- 1Institute for Environmental Design and Engineering, University College London, London, UK
- 2BuroHappold Engineering, London, UK
- 3Energy Institute, University College London, London, UK
This paper reviews the discrepancy between predicted and measured energy use in non-domestic buildings in a UK context with outlook to global studies. It explains differences between energy performance quantification and classifies this energy performance gap as a difference between compliance and performance modeling with measured energy use. Literary sources are reviewed in order to signify the magnitude between predicted and measured energy use, which is found to deviate by +34% with a SD of 55% based on 62 buildings. It proceeds in describing the underlying causes for the performance gap, existent in all stages of the building life cycle, and identifies the dominant factors to be related to specification uncertainty in modeling, occupant behavior, and poor operational practices having an estimated effect of 20–60, 10–80, and 15–80% on energy use, respectively. Other factors that have a high impact are related to establishing the energy performance target, such as early design decisions, heuristic uncertainty in modeling, and occupant behavior. Finally, action measures and feedback processes in order to reduce the performance gap are discussed, indicating the need for energy in-use legislation, insight into design stage models, accessible energy data, and expansion of research efforts toward building performance in-use in relation to predicted performance.
Highlights
1. Classifies the performance gap and analyses its magnitude and underlying causes.
2. The regulatory energy gap is found to deviate by +34% with a SD of 55% based on 62 case study buildings.
3. Specification uncertainty, occupant behavior, and poor practice are dominant underlying causes with an estimated effect of 20–60, 10–80, and 15–80% on energy use, respectively.
4. Action measures to reduce the energy performance gap in contrast to the building life cycle are discussed.
5. There is a need to develop techniques to mitigate the magnitude and underlying causes of the performance gap.
Introduction
According to the International Energy Agency (IEA), energy consumption in buildings represent one-third of the global energy consumption and is predicted to grow at an average rate of 1.0% per year until 2035 (IEA, 2010). Efforts on making buildings more energy efficient will therefore help to reduce carbon emissions and address climate change (European Commission, 2011). To meet targets set by the government, building regulations require new and existing buildings to be energy efficient and/or carbon efficient to a certain degree.
The Energy Performance Gap
To design and operate more efficient buildings, many classification schemes have been established, providing a means to communicate a building’s relative energy efficiency and carbon emissions (Wang et al., 2012b). These assessment schemes are related to the energy consumption of a building and can be quantified using different methods, both in the design stage [e.g., asset ratings, energy performance certificates (EPC), and part L calculations in the UK] and operational stage [e.g., operational ratings, display energy certificates (DEC) in the UK] of a building. Accredited performance assessment tools, ranging from steady-state calculations to dynamic simulation methods are utilized to predict the energy consumption of a building, to comply with regulated targets using standardized procedures. Both classification schemes (such as the EPC) and standard calculation procedures for quantifying the energy use of a building have been reported to show significant discrepancies to measured energy use during occupation, which risk not achieving regulated targets. This phenomenon has been termed previously as “the performance gap” (Carbon Trust, 2012; Menezes et al., 2012; Burman et al., 2014; de Wilde, 2014; Cohen and Bordass, 2015). Although a margin of error between predicted and measured energy use is inevitable due to uncertainties in design and operation, as well as limitations of measurements systems, explaining its magnitude and underlying causes are necessary to more confidently forecast and understand energy use in buildings.
Classification of the Gap
Building energy modeling is an integral part of today’s design process; however, research has shown that buildings can use twice the amount of their theoretical energy performance (Norford et al., 1994; Pegg et al., 2007). One of the first major post-occupancy evaluation studies was the PROBE studies, which found little connection between values assumed in design estimations and actual values found in existing buildings (Bordass et al., 2001). This makes it unlikely that the building industry achieves model-based targets (UKGBC, 2007). In the UK and most other countries, regulatory performance is determined through compliance modeling, which is the implementation of thermal modeling to calculate the energy performance of a building under standardized operating conditions (occupant density, set-points, operating schedules, etc.), set out in national calculation methodologies. Compliance modeling is useful to assess the energy efficiency of buildings under standardized conditions to determine if minimum performance requirements are met. However, such calculations should not be used as baselines for actual performance (Burman et al., 2014). Using the outcomes of compliance modeling to evaluate actual energy performance creates a significant risk for energy-related issues to go unnoticed, as the discrepancy between measured and modeled energy may be understood as the result of expected differences in operating conditions and exclusion of non-regulated loads from compliance modeling. This type of comparison has often been used to define the term “the performance gap” (Carbon Trust, 2012; Menezes et al., 2012; Cohen and Bordass, 2015). This was to some extent, inevitable due to the dominance of compliance modeling in the context of the current regulatory framework in the UK and European Union. However, comparing compliance modeling with measured energy use may lead to a distorted view of the energy performance gap. Theoretically, a gap could significantly be reduced if a building is simulated with actual operating conditions, in other words, when attention is paid to the building context, defined here as performance modeling. The term performance modeling in this context includes all energy quantification methods that aim to accurately predict the performance of a building. The difference between compliance modeling and performance modeling is further illustrated in Figure 1.

Figure 1. Representation of compliance modelling and its exclusion of different end-uses in energy calculations of a building.
On-going efforts to understand the energy performance gap have utilized calibration techniques to fine-tune a building energy model to actual operating conditions and energy use, ideally over a longer period of time. This method gives insights into the operational inefficiencies of a building and can pinpoint underlying reasons for differences between design estimations and actual use. Subsequently, a calibrated model could reintroduce design assumptions to quantify impacts of any underlying causes and their effect on energy performance. As such, a distinction can be made between three types of modeling efforts, which can be classified in three different ways to interpret the energy performance gap. These are the gap between compliance modeling and measured energy use, performance modeling and measured energy use and calibration and energy use with a longitudinal perspective (Burman, 2016):
1. Regulatory performance gap, comparing predictions from compliance modeling to measured energy use.
2. Static performance gap, comparing predictions from performance modeling to measured energy use.
3. Dynamic performance gap, utilizing calibrated predictions from performance modeling with measured energy use taking a longitudinal perspective to diagnose underlying issues and their impact on the performance gap.
Importance of Reducing the Gap
There is a need for design stage calculation methodologies to address all aspects of building energy consumption for whole-building simulation, including regulated and unregulated uses and predictions of actual operation (Norford et al., 1994; Diamond et al., 2006; Torcellini et al., 2006; Turner and Frankel, 2008). Building energy simulation models need to closely represent the actual behavior of the building under study for them to be used with any degree of confidence (Coakley et al., 2011). These models contain the design goals and should therefore be the basis for an assessment to determine whether the completed product complies with the design goals (Maile et al., 2012). Underperformance in design may soon be met by legal, financial implications (Daly et al., 2014), and demands for compensation and rectification work (ZCH and NHBC Foundation, 2010).
Investigation of predicted and measured energy use is necessary in order to understand the underlying causes of the performance gap. Furthermore, feedback helps improving the quality of future design stage models by identifying common mistaken assumption and by developing best-practice modeling approaches (Raftery et al., 2011). This also guides the development of simulation tools and identifies areas requiring research (Raftery et al., 2011), such as uncertainty and sensitivity analysis, parametric modeling, geometry creation, and system modeling. Furthermore, it can help policy-makers define performance targets more accurately (Government HM, 2010; ZCH, 2010), which then assist in mitigating climate change. In operation, methodologies that analyze a discrepancy and related issues can help in understanding how a specific building is operating, highlighting poor-performing and well-performing buildings, and identifying areas where action is required. Investigating a discrepancy between design and operation can also support in identifying retrofit options to reduce energy use. In order to more accurately predict energy savings from a set of proposed retrofit technologies, the simulation model must represent a building as operated (Heo et al., 2012). Building audits and monitored energy consumption should become integral to the modeling process.
Magnitude and Underlying Causes
Different procedures can be used for calculating the energy performance in both the design and operational stage of a building. Wang et al. (2012b) provide an extensive overview of energy performance quantification and assessment methods. These methods use mathematical equations to relate physical properties of the building, system, and equipment specifications to its external environment. They can help prospective occupiers, building owners, designers, and engineers in giving an indication of building energy use, carbon dioxide emissions, and operational costs. Furthermore, it allows a better understanding of where and how energy is used in a building and which measures have the greatest impact on energy use (CIBSE, 2013). In operation, it can identify energy-saving potentials and evaluate the energy performance and cost-effectiveness of energy-saving measures to be implemented (Pan et al., 2007). However, the built environment is complex and influenced by a large number of independent and interdependent variables, making it difficult to represent real-world building energy in-use (Coakley et al., 2011). Thus, models represent a simplification of reality, therefore, it is necessary to quantify to what degree they are inaccurate before employing them in design, prediction, and decision-making processes (Manfren et al., 2013). Comparing measured values to modeled or estimated values does therefore not offer a valid comparison and should be avoided whenever possible (Fowler et al., 2010). This perception is by ASHRAE (2004) as it states in its Energy Standard 90.1 Appendix G – “neither the proposed building performance nor the baseline building performance are predictions of actual energy consumption, due to variations such as occupancy, building operation and maintenance, weather, and the precision of the calculation tool.” Indeed, the modeled or baseline performance here refers to compliance modeling, which is not a representation of reality. Nevertheless, it is useful to signify the regulatory performance gap and to understand how compliance modeling is different to measured energy use. Especially since performance modeling is rarely used to predict the actual energy use of a building, as such, a comparison is unable to ever validate what has been designed.
