- 1Vector Genetics Laboratory, Department of Pathology, Microbiology and Immunology, University of California, Davis, Davis, CA, United States
- 2Global Health and Tropical Medicine, Instituto de Higiene e Medicina Tropical, Universidade Nova de Lisboa, Lisboa, Portugal
- 3Programa Nacional de Luta Contra o Paludismo, São Tomé, São Tomé and Príncipe
Islands possess physical characteristics that make them uniquely well-suited for initial field trials of new genetic-based technologies applied to African malaria vectors. This has led to efforts to characterize the degree of isolation of island mosquito populations. São Tomé and Príncipe (STP) is a country composed of two small islands in the Gulf of Guinea (Central Africa) where Anopheles coluzzii is the primary malaria vector. Several studies have shown a relatively high degree of genetic isolation between A. coluzzii populations in STP and the mainland compared with pairs of mainland populations separated by equivalent distances. Here, we analyzed complete mitochondrial genomes of individual A. coluzzii specimens from STP and neighboring mainland countries. The objectives are to describe the history of A. coluzzii establishment in STP, specifically to address several questions germane to their suitability as sites for a field trial release of genetically engineered mosquitoes (GEMs). These questions include: (i) What are the origins of A. coluzzii populations in STP?; (ii) How many introductions occurred?; (iii) When was A. coluzzii introduced into STP? and (iv) Is there ongoing, contemporary gene flow into STP from mainland populations? Phylogenetic analysis and haplotype networks were constructed from sequences of 345 A. coluzzii from STP, and 107 individuals from 10 countries on or near the west coast of Africa. Analysis of these data suggest that there have been two introductions of A. coluzzii onto the island of São Tomé that occurred roughly 500 years ago and that these originated from mainland West Africa. It appears that A. coluzzii has never been introduced into Príncipe Island directly from mainland Africa, but there have been at least four introductions originating from São Tomé. Our findings provide further support for the notion that contemporary populations of A. coluzzii on São Tomé and Príncipe are genetically isolated from mainland populations of this mosquito species.
Introduction
Malaria is a life-threatening disease caused by parasites in the genus Plasmodium, that in 2020 resulted in an estimated 627,000 deaths, 80% were in children under 5 years old (1). Sub-Saharan Africa continues to account for about 95% of malaria cases and 94% of deaths that occur annually worldwide (1). Current strategies to eliminate malaria greatly rely on vector control methods, including insecticide-treated mosquito nets (ITNs) and indoor residual spraying (IRS) (2). However, modelling studies have shown that these intervention methods are insufficient for malaria elimination (3, 4), and that development of innovative approaches are urgently needed (5–8). One of the emerging control methods under development is genetically engineered mosquitoes (GEMs) with gene drive for population modification or suppression (9, 10).
Strategies based on GEMs are designed to be high-impact, cost-effective, sustainable, and when integrated with ongoing malaria programs, can lead to malaria elimination (11). The World Health Organization (WHO) Guidance Framework for evaluating GEMs describes a phased process for testing and regulating their use (12). Ecologically confined field-testing sites are considered for the 2nd phase which proceeds confined laboratory and insectary testing (phase 1) and precedes the final phase of open-field deployment. In this context, physical islands have been suggested as ideal sites for phase 2 field trials (13, 14). More specifically, oceanic islands have been considered the best option due to their relatively small size, geographic and genetic isolation, and low genetic complexity of resident mosquito populations (15).
The islands of São Tomé and Príncipe (STP) constitute an African nation located in the Gulf of Guinea (Central Africa), about 250 and 225 km, respectively, off the coast of Gabon, the nearest continental landfall. There are only two species of anopheline mosquitoes in these islands: Anopheles coluzzii, which is a main malaria vector in West and Central Africa; and Anopheles coustani a species whose role as a malaria vector is unclear (16). Several previously published studies suggest genetic isolation between A. coluzzii populations in STP and the mainland (17–21). Here we analyzed A. coluzzii mitogenomes from specimens collected in STP and neighboring continental countries. The objectives of the work were to use haplotype profiles of A. coluzzii to determine: i) the ancestral origin of island populations; ii) the number of introductions, iii) an estimation of when they occurred, and iv) the level of ongoing, contemporary gene flow into the islands.
Materials and Methods
Sample Collection and DNA Extraction
Mitogenome analysis included both newly acquired and sequenced samples of A. coluzzii from São Tomé and Príncipe islands, and publicly available data generated previously for this species (Figure 1; Table S1). The former sample set included immature stages of A. coluzzii collected from a total of 73 breeding sites in STP (38 localities on São Tomé and 35 on Príncipe) (Table S1). The latter sample set was comprised of A. coluzzii from the UC-Davis Vector Genetics Laboratory (VGL) archive (21) or from the Ag1000G database, phase 2 (22). These samples originated from STP (São Tomé, N=14; Príncipe, N=15) and 10 countries in West and Central Africa: Angola (N=15), Benin (N=10), Burkina Faso (N=15), Cameroon (N=9), Côte d’Ivoire (N=15), Equatorial Guinea (here after Bioko Island, N=5), Gabon (N=5), Ghana (N=15), Guinea (N=4), Mali (N=14).
