher levels of immune checkpoint protein expression and a greater abundance of immune cell infiltration. Additionally, Previous studies have shown that T cells can induce a series of immune responses and clear tumor cells by binding to major MHC molecules (30). Therefore, we investigated the correlation between TLR3 and five immunomodulatory factors (chemokines, immune inhibitor, immune stimulators, MHC, receptors). Our data showed that TLR3 had the strongest positive correlation with MHC molecules involved in antigen presentation (r = 0.52, P < 0.001) (Figures 4D, E).
3.3 TLR3 enhance the anticancer effects of sintilimab in LUAD
To examine how TLR3 influences the anticancer activity of PBMCs with or without the presence of ICIs, we established an LUAD cells/PBMCs co-culture system in vitro. The schematic diagram is shown in Figure 5A. The co-culture system of PBMCs and LUAD cells was supplemented with sintilimab. The results showed that LUAD cells treated with TLR3 agonists were more susceptible to activated PBMCs under PD1 inhibitor therapy, leading to inhibited cell proliferation (Figures 5B, C). The trend was further validated by colony formation assay (Figures 5D, E). Additionally, we found that PBMCs inhibited the migration and invasion potential of LUAD cells treated with TLR3 agonists, and the addition of PD1 inhibitors further enhanced this inhibitory effect (Figures 5F, G). These findings suggest that TLR3 agonists promote the lymphocytoxicity of PBMCs and elicit a more robust antitumor immune response in LUAD cells.
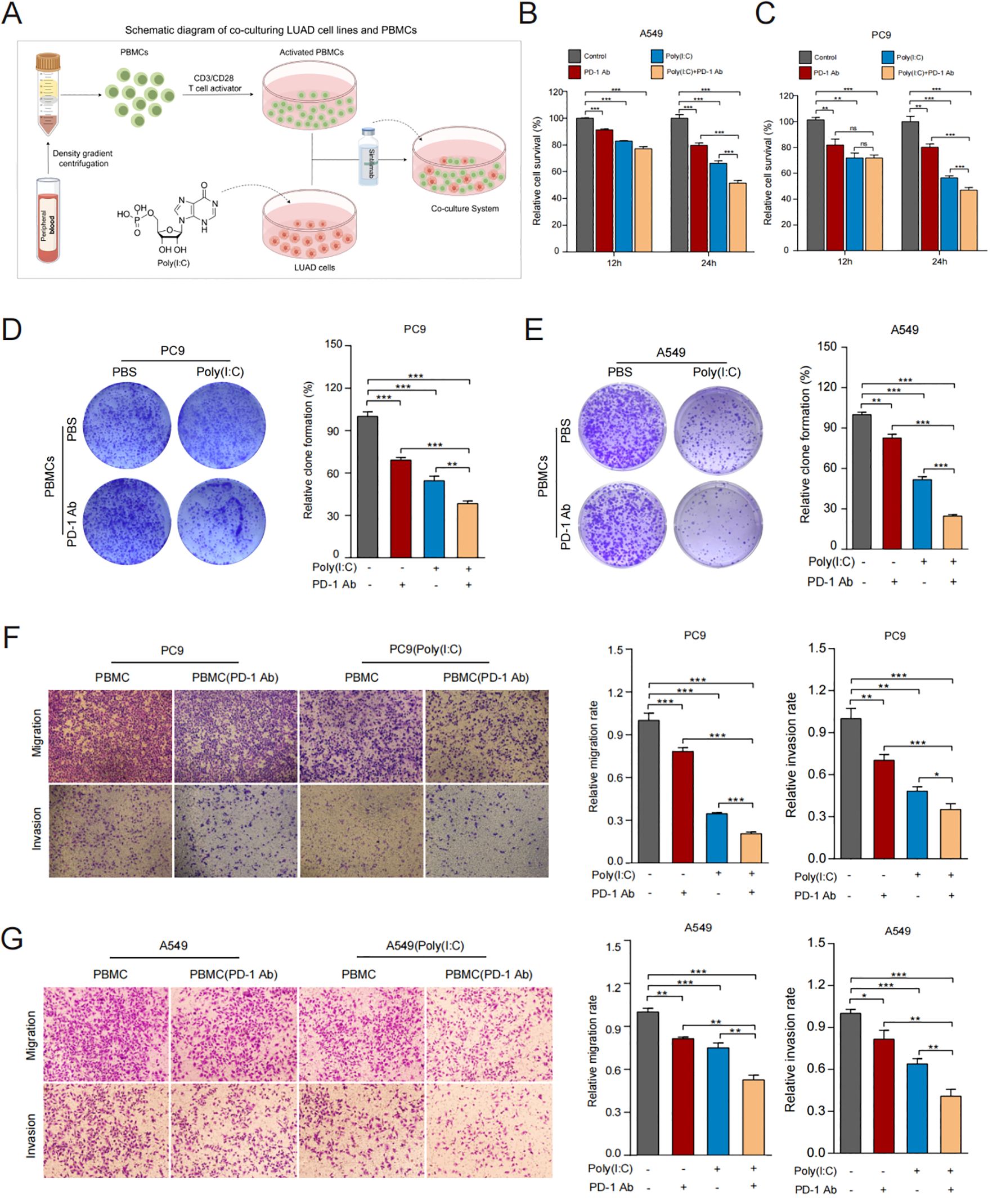
Figure 5. TLR3 promotes anti-tumor immune response in vitro. (A) Schematic diagram of co-culturing LUAD cell lines and PBMCs. (B, C) Cell viability of A549 and PC9 cells measured by CCK8 after co-culturing. (D, E) Proliferative ability of A549 and PC9 cells measured by colony formation assay after co-culturing. (F, G) Migration and invasion ability of A549 and PC9 cells measured by Transwell assay after co-culturing. Data shown as mean ± SD. *P<0.05, **P < 0.01, ***P < 0.001.
