- 1Department of Biological Sciences, National University of Singapore, Singapore, Singapore
- 2Military Medicine Institute (MMI), Singapore Armed Forces, Singapore, Singapore
- 3Tropical Marine Science Institute, National University of Singapore, Singapore, Singapore
Mosquitoes (Family: Culicidae) are dominant vectors of pathogens, and their surveillance has been incorporated into major disease control programs worldwide. However, routine, species-level identification of mosquitoes is often a bottleneck for management, and Next Generation Sequencing (NGS) platforms and DNA metabarcoding can revolutionize this process. MinION nanopore technologies promise on-site sequencing and rapid sample processing rates ideal for time-sensitive biosurveillance. Here, we benchmark the results of DNA metabarcoding on the MinION against the Illumina MiSeq platform, which is known for its higher sequencing accuracy. We used metazoan COI mini-barcode primers to carry out DNA metabarcoding of mosquito bulk samples caught during a real vector survey, then compared the mosquito species profiles recovered on each sequencing platform. We also tested the influence of using different trap lures, storage methods, and pooling different specimen body parts on the number of species recovered. We report that mosquito species-level identifications were highly congruent between MinION and Illumina (93% overlap). We also find that CO2 gas cylinders outperformed biogenic CO2 sources significantly, by two-fold. Notably, we demonstrated the feasibility of detecting zoonotic reservoirs and pathogen signals from mosquito bulk samples. We present the first use of DNA metabarcoding on the MinION for vector surveillance and discuss future applications.
Introduction
Vector-borne diseases pose significant health and economic challenges. They account for 17% of all infectious diseases globally, resulting in more than 700,000 deaths annually (1). Mosquitoes are vectors for more dreadful arthropod-borne diseases than any other taxon (1–3). Examples include malaria, dengue, chikungunya, Japanese encephalitis, Zika, and West Nile (2, 4). Such mosquito-borne diseases also cause huge economic burdens — for instance, the estimated aggregated global cost of dengue illness was US$ 8.9 billion in 2013 (5).
Despite recent advances in vaccine technologies, vaccines for many mosquito-borne diseases remain unavailable (6). At present, the most significant reductions in disease burden have been attributed to control measures that target mosquito vectors (7, 8). Pre-emptive surveillance and suppression measures have been routinely incorporated into major mosquito control programs and guidelines globally (USA: 9; Australia: 10; Europe: 11; Hong Kong: 12; Singapore: 13; Taiwan: 14).
One of the biggest bottlenecks in routine mosquito surveillance programs is specimen sorting and subsequent taxonomic identification (preferably to species-level resolution). According to mosquito control guidelines, control strategies need to be implemented in response to surveillance results and the most crucial indicators are the presence and abundance of particularly high-risk vector species [USA: (9); Australia: (10); Europe: (11); Hong Kong: (12); Singapore: (13); Taiwan: (14)]. Traditionally, vector surveys involve several components: i) sampling adult mosquitoes using traps like the BG-Sentinel traps, ii) counting the number of individuals (i.e., abundance) in the bulk sample, and most importantly iii) identifying them (preferably) to species-level resolution based on diagnostic morphological characteristics. The last step is particularly time-consuming, tedious, costly, and sometimes made impossible due to several reasons. Firstly, mosquito specimens are tiny, and identification of morphological characteristics under the microscope is often a laborious and time-intensive process (15, 16). Additionally, mosquito scales and setae, which are crucial diagnostic features, are frequently damaged during specimen handling and storage (17–20) thus impeding species identification. Mosquito identification is also limited by the availability and/or quality of published dichotomous taxonomic keys and/or local species checklists (15, 17, 19). Furthermore, in recent years, insect taxonomic expertise is becoming scarce and requires substantial investment in training manpower (21–24). These issues are major hurdles for frequent and routine species-level identification, especially in many tropical countries that suffer from high incidences of mosquito-borne disease transmission and where mosquitoes are also the most biodiverse but understudied (25). Such problems are further exacerbated by climate change and the recent range expansion of invasive yet cryptic mosquito species (26, 27), especially since most non-native species may not be represented in local identification keys.
New molecular methods, such as DNA barcoding, could circumvent several challenges in routine mosquito surveillance (28). Whereas routine specimen identifications in traditional surveillance methods are limited by the quality of samples, processing speed, cost and availability of taxonomic expertise or taxonomic information, a promising alternative could be to rely on DNA barcoding and Next Generation Sequencing (NGS) technologies. DNA barcoding can be done by a non-specialist with general laboratory and bioinformatics skills, and not limited to someone with specific taxonomic knowledge in mosquitoes (24, 29). There are also DNA barcode databases of mosquito species from all around the world, readily available online (such as NCBI GenBank and BOLD Systems), which one could use as references to attain species-level identities for some specimens. However, it is crucial to exercise caution, as misidentified species are prevalent in these databases and it is important to validate DNA barcodes for local mosquito specimens together with morphological documentation (16, 30). Researchers bear the responsibility of diligently checking the publication record of all sequences sourced from such databases. Developing molecular identification tools should rely on a sequence library carefully curated by experts in order to mitigate the risk of misidentifications down the line. The per-specimen cost of DNA barcoding has dropped drastically since the advent of NGS technologies, hence processing specimen-rich samples has become more feasible (29). DNA barcoding can also be applied to damaged specimens without defining diagnostic characters (24, 29).
The same advantages are extended to DNA metabarcoding, which allows the species profile of a bulk sample to be characterized quickly (31). It is also more efficient than DNA barcoding of specimens one-by-one (31), or qPCR which relies on species-specific primers (32). A caveat of DNA metabarcoding is that read abundances can be unreliable to estimators of species abundance due to multiple sources of artifacts and biases (33, 34). However, it can provide a list of species present in each sample (35), which complements mosquito control strategies that are based on responding to the presence/absence of specific mosquito species at a location. At present, DNA metabarcoding of mosquito bulk samples has been tested on NGS platforms like the Illumina platforms, with encouraging results — these studies compare DNA metabarcoding of bulk samples with morphological species identification and report significant overlaps in detected species composition (24, 35–37).
DNA sequencing which employs nanopore technology, such as the MinION (Oxford Nanopore Technologies), represents a relatively recent technological advancement. To date, this technology has not been used with DNA metabarcoding in the vector surveillance context. Using the MinION boasts several advantages over Illumina platforms. For instance, this device, which is the size of a portable USB drive, facilitates on-site sequencing of samples. With real-time sequencing and analysis, the turnaround time for generating DNA sequence data can be substantially reduced (38). In addition, MinION sequencing has also become more affordable in recent years and this enables researchers to perform in-house sequencing studies without relying on external sequencing companies or service providers. This is advantageous since they usually require longer turnaround times for DNA sequencing (from several weeks to months at a time) (39). In the context of biosurveillance, this translates to improved, time-efficient processing of samples, higher sampling frequencies and/or shorter sampling intervals as well as faster implementation of vector control measures. It is important to note that some earlier studies reported higher sequence error rates than Illumina platforms (40, 41). However, such error rates are dropping with newer flow cell iterations and library prep kit chemistries (42–44). Here, we present the first vector surveillance study which employs the novel methodology of combining MinION sequencing and DNA metabarcoding to characterize bulk mosquito samples.