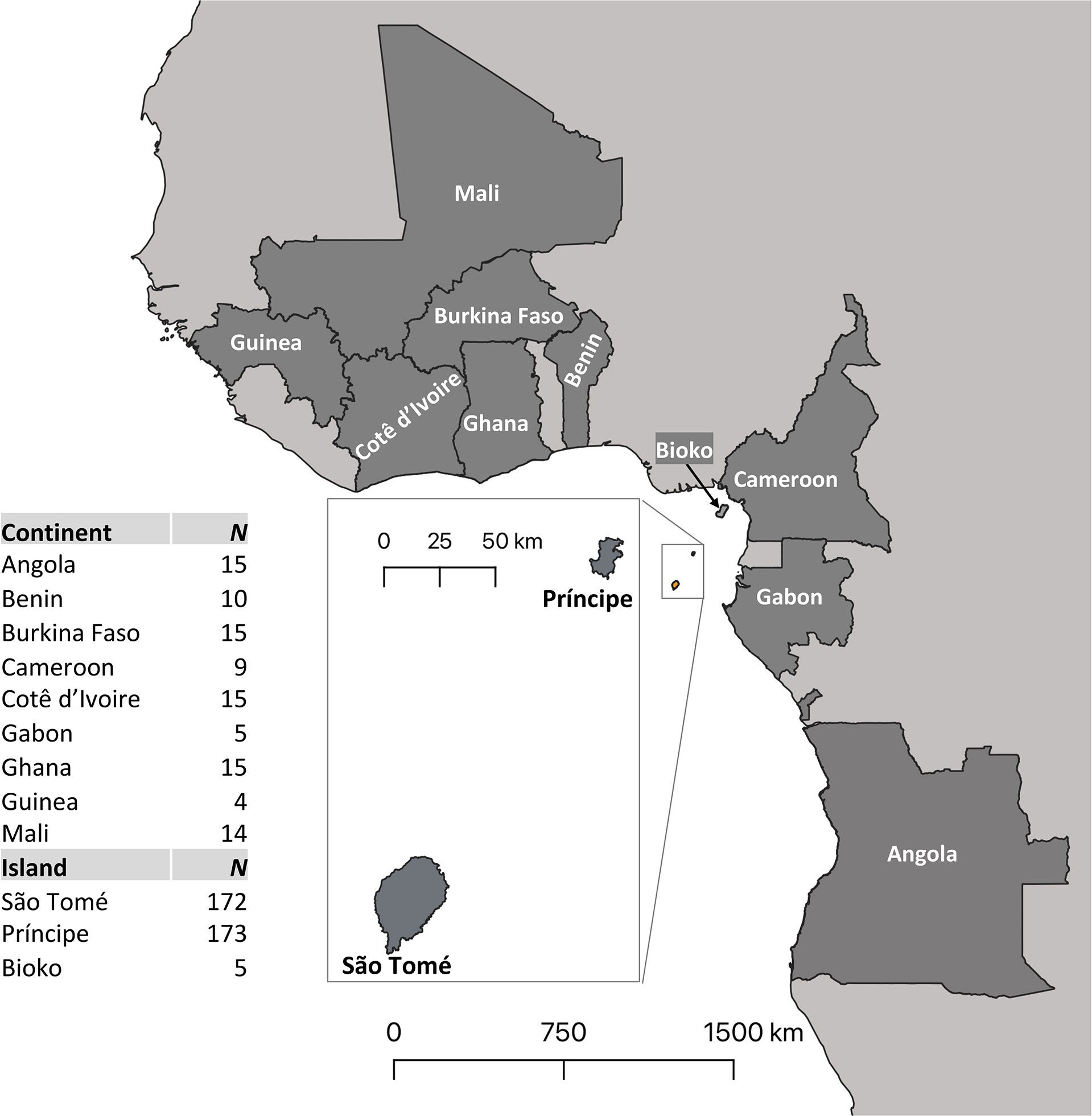
Figure 1 Sampling locations and sample sizes. In addition to São Tomé and Príncipe, Anopheles coluzzii samples originating from nine mainland African countries (Angola, Benin, Burkina Faso, Cameroon, Cotê d’Ivoire, Gabon, Ghana, Guinea and Mali) were used in this study. Samples from the island of Bioko (Equatorial Guinea) were also included. The insert map shows São Tomé and Príncipe islands. Table on the left displays the sample size (N) for each population.
Individual mosquito genomic DNA from specimens taken from the VGL sample archive were extracted using a Qiagen Biosprint following our established protocol (23). Species diagnostics was performed using species-specific SNPs included in the DIS assay (24). The methods used for DNA extraction, species identification and genome sequencing of individuals from Ag1000G were as described by The Anopheles gambiae 1000 Genomes Consortium (22).
Mitogenome Sequencing and Assembly
Individual mosquito DNA from the VGL samples was measured using a dsDNA high sensitivity assay kit on a Qubit instrument (Thermo Fisher Scientific, Waltham, MA, USA). Individual library preparation was done with 10 ng of genomic DNA as input and using KAPA HyperPlus Kit (Roche Sequencing Solutions, Indianapolis, Indiana, USA) following our protocol (25). AMPure SPRI beads (Beckman Coulter Life Sciences, Indianapolis, Indiana, USA) were used for library size selection and clean-up. Sequencing was performed on an Illumina HiSeq 4000 instrument at the UC Davis DNA Technologies Core facility for a pooled library of equal amounts of individual indexed libraries.
After demultiplex and adapter removal, raw-sequencing reads were used to assemble the mitochondria contig using NOVOPlasty version 2.6.7 (26) and 33 as K-mer’s value and default settings. Samples from the Ag1000G were downloaded as binary alignment map (BAM) files from the European Nucleotide Archive (ENA) under accession number PRJEB36277. BAM files were converted to FastQ format using BEDTools (27) which were then used for mitochondria assembly as described above.
Data Analysis
De novo assembled mitochondrial genomes were imported into Geneious (2021.1.1) and aligned with a reference mitogenome sequence of Anopheles coluzzii (JADFTP010000004.1) acquired from GenBank. The alignment was visually inspected to confirm that no missing data, singletons or polymorphism/ambiguities were present in the sequences. Genetic diversity indices such as nucleotide diversity (π) and haplotype diversity (Hd) were assessed for each population using DnaSP 6.12.03 (28).
Phylogenetic Analysis
The annotated mtGenome of A. gambiae (NCBI Ref. Seq. NC_002084.1) was used to partition the aligned mitogenomes. We conducted a partition test of heterogeneity to determine if the complete mtGenome was appropriate to use for phylogenetic analysis, as implemented in PartitionFinder 2.7.1 (29). The models of evolution that best fit each partition were determined by PartitionFinder 2.7.1 (Table S1). The A. coluzzii sequence alignment was aligned with mitogenome sequences of A. melas (KT382823.1), A. arabiensis (KT382816.1), A. gambiae (NC_002084.1) (Table S1) to use as additional outgroups for phylogenetic analyses using MAFFT v7.450 (30, 31).
A maximum likelihood (ML) analysis was conducted using IQ-TREE v2.1.3 (32) to estimate a ML tree with 1,000 ultrafast bootstrap replicates and resampled partitions and then sites within resampled partitions (32–36). Likelihood settings followed the general time reversible model (GTR) with a gamma distribution and estimates of the proportion of invariable sites (+I+G). Confidence in the resulting topologies was assessed using rapid bootstrapping and a search for the best-scoring tree with 1,000 replicates. We performed Bayesian inference (BI) analyses using parameters selected by PartitionFinder 2.7.1 (37) and conducted in MrBayes 3.2.7a (38). An MCMC algorithm ran for 100,000,000 generations, sampling one tree every 10,000 generations. Likelihood scores were used to determine the value of burn-in and stationary distributions. When split frequency in the Bayesian analyses reached <0.01, a 50% majority-rule consensus tree was obtained from the remaining trees. Posterior probabilities for clades were compared for congruence between analyses, bootstrap values ML > 70 and BI > 0.90 are presented on the phylograms.
Haplotype Networks and Migration Patterns
Haplotypes were identified and corrected using Poppr 2.0 and DnaSP 6.12.03 (39). An initial TCS haplotype network was constructed using POPArt 1.7 (40). Mainland Africa samples that differed by >25 mutations from individuals from São Tomé and Príncipe were removed and a second haplotype network constructed. A third haplotype network including only individuals from São Tomé and Príncipe Islands was constructed to identify the number of haplotypes and their geographic distribution.
Patterns of migration between mainland Africa and STP were investigated using a Bayesian approach compiled in Migrate-n v. 4.4.3 (41). Parameters were estimated under a full migration model that allowed gene flow to occur among STP and their most recent common ancestral populations. Migrate-n analyses were conducted using default parameters with two independent runs sampling every 200 steps for 200,000 recorded steps and a burn-in of 20,000. The migration models were compared based on their marginal likelihood and probability using thermodynamic integration with Bezier approximation as implemented in Migrate-n (42). Visualization of the migration pattern inferred by Migrate-n analysis was constructed using the Migest Package in R (43).
