- 1Lawrence Livermore National Laboratory, Livermore, CA, United States
- 2Sandia National Laboratories, Livermore, CA, United States
- 3Department of Mechanical and Aerospace Engineering, University of California, Davis, Davis, CA, United States
We performed Computational Fluid Dynamics (CFD) simulations using a Reynolds-Averaged Navier-Stokes (RANS) turbulence model of high-pressure spray pyrolysis with a detailed chemical kinetic mechanism encompassing pyrolysis of n-dodecane and formation of polycyclic aromatic hydrocarbons. We compare the results using the detailed mechanism and those found using several different reduced chemical mechanisms to experiments carried out in an optically accessible, high-pressure, constant-volume combustion chamber. Three different soot models implemented in the CONVERGE CFD software are used: an empirical soot model, a method of moments, and a discrete sectional method. There is a large variation in the prediction of the soot between different combinations of chemical mechanisms and soot model. Furthermore, the amount of soot produced from all models is substantially less than experimental measurements. All of this indicates that there is still substantial work that needs to be done to arrive at simulations that can be relied on to accurately predict soot formation.
1 Introduction
The emissions from power generation and transportation are major contributors to climate change and the production of particulate matter has a detrimental effect on human health. While there is a strong drive to reduce the number of combustion engines in use, they will remain the principal mode of transportation and power generation for many decades (Newell et al., 2019), and understanding the processes that govern the formation of particulate matter is likely to lead to many benefits.
Soot formation is a complex phenomenon that does not readily lend itself to experimental observation due to the short time scales of intermediate species and the small size of initial soot particles and their precursors (Wang, 2011). The presence of turbulence can further complicate these processes by introducing the effects of strain rate on the soot formation process (Bisetti et al., 2012). Computational Fluid Dynamics (CFD) simulations can capture these length and time scales, while also providing a means of understanding the time-history of short lived and intermediate species (Duvvuri et al., 2019). There have been several studies through the Engine Combustion Network that have leveraged the extensive experimental database and explored the use of a number of different turbulence models (Skeen et al., 2016; Chishty et al., 2018) and soot models (Duvvuri et al., 2021; Ong et al., 2021).
However, most chemical kinetic mechanisms used in CFD codes are reduced in size to cut down on the computational cost of running the simulation and optimized to capture global metrics such as the ignition delay and the flame speed (Pang et al., 2014). Accurately capturing global metrics and the formation and destruction of the large polycyclic aromatic hydrocarbon (PAH) species that play a key role in soot formation is difficult and requires larger mechanisms which can be computationally prohibitive. Furthermore, soot model development has focused on simple fuels such as ethylene under atmospheric conditions (International Sooting Flame workshop, 2021).
One path to simplifying the problem is to study pyrolysis conditions void of oxygen where the competing effects between soot inception and oxidation processes are eliminated. There is a long history (Graham et al., 1975) of using shock tubes to better understand the kinetic processes of a range of hydrocarbon fuels under pyrolysis conditions (Frenklach et al., 1985; Frenklach et al., 1988; Colket and Seery, 1994) and more recent studies that investigate pyrolysis of n-dodecane–a common surrogate for diesel and rocket fuel–by Malewicki and Brezinsky (2013) and MacDonald et al. (2013). Jet stirred reactors at atmospheric conditions have also been used to better understand PAH formation under pyrolysis conditions with n-dodecane and validate chemical mechanisms (Herbinet, et al., 2007) along with data from other experimental setups under pyrolysis conditions in Ranzi et al. (2005).
In this work, we perform CFD simulations of n-dodecane soot formation process under engine relevant conditions using the CONVERGE commercial software (Richards et al., 2020). The simulations are setup to model the pyrolysis experiments in a constant-volume chamber of Skeen and Yasutomi (2018), which are at higher pressures than most other data in the literature. Examining the soot formation process under oxygen-starved pyrolysis conditions alleviates the difficulties associated with the competing effects between soot growth and oxidation. A short injection of a small amount of fuel into a comparably large constant-volume chamber is used in these experiments to minimize spray vaporization and mixing effects on the soot formation process. These advantages enable systematic evaluation of different sub-processes associated with modeling soot. Different industry-relevant soot models available in CONVERGE are examined. Validation against time-resolved soot measurements is also performed. A recent reaction mechanism developed by Lawrence Livermore National Laboratory (LLNL), which provides a detailed description of the formation of PAHs, is compared with various chemical mechanisms in the literature.
2 Experimental and Numerical Methods
2.1 Experiments
The simulation results target and are compared to the experimental measurements of Skeen and Yasutomi (2018). In these experiments, n-dodecane fuel sprays were injected into an optically accessible, high-pressure, constant-volume combustion chamber capable of emulating ambient conditions up to 1,800 K and 350 atm. The desired conditions are achieved by spark-igniting a premixed charge of acetylene, hydrogen, nitrogen, and oxygen. A 0.186-mm diameter single-hole research nozzle from the Spray D set of Engine Combustion Network (ECN) injectors was used to inject the fuel. The objective of the injection system and its operation was to produce small quantities of fuel injected over a relatively short period (less than 0.2 ms) to decouple injection, evaporation and mixing to soot formation, as much as possible.
Soot volume fraction measurements were performed via diffuse back-illumination extinction imaging (DBI-EI). The reader is referred to Skeen and Yasutomi (2018) for details of the experimental setup and procedures to extract soot mass. There are many sources of experimental uncertainties such as soot density, the dimensionless extinction coefficient of soot, or the injected fuel mass. The first two sources have been the subject of countless publications, including the aforementioned work (Skeen and Yasutomi, 2018) and will not be discussed here. On the other hand, the uncertainty associated with the injected fuel quantity is a crucial boundary condition to the current CFD study and is described in detail in Section 2.3.
2.2 Computational Setup
Simulations of the experimental configuration are performed using the commercial CFD code CONVERGE v3.0 (Richards et al., 2020). Turbulence is modeled using a standard k-ε Reynolds-averaged Navier–Stokes (RANS) (Launder and Sharma, 1974) model. The authors have found that this turbulence model is less sensitive to the small values of the turbulent kinetic energy used to initialize simulations of the constant volume chamber. The domain and grid are shown in Figure 1, a base grid size of 4 mm is used throughout. A conical fixed embedding region with a refining scale of 5 is applied between the injector outlet to 7 mm downstream. The same refining scale is used for Adaptive Mesh Refinement (AMR) in the regions where either the sub-grid velocity or temperature exceeds 0.1% or 2.5% of the respective variable characteristic scale in the domain. The finest resolution of the simulation is thus 62.5 µm. The peak cell count is around 5 million cells for most simulations. Unity Lewis number is assumed.