3.4 TLR3 regulates PD-L1 expression through NF-κB pathway
To explore the immune signaling pathways affected by TLR3, we reviewed the literature and found that NF-κB signaling essential for immune responses, inflammatory response and cancer therapy (31). Additionally, previous researchs indicate that elevated levels of PD-L1 expression and activation of tumor infiltrating lymphocytes (TILs) enhance the efficacy of PD-L1/PD-L1 immunotherapy (32). Based on correlation analysis utilizing the TCGA database revealed a positive relationship between the expression of PD-L1 and TLR3 (r = 0.302, P < 0.001) (Figure 6A). In addition, the treatment of LUAD cells with TLR3 agonists leads to a notable enhancement of cytokines expression compare to the control group (Figure 6B) and increase in PD-L1 expression (Figures 6C, D).
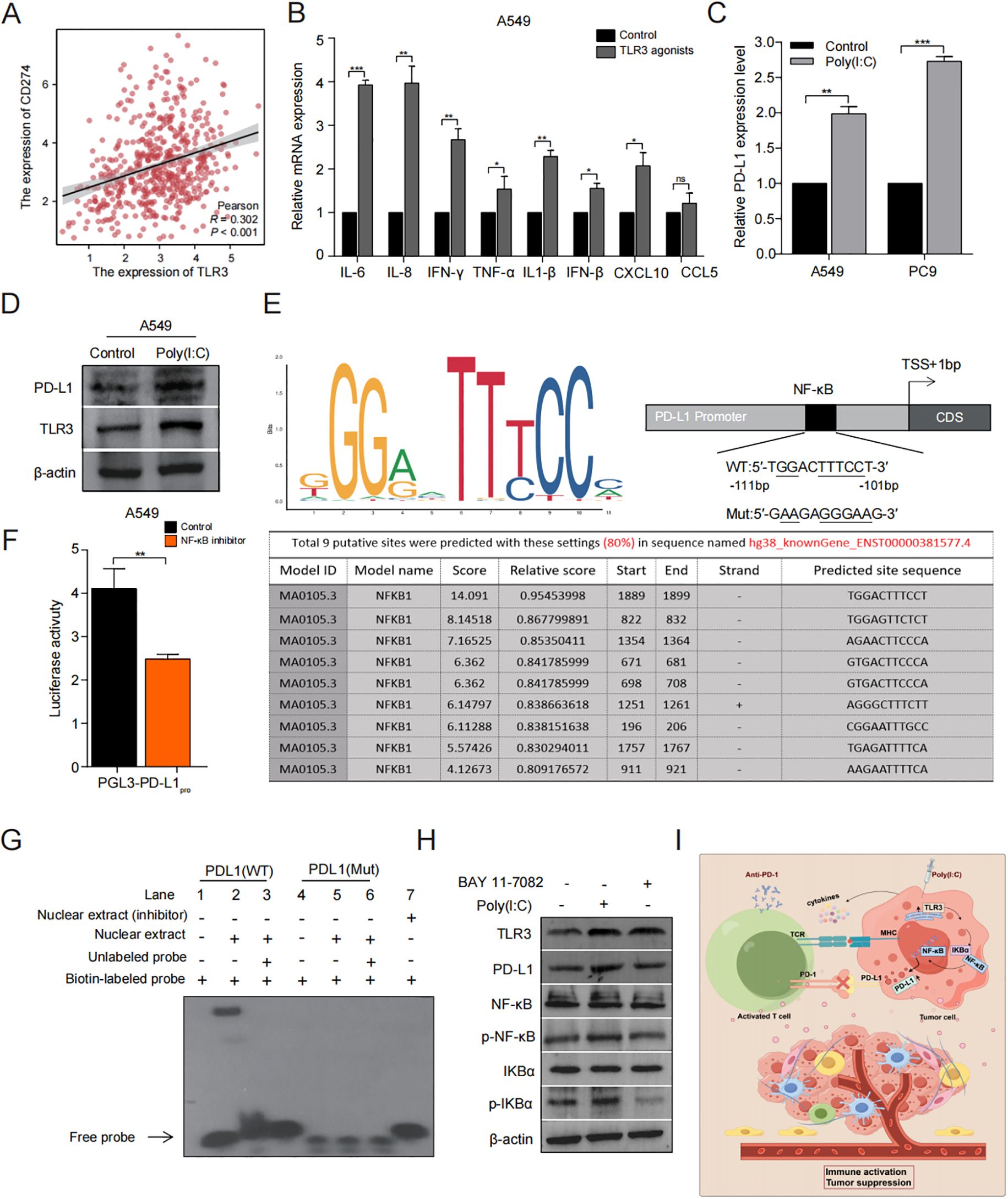
Figure 6. TLR3 regulates PD-L1 expression through NF-κB pathway. (A) Correlation between TLR3 and PD-L1 expression in the TCGA cohort. (B) The effect of TLR3 expression on cytokine release of A549 cells. (C, D) qRT-PCR and Western blotting analyses of PD-L1 regulated by TLR3. (E) JASPAR2024 prediction of the binding sequence of NF-κB to PD-L1. (F) Luciferase activity of pGL3-PD-L1pro in A549 cells treated with or without NF-κB inhibitor. (G) The binding activity of NF-κB to PD-L1 promoter identified by EMSA. (H) Western blotting analyses of the impact of TLR3 agonists and Bay-11 on NF-κB signaling. (I) Schematic diagram depicting the regulatory role of TLR3 in the TME immune landscapes of LUAD. Data shown as mean ± SD. *P < 0.05, **P < 0.01, ***P < 0.001, ns, no significance.
Based on the primary binding attributes of common transcription factors (TFs) as detailed in the JASPAR2024 database, NF-κB has been recognized as the most probable binder to PD-L1 (Figure 6E). Subsequently, we assessed how NF-κB influences the activity of PD-L1 promoter through luciferase assay and found that the promoter activity of PD-L1 decreased significantly when treated with NF-κB inhibitor (P < 0.001) (Figure 6F). Further analysis via EMSA showed that NF-κB can bind at the motif identified upstream of the PD-L1 (Figure 6G). These results demonstrate that NF-κB can regulate PD-L1 at the transcriptional level.