Divergence Time Estimates
In order to create a mitogenome-based timeline for the introduction of A. coluzzii onto STP, as reflected in their mitogenome sequences, additional phylogenetic trees were estimated. For this purpose, a multi-locus coalescent model was applied to the data using the software BEAST2 [BEAST 2.5; (44)]. Intraspecific divergence times were concurrently estimated using a substitution rate of 1.2 x 10-8 mutations per site per year and assuming a generation time of three weeks (45). BEAST2 analyses were performed in triplicate at the University of California – Davis Genome Center High Performance Computing cluster with Markov chains run for 100 million generations or until convergence, with 10 million generations of each run discarded as burn in and chains sampled every 10,000 generations. Based on the results from PartitionFinder 2.7.1 the HKY substitution model was used in combination with gamma site-specific rate variation and a proportion of invariant sites parameters with a relaxed log normal molecular clock. A strict clock was selected over a relaxed clock comparing the likelihood of the results of 10,000,000 generation test runs. Tracer v1.7.1 (46) was used to assess convergence. LogCombiner (47) was used to resample 10000 subtrees from BEAST2 analyses and then TreeAnnotator (44) was used to generate Maximum Clade Credibility (MCC) trees (44).
Results
Mitogenome Assemblies and Genetic Diversity
A total of 456 mitochondrial genomes were used to investigate the origin of A. coluzzii in São Tomé and Príncipe islands. New complete A. coluzzii mitochondrial genome sequences were generated for 158 individuals from São Tomé and 158 individuals from Príncipe. After multi-alignment, variable sites were identified in each sequence. São Tomé and Príncipe presented the lowest number of polymorphisms and nucleotide diversity estimates, despite being the largest sampling dataset (Table 1). Haplotype diversity ranged from 0.134 to 1, in which the Príncipe population had the lowest value (Table 1).
Phylogenetics
This combined phylogenetic analysis (Figure S1A) represents 414 terminals from the genus Anopheles, representing four species (A. melas, A. arabiensis, A. gambiae and A. coluzzii) and 12 western African (WA) sites (Angola, Benin, Bioko, Burkina Faso, Cameroon, Cotê d’Ivoire, Gabon, Ghana, Guinea, Mali, São Tomé, and Príncipe), and includes at least one representative of every unique haplotype (Figure S1B).
All relationships within the mitogenome phylogeny were resolved with significant support using ML and Bayesian analyses (ML > 70 and BI > 0.90; Figure 2). Anopheles arabiensis is recovered as the earliest branching lineage and is a sister clade to A. gambiae + A. coluzzii (Figure 2). The A. coluzzii reference sequence (JADFTP010000004.1) is recovered as the earliest branching lineage within A. coluzzii and is sister to five WA clades. Individuals from São Tomé and Príncipe are recovered as a monophyletic clade and are sister to individuals from only the northwestern region of West Africa (NW: Benin, Burkina Faso, Cotê d’Ivoire, Ghana, Guinea, and Mali) with strong support (Figures 2 and S1).
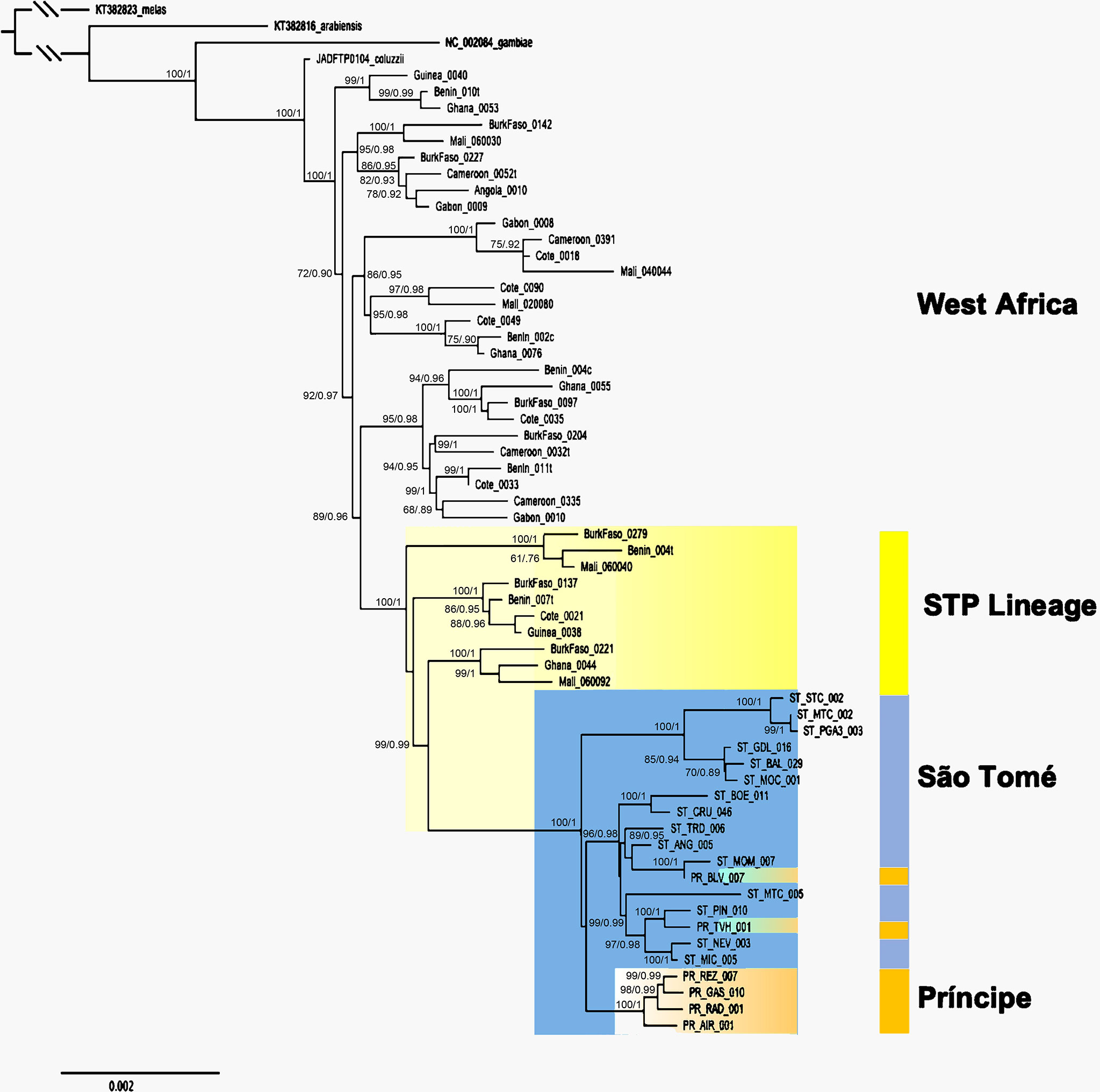
Figure 2 Phylogram of Anopheles coluzzii using complete mitogenome of a subset of individuals and only unique sequences. Values > 0.7 for ML and 90% for BI are shown and represented by percentages. Gray highlighted clades with mixed geographic localities from mainland West Africa (WA). A. coluzzii individuals from WA that share a most recent common ancestor with individuals from São Tomé and Príncipe are highlighted in yellow. Distinct clades containing individuals from São Tomé (in blue) and Príncipe (in orange). Phylogram including the complete dataset is presented in Figure S1.