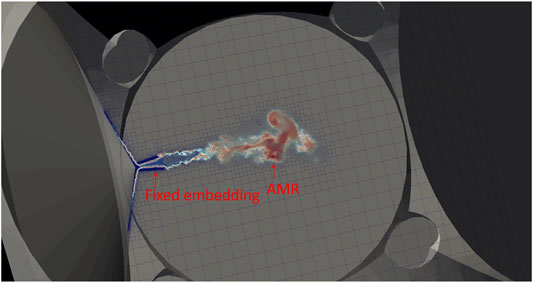
FIGURE 1. Schematic of the numerical setup used in this work. The computational domain is the Sandia Constant Volume chamber. Mesh refinement based on both fixed embedding and AMR are used to ensure a well resolved flow field.
A Lagrangian parcel method is used to simulate liquid spray with droplet breakup modelled using the Kelvin-Helmholtz (KH) and Rayleigh-Taylor (RT) models (Reitz, 1987; Senecal et al., 2007). Droplet collisions are modelled using the no time counter algorithm (Schmidt and Rutland, 2000). Droplet drag and evaporation are modeled using the Corrected Distortion framework (Nguyen et al., n.d.)1.
Combustion is modeled using a well-mixed reactor model with a multi-zone scheme to group similar computational cells together based on the temperature and mass fraction of two species. The chemistry solver is therefore called once per group rather than for each individual cell, which greatly improves computational efficiency (Babajimopoulos et al., 2005). Temperature is grouped in 5-K bins, and mass fractions in 0.001 bin-width for n-dodecane (C12H26) and acetylene (C2H2). Acetylene was chosen for its importance in the soot formation process (Frenklach and Mebel, 2020), while providing a good way to segregate cells into bins that differ in their chemical reactivity under pyrolysis conditions. The largest chemical mechanism used here consists of more than 800 species, which results in a sparse Jacobian matrix. Therefore, the preconditioned iterative SAGE kinetics solver is used (McNenly et al., 2015; Richards et al., 2020).
It should be noted that because of the injection strategy used in the experiments, only a small amount of liquid fuel is injected into a much larger constant volume chamber. Therefore, there is far less energy imparted on the flow than is the case for a long duration injection and the effects of Turbulence Chemistry Interaction (TCI) or Turbulence-Soot Interaction are expected to be minimal. The validity of the well-mixed reactor model for high-speed reacting RANS calculations has been well documented (Som et al., 2012). Given the fine resolution of 62.5 µm employed in this work, the usage of the well-mixed reactor is therefore justified. The effects of radiation are not modeled in this work, Bolla et al. (2017) and Fernandez et al. (2018) both found that including radiation had only a minor effect on the soot temperature at similar pressures and higher temperatures than in this study.
2.3 CFD Boundary Conditions
As mentioned earlier, the analysis carried out by Skeen and Yasutomi (2018) revealed uncertainties in the injected fuel mass. Because no experimental data were available to extract accurate rate of injection information under these conditions, internal flow CFD simulations were performed using findings from previous studies about the start and end of needle injection dynamics (Manin et al., 2016; Manin et al., 2020),. The internal flow simulation results provide the necessary guidance to construct different rate of injection profiles. These profiles, together with ambient density and temperature, fuel density, orifice diameter, hydraulic coefficients, and spreading angle are then used as inputs to the Musculus and Kattke jet model (Musculus and Kattke, 2009). This model has demonstrated good agreement when compared to detailed measurements combining high-sensitivity schlieren imaging and planar laser Rayleigh scattering to correlate mixing field to spray penetration rate (Pickett et al., 2011). The modeled penetration rate was then compared to the measurements, and the rate of injection iteratively adjusted until the best match was obtained. The rate of injection profile described in this section is used as boundary conditions the CFD simulations presented in this work. The injection duration was estimated to be 140 µs, with an injected mass around 0.455 mg; the rest of the relevant boundary conditions are listed in Table 1.
As noted in Table 1, the simulations focus on an ambient temperature of 1,500 K, which is slightly above the 1,450 K soot onset temperature measured in the experiments. The ambient pressure of 76 bar aims at being representative of a typical of modern diesel engine for light and medium duty applications. The gas composition for the simulations assumes complete reaction of the reactants during the pre-combustion event of the experiments, leaving no oxygen in the chamber.
2.4 Chemical Mechanisms
In this section we describe the various n-dodecane chemical kinetic mechanisms used in this study to model fuel pyrolysis. Simulations were conducted with three mechanisms from the literature, including the mechanisms of Wang et al. (2014) and Narayanaswamy et al. (2014), which are popularly used in the literature for spray simulations, and the recent LLNL mechanism of Kukkadapu et al. (2021). The mechanisms of Wang et al. and Narayanaswamy et al. are reduced mechanisms and include both low temperature oxidation and PAH chemistry. Pyrene isomers (A4) are the largest PAHs modelled in the mechanism of Wang et al., while the mechanism of Narayanaswamy et al. also describes the formation of PAHs up to cyclopenta-pyrene (A4R5). All three mechanisms were validated against shock tube data from Malewicki and Brezinsky (2013). The mechanism of Narayanaswamy et al. (2014) and Kukkadapu et al. (2021) were validated against data at both oxidizing and pyrolytic conditions, however the mechanism by Wang et al. was only validated against data under oxidizing conditions.
The recent mechanism of Kukkadapu et al. (2021) is a detailed high temperature mechanism of n-C12 and includes PAH chemistry. The original mechanism was built in a modular fashion and developed to capture the pyrolysis chemistry of C1-C12 n-alkane, iso-alkane, olefinic, alkynes, and aromatic hydrocarbons, and consists of about 1,500 species. As the objective of the present study is to study the pyrolysis of n-C12, the mechanism was manually reduced by removing the sub-modules deemed unnecessary (e.g., iso-dodecane, iso-octane, iso-nonane, trimethylbenzene etc). Furthermore, the mechanism of Kukkadapu et al. (2021) modelled formation of PAHs larger than A4R5 such as bi-naphthalene isomers, chrysene, and triphenylene. It is well known that certain soot models available in CONVERGE (such as the Gokul model) were developed assuming pyrene as the nucleating species, and the contribution to soot from PAHs larger than pyrene are not always modelled accurately, or even contribute to soot formation. To get around this problem, species lumping was implemented to modify the description of reactions leading to the formation of larger PAHs resulting in the production of A4R5/pyrene, hydrogen molecules as products. With these changes the mechanism proposed by Kukkadapu et al. was reduced to 872 species and 5,611 reactions.
