- 1Cooperative Oxford Laboratory, National Ocean Service/National Centers for Coastal Ocean Science, National Oceanic and Atmospheric Administration (NOAA), Oxford, MD, United States
- 2Horn Point Laboratory, University of Maryland Center for Environmental Science, Cambridge, MD, United States
- 3College of Earth, Ocean, and Atmospheric Sciences, Oregon State University, Corvallis, OR, United States
Routine monitoring of shellfish growing waters for bacteria indicative of human sewage pollution reveals little about the bacterial communities that co-occur with these indicators. This study investigated the bacterial community, potential pathogens, and fecal indicator bacteria in 40 water samples from a shellfish growing area in the Chesapeake Bay, USA. Bacterial community composition was quantified with deep sequencing of 16S rRNA gene amplicons, and absolute gene abundances were estimated with an internal standard (Thermus thermophilus genomes). Fecal coliforms were quantified by culture, and Vibrio vulnificus and V. parahaemolyticus with quantitative PCR. Fecal coliforms and V. vulnificus were detected in most samples, and a diverse assemblage of potential human pathogens were detected in all samples. These taxa followed two general patterns of abundance. Fecal coliforms and 16S rRNA genes for Enterobacteriaceae, Aeromonas, Arcobacter, Staphylococcus, and Bacteroides increased in abundance after a 1.3-inch rain event in May, and, for some taxa, after smaller rain events later in the season, suggesting that these are allochthonous organisms washed in from land. Clostridiaceae and Mycobacterium 16S rRNA gene abundances increased with day of the year and were not positively related to rainfall, suggesting that these are autochthonous organisms. Other groups followed both patterns, such as Legionella. Fecal coliform abundance did not correlate with most other taxa, but were extremely high following the large rainstorm in May when they co-occurred with a broad range of potential pathogen groups. V. vulnificus were absent during the large rainstorm, and did not correlate with 16S rRNA abundances of Vibrio spp. or most other taxa. These results highlight the complex nature of bacterial communities and the limited utility of using specific bacterial groups as indicators of pathogen presence.
Introduction
Monitoring for indicator microorganisms, such as fecal coliforms, Escherichia coli, and Enterococcus spp., at shellfish beds and swimming beaches has been conducted since the early 1900's to assess the likelihood of encountering human pathogens from fecal pollution (EPA, 1986; Ashbolt et al., 2001). However, the presence and abundance of these indicator bacteria are not always correlated with the presence of human pathogens (Noble and Fuhrman, 2001) and their strength in risk assessments depends on cell abundance (EPA, 1986; FDA, 2013) and the types of pathogens present (Wade et al., 2003). More reliable indicators of fecal pollution and human pathogens are being sought and several other bacterial groups have been proposed, including several members of the order Bacteriodales (Bernhard and Field, 2000; Walters et al., 2007) and the family Lachnospiraceae (Newton et al., 2011).
Several studies have explored the co-occurrence of pathogens and indicator bacteria in bivalves or their surrounding waters. For example, Walters et al. (2007) found that Salmonella, Campylobacter, and E. coli O157:H7 did not commonly co-occur in water samples, suggesting different sources or life-histories for these pathogens. While Hood et al. (1983) found that high levels of fecal coliforms and E. coli were about equally predictive of the presence of the specific pathogen group Salmonella in oyster tissue, a single indicator for the diverse types of pathogens found at shellfish beds or swimming beaches may not be feasible (Wade et al., 2003).
In the Chesapeake Bay, monitoring for indicator bacteria over natural shellfish beds occurs year-round. While the harvest of oysters from these natural beds is currently prohibited from late spring to early fall, harvest from aquaculture is permissible year-round, with additional post-harvest handling requirements during summer months (MDDNR, 2015). In Maryland, fecal indicators in waters over natural shellfish beds are monitored with measurements of fecal coliform densities twice a month. Local health agencies conduct similar routine monitoring during the summer in recreational areas of the Chesapeake Bay used for boating and swimming.
Despite these monitoring efforts, little is known about the bacterial communities that co-occur with fecal indicator bacteria. Bacterial communities in natural waters tend to be very diverse (Kan et al., 2006) and have complex and redundant functional groups (Comte and del Giorgio, 2010). Until recently, studies of bacterial communities in shellfish growing areas have relied on culture-based methods that exclude a large number of bacteria and require focused analyses of particular bacterial groups. A study in the Santa Anna River, California using 16S rRNA gene amplicon pyrosequencing detected higher percentages of genera that contain human pathogens in areas of urban runoff and agriculture (Ibekwe et al., 2013). Likewise, a recent study of bacterial community variability at a shellfish growing area in Spain, using denaturing gradient gel electrophoresis (DGGE), noted seasonal shifts in the bacterial community and the presence of genera containing human pathogens (Pereira et al., 2015). However, this study included samples from only six time points between February and December. The occurrence and residence time of bacterioplankton in rivers and estuaries can change over time periods of hours and days (Heidelberg et al., 2002; Crump et al., 2004) and the survival and abundance of human pathogens in natural waters may relate to temporal changes in water temperatures and salinities (Rhodes and Kator, 1988; Jacobs et al., 2014). Several more recent studies have used next-generation sequencing to investigate the presence of human pathogens in drinking water and to compare the occurrence of these pathogens to indicator bacteria (Inkinen et al., 2016; Shrestha et al., 2017). Therefore, additional studies using next-generation sequencing and focused on more highly resolved temporal changes in estuarine bacterial communities and potential pathogenic members at shellfish growing areas, would help inform human health risks relative to traditional indicators and potentially improve decisions about restricting shellfish harvest.
The presence of human pathogens that are not of fecal origin represent a significant concern in many estuarine areas. Pathogenic groups of Vibrio bacteria, such as V. vulnificus and V. parahaemolyticus, for example, are naturally-occurring and cause numerous cases of gastroenteritis and wound infections (Johnson et al., 2012). Environmental factors influencing their abundance, such as water temperature and salinity, are known (Johnson et al., 2012; Jacobs et al., 2014), but the co-occurrence of these pathogens with other bacterial members of the plankton is poorly understood.
The objectives of this study were to assess the abundances of fecal indicator bacteria and specific Vibrio bacteria in a shellfish growing area of the Chesapeake Bay over time, and to identify members of the bacterial community, including potential pathogen groups, that co-occur with these organisms. An additional objective was to relate the occurrence of fecal coliform and Vibrio species with the greater bacterial community and with potential drivers of the temporal changes detected in these taxa.
In order to address these objectives, this study used high-throughput DNA sequencing of 16S rRNA gene PCR amplicons to identify and quantify bacterial community members. High-throughput sequencing techniques typically only provide estimates of relative abundance, and not absolute abundance, but some recent studies have overcome this limitation using internal controls (Moran et al., 2013; Satinsky et al., 2013; Smets et al., 2016). Moran et al. (2013) and Satinsky et al. (2013) added a known concentration of Thermus thermophilus HB-8 genomes to filter-concentrated plankton samples prior to DNA extraction in order to estimate the absolute abundance of genes identified in metagenome sequence libraries. Smets et al. (2016) used a similar approach to estimate the absolute abundance of 16S rRNA genes identified in PCR amplicon libraries from soils, and found that these abundances correlated with the mass of soil extracted and the abundance of bacterial cells. We used internal controls to estimate absolute abundances of 16S rRNA genes in plankton samples in order to compare the abundance of bacterial community members identified with high-throughput sequencing, with culture-derived abundances of indicator bacteria and quantitative PCR-based abundances of specific Vibrio taxa.