Haplotype Network and Migration
Individuals from Central Africa (Angola, Bioko, Cameroon, Gabon) form an unresolved cluster from haplotypes within the network, with northern West African (NW) haplotypes appearing as the closest relatives of haplotypes present in STP (Figure S1). No shared haplotype between any west African country and STP were identified (Figures 3A and S1). São Tomé and Príncipe haplotypes are separated from the closest mainland haplotypes by a least 11 mutations (Figure S1). We identified 5 unique haplotypes present in São Tomé (ST-1, 2, 3, 5 and 6), two unique haplotypes in Príncipe (PR-1and 2) and three shared haplotypes between the two islands (Figure 3A). We found no geographic substructure of haplotypes within either island. Migrate-n analysis indicates similar migration estimates of A. coluzzii among the west African populations in the countries of Benin, Burkina Faso, Cotê d’Ivoire, Ghana, Guinea, and Mali, and from these populations to those in São Tomé (Figure 4). This analysis also indicates migration from São Tomé to Príncipe, with an insignificant chance of migration in the opposite direction (from Príncipe to São Tomé). Migration from both islands to any of continental populations also appears highly unlikely (Figure 4).
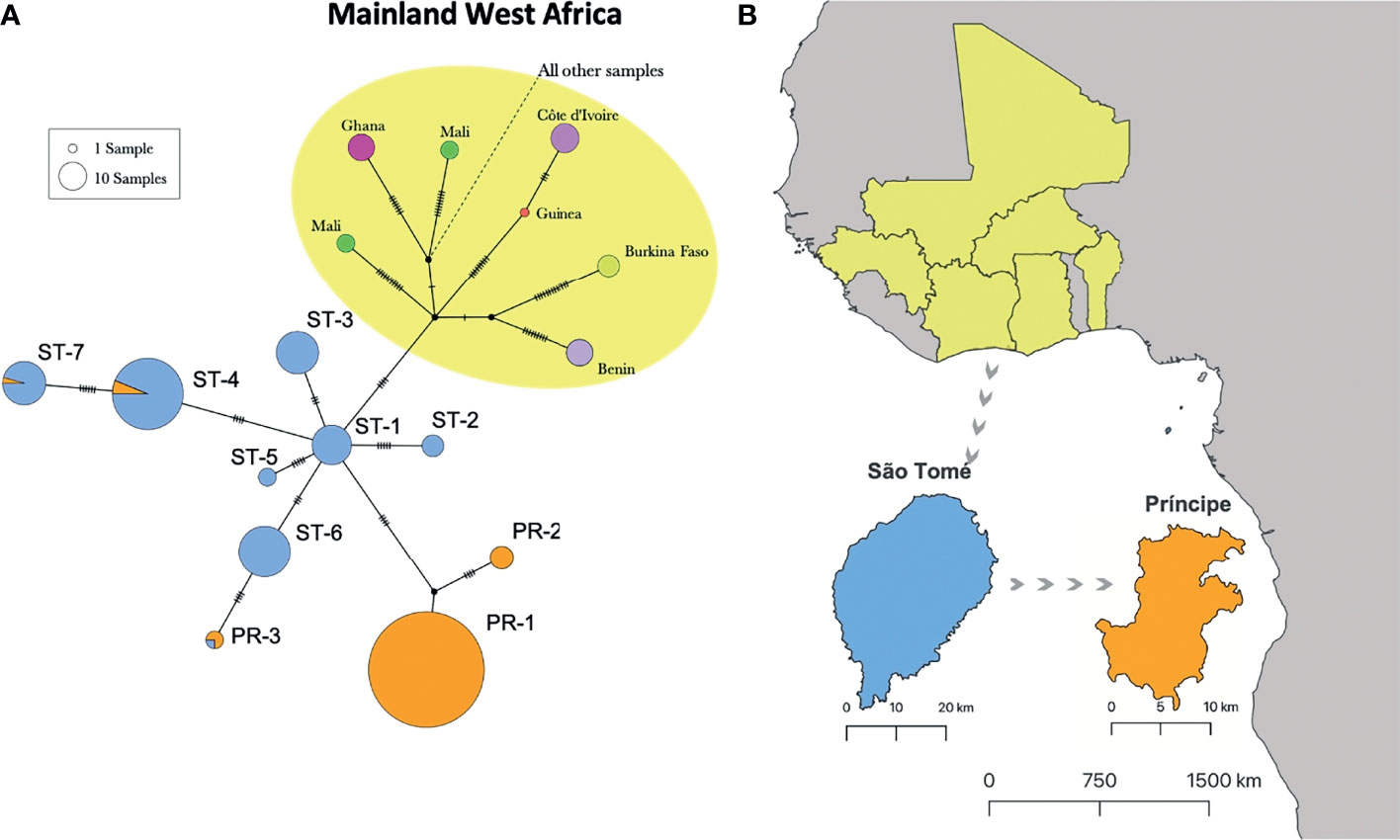
Figure 3 Haplotype network of A. coluzzii from São Tomé and Príncipe (STP) and their West Africa ancestral lineage. (A) Haplotype network with individuals from São Tomé (blue) and Príncipe (orange), and individuals from mainland West Africa (yellow shade) that share a recent common ancestor with STP individuals. Haplotypes labeled ST-1 to ST-7 are mainly found in São Tomé Island. Haplotype labeled PR-1 to PR-3 are found in Príncipe Island. (B) Map highlighting the geographic locations of STP ancestral populations. Arrows indicate direction of A. coluzzii introduction to São Tomé Island from the continent, and to Príncipe Island from São Tomé Island. Complete haplotype network is included in Figure S1.
