Effect of flow rate and CNM concentration in nanofluid on the performance of convective heat transfer coefficient
- 1Mechanical Engineering Department, Universitas Muhammadiyah Surakarta, Surakarta, Indonesia
- 2School of Applied Sciences and Mathematics, Universiti Teknologi Brunei, Gadong, Brunei Darussalam
The aim of this study is to investigate the thermal performance of water-based Carbon Nano Material (CNM) nanofluids, particularly in the convective heat transfer coefficient (h) parameter, and to analyze the effects of nanoparticle concentration and flow rate on an experimental heat transfer system. To achieve this, the researchers prepared the nanofluid through a 2-step method. In the first step, we mixed the nanoparticles with the base fluid using a magnetic stirrer to ensure homogenization, with the CNM-Water ratio set at 30, 75, 120, and 150 ppm. In the second step, we characterized the prepared samples, determining their size, composition, and particle morphology through PSA and SEM-EDX examination, as well as measuring their density (ρ) and specific heat (Cp). The experiments were carried out using a flow rate simulation test rig with a data acquisition system, at different flow rates of 0.2, 0.6, 1, and 1.4 L/min. Temperature (T) was measured at the inlet, outlet, and heater to determine the convective heat transfer coefficient value. The study successfully investigated the direct impact of CNM concentration and flow rate, with the results showing a consistent and expected value of the coefficient compared to previous studies. The highest coefficient value was observed at 150 ppm CNM-Water ratio and a flow rate of 1.4 L/min, with a value of 1825.37 W/(m2.K).
1 Introduction
High-performance cooling is a very vital requirement in various industries, cooling is needed to maintain the performance and reliability expected from a product such as computers, high-power electronic equipment, and vehicle engines. Conventional techniques commonly used for the optimization of heat transfer systems include increasing the surface area of the device and adjusting the fluid flow velocity. The main limitation in the previous cooling technology was related to the low thermal conductivity of the base fluid used, so that new technologies such as conventional solid-liquid suspension were developed by adding millimeter or micrometer-sized solid particles to the base fluid and applications such as micro-solids channeling. The early generation technology is fraught with a range of issues such as rapid agglomeration rates, wear and tear on cooling installations due to friction, blockages in micro-channels, and limitations in the maximum performance of micro-channels. In addition, the conventional liquid suspension method that involves adding a large number of particles (>10% of the total volume) is considered inefficient, as it results in a decrease in pressure and requires greater pumping power. These challenges have necessitated the exploration of alternative approaches to cooling technology that are more efficient and effective. With the technological trend of device/product miniaturization, the complexity of cooling problems will also increase in various industries. Therefore, nanofluid applications are being developed to find solutions to problems in the heat transfer field. Nanofluid is a two-phase liquid and solid mixture that is engineered by dispersing nano-sized particles in a base fluid. The main dimensions of the particles used are less than 100 nm which can be in the form of nanoparticles, nanotubes, nanofibers, nanowires, or nano rods. It is known that several types of particles that have been studied in the literature include, non-metallic particles (SiO2 (Logesh et al., 2019), SiC (Huminic et al., 2017), TiO2 (Murshed et al., 2005), Al2O3 (Babu et al., 2020), ZnO (Kole and Dey, 2012), CuO (Said et al., 2019), Fe3O4 (Zhu et al., 2006) and AIN (Wozniak et al., 2013)) and metallic particles (Cu (Eastman et al., 2001), Ag (Patel et al., 2003) and Au (Chen et al., 2017)). While the basic fluid types commonly applied to cooling systems include oil, water, organic glycol liquid, refrigerant, polymer solution, biofluid, and mineral oil.
The focus of this research is to investigate the thermal performance of Carbon Nano Material (CNM) nanofluids, which has not been extensively explored in previous studies. The research novelty lies in the use of experimental methods to examine the effect of flow rate and CNM concentration on the base fluid, water. By conducting experiments to evaluate these parameters, the study aims to provide insights into the potential of CNM nanofluids in enhancing the heat transfer efficiency in various applications. CNM is a nanostructured material with good mechanical, thermal, and electrical properties, which can consist of single-walled or multi-walled nanotubes. CNM has unique properties that are considered suitable for optimization or improvement of device performance which include having the behavior of semiconductors or metals, being stronger than steel but lighter than aluminum, being able to conduct heat more efficiently, and having high stability with excellent electrical and unique optical properties.
Abed et al. (Abed et al., 2020) conducted a study on the heat transfer behavior of nanofluid superposed porous-nanofluid layer in a circular cylinder tube, where a significant increase in convective heat transfer was observed, depending on the flow conditions and CNT concentration. In another study, Nazari et al. (Nazari et al., 2014) aimed to investigate CPU cooling using alumina and CNT nanofluids, and compare the results with the cooling performance of pure base fluids, namely, water and ethylene glycol. Their findings showed an optimal heat increase of approximately 13% for a nanofluid CNT with a volume fraction of 0.25% at a flow rate of 21 mL/s. These studies demonstrate the potential of nanofluids in enhancing heat transfer in cooling systems and provide valuable insights for future research in this field.
Several studies related to CNTs have been conducted including by Kumaresan, V et al. (Kumaresan et al., 2013) who investigated the heat transfer characteristics of CNT-based nanofluids by varying the length of the tubular for cooling or heating applications. Nanofluid was prepared by dispersing Carbon Nanotube multi-wall (MWCNT) in a mixture of water-ethylene glycol with a volume ratio of 70:30, resulting in an increase in the Prandtl number compared to the base fluid of 115.8% at 0°C and 180.2% at 40°C with a volume of 0.45% MWCNT. Then (Van Trinh et al., 2018) conducted research on ethylene glycol (E.G.,)-based nanofluids made from hybrid Gr-CNT obtained by combining CNT-OH and Gr-COOH solutions using the ultrasonication method without surfactants. Nanofluid containing a volume of 0.07% Gr-CNT showed an increase in thermal conductivity of 18% and 50% at 30°C and 50°C, respectively. Dalkılıç, Ahmet Selim et al. (Dalkılıç et al., 2018) are also known conducted an experiment to optimize the thermal conductivity of CNT-SiO2 based on distilled water from previous research. The experimental results showed that all samples with a volume fraction of 1% or higher experienced an average increase of 20.13%. The maximum increase in thermal conductivity of 26.19% was found in the volume fraction of 0.8 CNT: 0.2 SiO2. Habeeb et al. (Habeeb et al., 2019) optimized the finned-tube with twisted tape by applying a Al2O3 - water nanofluid with CFD modeling of laminar flow and heat transfer, resulting in an increase in the nusselt number of 18% at Re = 2290 compared to distilled water without nanoparticles and has a further increase when using a wire coil with no significant pressure drop. Duan, (Chougule et al., 2016), analyzed the effect of deionized water-based CNT-Al2O3 nanofluids in a thermoelectric cooling system. This study concludes that nanofluids can significantly improve cooling performance in natural circulation mode. Under the same working conditions, the CNT nanofluid has the largest heat flux density, which is 55% higher than the base fluid and has the ability to withstand freezing so that it can work more effectively.