Next, we examined the expression levels of marker proteins associated with the NF-κB signaling pathway in A549 and PC9 cells with or without the addition of a TLR3 agonist (Poly(I:C)). This result was further validated by treatment with NF-κB inhibitor (BAY 11-7082). These results demonstrated that the level of TLR3, PD-L1, p-IκBα and p-NF-κB was significantly elevated in the Poly(I:C) treated group in comparison to the control group. However, after treatment with the addition of NF-κB inhibitor, these proteins were significantly inhibited (Figure 6H).
In conclusion, these findings indicate that TLR3 may regulate NF-κB signaling, thereby influencing the PD-L1 expression that could be pharmacologically targeted as an effective approach to sensitize patients with LUAD to ICIs (Figure 6I).
4 Discussion
Our study has revealed the pivotal role of TLR3 in regulating the immune landscape of LUAD, with particular emphasis on its potential to enhance the efficacy of immunotherapy. By analyzing multiple cohorts validation, we found that patients with higher levels of TLR3 demonstrated significantly improved survival, increased immune infiltration, and higher TMB, positioning TLR3 as a critical immune modulator in the tumor microenvironment.
Previous studies on TLR3 have primarily concentrated on its role in recognizing viral infections, mediating inflammation, and activating immune cells. For instance, TLR3 activation stimulates the production of various cytokines and chemokines via signal transduction pathways, including NF-κB and IRF3, thereby effectively counteracting viral invasion and regulating the homeostasis of the immune system (33, 34). As a crucial component of the immune system, the activation of the TLR3 receptor in dendritic cells significantly enhances the secretion efficiency of various cytokines, thereby effectively facilitating the activation process and functional differentiation of T lymphocytes (35, 36). However, recent studies have shown that the increased expression of endogenous TLR3 in tumor cells can improve the responses of CD4+ Th1 and CD8+ cytotoxic T cells (37, 38), and is used to improve immunotherapy by innate immunity in the TMEt (39, 40). Recently, increasing evidence has shown that combing TLR agonists with other therapies can effectively eliminate tumors (41). For example, combining Poly(I:C) with paclitaxel successfully increases tumor cytotoxicity in drug-resistant colon cancer cells through IFN-β secretion (42). Similarly, the combination of a TLR3 agonist with sorafenib can hinder the advancement of hepatocellular carcinoma through the activation of NK and CD8+ T cells (43). Additionally, a multi-center study also reported improved outcomes using a combined therapy of Poly(I:C), radiotherapy and temozolomide for glioblastoma treatment (44).