To ensure that the reduced n-C12 pyrolysis mechanism captures the formation of PAHs accurately, the Kukkadapu et al. (2021) mechanism is validated against the jet stirred reactor (JSR) pyrolysis data of Herbinet et al. (2007). The performance of the Kukkadapu et al. (2021) mechanism and the two other mechanisms considered in the present study are shown in Figure 2. As seen in Figure 2, the Kukkadapu et al. (2021) mechanism satisfactorily captures the formation of aromatics containing up to 4-rings with the difference in simulated and experimental concentrations of the aromatics within a factor of two. The mechanism by Narayanaswamy et al. (2014) was found to capture the formation of benzene, phenanthrene and pyrene satisfactorily, while a difference of about a factor of five was observed in predictions of naphthalene. The mechanism of Wang et al. (2014) was found to satisfactorily capture the formation of phenanthrene and benzene, but it significantly overpredicts naphthalene and pyrene. Overall, the Wang et al. (2014) mechanism is observed to show significant differences, while Kukkadapu et al. (2021) and Narayanaswamy et al. (2014) mechanisms were found to perform satisfactorily. Note that all data is well below the soot onset temperature for n-dodecane (Skeen and Yasutomi, 2018) and thus soot formation does not impact the formation or destruction of PAH molecules. A summary of the three mechanisms and the nucleation species used for each of them in the simulations is shown in Table 2.
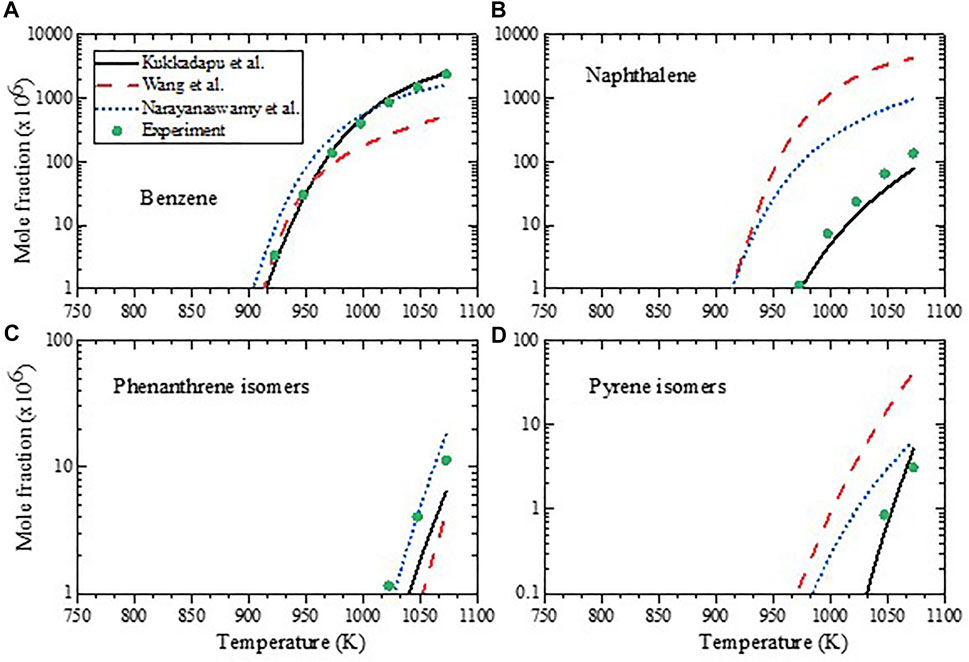
FIGURE 2. Plots showing the performance of the different mechanisms in capturing the formation of (A) benzene, (B) naphthalene, (C) phenanthrene isomers, and (D) pyrene Isomers during pyrolysis of n-dodecane in a JSR. Experimental data are from Herbinet et al. (2007).
2.5 Soot Models
Three different soot models, representative of the range of soot modeling approaches used in thermal engines, are tested in this work. The first model is a phenomenological model (called Gokul in CONVERGE) developed by Vishwanathan and Reitz (2010). This model is based on the work by Leung et al. (1991) and describes the overall soot behavior by simulating basic soot processes such as particle inception, surface growth, oxidation, and coagulation. In this model nucleation occurs through pyrene (A4), surface growth is due to acetylene, the coagulation model assumes that all particles in a cell are monodisperse and thus have the same size, and oxidation occurs through reactions with O2 and OH. This model utilizes two equations to estimate the overall soot mass and number density.
The second model, called the Particulate Mimic (PM), is a method of moments approach (Mauss, 1998). The method of moments uses equations for the moments of the particle size distribution function to model the dynamics of an ensemble of soot particles,
where
where Ni is the number density of soot particles of size class i. This formulation allows extraction of many of the major features of the particle size distribution. The zeroth moment is related to the mean number density and the first moment is related to the mean mass or mean volume of soot particles. In this work six moments were used.
The most advanced soot model implemented in CONVERGE v3.0, and used in this study, is called the Particulate Size Mimic (PSM) model. It is a discrete sectional method (Kumas and Ramkrishna, 1996; Wen et al., 2005), based on the methods of Netzell et al. (2007) and Marchal (2008). This model provides details about the soot particle distribution function not available from the other methods. For this model the particle size distribution function is discretized into bins, each bin contains particles of a similar volume. Particles appear in the first bin due to nucleation and then can move into other bins based on the solution of a transport equation. The particles can move up to another bin that contains larger volume particles due to surface growth, condensation, or coagulation. They can move down to a bin with smaller volume particles due to oxidation, or fragmentation.
The phenomenological Gokul soot model has a one-way coupling scheme with the gas phase chemistry such that PAH species and acetylene that form soot particles are not removed from the gaseous flow field. Consequently, heat and mass transfer are not strictly conserved in this model. In contrast, soot related heat and mass transfer are conserved in both the PM and PSM models. As one would expect, the computational costs of these different models scale roughly with the model’s complexity, thus the PSM model is the most computationally expensive model employed in this work. While more advanced soot models exist, the models used in this study are still relevant due to the tradeoff between computational cost and detail present in most soot models.
3 Results and Discussion
Simulations with various combinations of the chemical kinetic mechanisms and soot models discussed in the previous section were carried out in CONVERGE at the conditions listed in Table 1. Figure 3 shows the 3-D projected soot mass [kg-m] predicted using the PSM soot model at different time instances for all three chemical mechanisms. These images are obtained by integrating the raw 3-D Eulerian soot mass field in the transverse direction. They qualitatively capture the soot behavior observed from the experimental DBI-EI results (Figure 3 of Skeen and Yasutomi (2018)). The results show that the overall location of the soot field is similar amongst the different simulations, with the Wang et al. mechanism consistently predicting the highest soot mass for all time instances. All three mechanisms also present relative consistency regarding the temporal evolution of soot formation, with the first image showing soot occurring 2 ms ASOI. The soot concentration levels predicted by the Narayanaswamy et al. and Kukkadapu et al. mechanisms appear to be similar from these two-dimensional visualizations. However, summing the soot mass in the computational domain the Kukkadapu et al. mechanism predicts slightly less soot at 2 ms ASOI, but by 5 ms higher concentration pockets of soot form around 50 mm downstream and away from the jet axis leading to slightly higher overall soot mass compared to the Narayanaswamy et al. simulation results. Interestingly, and in agreement with the experiments used as the target, the formation of soot is uniform across the jet for all three mechanisms. This homogeneity in soot indicates that mixing does not play a major role under these conditions–an objective of the experiments–and support that a detailed turbulence model may not be needed to accurately capture the physics of soot formation.