Muruganandam, (Duan et al., 2017), conducted an experimental investigation of the two-step nanofluid method at volume concentrations of 0.1, 0.3, 0.5% with the addition of sodium dodecyl butane sulfonate (SDBS) as a stabilizer. 0.3% nanofluid shows the best stability, has better mechanical efficiency and fuel consumption than water as coolant. Masoud Hosseini, (Muruganandam and Mukesh Kumar, 2020), conducted a simulation-based analysis on a heat exchanger. In one study, the total heat transfer coefficient was found to increase by 6% at a CNT volume fraction of 0.278% and a mass flow rate of 45 kg/s. This result highlights the potential of nanofluids to enhance heat transfer in cooling systems. In another experiment, (Masoud Hosseini et al., 2016), investigated the thermal conductivity of three types of CNT nanofluid concentrations, ranging from 0.05% to 0.48% by volume, at temperatures of 10°C–60°C. These findings offer valuable insights into the relationship between CNT concentration and thermal conductivity, which is an important factor to consider when designing and optimizing cooling systems. The results show that the thermal conductivity capacity of nano CNT fluids is higher than that of base fluids and is highly dependent on aspect ratio, concentration, and temperature. (Xing et al., 2016). investigated the heat transfer coefficient and pressure drop characteristics in CNT-water-based nanofluids in a double pipe heat exchanger. The results show that the addition of CNT can increase the thermal conductivity up to 56% at a volume fraction of 0.3% and has a higher convective heat transfer coefficient than water as the base fluid. Babu, K (Sarafraz et al., 2016) studied the effect of concentration and agitation of Carbon Nanotubes on the rate of heat transfer during the cooling process. The results showed that the heat flux increased with increasing CNT concentration up to 0.5% then started to decrease with further increase in concentration.
Aghabozorg, (Babu and Prasanna Kumar, 2011), in their research investigated the optimization of Fe2O3 - CNT magnetic nanoparticles in horizontal shell and tube heat exchangers under laminar, transient, and turbulent flow with different temperature variations. From the observations, it is known that the Fe2O3 - CNT nanofluid hybrid shows a higher heat transfer coefficient than distilled water as the base fluid with the highest increase of 37.50% at a volume concentration of 0.2%. (Aghabozorg et al., 2016). investigated the effect of surface modifications (surfactants, acids, bases, amides, sulfates) on multi-walled Carbon Nanotubes regarding the stability of CNT dispersion in aqueous media and thermal conductivity. Treatment with Sodium Dodecyl Sulfate (SDS) showed an increase in conductivity, with the highest value of 0.765 W/mK and an increase of 24.9% compared to the base fluid. Kumaresan, V (Ghozatloo et al., 2014) conducted a study with the aim of measuring and analyzing the thermophysical properties of CNT-based water and ethylene glycol alloy experimentally at various temperatures. The maximum increase in thermal conductivity occurs at a temperature of 40°C with a volume fraction of 0.45%, which is 19.75%. (Kumaresan and Velraj, 2012). studied the thermal conductivity and viscosity of water-based multi-wall CNTs as base fluids on various geometric characteristics with a volume fraction of 0.5%. The experimental results show that the thermophysical properties of nanofluids are related to geometric characteristics. In his research, the maximum increase that can be achieved is 36% (Salawu et al., 2023) in their study, SWCNT-Ag and MWCNT-MoS4 based nanofluids were prepared in engine oil. The results show that the increase in Prandtl number, as well as the inclusion of the viscoelastic term and elastic deformation, result in decreased heat distribution for both SWCNT-Ag and MWCNT-MoS4 hybrid nanofluids. In order to understand the behavior of nanofluids, (Kiran Kumar et al., 2023), conducted an investigation into their movement, analyzing factors such as velocity, temperature and concentration. The findings indicated that elevated temperatures and concentrations yield slower velocities, while greater velocities are attained with higher radiative heat flux or nanoparticle volume fraction.
Based on the above literature descriptions, this study aims to investigate the effect of the heat transfer performance of CNM-Water nanofluids on a cooling simulation system by adding the volume of the CNM fraction and regulating the flow rate of nanofluids and then comparing it with the base fluid. The nanofluid used in this research shows potential for application as a cooling liquid in lithium-ion batteries.
2 Methods
This research uses literature study and experiment method. The nanoparticles prepared are Carbon Nano Material (CNM) produced by the Vendor. The characteristics to be studied in nanofluids are particle size, morphology, and chemical composition, and property measurements including density and specific heat, then temperature data collection at the inlet, outlet, and heating elements to determine the value of the heat transfer coefficient. The CNM-water nanofluid will be applied to a flow rate simulation tool with variations in CNM concentration and a certain flow rate with the aim of testing the effectiveness of the nanofluid used as a working fluid and compared with water.
2.1 Sample characterization
Particle size analysis (PSA) was carried out using a Photon Correlation Spectroscopy then the morphology and surface topology were examined with a Scanning Electron Microscopy (SEM) instrument by scanning a focused electron beam on the surface and chemical composition was examined by Energy Dispersion X-Ray (EDX) examination.
Identification of nanoparticle size was carried out using the Photon Correlation Spectroscopy instrument. Measurements took place at a temperature condition of 25.1°C, refractive index 1.3328, viscosity 0.8858 cP, and scattering intensity 11,001 cps in a water solvent. It is known from the measurement results as can be observed in Figure 1 that the size of the nanoparticles used in this study has an average size of 74.7 nm with a polydispersity index of 0.323.
In SEM analysis, the electron beam is scanned in a rectangular pattern (raster scan). In this process, the position of the beam is combined with the detection signal to produce a high-resolution image. During this process, the sample is observed at a magnification of up to 50 nm to determine the shape of the particles. EDX analysis was carried out in the range of 0–20 keV, the results revealed the presence of carbon (C) elements and it was known that there were impurities including aluminum oxide (Al2O3), Calcium Oxide (CaO), and Zinc Oxide (ZnO). The percentage by weight of Carbon calculated from the EDX analysis was 96.61% by weight (0.277 keV). SEM-EDX analysis confirmed that the sample prepared was CNM with a purity level >90% as can be seen in Table 1.