Our data show that TLR3 activation may enhance the effectiveness of PD1 inhibitors by amplifying the response within the TME. Given that ICIs have significantly prolonged the OS of patients with various cancers, including lung adenocarcinoma (45–47). However, many patients exhibit limited or no response to ICI therapies (3, 48, 49). Studies have shown that after treatment with anti-PD1 antibodies, the survival rate of patients with high expression of PD-L1 has been significantly improved (17–20). But PD-L1 expression levels vary greatly between individuals (50, 51). Therefore, PD-L1 expression serves as a critical determinant for evaluating clinical responses to anti- PD1 therapy (52, 53).
Multiple mechanisms are involved in the regulation of PD-L1 expression, with the regulation by transcription factors such as STAT3, AP-1, HIF-1α and NF-κB being included (54–58), as well as the regulation of histones and post-translational modifications (ubiquitination and acetylation) (59). Several studies have shown that TLR3 can activate nuclear NF-κB (60), which leads to the production of type I interferons and chemokines (61, 62). It is reported that the PARP1 inhibitor Olaparib can promotes the binding of the transcription factor nuclear phosphoprotein (NPM1) to the PD-L1 promoter in triple-negative breast cancer cells,thereby activating PD-L1 transcription (63). Which boosts the effectiveness of anti-PD-1 treatment. Similarly, our study observed a greater presence of CD8+T cells, CTLs, and NK cells in LUAD patients with elevated TLR3 expression, and to enhance the sensitivity of immune-tolerant LUAD to immunotherapy by regulating PD-L1 expression via the NF-κB pathway.
While these models provide valuable mechanistic insights, a limitation of our study is the reliance on in vitro and in silico data to define the relationship between TLR3 and immunotherapy response. Our future research will involve clinical validation in larger cohorts or prospective trials to confirm the utility of TLR3 as a biomarker or therapeutic target. Furthermore, we aim to elucidate the complex tumor microenvironment and associated mechanisms in vivo, particularly in response to combined immunotherapy, through experiments conducted in animal models.
5 Conclusion
In conclusion, this study offers a thorough analysis of the role of TLR3 in LUAD, demonstrating its potential to improve immunotherapy outcomes. By enhancing immune infiltration, TMB, and PD-L1 expression via the NF-κB pathway, TLR3 functions as both a biomarker for prognosis and a prospective therapeutic target.
Data availability statement
The original contributions presented in the study are included in the article/Supplementary Material. Further inquiries can be directed to the corresponding author.
Author contributions
AL: Conceptualization, Validation, Writing – original draft. ML: Conceptualization, Visualization, Writing – original draft. XL: Conceptualization, Visualization, Writing – original draft. HW: Methodology, Validation, Writing – original draft. XGL: Methodology, Validation, Writing – review & editing. ZZ: Data curation, Software, Writing – review & editing. XZ: Funding acquisition, Project administration, Supervision, Writing – review & editing.
Funding
The author(s) declare that financial support was received for the research and/or publication of this article. This work was supported by the Foundation for Talents by Tangshan Human Resources and Social Security Bureau (grant numbers A202110007), and the Clinical Research Special Funding Fund of Wu Jieping Medical Foundation (grant numbers 320.6750.2021-02-100), the Central Guided Local Science and Technology Development Fund Project (grant numbers 246Z7727G) and the Graduate Innovation Funding Project in Hebei Province (grant numbers CXZZBS2023119).
Acknowledgments
We thank the study participants and research staff for their contributions to this study.
Conflict of interest
The authors declare that the research was conducted in the absence of any commercial or financial relationships that could be construed as a potential conflict of interest.
Generative AI statement
The author(s) declare that no Generative AI was used in the creation of this manuscript.
Publisher’s note
All claims expressed in this article are solely those of the authors and do not necessarily represent those of their affiliated organizations, or those of the publisher, the editors and the reviewers. Any product that may be evaluated in this article, or claim that may be made by its manufacturer, is not guaranteed or endorsed by the publisher.