Besides the choice of DNA sequencing platform, there are several components of a mosquito biosurveillance program that often require optimization. For instance, an important consideration is the use of an optimal source of carbon dioxide lure for mosquito traps. Carbon dioxide is a major constituent of vertebrate breath that plays a key role in a mosquito’s host-seeking behavior, hence utilizing CO2 in traps increases the catch rate (45). Microbially generated CO2 such as that by yeast, commonly referred to as biogenic carbon sources, serves as a convenient alternative to CO2 gas cylinders and has been shown to be an effective lure (45). However, to the best of our knowledge, the two have not been directly compared. Another concern in adopting a molecular-based surveillance pipeline, is the requirements for specimen storage and preservation. Traditionally, specimens need to be kept dry to preserve important morphological features (23). It is therefore crucial to avoid liquid preservatives like ethanol until they have been identified and/or the features are no longer needed. This requirement is relaxed in molecular species identification, which does not rely on morphological characteristics, and it remains to be tested if the inclusion of ethanol to preserve DNA in addition to cold storage will improve the species detection rates. Another consideration in DNA metabarcoding is how to prevent specimens with high biomass or in high abundances from obscuring the detection of rare or small specimens, due to random subsampling at various stages of a molecular protocol (46–51). This can occur during PCR amplification (e.g., polymerases randomly ligating to DNA templates), library preparation (e.g., random adaptor ligation), and so on. Tissue types/sizes have been shown to influence the taxon recovery rate in other arthropods (52). The effects of unequal biomass in our samples could be alleviated by standardizing the tissue size pooled for DNA metabarcoding — for instance, by pooling only the heads of each mosquito specimen, since they generally do not vary in biomass across different-sized mosquito specimens.
In this comparative biosurveillance study, we benchmark the results of DNA metabarcoding using MinION against an Illumina sequencing platform. We sampled mosquitoes from an active biosurveillance site in Singapore and used a universal, metazoan COI mini-barcode to compare the mosquito vector detection between sequencing platforms. This endeavor was facilitated by a carefully curated reference library containing individual DNA barcodes of more than 150 locally captured mosquito species. These species were identified by expert taxonomists, which we use as our ‘gold standard’ of reference. Notably, this is the first study demonstrating the use of nanopore technologies and DNA metabarcoding for surveying mosquito vectors in bulk samples. Additionally, we assessed whether the species richness and profile of mosquitoes detected via metabarcoding were influenced by the following factors in a biosurveillance workflow: (A) source of CO2 lures (gas cylinders versus biogenic sources), (B) tissue biomass (specimen heads versus the rest of the body), as well as (C) specimen storage methods (cold storage, with or without ethanol preservative). Our analyses of results from both sequencing platforms revealed a high congruence between the results of Illumina and MinION sequencing, positioning nanopore technologies as a promising tool for mosquito biosurveillance in the future.
Materials and methods
Sample collection and experimental design
We conducted mosquito sampling over five days within a routinely surveyed forest area in Singapore (Supplementary Table 1 and Text S1), in collaboration with the Military Medicine Institute of the Singapore Armed Forces and a biosurveillance company (Figure 1). To test the influence of different sources of CO2 used as trap lures, we used BG-Sentinel traps baited with CO2 released from either sugar-activated yeast in BG-CO2 generator kits (Biogents, Germany) or CO2 gas cylinders (Air Liquide, France). Mosquito traps were left at the collection site overnight, for at least 24 hours. Samples were kept on ice and transported back to the lab for presorting immediately. Non-targets (i.e., taxa that are not mosquitoes) were discarded in this process. To assess the effect of pooling different segments of a specimen, the heads of every mosquito specimen from the same trap were dissected and pooled to create a bulk sample consisting of only heads, while the rest of the specimen was pooled separately to create another bulk sample. To find out the influence of storage conditions, we randomly assigned specimens in each bulk sample to one of two tubes, then one tube had 70% ethanol added to it while the other was kept dry. These samples were collected from forested sites with limited access to freezers colder than 0°C. Due to this limitation, all samples were immediately stored at 0°C upon collection for less than 12hrs, before being transferred to the research facility at the National University of Singapore and stored at -80°C until DNA was extracted. We expected to have 40 mixed or bulk samples in total (5 sampling points × 2 sources of CO2 tested = 10 mosquito traps. 10 traps × 2 different specimen body segments tested × 2 storage conditions tested = 40 bulk samples). However, one mosquito trap was destroyed due to natural causes, resulting in 36 bulk samples only (9 traps×2×2 = 36).
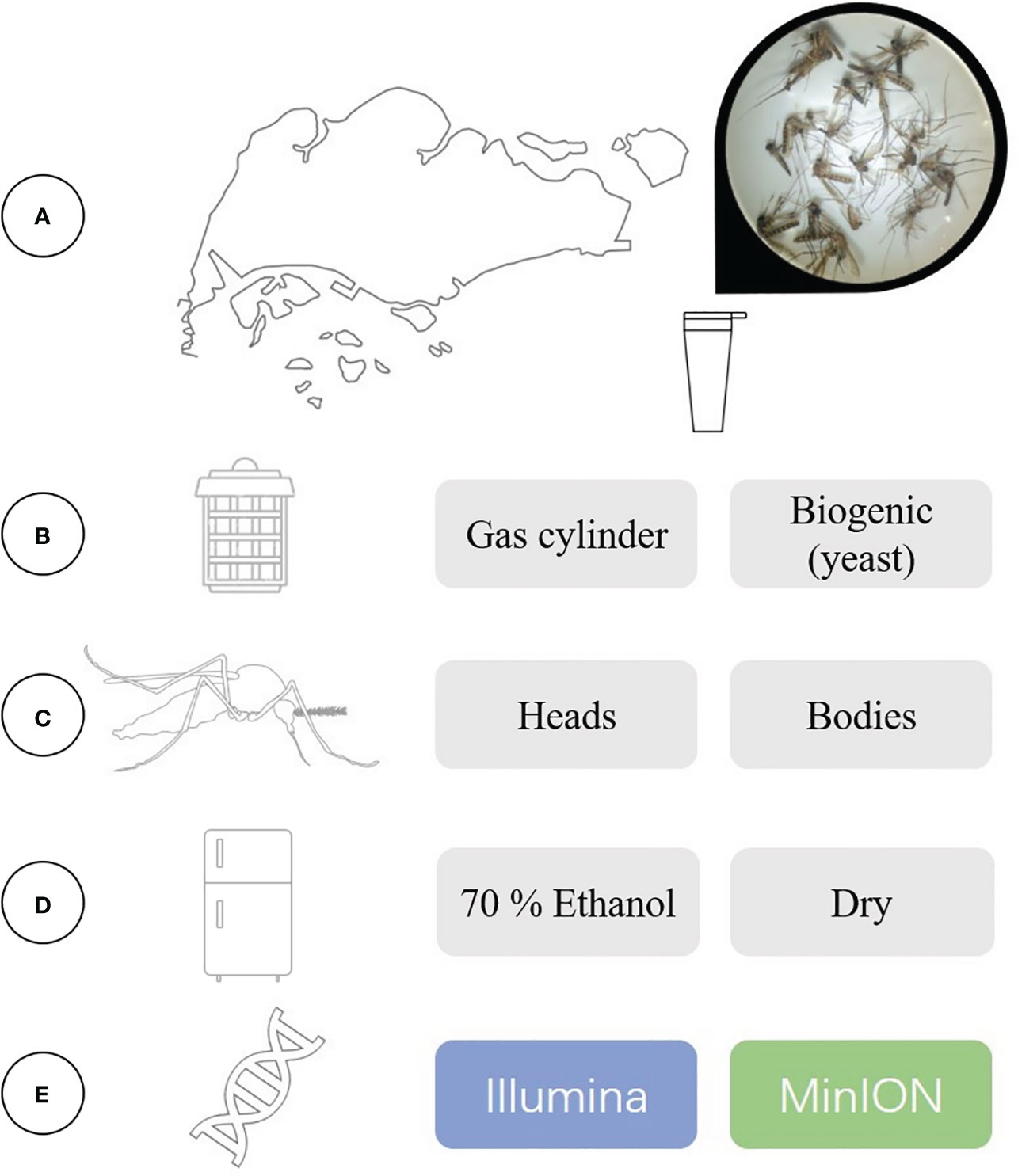
Figure 1 A summary of our experimental set-up and the variables that were evaluated in this study. (A) We trapped and collected mosquitoes at active surveillance sites in Singapore, and assessed the effectiveness of using (B) two different sources of CO2 for trap lures. Bulk mosquito samples were sent for DNA metabarcoding, where we compared the effects of using (C) different specimen body segments to create the bulk sample, (D) different storage methods and (E) different sequencing platforms.