Materials and Methods
Water Sampling
The sampling location was a long-term Maryland Department of the Environment monitoring station near the mouth of Town Creek (Figure S1), surrounded by the Town of Oxford (Maryland, USA) to the west and agricultural fields and low density residential areas to the east. The Town of Oxford manages a secondary wastewater treatment facility that releases approximately 125,000 gal/day of effluent (Scott Delude, Public Works Director, personal communication). Because of proximity to the treatment plant, shellfish harvest and aquaculture are currently forbidden in Town Creek, though routine culture-based testing of treatment plant effluent shows fecal bacteria levels below the detection limit (<1.8 MPN (Most Probable Number) per 100 mL of sample, (standard method 9221E; APHA, 1998)). Total depth at the sampling location is approximately 3.0 m, tidal amplitude at this location averages about 0.6 m (NOAA, 2015), and salinity averages 10 ppt (current study).
Water samples were collected on 14 days between April 14 and September 3, 2014, from both surface and bottom waters, and replicate samples were collected on 9 days. Water was collected in sterile polypropylene bottles (500 mL) by hand from surface waters and by Van Dorn sampler (Wildco, Inc.) from bottom waters (0.25 m above bottom), placed on ice, and processed within 30 min of collection. Water physicochemical measurements were collected with a YSI 6600 datasonde (YSI, Inc., Yellow Springs, OH). Precipitation was measured with a tipping bucket rain gauge (Onset, Inc.) stationed approximately 1.6 km from the water collection site (Figure S1).
Recovery of DNA by water filtration was performed using established methods (Fortunato and Crump, 2011). Between 250 and 300 mL of water was filtered through a 0.2 μm Sterivex-GP filter (EMD Millipore, Darmstadt, Germany), the volume filtered was recorded, and 1 mL of filter-sterilized DNA extraction buffer (DEB; 0.1M Tris-HCL (pH 8), 0.1M Na-EDTA (pH 8), 1.5M NaCl, 5% Cetyltrimethyl ammonium bromide) was added to each filter cartridge. The filters were then stored at −80°C, and later extracted according to published methods (Crump et al., 2003), including several freeze/thaw cycles and final DNA isolation by isopropanol precipitation. Prior to extraction, approximately 60 million copies of the T. thermophilus genome (i.e., 250 ng T. thermophilus DNA) were added to each sample, in order to approximate a final ratio of 1.00:0.04 natural bacterial cells to T. thermophilus genomes, based on expected cell abundance in Chesapeake Bay waters (Heidelberg et al., 2002; Kan et al., 2006).
DNA Sequencing
DNA library preparation and sequencing followed Fadrosh et al. (2014). A segment of the 16S rRNA gene including the V3 and V4 hypervariable regions was amplified using PCR. Amplification was confirmed by running several samples on an electrophoresis gel and checking for bands with an approximate length of 469 base pairs. Universal 16S rRNA gene primers (319F and 806R) were complemented with a heterogeneity spacer sequence of variable length and an Illumina (Illumina, Inc., San Diego, CA) index sequence. Sequencing was performed on the MiSeq platform (Illumina, Inc., San Diego, California, USA), which produced an average of 131,000 reads per sample. Processing of reads was conducted using Mothur (Schloss et al., 2009; Mothur, 2015) and following Kozich et al. (2013). All reads were archived in the Sequence Read Archive (accession #SRP073436).
A phylotypic approach (Mothur command “phylotype”) was used for clustering sequences into operational taxonomic units (OTUs), with a cutpoint of 97% similarity for classification (Mothur, 2015). Chimeric sequences were identified and removed using the function chimera.uchime. OTU representative sequences were classified by comparison to the Silva database, version 1.19 (Silva, 2015), and OTUs not classified as Eubacteria (i.e., Eukaryota, mitochondria, chloroplasts, or unclassified) were deleted. The per liter abundance of 16S rRNA gene copies per OTU in the original sample population was estimated from the number of reads of the internal control, T. thermophilus. A correction factor was applied to the number of reads in each sample based on the ratio of 16S rRNA genomes added to original samples compared to the number of reads for T. thermophilus after sequence processing (Equation 1). Although this approach does not account for gene copy number per cell or differential amplification success of different bacteria, it provided an estimate of the density of 16S rRNA genes present in the samples for comparison across samples. A similar approach was taken by Smets et al. (2016) to estimate 16S rRNA gene abundances in soil samples.
Where Tt = T. thermophilus and seqs = sequences.
Fecal Coliform and Vibrio Abundance Estimations
Fecal coliform densities were estimated using the standard three-tube culture method (APHA, 1998). Test tubes containing inverted gas-tubes and various concentrations of A1 broth were inoculated with aliquots of sample water, incubated in a 35°C water bath for 3 h (±30 min), and then in a 44.5°C water bath for 21 h (±2 h). Tubes showing turbidity and gas production were counted as positive for growth of fecal coliforms. Samples were tested for fecal coliforms on all dates except August 22 when an equipment failure compromised the cultures. An estimate of the number of fecal coliform bacteria in 100 mL of water was made by dividing the Most Probable Number (MPN) by 100.
Estimates of V. vulnificus (Vv) and V. parahaemolyticus (Vp) abundance, independent of the 16S rRNA community analysis, were conducted using quantitative PCR (qPCR) (Jacobs et al., 2014), with primers and probes adapted from Panicker and Bej (2005) and Nordstrom et al. (2007), respectively (Table S1). A unique internal control was incorporated simultaneously into the qPCR assay to test for the presence and influence of inhibitors (Nordstrom et al., 2007). The V. vulnificus and V. parahaemolyticus standard curves were developed separately using optical density and spread plate cultures to estimate the Vibrio cells per milliliter of water, allowing the reporting of qPCR results as Vibrio cells per milliliter of sample. DNA for the standard curve was filtered from the spiked water samples that were diluted for spread plating, and extracted using the same protocol as the environmental samples (described in Section Water sampling). The standard curve was included on each microplate. All qPCR assays had efficiencies between 97 and 100%, standard curve R2 values greater than 0.990, and limits of detection of 2 cells per mL (200 copies per assay, assuming one copy per cell). Each assay included a negative control that was always below the limit of detection.
Selection of Potential Pathogen Groups
Bacterial genera that largely contain human pathogens or include species endemic to the human gut were identified. These taxonomic groups included Aeromonas, Arcobacter, Campylobacter, Legionella, Helicobacter, Toxoplasma, and members of the Enterobacteriaceae (e.g., Yersinia, Klebsiella, Escherichia, Shigella). In addition, genera that contain common foodborne (Scallan et al., 2011) and waterborne (Pond, 2005) human pathogens, but also include non-pathogenic members, were identified. These genera included Clostridium, Leptospira, Listeria, Mycobacterium, Staphylococcus, and Vibrio. The genera Coxiella, Rickettsia, and Franciscella, were also included in our screening of 16S rRNA gene sequences because they are listed by the United States Health and Human Services Administration as important bacterial pathogens (HHS, 2017).
Data Analyses
Prior to diversity calculations, the number of sequences per sample was rarefied to 17,500. Alpha diversity measurements (Shannon Diversity and the Chao1 estimate of taxon richness) were calculated for each sample using R (The R Foundation for Statistical Computing, version 3.1.2). Beta diversity was compared between samples using Bray-Curtis Similarity matrices and plotted with non-metric multi-dimensional scaling (nMDS) using R and by cluster analysis using PRIMER for Windows (PRIMER-E Ltd, version 6). Comparison of community composition to environmental variables was conducted via distance-based linear modeling (DistLM) using PRIMER. All other comparisons involved estimated 16S rRNA gene densities. For rare taxa, with 16S rRNA genes detected in only one or two samples, presence vs. absence was compared between 16S rRNA phylotypes, fecal coliforms (culture method), V. vulnificus and V. parahaemolyticus (qPCR) estimates. For more commonly occurring potential pathogen groups (detected in three or more samples), estimated abundances of bacterial genera (16S rRNA sequences), fecal coliforms (culture method), V. vulnificus and V. parahaemolyticus (qPCR), and environmental factors (e.g., water clarity, rainfall) were compared using Spearman correlation analysis in SAS (SAS Institute, Inc.; version 9.4). Significant correlations were then compared visually using a network analysis (R, package iGraph). All statistical tests were considered significant at p < 0.05.