2.2 Sample property measurement
Measurement of water quality that will be used as the base fluid in this study using a TDS meter, the TDS value is limited to no more than 50 ppm. The density value of the base fluid was measured using a pycnometer or a specific gravity bottle volume of 25 mL. To measure the density of a sample, a pycnometer was used. The weight of the pycnometer and cover was measured before inserting the sample. The sample was inserted until the capillary tube was filled, and the weight was measured again. At least three measurements were taken to ensure accuracy before calculating the density of the sample.Figure 2, Figure 3.
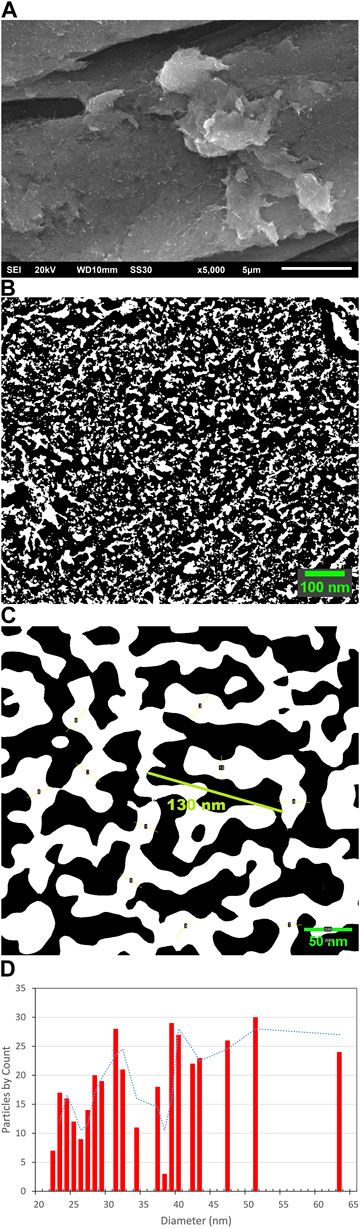
FIGURE 2. SEM Image CNM, (A)-(B) Surface morphology of CNM on a scale of 5 m and 100 nm, (C) Dimensions of length and size of CNM nanoparticles on a scale of 50 nm, (D) Histogram of diameter distribution of CNM nanoparticles.
In this study, the CNM density value obtained theoretically through literature study, it is known that in graphite, the graphene layers are connected by brittle van der Waals (vdW) bonds. vdW bonds exist in various molecules and nanostructures (D'Amico and Sharapov, 2004), these bonds are generally longer than covalent bonds, vdW bonds arise from attractive and repulsive forces while the radius vdW is half of the bond length (Holwill, 2019). In the Carbon study, the value of the radius vdW that has been reported is around 0.17 nm (Pauling, 1931). The unit cell of graphite has a lattice constant, a = 0.246 nm, c = 0.671 nm and the distance between planes in graphite is c/2 = 0.335 nm, which is the bond length vdW. Half of this value is the radius vdW, R vdW = 0.168 nm. Then through equations 7, equations 8, and equations 9 mathematically obtained the value of CNM density is 2.28 g/cm3. The measurement of the specific heat of the basic fluid in this study uses an aluminum calorimeter as shown in Figure 3.15 with a minimum of 3 data collection times. The data will be used is the mass of a substance (empty mass of calorimeter, the sample mass of cold, heat the sample mass), temperature (the temperature of the cold early, early summer temperature, and the temperature of the mixture) and Cp Calorimeter which the value of the amount already known. Calculation of specific heat is based on the black principle where Q received is proportional to Q released. In this study, the specific heat value of CNM was obtained through data from previous research conducted by, which was 0.73 J/gr°C (Alvarez, 2013). The measurement results can be observed in Tables 2, Table 3.
2.3 Nanofluid preparation
A two-step method was employed to produce the nanofluid. In the first stage, a water-based fluid and CNT dispersion were prepared with concentrations of 30, 75, 120, and 150 ppm, which were determined based on previous studies. For the second stage, a magnetic stirrer was used to mix the nanofluid for approximately 15 min to ensure homogeneity, as illustrated in Figure 4. The deposition rate of the sample was then observed, and precipitation was detected when the liquid boundary line began to form. Table 4.
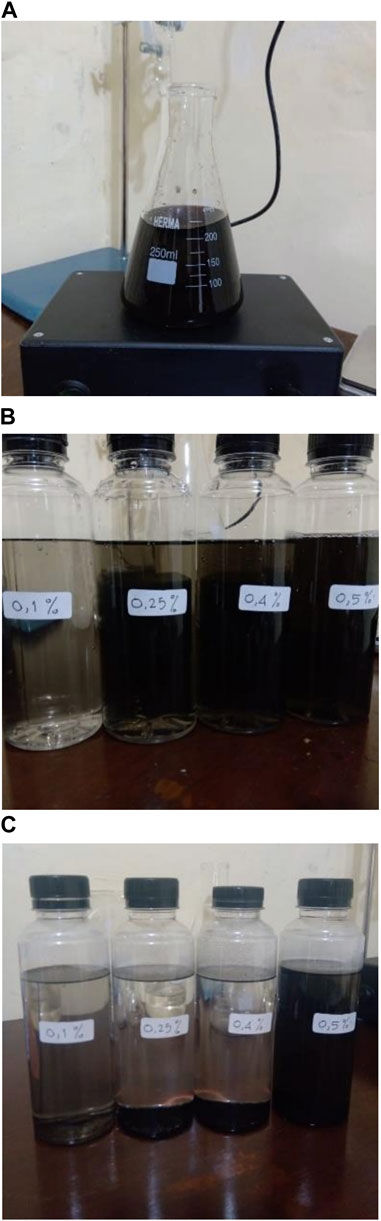
FIGURE 4. Preparation of nanofluid, (A) Magnetic stirrer, (B) Nanofluid CNM-Water 1 day old, (C) 30 days old.
2.4 Experimental process
The research investigates the performance of nanofluids using a specially designed test device that simulates a cooling system. The device, shown in Figure 5, comprises a closed-loop flow with a circulation pump, flowmeter, water block, air cooler, thermoelectric cooler, vibration bath, and four temperature sensors. Two ds18b20 sensors are located at the water block inlets, one ds18b20 sensor is placed in the microchannel heat sink area to measure the fluid temperature, and a max-6675 sensor is used to measure the heating element’s wall temperature. The heating element is made of an aluminum heater plate that measures 21 × 36 × 5 mm and operates at a constant power of 30 W. To ensure efficient heat transfer, the heater plate is coated with thermal paste and affixed to the water block wall.