Magnitude
To date, many studies have focused on understanding the relationship between predicted and measured energy use. Recently, this has become more important due to the increasing need to reduce energy consumption in buildings and their apparent differences.
Table 1 gives an overview of reported discrepancies between predicted and measured energy use found in the literature. An indication of the discrepancy is given by a percentage deviation from the predicted baseline value for a range of different non-domestic buildings. The magnitude of the performance gap is typically reported using percentages, given as an increase or decrease from predicted. Case study buildings are located in different climates and have been predicted through different assessment methods using various simulation software. In this analysis, averages from the CarbonBuzz database are used as reported by Ruyssevelt (2014), who analyzed 408 buildings for different buildings. A more in-depth analysis is given in Robertson and Mumovic (2013). CarbonBuzz (2015) is a platform established in order to benchmark and track energy use in project from design to operation. The type of prediction method used is given in Table 2.
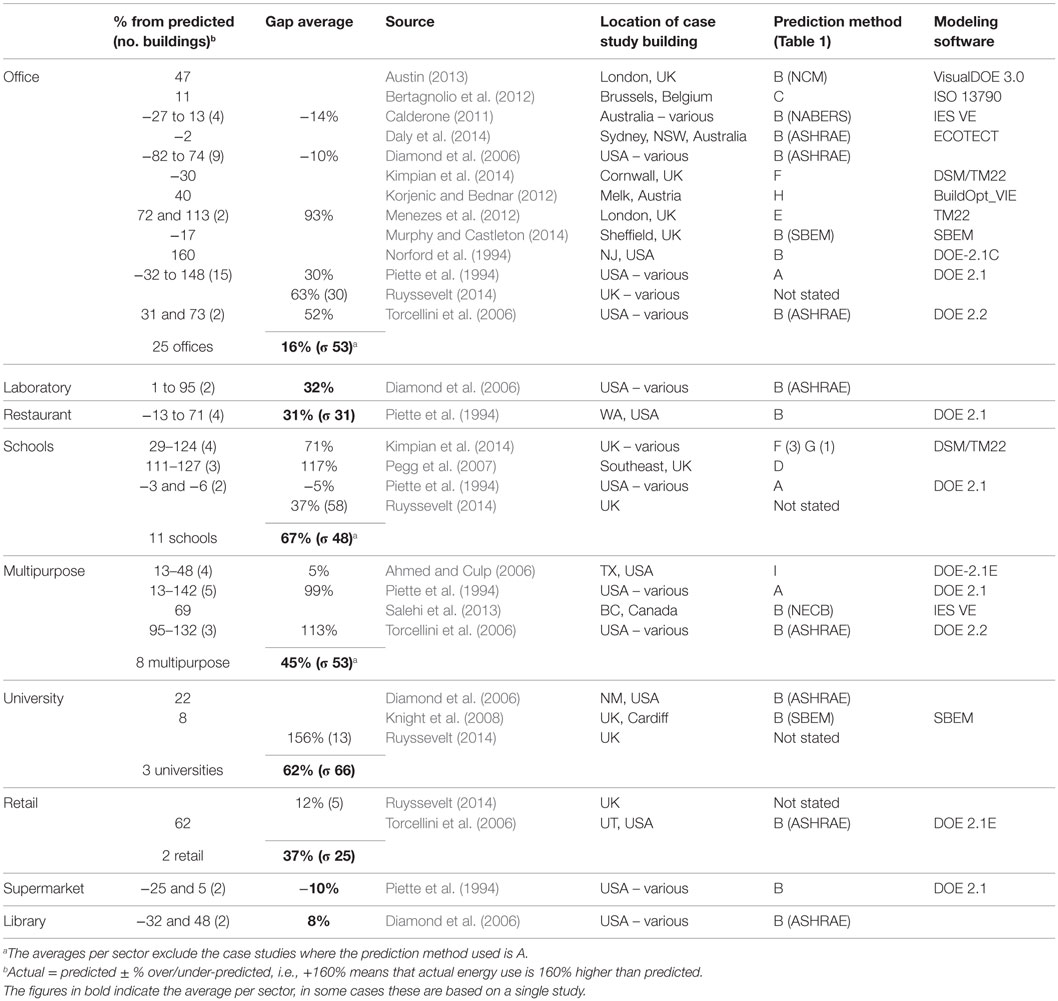
Table 1. Magnitude in discrepancy reported in the literature (regulatory performance gap including equipment energy use).
In Figure 2, absolute values for the case studies are visualized, and Figure 3 illustrates the percentage differences from measured to predicted energy use, segmented by building function. The +100% means that measured energy use is twice the amount predicted, whereas −100% means that measured energy use is 0 kWh/m2a.
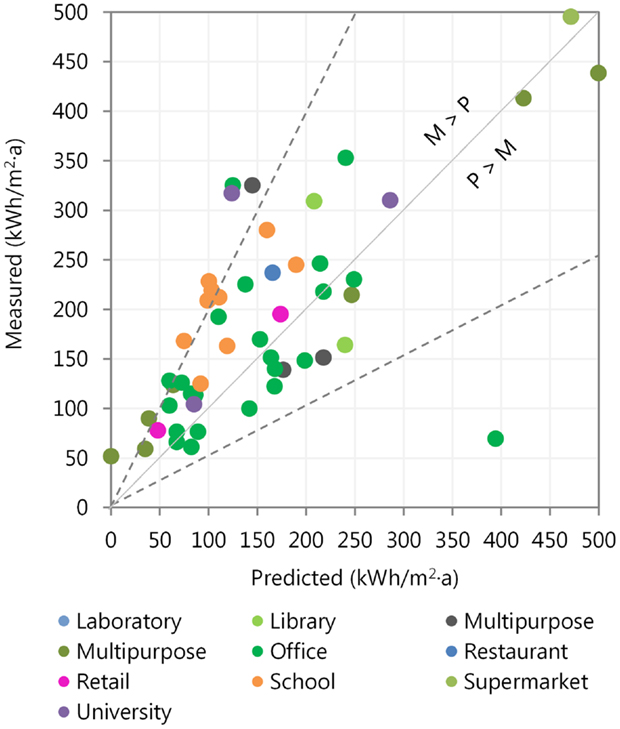
Figure 2. Predicted and measured energy use intensities of reviewed case studies for different building functions.
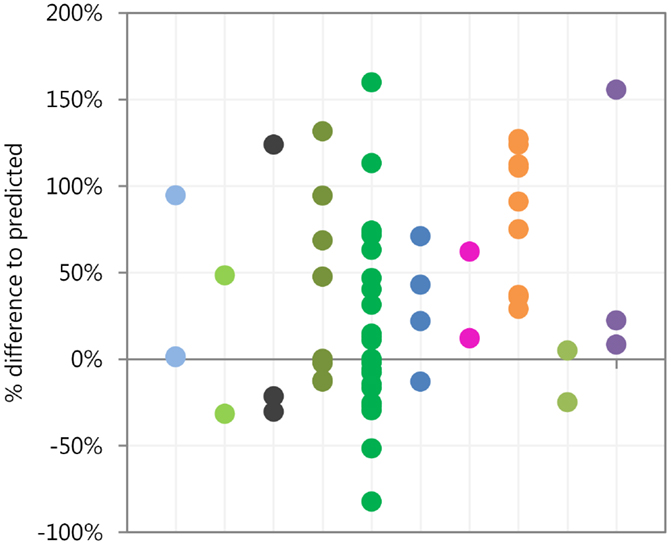
Figure 3. Difference in percentages of measured to predicted energy use of reviewed case studies for different building functions.
In Figure 2, several of the buildings have high energy use intensities (>1000 kWh/m2a) and are therefore not shown. The two dashed lines indicate if measured energy use in specific case studies is double the predicted value (top left) or is less than half the predicted value (bottom right). This visualization emphasizes that measured energy use in most case studies is higher than predicted energy use. While 15% (9 of 62) of the case studies use double the amount of energy initially predicted in contrast to one significant outlier that uses less than half the energy initially predicted. In particular, Ruyssevelt (2014) reported university buildings to use 156% more energy than initially predicted, an average based on 13 individual studies. This percentage is, however, not supported by Knight et al. (2008) and Diamond et al. (2006) who report a difference of only 8 and 22% for university buildings, respectively. Similarly, Ruyssevelt (2014) reported schools to use 37% more energy than initially predicted, based on an average of 58 individual studies, whereas Pegg et al. (2007) and Kimpian et al. (2014) report much higher average percentages of 117% (3 schools) and 71% (5 schools), respectively.
Figure 3 shows that the number of case studies for the different building functions is not well distributed, the dataset consists mainly of offices, schools, and multipurpose buildings. Although the sample size is small, analysis indicates that schools generally consume more energy than predicted, which is likely due to underspecified assumptions for equipment and occupancy hours. The discrepancies for offices are much more variable with an average of +22% and SD of 50%.