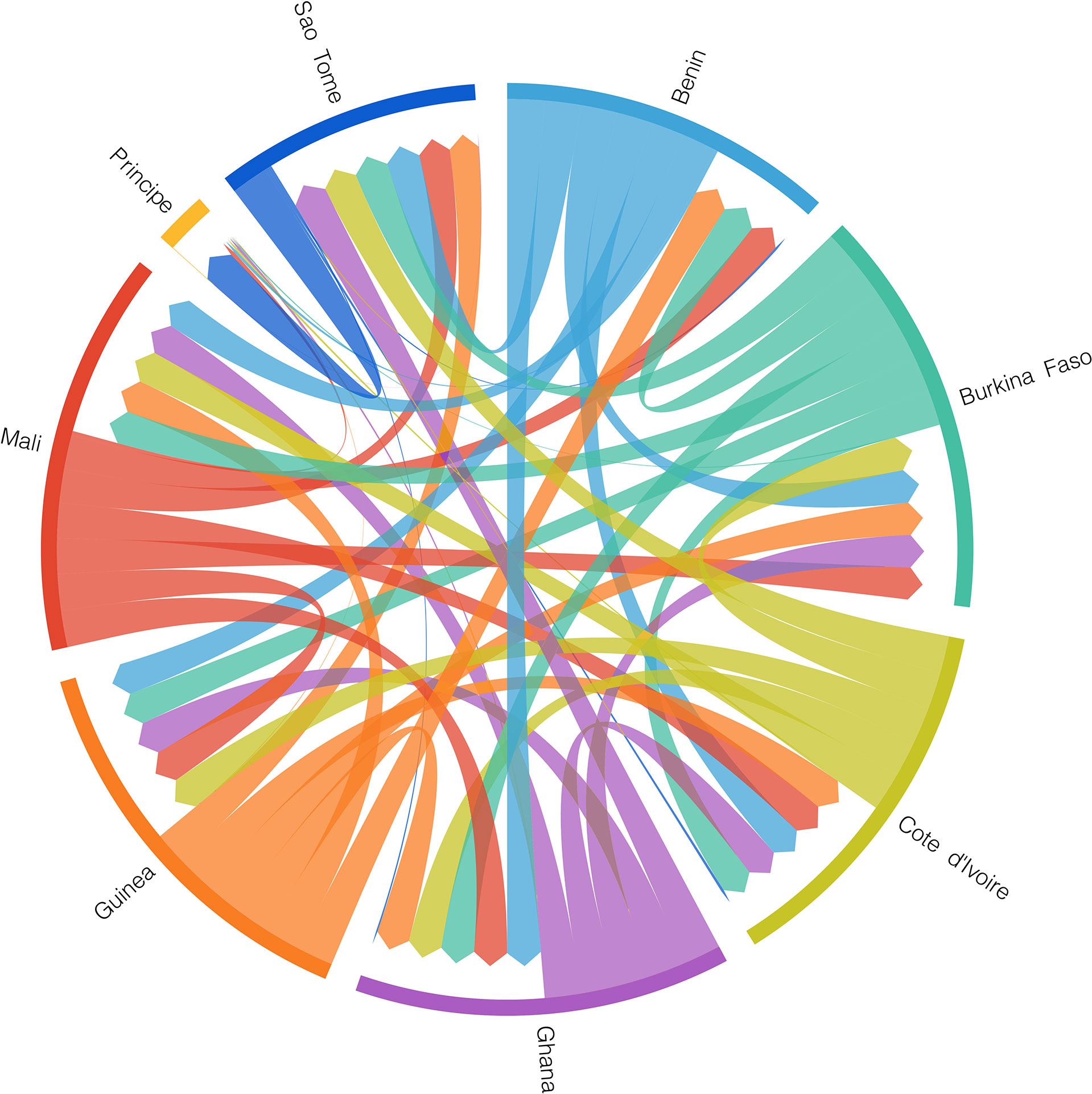
Figure 4 Migration pattern of A. coluzzii populations using Migrate-n analysis. The color of each arrow corresponds to the color of the source population locality listed outside the circle. The width of each stripe is proportional to the estimated number of migrants.
Divergence Time Estimate
We identified a well-supported phylogeny generated by BEAST2 that is concordant with the ML and BI mitogenomic phylogenetic trees. The final BEAST2 trees support a recent divergence of the São Tomé and Príncipe populations from ancestral West African populations (Figures 3B and 5). Divergence time estimates reveal that São Tomé and Príncipe populations began differentiating from West African populations around 500 years before present (Figure 5). Our BEAST2 trees suggest that Príncipe populations represent a contemporary divergence from São Tomé. Furthermore, the BEAST2 trees support at least two introductions of A. coluzzii into São Tomé and at least four introductions from there to Príncipe (Figure 5).
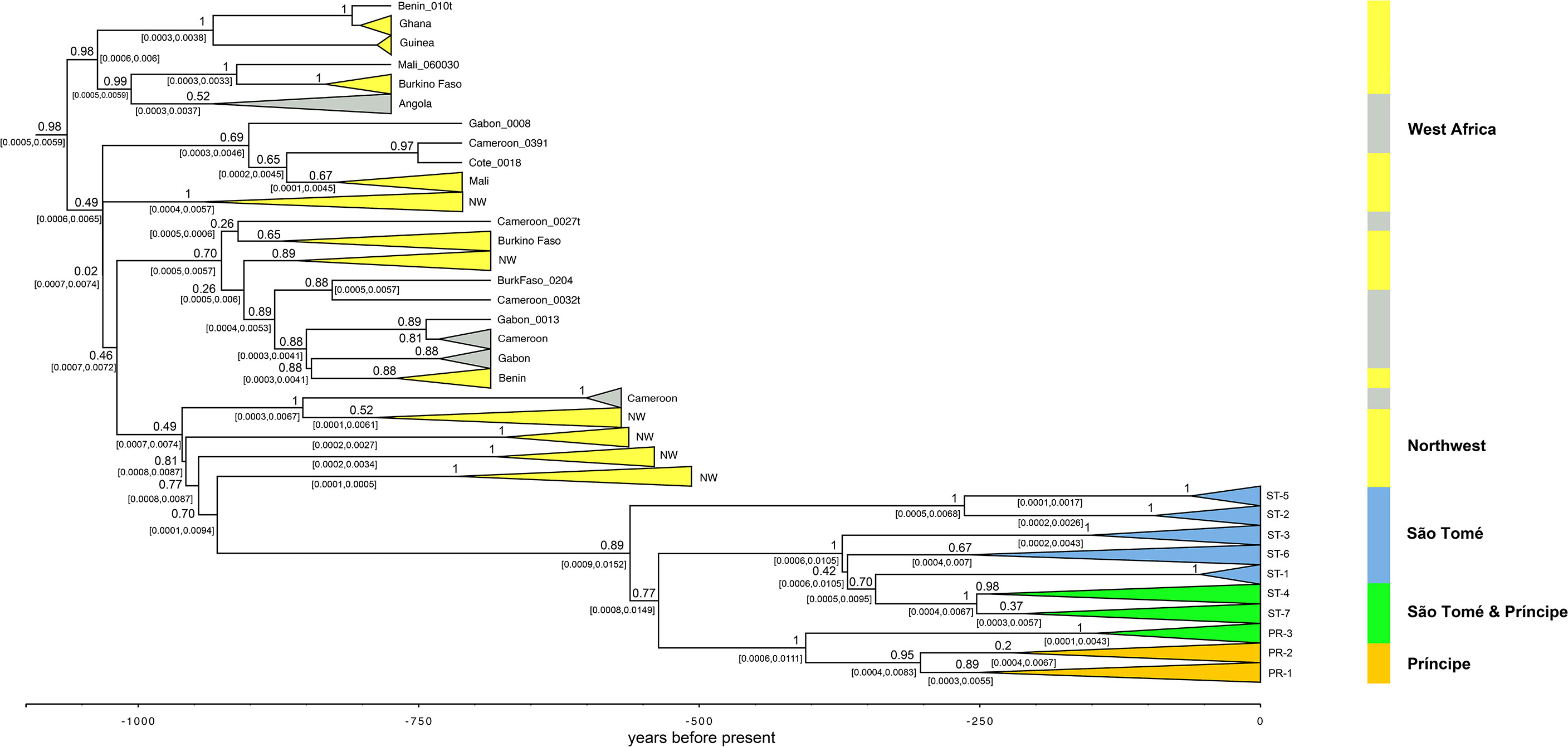
Figure 5 Divergence time estimates (x axis in years before present) of A. coluzzii populations from São Tomé, Príncipe and West & Central Africa (calculated with a complete mitogenome coalescent model in *BEAST; outgroups not shown). Labels from São Tome and Príncipe correspond to haplotypes in Figure 2. Grey highlighted terminals indicate samples from Central Africa; yellow highlighted terminals indicate samples from West Africa; blue highlighted terminals indicate individuals from São Tomé; green highlighted terminals indicate clades found in individuals from São Tomé and Príncipe; orange highlighted terminals indicate samples exclusively from Príncipe. Numbers on branches are the posterior probability values and the numbers within brackets are the 95% HPC intervals.