DNA extraction, PCR amplification, and DNA sequencing
Samples were digested using Proteinase K and genomic DNA was extracted with DNeasy® Blood & Tissue Kit (QIAGEN, Germany) following the manufacturer’s instructions. To mitigate the pronounced clogging of filter columns, samples were divided into subsamples before DNA extraction. It is important to emphasize that this measure was taken to streamline the specimen processing workflow, the subsamples were later combined since they are pseudo-replicates. The allocation of specimens to their respective subsample tubes was executed in a randomized manner. Division was performed in such a way that head-only samples were restricted to a maximum of 50 heads, while body-only samples were limited to a maximum of 25 bodies. We employed the QIAcube Connect device (QIAGEN, Germany) for DNA extraction, and this automated system allowed processing in batches of 12 samples. For every batch of 12, a negative control was included, where mosquito specimens were replaced with molecular-grade water. After extraction, the DNA extracts derived from subsamples were combined prior to PCR amplification and sequencing, with one notable exception. In one of our nine traps, the specimen count was higher, comprising 356 specimens, which is approximately 3x more abundant than the next most specimen-rich sample (see Supplementary Table 1). To prevent the potential issue of highly abundant species overwhelming the signals of rare species, each sample from that particular trap was kept as three distinct DNA extracts and processed separately (i.e., instead of 36 bulk samples corresponding to 36 DNA extracts, we had 44 DNA extracts. This is the breakdown: 32 samples × 1 DNA extract + 4 samples × 3 DNA extracts = 44). Data from pseudo-replicate DNA extracts were subsequently merged during data analysis.
All samples were PCR amplified using the universal metazoan primers mICOIintF: 5’- GGWACWGGWTGAACWGTWTAYCCYCC (53) and jgHCO2198: 5’-TAIACYTCIGGRTGICCRAARAAYCA (54), which target the 313bp COI minibarcode. For our detailed PCR protocol, see Text S2. We used tagged primers for each PCR sample, where a unique 13-bp sequence (or tag) is attached to the 5’-end of primers (Supplementary Table 2), to allow for multiplexing large numbers of samples on Next Generation Sequencing platforms (55). For each DNA extract triplicate PCRs were performed, each with uniquely tagged forward and reverse primers. The tags on both the forward and the reverse primers are the same for each PCR sample. For every batch of 96 PCR samples, a negative control using molecular-grade water was included. After amplification, PCR products were equivolume pooled and purified using Ampure XP beads (Beckman Coulter, USA) following manufacturer’s instructions. In order to compare the results of using different sequencing platforms, we homogenized the same pool of cleaned PCR products by pipetting and made two aliquots. One was sequenced on an Illumina MiSeq platform to obtain 250 × 2 paired-end sequences, the other was sequenced on an R10.3 flow cell using a MinION Mk1B (Oxford Nanopore Technologies, UK) and the SQK-LSK112 Ligation Sequencing Kit. MinION Library preparation follows the manufacturer’s protocol, with some modifications (see Text S3 for details).
Bioinformatics
FASTQ files for forward and reverse reads of Illumina sequencing were merged using fastp (56) with a minimum length filter of ≥200bp. Sequences were processed using OBITools v 1.2 (57) and demultiplexed (i.e., assigned to PCR samples) using ngsfilter, allowing for up to 2bp mismatches. Sequences with one read count across all PCR samples were discarded using obigrep. PCR and sequencing errors were identified using obiclean by tagging sequence variants (“internal sequences”) and subsequently discarded. Curated sequences were subjected to a clustering and denoising pipeline using cluster_otus as implemented in USEARCH (58). In this process, chimeric sequences were flagged and discarded. The remaining sequences were assigned to putative species clusters (referred to as molecular operational taxonomic units, or mOTUs) based on a 97% sequence similarity threshold within each cluster. Sequences were retained for further analysis only if there were at least two reads per PCR sample, and if the sequence was present in at least two out of three PCR replicates. Finally, sequences with the same mOTU cluster assignment had read counts combined. The result is a community matrix of mOTUs × samples. To assign mosquito identities to all mOTUs, representative sequences of each mOTU were first queried against an internal DNA barcode library containing DNA barcodes of mosquitoes in the region, using MegaBLAST from BLAST 2.10.0+ (59). This carefully curated library comprises DNA barcodes of more than 150 mosquitoes captured locally and identified by expert taxonomists (15; Yeo et al., unpublished), or collated from regional DNA barcoding projects in the literature (17). We have tested the effectiveness of the reference library in a proof-of-concept experiment (see Text S4 for a brief overview). BLAST matches were filtered using ≥ 250 bp query cover and ≥ 97% identity match for species level mosquito matches. Finally, mOTU sequences were submitted to GBIF’s online search engine (https://www.gbif.org/tools/sequence-id to attain additional identity matches.
We used a simple bioinformatics pipeline for MinION data. FAST5 data was basecalled using Guppy and the r10.3 high accuracy model. The resulting FASTQ files were demultiplexed and underwent quality control processing using the default settings of ONTbarcoder (v 0.1.9) (60). To the internal DNA barcode reference library mentioned earlier, we identified and added all mosquito sequences from the GBIF search results with ≥97% identity match and ≥250 query cover to our Illumina dataset. 44 DNA barcodes from BOLD Systems met these criteria. All MinION sequences were then BLAST matched against this final, curated DNA barcode library. Strong mosquito species matches (≥97% identity match and ≥250 bp query cover) were accepted as positive detections in a PCR sample, provided that they were represented by more than one read. Data for the three PCR replicates per sample were combined. We used statistical modeling to determine the effect of DNA sequencing platforms and other biosurveillance workflow optimizations on the eventual species richness and composition of mosquitoes detected (see Text S5 for details on data analysis).