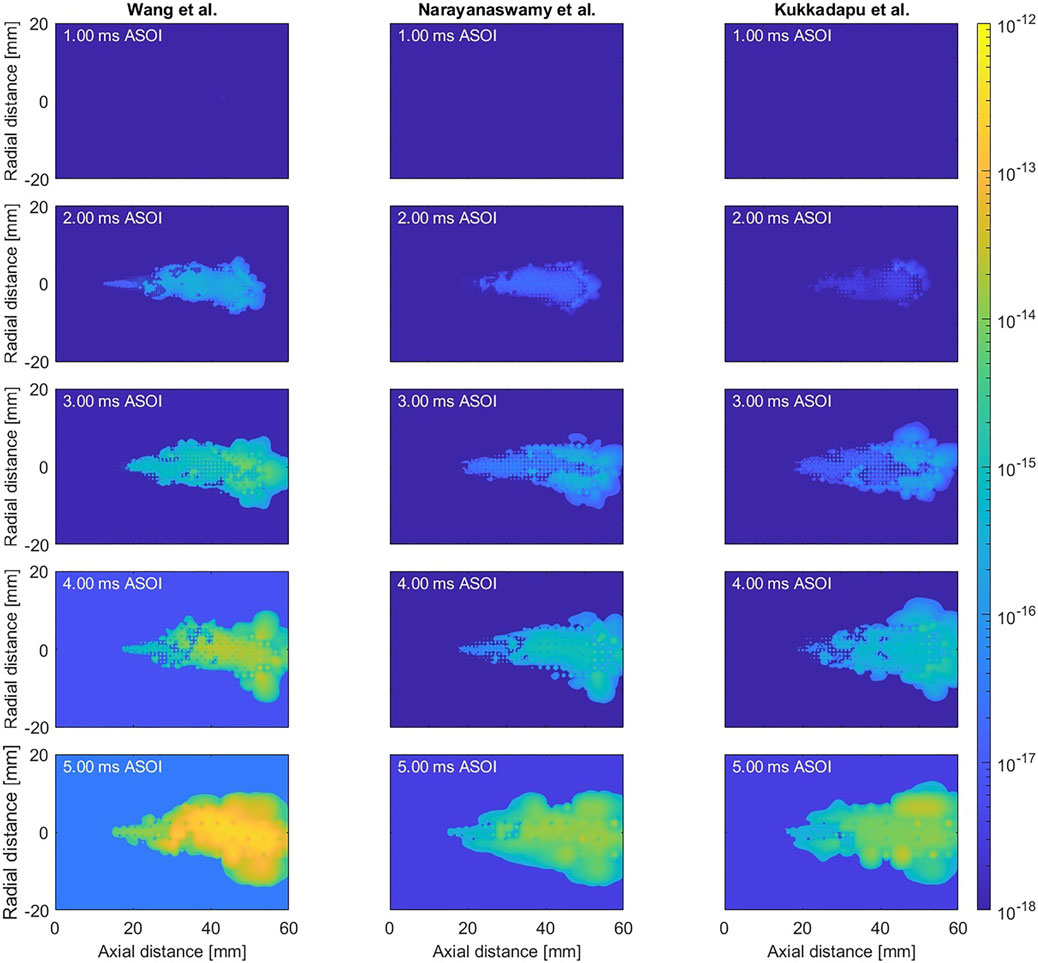
FIGURE 3. Projections of soot mass [kg-m] at select timings after the start of injection for simulations using the three mechanisms with the PSM soot model.
We will now explore the effect of the three different soot models listed in Table 3 using the Wang et al. mechanism. The overall soot mass is also compared to the experimental results of Skeen and Yasutomi (2018). All soot models use isomers of pyrene, A4 as the soot precursor. Figure 4A shows profiles of the mass of pyrene normalized by the mass of fuel injected for the three simulations. Because of the one-way coupling in the Gokul model, meaning that mass conservation of combined gas phase species and soot is not strictly enforced, the pyrene concentration continuously increases since it is not consumed. Consequently, this model predicts over two orders of magnitude more pyrene compared to the two-way coupled models. The soot mass predicted by the Gokul model grows exponentially, violating mass conservation. For simulations that include oxidation, this growth could be balanced by soot oxidation, resulting in better agreement with engine measurements despite not being physically accurate (Vishwanathan and Reitz, 2010).
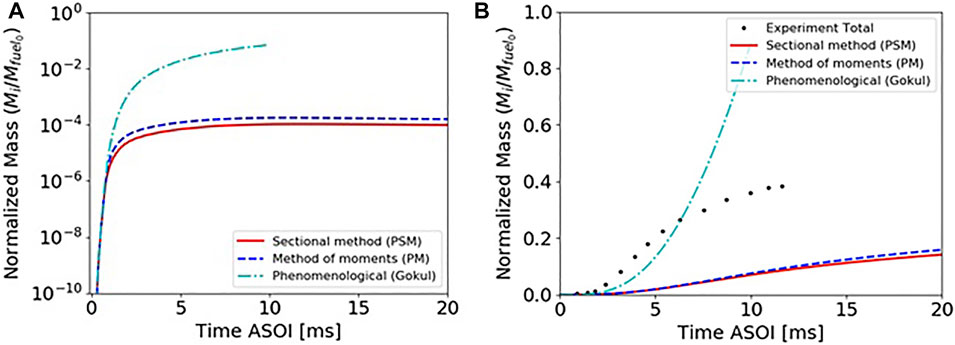
FIGURE 4. (A) Time-resolved profiles of the mass of pyrene normalized by the mass of injected fuel and (B) total soot mass profiles normalized by the injected fuel mass predicted by different soot models using the Wang et al. (2014) mechanism, and compared to the experimental data of Skeen and Yasutomi (2018).