Discussion
Origin of A. coluzzii in São Tomé and Príncipe
Our phylogenetic analyses and haplotype networks using whole mitochondrial sequences support a West African (Mali, Burkina Faso, Côte d’Ivoire, Guinea, Ghana, Benin) ancestry of A. coluzzii on the islands. Similarly, a population genetic study has presented the smallest FST values between STP populations and West African populations from Ghana, Senegal and Côte d’Ivoire using a region of the mitochondrial NADH dehydrogenase subunit 5 (ND5) gene (19). Discordant results were found using nuclear sequences that suggested the population of A. coluzzii in Angola and Central Africa (Bioko, Cameroon, and Gabon) as the genetically closest populations to STP (19, 21). The biology of mitochondrial and nuclear DNA (nDNA) includes fundamental differences such as ploidy, degree of recombination, number of introns, effective population size and mutation rate (48, 49). For A. coluzzii, it is a comparison between the 15,000 base pair mitochondrial genome and 278 million base pair nuclear genome (50). However, mtDNA has been extensively used to evaluate ancestry and demographic changes in populations (51, 52).
Our analyses suggest that A. coluzzii was introduced onto the island of São Tomé from West Africa on at most two occasions. Historical information suggests this introduction occurred during the first major wave of human migration associated with the Portuguese colonization and subsequent slave trade supporting sugar production around 1500-1600AD (53). The introduction of malaria into São Tomé reported in 1493 (54) supports the presence of the vector at around that time. Our results also suggest that A. coluzzii was only introduced to Príncipe Island from São Tomé four or possibly five times. Of critical importance, we also found no evidence of contemporary genetic exchange between STP and mainland Africa, or between São Tomé and Príncipe which supports the suitability of STP for early field trials of genetically engineered mosquitoes for malaria elimination from the islands.
Haplotype Diversity Among A. coluzzii Populations in São Tomé and Príncipe
Within the island of São Tomé, we identified five unique haplotypes (ST-1, 2, 3, 5 and 6) which appear to be diverged from a single ancestral lineage, designated lineage ST-1, (Figure 3A). On Príncipe we identified 2 unique haplotypes that likely originated on São Tomé from one to two introductions (Figure 3A). We also observed three shared haplotypes between the islands that, combined with our bidirectional migration analysis, likely diverged on São Tomé and were subsequently introduced to Príncipe (Figures 3A; 4). The presence of multiple haplotypes on each island suggests that at some point populations were isolated. However, the lack of geographic substructure in haplotype distributions suggests that contemporary gene flow among subpopulations is occurring within each island. The small number of haplotypes (Figure 3A) and absence haplotype divergence among subpopulations within each island agrees with a very recent colonization of STP, resulting in the observed population bottleneck (founder effect) of A. coluzzii in STP (21).
As geographic distance from a source population increases, overall genetic diversity decreases, resulting in fewer observed mtDNA haplotypes. This can be the result of colonization events on islands causing population bottlenecks (founder effect) (21, 55). The founder effect is amplified as the number of islands within a chain increases, because colonization typically occurs in a stepwise pattern moving away from the island first colonized, resulting in multiple population bottlenecks. The presence of a greater number of haplotypes (8 vs 5) on the larger of the two islands (São Tomé vs Príncipe) fits within island biogeography theory and lends further support to São Tomé as the initial island of A. coluzzii introduction (56). The results reported here provide additional evidence that populations of the malaria vector A. coluzzii are genetically isolated from mainland conspecific populations. In addition, our results suggest that introductions of this species into STP are rare events and that there is little or no contemporary dispersal of this species into the islands from mainland populations.
Data Availability Statement
The datasets presented in this study can be found in online repositories. The names of the repository/repositories and accession number(s) can be found below: https://www.ncbi.nlm.nih.gov/, PRJNA779397.
Author Contributions
MC designed research, analyzed data, wrote the paper. RD designed research, analyzed data, wrote the paper. AC coordinated field collection. JP coordinated field collection. GL designed research, wrote the paper. All authors contributed to the article and approved the submitted version.
Funding
This work was supported by grants from the UC Irvine Malaria Initiative Program and Open Philanthropy.
Conflict of Interest
The authors declare that the research was conducted in the absence of any commercial or financial relationships that could be construed as a potential conflict of interest.
Publisher’s Note
All claims expressed in this article are solely those of the authors and do not necessarily represent those of their affiliated organizations, or those of the publisher, the editors and the reviewers. Any product that may be evaluated in this article, or claim that may be made by its manufacturer, is not guaranteed or endorsed by the publisher.
Acknowledgments
We thank National Malaria Control Program personnel from São Tomé and Príncipe and, the Ministry of Health in São Tomé and Príncipe who facilitated our field collections in São Tomé.
Supplementary Material
The Supplementary Material for this article can be found online at: https://www.frontiersin.org/articles/10.3389/fitd.2022.855272/full#supplementary-material
Supplementary Figure 1 | Results of analyses that include all 456 Anopheles mitogenomes from western Africa (A) haplotype network (B) Maximum Likelihood phylogram with at least one representative of each haplotype. Values > 0.7 for ML are shown.
References
1. World Health Organization. World Malaria Report 2021. Geneva: World Health Organization (2021). Licence: CC BY-NC-SA 3.0 IGO.