To assess the potential for pathogens to be detected in DNA metabarcoding data of mosquito bulk samples, demultiplexed but unfiltered Illumina sequences were BLAST matched against the NCBI nt database. We used MegaBLAST to identify the top 10 hits with e-value parameter set at 1e-5, then parsed results using readsidentifier to obtain the best match under the criterion of ≥250 bp query cover and identity match of ≥85%. We chose avian haemosporidians to be screened as the samples were not stored in appropriate conditions for detecting RNA viruses. Additionally, Singapore is a Southeast Asian rest location for multiple flyways (61) resulting in a presence of avian haemosporidians in avian host and mosquito vectors. Thus, the best matches were screened using an adapted protocol (62).
Results and discussion
High congruence between sequencing platforms
We found high congruence in mosquito species richness and profiles (presence/absence) between sequencing platforms, suggesting that the use of MinION for biosurveillance is highly promising. We used a carefully curated reference library comprising DNA barcodes of mosquitoes captured locally and identified by expert taxonomists (15; Yeo et al., unpublished), or collated from regional DNA barcoding projects in the literature (17). The number of species detected per sample was similar (Figure 2D): Statistical models showed that the sequencing platform (MinION or Illumina) was not a significant variable in explaining species richness (p-value=0.453, see Supplementary Table 5) and accounted for only <4% of the model variance (marginal R2 = 0.003, conditional R2 = 0.462; see Supplementary Table 5). The samples sequenced on the Illumina platform exhibited only a marginal increase in the number of identified mosquito species, potentially attributed to its deeper sequencing coverage (Illumina: 46,085 ± 24,237 reads per PCR sample; MinION: 17,486 ± 12,154 reads per PCR sample). However, no mosquito species were consistently missed by either platform (see Supplementary Figure 1). The species profiles were similar between sequencing platforms: PERMANOVA analysis also showed that there were no significant differences (p-value=0.962). On average, the mean overlap between MinION and Illumina sequencing results for all mosquito bulk samples was 93% (Figure 3, see Supplementary Figure 1), which is a lot higher than the findings of Egeter and colleagues (39). In their study, they conducted water environmental DNA (eDNA) metabarcoding, utilizing bivalve-specific primers and sequenced the PCR products on MinION and Illumina platforms, However, they reported a mean sample overlap of only 69%. Despite the differences in sample type and molecular protocols used in their study (eDNA, and taxon-specific primers), they also tested the results of DNA metabarcoding sample mixtures on different sequencing platforms. The most plausible explanation for the observed discrepancy in overlap is that we used a newer MinION flow cell version (10.3) which had lower error rates, compared to Egeter and colleagues (39) (version 9.4). This could have resulted in fewer instances of tag jumping and erroneous sample assignment, and/or erroneous species ID assignment. As newer flow cells and more sophisticated bioinformatic pipelines become available, we expect the agreement between sequencing platforms to increase further. We demonstrate in our study, that using the MinION and a basic pipeline with minimal bioinformatic processing, we can attain very similar results to that of Illumina sequencing. This finding suggests a promising application of MinION bulk sample DNA metabarcoding for mosquito vector surveillance purposes in the future.
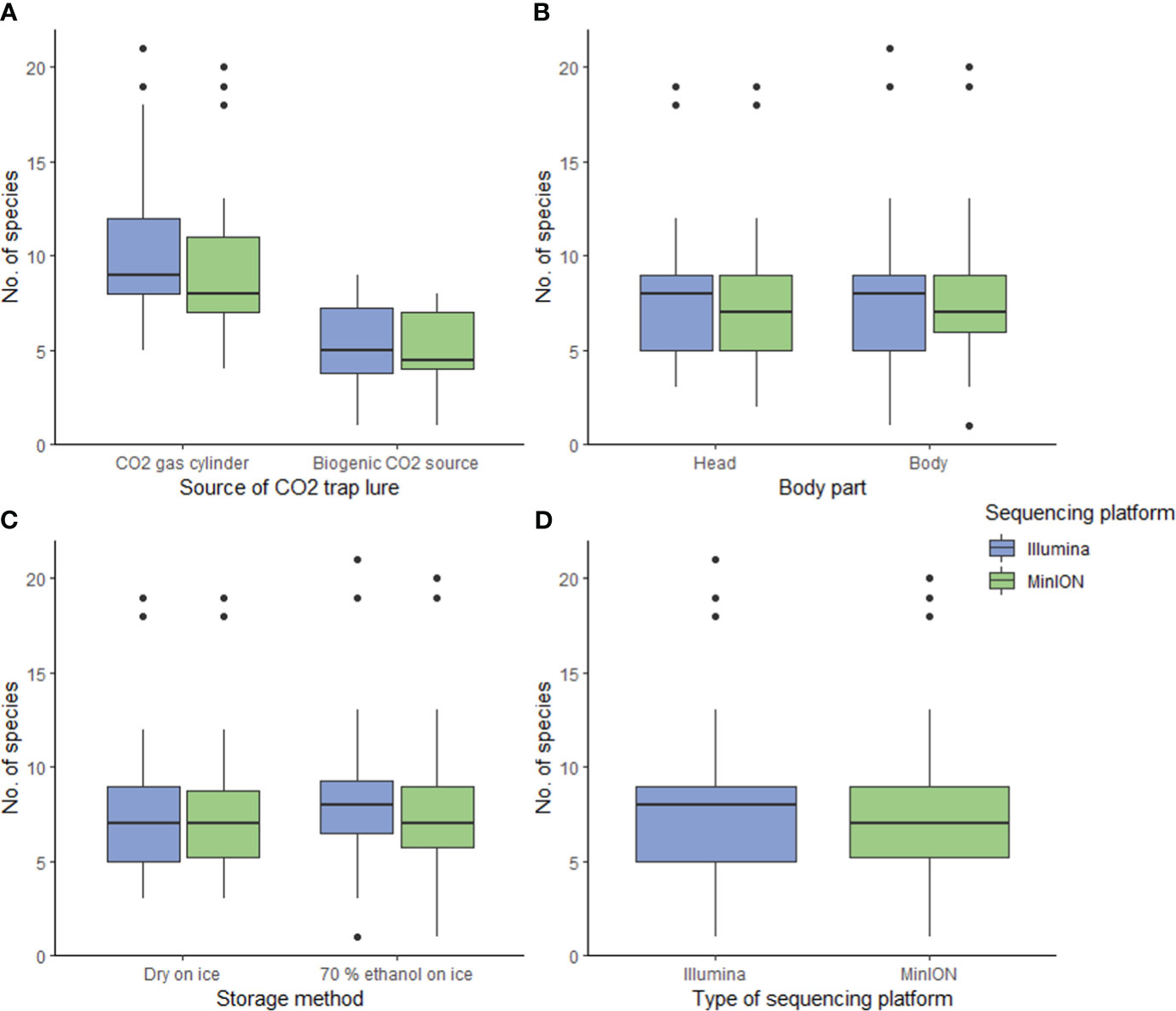
Figure 2 Boxplots show that only (A) the source of CO2 trap lure had a significant impact on the number of mosquito species detected from DNA metabarcoding bulk samples (p-value<0.05). The other variables did not influence the species richness recovered significantly, including (B) the specimen body segment used to assemble a bulk sample, (C) the storage methods, and (D) the sequencing platform.