Supplementary material
The Supplementary Material for this article can be found online at: https://www.frontiersin.org/articles/10.3389/fimmu.2025.1585747/full#supplementary-material
References
1. Bray F, Laversanne M, Sung H, Ferlay J, Siegel RL, Soerjomataram I, et al. Global cancer statistics 2022: GLOBOCAN estimates of incidence and mortality worldwide for 36 cancers in 185 countries. CA Cancer J Clin. (2024) 74:229–63. doi: 10.3322/caac.21834
2. Chen D, Wang R, Yu C, Cao F, Zhang X, Yan F, et al. FOX-A1 contributes to acquisition of chemoresistance in human lung adenocarcinoma via transactivation of SOX5. EBioMedicine. (2019) 44:150–61. doi: 10.1016/j.ebiom.2019.05.046
3. Brahmer JR, Tykodi SS, Chow LQ, Hwu WJ, Topalian SL, Hwu P, et al. Safety and activity of anti-PD-L1 antibody in patients with advanced cancer. N Engl J Med. (2012) 366:2455–65. doi: 10.1056/NEJMoa1200694
4. Garon EB, Rizvi NA, Hui R, Leighl N, Balmanoukian AS, Eder JP, et al. Pembrolizumab for the treatment of non-small-cell lung cancer. N Engl J Med. (2015) 372:2018–28. doi: 10.1056/NEJMoa1501824
5. Zou W, Wolchok JD, Chen L. PD-L1 (B7-H1) and PD-1 pathway blockade for cancer therapy: Mechanisms, response biomarkers, and combinations. Sci Transl Med. (2016) 8:328rv4. doi: 10.1126/scitranslmed.aad7118
6. Boussiotis VA. Molecular and biochemical aspects of the PD-1 checkpoint pathway. N Engl J Med. (2016) 375:1767–78. doi: 10.1056/NEJMra1514296
7. Yarchoan M, Hopkins A, Jaffee EM. Tumor mutational burden and response rate to PD-1 inhibition. N Engl J Med. (2017) 377:2500–1. doi: 10.1056/NEJMc1713444
8. Pitt JM, Vetizou M, Daillere R, Roberti MP, Yamazaki T, Routy B, et al. Resistance mechanisms to immune-checkpoint blockade in cancer: tumor-intrinsic and -extrinsic factors. Immunity. (2016) 44:1255–69. doi: 10.1016/j.immuni.2016.06.001
9. Chen JQ, Szodoray P, Zeher M. Toll-like receptor pathways in autoimmune diseases. Clin Rev Allergy Immunol. (2016) 50:1–17. doi: 10.1007/s12016-015-8473-z
10. Zhang Y, Liu J, Wang C, Liu J, Lu W. Toll-like receptors gene polymorphisms in autoimmune disease. Front Immunol. (2021) 12:672346. doi: 10.3389/fimmu.2021.672346
11. Bonnin M, Fares N, Testoni B, Estornes Y, Weber K, Vanbervliet B, et al. Toll-like receptor 3 downregulation is an escape mechanism from apoptosis during hepatocarcinogenesis. J Hepatol. (2019) 71:763–72. doi: 10.1016/j.jhep.2019.05.031
12. Fan L, Zhou P, Hong Q, Chen AX, Liu GY, Yu KD, et al. Toll-like receptor 3 acts as a suppressor gene in breast cancer initiation and progression: a two-stage association study and functional investigation. Oncoimmunology. (2019) 8:e1593801. doi: 10.1080/2162402X.2019.1593801
13. Pradere JP, Dapito DH, Schwabe RF. The Yin and Yang of Toll-like receptors in cancer. Oncogene. (2014) 33:3485–95. doi: 10.1038/onc.2013.302
14. Guillerey C, Chow MT, Miles K, Olver S, Sceneay J, Takeda K, et al. Toll-like receptor 3 regulates NK cell responses to cytokines and controls experimental metastasis. Oncoimmunology. (2015) 4:e1027468. doi: 10.1080/2162402X.2015.1027468
15. Papafragkos I, Grigoriou M, Boon L, Kloetgen A, Hatzioannou A, Verginis P. Ablation of NLRP3 inflammasome rewires MDSC function and promotes tumor regression. Front Immunol. (2022) 13:889075. doi: 10.3389/fimmu.2022.889075
16. Theivanthiran B, Evans KS, DeVito NC, Plebanek M, Sturdivant M, Wachsmuth LP, et al. A tumor-intrinsic PD-L1/NLRP3 inflammasome signaling pathway drives resistance to anti-PD-1 immunotherapy. J Clin Invest. (2020) 130:2570–86. doi: 10.1172/JCI133055
17. Smit EF, de Langen AJ. Pembrolizumab for all PD-L1-positive NSCLC. Lancet. (2019) 393:1776–8. doi: 10.1016/S0140-6736(18)32559-5