The experimental setup involves circulating 200 mL of fluid through the system using a DC pump with a maximum capacity of 4 L/min. The fluid flows from the vibration bath to the water block to absorb heat, then passes through the air cooler and TEC cooler for cooling. Multiple sensors are integrated with an Arduino-based data acquisition system, programmed using node-red, a flow-based programming tool. The system can be monitored in real-time via the node-red dashboard. To vary the flow rate, the pump motor’s rotation is adjusted using a variable speed drive with four flow rate variations (0.2, 0.6, 1, and 1.4 L/min). After ensuring steady-state conditions for 600 s, sensor data is collected for each flow rate and concentration volume, and the readings are saved automatically in a CSV file.
3 Data processing
Equation 1 is used to determine the nano volume concentration of the fluid.
Where θ are the volume fractions of the particles, mn and ml are the masses of the nanoparticles and the base fluid, respectively, while ρn dan ρl are the densities of the nanoparticles and the base fluid, respectively.
The heat transfer coefficient from the working fluid to the heat sink or water block is one of the important parameters in the investigation of the performance of nanofluids, it can be determined using a theoretical approach through Equation (2) (Pradhan et al., 2009) based on Newton’s law:
Where q is the heat transfer rate, h is the convective heat transfer coefficient, A is the area and ΔTm is the average temperature difference between the wall and the fluid in direct contact with the wall.
In this study, the temperature of the wall and heating element is assumed to be the same and applied using thermal paste, so that Twall = Theater. While ΔTm can be known through Equation (3) (Pradhan et al., 2009).
Where Tin and Tout are the temperatures of the fluid at the inlet and outlet of the water block, while Theater is the temperature of the heating element.
The temperature of the nanofluid will increase when it passes through the water block, so the heat absorbed by the nanofluid can be expressed in Equation (4) (Pradhan et al., 2009).
Where Q is the flow rate, while ρnf and Cnf are the density and specific heat of the nanofluid, each of which values are obtained through sample testing with a pycnometer and calorimeter. It can be expressed through Eq. 5, Eq. 6 (Rafati et al., 2012).
CNM density in this study is defined as the total mass of carbon atoms in a closed volume. To calculate the hexagon area (A) and surface density (Sd) expressed in Eq. 7 and Eq. 8:
It is known that in each hexagon there are 2 full carbon atoms. The CNM density can be expressed through Equation (8):
Where h is the distance between layers known to 0.335 nm (Zhao et al., 2019), Mw is the mass of a carbon atom and NA is Avogadro Number. b is the CC bond length which is equivalent to 0.142 nm (RozpŁoch et al., 2007).
Through Eq. 2, Eq. 3, the convective heat transfer coefficient can be expressed in Equation (9) (Pradhan et al., 2009).
The convective heat transfer coefficient is known to be a function of the Reynolds Number, Prandtl Number, and Nusselt Number, the correlation is shown through Equations 10–13:
Where, Q is the flow rate, Dh is the hydraulic diameter, v is the kinematic viscosity, A is the cross-sectional area, is the dynamic viscosity, Cp is the specific heat and k is the thermal conductivity.
4 Uncertainty analysis
The lack of precise knowledge about the true value of the measurand is reflected in the uncertainty associated with the measurement result. Even after correcting for known systematic effects, the measurement result remains an estimation of the true value of the measurand due to uncertainties arising from random effects and imperfect correction for systematic effects. To assess the uncertainty in the experimental data, a type A evaluation method was employed, which involves statistical analysis of a series of observations (JCGM, 2008:100, 2008; Willink, 2022).
The quantity Y, which represents the measurand, is not directly measured but is instead calculated based on N other quantities (X1, X2, ., XN) using a functional relationship denoted as f.
Equation 14 can be used to estimate the value of the measurand Y, indicated by the symbol y, by providing input estimations for the values of the N quantities X1, X2,., XN. As a result, Eq. 15 provides the output estimate y, which is the outcome of the measurement. The estimate y may be obtained from Equation 16.
In other words, y is considered to be the arithmetic mean or average of n independent determinations of Yk, each of which was based on a full set of observed values for the N input quantities Xi collected at the same time and had the same level of uncertainty. Instead of y = f (
The estimated standard deviation associated with each input estimate xi, known as standard uncertainty and represented by u(xi), is used to calculate the estimated standard deviation associated with the output estimate or measurement result y, known as the combined standard uncertainty and denoted by uc(y).
The arithmetic mean or average
Equation 19 provides the experimental variance of the observations, which approximates the variance σ2 of the probability distribution of q. Due to random fluctuations in the influence quantities, each observation qk has a different value. The variability of the observed values qk, or more precisely, their dispersion around their mean
Equation 20 provides the most accurate estimate of σ2(
As a result, the standard uncertainty u(xi) of an estimate of an input quantity Xi derived from n independent repeated observations Xi,k is u(xi) = s(
5 Result and discussion
This study experimentally investigates the impact of varying concentrations of CNM nanoparticles in water-based fluids and flow rate on thermal performance. The convective heat transfer coefficient is the key parameter used to assess the thermal performance, and its value is determined using experimental and mathematical data (Patel et al., 2003; Nazari et al., 2014). Figure 6 depicts the convective heat transfer coefficient of water-based CNM nanofluids at different mass-volume ratios and flow rates. The experimental findings indicate that the convective heat transfer coefficient increases considerably with an increase in the mass-volume ratio and flow rate. For instance, at a flow rate of 0.2 L/min, the addition of 30 ppm CNM nanofluid led to a 41.6% increase in the convective heat transfer coefficient, while the addition of 75 ppm, 120 ppm, and 150 ppm increased the coefficient by 44.8%, 15.1%, and 10.4%, respectively. Notably, the water-based fluid demonstrated better performance than the CNM fluid with a ratio of 30 ppm at a flow rate of 0.2 L/min and 0.6 L/min, while a higher flow rate of 1.4 L/min increased the heat absorption rate of the working fluid and improved the heat transfer process. Another researchers were given the same results (Kumaresan et al., 2013; Van Trinh et al., 2018).