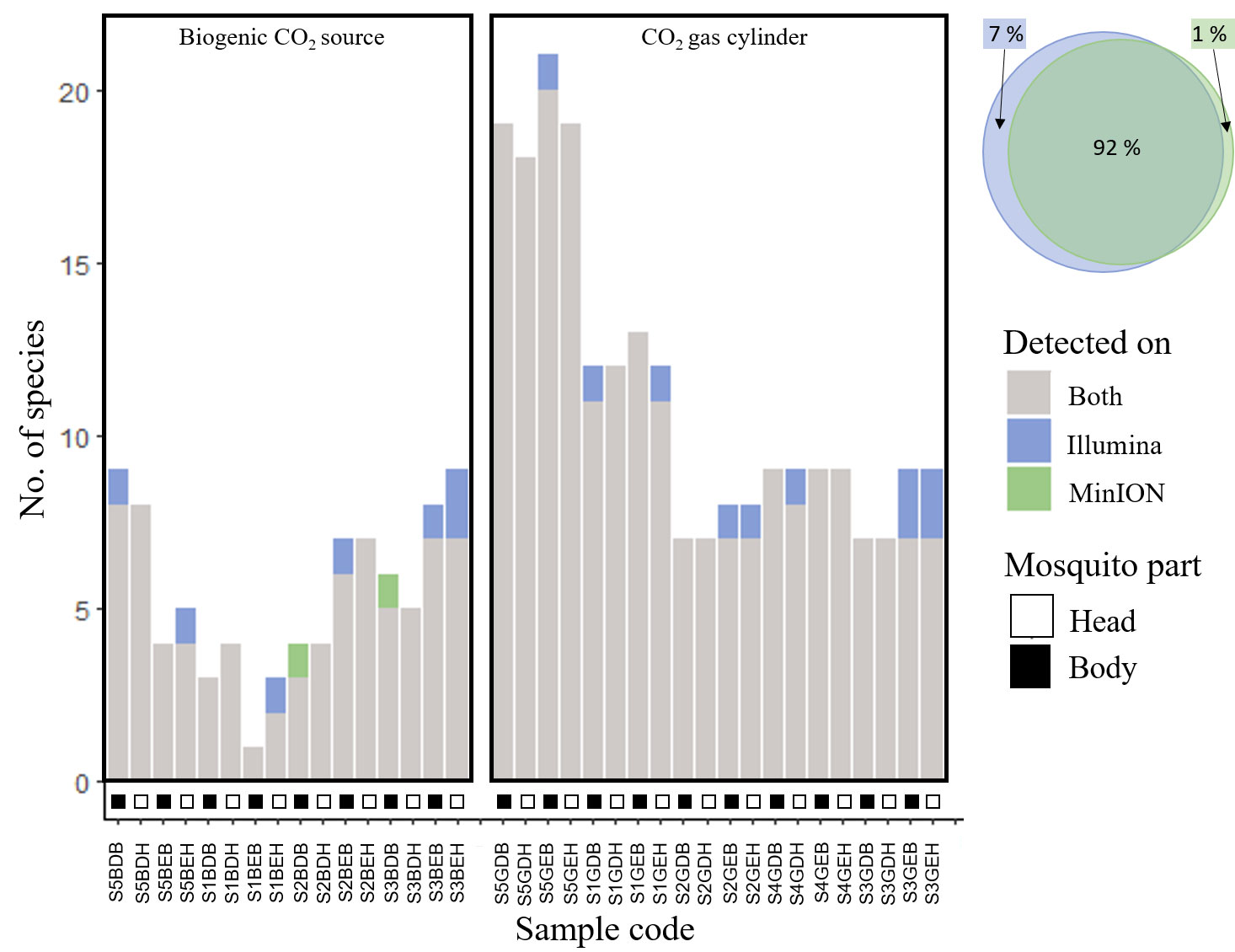
Figure 3 Graphs and Venn diagram demonstrating high levels of congruence in the number of mosquito species detected between sequencing platforms (Illumina v.s. MinION), for DNA metabarcoding of bulk samples.
Different CO2 sources had significantly different mosquito catching performance. CO2 gas released by gas cylinders outperformed CO2 biogenically produced using yeast and sugar substrates, in luring a higher number of mosquito species. The utilization of CO2 gas cylinders resulted in a roughly two-fold increase in the average number of mosquito species captured per sample (Figure 2A), and this trend is consistent across both sequencing platforms (MinION: p-value<0.05, marginal R2 = 0.465, conditional R2 = 0.646; Illumina: p-value<0.05, marginal R2 = 0.472, conditional R2 = 0.646; MinION data and Illumina data: ΔAICc<2 (63); Figure 2A, see Supplementary Tables 3–5). The additional species observed spanned multiple genera without displaying a discernable pattern (see Supplementary Figure 1). These findings suggest that while biogenic CO2 sources may offer convenience in deployment (45), gas cylinders prove to be more efficient for mosquito trapping. One plausible explanation is that gas cylinders release CO2 more consistently than yeast, which ferments its substrate and result in fluctuating CO2 production rates (64). Another possibility is that non-target taxa were drawn to the sugar substrates in the traps, added for yeast biogenic production of CO2. Initial, visual assessment of the mosquito traps corroborated this, revealing that more non-target taxa were caught when yeast was employed.
The employment of different body segments to create a bulk sample and the utilization of different storage methods did not exert a significant influence on species richness. Results show that dissecting and pooling only the specimen heads to create a bulk sample did not improve species detection (MinION and Illumina: p-value<0.05 and ΔAICc>2; Figure 2B, see Supplementary Tables 3–5). This suggests that pooling whole mosquito specimens is as effective as pooling only the heads in preparing a bulk sample for DNA metabarcoding. Consequently, for future mosquito biosurveillance applications, we recommend pooling whole specimens without dissection to reduce processing time. Storing specimens in ethanol in addition to freezing, did not yield a significant improvement in species detection (MinION data and Illumina data: p-value<0.05. Only Illumina data: ΔAICc=1.99 (very close to 2); Figure 2C, see Supplementary Tables 3–5). This implies that for sample storage, freezing at 0°C alone is adequate for short-term storage of samples (<12 hrs) which is beneficial for remote field sites with no accessibility to more advanced infrastructure. The efficacy of keeping specimens in ethanol at room temperature (or warmer) compared to cold storage remains a subject for future investigation, especially since ice and refrigerators or freezers may not be readily available at or near survey sites (23). It is worth noting that for longer-term storage of samples, ethanol is more likely to be crucial (65).
Additional applications: detecting hosts and pathogens
A number of non-mosquito detections in our study demonstrate that identifying hosts and pathogens in mosquito bulk samples is a possible application of the DNA metabarcoding toolbox. Results from quality-filtered, Illumina COI metabarcoding sequences of mosquito bulk samples, revealed mOTU BLAST matches to Sus scrofa and Otus lettia, which are likely to be DNA signals from the mosquito’s bloodmeals, and hence, potential zoonotic reservoirs. NCBI BLAST matches of demultiplexed but unfiltered data also revealed DNA signals of avian haemosporidian parasites in one mosquito bulk sample. Although the identity match was very low (94%), this is not surprising considering that COI is not the most ideal DNA barcoding marker for protists (66). Further preliminary tests also corroborated the identity of these parasites (i.e., PCR amplification using parasite-specific primers and checking PCR success via gel electrophoresis) (Yeo et al., unpublished). These unintended detections suggests that mosquito bulk samples can not only be used for vector surveillance, but also for detecting hosts and pathogens. In the future, host-specific and pathogen-specific primers could be applied to increase their respective detections.
