- 1Friedrich Alexander University Erlangen-Nürnberg, Erlangen, Germany
- 2Washington University in St. Louis, St. Louis, MO, United States
- 3Graz University of Technology, Austria and Norwegian University of Science and Technology (NTNU), Trondheim, Norway
Editorial on the Research Topic
Advances in Brain Mechanics
Increasing evidence confirms that mechanics plays a critical role in brain function and dysfunction. In recent years, computational mechanics has become a powerful tool for studying and predicting the behavior of the human brain under both physiological and pathological conditions. Yet, important challenges that have hindered realistic and reliable numerical predictions remain unresolved. This Frontiers Research Topic covers recent advances, current challenges, and perspectives in brain mechanics modeling. It encompasses novel experimental and modeling approaches, including computational solid and fluid mechanics as well as data-driven modeling, which ultimately target at personalized simulations that add value to clinicians.
The mechanical response of brain tissue is highly complex: it is ultra-soft with a shear modulus on the order of 1kPa, biphasic, and heterogeneous; different brain regions show different mechanical behavior, for example in gray matter versus white matter. Therefore, the measured properties strongly depend on the length and time scale of the specific experimental setup. Common techniques include large-strain compression, tension, and shear experiments, which are typically performed ex vivo, as well as indentation measurements and magnetic resonance elastography, which can be performed in vivo. In addition to the loading and boundary conditions, assumptions made when analyzing the corresponding data can affect the reported values for properties like stiffness and viscosity. These difficulties have led to apparently contradicting experimental results in the literature. What may seem like inconsistencies at first glance, however, can often be brought together by nonlinear continuum mechanics modeling and finite element simulations, providing material models that capture the behavior of brain tissue across different time scales and loading conditions. Greiner et al. demonstrate, for example, that a poro-viscoelastic model can explain why indentation experiments suggest that white matter tissue in the human brain is stiffer than gray matter tissue, while large-strain compression experiments show the opposite trend. This study highlights the potential of mechanical modeling and simulation to standardize and help interpret experimental observations in the future.
Well-designed mechanical experiments are critical to properly calibrate models for finite element simulations of brain mechanics. So far, mechanical ex vivo testing techniques have mainly been used to identify material parameters. However, in order to achieve the goal of establishing personalized finite element models of the human brain, the in vivo characterization of human brain tissue properties becomes important. The latest developments in this direction enable the live assessment of mechanical properties using multi-modal magnetic resonance elastography (MRE), as presented in Herthum et al. While this technique is currently limited to small deformations and relatively high frequencies, which has limited its suitability for calibrating nonlinear material models at large strains, Giudice et al. are now introducing a multi-stage inverse finite element approach to calibrate heterogeneous subject-specific material parameters for injury prediction from MRE data. The parameters define the underlying nonlinear deviatoric response by minimizing the error between model-predicted brain displacements and experimental displacement data. In the future, such MRE-based, heterogeneous, subject-specific material properties can serve as a basis for improving the biofidelity of finite element brain models and enhance their potential as a tool for predicting injuries, assisting the diagnosis of diseases and further developing current treatment strategies.
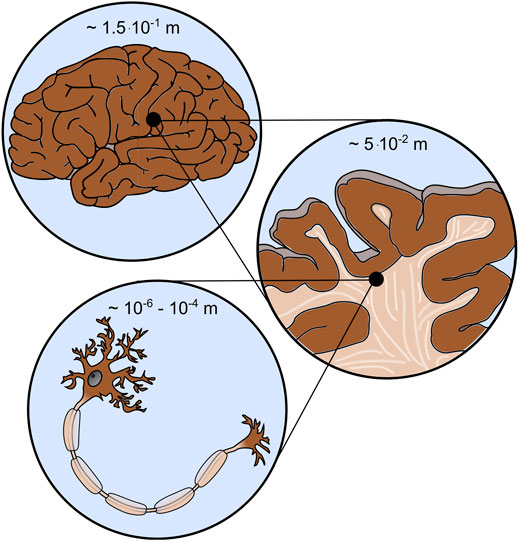
FIGURE 1. Brain mechanics involves different scales, e.g., the cell (bottom), tissue (middle), and organ (top) scale.
To provide personalized predictions, subject-specific head models are also required. Xiaogai Li presents a methodology for personalizing a baseline model through hierarchical imaging registrations with multiple features and multiple modalities, mesh morphing, and mesh grouping. The efficient generation of subject-specific finite element head models will be an important step for personalized simulations aimed at addressing clinical questions.