By contrast, the PM and PSM models predict that just over 15% of the injected fuel is converted to soot, which is significantly lower than the 40% value measured in the experiments and well outside of the expected experimental uncertainty (Skeen and Yasutomi, 2018). In addition, to the lower conversion efficiency from fuel to soot, or soot yield, the simulations predict a longer soot onset time compared to the experiments. These large differences highlight the shortcomings of the current models. One contributor is that some kinetic mechanisms and soot models are tuned to capture PAH formation under only oxidizing engine conditions (Wang et al., 2014), which can negatively affect their performance under pure pyrolysis conditions. Additionally, Duvvuri et al. (2021) pointed out that kinetic mechanisms are sometimes used without a coupled soot model during validation in which they are used to reproduce the species concentrations from shock tube experiments. Because the formation and destruction of PAH species are tied to the destruction and formation of soot, failing to use a coupled soot model during validation could lead to mechanisms with reduced formation of PAH species. This effect can be seen in Figure 4 where the two simulations with coupled soot models produce far less pyrene than the simulation with the phenomenological soot model. If pyrene was measured in the experiments, the formation of pyrene might be detuned to match the pyrene formation from the experiments when a soot model without coupling is used. Another contributor for the discrepancy could be short comings in the soot models used in this study.
We compare the effect of the three different chemical mechanisms when using the PSM soot model in Figure 5, which shows the cumulative soot mass predicted by different soot sub-models. As anticipated based on Figure 3, the Wang et al. mechanism results in more soot mass compared to the other two mechanisms with substantial differences in the early stages, and still about an order of magnitude gap by 20 ms between the Wang et al. (highest) and Narayanaswamy et al. (lowest). The relative amounts of soot produced by the three mechanisms could be understood by comparing the pyrene predictions shown in Figure 2D. Specifically, the mechanism of Wang et al. was found to predict higher concentrations of pyrene, which would lead to faster inception and higher soot mass. Inception and condensation drive soot growth with the contributions from the two terms being of the same order. There is a time delay between inception and condensation since inception is the initial source of soot and condensation requires a pool of soot particles that can collide with nucleation species. The Wang et al. mechanism shows a cross-over at around 8 ms, with condensation becoming the dominating contributor, but the other two mechanisms show nearly matched contributions for these two processes by the end of the simulation time. Surface growth is the next largest term, but several orders of magnitude smaller and does not make a large contribution to the total soot mass.
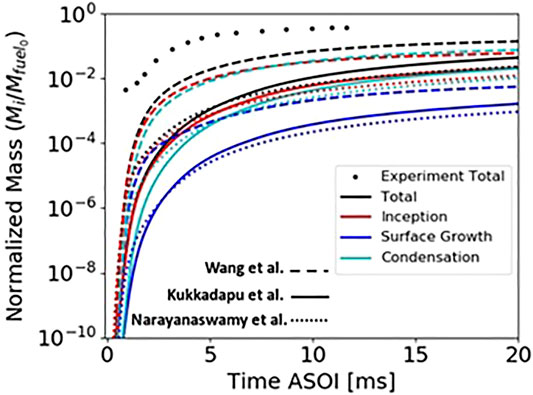
FIGURE 5. Cumulative soot mass normalized by mass of injected fuel versus time ASOI with contributions from different sub-models for the PSM soot model with the Kukkadapu et al. (2021) Narayanaswamy et al. (2014), and Wang et al. (2014) mechanisms.
Some of the differences described above are due to the variations in gas phase species predicted by the chemical mechanisms and how they interact with the soot models. To study this effect, Figure 6 shows the mass of various species normalized by the mass of injected fuel for the three different mechanisms considered in this work with the PSM soot model. Starting with the fuel, we can see that the n-dodecane fuel breaks down more quickly for the two more detailed chemical mechanisms. These mechanisms contain a larger number of pathways that more accurately capture the fuel molecular breakdown process. Note the Y-axis uses a log scale which can make small changes in the mass appear large for smaller absolute values such as the fuel as it is consumed at later times. More C2H2 is formed initially by the Wang et al. mechanism compared to the other two mechanisms, which likely spurs the greater initial increase in soot mass, as suggested by the larger contribution of surface growth especially in early stages with the Wang et al. mechanism. However, the Kukkadapu et al. mechanism predicts a higher normalized mass of C2H2 compared to the Wang et al. mechanism beyond 7 ms, leading to the soot mass increase driven by surface growth observed in Figure 5. Despite this increase, soot mass via surface growth remains higher for the Wang et al. mechanism throughout the simulated time.
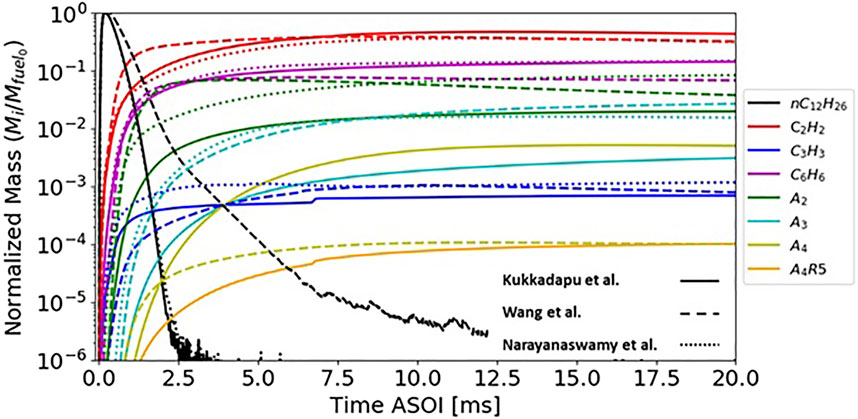
FIGURE 6. The mass for a number of species normalized by the mass of injected fuel for simulations with the Kukkadapu et al. (2021), Wang et al. (2014) and Narayanaswamy et al. (2014) mechanisms with the PSM soot model versus time ASOI.
The contribution to the total soot mass from surface growth is an order of magnitude small than that by inception and condensation indicating that smaller gas phase species such as C2H2 react primarily with other gas phase species rather than soot particles. These gas phase reactions are driven by the chemical mechanisms whereas surface growth is modeled via the Hydrogen Abstraction Acetylene Addition Ring Closure (HACARC) mechanism (Mauss, 1998) within the soot model. The HACARC mechanism captures the reaction of smaller gas phase species with solid soot particles.
Pyrene (A4) is used as a precursor for simulations using the Wang et al. and Narayanaswamy et al. mechanisms along with other precursors for the Narayanaswamy et al. mechanism (see Table 2). Pyrene mass is below the range displayed in this plot for the Narayanaswamy et al. mechanism, expectedly because of rapid conversion to soot. In contrast, there is a higher amount of pyrene predicted by the Wang et al. mechanism, remaining at a near constant level until the end of the simulations. This indicates that the formation rate of pyrene predicted by the gas phase kinetic mechanism is greater than or similar to the consumption rate within the PSM soot model throughout the simulation. The Wang et al. mechanism results in the highest mole fraction of pyrene isomers in the JSR simulations shown in Figure 2. The Kukkadapu et al. mechanism uses A4R5 as the soot precursor, so direct comparison to the four-ring aromatic species is difficult. The simulation results for this mechanism show that A4R5 forms later than pyrene, and with a lower rate of formation, which provides a sound explanation regarding the delay in soot formation for this mechanism compared to the other two, as seen in Figure 5. These differences caused by the gas phase chemistry likely explain why the simulations with the Narayanaswamy et al. mechanism predict more soot in the early stages and the Kukkadapu et al. mechanism predicts higher soot mass after about 3 ms as seen in Figures 3, 5. Furthermore, Figure 5 shows that the contribution of both inception and condensation processes when using the Kukkadapu et al. mechanism surpasses that predicted using the Narayanaswamy et al. mechanism at these later times.