In summary, our study investigates various factors influencing the efficacy of biosurveillance and offers recommendations for optimizing the molecular detection workflow, with broader applicability beyond Singapore particularly in regions which are lacking taxonomic expertise. We show that utilizing the MinION and employing a basic bioinformatic pipeline yields results that are highly consistent with Illumina sequencing, positioning it as a promising addition to the biosurveillance toolbox. We also found that CO2 gas cylinders outperformed biogenic sources in attracting more mosquito species, while strategies such as pooling only the head segment for DNA metabarcoding or incorporating ethanol preservative for short-term cold storage did not significantly enhance species detection rates. Finally, our study demonstrates the feasibility of identifying hosts and pathogens from mosquito bulk samples.
Data availability statement
DNA barcode matches to our internal, Culicidae reference database have been published on NCBI GenBank, accession numbers OQ730546 – OQ730549. Raw sequencing data in fastq (Illumina MiSeq) and fast5/fastq (MinION) formats have been uploaded to the NCBI Sequence Read Archive, BioProject accession number PRJNA956466.
Ethics statement
The manuscript presents research on animals that do not require ethical approval for their study.
Author contributions
NP, HY, and SK contributed to conception and design of the study. HY, TY, TT, and TL were responsible for the collection and organization of field samples. RL, HY, TY, and TT were responsible for the molecular work. RL, HY, and SK were responsible for the bioinformatic analyses. RL, HY, TT, and NP were responsible for the statistical work and figures. TY and HY wrote the first draft of the manuscript. RL, TT, and NP were responsible for major revisions. All authors contributed to the article and approved the submitted version.
Conflict of interest
The authors declare that the research was conducted in the absence of any commercial or financial relationships that could be construed as a potential conflict of interest.
Publisher’s note
All claims expressed in this article are solely those of the authors and do not necessarily represent those of their affiliated organizations, or those of the publisher, the editors and the reviewers. Any product that may be evaluated in this article, or claim that may be made by its manufacturer, is not guaranteed or endorsed by the publisher.
Supplementary material
The Supplementary Material for this article can be found online at: https://www.frontiersin.org/articles/10.3389/fitd.2024.1223435/full#supplementary-material
References
1. WHO. Vector-borne diseases. (2020). Available at: https://www.who.int/news-room/fact-sheets/detail/vector-borne-diseases.
2. Durden LA, Mullen GR. Chapter 1—Introduction. In: Mullen GR, Durden LA, editors. Medical and veterinary entomology, 3rd ed. Massachusetts: Academic Press (2019). p. 1–16. doi: 10.1016/B978-0-12-814043-7.00001-7
3. Breedlove B. Deadly, dangerous, and decorative creatures. Emerging Infect Dis (2022) 28(2):495–6. doi: 10.3201/eid2802.AC2802
4. Benelli G. Research in mosquito control: Current challenges for a brighter future. Parasitol Res (2015) 114(8):2801–5. doi: 10.1007/s00436-015-4586-9
5. Shepard DS, Undurraga EA, Halasa YA, Stanaway JD. The global economic burden of dengue: A systematic analysis. Lancet Infect Dis (2016) 16(8):935–41. doi: 10.1016/S1473-3099(16)00146-8
6. Carvalho VL, Long MT. Perspectives on new vaccines against arboviruses using insect-specific viruses as platforms. Vaccines (2021) 9(3):263. doi: 10.3390/vaccines9030263
7. Tomerini DM, Dale PE, Sipe N. Does mosquito control have an effect on mosquito-borne disease? The case of ross river virus disease and mosquito management in Queensland, Australia. J Am Mosq Control Assoc (2011) 27(1):39–44. doi: 10.2987/10-6038.1
8. WHO. Global vector control response 2017-2030. Geneva: World Health Organization (2017). Available at: https://apps.who.int/iris/handle/10665/259205. Licence: CC BY-NC-SA 3.0 IGO.
9. American Mosquito Control Association (AMCA). Best practices for integrated mosquito management. Sacramento, CA: American Mosquito Control Association (2021). Available at: https://cdn.ymaws.com/www.mosquito.org/resource/resmgr/docs/publications/hr_november_2021_amca_bmp_ma.pdf.
10. Environmental Health Service, Department of Health (EHSDH). South Australian Integrated Mosquito Management Resource Package 2006: An informative guide for mosquito management practitioners. South Australia: Environmental Health Service, South Australian Department of Health (2006). Available at: https://www.sahealth.sa.gov.au/wps/wcm/connect/6f5e908045c6d6e18dceefac725693cd/mozzie-resource-aug06.pdf?MOD=AJPERES&CACHEID=ROOTWORKSPACE-6f5e908045c6d6e18dceefac725693cd-nKQfCUD.
11. European Centre for Disease Prevention and Control (ECDPC). Guidelines for the surveillance of invasive mosquitoes in Europe. Stockholm: ECDC (2012). Available at: https://data.europa.eu/doi/10.2900/61134.
12. Food and Environmental Hygiene Department - The Government of the Hong Kong Special Administrative Region (FEHD). Mosquito pests and their control. (2022). Available at: https://www.fehd.gov.hk/english/pestcontrol/risk-pest-mosquito.html.
13. National Environmental Agency (NEA). Surveillance and epidemiology programme. (2020). Available at: https://www.nea.gov.sg/corporate-functions/resources/research/surveillance-and-epidemiology-programme.
14. Taiwan Centres for Disease Control (TCDC). Dengue fever. (2014). Available at: https://www.cdc.gov.tw/En/Category/ListContent/bg0g_VU_Ysrgkes_KRUDgQ?uaid=9_Oq7OYHa-l8B05iUwyVvQ.