The complex mechanical properties of brain tissue are closely coupled to biochemical and biological processes. Therefore, multiphysics modeling has proven to be a valuable tool for predictively understanding phenomena related to development, aging and disease. Blinkouskaya et al., for example, present a model that couples tissue atrophy, the shrinking of our brain and biomarker progression in Alzheimer’s disease. Their ultimate goal is to develop a diagnostic tool able of distinguishing between healthy aging and accelerated aging typically seen in Alzheimer’s disease and related dementias. In this regard, the proposed approach based on continuum mechanics could allow earlier and more effective interventions. Another example of a coupled problem in the brain involves the smaller blood vessels, which, due to the pulsating blood flow, experience not only oscillatory forces, but also structural and morphological changes that are controlled by the surrounding brain cells. Corina S. Drapaca introduces a variable-order, fractional viscoelastic model to predict how the mechanical deformation of the cerebral artery wall is caused by pulsating blood flow and the dynamics of the neuronal nitric oxide. The model could prove to be a valuable tool to better understand the dynamic interaction between nitric oxide and brain microvessels related to neurometabolism in healthy and diseased states.
Another promising way to advance brain mechanics modeling in the future is to complement physics-based models with machine learning techniques. In Schroder et al. a machine learning layer is integrated into a mechanical damage model in order to accelerate the prediction of functional deficits after traumatic brain injury. The machine learning prediction closely matches the full simulation results and opens the door to live predictions of changes in the functional activity of the brain or to reverse engineering the mechanisms of an accident. Similarly, Menichetti et al. present an artificial neural network as a computationally efficient surrogate of a finite element porcine brain model to predict the localized brain strain and strain rate resulting from experiments with controlled cortical influence. The model is used to create tissue-level injury metrics and corresponding thresholds for cerebral contusion. Finally, in Linka et al. a “constitutive artificial neural network” is being established to predict the viscoelastic stress-strain response of brain tissue solely on the basis of microstructural data. The network then enables the modeler to assess the relevance of certain features such as cellular and extracellular tissue components for the macroscopic large-strain mechanical response and thus gain new insights into the microstructure-mechanics relationships in the brain. These studies highlight how the combination of physics-based modeling and machine learning techniques can advance the field of brain mechanics.
In general, the integration of both mechanical data and modeling, as well as imaging or medical data, will be key to bringing computational brain mechanics models closer to their use in clinical practice. Tan et al. present, for example, a methodology to combine computational simulations with clinical data for the interpretation of blunt traumatic brain injury. This study demonstrates how the integration of techniques from computational biomechanics and medical data assessed in the clinic (e.g., magnetic resonance imaging) can help predict injuries, support early medical diagnosis, or evaluate the effectiveness of personal protective equipment. This approach can lead to improved bicycle helmet designs that are effective in preventing traumatic brain injury (TBI), as discussed in Abderezaei et al.
By gathering these recent advances in the rapidly evolving field of brain mechanics, we aim to foster further synergies between various experimental and modeling approaches that will help overcome remaining challenges and frontiers. Together, these advances are moving the field towards its goal of generating high-fidelity models that can provide novel insights into brain injuries and disease and ultimately improve strategies for their diagnosis and treatment.
Author Contributions
SB wrote the original draft, PB and GH carefully revised the article. All authors approved the final version.
Conflict of Interest
The authors declare that the research was conducted in the absence of any commercial or financial relationships that could be construed as a potential conflict of interest.
Publisher’s Note
All claims expressed in this article are solely those of the authors and do not necessarily represent those of their affiliated organizations, or those of the publisher, the editors and the reviewers. Any product that may be evaluated in this article, or claim that may be made by its manufacturer, is not guaranteed or endorsed by the publisher.
Keywords: Brain mechanics, data-driven modeling, finite element method, nonlinear continuum mechanics, personalized simulations, traumatic brain injury, multiphysics modeling
Citation: Budday S, Bayly PV and Holzapfel GA (2021) Editorial: Advances in Brain Mechanics. Front. Mech. Eng 7:803151. doi: 10.3389/fmech.2021.803151
Received: 27 October 2021; Accepted: 22 November 2021;
Published: 17 December 2021.
Edited and Reviewed by:
Juergen Brugger, Swiss Federal Institute of Technology Lausanne, SwitzerlandCopyright © 2021 Budday, Bayly and Holzapfel. This is an open-access article distributed under the terms of the Creative Commons Attribution License (CC BY). The use, distribution or reproduction in other forums is permitted, provided the original author(s) and the copyright owner(s) are credited and that the original publication in this journal is cited, in accordance with accepted academic practice. No use, distribution or reproduction is permitted which does not comply with these terms.
*Correspondence: Silvia Budday, c2lsdmlhLmJ1ZGRheUBmYXUuZGU=