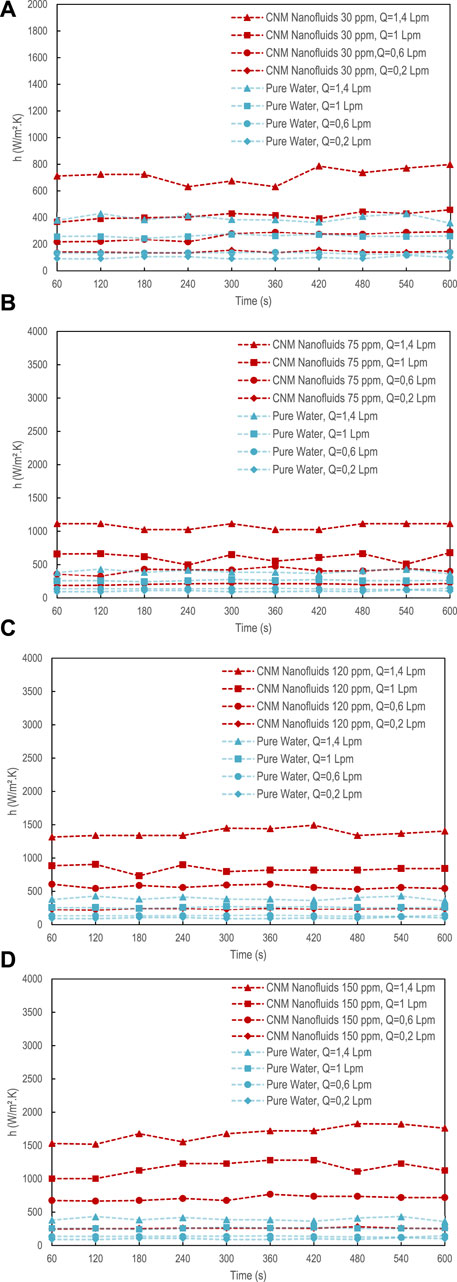
FIGURE 6. Comparison of convective heat transfer coefficient values for base fluids and CNM Nanofluids at the ratio: (A) 30 ppm, (B) 75 ppm, (C) 120 ppm, (D) 150 ppm.
Figure 6B shows the increase in heat transfer at a flow rate of 0.6 L/min. The CNM fluid with a concentration of 30 ppm showed a 95.6% increase in comparison to the base fluid. With the addition of a mass-volume ratio of 75 ppm, the increase was 57.1%. For the concentration range of 75–120 ppm and 120–150 ppm, the increase was 39.7% and 24.4%, respectively. It can be observed that at a flow rate of 1.4 L/min, the pure water base fluid has a higher thermal performance compared to CNM fluid with a concentration of 75 ppm at a flow rate of 0.2 L/min. The increase in thermal performance can be further observed in Figure 4.6.c. For a flow rate of 1 L/min, there is an increase in the convective heat transfer coefficient of 59.4% at a ratio of 30 ppm compared to pure water as a working fluid. The ratio range of 30–75 ppm of CNM fluid increased by 47.1%. The addition ranges of 75–120 ppm and 120–150 ppm increased by 37% and 38.9%, respectively. According to Figure 6C, the base water was delivered at a concentration higher than 120 ppm CNM nanofluid at flow rates of 1.4 and 0.2 L/min. The thermal performance of the base fluid was increased by 82.5% with the addition of a mass-volume ratio of 30 ppm at a flow rate of 1.4 L/min, as shown in Figure 6D. Furthermore, an addition of 30–75 ppm increased the thermal performance by 50%. The increase in thermal performance can be observed with the addition of 75–120 ppm and 120–150 ppm, which resulted in a 28.1% and 21.5% improvement, respectively. However, at flow rates of 0.4 and 0.2 L/min, the thermal performance of the 150 ppm CNM nanofluid was lower than that of pure water. The thermal performance was enhanced by the inclusion of 30, 75, 120, and 150 ppm of CNM, with values of 72%, 74.9%, 84.3%, and 93.2%, respectively, observed within the flow rate range of 1–1.4 L/min. These findings indicate that changes in flow rate can significantly impact the thermal performance of the system.
Previous studies have examined the mechanism for increasing the thermal performance of nanofluids, particularly in regard to the intrinsic thermal performance of nanoparticles, their size and shape, their dispersion, and Brownian motion. The most important and primary factor is the high thermal performance of the nanoparticles themselves, with C, Al2O3, CaO, and ZnO known to have high thermal performance, and with a synergistic effect among these nanoparticles producing nanofluids with higher thermal performance (Dresselhaus et al., 1995). More specifically, this increase in thermal performance can be attributed to several factors. One is the excitation of Low-Frequency Phonon (LFP) induced by Carbon Nano Material, which redistributes LFP to the carbon atom interface, activating LFP resonance with other nanoparticles, such as Al2O3, CaO, and ZnO, resulting in more heat transfer upon contact (Ma et al., 2020). Another factor is related to mass concentration, where as the distance between particles decreases, more particles come into contact with each other, increasing the frequency of lattice vibrations, and thus thermal performance (Qiu et al., 2020). A third factor is the effect of particle size; according to Brown’s theory, smaller particles in a fluid move faster, leading to higher energy transfer within the fluid (Manasrah et al., 2016; Qiu et al., 2019). As the flow rate increases, the Brownian motion of the nanoparticles becomes more active, resulting in a significant increase in the convective heat transfer coefficient. The flow rate is related to the Reynolds number, and according to Equation (11), the microchannel area exhibits characteristics of turbulent flow, with intense mixing and disorderly vectors, leading to increased friction between the dispersed C, Al2O3, CaO, and ZnO particles in the water-based fluid and the microchannel walls. The higher the particle concentration and flow rate, the greater the frictions, resulting in an increase in the heat transfer coefficient. The result was confirmed by (She and Fan, 2018).
Figure 7 illustrates the changes in the heat transfer coefficient resulting from the addition of mass-volume ratios of 30, 75, 120, and 150 ppm to the water-based fluid. Adding CNM mass-volume ratios is known to enhance the convective heat transfer coefficient parameters, as expected and confirmed by the experimental results of several previous researchers.
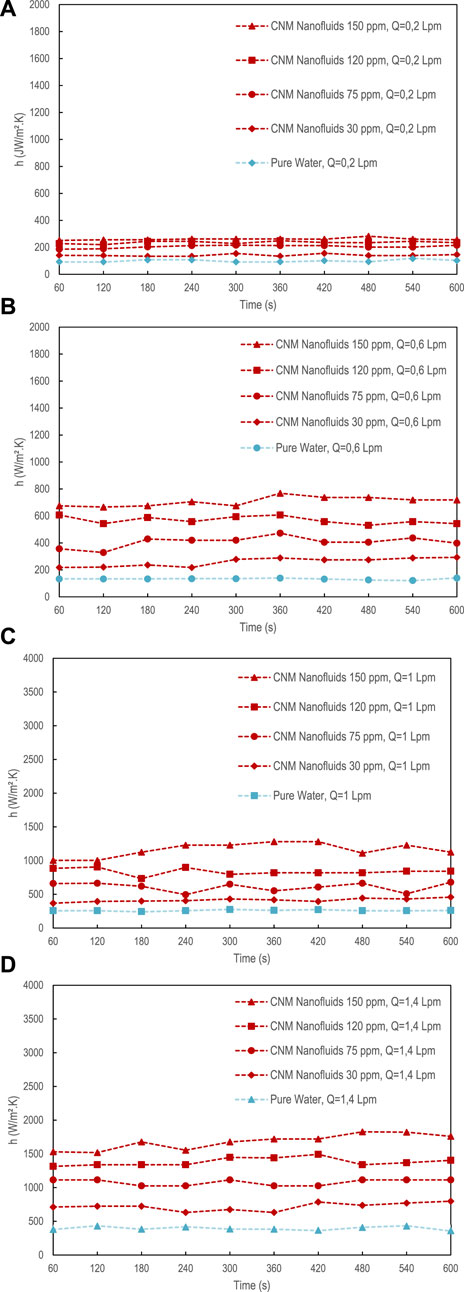
FIGURE 7. Function of mass-volume ratio of CNM to convective heat transfer coefficient at flow rates: (A) 0.2 L/min, (B) 0.6 L/min, (C) 1 L/min, (D) 1, 4 L/min.