15. Yeo H, Yeoh TX, Ding H, Lee TTM, Puniamoorthy N. Morphology and mini-barcodes: The inclusion of larval sampling and NGS-based barcoding improves robustness of ecological analyses of mosquito communities. J Appl Ecol (2021) 58(10):2087–100. doi: 10.1111/1365-2664.13966
16. Montalvo-Sabino E, Abílio AP, Guarido MM, Valadas V, Novo MT, Kampango A, et al. Morphological and molecular characterization using genitalia and CoxI barcode sequence analysis of afrotropical mosquitoes with arbovirus vector potential. Diversity (2022) 14(11):11. doi: 10.3390/d14110940
17. Chan A, Chiang L-P, Hapuarachchi HC, Tan C-H, Pang S-C, Lee R, et al. DNA barcoding: Complementing morphological identification of mosquito species in Singapore. Parasites Vectors (2014) 7(1):569. doi: 10.1186/s13071-014-0569-4
18. van de Vossenberg BTLH, Ibáñez-Justicia A, Metz-Verschure E, van Veen EJ, Bruil-Dieters ML, Scholte EJ. Real-time PCR Tests in Dutch Exotic Mosquito Surveys; Implementation of Aedes aEgypti and Aedes albopictus Identification Tests, and the Development of Tests for the Identification of Aedes atropalpus and Aedes japonicus japonicus (Diptera: Culicidae). J Med Entomology (2015) 52(3):336–50. doi: 10.1093/jme/tjv020
19. Talaga S, Leroy C, Guidez A, Dusfour I, Girod R, Dejean A, et al. DNA reference libraries of French Guianese mosquitoes for barcoding and metabarcoding. PloS One (2017) 12(6):e0176993. doi: 10.1371/journal.pone.0176993
20. Beebe NW. DNA barcoding mosquitoes: Advice for potential prospectors. Parasitology (2018) 145(5):622–33. doi: 10.1017/S0031182018000343
21. Besansky NJ, Severson DW, Ferdig MT. DNA barcoding of parasites and invertebrate disease vectors: What you don’t know can hurt you. Trends Parasitol (2003) 19(12):545–6. doi: 10.1016/j.pt.2003.09.015
22. Almeida APG, Fouque F, Launois P, Sousa CA, Silveira H. From the laboratory to the field: updating capacity building in medical entomology. Trends Parasitol (2017) 33(9):664–8. doi: 10.1016/j.pt.2017.05.011
23. Boerlijst SP, Trimbos KB, van der Beek JG, Dijkstra KDB, van der Hoorn BB, Schrama M. Field evaluation of DNA based biodiversity monitoring of caribbean mosquitoes. Front Ecol Evol (2019) 7:240. doi: 10.3389/fevo.2019.00240
24. Pedro PM, Rodrigues de Sá IL, Rojas MVR, Amorim JA, Ribeiro Galardo AK, Santos Neto NF, et al. Efficient monitoring of adult and immature mosquitoes through metabarcoding of bulk samples: A case study for non-model culicids with unique ecologies. J Med Entomology (2021) 58(3):1210–8. doi: 10.1093/jme/tjaa267
25. Harbach R. The culicidae (Diptera): A review of taxonomy, classification and phylogeny. Zootaxa (2007) 1668:591–638. doi: 10.5281/zenodo.180118
26. Thielman A, Hunter FF. Establishment of ochlerotatus japonicus (Diptera: culicidae) in ontario, Canada. J Med Entomology (2006) 43(2):138–42. doi: 10.1093/jmedent/43.2.138
27. Peach DAH, Matthews BJ. The invasive mosquitoes of Canada: an entomological, medical, and veterinary review. Am J Trop Med Hygiene (2022) 107(2):231–44. doi: 10.4269/ajtmh.21-0167
28. Klink R, August T, Bas Y, Bodesheim P, Bonn A, Fossøy F, et al. Emerging technologies revolutionise insect ecology and monitoring. Trends Ecol Evol (2022). doi: 10.1016/j.tree.2022.06.001
29. Meier R, Wong W, Srivathsan A, Foo M. $1 DNA barcodes for reconstructing complex phenomes and finding rare species in specimen-rich samples. Cladistics (2016) 32(1):100–10. doi: 10.1111/cla.12115
30. Cheng Z, Li Q, Deng J, Liu Q, Huang X. The devil is in the details: Problems in DNA barcoding practices indicated by systematic evaluation of insect barcodes. Front Ecol Evol (2023) 11:1149839. doi: 10.3389/fevo.2023.1149839
31. Piper AM, Batovska J, Cogan NOI, Weiss J, Cunningham JP, Rodoni BC, et al. Prospects and challenges of implementing DNA metabarcoding for high-throughput insect surveillance. GigaScience (2019) 8(8):giz092. doi: 10.1093/gigascience/giz092
32. Schneider J, Valentini A, Dejean T, Montarsi F, Taberlet P, Glaizot O, et al. Detection of invasive mosquito vectors using environmental DNA (eDNA) from water samples. PloS One (2016) 11(9):e0162493. doi: 10.1371/journal.pone.0162493
33. Zinger L, Bonin A, Alsos IG, Bálint M, Bik H, Boyer F, et al. DNA metabarcoding—Need for robust experimental designs to draw sound ecological conclusions. Mol Ecol (2019) 28(8):1857–62. doi: 10.1111/mec.15060
34. van der Loos LM, Nijland R. Biases in bulk: DNA metabarcoding of marine communities and the methodology involved. Mol Ecol (2021) 30(13):3270–88. doi: 10.1111/mec.15592
35. Mechai S, Bilodeau G, Lung O, Roy M, Steeves R, Gagne N, et al. Mosquito identification from bulk samples using DNA metabarcoding: A protocol to support mosquito-borne disease surveillance in Canada. J Med Entomology (2021) 58(4):1686–700. doi: 10.1093/jme/tjab046
36. Lilja T, Nylander JAA, Troell K, Lindström A. Species identification of Swedish mosquitoes through DNA metabarcoding. J Eur Mosq Control Assoc (2017) 35:9.
37. Batovska J, Lynch SE, Cogan NOI, Brown K, Darbro JM, Kho EA, et al. Effective mosquito and arbovirus surveillance using metabarcoding. Mol Ecol Resour (2018) 18(1):32–40. doi: 10.1111/1755-0998.12682
38. Srivathsan A, Feng V, Suárez D, Emerson B, Meier R. (2023). ONTbarcoder 2.0: Rapid species discovery and identification with real-time barcoding facilitated by Oxford Nanopore R10.4. Cladistics. doi: 10.1111/cla.12566
39. Egeter B, Veríssimo J, Lopes-Lima M, Chaves C, Pinto J, Riccardi N, et al. Speeding up the detection of invasive bivalve species using environmental DNA: A Nanopore and Illumina sequencing comparison. Mol Ecol Resour (2022) 22(6):2232–47. doi: 10.1111/1755-0998.13610
40. Laver T, Harrison J, O’Neill PA, Moore K, Farbos A, Paszkiewicz K, et al. Assessing the performance of the oxford nanopore technologies minION. Biomolecular Detection Quantification (2015) 3:1–8. doi: 10.1016/j.bdq.2015.02.001
41. Sauvage V, Boizeau L, Candotti D, Vandenbogaert M, Servant-Delmas A, Caro V, et al. Early MinION™ nanopore single-molecule sequencing technology enables the characterization of hepatitis B virus genetic complexity in clinical samples. PloS One (2018) 13(3):e0194366. doi: 10.1371/journal.pone.0194366
42. Jain M, Tyson JR, Loose M, Ip CLC, Eccles DA, O’Grady J, et al. MinION Analysis and Reference Consortium: Phase 2 data release and analysis of R9.0 chemistry. F1000Research (2017) 6:760. doi: 10.12688/f1000research.11354.1
43. Chang JJM, Ip YCA, Ng CSL, Huang D. Takeaways from mobile DNA barcoding with bentoLab and minION. Genes (2020) 11(10):1121. doi: 10.3390/genes11101121
44. Oxford Nanopore Technologies. R10.3: The newest nanopore for high accuracy nanopore sequencing – now available in store. (2020). Available at: http://nanoporetech.com/about-us/news/r103-newest-nanopore-high-accuracy-nanopore-sequencing-now-available-store.