Results
Environmental Conditions
Conditions changed seasonally and were similar between surface and bottom waters, with a small decrease in dissolved oxygen in bottom waters during May and August (Table S2). Mean water temperatures increased from 15°C in April to 26°C in June, and remained high through September. Salinity ranged from 9.1 to 11.7 ppt, with the lowest values in June and highest values in May and September. Water clarity (Secchi depth) decreased in June and remained low thereafter. Fifteen precipitation events occurred during the study, ranging from 0.05 to 1.30 inches of rain (Figure S2). Four events exceeding 0.50 inches of rain in the previous 24 h were sampled (May 1, May 28, June 13, and June 24), including one event (May 1) that exceeded 1 inch of rain.
Bacterial Community Composition
Using the phylotypic approach to sequence classification (“phylotype” command in Mothur software package) (Schloss et al., 2009), the samples contained an average of 541 operational taxonomic units (OTUs), and were dominated by Actinobacteria, Bacteriodetes, Cyanobacteria, and Proteobacteria (Figure 1). Temporal trends were detected for several bacteria phyla and classes, highlighted by an increasing proportion of Cyanobacteria and a decreasing proportion of Actinobacteria from May through August. Replicate samples were very similar in composition, except for surface water samples collected July 9, with replicate S1 having a notably higher percentage of Cyanobacteria than replicate S2. Shannon diversity ranged from 3.4 to 4.2 with a trend toward increased diversity over time, except for one replicate on July 9 sample (Figure 2). The Chao1 estimate of taxonomic richness changed little over time with the exceptions of high Chao1 in surface waters during the large precipitation event on May 1, and low Chao1 in surface waters on August 22 following a 10-day period of less than 0.01 inches of precipitation total (Figure 2).
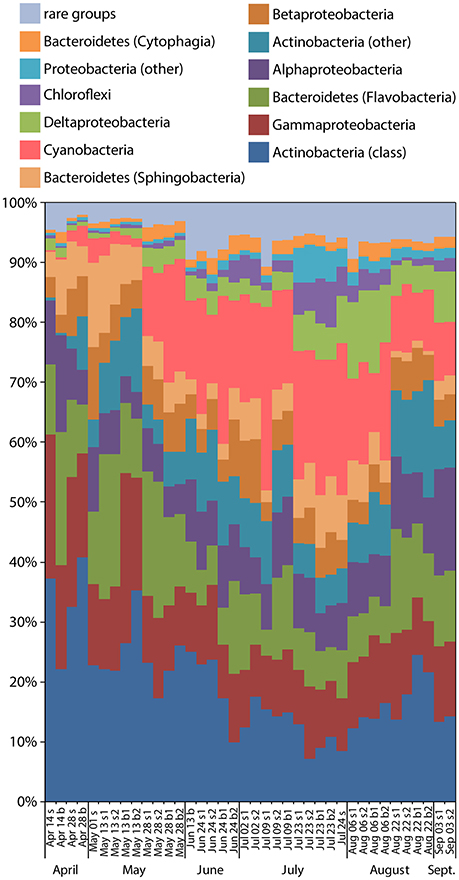
Figure 1. Relative abundances of 16S rRNA genes for the 12 most common taxonomic groups by sample. The phyla Proteobacteria were separated into Alpha-, Beta-, Gamma-, Delta-, and “other” classes; Bacteroidetes into Cytophagia, Sphingobacteriia, Flavobacteria, and “other” classes; and Actinobacteria into the class Actinobacteria and “other” classes.
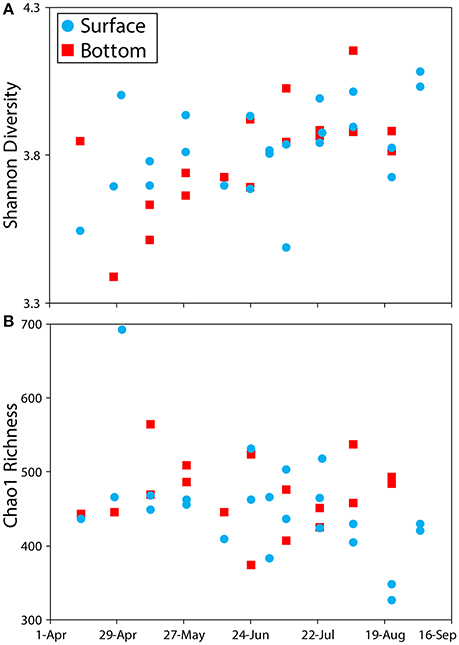
Figure 2. Measurements of alpha diversity by sample date and sample depth (surface and bottom). Panel (A) shows Shannon diversity values and panel (B) shows Chao1 richness.
Patterns in betadiversity showed that bacterial community composition was more variable in April–May, and relatively consistent in June–September (Figure 3). Bacterial community composition was significantly related to water temperature, turbidity, and salinity, which explained 29, 19, and 14% of variability in bacterial community composition, respectively (Table 1). Dissolved oxygen, wind direction, and rain in the previous 24 h were also significantly related to community composition, but they explained less of the variability (Table 1). Other variables (tide state, wind speed, cloud cover) were not significantly related bacterial community composition.
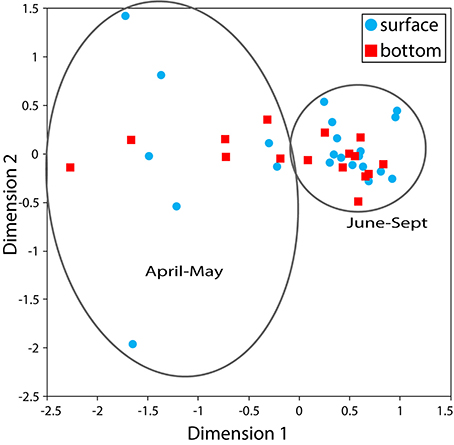
Figure 3. nMDS plot of beta diversity using Bray-Curtis similarity comparisons of log transformed 16S rRNA copy abundance. Surface nMDS plot based on 25 iterations with a final stress level of 0.01. Surface community composition (blue circles) showed higher variability in spring than in summer of fall.
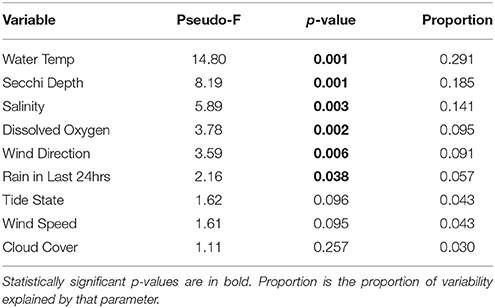
Table 1. Associations between environmental variables and patterns of community composition, as determined by distance-based linear modeling (DistLM).
Internal Control Recovery and Estimation of 16S rRNA Gene Abundances
After removing unclassified sequences and those classified as Eukaryota, mitochondria, and chloroplasts, the 16S rRNA dataset contained 5,190,588 reads classified to 1,429 phylotypic OTUs. Converting the read counts to estimates of 16S rRNA gene abundances produced similar total 16S rRNA gene densities across samples (Figure 4). Despite notable variability in T. thermophilus sequence numbers between samples, all but two samples were estimated as having between 5.4 × 105 and 5.1 × 106 16S genes/mL. These two samples (Jun13S and Jul09B2), contained very few T. thermophilus sequences, resulting in very high 16S gene abundances, and thus were eliminated from further, density-based analyses. The estimated abundance of 16S rRNA genes is similar to findings from other studies of Chesapeake Bay bacterial communities that have been measured as ranging from 2.0 to 24 × 106 cells/mL (Shiah and Ducklow, 1994; Heidelberg et al., 2002).