Other key species related to soot formation, such as naphthalene (A2) or anthracene (A3), present substantial differences amongst the three mechanisms. This is likely the result of different pathways available in the kinetic mechanisms, with more detailed mechanisms generally leading to more reliable species concentrations as shown in Figure 2. The present results show the simpler Wang et al. mechanism producing soot mass in better agreement with the experiments compared to the other two, more detailed, kinetic mechanisms, which is in opposition to the previous statement. To better understand this contradiction, future work will investigate fundamentals of species concentration for the different mechanisms, as well as the role of PAH precursors in the soot formation process.
A comparison between the two detailed soot models studied in this work is shown in Figure 7. The cumulative soot mass predicted by different soot sub-models: inception, surface growth, fragmentation, oxidation, condensation, and coagulation are plotted for the detailed chemical mechanisms of Kukkadapu et al. (2021). While the total soot mass predicted is similar between the PSM and PM models, their respective sub-models behave differently. In both models, the inception process is a major contributor to soot mass. The PSM model predicts a higher rate of inception than the PM model by at least an order of magnitude by the end of the simulation. The PM model shows little contribution to total soot mass from the inception process after about 3 ms. This is expected due to the model’s two-way coupling implementation that leads to the depletion of the soot precursor. While inception drives soot mass increase in the early stage, condensation eventually dominates soot formation.
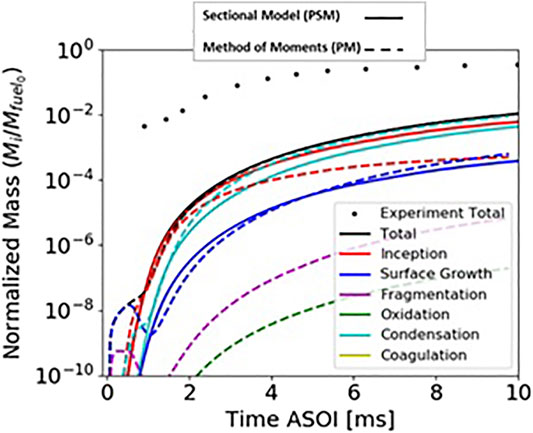
FIGURE 7. Cumulative soot mass normalized by mass of injected fuel versus time ASOI with contributions from different sub-models for the PSM and PM soot models with the detailed kinetic mechanism of (Kukkadapu et al., 2021).
Soot mass from condensation is higher for the PM model compared to the PSM model. There are also differences in the surface growth, but the condensation term is a couple orders of magnitude higher. Due to the oxygen deficient nature of the charge gas, soot oxidation is not a significant process. It should be noted, however, that there is some oxygen in the simulation domain as a result of molecular disassociation of the combustion products from the pre-combustion process used to achieve diesel like conditions in the combustion vessel (see Table 1). While the PM model shows a small amount of soot oxidation, it is still at least two orders of magnitude below the other soot related phenomena. The PM model also shows a small contribution from the fragmentation sub-model. In contrast, the contribution from both the fragmentation and oxidation sub-models in the PSM are below the range displayed in this plot. These contributions are small, and while they show that the soot models differ, they should not be used alone to draw conclusions on their capabilities or performance.
4 Conclusion
3-D CFD simulations of soot formation via fuel pyrolysis in a constant volume combustion chamber were carried out with finite rate chemistry using three detailed chemical kinetics models and three soot models available in CONVERGE. The simulation results were compared to experimental measurements to provide a frame of reference for the soot mass predicted by the different kinetic mechanisms and soot models. Chemical mechanisms containing PAH molecules are often tuned using experimental data under conditions that contain oxygen. Oxygen-deficient, pyrolysis conditions serve as a good benchmark by eliminating the complexities associated with the competing processes driving soot formation and oxidation. Additionally, mechanisms validated under sooting conditions should have a coupled soot model to ensure that they are not biased to compensate for the inability of the model to utilize gas phase species to form soot. All simulation results predict lower soot mass compared to the experiments, as well as later soot inception/formation indicating that further work is needed to improve chemical mechanisms in tandem with soot models under pyrolysis conditions. Simulations of these experiments provide a promising path to make progress towards improving both chemical mechanisms and soot models and how they are tied together. An important finding is that soot inception time depends on the species chosen as precursor(s), which link the gas phase chemical kinetics to the soot model. Choosing larger PAH molecules can lead to a delay in the prediction of soot onset compared to a smaller molecule. The simulations showed that the soot inception, condensation, and surface growth processes are highly dependent on the gas phase chemical mechanism. The formation of soot was somewhat uniform across the jet, which agrees with the experiments used as the target. This homogeneity indicates that mixing does not play a major role under these conditions, and support that a detailed turbulence model may not be needed to accurately capture the physics of soot formation.
Data Availability Statement
The raw data supporting the conclusion of this article will be made available by the authors, without undue reservation.
Author Contributions
TN, CB, and NK setup and carried out the simulations. GK reduced the detailed chemical mechanism. All authors contributed to the interpretation of the results, provided critical feedback, and helped shape the research, analysis and manuscript.
Funding
This work was conducted as part of the Partnership for Advanced Combustion Engine (PACE) project sponsored by the U.S. Department of Energy (DOE) Office of Energy Efficiency and Renewable Energy (EERE), Bioenergy Technologies and Vehicle Technologies Offices. Lawrence Livermore National Laboratory is operated by Lawrence Livermore National Security, LLC, for the U.S. Department of Energy, National Nuclear Security Administration under Contract DE-AC52-07NA27344. Sandia National Laboratories is a multi-mission laboratory managed and operated by National Technology and Engineering Solutions of Sandia, LLC., a wholly owned subsidiary of Honeywell International, Inc., for the U.S. Department of Energy’s National Nuclear Security Administration under contract DE-NA0003525.
Conflict of Interest
The authors declare that the research was conducted in the absence of any commercial or financial relationships that could be construed as a potential conflict of interest.
Publisher’s Note
All claims expressed in this article are solely those of the authors and do not necessarily represent those of their affiliated organizations, or those of the publisher, the editors and the reviewers. Any product that may be evaluated in this article, or claim that may be made by its manufacturer, is not guaranteed or endorsed by the publisher.