Most studies use compliance modeling with the inclusion of equipment energy use, and use model calibration to further understand the underlying causes of a difference. It therefore remains unclear how significant the energy performance gap would be when performance modeling is compared with measured energy use. Although it should theoretically reduce a discrepancy by taking into account unregulated factors, the underlying causes that impact measured energy use might be very erratic and unmanageable, still leaving a significant gap in place.
Underlying Causes
A building energy model represents the speculative design of a building and is a simplification of reality. It is therefore important to quantify to what degree it is imperfect (Manfren et al., 2013). In Figure 4 an overview is given of all the underlying causes of the performance gap existent in the different stages of the building life cycle according to Royal Institute of British Architects’ (RIBA) plan of work (RIBA, 2013) and drawn in relation to an S-curve visualization of building performance proposed by Bunn and Burman (2015). The S-curve model allows for the transient and unstable nature of building performance during design stages and early stages of operation before the building reaches steady operation and can help visualize performance issues. These performance issues are identified as underlying causes of the energy performance gap, some directly related to the regulatory performance gap, whereas others are more applicable to the static performance gap, such as the simplification of system design in modeling. These issues are discussed and qualitatively analyzed to understand their importance.
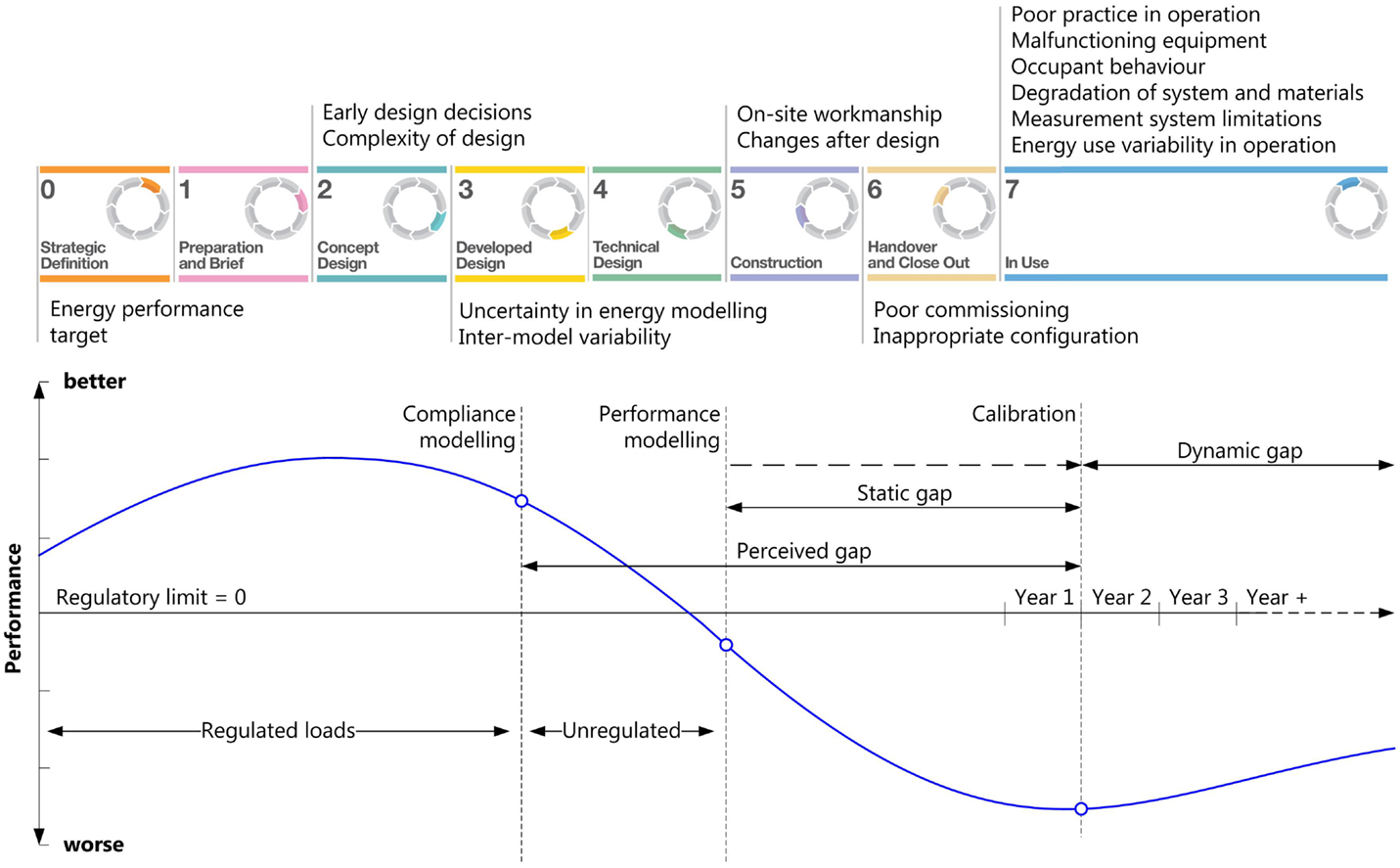
Figure 4. Underlying causes existent in different RIBA stages [adapted from RIBA (2013)] and S-curve visualization of performance throughout the life cycle [adapted from Bunn and Burman (2015)].
Addressing every available source will help in assessing evidence on the impact of these issues; therefore, it is also useful to look at domestic experiences in this area.
Limited Understanding of Impact of Early Design Decisions
During the early design stage, there is a lack of focus and understanding on the energy implications of design decisions (ZCH, 2014a,b). Choices, such as form, orientation, materials, use of renewables, passive strategies, innovative solutions, and others, should be critically addressed during the concept design. Uncertainty and sensitivity analysis that determine the impact of design parameters can guide the design process through identifying and preventing costly design mistakes before they occur (Bucking et al., 2014). The impact of such an issue can be highly dependent on the project team and is likely to influence various aspects of the energy performance of the building.
Complexity of Design
Complexity of design can introduce problems during building construction, affecting building performance. For example, mistakes in construction become more frequent and complex systems are less well understood (Bunn and Burman, 2015). Simplicity should be the aim of the design as many of the underlying issues are related to the complexity of the building (Williamson, 2012).
Uncertainty in Building Energy Modeling
In the detailed design stage, building energy modeling requires a high level of detail in order predict energy use of a building. Myriad parameters with a certain level of uncertainty can have a large effect on the final performance due to the aggregated effect of uncertainties. Among uncertainties in design, those related to natural variability, such as material properties are relatively well covered (de Wit and Augenbroe, 2002). Other uncertainties are less well understood and need a strong basis for research to be established in modeling procedures. Investigation toward well-defined assumptions can assist in more accurately and confidently predict performance of a building (Heidarinejad et al., 2013). Different sources of uncertainty exist in the use of building simulation. de Wit (2001) classified specification, modeling, numerical, and scenario uncertainties, where heuristic uncertainty has been added to describe human-introduced errors as reported by Kim and Augenbroe (2013).
Specification Uncertainty
Specification uncertainty arises from incomplete or inaccurate specification of the building or systems modeled. This refers to the lack of information on the exact properties and may include model parameters, such as geometry, material properties, HVAC specifications, plant and system schedules, and casual gains. Parameters related to specification uncertainty are often “highly unknown” during the early design stage and can have a large effect on the predicted energy use, assumptions for such parameters are often not representative of actual values in operation. For example, Burman et al. (2012) identified that the values assumed for specific fan powers were often much lower than in operation. Similarly, Salehi et al. (2013) identified that underlying equipment and lighting loads were significantly underestimated.
Modeling Uncertainty
Modeling uncertainty arises from simplifications introduced in the development of the model. These include system simplification, zoning, stochastic process scheduling, and also calculation algorithms. Wetter (2011) asserts that mechanical systems and their control systems are often so simplified that they do not capture dynamic behavior and part-load operation of the mechanical system or the response of feedback control systems. This is further supported by Salehi et al. (2013) who were unable to model the unconventional heating system of a building in utilized modeling software, which may lead to wrong performance prediction. Also, Burman et al. (2012) found that pumps’ auxiliary power could not be modeled for compliance purposes and had to apply default values based on HVAC system type. Such tool limitations are extensively reported and contrasted and also highlight that certain systems and its configurations are not supported by building simulation software (Crawley et al., 2005).
Numerical Uncertainty
Errors introduced in the discretization and simulation of the model. Neymark et al. (2002) performed a comparative analysis of whole-building simulation programs and found that prominent bugs and faulty algorithms caused errors of up to 20–45% in predicting energy consumption or COP values.
Scenario Uncertainty
Uncertainty related to the external environment of a system and its effects on the system. The specification of weather, building operation, and occupant behavior is in the design model. Accuracy of design weather data can have a large effect on the predicted energy performance of a building. According to Bhandari et al. (2012), the predicted annual building energy consumption can vary up to 7% as a function of the provided location’s weather data. While Wang et al. (2012a) showed that the impact of year-to-year weather fluctuation on the energy use of a building ranges from −4 to 6%. Knowing the uncertainty of related microclimate variables is necessary to understand its impact on energy prediction (Sun et al., 2014). Similarly, occupants are an uncertainty external to the system and play a major role in the operation of a building. Occupants operate the building through adjusting lighting levels, operate electrical devices, open windows, and possibly control HVAC operations. In design calculations, occupancy is normally accounted for through a fraction profile, which determines their presence in the model and separately determines when they can operate building equipment. This profile is simplified by taking the average behavior of the occupants, and therefore, neglects temporal variations and atypical behavior (Kim and Augenbroe, 2013). Furthermore, occupant effects are related to specification uncertainty through assumed base loads (e.g., lighting and equipment), which make it difficult to determine how occupant profiles or wrong base load assumptions impact the energy performance. Murphy and Castleton (2014) identified lower lighting energy use than predicted in their case study building due to unanticipated unoccupied hours.