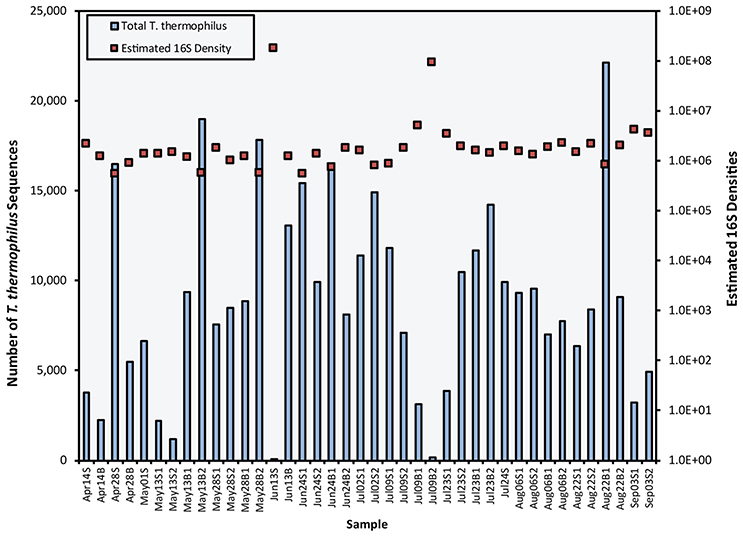
Figure 4. Comparison of the number of T. thermophilus (Tt) 16S rRNA reads (blue bars) and the estimated total 16S rRNA gene densities (#/mL) (red squares) for the 40 samples in this study. Sample names include month and day followed by S (surface) or B (bottom) and replicate number. Jun13S and Jul09B2 had unusually low numbers of Tt reads, indicating an unusually high density of bacteria or some error in the addition of Tt genes to those samples.
Genera With Pathogenic Members
A number of bacterial genera that include human pathogens were detected in the 16S rRNA gene sequences. All 38 samples contained members of the genus Vibrio, Arcobacter (Epsilonproteobacteria) spp., Clostridium spp. (Firmicutes), and Legionella spp. (Gammaproteobacteria) (Figure 5, Table 2), and most samples contained members of Pseudomonas spp. (Gammaproteobacteria), Aeromonas spp. (Gammaproteobacteria) and Mycobacterium spp. (Actinobacteria). Bacteria were also detected from 11 genera of the family Enterobacteriaceae, which includes most genera considered fecal coliform bacteria. Enterococcus spp. were detected in two samples (Table 2). The 16S rRNA gene densities for a number of individual Enterobacteriaceae groups as well as other potential pathogen containing groups, including Aeromonas, Arcobacter, Bacteroides, Legionella, and several genera of Clostridium were notably elevated or were exclusively detected on May 1 (Figure 5, Table 2). Bacterial groups not detected in these samples included E. coli, Campylobacter, Salmonella, Listeria, and Helicobacter.
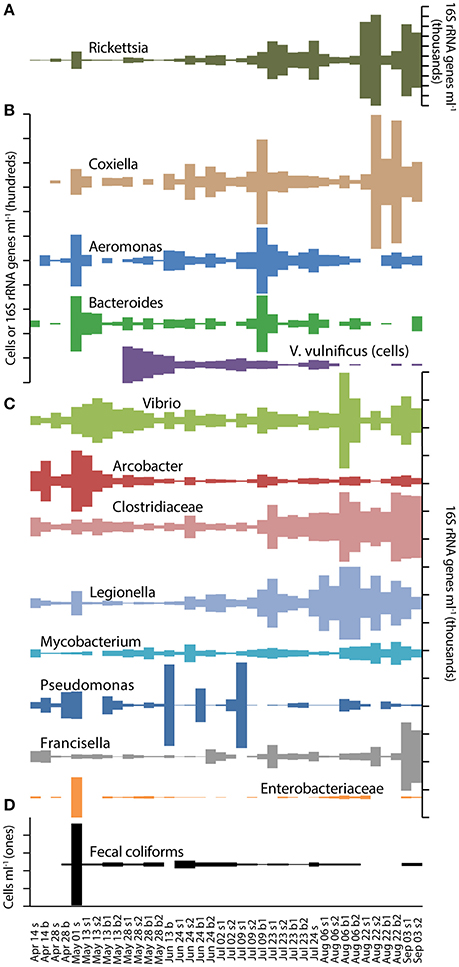
Figure 5. Abundances of V. vulnificus cells (qPCR), pathogen-containing phylogenetic groups (16S amplicons), and fecal coliforms (culturing) on scales of (A) thousands per ml, (B) hundreds per ml, (C) thousands per ml, and (D) individuals per ml on each sampling date.
Significant correlations (Spearman rank correlation, p < 0.05) were detected between the abundances of pathogen containing groups, fecal coliforms and Vibrio vulnificus (Figure 6, Table S3). These groups fall into two clusters, which show the allochthonous community members correlating with fecal coliforms, and the more persistent members forming a separate cluster with Vibrio spp. The abundance of V. vulnificus was negatively correlated with two members of this second cluster, reflecting the persistent nature of V. vulnificus but its inverse abundance compared to these two other pathogens (Figure 5).
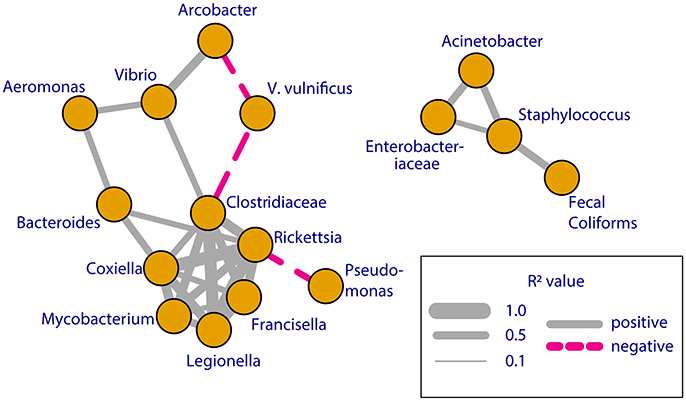
Figure 6. Correlation network of select pathogens (16S rRNA), fecal coliforms (culture), and V. vulnificus (qPCR) found in three or more samples. Connections represent significant (p < 0.05) Spearman rank correlations between pairs of taxa. Line thickness reflects the strength of this relationship (R2-value) and line color reflects whether the relationship was positive (solid, gray) or negative (dashed, red). All taxa included were correlated to at least one other taxa (Table S3). Line distance is not reflective of strength of correlation.
Several significant correlations (p < 0.05) occurred between the abundances of potential pathogen OTUs and environmental factors (Table S4). Clostridiaceae, Legionella, and Mycobacterium were positively correlated with the day of the year (DOY). Bacteroides and Legionella were positively correlated to water temperature, and several taxa were correlated with water temperature and Secchi depth, although some relationships were negative and others positive.
Fecal Coliform Bacteria and Vibrio Species
Fecal coliforms were detected on 11 of 14 sampling dates in both surface and bottom waters using standard culture methods (Figure 5C). All samples contained less than 0.5 cells per mL except on May 1 when fecal coliform abundance spiked to 4.5 cells/mL during the largest rain event. In contrast, samples collected on May 28, June 13 and July 24 contained relatively low fecal coliform densities, despite occurring soon after smaller rain events. Fecal coliforms were positively correlated with Staphylococcus 16S rRNA gene abundance, but not with any other potential pathogen OTUs (Table S3).
V. vulnificus was detected by qPCR assay in most samples after May 13 (Figure 5A), with greater than 100 cells per mL in the May 28 surface samples. V. vulnificus densities declined in mid-summer, and then increased in bottom water samples on August 6. V. vulnificus abundance did not correlate with Vibrio 16S rRNA gene abundance (Table S3). Vibrio parahaemolyticus was only detected in four samples with a maximum abundance of 4.7 cells/mL in one of the August 6 samples.
Detection of Potential and Existing Indicators
Several bacterial genera belonging to families that have been proposed as indicators of fecal pollution, several families of the order Bacteroidales and the family Lachnospiraceae, peaked in abundance on May 1 (Table 3), concurrent with a number of potential pathogen groups (Table 2).