The flow rates of basic fluid and nanofluid in this study were determined respectively 0.2, 0.6, 1, and 1.4 L/min. The experiment was carried out at room temperature 26°C. It can be observed at a flow rate of 0.2 L/min, it is known that the average value of the heat transfer coefficient for pure water is 100.02 W/(m2.K). and increased with the addition of the CNM mass-volume ratio of 30, 75, 120, and 150 ppm respectively as shown in Figure 7A. The highest coefficient value was obtained for nanofluids with a CNM ratio of 150 ppm of 281.72 W/(m2.K). Figure 7B shows that an increase in the coefficient of heat transfer occurs as the flow rate increases, with the maximum value observed at 0.6 L/min. This increase is related to the presence of Brownian motion resulting from the small size of the nanoparticles, which accelerates heat transfer through intermolecular motion. The highest coefficient value occurs at the 150 ppm CNM ratio, with an average of 766.82 W/(m2.K).
Figures 7B,C show a tendency for the performance trend of the CNM nanofluid with a 75 ppm ratio to decrease at flow rates of 0.6 L/min and 1 L/min, although the decrease is insignificant. This tendency may be related to the instability of the suspension of nanoparticles in the base fluid, with the possibility of agglomeration along the tube path and in the microchannel area. A similar observation was made for the CNM ratio of 120 ppm at a flow rate of 1 L/min. This observation is consistent with the data on nanofluid samples, where separation occurs or a boundary line appears at the CNM ratio of 75 and 120 ppm after 30 days of sample age. The highest heat transfer coefficient value at a flow rate of 1 L/min occurs at a 150 ppm CNM ratio, with a value of 1280.26 W/(m2.K). At a flow rate of 1.4 L/min, Figure 7D shows a significant increase in the coefficient value for each addition of the CNM mass-volume ratio. The highest value is obtained with the addition of the 150 ppm CNM ratio, which is 1825.37 W/(m2.K).
It is known from the results of this study that the heat transfer rate increases with the addition of the mass of nanoparticles and the flow rate compared to the water-based fluid. The amount of heat that can be transferred per unit time is also greater, and this has an impact on decreasing the temperature on the heating element side, as observed in Figure 8. It can be said that the addition of CNM mass to the base fluid and adjusting the flow rate can improve the heat transfer performance.
Based on the study, the most optimal thermal performance was achieved using a 150 ppm CNM fluid at a flow rate of 1.4 L/min, which resulted in a convective heat transfer coefficient 1825.37 W/(m2.K) higher than water at the same flow rate of 426.75 W/(m2.K).
6 Conclusion
The experimental results of this study on CNM-Water nanofluids in a cooling system, prepared using a one-step magnetic stirrer method, revealed a significant increase in convective heat transfer coefficient compared to water, particularly at a mass-volume ratio of 150 ppm and a flow rate of 1.4 L/min, with a heat transfer coefficient value of 1825.37 W/(m2.K). Nano fluids containing more than 120 ppm CNM have a higher thermal coefficient compared to pure water. Although cost and stability are important factors, the findings suggest that CNM nanofluids have great potential for use in heat-generating industrial or electrical systems, and further research is needed to assess their long-term stability in heat transfer systems. Additionally, plausible mechanisms, such as the impact of shear-induced on thermal conductivity and the reduction of the thermal boundary layer thickness, cannot fully account for the considerable heat transfer enhancement observed in this work.
Data availability statement
The original contributions presented in the study are included in the article/supplementary material, further inquiries can be directed to the corresponding author.
Author contributions
AA: Data curation, Writing—Original draft preparation. DR: Supervision. Mas: Conceptualization, Methodology. AH: Visualization, Investigation. JS: Writing—Reviewing and Editing. MI: Nanofluid design. All authors contributed to the article and approved the submitted version.
Funding
The research was funded by the Innovation and Research Centre of Universitas Muhammadiyah Surakarta under the contract number 145.5/A.3-III/LRI/VII/2022.
Acknowledgments
The writers wish to express their gratitude to the Universitas Muhammadiyah Surakarta for providing financial assistance for the research project through the Innovative Research program. The project was assigned contract number 145.5/A.3-III/LRI/VII/2022. Additionally, they would like to thank the Mechanical Engineering Department and Material Laboratory at Universitas Muhammadiyah Surakarta for their contributions to the project. We would like to express our gratitude to the organization for their generous support.
Conflict of interest
The authors declare that the research was conducted in the absence of any commercial or financial relationships that could be construed as a potential conflict of interest.
Publisher’s note
All claims expressed in this article are solely those of the authors and do not necessarily represent those of their affiliated organizations, or those of the publisher, the editors and the reviewers. Any product that may be evaluated in this article, or claim that may be made by its manufacturer, is not guaranteed or endorsed by the publisher.