Heuristic Uncertainty
Human-introduced error is in the form of modeler’s bias or mistakes. User errors are inevitably quite common due to the complexity of building energy simulation and its tools, these errors range from modelers setting up a building system in different ways, forgetting to correctly apply operation or occupancy profiles to the correct zones or can be related to geometry creation. Some of these errors can have a negligible effect on the predicted energy use, whereas others can significantly change the final outcome. Guyon (1997) investigated the influence of 12 energy modelers on prediction of energy consumption of a residential house and found a 40% variability in their final predictions. A similar observation was made by the building research establishment (BRE) where 25 users predicted the energy consumption of a large complex building and found that their results varied from −46 to +106% (Bloomfied, 1988). Although these studies show significant differences, not much evidence is available to further support these results.
Inter-Model Variability
Energy use prediction is performed using different tools, developed in different countries, for different reasons and as such introduce variability in the results when modeling the same building, i.e., inter-model variability. This is directly related to uncertainties in building energy simulation; model simplification, user error, and numerical uncertainties will drive the variability between different tools. These tools are utilized for the purpose of building performance prediction and thus have to give credible and relatively accurate results. Raslan and Davies (2010) compared 13 different accredited software tools and highlight a large degree of variability in the results produced by each of the tools and consistency in achieving compliance with the building regulations for the same building. In a more recent study, Schwartz and Raslan (2013) performed an inter-model comparative analysis of three different dynamic simulation tools using a single case study and found a 35% variability in the total energy consumption. Similarly, Neymark et al. (2002) compared seven different tools and indicated a 4–40% disagreement in energy consumption.
On-site Workmanship
As building regulations become more stringent and new technologies are introduced, the quality of construction has to be improved. On-site workmanship needs to adapt and be trained to these increasing levels of complexity in building construction. New skills such as extreme air tightness for limiting air infiltration give rise to performance issues as air tightness is compromised during construction by discontinuous insulation or punctured airtight barriers (Williamson, 2012), whereas Olivier (2001) reports that UK figures for construction U-values are optimistic. Installation of services, such as drainage, air ducts, and electrical pipe work, can often leave gaps that also reduce air tightness and induce thermal loss (Morant, 2012). Other common issues related to on-site workmanship are eaves to wall junction insulation, incorrect positioning of windows and doors that reduce the actual performance of the thermal envelope (ZCH, 2014a,b). These issues are more prone to affect the energy performance in domestic buildings, where usually the performance of the thermal envelope is more significant.
Changes after Design
During building design and construction, often products or changes are value engineered, affecting building performance, while not being fed back to the design team for evaluation against the required performance standard (ZCH, 2014a,b). These changes can occur due to site constraints, not well thought of integration of design modules problems with detailing and budget issues. Morant (2012) reported inconsistencies between design specified and installed lighting loads in an office, which had a considerable impact on the discrepancy between predicted and measured electricity use. Good communication and coordination by the contractor are essential to prevent changes in design changes to influence the energy performance.
Poor Commissioning
When a building is constructed, it is handed over, a separate stage that includes the installation and commissioning of building services, done poorly this results in reduced system efficiency and compromising the air tightness and ventilation strategies. Piette et al. (1994) and Pang et al. (2012) reported poor commissioning of control measures, which were not set up for proper control, and operation. Kimpian et al. (2014) identified that inverters for supply and extract fans were provided to AHUs but were not enabled during commissioning, resulting in fans operating at maximum speed at all times. In operation, such issues persist and require continuous commissioning.
Poor Practice and Malfunctioning Equipment
The actual operation of a building is idealized during design by making assumptions for temperature set-points, control schedules, and general performance of HVAC systems. In practice, however, it is often the case that many of these assumptions deviate and directly influence a building’s energy use. Kleber and Wagner (2007) monitored an office building and found that failures in operating the building’s facilities caused higher energy consumption, and they underline the importance of continuous monitoring of a building. Wang et al. (2012a) showed that poor practice in building operations across multiple parameters results in an increase in energy use of 49–79%, while good practice reduces energy consumption by 15–29%. Piette et al. (1994) suggest that building operators do not necessarily possess the appropriate data, information, and tools needed to provide optimal results. As such, operational assumptions made in the design stage may not be met by building operators (Moezzi et al., 2013).
Occupant Behavior
Another dynamic factor for a building in-use is occupants. They have a substantial influence on the energy performance of a building by handling controls, such as those for lighting, sun shading, windows, set-points, and office equipment, and also through their presence, which may deviate from assumed schedules. People are very different in their behavior through culture, upbringing and education, making their influence on energy consumption highly variable. One of the major factors that has been reported to have a large influence on the discrepancy between predicted and measured energy use is the issue of night-time energy use related to leaving office equipment on (Kawamoto et al., 2004; Masoso and Grobler, 2010; Zhang et al., 2011; Mulville et al., 2014), this can be both related to occupant behavior (not turning off equipment) and assumptions for operational schedules, extended working hours not taken into account in the design model. In an uncontrolled environment (not extensively monitored), it is impossible to determine how one or the other is influencing the discrepancy. Azar and Menassa (2012) investigated 30 typical office buildings and found that occupancy behavioral parameters significantly influence energy use. Parys et al. (2010) reported a SD of up to 10% on energy use to be related to occupant behavior. A more significant value is reported by Martani et al. (2012) who studied two buildings and found a 63 and 69% variation in electricity consumption due to occupant behavior. Using modeling, Hong and Lin (2013) investigated different work styles in an office space and found that an austere work style consumes up to 50% less energy, whereas a wasteful work style consumed 90% more energy than typical behavior. Such work styles were defined by modeling parameters for schedules and loads that people have an influence on.
Measurement System Limitations
Similar to predicting energy use using building energy models, metered energy use obtained from measurement systems needs to be validated to ensure accuracy of the data. Limitations of measurement systems make adequate assessment of energy use inaccurate (Maile, 2010). For energy measurement system, the accuracy is the sum of all its components and has an error percentage of up to about 1% (IEC, 2003). For monitoring environmental variables, typical sensor accuracies lie within 1–5% for normal operating conditions, whereas incorrectly placed sensors will have increased levels of error (Maile et al., 2010). Most common sources are calibration errors, or the absence of calibration (Palmer and Armitage, 2014). Fedoruk et al. (2015) identified that system measurements were not accurately representing its performance due to mislabeling, incorrect installation, and not being calibrated. They report that simply having access to large amounts of data may actually result in more confusion and operational problems.
Longitudinal Variability in Operation
Finally, commonly the energy performance gap is generally assessed for a year of measured data. However, longitudinal performance is affected by factors such as building occupancy, deterioration of physical elements, climatic conditions, and building maintenance processes and policies (de Wilde et al., 2011). Brown et al. (2010) present a longitudinal analysis of 25 buildings in the UK and found an increase of 9% in energy use on average per year over 7 years, with a SD of 18%. Similarly, Piette et al. (1994) analyzed 28 buildings in the US and found an average increase of 6% between the third and fourth year, with no average increase during the fifth year. Thus, a longitudinal variability in operational energy use has to be taken into account when investigating the energy performance gap. It should be noted here that longitudinal variability can be related to many of the previous factors mentioned; also, sometimes such increase in energy use is related to an expected increase of equipment loads or changes in building function.
Assessing the Underlying Causes
All of these causes combined can have a large influence on the final energy performance of a building. Table 3 shows a risk matrix that defines the potential-associated risks of the discussed underlying causes based on general consensus in the literature. An overview of several of the reviewed case studies and their reported underlying reasons for a discrepancy are given in the Supplementary Material.
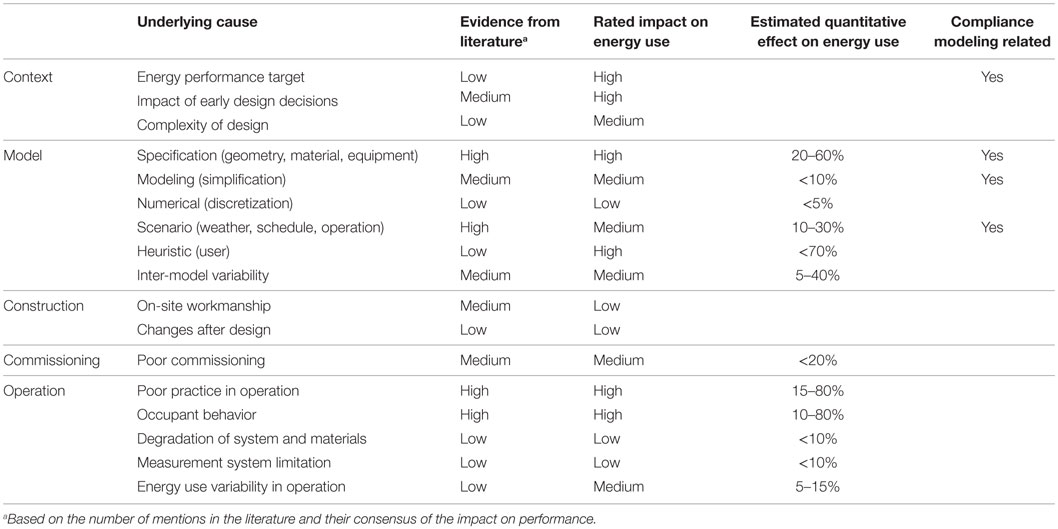
Table 3. Potential risk on energy use from reported underlying causes assessed based on general consensus in the literature.