3. Griffin JT, Hollingsworth TD, Okell LC, Churcher TS, White M, Hinsley W, et al. Reducing Plasmodium Falciparum Malaria Transmission in Africa: A Model-Based Evaluation of Intervention Strategies. PloS Med (2010) 7:e1000324. doi: 10.1371/journal.pmed.1000324
4. Walker PG, Griffin JT, Ferguson NM, Ghani AC. Estimating the Most Efficient Allocation of Interventions to Achieve Reductions in Plasmodium Falciparum Malaria Burden and Transmission in Africa: A Modelling Study. Lancet Glob Health (2016) 4:e474–84. doi: 10.1016/S2214-109X(16)30073-0
5. Gantz VM, Jasinskiene N, Tatarenkova O, Fazekas A, Macias VM, Bier E, et al. Highly Efficient Cas9-Mediated Gene Drive for Population Modification of the Malaria Vector Mosquito Anopheles Stephensi. Proc Natl Acad Sci U S A (2015) 112:E6736–43. doi: 10.1073/pnas.1521077112
6. Hammond A, Galizi R, Kyrou K, Simoni A, Siniscalchi C, Katsanos D, et al. A CRISPR-Cas9 Gene Drive System Targeting Female Reproduction in the Malaria Mosquito Vector Anopheles Gambiae. Nat Biotechnol (2016) 34(1):78–83. doi: 10.1038/nbt.3439
7. Kyrou K, Hammond AM, Galizi R, Kranjc N, Burt A, Beaghton AK, et al. A CRIS PR–Cas9 Gene Drive Targeting Doublesex Causes Complete Population Suppression in Caged Anopheles Gambiae Mosquitoes. Nat Biotech (2018) 36:1062. doi: 10.1038/nbt.4245
8. Macias VM, McKeand S, Chaverra-Rodriguez D, Hughes GL, Fazekas A, Pujhari S, et al. Cas9-Mediated Gene-Editing in the Malaria Mosquito Anopheles Stephensi by ReMOT Control. G3 (Bethesda) (2020) 10:1353–60. doi: 10.1534/g3.120.401133
9. Carballar-Lejarazú R, James AA. Population Modification of Anopheline Species to Control Malaria Transmission. Pathog Glob Health (2017) 111:424–35. doi: 10.1080/20477724.2018.1427192
10. Simoni A, Hammond AM, Beaghton AK, Galizi R, Taxiarchi C, Kyrou K, et al. A Male-Biased Sex-Distorter Gene Drive for the Human Malaria Vector Anopheles Gambiae. Nat Biotechnol (2020) 38:1054–60. doi: 10.1038/s41587-020-0508-1
11. James S, Collins FH, Welkhoff PA, Emerson C, Godfray HCJ, Gottlieb M, et al. Pathway to Deployment of Gene Drive Mosquitoes as a Potential Biocontrol Tool for Elimination of Malaria in Sub-Saharan Africa: Recommendations of a Scientific Working Group†. Am J Trop Med Hyg (2018) 98(6_Suppl):1–49. doi: 10.4269/ajtmh.18-0083
12. WHO/TDR, FNIH. The Guidance Framework for Testing Genetically Modified Mosquitoes [Framework] (2014). Available at: https://www.who.int/tdr/publications/year/2014/Guidance_frame_work_mosquitoes.pdf.
13. Scott TW, Takken W, Knols BG, Boëte C. The Ecology of Genetically Modified Mosquitoes. Science (2002) 298:117–9. doi: 10.1126/science.298.5591.117
14. Committee on Gene Drive Research in Non-Human Organisms: Recommendations for Responsible Conduct; Board on Life Sciences; Division on Earth and Life Studies; National Academies of Sciences, Engineering, and Medicine. Gene Drives on the Horizon: Advancing Science, Navigating Uncertainty, and Aligning Research With Public Values. Washington (DC): National Academies Press (US) (2016).
15. Lanzaro GC, Campos M, Crepeau M, Cornel A, Estrada A, Gripkey H, et al. Selection of Sites for Field Trials of Genetically Engineered Mosquitoes With Gene Drive. Evol Appl (2021) 14:2147–61. doi: 10.1111/eva.13283
16. Pinto J, Sousa CA, Gil V, Ferreira C, Gonçalves L, Lopes D, et al. Malaria in São Tomé and Príncipe: Parasite Prevalences and Vector Densities. Acta Trop (2000) 76:185–93. doi: 10.1016/s0001-706x(00)00100-5
17. Pinto J, Donnelly MJ, Sousa CA, Gil V, Ferreira C, Elissa N, et al. Genetic Structure of Anopheles Gambiae (Diptera: Culicidae) in São Tomé and Príncipe (West Africa): Implications for Malaria Control. Mol Ecol (2002) 11:2183–7. doi: 10.1046/j.1365-294x.2002.01587.x
18. Moreno M, Salgueiro P, Vicente JL, Cano J, Berzosa PJ, de Lucio A, et al. Genetic Population Structure of Anopheles gambiae in Equatorial Guinea. Malar J (2007) 6:137. doi: 10.1186/1475-2875-6-137
19. Marshall JC, Pinto J, Charlwood JD, Gentile G, Santolamazza F, Simard F, et al. Exploring the Origin and Degree of Genetic Isolation of Anopheles Gambiae From the Islands of São Tomé and Príncipe, Potential Sites for Testing Transgenic-Based Vector Control. Evol Appl (2008) 1:631–44. doi: 10.1111/j.1752-4571.2008.00048.x
20. Salgueiro P, Moreno M, Simard F, O’Brochta D, Pinto J. New Insights Into the Population Structure of Anopheles Gambiae s.s. In the Gulf of Guinea Islands Revealed by Herves Transposable Elements. PloS One (2013) 8:e62964. doi: 10.1371/journal.pone.0062964
21. Campos M, Hanemaaijer M, Gripkey H, Collier TC, Lee Y, Cornel AJ, et al. The Origin of Island Populations of the African Malaria Mosquito, Anopheles Coluzzii. . Commun Biol (2021) 4:630. doi: 10.1038/s42003-021-02168-0
22. Anopheles gambiae 1000 Genomes Consortium. Genome Variation and Population Structure Among 1142 Mosquitoes of the African Malaria Vector Species Anopheles Gambiae and Anopheles Coluzzii. Genome Res (2020) 30:1533–46. doi: 10.1101/gr.262790.120
23. Nieman CC, Yamasaki Y, Collier TC, Lee Y. A DNA Extraction Protocol for Improved DNA Yield From Individual Mosquitoes. F1000Res (2015) 4:1314. doi: 10.12688/f1000research.7413.1
24. Lee Y, Weakley AM, Nieman CC, Malvick J, Lanzaro GC. A Multi-Detection Assay for Malaria Transmitting Mosquitoes. J Vis Exp (2015) 28:e52385. doi: 10.3791/52385
25. Yamasaki YK, Nieman CC, Chang AN, Collier TC, Main BJ and Lee Y. Improved tools for genomic DNA library construction of small insects [version 1; not peer reviewed]. F1000Research (2016) 211(poster):5. doi: 10.7490/f1000research.1111322.1
26. Dierckxsens N, Mardulyn P, Smits G. NOVOPlasty: De Novo Assembly of Organelle Genomes From Whole Genome Data. Nucleic Acids Res (2017) 45:e18. doi: 10.1093/nar/gkw955
27. Quinlan AR, Hall IM. BEDTools: A Flexible Suite of Utilities for Comparing Genomic Features. Bioinformatics (2010) 26:841–2. doi: 10.1093/bioinformatics/btq033
28. Rozas J, Ferrer-Mata A, Sánchez-Del Barrio JC, Guirao-Rico S, Librado P, Ramos-Onsins S, et al. DnaSP 6: DNA Sequence Polymorphism Analysis of Large Datasets. Mol Biol Evol (2019) 34:3299–302. doi: 10.1093/molbev/msx248