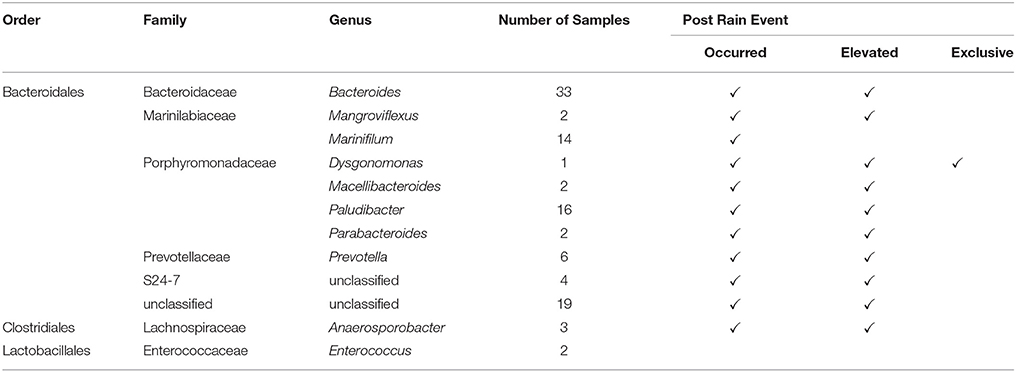
Table 3. The occurrence of potential (Bacteroidales and Clostridiales) and existing (Enterococcus) fecal indicator groups, the number of samples (out of 38) in which they occurred, those that were detected on May 1 (Occurred), those that were elevated on May 1 at least 2 times the standard deviation above their mean (Elevated), and those that were only detected on May 1 (Exclusive).
Discussion
Deep amplicon sequencing of 16S rRNA genes at the shellfish monitoring station examined in this study identified many pathogen-containing bacterial families and genera that were present throughout the spring and summer. Other pathogen containing groups identified by 16S rRNA sequencing were greatly elevated in abundance and diversity on May 1 following the only rainstorm that exceeded 1 inch in the previous 24 h. Fecal coliforms were also in high abundance after this rainstorm, and, over time, their abundance correlated with the abundance of Staphylococcus sp. 16S genes. In contrast, V. vulnificus (quantified via qPCR) were absent after this rainstorm, and did not appear until May 28 when water temperature increased to 24.2°C and salinity decreased to 9.3 ppt. V. vulnificus were detected in most samples after May 28, but did not correlate with fecal coliform abundance, Vibrio sp. 16S rRNA genes, or with any other taxa except for weak negative correlations with Arcobacter sp. and Pseudomonas sp. 16S rRNA genes. Thus, while 16S rRNA gene amplicon sequencing detected potential pathogens in Chesapeake Bay waters, elevated abundances of some of these organisms only corresponded to elevated abundances of the fecal coliform indicator method following a major rainstorm. These results demonstrate that many potential pathogens co-occur with high densities of fecal coliform bacteria following large storms, but are poorly correlated at other times, and do not include autochthonous potential pathogens like V. vulnificus.
Changes in the broader bacterial community over time correlated with seasonal changes in environmental factors including water temperature, Secchi depth, dissolved oxygen, and salinity. Similar seasonal patterns have been seen in other estuaries (Crump et al., 2003; Fortunato and Crump, 2011; Pereira et al., 2015), and are likely driven by species sorting of microbial communities in order to adapt to multiple environmental factors that change as seasons shift from spring to summer to fall. This is consistent with Kan et al. (2006) who found that seasonal variability in bacterial communities at any particular location in Chesapeake Bay far exceeded spatial variability across the entire salinity gradient of Chesapeake Bay. Kan et al. (2006) found that this variability was most strongly correlated with seasonal changes in temperature and chlorophyll a concentration, matching well with the top environmental correlates from the shellfish monitoring station (temperature and Secchi depth). This suggests that the microbial community at the shellfish monitoring station is representative of microbial communities throughout the Chesapeake Bay, and that variability driven by inputs of allochthonous organisms from land is infrequent and temporary.
The greatest change in community composition at the monitoring station occurred after a 24-h rainfall of 1.3 inches, and featured a decrease in typical planktonic taxa (Actinobacteria, Gammaproteobacteria), and an increase in Bacteroidetes, Cyanobacteria, and several taxa typical of soils (Acidobacteria, Firmicutes). Alpha diversity of the bacterial community was also highest on that day, further supporting the idea that this rainfall introduced allochthonous (i.e., non-endemic) bacteria to the community. This suggests that runoff from land can influence the chance of encountering pathogen-containing phylogenetic groups in nearshore water, but only for short periods of time. It also suggests a nonlinear relationship between precipitation and the bacterial community, with some threshold below 1.3 inches of rain capable of causing a distinct shift in the community. Salinity at the site was lowered by 1.4 ppt following this storm, suggesting a 13% dilution with rainwater. This level of dilution was not seen following any of the smaller storms.
Trends in the abundance of bacterial taxa of concern to shellfish harvest managers followed two general patterns that appear to distinguish between autochthonous (endemic) bacteria and allochthonous (land-based or benthic) bacteria. Mycobacterium, Legionella, and Clostridiaceae are autochthonous taxa commonly found in fresh and estuarine waters, with densities driven by water conditions like temperature and salinity (Grimes, 1991). In samples where they were detected, 16S rRNA gene abundances for many of these groups were positively correlated with each other, and were positively correlated with temperature and day of the year. Similarly, the abundances of potential pathogen groups typically associated with runoff and land-based sources (Ferguson et al., 1996; Ackerman and Weisberg, 2003), such as Enterobacteriaceae, Aeromonas, Arcobacter and Bacteroides did not correlate with the autochthonous groups but some correlated with each other. However, few of these allochthonous taxa correlated positively with temperature because they spiked in abundance in cooler water following the large rain event on May 1, and several were also abundant in bottom waters on July 9. Vibrio sp. abundance was correlated with members of both of these groups of taxa, including Clostridiaceae and Arcobacter, likely reflecting the highly variable characteristics and life cycles of diverse Vibrio species (Thompson et al., 2004). Several pathogen groups, such as Salmonella, Campylobacter, and Listeria were never detected, despite previous evidence of the presence of some of these groups in Chesapeake Bay waters (Sayler et al., 1975) and shellfish meat (Rawles et al., 1995). The detection of Coxiella, Francisella, and Rickettsia bacteria in most samples despite their lack of association with waterborne diseases advocates for the monitoring of these pathogen groups in natural water bodies.
The occurrence of both autochthonous and allochthonous pathogen-containing genera underscores the need for a management strategy capable of assessing potential pathogens from multiple sources in shellfish and recreational waters. For example, the appearance or increase in abundance of several members of the groups Enterobacteriaceae, Acrobacter, Aeromonas, and Bacteroides, and their co-occurrence with a spike in culture-based fecal coliform densities supports the use of these indicators as criteria for closing shellfish beds to harvest (FDA, 2013). Also, the co-occurrence of several genera of Bacteriodales and Lachnospiraceae with pathogen-containing groups encourages further study into using these families as alternative indicators of human waste (Bernhard and Field, 2000; Newton et al., 2011). However, a different strategy is required for pathogen groups that are not related to precipitation. The ubiquity of some of these groups, such as V. vulnificus, is known (Wright et al., 1996; Jacobs et al., 2014), particularly in summer months. The detection of Vibrio, Pseudomonas, Legionella, Mycobacterium, and Clostridiaceae 16S rRNA genes in most of the samples is also consistent with their ubiquitous presence in aquatic environments. The strategy for assessing the presence and/or abundance of these endemic pathogen-containing groups may rely upon qPCR detection in shellfish tissues (Brasher et al., 1998) or the use of spatially-explicit predictive models (Jacobs et al., 2014). The challenge to the management community is to discover and implement cost-effective monitoring tools that will more accurately assess the relative risk of encountering both allochthonous and autochthonous pathogens.