Important underlying causes identified in the literature are those that a high impact and high evidence rating. These are specifically related to specification uncertainty in building modeling, occupant behavior, and poor practice in operation, with an estimated effect of 20–60, 10–80, and 15–80% on energy use, respectively. Other important factors that are likely to have a high-rated impact are the energy performance target, impact of early design decisions and heuristic uncertainty in modeling.
An assessment of the underlying causes of the energy performance gap has shown that there is a need for both action and further research to be undertaken. Detailed building prediction methods and post-occupancy evaluation have proven to be essential in the understanding of building assumptions, occupant behavior, systems, and the discrepancy between predicted and measured energy use.
Reducing the Energy Performance Gap
A major concern in the built environment is the segmentation of disciplines involved in the building life cycle stages. Traditionally, designers, engineers, and contractors are all involved in the building development process, but leave once the building is physically complete, leaving the end-users with a building they are unlikely to fully understand. The design community rarely goes back to see how buildings perform after they have been constructed (Torcellini et al., 2006). Feedback mechanisms on energy performance are not well developed, and it is generally assumed that buildings perform as designed, consequently there is little understanding of what works and what does not, which makes it difficult to continuously improve performance (ZCH and NHBC Foundation, 2010). Gathering more evidence on both the performance gap and its underlying issues can support feedback mechanisms and prioritize principle issues. For this, the primary requirement is the collection of operational performance data, which can be fed back to design teams to ensure lessons are learnt and issues are avoided in future designs. It can help policy-makers understand the trend of energy use and support the development of regulations. Finally, operational data are valuable to facilities management in order to efficiently operate the building. This feedback process is illustrated in Figure 5.
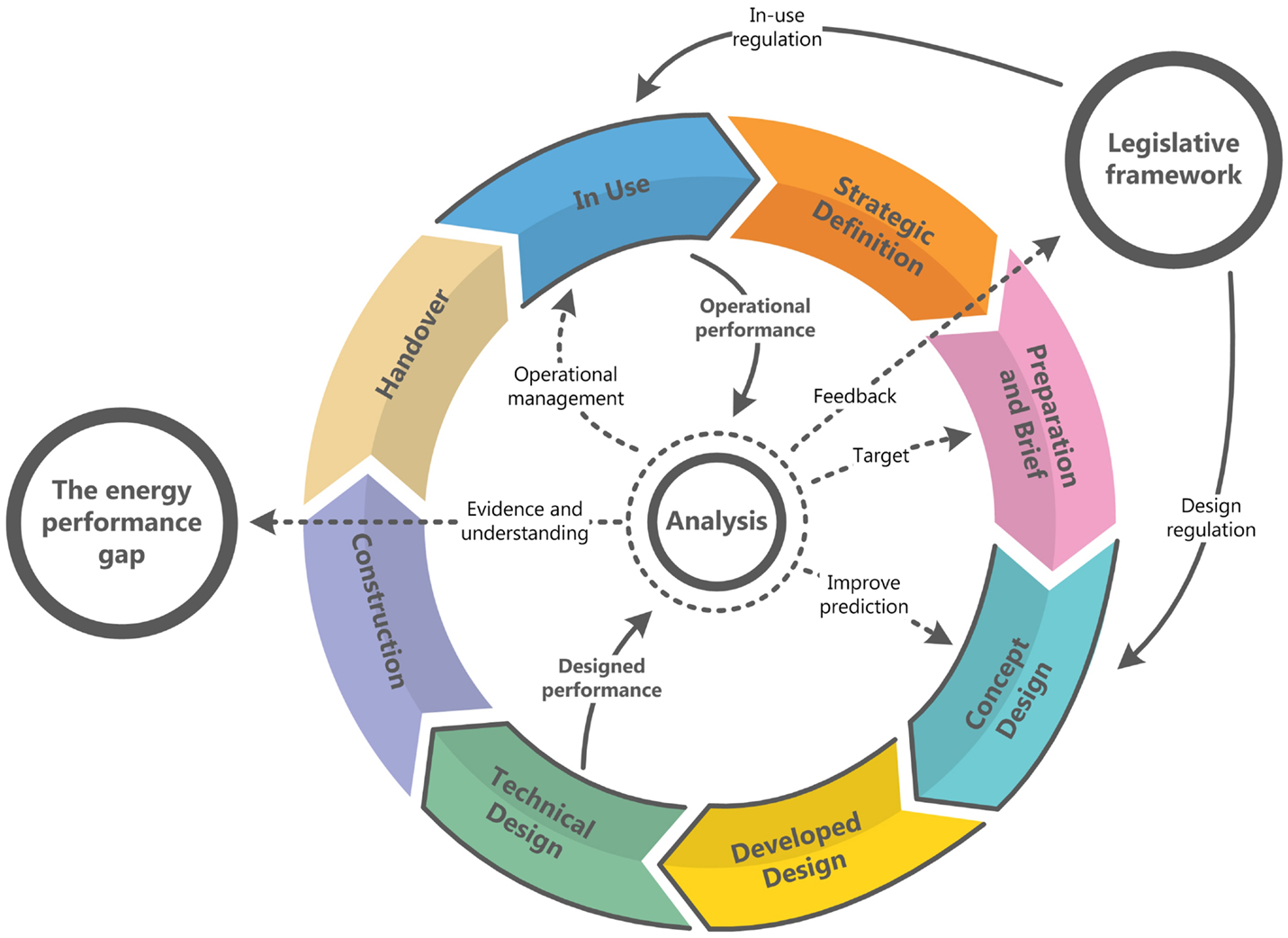
Figure 5. Feedback process in relation to the RIBA plan of work stages [adapted from RIBA (2013)].
Legislative Frameworks
Recently, the UK department of Energy and Climate Change introduced the energy-saving opportunity scheme (ESOS) in order to promote operational management in buildings. A mandatory energy assessment to identify energy savings in corporate undertakings that either employ more than 250 or have an annual turnover in excess of ~38 million pounds (50 million Euros). An assessor should calculate how much can be saved from improved efficiency. How these savings are predicted is, however, left open and could entail simple hand calculations instead of the more detailed dynamic thermal simulations. Furthermore, implementing proposed energy savings is voluntary. In the same context, energy performance contracts are legally binding a third party for predicted savings to be realized, otherwise equivalent compensation needs to be provided. It thus becomes important to make accurate predictions of energy-saving measures as their reliability directly influences the profit of the businesses providing these contracts. Therefore, building energy modeling is normally applied in order to take into account all aspects of energy use. Typically, performance modeling is supported by measured data to make such predictions. The EU energy efficiency directive calls for a need to remove regulatory and non-regulatory barriers to the use of energy performance contracting to stimulate its use as an effective measure to improve efficiency of the existing building stock (European Parliament and Council, 2012).
For new buildings, regulatory limits become ever more stringent in order to mitigate climate change. Evidently, these regulatory limits are not achieved in practice, which can foster a lack of confidence in simulation and may soon be met by legal and financial implications (Daly et al., 2014). Burman et al. (2014) propose a framework that would enable effective measurement of any excess in energy use over the regulatory limit set out for a building. This excess in energy use could cause disproportionate environmental damage and it could be argued that it should be charged at a different rate or be subject to an environmental tax. Kimpian et al. (2014) suggest mandating the disclosure of design stage calculations and assumptions as well as operational energy use outcomes in building regulations, such data would significantly support the understanding of the energy performance gap. Furthermore, addressing all aspects of energy use beyond regulated energy use for compliance purposes would resolve the regulatory performance gap. However, such changes would make it difficult for regulators to assess energy efficiency of buildings under standardized conditions. Governments continue to face the difficult task of balancing the principal of not interfering in the affairs of businesses with the recognition of serious consequences of energy waste and climate change (Jonlin, 2014).
Data Collection
Accessible meter data are mandatory to confirm that buildings really do achieve their designed and approved goals (UKGBC, 2007). In the European Union, automatic meter readings (AMR) are now widely used to record such data sub-hourly. However, a lack of meter data is likely to lead to a progressive widening of the gap between predicted and measured energy use (Oreszczyn and Lowe, 2009). Energy performance data can be used by design teams to enable them to deliver better designs, clients to enable benchmarking and develop a lower carbon building brief, building users to drive change and management in operation, policy-makers to target plans and incentives and monitor the trend of energy use (Government HM, 2010). To this end, sub-metering is now mandatory in the UK for new builds. It is common, however, to find meters installed, but not properly commissioned and validated, which can make data futile (Austin, 2013). Without data collection there would be no feedback loop to inform future policy and regulation (ZCH, 2010).