References
Abed, I. M., Abdulkadhim, A., Hamzah, R. A., Hamzah, H. K., and Ali, F. H. (2020). Natural convection heat transfer for adiabatic circular cylinder inside trapezoidal enclosure filled with nanofluid superposed porous-nanofluid layer. FME Trans. 48 (1), 82–89. doi:10.5937/fmet2001082M
Aghabozorg, M. H., Rashidi, A., and Mohammadi, S. (2016). Experimental investigation of heat transfer enhancement of Fe2O3-CNT/water magnetic nanofluids under laminar, transient, and turbulent flow inside a horizontal shell and tube heat exchanger. Exp. Therm. Fluid Sci. 72, 182–189. doi:10.1016/j.expthermflusci.2015.11.011
Alvarez, S. (2013). A cartography of the van der Waals territories. Dalton Trans. 42 (24), 8617–8636. doi:10.1039/c3dt50599e
Babu, E. R., Reddy, N. C., Reddappa, H. N., Shivananda Murthy, K. V., Gnanendra Reddy, G. V., and Koppad, P. G. (2020). Influence of Al2O3 nanoparticles on thermal performance of closed loop pulsating heat pipe. FME Trans. 48 (1), 143–148. doi:10.5937/fmet2001143B
Babu, K., and Prasanna Kumar, T. S. (2011). Effect of CNT concentration and agitation on surface heat flux during quenching in CNT nanofluids. Int. J. Heat Mass Transf. 54 (1–3), 106–117. doi:10.1016/j.ijheatmasstransfer.2010.10.003
Chen, M., He, Y., Huang, J., and Zhu, J. (2017). Investigation into Au nanofluids for solar photothermal conversion. Int. J. Heat Mass Transf. 108, 1894–1900. doi:10.1016/j.ijheatmasstransfer.2017.01.005
Chougule, S. S., Nirgude, V. V., Gharge, P. D., Mayank, M., and Sahu, S. K. (2016). Heat transfer enhancements of low volume concentration CNT/water nanofluid and wire coil inserts in a circular tube. Energy Procedia 90 (2015), 552–558. doi:10.1016/j.egypro.2016.11.223
D'Amico, I., and Sharapov, S. G. (2004). Universal scaling of Coulomb drag in graphene layers. Retrieved from: http://arxiv.org/abs/cond-mat/0410769.
Dalkılıç, A. S., Yalçın, G., Küçükyıldırım, B. O., ztuna, S., Akdoğan Eker, A., Jumpholkul, C., et al. (2018). Experimental study on the thermal conductivity of water-based CNT-SiO2 hybrid nanofluids. Int. Commun. Heat Mass Transf. 99, 18–25. doi:10.1016/j.icheatmasstransfer.2018.10.002
Dresselhaus, M. S., Dresselhaus, G., and Saito, R. (1995). Physics of carbon nanotubes. Carbon 33 (7), 883–891. doi:10.1016/0008-6223(95)00017-8
Duan, L., Han, J., Cao, L., and Huo, C. (2017). Experimental study on heat transfer characteristics of CNTs/Al2O3 nanofluids in personal cooling system based on thermoelectric refrigeration. Procedia Eng. 205, 588–595. doi:10.1016/j.proeng.2017.10.421
Eastman, J. A., Choi, S. U. S., Li, S., Yu, W., and Thompson, L. J. (2001). Anomalously increased effective thermal conductivities of ethylene glycol-based nanofluids containing copper nanoparticles. Appl. Phys. Lett. 78 (6), 718–720. doi:10.1063/1.1341218
Ghozatloo, A., Rashidi, A. M., and Shariaty-Niasar, M. (2014). Effects of surface modification on the dispersion and thermal conductivity of CNT/water nanofluids. Int. Commun. Heat Mass Transf. 54, 1–7. doi:10.1016/j.icheatmasstransfer.2014.02.013
Habeeb, L. J., Saleh, F. A., and Maajel, B. M. (2019). CFD modeling of laminar flow and heat transfer utilizing Al2O3/water nanofluid in a finned-tube with twisted tape. FME Trans. 47 (1), 89–100. doi:10.5937/fmet1901089H
Holwill, M. (2019). van der Waals heterostructures. Nature 499, 19–31. doi:10.1007/978-3-030-18529-9_3
Huminic, G., Huminic, A., Fleaca, C., Dumitrache, F., and Morjan, I. (2017). Thermo-physical properties of water based SiC nanofluids for heat transfer applications. Int. Commun. Heat Mass Transf. 84, 94–101. doi:10.1016/j.icheatmasstransfer.2017.04.006
JCGM 2008:100 (2008). Evaluation of measurement data — guide to the expression of uncertainty in measurement. Geneva: Int. Organ. Stand. Available: http://www.bipm.org/en/publications/guides/gum.html.
Kiran Kumar, T., Srinivasa Rao, P., and Shamshuddin, M. D. (2023). Effect of thermal radiation on nonDarcy hydromagnetic convective heat and mass transfer flow of a water–SWCNT’s and MWCNT’s nanofluids in a cylindrical annulus with thermo-diffusion and chemical reaction. Int. J. Mod. Phys. B 0 (0), 2450011. doi:10.1142/S0217979224500115
Kole, M., and Dey, T. K. (2012). Thermophysical and pool boiling characteristics of ZnO-ethylene glycol nanofluids. Int. J. Therm. Sci. 62, 61–70. doi:10.1016/j.ijthermalsci.2012.02.002
Kumaresan, V., Mohaideen Abdul Khader, S., Karthikeyan, S., and Velraj, R. (2013). Convective heat transfer characteristics of CNT nanofluids in a tubular heat exchanger of various lengths for energy efficient cooling/heating system. Int. J. Heat Mass Transf. 60 (1), 413–421. doi:10.1016/j.ijheatmasstransfer.2013.01.021
Kumaresan, V., and Velraj, R. (2012). Experimental investigation of the thermo-physical properties of water-ethylene glycol mixture based CNT nanofluids. Thermochim. Acta 545, 180–186. doi:10.1016/j.tca.2012.07.017
Logesh, K., Ramesh, V., Kumar, T. P. B., Kumar, V. P., and Akash, B. (2019). Preparation and property studies of SiO2/H2O nanofluid. Mater. Today Proc. 18, 4816–4820. doi:10.1016/j.matpr.2019.07.470
Ma, M., Zhai, Y., Yao, P., Li, Y., and Wang, H. (2020). Synergistic mechanism of thermal conductivity enhancement and economic analysis of hybrid nanofluids. Powder Technol. 373, 702–715. doi:10.1016/j.powtec.2020.07.020
Manasrah, A. D., Al-Mubaiyedh, U. A., Laui, T., Ben-Mansour, R., Al-Marri, M. J., Almanassra, I. W., et al. (2016). Heat transfer enhancement of nanofluids using iron nanoparticles decorated carbon nanotubes. Appl. Therm. Eng. 107, 1008–1018. doi:10.1016/j.applthermaleng.2016.07.026
Masoud Hosseini, S., Vafajoo, L., and Salman, B. H. (2016). Performance of CNT-water nanofluid as coolant fluid in shell and tube intercooler of a LPG absorber tower. Int. J. Heat Mass Transf. 102, 45–53. doi:10.1016/j.ijheatmasstransfer.2016.05.071