18. Herbst RS, Baas P, Kim DW, Felip E, Perez-Gracia JL, Han JY, et al. Pembrolizumab versus docetaxel for previously treated, PD-L1-positive, advanced non-small-cell lung cancer (KEYNOTE-010): a randomised controlled trial. Lancet. (2016) 387:1540–50. doi: 10.1016/S0140-6736(15)01281-7
19. Huang M, Lopes GL, Insinga RP, Burke T, Ejzykowicz F, Zhang Y, et al. Cost-effectiveness of pembrolizumab versus chemotherapy as first-line treatment in PD-L1-positive advanced non-small-cell lung cancer in the USA. Immunotherapy. (2019) 11:1463–78. doi: 10.2217/imt-2019-0178
20. Velcheti V, Chandwani S, Chen X, Pietanza MC, Burke T. First-line pembrolizumab monotherapy for metastatic PD-L1-positive NSCLC: real-world analysis of time on treatment. Immunotherapy. (2019) 11:889–901. doi: 10.2217/imt-2019-0061
21. Garcia-Diaz A, Shin DS, Moreno BH, Saco J, Escuin-Ordinas H, Rodriguez GA, et al. Interferon receptor signaling pathways regulating PD-L1 and PD-L2 expression. Cell Rep. (2017) 19:1189–201. doi: 10.1016/j.celrep.2017.04.031
22. Concha-Benavente F, Srivastava RM, Trivedi S, Lei Y, Chandran U, Seethala RR, et al. Identification of the cell-intrinsic and -extrinsic pathways downstream of EGFR and IFNgamma that induce PD-L1 expression in head and neck cancer. Cancer Res. (2016) 76:1031–43. doi: 10.1158/0008-5472.CAN-15-2001
23. Boes M, Meyer-Wentrup F. TLR3 triggering regulates PD-L1 (CD274) expression in human neuroblastoma cells. Cancer Lett. (2015) 361:49–56. doi: 10.1016/j.canlet.2015.02.027
24. Hanzelmann S, Castelo R, Guinney J. GSVA: gene set variation analysis for microarray and RNA-seq data. BMC Bioinf. (2013) 14:7. doi: 10.1186/1471-2105-14-7
25. Yoshihara K, Shahmoradgoli M, Martinez E, Vegesna R, Kim H, Torres-Garcia W, et al. Inferring tumour purity and stromal and immune cell admixture from expression data. Nat Commun. (2013) 4:2612. doi: 10.1038/ncomms3612
26. Geeleher P, Cox NJ, Huang RS. Clinical drug response can be predicted using baseline gene expression levels and in vitro drug sensitivity in cell lines. Genome Biol. (2014) 15:R47. doi: 10.1186/gb-2014-15-3-r47
27. Geeleher P, Cox N, Huang RS. pRRophetic: an R package for prediction of clinical chemotherapeutic response from tumor gene expression levels. PloS One. (2014) 9:e107468. doi: 10.1371/journal.pone.0107468
28. McGranahan N, Furness AJ, Rosenthal R, Ramskov S, Lyngaa R, Saini SK, et al. Clonal neoantigens elicit T cell immunoreactivity and sensitivity to immune checkpoint blockade. Science. (2016) 351:1463–9. doi: 10.1126/science.aaf1490
29. Lu X, Jiang L, Zhang L, Zhu Y, Hu W, Wang J, et al. Immune signature-based subtypes of cervical squamous cell carcinoma tightly associated with human papillomavirus type 16 expression, molecular features, and clinical outcome. Neoplasia. (2019) 21:591–601. doi: 10.1016/j.neo.2019.04.003
30. Yarchoan M, Johnson BA 3rd, Lutz ER, Laheru DA, Jaffee EM. Targeting neoantigens to augment antitumour immunity. Nat Rev Cancer. (2017) 17:209–22. doi: 10.1038/nrc.2016.154
31. Li Q, Verma IM. NF-kappaB regulation in the immune system. Nat Rev Immunol. (2002) 2:725–34. doi: 10.1038/nri910
32. Doroshow DB, Sanmamed MF, Hastings K, Politi K, Rimm DL, Chen L, et al. Immunotherapy in non-small cell lung cancer: facts and hopes. Clin Cancer Res. (2019) 25:4592–602. doi: 10.1158/1078-0432.CCR-18-1538
33. Liu Y, Mo CF, Luo XY, Li H, Guo HJ, Sun H, et al. Activation of toll-like receptor 3 induces interleukin-1 receptor antagonist expression by activating the interferon regulatory factor 3. J innate immunity. (2020) 12:304–20. doi: 10.1159/000504321
34. Chen CY, Hung YF, Tsai CY, Shih YC, Chou TF, Lai MZ, et al. Transcriptomic analysis and C-terminal epitope tagging reveal differential processing and signaling of endogenous TLR3 and TLR7. Front Immunol. (2021) 12:686060. doi: 10.3389/fimmu.2021.686060