Data itself do not solve any of the underlying issues of the energy performance gap as it is not directly visible how a building is working. It needs to be clear what this data represent. The issue of determining where errors exist between measured and simulated performance is simple when using monthly or diurnal plots. At hourly levels, many of the traditional graphical techniques become overwhelmed with too many data points, making it difficult to determine the tendency of black clouds of data points (Haberl and Bou-Saada, 1998; Coakley et al., 2011). Tools to support intuitive visualization are needed to disaggregate and display energy uses at detailed levels (building, sub-zone, system) and for different time granularity (yearly, monthly, weekly, and sub-hourly) comparing predicted and measured energy use taking a longitudinal approach.
Design Improvements
Negating the performance gap starts at the beginning of a project. At this point, it is important to set a stringent energy performance in-use target, which can assist in a more rigorous review of system specifications and operational risks (Kimpian et al., 2014). With such in-use expectations, it becomes necessary to carry out performance modeling, validate assumptions made in the building model, make sure that building fabric is constructed to a high standard, systems are properly commissioned and that the building is operated as efficiently and effectively as possible. Making an accurate prediction of building energy performance then becomes an integral part of the design process. A building design, however, is based on thousands of input parameters, often obtained from guidelines or building regulations, some of which have extensive background research, while others are only best-guess values. In particular, during the early design stage, these values have a major influence on the design and its final performance. Pegg et al. (2007) argue for the use of feedback to inform design and need for realistic and relevant benchmarks. Mahdavi and Pröglhöf (2009) suggest the collection of occupancy behavior information to derive generalized models and utilize such models in building energy simulation. Capturing user-based control actions and generalizing these as simulation inputs can provide more accuracy in performance modeling predictions, and ideally such results are fed back to improve compliance modeling processes as well. Menezes et al. (2012) used basic monitoring results to feed into energy models in order to gain a more accurate prediction of a building’s actual performance (within 3% of actual consumption for a specific study). Similarly, Daly et al. (2014) showed the importance of using accurate assumption in building performance simulation, and identified the risks associated with such assumptions. They examined the sensitivity of assumptions on predicted energy use by using high and low assumptions and found that payback periods of simple retrofits could vary by several years depending on the simulation assumptions used.
Continuous feedback can improve the design process and more accurately predict actual in-use performance. Such predictions can be further supported by introducing well-defined uncertainties in design, improving the robustness of the building design, reliability of energy simulation, and enable design decision support, in particular when supported by sensitivity analysis (Hopfe and Hensen, 2011).
Training and Education
Often the real performance of building elements are underestimated, as they are taken from lab tests and omit, for example, the occurrence of thermal bridging during construction, which are more common with a higher design complexity. During construction, robust checking and testing is necessary to ensure that the quality of construction is maintained (Morant, 2012). Clear guidance on thermal bridging should therefore be provided to the construction industry (ZCH, 2014a,b). Training and education are needed to increase skills in the construction industry and ensure better communication and quality of construction. Similarly, training and education should be enhanced for facility managers, to more strictly perform maintenance and operation of buildings. Whereas in the design stage, it is important to create awareness to energy modelers of the energy performance discrepancy, while promoting skills, innovation, and technological development in order to deal more appropriately with creating a robust design.
Operational Management
After construction of a building, its systems are commissioned in order to perform as expected. However, post-occupancy evaluation has shown that this is often poorly done and that there is a lack of fine-tuning during operation (Kimpian et al., 2014). Frequent re-commissioning exercises can help maximize the efficiency of building services, avoiding unnecessary energy use (Morant, 2012). For guidance in this process, the soft landings framework was developed in order to provide extended aftercare, through monitoring, performance reviews and feedback. Aftercare and professional assistance are required as technologies and solutions made during the design often prove too complicated to be manageable (Way et al., 2014). Continual monitoring of the performance during operation is thus important in order to ensure that design goals are met under normal operating conditions (Torcellini et al., 2006). It is essential that facilities managers take ownership of energy consumption in buildings as they have detailed information of operational issues (CIBSE, 2015).
When a building is in-use, a discrepancy between predicted and measured energy use can be identified by representing the operation using advanced and well-documented simulation tools. A calibrated energy model can pinpoint differences between how a building was designed to perform and how it is actually functioning (Norford et al., 1994), this can then allow operational issues to be identified and solved, assisting facilities managers in the operation of their building. Furthermore, it can be used to assess the feasibility of energy conservation measures (ECMs) through forecasting energy savings (Raftery et al., 2011). However, model calibration intends to compensate errors that can mask modeling inaccuracies at the whole-building level (Clarke, 2001). A well set up methodology should therefore be established. Maile et al. (2012) developed such a method using a formal representation of building objects to capture relationships between predicted and measured energy use on a detailed level and were able to identify and solve operational issues. Raftery et al. (2011) argue that these calibration methods improve the quality of future models by identifying common mistaken assumptions and by developing best-practice modeling approaches. Reliability and accuracy of calibrated models depend on the quality of measured data used to create the model as well as the accuracy and limitation of the tools used to simulate the building and its systems (Coakley et al., 2012). In addition, there are often many constraints to going back to the building in order to make it more efficient, such as cost, reputational concerns, and liability (Robertson and Mumovic, 2013). A review of and methodology for calibration techniques are presented by Coakley et al. (2014) and Raftery et al. (2011), respectively.
Conclusion
Predicted and measured energy use have been shown to deviate significantly, also termed “the performance gap.” This paper classifies this gap as either a regulatory, static, or dynamic performance gap. Their differences are explained by the underlying approach taken to predict energy use and its comparison with measured energy use. The significance of the regulatory energy performance gap and its underlying causes have been analyzed:
• From 62 case study buildings, the average discrepancy between predicted and measured energy use is +34%, with a SD of 55%. These studies include a prediction of equipment energy use.
• The most important underlying causes identified in the literature are specification uncertainty in building modeling, occupant behavior, and poor practice in operation, with an estimated effect of 20–60, 10–80, and 15–80% on energy use, respectively. Other important factors are the energy performance target, impact of early design decisions, heuristic uncertainty in modeling.
Understanding and mitigating differences between predicted and measured energy use require an expansion of research efforts and focus on underlying causes that have a medium to high impact on energy use. Detailed energy audits and model calibration are invaluable techniques in order to quantify these causes. Furthermore, tools are necessary to support intuitive visualization and data disaggregation to display energy uses at detailed levels and for different time granularity comparing predicted and measured energy use taking a longitudinal approach. To successfully reduce the energy performance gap, key measures for further work and research by the building industry need to be established:
(1) Accessible energy data are required for a continued gathering of evidence on the energy performance gap, and this can be established through collaborative data gathering platforms, such as CarbonBuzz.
(2) Legislative frameworks set limits for predicted performance and penalize buildings for high operational energy use. More effectively, however, governments should relate predicted to measured performance through predictive modeling and in-use regulation. Furthermore, it should consider mandating the disclosure of design stage calculations and assumptions as well as operational energy use outcomes in building regulations.
(3) Monitoring and data analysis of operational building performance are imperative to driving change and management in operation. While well-defined assumptions need to be established through detailed calibration studies identifying the driving factors of energy use in buildings.
Author Contributions
CD, the main author, is a doctoral researcher and is the main contributor to this review paper, having done the literature research and writing. MD is CD’s industrial supervisor during his doctoral studies and has reviewed the paper and provided feedback for initial revision. EB is CD’s academic supervisor during his doctoral studies and has reviewed the paper and provided feedback on the work, including direct insights from his own work. CS is CD’s secondary academic supervisor during his doctoral studies and has reviewed the paper and provided feedback for initial revision. DM is CD’s first academic supervisor during his doctoral studies and has reviewed the paper and provided feedback for initial revision; DM is an Associate Editor for the HVAC Journal.
Conflict of Interest Statement
The authors declare that the research was conducted in the absence of any commercial or financial relationships that could be construed as a potential conflict of interest.
Funding
This research has been made possible through funding provided by the Engineering and Physical Sciences Research Council (EPSRC) and BuroHappold Engineering.
Supplementary Material
The Supplementary Material for this article can be found online at http://journal.frontiersin.org//article/10.3389/fmech.2015.00017
References
Ahmed, M., and Culp, C. H. (2006). Uncalibrated building energy simulation modeling results. HVAC&R Res. 12, 1141–1155. doi: 10.1080/10789669.2006.10391455
ASHRAE. (2004). ASHRAE Standard 90.1: Energy Standard for Buildings Except Low-Rise Residential Buildings. Atlanta, GA: American Society of Heating, Refrigerating and Air-Conditioning Engineers, Inc.
Austin, B. (2013). The Performance Gap – Causes and Solutions. London: Green Construction Board – Buildings Working Group.
Azar, E., and Menassa, C. C. (2012). A comprehensive analysis of the impact of occupancy parameters in energy simulation of office buildings. Energy Build. 55, 841–853. doi:10.1016/j.enbuild.2012.10.002
Bertagnolio, S., Randaxhe, F., and Lemort, V. (2012). “Evidence-based calibration of a building energy simulation model: application to an office building in Belgium,” in Proceedings of the Twelfth International Conference for Enhanced Building Operations (Manchester).