45. Sukumaran D, Ponmariappan S, Sharma AK, Jha HK, Wasu YH, Sharma AK. Application of biogenic carbon dioxide produced by yeast with different carbon sources for attraction of mosquitoes towards adult mosquito traps. Parasitol Res (2016) 115(4):1453–62. doi: 10.1007/s00436-015-4879-z
46. Zhou J, Wu L, Deng Y, Zhi X, Jiang Y-H, Tu Q, et al. Reproducibility and quantitation of amplicon sequencing-based detection. ISME J (2011) 5(8):1303–13. doi: 10.1038/ismej.2011.11
47. Kebschull JM, Zador AM. Sources of PCR-induced distortions in high-throughput sequencing data sets. Nucleic Acids Res (2015) 43(21):e143–3. doi: 10.1093/nar/gkv717
48. Leray M, Knowlton N. Random sampling causes the low reproducibility of rare eukaryotic OTUs in Illumina COI metabarcoding. PeerJ (2017) 5:e3006. doi: 10.7717/peerj.3006
49. Krehenwinkel H, Fong M, Kennedy S, Huang EG, Noriyuki S, Cayetano L, et al. The effect of DNA degradation bias in passive sampling devices on metabarcoding studies of arthropod communities and their associated microbiota. PloS One (2018) 13(1):e0189188. doi: 10.1371/journal.pone.0189188
50. Loh RK, Kutty SN, Yeo DCJ, Meier R. Toward eDNA-based bioassessment of freshwater reservoirs with small volumes of water: Robust molecular protocols. Environmental DNA (2021) 5(2):264–81. doi: 10.1002/edn3.373
51. Shirazi S, Meyer RS, Shapiro B. Revisiting the effect of PCR replication and sequencing depth on biodiversity metrics in environmental DNA metabarcoding. Ecol Evol (2021) 11(22):15766–79. doi: 10.1002/ece3.8239
52. Braukmann TWA, Ivanova NV, Prosser SWJ, Elbrecht V, Steinke D, Ratnasingham S, et al. Metabarcoding a diverse arthropod mock community. Mol Ecol Resour (2019) 19(3):711–27. doi: 10.1111/1755-0998.13008
53. Leray M, Yang JY, Meyer CP, Mills SC, Agudelo N, Ranwez V, et al. A new versatile primer set targeting a short fragment of the mitochondrial COI region for metabarcoding metazoan diversity: Application for characterizing coral reef fish gut contents. Front Zoology (2013) 10(1):34. doi: 10.1186/1742-9994-10-34
54. Geller J, Meyer C, Parker M, Hawk H. Redesign of PCR primers for mitochondrial cytochrome c oxidase subunit I for marine invertebrates and application in all-taxa biotic surveys. Mol Ecol Resour (2013) 13(5):851–61. doi: 10.1111/1755-0998.12138
55. Srivathsan A, Hartop E, Puniamoorthy J, Lee WT, Kutty SN, Kurina O, et al. Rapid, large-scale species discovery in hyperdiverse taxa using 1D MinION sequencing. BMC Biol (2019) 17(1):96. doi: 10.1186/s12915-019-0706-9
56. Chen S, Zhou Y, Chen Y, Gu J. fastp: An ultra-fast all-in-one FASTQ preprocessor. Bioinformatics (2018) 34(17):i884–90. doi: 10.1093/bioinformatics/bty560
57. Boyer F, Mercier C, Bonin A, Le Bras Y, Taberlet P, Coissac E. obitools: A unix-inspired software package for DNA metabarcoding. Mol Ecol Resour (2016) 16(1):176–82. doi: 10.1111/1755-0998.12428
58. Edgar RC. Search and clustering orders of magnitude faster than BLAST. Bioinf (Oxford England) (2010) 26(19):2460–1. doi: 10.1093/bioinformatics/btq461
59. Camacho C, Coulouris G, Avagyan V, Ma N, Papadopoulos J, Bealer K, et al. BLAST+: architecture and applications. BMC Bioinf (2009) 10:421. doi: 10.1186/1471-2105-10-421
60. Srivathsan A, Lee L, Katoh K, Hartop E, Kutty SN, Wong J, et al. ONTbarcoder and MinION barcodes aid biodiversity discovery and identification by everyone, for everyone. BMC Biol (2021) 19(1):217. doi: 10.1186/s12915-021-01141-x
61. Yong DL, Liu Y, Low BW, Española CP, Choi C-Y, Kawakami K. Migratory songbirds in the East Asian-Australasian Flyway: A review from a conservation perspective. Bird Conserv Int (2015) 25(1):1–37. doi: 10.1017/S0959270914000276
62. Yeo H, Harjoko DN, Rheindt FE. Double trouble: Untangling mixed sequence signals in bird samples with avian haemosporidian co-infections. Parasitology (2022) 149(6):799–810. doi: 10.1017/S0031182022000245
63. Burnham KP, Anderson DR. Practical use of the information-theoretic approach. In: Burnham KP, Anderson DR, editors. Model Selection and Inference: A Practical Information-Theoretic Approach. New York: Springer (1998). p. 75–117. doi: 10.1007/978-1-4757-2917-7_3
64. Jerry DCT, Mohammed T, Mohammed A. Yeast-generated CO2: A convenient source of carbon dioxide for mosquito trapping using the BG-Sentinel® traps. Asian Pacific J Trop Biomedicine (2017) 7(10):896–900. doi: 10.1016/j.apjtb.2017.09.014
65. Straube D, Juen A. Storage and shipping of tissue samples for DNA analyses: A case study on earthworms. Eur J Soil Biol (2013) 57:13–8. doi: 10.1016/j.ejsobi.2013.04.001
Keywords: Illumina MiSeq System, MinION, Next Generation Sequencing, DNA metabarcoding, Vector Biology, nanopore technologies, Culicidae, mosquito
Citation: Loh RK, Tan TRH, Yeo H, Yeoh TX, Lee TTM, Kutty SN and Puniamoorthy N (2024) Metabarcoding mosquitoes: MinION sequencing of bulk samples gives accurate species profiles for vector surveillance (Culicidae). Front. Trop. Dis 5:1223435. doi: 10.3389/fitd.2024.1223435
Received: 18 May 2023; Accepted: 26 January 2024;
Published: 13 February 2024.
Edited by:
Paul O. Mireji, Kenya Agricultural and Livestock Research Organization, KenyaReviewed by:
Lawrence E. Reeves, University of Florida, United StatesA. Paulo Gouveia Almeida, New University of Lisbon, Portugal
Copyright © 2024 Loh, Tan, Yeo, Yeoh, Lee, Kutty and Puniamoorthy. This is an open-access article distributed under the terms of the Creative Commons Attribution License (CC BY). The use, distribution or reproduction in other forums is permitted, provided the original author(s) and the copyright owner(s) are credited and that the original publication in this journal is cited, in accordance with accepted academic practice. No use, distribution or reproduction is permitted which does not comply with these terms.
*Correspondence: Rebecca Ker Loh, cmViZWNjYWxvaGtlckBnbWFpbC5jb20=; Nalini Puniamoorthy, bmFsaW5pQG51cy5lZHUuc2c=
†These authors share first authorship