Our 16S rRNA gene classification of the bacterial community at a shellfish monitoring station identified a broad range of potential pathogen groups that may serve as useful indicators of poor water quality. However, all of these taxonomic groups include both virulent and non-virulent strains, suggesting that it is necessary to develop procedures that target species-specific genes and virulence genes from virulent members of these groups in order to make management decisions about water quality in shellfish growing areas. Although virulence factors are complex and subject to evolution and transfer between microbes (Hacker et al., 1997), future study of their occurrence, coupled with 16S rRNA gene diversity studies, in shellfish waters may improve our assessment of risks to human health.
Using an internal control to normalize sequences across samples and to estimate 16S rRNA gene densities is a powerful new approach for microbial ecology (Satinsky et al., 2013; Smets et al., 2016), because it provides per liter abundances of individual microbial taxa for comparison with qPCR and culture-based estimates. Estimates of total bacterial 16S rRNA genes in our samples were within the range of values for abundance of total bacterial cells in estuarine waters (Ducklow, 1982). However, the number of 16S rRNA genes per bacterial cell varies between 1 and 15 (Kembel et al., 2012), with greater numbers typical of copiotrophic bacteria that maintain large amounts of genetic material in order to quickly take advantage of nutrient introductions (Klappenbach et al., 2000). Although methods for estimating bacterial cell abundance based on 16S rRNA gene copies have been developed (Kembel et al., 2012), there are still large groups of uncultured bacteria for which the number of 16S rRNA genes per cell are unknown. This approach may be strengthened in future studies by making additional estimates of cell densities from staining and direct counting of total bacterial cells, from fluorescence in-situ hybridization using probes for specific groups, and from qPCR methods.
Although the use of an internal control to estimate the abundance of 16S rRNA genes in environmental samples shows some promise, differences in abundance estimates between 16S sequencing and the culture and qPCR-based methods suggest that there are some unquantified biases in these techniques. Enterobacteriaceae (16S sequencing) and fecal coliform (culture) bacteria co-occurred, due primarily to the peak in abundance on May 1, but cultured fecal coliform abundances were two to three orders of magnitude lower than the 16S rRNA gene densities (Figure 5). This discrepancy is likely a result of the fact that the family Enterobacteriaceae includes members that are not considered fecal coliforms and that do not grow optimally at 44°C. Additionally, Enterobacteriaceae densities based on 16S rRNA gene data may include bacteria that were non-culturable and therefore not represented in the fecal coliform counts, reflecting the well-known difficulties in quantitatively culturing bacteria (Staley and Konopka, 1985). Some of this discrepancy could also partially be explained if naturally-occurring Enterobacteriaceae have multiple 16S genes per genome (Stoddard et al., 2015).
Total Vibrio 16S rRNA gene abundance was up to approximately 500 times greater than V. vulnificus abundance estimated by qPCR (Aug 6 bottom sample), and averaged approximately 30 times greater. However, nine samples contained V. vulnificus estimates greater than the total Vibrio (16S) estimate suggesting that, for these samples, either the qPCR technique overestimated V. vulnificus abundance or amplicon sequencing underestimated Vibrio spp. 16S gene abundance. The qPCR method quantifies V. vulnificus by comparing sample data to a standard curve prepared with known densities of cultured V. vulnificus cells. This method could overestimate V. vulnificus if the copy number of genes targeted by qPCR is elevated in natural populations of V. vulnificus compared to the cultured representatives. It is also possible that the PCR used to amplify 16S rRNA genes prior to sequencing under-amplified Vibrio sp. 16S rRNA genes, although the 16S rRNA PCR primers used for this study exactly match T. thermophilus and all Enterobacteriaceae and Vibrio taxa in the Silva database (v1.19). However, PCR bias may have affected estimates of 16S rRNA genes due to differences in the secondary structure and the G:C content of the various amplicons (Suzuki and Giovannoni, 1996). Pinto and Raskin (2012) showed that, although increased sequencing depth corresponded to improved taxa detection frequency, amplicon structure can affect the number of reads. Suzuki et al. (1998) also showed that over-amplification of genes in a mixed bacterial community can cause over-representation of rare taxa and underrepresentation of more common taxa, but in this study, Vibrio would be considered rare and therefore unlikely to be under-amplified. An important next step in using T. thermophilus or similar internal control approaches for estimating cell abundances is the development of a quantitative PCR assay to assess the recovery of the internal control after sample extraction.
Despite these discrepancies, 16S rRNA gene amplicon sequencing allowed a comprehensive examination of bacterial communities in Chesapeake Bay waters, providing information useful in assessing potential human health risks associated with exposures to this water body. For example, Acinetobacter bacteria, detected in 15 of 38 samples, is a genus of bacteria found in soil and water at low densities (Baumann, 1968). Some members of this genus are known to express multi-drug resistance and virulence factors (Towner, 2009). Moreover, Zhang et al. (2009) showed that certain wastewater treatment processes may select for multi-drug-resistant Acinetobacter strains in receiving waters. Dysgonomonas, which were present on May 1, are much less common in aquatic environments, but are also known for multi-drug resistance and pathogenicity (Vaughan and Forbes, 2014). As sequencing methods produce larger numbers of reads per sample, and as read classification improves with expanding phylogenetic databases, assessments of bacterial communities and associated pathogens in shellfish growing and recreational-use areas will improve our understanding of the ecology of these bacterial groups and how to best manage human health risks.
Several conclusions may be drawn from this study. Significant changes in bacterial community composition, including fecal bacteria and fecal indicators may occur from single rain events with a threshold of precipitation occurring at this sampling location. Water temperature may also influence bacterial community composition over time. PCR quantification of V. vulnificus and V. parahaemolyticus showed that only a subset of samples contained these pathogens at densities above the limit of detection and that their abundances did not correlate with the ubiquitous Vibrio 16S rRNA gene densities. If coupled with virulence information, 16S rRNA gene sequencing studies may provide useful information regarding the types, amounts, and temporal dynamics of pathogenic bacteria that occur in managed waters and be used to inform decisions about seafood safety.
Author Contributions
AL, BC, and RH conceived and designed the study; AL performed sample collection and processing; AL and BC performed data analysis; AL, BC, and RH drafted and critically revised the paper. All authors read and approved the final version of the manuscript.
Funding
BC was supported by National Oceanic and Atmospheric Administration (NOAA) award EA133C07CN0163. RH's participation in this effort was supported by multiple awards from NOAA, NASA and NSF.
Disclaimer
The scientific results and conclusions, as well as any opinions expressed herein, are those of the author(s) and do not necessarily reflect the views of NOAA or the Department of Commerce. The mention of any commercial product is not meant as an endorsement by the Agency or Department.
Conflict of Interest Statement
The authors declare that the research was conducted in the absence of any commercial or financial relationships that could be construed as a potential conflict of interest.
Acknowledgments
Amy Sapkota of the University of Maryland, School of Public Health helped design the study and reviewed an earlier draft. The authors wish to thank Tsvetan Bachvaroff of the University of Maryland Center for Environmental Science, Institute of Marine and Environmental Technology for access to and assistance with the Linux machine used for bioinformatics. Jason Steele and Juan Maldonado of the Center for Personalized Diagnostics, The Biodesign Institute, Arizona State University developed the DNA library and conducted the 16S sequencing. Lindy Fine provided instruction and advice on DNA extraction. Thomas Sharpton and Ryan Mueller of the Department of Microbiology, School of Agricultural Sciences, Oregon State University consulted on the use of an internal control as a means of estimating 16S gene densities. Amy Freitag, of Jardon and Howard Technologies, Inc. assisted with the network analysis. This is UMCES contribution no. 5422.
Supplementary Material
The Supplementary Material for this article can be found online at: https://www.frontiersin.org/articles/10.3389/fmicb.2018.00384/full#supplementary-material
References
Ackerman, D., and Weisberg, S. B. (2003). Relationship between rainfall and beach bacterial concentrations on Santa Monica Bay beaches. J. Water Health 1, 85–89.
APHA (1998). Standard Methods for the Examination of Water and Wastewater, 20th Edn. Washington, DC: American Public Health Association.