Murshed, S. M. S., Leong, K. C., and Yang, C. (2005). Enhanced thermal conductivity of TiO2- Water based nanofluids. Int. J. Therm. Sci. 44 (4), 367–373. doi:10.1016/j.ijthermalsci.2004.12.005
Muruganandam, M., and Mukesh Kumar, P. C. (2020). Experimental analysis on internal combustion engine using MWCNT/water nanofluid as a coolant. Mater. Today Proc. 21, 248–252. doi:10.1016/j.matpr.2019.05.411
Nazari, M., Karami, M., and Ashouri, M. (2014). Comparing the thermal performance of water, Ethylene Glycol, Alumina and CNT nanofluids in CPU cooling: Experimental study. Exp. Therm. Fluid Sci. 57, 371–377. doi:10.1016/j.expthermflusci.2014.06.003
Patel, H. E., Das, S. K., Sundararajan, T., Sreekumaran Nair, A., George, B., and Pradeep, T. (2003). Thermal conductivities of naked and monolayer protected metal nanoparticle based nanofluids: Manifestation of anomalous enhancement and chemical effects. Appl. Phys. Lett. 83 (14), 2931–2933. doi:10.1063/1.1602578
Pauling, L. (1931). The nature of the chemical bond. Application of results obtained from the quantum mechanics and from a theory of paramagnetic susceptibility to the structure of molecules. J. Am. Chem. Soc. 53 (4), 1367–1400. doi:10.1021/ja01355a027
Pradhan, N. R., Duan, H., Liang, J., and Iannacchione, G. S. (2009). The specific heat and effective thermal conductivity of composites containing single-wall and multi-wall carbon nanotubes. Nanotechnology 20 (24), 245705. doi:10.1088/0957-4484/20/24/245705
Qiu, L., Zhu, N., Feng, Y., Michaelides, E. E., yła, G., Jing, D., et al. (2020). A review of recent advances in thermophysical properties at the nanoscale: From solid state to colloids. Phys. Rep. 843, 1–81. doi:10.1016/j.physrep.2019.12.001
Qiu, L., Zou, H., Wang, X., Feng, Y., Zhang, X., Zhao, J., et al. (2019). Enhancing the interfacial interaction of carbon nanotubes fibers by Au nanoparticles with improved performance of the electrical and thermal conductivity. Carbon 141, 497–505. doi:10.1016/j.carbon.2018.09.073
Rafati, M., Hamidi, A. A., and Shariati Niaser, M. (2012). Application of nanofluids in computer cooling systems (heat transfer performance of nanofluids). Appl. Therm. Eng. 45-46, 9–14. doi:10.1016/j.applthermaleng.2012.03.028
RozpŁoch, F., Patyk, J., and Stankowski, J. (2007). Graphenes bonding forces in graphite. Acta Phys. Pol. A 112 (3), 557–562. doi:10.12693/aphyspola.112.557
Said, Z., Rahman, S. M. A., El Haj Assad, M., and Alami, A. H. (2019). Heat transfer enhancement and life cycle analysis of a Shell-and-Tube Heat Exchanger using stable CuO/water nanofluid. Sustain. Energy Technol. Assessments 31, 306–317. doi:10.1016/j.seta.2018.12.020
Salawu, S. O., Obalalu, A. M., Fatunmbi, E. O., and Shamshuddin, M. D. (2023). Elastic deformation of thermal radiative and convective hybrid SWCNT-Ag and MWCNT-MoS4 magneto-nanofluids flow in a cylinder. Results Mater 17, 100380. doi:10.1016/j.rinma.2023.100380
Sarafraz, M. M., Hormozi, F., and Nikkhah, V. (2016). Thermal performance of a counter-current double pipe heat exchanger working with COOH-CNT/water nanofluids. Exp. Therm. Fluid Sci. 78, 41–49. doi:10.1016/j.expthermflusci.2016.05.014
She, L., and Fan, G. (2018). Numerical simulation of flow and heat transfer characteristics of CuO-water nanofluids in a flat tube. Front. Energy Res. 6, 1–8. doi:10.3389/fenrg.2018.00057
Van Trinh, P., Anh, N. N., Hong, N. T., Hong, P. N., Minh, P. N., and Thang, B. H. (2018). Experimental study on the thermal conductivity of ethylene glycol-based nanofluid containing Gr-CNT hybrid material. J. Mol. Liq. 269, 344–353. doi:10.1016/j.molliq.2018.08.071
Willink, R. (2022). On revision of the guide to the expression of uncertainty in measurement: Proofs of fundamental errors in bayesian approaches. Meas. Sensors 24, 100416. doi:10.1016/j.measen.2022.100416
Wozniak, M., Danelska, A., Kata, D., and Szafran, M. (2013). New anhydrous aluminum nitride dispersions as potential heat-transferring media. Powder Technol. 235, 717–722. doi:10.1016/j.powtec.2012.11.023
Xing, M., Yu, J., and Wang, R. (2016). Experimental investigation and modelling on the thermal conductivity of CNTs based nanofluids. Int. J. Therm. Sci. 104, 404–411. doi:10.1016/j.ijthermalsci.2016.01.024
Zhao, N., Guo, L., Qi, C., Chen, T., and Cui, X. (2019). Experimental study on thermo-hydraulic performance of nanofluids in CPU heat sink with rectangular grooves and cylindrical bugles based on exergy efficiency. Energy Convers. Manag. 181, 235–246. doi:10.1016/j.enconman.2018.11.076
Zhu, H., Zhang, C., Liu, S., Tang, Y., and Yin, Y. (2006). Effects of nanoparticle clustering and alignment on thermal conductivities of Fe3O4aqueous nanofluids. Appl. Phys. Lett. 89 (2), 023123–023127. doi:10.1063/1.2221905
Nomenclature
Keywords: thermal performance, water-based, carbon nano material (CNM), nanofluids, heat transfer coefficient, nanoparticle concentration
Citation: Anggono AD, Riswanto D, Ismoen M, Masyrukan , Hariyanto A and Sedyono J (2023) Effect of flow rate and CNM concentration in nanofluid on the performance of convective heat transfer coefficient. Front. Mech. Eng 9:1174185. doi: 10.3389/fmech.2023.1174185
Received: 26 February 2023; Accepted: 16 June 2023;
Published: 03 July 2023.
Edited by:
Andrey Mityakov, University of Turku, FinlandReviewed by:
MD. Shamshuddin, SR University, IndiaTitan C. Paul, University of South Carolina Aiken, United States
Copyright © 2023 Anggono, Riswanto, Ismoen, Masyrukan, Hariyanto and Sedyono. This is an open-access article distributed under the terms of the Creative Commons Attribution License (CC BY). The use, distribution or reproduction in other forums is permitted, provided the original author(s) and the copyright owner(s) are credited and that the original publication in this journal is cited, in accordance with accepted academic practice. No use, distribution or reproduction is permitted which does not comply with these terms.
*Correspondence: Agus Dwi Anggono, ada126@ums.ac.id