35. Seo JW, Yang EJ, Kim SH, Choi IH. An inhibitory alternative splice isoform of Toll-like receptor 3 is induced by type I interferons in human astrocyte cell lines. BMB reports. (2015) 48:696–701. doi: 10.5483/bmbrep.2015.48.12.106
36. Thierry S, Maadadi S, Berton A, Dimier L, Perret C, Vey N, et al. TL-532, a novel specific Toll-like receptor 3 agonist rationally designed for targeting cancers: discovery process and biological characterization. Microbial Cell. (2023) 10:117–32. doi: 10.15698/mic2023.06.797
37. Zhu M, Xu W, Su H, Huang Q, Wang B. Addition of CpG ODN and Poly (I:C) to a standard maturation cocktail generates monocyte-derived dendritic cells and induces a potent Th1 polarization with migratory capacity. Hum Vaccin Immunother. (2015) 11:1596–605. doi: 10.1080/21645515.2015.1046659
38. Lee BR, Jeong SK, Ahn BC, Lee BJ, Shin SJ, Yum JS, et al. Combination of TLR1/2 and TLR3 ligands enhances CD4(+) T cell longevity and antibody responses by modulating type I IFN production. Sci Rep. (2016) 6:32526. doi: 10.1038/srep32526
39. Amos SM, Pegram HJ, Westwood JA, John LB, Devaud C, Clarke CJ, et al. Adoptive immunotherapy combined with intratumoral TLR agonist delivery eradicates established melanoma in mice. Cancer Immunol Immunother. (2011) 60:671–83. doi: 10.1007/s00262-011-0984-8
40. Liu Z, Han C, Fu YX. Targeting innate sensing in the tumor microenvironment to improve immunotherapy. Cell Mol Immunol. (2020) 17:13–26. doi: 10.1038/s41423-019-0341-y
41. Adams S. Toll-like receptor agonists in cancer therapy. Immunotherapy. (2009) 1:949–64. doi: 10.2217/imt.09.70
42. Zhao J, Xue Y, Pan Y, Yao A, Wang G, Li D, et al. Toll-like receptor 3 agonist poly I:C reinforces the potency of cytotoxic chemotherapy via the TLR3-UNC93B1-IFN-beta signaling axis in paclitaxel-resistant colon cancer. J Cell Physiol. (2019) 234:7051–61. doi: 10.1002/jcp.27459
43. Schlom J, Arlen PM, Gulley JL. Cancer vaccines: moving beyond current paradigms. Clin Cancer Res. (2007) 13:3776–82. doi: 10.1158/1078-0432.CCR-07-0588
44. Rosenfeld MR, Chamberlain MC, Grossman SA, Peereboom DM, Lesser GJ, Batchelor TT, et al. A multi-institution phase II study of poly-ICLC and radiotherapy with concurrent and adjuvant temozolomide in adults with newly diagnosed glioblastoma. Neuro Oncol. (2010) 12:1071–7. doi: 10.1093/neuonc/noq071
45. Ribas A, Wolchok JD. Cancer immunotherapy using checkpoint blockade. Science. (2018) 359:1350–5. doi: 10.1126/science.aar4060
46. Singh S, Hassan D, Aldawsari HM, Molugulu N, Shukla R, Kesharwani P. Immune checkpoint inhibitors: a promising anticancer therapy. Drug Discov Today. (2020) 25:223–9. doi: 10.1016/j.drudis.2019.11.003
47. Li J, Wang G, Zhang W, Jin G, Zhang M, Jiang X, et al. Graphene film-functionalized germanium as a chemically stable, electrically conductive, and biologically active substrate. J Mater Chem B. (2015) 3:1544–55. doi: 10.1039/c4tb01510j
48. Park YJ, Kuen DS, Chung Y. Future prospects of immune checkpoint blockade in cancer: from response prediction to overcoming resistance. Exp Mol Med. (2018) 50:1–13. doi: 10.1038/s12276-018-0130-1
49. Hegde PS, Chen DS. Top 10 challenges in cancer immunotherapy. Immunity. (2020) 52:17–35. doi: 10.1016/j.immuni.2019.12.011
50. Yu H, Boyle TA, Zhou C, Rimm DL, Hirsch FR. PD-L1 expression in lung cancer. J Thorac Oncol. (2016) 11:964–75. doi: 10.1016/j.jtho.2016.04.014
51. Skov BG, Rorvig SB, Jensen THL, Skov T. The prevalence of programmed death ligand-1 (PD-L1) expression in non-small cell lung cancer in an unselected, consecutive population. Mod Pathol. (2020) 33:109–17. doi: 10.1038/s41379-019-0339-0