Ashbolt, N. J., Grabow, W. O. K., and Snozzi, M. (2001). “Indicators of microbial water quality,” in Water Quality: Guidelines, Standards and Health, eds. L. Fewtrell and J. Bartram (London: IWA Publishing), 289–315.
Bernhard, A. E., and Field, K. G. (2000). A PCR assay to discriminate human and ruminant feces on the basis of host differences in Bacteroides-Prevotella genes encoding 16S rRNA. Appl. Environ. Microbiol. 66, 4571–4574. doi: 10.1128/AEM.66.10.4571-4574.2000
Brasher, C. W., DePaola, A., Jones, D. D., and Bej, A. K. (1998). Detection of microbial pathogens in shellfish with multiplex PCR. Curr. Microbiol. 37, 101–107. doi: 10.1007/s002849900346
Comte, J., and del Giorgio, P. A. (2010). Linking the patterns of change in composition and function in bacterioplankton successions along environmental gradients. Ecology 91, 1466–1476. doi: 10.1890/09-0848.1
Crump, B. C., Hopkinson, C. S., Sogin, M. L., and Hobbie, J. E. (2004). Microbial biogeography along an estuarine salinity gradient: combined influences of bacterial growth and residence time. Appl. Environ. Microbiol. 70, 1494–1505. doi: 10.1128/AEM.70.3.1494-1505.2004
Crump, B. C., Kling, G. W., Bahr, M., and Hobbie, J. E. (2003). Bacterioplankton community shifts in an arctic lake correlate with seasonal changes in organic matter source. Appl. Environ. Microbiol. 69, 2253–2268. doi: 10.1128/AEM.69.4.2253-2268.2003
Ducklow, H. W. (1982). Chesapeake Bay nutrient and plankton dyanimcs. 1. Bacterial biomass and production during spring tidal destratification in the York River, Virginia, estuary. Limnol. Oceanogr. 27, 651–659.
Fadrosh, D. W., Ma, B., Gajer, P., Sengamalay, N., Ott, S., Brotman, R. M., et al. (2014). An improved dual-indexing approach for multiplexed 16S rRNA gene sequencing on the Illumina MiSeq platform. Microbiome 2, 7. doi: 10.1186/2049-2618-2-6
FDA (2013). National Shellfish Sanitation Program (NSSP) Guide for the Control of Molluscan Shellfish: 2013 Revision. Washington, DC: FDA.
Ferguson, C. M., Coote, B. G., Ashbolt, N. J., and Stevenson, I. M. (1996). Relationships between indicators, pathogens and water quality in an estuarine system. Water Res. 30, 2045–2054. doi: 10.1016/0043-1354(96)00079-6
Fortunato, C. S., and Crump, B. C. (2011). Bacterioplankton community variation across river to ocean environmental gradients. Microb. Ecol. 62, 374–382. doi: 10.1007/s00248-011-9805-z
Grimes, D. J. (1991). Ecology of Estuarine bacteria capable of causing human-disease - a review. Estuaries 14, 345–360. doi: 10.2307/1352260
Hacker, J., BlumOehler, G., Mühldorfer, I., and Tschäpe, H. (1997). Pathogenicity islands of virulent bacteria: structure, function and impact on microbial evolution. Mol. Microbiol. 23, 1089–1097. doi: 10.1046/j.1365-2958.1997.3101672.x
Heidelberg, J. F., Heidelberg, K. B., and Colwell, R. R. (2002). Seasonality of Chesapeake Bay bacterioplankton species. Appl. Environ. Microbiol. 68, 5488–5497. doi: 10.1128/AEM.68.11.5488-5497.2002
HHS (2017). Select Agents List [Online]. United States Health and Human Services Administration. Available online at: www.selectagents.gov/SelectAgentsandToxinsList (Accessed December 27, 2017).
Hood, M. A., Ness, G. E., and Blake, N. J. (1983). Relationship among fecal coliforms, Escherichia coli, and Salmonella spp. in shellfish. Appl. Environ. Microbiol. 45, 122–126.
Ibekwe, A. M., Leddy, M., and Murinda, S. E. (2013). Potential human pathogenic bacteria in a mixed urban watershed as revealed by pyrosequencing. PLoS ONE 8:e0794909. doi: 10.1371/journal.pone.0079490
Inkinen, J., Jayaprakash, B., Santo Domingo, J. W., Keinänen-Toivola, M. M., Ryu, H., and Pitkänen, T. (2016). Diversity of ribosomal 16S DNA- and RNA-based bacterial community in an office building drinking water system. J. Appl. Microbiol. 120, 1723–1738. doi: 10.1111/jam.13144
Jacobs, J. M., Rhodes, M., Brown, C. W., Hood, R. R., Leight, A., Long, W., et al. (2014). Modeling and forecasting the distribution of Vibrio vulnificus in Chesapeake Bay. J. Appl. Microbiol. 117, 1312–1327. doi: 10.1111/jam.12624
Johnson, C. N., Bowers, J. C., Griffitt, K. J., Molina, V., Clostio, R. W., Pei, S. F., et al. (2012). Ecology of Vibrio parahaemolyticus and Vibrio vulnificus in the coastal and estuarine waters of Louisiana, Maryland, Mississippi, and Washington (United States). Appl. Environ. Microbiol. 78, 7249–7257. doi: 10.1128/AEM.01296-12
Kan, J. J., Crump, B. C., Wang, K., and Chen, F. (2006). Bacterioplankton community in Chesapeake Bay: predictable or random assemblages. Limnol. Oceanogr. 51, 2157–2169. doi: 10.4319/lo.2006.51.5.2157
Kembel, S. W., Wu, M., Eisen, J. A., and Green, J. L. (2012). Incorporating 16S gene copy number information improves estimates of microbial diversity and abundance. PLoS Comput. Biol. 8:e1002743. doi: 10.1371/journal.pcbi.1002743
Klappenbach, J. A., Dunbar, J. M., and Schmidt, T. M. (2000). rRNA operon copy number reflects ecological strategies of bacteria. Appl. Environ. Microbiol. 66, 1328–1333. doi: 10.1128/AEM.66.4.1328-1333.2000
Kozich, J. J., Westcott, S. L., Baxter, N. T., Highlander, S. K., and Schloss, P. D. (2013). Development of a dual-Index sequencing strategy and curation pipeline for analyzing amplicon sequence data on the MiSeq Illumina sequencing platform. Appl. Environ. Microbiol. 79, 5112–5120. doi: 10.1128/AEM.01043-13
MDDNR (2015). Harvest Reporting [Online]. Maryland Department of Natural Resources. Available online at: http://dnr.maryland.gov/fisheries/Pages/aquaculture/harvest-reporting.aspx (Accessed December 30, 15).
Moran, M. A., Satinsky, B., Gifford, S. M., Luo, H., Rivers, A., Chan, L. K., et al. (2013). Sizing up metatranscriptomics. ISME J. 7, 237–243. doi: 10.1038/ismej.2012.94
Mothur (2015). MiSeq SOP [Online]. Available online at: www.mothur.org/wiki/MiSeq_SOP (Accessed June 4, 2015).
Newton, R. J., VandeWalle, J. L., Borchardt, M. A., Gorelick, M. H., and McLellan, S. L. (2011). Lachnospiraceae and Bacteroidales alternative fecal indicators reveal chronic human sewage contamination in an urban harbor. Appl. Environ. Microbiol. 77, 6972–6981. doi: 10.1128/AEM.05480-11
NOAA (2015). Tides and Currents [Online]. Available online at: https://tidesandcurrents.noaa.gov/ (Accessed December 22, 15).