Acknowledgments
The authors would like to thank Convergent Sciences Inc. for licenses.
Footnotes
1It should be noted however that Panamá´s science diplomacy strategy was launched in 2018 in spite of this particular challenge.
References
Babajimopoulos, A., Assanis, D. N., Flowers, D. L., Aceves, S. M., and Hessel, R. P. (2005). A Fully Coupled Computational Fluid Dynamics and Multi-Zone Model with Detailed Chemical Kinetics for the Simulation of Premixed Charge Compression Ignition Engines. Int. J. Engine Res. 6 (5), 497–512. doi:10.1243/146808705x30503
Bisetti, F., Blanquart, G., Müeller, M. E., and Pitsch, H. (2012). On the Formation and Early Evolution of Soot in Turbulent Nonpremixed Flames. Combust. Flame 159 (1), 317–335. doi:10.1016/j.combustflame.2011.05.021
Bolla, M., Chishty, M. A., Hawkes, E. R., Chan, Q. N., and Kook, S. (2017). Influence of Turbulent Fluctuations on Radiation Heat Transfer, NO and Soot Formation under ECN Spray A Conditions. Proc. Combust. Inst. 36 (3), 3551–3558. doi:10.1016/j.proci.2016.06.173
Chishty, M. A., Bolla, M., Hawkes, E. R., Pei, Y., and Kook, S. (2018). Soot Formation Modelling for N-Dodecane Sprays Using the Transported PDF Model. Combust. Flame 192, 101–119. doi:10.1016/j.combustflame.2018.01.028
Colket, M. B., and Seery, D. J. (1994). Reaction Mechanisms for Toluene Pyrolysis. Symp. Combust. 25 (1), 883–891. doi:10.1016/s0082-0784(06)80723-x
Duvvuri, P. P., Shrivastava, R. K., and Sreedhara, S. (2019). “Numerical Modelling of Soot in Diesel Engines,” in Engine Exhaust Particulates (Singapore: Springer), 71–119. doi:10.1007/978-981-13-3299-9_5
Duvvuri, P. P., Shrivastava, R. K., and Sreedhara, S. (2021). Comparison of Soot Models for Reacting Sprays in Diesel Engine-like Conditions. Proc. Inst. Mech. Eng. D: J. Automobile Eng. doi:10.1177/09544070211015490
Fernandez, S. F., Paul, C., Sircar, A., Imren, A., Haworth, D. C., Roy, S., et al. (2018). Soot and Spectral Radiation Modeling for High-Pressure Turbulent spray Flames. Combust. Flame 190, 402–415. doi:10.1016/j.combustflame.2017.12.016
Frenklach, M., and Mebel, A. M. (2020). On the Mechanism of Soot Nucleation. Phys. Chem. Chem. Phys. 22 (9), 5314–5331. doi:10.1039/d0cp00116c
Frenklach, M., Clary, D. W., Gardiner, W. C., and Stein, S. E. (1985). Detailed Kinetic Modeling of Soot Formation in Shock-Tube Pyrolysis of Acetylene. Symp. Combust. 20 (1), 887–901. doi:10.1016/s0082-0784(85)80578-6
Frenklach, M., Clary, D. W., Gardiner, W. C., and Stein, S. E. (1988). Effect of Fuel Structure on Pathways to Soot. Symp. Combust. 21 (1), 1067–1076. doi:10.1016/s0082-0784(88)80337-0
Graham, S. C., Homer, J. B., and Rosenfeld, J. L. J. (1975). The Formation and Coagulation of Soot Aerosols Generated by the Pyrolysis of Aromatic Hydrocarbons. Proc. R. Soc. A: Math. Phys. Eng. Sci. 344 (1637), 259–285.
Herbinet, O., Marquaire, P.-M., Battin-Leclerc, F., and Fournet, R. (2007). Thermal Decomposition of N-Dodecane: Experiments and Kinetic Modeling. J. Anal. Appl. Pyrolysis 78 (2), 419–429. doi:10.1016/j.jaap.2006.10.010
International Sooting Flame workshop (2021). International Sooting Flame (ISF) Workshop. Available at: https://www.adelaide.edu.au/cet/isfworkshop/about-isf.
Kukkadapu, G., Wagnon, S. W., Pitz, W. J., and Hansen, N. (2021). Identification of the Molecular-Weight Growth Reaction Network in Counterflow Flames of the C3H4 Isomers Allene and Propyne. Proc. Combust. Inst. 38 (1), 1477–1485. doi:10.1016/j.proci.2020.07.130
Kumas, S., and Ramkrishna, D. (1996). On the Solution of Population Balance Equations by Discretization-II. A Moving Pivot Technique. Chem. Eng. Sci. 51 (8), 1333–1342.
Launder, B. E., and Sharma, B. I. (1974). Application of the Energy-Dissipation Model of Turbulence to the Calculation of Flow Near a Spinning Disc. Lett. Heat Mass Transfer 1 (2), 131–137. doi:10.1016/0094-4548(74)90150-7
Leung, K. M., Lindstedt, R. P., and Jones, W. P. (1991). A Simplified Reaction Mechanism for Soot Formation in Nonpremixed Flames. Combust. Flame 87, 289–305. doi:10.1016/0010-2180(91)90114-q
MacDonald, M. E., Ren, W., Zhu, Y., Davidson, D. F., and Hanson, R. K. (2013). Fuel and Ethylene Measurements during N-Dodecane, Methylcyclohexane, and Iso-Cetane Pyrolysis in Shock Tubes. Fuel 103 (103), 1060–1068. doi:10.1016/j.fuel.2012.09.068
Malewicki, T., and Brezinsky, K. (2013). Experimental and Modeling Study on the Pyrolysis and Oxidation of N-Decane and N-Dodecane. Proc. Combust. Inst. 34 (1), 361–368. doi:10.1016/j.proci.2012.06.156
Manin, J., Bardi, M., Pickett, L. M., and Payri, R. (2016). Boundary Condition and Fuel Composition Effects on Injection Processes of High-Pressure Sprays at the Microscopic Level. Int. J. Multiphase Flow 83, 267–278. doi:10.1016/j.ijmultiphaseflow.2015.12.001
Manin, J., Pickett, L. M., and Yasutomi, K. (2020). Stereoscopic High-Speed Microscopy to Understand Transient Internal Flow Processes in High-Pressure Nozzles. Exp. Therm. Fluid Sci. 114, 110027. doi:10.1016/j.expthermflusci.2019.110027
Marchal, C. (2008). Modélisation de la formation et de l'oxydation des suies dans un moteur automobile. Available at: https://academic.microsoft.com/paper/113538038.
Mauss, F. (1998). Entwicklung eines Kinetischen Modells der Russbildung mit Schneller. Ph.D. Thesis. Aachen: RWTH Aachen.