29. Lanfear R, Hua X, Warren DL. Estimating the Effective Sample Size of Tree Topologies From Bayesian Phylogenetic Analyses. Genome Biol Evol (2016) 8(8):2319–32. doi: 10.1093/gbe/evw171
30. Katoh K, Misawa K, Kuma K, Miyata T. MAFFT: A Novel Method for Rapid Multiple Sequence Alignment Based on Fast Fourier Transform. Nucleic Acids Res (2002) 30:3059–66. doi: 10.1093/nar/gkf436
31. Katoh K, Standley DM. MAFFT Multiple Sequence Alignment Software Version 7: Improvements in Performance and Usability. Mol Biol Evol (2013) 30(4):772–80. doi: 10.1093/molbev/mst010
32. Chernomor O, von Haeseler A, Minh BQ. Terrace Aware Data Structure for Phylogenomic Inference From Supermatrices. Syst Biol (2016) 65:997–1008. doi: 10.1093/sysbio/syw037
33. Gadagkar SR, Rosenberg MS, Kumar S. Inferring Species Phylogenies From Multiple Genes: Concatenated Sequence Tree Versus Consensus Gene Tree. J Exp Zool (2005) 304B:64–74. doi: 10.1002/jez.b.21026
34. Seo T-K, Kishino H, Thorne JL. Incorporating Gene-Specific Variation When Inferring and Evaluating Optimal Evolutionary Tree Topologies From Multilocus Sequence Data. Proc Natl Acad Sci USA (2005) 102:4436–41. doi: 10.1073/pnas.0408313102
35. Stamatakis A. Raxml-Vi-Hpc: Maximum Likelihood-Based Phylogenetic Analyses With Thousands of Taxa and Mixed Models. Bioinformatics (2006) 22:2688–90. doi: 10.1093/bioinformatics/btl446
36. Hoang DT, Chernomor O, Haeseler A, Minh BQ, Vinh LS. UFBoot2: Improving the Ultrafast Bootstrap Approximation. Mol Biol Evol (2018) 35:518–22. doi: 10.1093/molbev/msx281
37. Lanfear R, Frandsen PB, Wright AM, Senfeld T, Calcott B. PartitionFinder 2: New Methods for Selecting Partitioned Models of Evolution for Molecular and Morphological Phylogenetic Analyses. Mol Biol Evol (2017) 34:772–3. doi: 10.1093/molbev/msw260
38. Huelsenbeck JP, Ronquist F. MRBAYES: Bayesian Inference of Phylogenetic Trees. Bioinformatics (2001) 17(8):754–5. doi: 10.1093/bioinformatics/17.8.754
39. Kamvar ZN, Tabima JF, Grünwald NJ. Poppr: An R Package for Genetic Analysis of Populations With Clonal, Partially Clonal, and/or Sexual Reproduction. PeerJ (2014) 2:e281. doi: 10.7717/peerj.281
40. Leigh JW, Bryant D. Popart: Full-Feature Software for Haplotype Network Construction. Methods Ecol Evol (2015) 6:1110–6. doi: 10.1111/2041-210X.12410
41. Beerli P, Felsenstein J. Maximum Likelihood Estimation of a Migration Matrix and Effective Population Sizes in N Subpopulations by Using a Coalescente Approach. Proc Natl Acad Sci USA (2001) 98(8):4563–8. doi: 10.1073/pnas.081068098
42. Beerli P, Palczewski M. Unified Framework to Evaluate Panmixia and Migration Direction Among Multiple Sampling Locations. Genetics (2010) 185(1):313–26. doi: 10.1534/genetics.109.112532
43. Abel GJ, Sander N. Quantifying Global International Migration Flows. Science (2014) 343(6178):1520–2. doi: 10.1126/science.1248676
44. Bouckaert R, Vaughan TG, Barido-Sottani J, Duchêne S, Fourment M, Gavryushkina A, et al. BEAST 2.5: An Advanced Software Platform for Bayesian Evolutionary Analysis. PloS Comput Biol (2019) 15:e1006650. doi: 10.1371/journal.pcbi.1006650
45. Jones CM, Lee Y, Kitchen A, Collier T, Pringle JC, Muleba M, et al. Complete Anopheles Funestus Mitogenomes Reveal an Ancient History of Mitochondrial Lineages and Their Distribution in Southern and Central Africa. Sci Rep (2018) 8:9054. doi: 10.1038/s41598-018-27092-y
46. Rambaut A, Drummond AJ, Xie D, Baele G, Suchard MA. Posterior Summarization in Bayesian Phylogenetics Using Tracer 1.7. Syst Biol (2018) 67:901–4. doi: 10.1093/sysbio/syy032
47. Drummond AJ, Rambaut A. BEAST: Bayesian Evolutionary Analysis by Sampling Trees. BMC Evol Biol (2007) 7:214. doi: 10.1186/1471-2148-7-214
49. Ballard JWO, Whitlock MC. The Incomplete Natural History of Mitochondria. Mol Ecol (2004) 13:729–44. doi: 10.1046/j.1365-294X.2003.02063.x
50. Holt RA, Subramanian GM, Halpern A, Sutton GG, Charlab R, Nusskern DR, et al. The Genome Sequence of the Malaria Mosquito Anopheles Gambiae. Science (2002) 298(5591):129–49. doi: 10.1126/science.1076181
52. Avise JC. Mitochondrial DNA Polymorphism and a Connection Between Genetics and Demography of Relevance to Conservation. Conserv Biol (1995) 9:686–90. doi: 10.1046/j.1523-1739.1995.09030686.x
53. Tenreiro F. A Ilha De São Tomé. In: Memórias Da Junta De Investigação do Ultramar 2a Série N°24, Lisboa. Junta de Investigações do Ultramar: Lisbon (1961). p. 279.
54. Baptista JL. Subsidio Para a História do Paludismo Em S. Tomé. Acta Médoca Portuguesa (1996) 9:259–65.
55. Johnson KP, Adler FR, Cherry JL. Genetic and Phylogenetic Consequences of Island Biogeography. Evolution (2007) 54(2):387–96. doi: 10.1111/j.0014-3820.2000.tb00041.x
Keywords: island biogeography, gene-drive, field trial, malaria, population genetics, genomics
Citation: Ditter RE, Campos M, Pinto J, Cornel AJ, Rompão H and Lanzaro GC (2022) Mitogenome Analyses Reveal Limited Introduction of Anopheles coluzzii Into the Central African Islands of São Tomé and Príncipe. Front. Trop. Dis 3:855272. doi: 10.3389/fitd.2022.855272
Received: 15 January 2022; Accepted: 10 March 2022;
Published: 29 April 2022.
Edited by:
Janet Midega, Wellcome Trust (WT), United KingdomReviewed by:
Charles Mbogo, Kenya Medical Research Institute (KEMRI), KenyaMárcio Galvão Pavan, Oswaldo Cruz Foundation (Fiocruz), Brazil
Copyright © 2022 Ditter, Campos, Pinto, Cornel, Rompão and Lanzaro. This is an open-access article distributed under the terms of the Creative Commons Attribution License (CC BY). The use, distribution or reproduction in other forums is permitted, provided the original author(s) and the copyright owner(s) are credited and that the original publication in this journal is cited, in accordance with accepted academic practice. No use, distribution or reproduction is permitted which does not comply with these terms.
*Correspondence: Gregory C. Lanzaro, Z2NsYW56YXJvQHVjZGF2aXMuZWR1
†These authors share first authorship