Bhandari, M., Shrestha, S., and New, J. (2012). Evaluation of weather datasets for building energy simulation. Energy Build. 49, 109–118. doi:10.1016/j.enbuild.2012.01.033
Bloomfied, D. P. (1988). An Investigation into Analytical and Empricial Validation Techniques for Dynamic Thermal Models of Buildings. London: BRE (Building Research Establishment).
Bordass, B., Cohen, R., Standeven, M., and Leaman, A. (2001). Assessing building performance in use 3: energy performance of the probe buildings. Build. Res. Inform. 29, 114–128. doi:10.1080/09613210010008027
Brown, N., Wright, A. J., Shukla, A., and Stuart, G. (2010). Longitudinal analysis of energy metering data from non-domestic buildings. Build. Res. Inform. 38, 80–91. doi:10.1080/09613210903374788
Bucking, S., Zmeureanu, R., and Athienitis, A. (2014). A methodology for identifying the influence of design variations on building energy performance. J. Build. Perform. Simul. 7, 411–426. doi:10.1080/19401493.2013.863383
Bunn, R., and Burman, E. (2015). “S-curves to model and visualise the energy performance gap between design and reality – first steps to a practical tool,” in CIBSE Technical Symposium (London).
Burman, E. (2016). Assessing the Operational Performance of Educational Buildings against Design Expectations – A Case Study Approach. Doctoral thesis, UCL (University College London), London.
Burman, E., Mumovic, D., and Kimpian, J. (2014). Towards measurement and verification of energy performance under the framework of the European directive for energy performance of buildings. Energy 77, 153–163. doi:10.1016/j.energy.2014.05.102
Burman, E., Rigamonti, D., Kimpain, J., and Mumovic, D. (2012). “Performance gap and thermal modelling: a comparison of simulation results and actual energy performance for an academy in North West England,” in First Building Simulation and Optimization Conference (London: Loughborough).
Calderone, A. (2011). “Simulation versus reality – 4 case studies,” in 12th Conference of International Building Performance Simulation Association, 14-16 November (Sydney, NSW).
Carbon Trust. (2012). Closing the Gap – Lesson Learned on Realising the Potential of Low Carbon Building Design. London: The Carbon Trust
CarbonBuzz. (2015). CarbonBuzz. Available at: www.carbonbuzz.org
CIBSE. (2013). CIBSE TM54: Evaluating Operational Energy Performance of Buildings at the Design Stage. London: The Chartered Institution of Building Services Engineers.
CIBSE. (2015). CIBSE TM57: Integrated School Design. Guide. London: The Chartered Institution of Building Services Engineers.
Coakley, D., Raftery, P., and Keane, M. (2014). A review of methods to match building energy simulation models to measured data. Renew. Sustain. Energ. Rev. 37, 123–141. doi:10.1016/j.rser.2014.05.007
Coakley, D., Raftery, P., and Molloy, P. (2012). “Calibration of whole building energy simulation models: detailed case study of a naturally ventilated building using hourly measured data,” in First Building Simulation and Optimization Conference (Loughborough).
Coakley, D., Raftery, P., Molloy, P., and White, G. (2011). Calibration of a Detailed BES Model to Measured Data Using an Evidence-Based Analytical Optimisation Approach. Galway: Irish Research Unit for Sustainable Energy (IRUSE).
Cohen, R., and Bordass, B. (2015). Mandating transparency about building energy performance in use. Build. Res. Inform. 43, 534–552. doi:10.1080/09613218.2015.1017416
Crawley, D. B., Hand, J. W., and Kummert, M. (2005). Contrasting the Capabilities of Building Energy Performance Simulation Programs. Technical Report. Washington, DC: U.S. Department of Energy, University of Strathclyde, University of Wisconsin.
Daly, D., Cooper, P., and Ma, Z. (2014). Understanding the risks and uncertainties introduced by common assumptions in energy simulations for Australian commercial buildings. Energy Build. 75, 382–393. doi:10.1016/j.enbuild.2014.02.028
de Wilde, P. (2014). The gap between predicted and measured energy performance of buildings: a framework for investigation. Autom. Constr. 41, 40–49. doi:10.1016/j.autcon.2014.02.009
de Wilde, P., Tian, W., and Augenbroe, G. (2011). Longitudinal prediction of the operational energy use of buildings. Build. Environ. 46, 1670–1680. doi:10.1016/j.buildenv.2011.02.006
de Wit, S., and Augenbroe, G. (2002). Analysis of uncertainty in building design evaluations and its implications. Energy Build. 34, 9510958. doi:10.1016/S0378-7788(02)00070-1
de Wit, S. M. (2001). Uncertainty in Predictions of Thermal Comfort in Buildings. PhD Thesis. Delft: Technische Universiteit Delft.
Diamond, R. Berkeley, L., Opitz, M., Hicks, T., Von Neida, B., and Herrera, S. (2006). Evaluating the Energy Performance of the First Generation of LEED-Certified Commercial Buildings. Proceedings of the 2006 American Council for an Energy-Efficient Economy Summer Study on Energy Efficiency in Buildings. Berkeley, CA.
European Commission. (2011). Energy Roadmap 2050 – Communication from the Commission to the European Parliament, the Council, the European Economic and Social Committee and the Committee of the Regions. Brussels.
European Parliament and Council. (2012). Directive 2012/27/EU of the European parliament and of the council. Off. J. Eur. Union.
Fedoruk, L. E., Cole, R. J., Robinson, J. B., and Cayuela, A. (2015). Learning from failure: understanding the anticipated-achieved building energy performance gap. Build. Res. Inform. 43, 750–763. doi:10.1080/09613218.2015.1036227
Fowler, K., Rauch, E., Henderson, J., and Kora, A. (2010). Re-Assessing Green Building Performance: A Post Occupancy Evaluation of 22 GSA Buildings. Richland: Pacific Northwest National Laboratory.
Guyon, G. (1997). Role of the Model User in Results Obtained From Simulation Software Program. Moret-sur-Loing: International Building Performance Simulation Association.
Haberl, J. S., and Bou-Saada, T. E. (1998). Procedures for calibrating hourly simulation models to measured building energy and environmental data. ASME J. Solar Energy Eng. 120, 193–204. doi:10.1115/1.2888069
Heidarinejad, M., Dahlhausen, M., McMahon, S., Pyke, C., and Srebric, J. (2013). “Building classification based on simulated annual results: towards realistic building performance expectations,” in 13th Conference of International Building Performance Simulation Association (Chambéry).
Heo, Y., Choudhary, R., and Augenbroe, G. A. (2012). Calibration of building energy models for retrofit analysis under uncertainty. Energy Build. 47, 550–560. doi:10.1016/j.enbuild.2011.12.029
Hong, T., and Lin, H. (2013). Occupant Behavior: Impact on Energy Use of Private Offices. Report. Berkeley, CA: Ernest Orlando Lawrence Berkeley National Laboratory.
Hopfe, C. J., and Hensen, J. L. M. (2011). Uncertainty analysis in building performance simulation for design support. Energy Build. 43, 2798–2805. doi:10.1016/j.enbuild.2011.06.034
IEC. (2003). IEC Standard Electricity Metering Equipment – Static Meters for Active Energy – Part 21 and 22. Geneva: International Electrotechnical Commission.
Jonlin, D. (2014). “Bridging the energy performance gap: real-world tools,” in ACCEEE Summer Study on Energy Efficiency in Buildings. Pacific Grove, CA.
Kawamoto, K., Shimoda, Y., and Mizuno, M. (2004). Energy saving potential of office equipment power management. Energy Build. 36, 915–923. doi:10.1016/j.enbuild.2004.02.004
Kim, S. H., and Augenbroe, G. (2013). Uncertainty in developing supervisory demand-side controls in buildings: a framework and guidance. Autom. Constr. 35, 28–43. doi:10.1016/j.autcon.2013.02.001
Kimpian, J., Burman, E., Bull, J., Paterson, G., and Mumovic, D. (2014). “Getting real about energy use in non-domestic buildings,” in CIBSE ASHRAE Technical Symposium (Dublin).
Kleber, M., and Wagner, A. (2007). “Results of monitoring a naturally ventilated and passively cooled office building in Frankfurt a.M., Germany,” in Proceedings of Clima 2007 Wellbeing Indoors. Karlsruhe.
Knight, I., Stravoravdis, S., and Lasvaux, S. (2008). Predicting operational energy consumption profiles – finding from detailed surveys and modelling in a UK educational building compared to measured consumption. Int. J. Ventil. 7, 49–57. doi:10.5555/ijov.2008.7.1.49
Korjenic, A., and Bednar, T. (2012). Validation and evaluation of total energy use in office buildings: a case study. Autom. Constr. 23, 64–70. doi:10.1016/j.autcon.2012.01.001
Mahdavi, A., and Pröglhöf, C. (2009). “User behavior and energy performance in buildings,” in Internationalen Energiewirtschaftstagung an der TU Wien (IEWT 2009). Vienna.
Maile, T. (2010). Comparing Measured and Simulated Building Energy Performance Data. PhD Thesis. Stanford, CA: Stanford University.