McNenly, M. J., Whitesides, R. A., and Flowers, D. L. (2015). Faster Solvers for Large Kinetic Mechanisms Using Adaptive Preconditioners. Proc. Combust. Inst. 35 (1), 581–587. doi:10.1016/j.proci.2014.05.113
Musculus, M. P. B., and Kattke, K. (2009). Entrainment Waves in Diesel Jets. SAE Int. J. Engines 2 (1), 1170–1193. doi:10.4271/2009-01-1355
Narayanaswamy, K., Pepiot, P., and Pitsch, H. (2014). A Chemical Mechanism for Low to High Temperature Oxidation of N-Dodecane as a Component of Transportation Fuel Surrogates. Combust. Flame 161 (4), 866–884. doi:10.1016/j.combustflame.2013.10.012
Netzell, K., Lehtiniemi, H., and Mauss, F. (20072007). Calculating the Soot Particle Size Distribution Function in Turbulent Diffusion Flames Using a Sectional Method. Proc. Combust. Inst. 3131 (1), 667667–674674. doi:10.1016/j.proci.2006.08.081
Newell, R. G., Raimi, D., and Aldana, G. (2019). Global Energy Outlook 2019: The Next Generation of Energy. Resources for the Future.
Nguyen, T. M., Dahms, R. N., Pickett, L. M., and Tagliante, F. The Corrected Distortion Model for Lagrangian Spray Simulation of Transcritical Fuel Injection. Int. J. Multiphase Flow. In Revision.
Ong, J. C., Pang, K. M., Ho, J. H., and Ng, H. K. (2021). Effects of Ambient Oxygen and Density on Primary Soot Size under Diesel-like Conditions Using a Lagrangian Soot Tracking Model. SAE Int. J. Engines 14 (2), 301–315. doi:10.4271/03-14-02-0018
Pang, K. M., Jangi, M., Bai, X. S., and Schramm, J. (2014). “Investigation of Chemical Kinetics on Soot Formation Event of N-Heptane spray Combustion,” in SAE 2014 World Congress & Exhibition, Detroit, MI, 8-10 April 2014. Available at: https://academic.microsoft.com/paper/1965490332. doi:10.4271/2014-01-1254
Pickett, L. M., Manin, J., Genzale, C. L., Siebers, D. L., Musculus, M. P. B., and Idicheria, C. A. (2011). Relationship between Diesel Fuel Spray Vapor Penetration/Dispersion and Local Fuel Mixture Fraction. SAE Int. J. Engines 4 (1), 764–799. doi:10.4271/2011-01-0686
Ranzi, E., Frassoldati, A., Granata, S., and Faravelli, T. (2005). Wide-Range Kinetic Modeling Study of the Pyrolysis, Partial Oxidation, and Combustion of Heavy N-Alkanes. Ind. Eng. Chem. Res. 44 (14), 5170–5183. doi:10.1021/ie049318g
Reitz, R. D. (1987). Modeling Atomization Processes in High-Pressure Vaporizing Sprays. Atomisation Spray Techn. 3, 309.
Richards, K. J., Senecal, P. K., and Pomraning, E. (2020). Converge (v3.0). Madison, WI: Convergent Science.
Schmidt, D. P., and Rutland, C. J. (2000). A New Droplet Collision Algorithm. J. Comput. Phys. 164 (1), 62–80. doi:10.1006/jcph.2000.6568
Senecal, P. K., Richards, K. J., Pomraning, E., Yang, T., Dai, M. Z., McDavid, R. M., et al. (2007). “A New Parallel Cut-Cell Cartesian CFD Code for Rapid Grid Generation Applied to in-Cylinder Diesel Engine Simulations,” in SAE World Congress & Exhibition, Detroit, April 16, 2007 Available at: https://academic.microsoft.com/paper/1537868910. doi:10.4271/2007-01-0159
Skeen, S. A., and Yasutomi., K. (2018). Measuring the Soot Onset Temperature in High-Pressure N-Dodecane spray Pyrolysis. Combust. Flame 188, 483–487. doi:10.1016/j.combustflame.2017.09.030
Skeen, S. A., Manin, J., Pickett, L. M., Cenker, E., Bruneaux, G., Kondo, K., et al. (2016). A Progress Review on Soot Experiments and Modeling in the Engine Combustion Network (ECN). SAE Int. J. Engines 9 (2), 883–898. doi:10.4271/2016-01-0734
Som, S., D'Errico, G., Douglas, L., and Lucchini, T. (2012). Comparison and Standardization of Numerical Approaches for the Prediction of Non-reacting and Reacting Diesel Sprays. SAE Technical Paper 2012-01-1263. doi:10.4271/2012-01-1263
Vishwanathan, G., and Reitz, R. D. (2010). Development of a Practical Soot Modeling Approach and its Application to Low-Temperature Diesel Combustion. Combust. Sci. Techn. 182 (8), 1050–1082. doi:10.1080/00102200903548124
Wang, H., Ra, Y., Jia, M., and Reitz, R. D. (2014). Development of a Reduced N-Dodecane-PAH Mechanism and its Application for N-Dodecane Soot Predictions. Fuel 136, 25–36. doi:10.1016/j.fuel.2014.07.028
Wang, H. (2011). Formation of Nascent Soot and Other Condensed-phase Materials in Flames. Proc. Combust. Inst. 33 (1), 41–67. doi:10.1016/j.proci.2010.09.009
Keywords: soot, pyrolysis, spray, CFD, chemical kinetics
Citation: Killingsworth NJ, Nguyen TM, Brown C, Kukkadapu G and Manin J (2021) Investigating the Effects of Chemical Mechanism on Soot Formation Under High-Pressure Fuel Pyrolysis. Front. Mech. Eng 7:765478. doi: 10.3389/fmech.2021.765478
Received: 27 August 2021; Accepted: 09 November 2021;
Published: 30 November 2021.
Edited by:
Mariano Sirignano, University of Naples Federico II, ItalyReviewed by:
Matteo Pelucchi, Politecnico di Milano, ItalyJean-Louis Consalvi, Aix-Marseille Université, France
Raghu Sivaramakrishnan, Argonne National Laboratory (DOE), United States
Copyright © 2021 Killingsworth, Nguyen, Brown, Kukkadapu and Manin. This is an open-access article distributed under the terms of the Creative Commons Attribution License (CC BY). The use, distribution or reproduction in other forums is permitted, provided the original author(s) and the copyright owner(s) are credited and that the original publication in this journal is cited, in accordance with accepted academic practice. No use, distribution or reproduction is permitted which does not comply with these terms.
*Correspondence: Nick J. Killingsworth, a2lsbGluZ3N3b3J0aDJAbGxubC5nb3Y=