Noble, R. T., and Fuhrman, J. A. (2001). Enteroviruses detected by reverse transcriptase polymerase chain reaction from the coastal waters of Santa Monica Bay, California: low correlation to bacterial indicator levels. Hydrobiologia 460, 175–184. doi: 10.1023/A:1013121416891
Nordstrom, J. L., Vickery, M. C., Blackstone, G. M., Murray, S. L., and DePaola, A. (2007). Development of a multiplex real-time PCR assay with an internal amplification control for the detection of total and pathogenic Vibrio parahaemolyticus bacteria in oysters. Appl. Environ. Microbiol. 73, 5840–5847. doi: 10.1128/AEM.00460-07
Panicker, G., and Bej, A. K. (2005). Real-time PCR detection of Vibrio vulnificus in oysters: comparison of oligonucleotide primers and probes targeting vvhA. Appl. Environ. Microbiol. 71, 5702–5709. doi: 10.1128/AEM.71.10.5702-5709.2005
Pereira, C., Santos, L., Silva, A. P., Silva, Y. J., Cunha, A., Romalde, J. L., et al. (2015). Seasonal variation of bacterial communities in shellfish harvesting waters: preliminary study before applying phage therapy. Mar. Pollut. Bull. 90, 68–77. doi: 10.1016/j.marpolbul.2014.11.019
Pinto, A. J., and Raskin, L. (2012). PCR biases distort bacterial and archaeal community structure in pyrosequencing datasets. PLoS ONE 7:e43093. doi: 10.1371/journal.pone.0043093
Pond, K. (2005). Water Recreation and Disease: Plausibility of Associated Infections: Acute Effects, Sequalae and Mortality. Geneva: World Health Organization.
Rawles, D. D., Flick, G., Diallo, A., and Croonenberghs, R. (1995). Growth of mixed cultures of Listeria-monocytogenes and listeria-innocua in blue-crab (Callinectes-Sapidus) meat. J. Food Prot. 58, 1268–1270. doi: 10.4315/0362-028X-58.11.1268
Rhodes, M. W., and Kator, H. (1988). Survival of Escherichia coli and Salmonella spp. in estuarine environments. Appl. Environ. Microbiol. 54, 2902–2907.
Satinsky, B. M., Gifford, S. M., Crump, B. C., and Moran, M. A. (2013). Use of internal standards for quantitative metatranscriptome and metagenome analysis. Methods Enzymol. 531, 237–250. doi: 10.1016/B978-0-12-407863-5.00012-5
Sayler, G. S., Nelson, J. D. Jr., Justice, A., and Colwell, R. R. (1975). Distribution and significance of fecal indicator organisms in the upper Chesapeake Bay. Appl. Environ. Microbiol. 30, 625–638.
Scallan, E., Hoekstra, R. M., Angulo, F. J., Tauxe, R. V., Widdowson, M. A., Roy, S. L., et al. (2011). Foodborne illness acquired in the United States–major pathogens. Emerging Infect. Dis. 17, 7–15. doi: 10.3201/eid1701.P11101
Schloss, P. D., Westcott, S. L., Ryabin, T., Hall, J. R., Hartmann, M., Hollister, E. B., et al. (2009). Introducing mothur: open-source, platform-independent, community-supported software for describing and comparing microbial communities. Appl. Environ. Microbiol. 75, 7537–7541. doi: 10.1128/AEM.01541-09
Shiah, F. K., and Ducklow, H. W. (1994). Temperature and substrate regulation of bacterial abundance, production and specific growth-rate in Chesapeake Bay, U.S.A. Mar. Ecol. Prog. Series 103, 297–308. doi: 10.3354/meps103297
Shrestha, R. G., Tanaka, Y., Malla, B., Bhandari, D., Tandukar, S., Inoue, D., et al. (2017). Next-generation sequencing identification of pathogenic bacterial genes and their relationship with fecal indicator bacteria in different water sources in the Kathmandu Valley, Nepal. Sci. Total Environ. 601, 278–284. doi: 10.1016/j.scitotenv.2017.05.105
Silva (2015). Silva Ribosomal RNA Database, Version 1.19 [Online]. Available online at: www.arb-silva.de (Accessed February 18, 2015).
Smets, W., Leff, J. W., Bradford, M. A., McCulley, R. L., Lebeer, S., and Fierer, N. (2016). A method for simultaneous measurement of soil bacterial abundances and community composition via 16S rRNA gene sequencing. Soil Biol. Biochem. 96, 145–151. doi: 10.1016/j.soilbio.2016.02.003
Staley, J. T., and Konopka, A. (1985). Measurement of in situ activities of nonphotosynthetic microorganisms in aquatic and terrestrial habitats. Annu. Rev. Microbiol. 39, 321–346. doi: 10.1146/annurev.mi.39.100185.001541
Stoddard, S. F., Smith, B. J., Hein, R., Roller, B. R. K., and Schmidt, T. M. (2015). rrnDB: improved tools for interpreting rRNA gene abundance in bacteria and archaea and a new foundation for future development. Nucleic Acids Res. 43, D593–D598. doi: 10.1093/nar/gku1201
Suzuki, M., and Giovannoni, S. J. (1996). Bias caused by template annealing in the amplification of mixtures of 16S rRNA genes by PCR. Appl. Environ. Microbiol. 62, 625–630.
Suzuki, M., Rappe, M. S., and Giovannoni, S. J. (1998). Kinetic bias in estimates of coastal picoplankton community structure obtained by measurements of small-subunit rRNA gene PCR amplicon length heterogeneity. Appl. Environ. Microbiol. 64, 4522–4529.
Thompson, J. R., Randa, M. A., Marcelino, L. A., Tomita-Mitchell, A., Lim, E., and Polz, M. F. (2004). Diversity and dynamics of a north Atlantic coastal Vibrio community. Appl. Environ. Microbiol. 70, 4103–4110. doi: 10.1128/AEM.70.7.4103-4110.2004
Towner, K. J. (2009). Acinetobacter: an old friend, but a new enemy. J. Hospital Infect. 73, 355–363. doi: 10.1016/j.jhin.2009.03.032
Vaughan, L. B., and Forbes, B. A. (2014). A 50-year-old with a 2-day history of right upper quadrant tenderness and septicemia caused by a gram-negative organism answer to photo quiz: Dysgonomonas capnocytophagoides. J. Clin. Microbiol. 52, 1811. doi: 10.1128/jcm.00385-13
Wade, T. J., Pai, N., Eisenberg, J. N., and Colford, J. M. Jr. (2003). Do, U.S. Environmental Protection Agency water quality guidelines for recreational waters prevent gastrointestinal illness? a systematic review and meta-analysis. Environ. Health Perspect. 111, 1102–1109. doi: 10.1289/ehp.6241
Walters, S. P., Gannon, V. P. J., and Field, K. G. (2007). Detection of Bacteroidales fecal indicators and the zoonotic pathogens E. coli O157 : H7, Salmonella, and Campylobacter in river water. Environ. Sci. Technol. 41, 1856–1862. doi: 10.1021/es0620989
Wright, A. C., Hill, R. T., Johnson, J. A., Roghman, M. C., Colwell, R. R., and Morris, J. G. Jr. (1996). Distribution of Vibrio vulnificus in the Chesapeake Bay. Appl. Environ. Microbiol. 62, 717–724.
Keywords: microbiome, estuary, human health, shellfish closures, pathogens
Citation: Leight AK, Crump BC and Hood RR (2018) Assessment of Fecal Indicator Bacteria and Potential Pathogen Co-Occurrence at a Shellfish Growing Area. Front. Microbiol. 9:384. doi: 10.3389/fmicb.2018.00384
Received: 02 November 2017; Accepted: 20 February 2018;
Published: 14 March 2018.
Edited by:
Raquel Peixoto, Universidade Federal do Rio de Janeiro, BrazilReviewed by:
Jer-Horng Wu, National Cheng Kung University, TaiwanJanelle Renee Thompson, Massachusetts Institute of Technology, United States
Copyright © 2018 Leight, Crump and Hood. This is an open-access article distributed under the terms of the Creative Commons Attribution License (CC BY). The use, distribution or reproduction in other forums is permitted, provided the original author(s) and the copyright owner are credited and that the original publication in this journal is cited, in accordance with accepted academic practice. No use, distribution or reproduction is permitted which does not comply with these terms.
*Correspondence: Andrew K. Leight, YWsubGVpZ2h0QG5vYWEuZ292