52. Chen Y, Liu Q, Chen Z, Wang Y, Yang W, Hu Y, et al. PD-L1 expression and tumor mutational burden status for prediction of response to chemotherapy and targeted therapy in non-small cell lung cancer. J Exp Clin Cancer Res. (2019) 38:193. doi: 10.1186/s13046-019-1192-1
53. Clark CA, Gupta HB, Sareddy G, Pandeswara S, Lao S, Yuan B, et al. Tumor-intrinsic PD-L1 signals regulate cell growth, pathogenesis, and autophagy in ovarian cancer and melanoma. Cancer Res. (2016) 76:6964–74. doi: 10.1158/0008-5472.CAN-16-0258
54. Maeda T, Hiraki M, Jin C, Rajabi H, Tagde A, Alam M, et al. MUC1-C induces PD-L1 and immune evasion in triple-negative breast cancer. Cancer Res. (2018) 78:205–15. doi: 10.1158/0008-5472.CAN-17-1636
55. Noman MZ, Desantis G, Janji B, Hasmim M, Karray S, Dessen P, et al. PD-L1 is a novel direct target of HIF-1alpha, and its blockade under hypoxia enhanced MDSC-mediated T cell activation. J Exp Med. (2014) 211:781–90. doi: 10.1084/jem.20131916
56. Green MR, Rodig S, Juszczynski P, Ouyang J, Sinha P, O’Donnell E, et al. Constitutive AP-1 activity and EBV infection induce PD-L1 in Hodgkin lymphomas and posttransplant lymphoproliferative disorders: implications for targeted therapy. Clin Cancer Res. (2012) 18:1611–8. doi: 10.1158/1078-0432.CCR-11-1942
57. Chung SS, Giehl N, Wu Y, Vadgama JV. STAT3 activation in HER2-overexpressing breast cancer promotes epithelial-mesenchymal transition and cancer stem cell traits. Int J Oncol. (2014) 44:403–11. doi: 10.3892/ijo.2013.2195
58. Sun C, Mezzadra R, Schumacher TN. Regulation and function of the PD-L1 checkpoint. Immunity. (2018) 48:434–52. doi: 10.1016/j.immuni.2018.03.014
59. Woods DM, Sodre AL, Villagra A, Sarnaik A, Sotomayor EM, Weber J. HDAC inhibition upregulates PD-1 ligands in melanoma and augments immunotherapy with PD-1 blockade. Cancer Immunol Res. (2015) 3:1375–85. doi: 10.1158/2326-6066.CIR-15-0077-T
60. Doyle S, Vaidya S, O’Connell R, Dadgostar H, Dempsey P, Wu T, et al. IRF3 mediates a TLR3/TLR4-specific antiviral gene program. Immunity. (2002) 17:251–63. doi: 10.1016/s1074-7613(02)00390-4
61. Yamamoto M, Sato S, Hemmi H, Hoshino K, Kaisho T, Sanjo H, et al. Role of adaptor TRIF in the MyD88-independent toll-like receptor signaling pathway. Science. (2003) 301:640–3. doi: 10.1126/science.1087262
62. Oshiumi H, Matsumoto M, Funami K, Akazawa T, Seya T. TICAM-1, an adaptor molecule that participates in Toll-like receptor 3-mediated interferon-beta induction. Nat Immunol. (2003) 4:161–7. doi: 10.1038/ni886
Keywords: toll-like receptor 3, lung adenocarcinoma, PD-L1, tumor microenvironment, immunotherapy
Citation: Li A, Luo M, Liu X, Wu H, Liu X, Zhang Z and Zhang X (2025) Toll-like receptor 3 activation enhances antitumor immune response in lung adenocarcinoma through NF-κB signaling pathway. Front. Immunol. 16:1585747. doi: 10.3389/fimmu.2025.1585747
Received: 01 March 2025; Accepted: 21 April 2025;
Published: 08 May 2025.
Edited by:
Jinghua Pan, Jinan University, ChinaReviewed by:
Alex Braiman, Ben-Gurion University of the Negev, IsraelRan Tao, Texas A&M University Baylor College of Dentistry, United States
Copyright © 2025 Li, Luo, Liu, Wu, Liu, Zhang and Zhang. This is an open-access article distributed under the terms of the Creative Commons Attribution License (CC BY). The use, distribution or reproduction in other forums is permitted, provided the original author(s) and the copyright owner(s) are credited and that the original publication in this journal is cited, in accordance with accepted academic practice. No use, distribution or reproduction is permitted which does not comply with these terms.
*Correspondence: Xuemei Zhang, emhhbmd4dWVtZWlAbmNzdC5lZHUuY24=
†These authors have contributed equally to this work and share first authorship