Maile, T., Bazjanac, V., and Fischer, M. (2012). A method to compare simulated and measured data to assess building energy performance. Build. Environ. 56, 241–251. doi:10.1016/j.buildenv.2012.03.012
Maile, T., Fischer, M., and Bazjanac, V. (2010). Formalizing Assumptions to Document Limitations of Building Performance Measurement Systems. Stanford, CA: Stanford University.
Manfren, M., Aste, N., and Moshksar, R. (2013). Calibration and uncertainty analysis for computer models – a meta-model based approach for integrated building energy simulation. Appl. Energy 103, 627–641. doi:10.1016/j.apenergy.2012.10.031
Martani, C., Lee, D., Robison, P., Britter, R., and Ratti, C. (2012). ENERNET: studying the dynamic relationship between building occupancy and energy consumption. Energy Build. 47, 584–591. doi:10.1016/j.enbuild.2011.12.037
Masoso, O. T., and Grobler, L. J. (2010). The dark side of occupants’ behaviour on building energy use. Energy Build. 42, 173–177. doi:10.1016/j.enbuild.2009.08.009
Menezes, A. C., Cripps, A., Bouchlaghem, D., and Buswell, R. (2012). Predicted vs. actual energy performance of non-domestic buildings: using post-occupancy evaluation data to reduce the performance gap. Appl. Energy 97, 355–364. doi:10.1016/j.apenergy.2011.11.075
Moezzi, M., Hammer, C., Goins, J., and Meier, A. (2013). Behavioral Strategies to Bridge the Gap Between Potential and Actual Savings in Commercial Buildings. Berkeley, CA: Air Resources Board.
Morant, M. (2012). The Performance Gap – Non Domestic Building: Final Report (CEW1005). Cardiff: AECOM/Constructing Excellence Wales.
Mulville, M., Jones, K., and Heubner, G. (2014). The potential for energy reduction in UK commercial offices through effective management and behaviour change. Archit. Eng. Des. Manag. 10, 79–90. doi:10.1080/17452007.2013.837250
Murphy, E., and Castleton, H. (2014). “Predicting the energy performance of a new low carbon office building: a case study approach,” in Second IBPSA – England Conference – Building Simulation and Optimization (London).
Neymark, J., Judkoff, R., Knabe, G., Le, H.-T., Dürig, M., Glass, A., et al. (2002). Applying the building energy simulation test (BESTEST) diagnostic method to verification of space conditioning equipment models used in whole-building energy simulation programs. Energy Build. 34, 917–931. doi:10.1016/S0378-7788(02)00072-5
Norford, L. K., Socolow, R. H., Hsieh, E. S., and Spadaro, G. V. (1994). Two-to-one discrepancy between measured and predicted performance of a ‘low-energy’ office building: insights from a reconciliation based on the DOE-2 model. Energy Build. 21, 121–131. doi:10.1016/0378-7788(94)90005-1
Olivier, D. (2001). Building in Ignorance – Demolising Complacency: Improving the Energy Performance of 21st Century Homes. Leominster: Report. Association for the Conservation of Energy.
Oreszczyn, T., and Lowe, R. (2009). Challenges for energy and buildings research: objectives, methods and funding mechanisms. Build. Res. Inform. 38, 107–122. doi:10.1080/09613210903265432
Palmer, J., and Armitage, P. (2014). Building Performance Evaluation Programme – Early Findings from Non-Domestic Projects. Report. London: Innovate UK.
Pan, Y., Huang, Z., and Wu, G. (2007). Calibrated building energy simulation and its application in a high-rise commercial building in Shanghai. Energy Build. 39, 651–657. doi:10.1016/j.enbuild.2006.09.013
Pang, X., Wetter, M., Bhattacharya, P., and Haves, P. (2012). A framework for simulation-based real-time whole building performance assessment. Build. Environ. 54, 100–118. doi:10.1016/j.buildenv.2012.02.003
Parys, W., Saelens, D., and Hens, H. (2010). “Implementing realistic occupant behavior in building energy simulations – the effect on the results of an optimization of office buildings,” in CLIMA (Antalya).
Pegg, I. M., Cripps, A., and Kolokotroni, A. (2007). Post-Occupancy Performance of Five Low-Energy Schools in the UK. London: ASHRAE Transactions.
Piette, M. A., Nordman, B., deBuen, O., and Diamond, R. (1994). “Over the energy edge: results from a seven year new commercial buildings research and demonstration project,” in LBNL-35644 (Berkeley, CA).
Raftery, P., Keane, M., and O’Donnell, J. (2011). Calibrating whole building energy models: an evidence-based methodology. Energy Build. 43, 2356–2364. doi:10.1016/j.enbuild.2011.05.020
Raslan, R., and Davies, M. (2010). Results variability in accredited building energy performance compliance demonstration software in the UK: an inter-model comparative study. J. Build. Perform. Simul. 3, 63–85. doi:10.1080/19401490903477386
RIBA. (2013). RIBA Plan of Work 2013. Available at: http://www.ribaplanofwork.com/
Robertson, C., and Mumovic, D. (2013). “Assessing building performance: designed and actual performance in context of industry pressures,” in Sustainable Building Conference 2013 (Coventry).
Salehi, M. M., Cavka, B. T., Fedoruk, L., Frisque, A., Whitehead, D., and Bushe, W. K. (2013). “Improving the performance of a whole-building energy modeling tool by using post-occupancy measured data,” in 13th Conference of International Building Performance Simulation Association (Chambéry).
Schwartz, Y., and Raslan, R. (2013). Variations in results of building energy simulation tools, and their impact on BREEAM and LEED ratings: a case study. Energy Build. 62, 350–359. doi:10.1016/j.enbuild.2013.03.022
Sun, Y., Heo, Y., Tan, M., Xie, H., Jeff Wu, C. F., and Augenbroe, G. (2014). Uncertainty quantification of microclimate variables in building energy models. J. Build. Perform. Simul. 7, 17–32. doi:10.1080/19401493.2012.757368
Torcellini, P., Deru, M., Griffith, B., Long, N., Pless, S., Judkoff, R., et al. (2006). NREL/CP-550-36290 Lessons Learned from Field Evaluation of Six High-Performance Buildings. Technical Report. Golden: National Renewable Energy Laboratory.
Turner, C., and Frankel, M. (2008). Energy Performance of LEED for New Construction Buildings. Technical Report. Washington, DC: New Buildings Institute.
UKGBC. (2007). Carbon Reductions in New Non-Domestic Buildings. Report. London: Queen’s Printer United Kingdom Green Building Council.
Wang, L., Mathew, P., and Pang, X. (2012a). Uncertainties in energy consumption introduced by building operations and weather for a medium-size office building. Energy Build. 53, 152–158. doi:10.1016/j.enbuild.2012.06.017
Wang, S., Yan, C., and Xiao, F. (2012b). Quantitative energy performance assessment methods for existing buildings. Energy Build. 55, 873–888. doi:10.1016/j.enbuild.2012.08.037
Way, M., Bordass, B., Leaman, A., and Bunn, R. (2014). The Soft Landing Framework – for Better Briefing, Design, Handover and Building Performance In-Use. Bracknell: BSRIA/Usable Buildings Trust.
Wetter, M. (2011). A View on Future Building System Modeling and Simulation. Berkeley, CA: Lawrence Berkeley National Laboratory.
Williamson, B. (2012). The Gap Between Design and Build: Construction Compliance Towards 2020 in Scotland, Edinburgh. Edinburgh: Napier University.
ZCH. (2010). A Review of the Modelling Tools and Assumptions: Topic 4, Closing the Gap Between Designed and Built Performance. London: Zero Carbon Hub.
ZCH. (2014a). Closing the Gap Between Design and As-Built Performance – End of Term Report. London: Zero Carbon Hub.
ZCH. (2014b). Closing the Gap Between Design and As-Built Performance – Evidence Review Report. London: Zero Carbon Hub.
ZCH and NHBC Foundation. (2010). Carbon Compliance for Tomorrow’s New Homes – A Review of the Modelling Tool and Assumptions – Topic 4 – Closing the Gap Between Design and Built Performance. London: Zero Carbon Hub & NHBC Foundation.
Keywords: energy performance gap, energy use in buildings, predictions, measurements, feedback, post-occupancy evaluation
Citation: van Dronkelaar C, Dowson M, Burman E, Spataru C and Mumovic D (2016) A Review of the Energy Performance Gap and Its Underlying Causes in Non-Domestic Buildings. Front. Mech. Eng. 1:17. doi: 10.3389/fmech.2015.00017
Received: 10 November 2015; Accepted: 21 December 2015;
Published: 13 January 2016
Edited by:
Hasim Altan, The British University in Dubai, United Arab EmiratesReviewed by:
Xiufeng Pang, Lawrence Berkeley National Laboratory, USAZhenjun Ma, University of Wollongong, Australia
Andrew John Wright, De Montfort University, UK
Copyright: © 2016 van Dronkelaar, Dowson, Burman, Spataru and Mumovic. This is an open-access article distributed under the terms of the Creative Commons Attribution License (CC BY). The use, distribution or reproduction in other forums is permitted, provided the original author(s) or licensor are credited and that the original publication in this journal is cited, in accordance with accepted academic practice. No use, distribution or reproduction is permitted which does not comply with these terms.
*Correspondence: Chris van Dronkelaar, Y2hyaXN2YW5kckBnbWFpbC5jb20=