- 1Departamento de Biología, Facultad de Química y Biología, Universidad de Santiago de Chile, Santiago, Chile
- 2Centro de Estudios en Ciencia y Tecnología de Alimentos, Universidad de Santiago de Chile, Santiago, Chile
- 3Millennium Institute for Integrative Systems and Synthetic Biology, Santiago, Chile
- 4Departamento de Genética Molecular y Microbiología, Facultad de Ciencias Biológicas, Pontificia Universidad Católica de Chile, Santiago, Chile
- 5Departamento de Ciencia y Tecnología de los Alimentos, Facultad Tecnológica, Universidad de Santiago de Chile, Santiago, Chile
Glycerol is one of the most important by-products of alcohol fermentation, and depending on its concentration it can contribute to wine flavor intensity and aroma volatility. Here, we evaluated the potential of utilizing the natural genetic variation of non-coding regions in budding yeast to identify allelic variants that could modulate glycerol phenotype during wine fermentation. For this we utilized four Saccharomyces cerevisiae strains (WE - Wine/European, SA – Sake, NA – North American, and WA – West African), which were previously profiled for genome-wide Allele Specific Expression (ASE) levels. The glycerol yields under Synthetic Wine Must (SWM) fermentations differed significantly between strains; WA produced the highest glycerol yields while SA produced the lowest yields. Subsequently, from our ASE database, we identified two candidate genes involved in alcoholic fermentation pathways, ADH3 and GPD1, exhibiting significant expression differences between strains. A reciprocal hemizygosity assay demonstrated that hemizygotes expressing GPD1WA, GPD1SA, ADH3WA and ADH3SA alleles had significantly greater glycerol yields compared to GPD1WE and ADH3WE. We further analyzed the gene expression profiles for each GPD1 variant under SWM, demonstrating that the expression of GPD1WE occurred earlier and was greater compared to the other alleles. This result indicates that the level, timing, and condition of expression differ between regulatory regions in the various genetic backgrounds. Furthermore, promoter allele swapping demonstrated that these allele expression patterns were transposable across genetic backgrounds; however, glycerol yields did not differ between wild type and modified strains, suggesting a strong trans effect on GPD1 gene expression. In this line, Gpd1 protein levels in parental strains, particularly Gpd1pWE, did not necessarily correlate with gene expression differences, but rather with glycerol yield where low Gpd1pWE levels were detected. This suggests that GPD1WE is influenced by recessive negative post-transcriptional regulation which is absent in the other genetic backgrounds. This dissection of regulatory mechanisms in GPD1 allelic variants demonstrates the potential to exploit natural alleles to improve glycerol production in wine fermentation and highlights the difficulties of trait improvement due to alternative trans-regulation and gene-gene interactions in the different genetic background.
Introduction
Glycerol production is one of the most important by-products generated during alcohol fermentation. Depending on the quantity and wine type, glycerol can contribute to wine flavor intensity and impact aroma volatility (Gawel et al., 2007; Marchal et al., 2011; Zhao et al., 2015). In budding yeast, glycerol is synthesized by the reduction of dihydroxyacetone phosphate followed by dephosphorylation catalyzed by glycerol-3-phosphate dehydrogenase (GPD1) and glycerol-3-phosphatase (GPP1) (Albertyn et al., 1994). Genetic modification has been used to engineer yeast that produces more glycerol (Steensels et al., 2014). Despite this, the application of genetically modified organisms (GMOs) in the industry is restricted by the lack of policies that regulate their use and by negative public perception (Steensels et al., 2014). This has inspired the development of alternative strategies for the generation of new strains, such as experimental evolution (Steensels et al., 2014; Tilloy et al., 2014). For example, the wine strain EC1118 has been genetically improved to produce more glycerol through constant exposure to osmotic stress (Tilloy et al., 2014). Nevertheless, the use of experimental evolution to obtain specific phenotypes is time-consuming, and undesirable mutations can complicate industrial applications. Thus, non-invasive nor mutagenic strategies represent an alternative where variants of interest are selected from standing natural genetic variation (Cubillos, 2016). S. cerevisiae strains are genotypically and phenotypically highly variable, and thus are an ideal model for studying trait improvement (Thompson and Cubillos, 2017; Peter et al., 2018).
Natural and commercial S. cerevisiae isolates differ largely in a series of traits (Crepin et al., 2012; Salinas et al., 2016; Cubillos et al., 2017). In this context, it has been reported that depending on the genetic background, isolates can yield different concentrations of acetic acid, glycerol, ethanol, and other secondary metabolites (Salinas et al., 2012). Efforts aimed at deciphering the genetic basis underlying some of these phenotypic differences in isolate types have demonstrated the existence of a wide set of quantitative trait loci (QTLs), for example: ethanol production (Katou et al., 2009; Pais et al., 2013), ethanol tolerance (Swinnen et al., 2012), glycerol production (Hubmann et al., 2013b), asparagine assimilation (Marullo et al., 2007), low temperature fermentation (Garcia-Rios et al., 2017), and nitrogen assimilation (Brice et al., 2014, 2018; Cubillos et al., 2017). In most of these cases, QTLs are down to non-synonymous changes which significantly impact protein structure and gene function. For example, a series of aminoacidic changes in SSK1, GPD1, HOT1, and SMP1 genes have been found as responsible for low glycerol and high ethanol yield differences between CBS6412 and Ethanol Red strains (Hubmann et al., 2013a,b). Yet, the molecular mechanisms and the effect of these polymorphisms upon protein activity and stability are unknown.
Although, these regions explain a substantial fraction of the natural phenotypic variation between individuals, a wide set of variants across eukaryotes are located within non-coding regions and finely modulate gene expression and ultimately phenotypes (Wray, 2007). In this context, non-coding regions have been less explored in yeast and could be useful for genetic breeding and industrial applications via the modulation of gene regulation and expression (Thompson and Cubillos, 2017). Previous expression profiles of S. cerevisiae isolates obtained from different ecological niches have demonstrated that the genetic control of expression is well-defined (Fay et al., 2004; Kvitek et al., 2008; Ehrenreich et al., 2009; Zhu et al., 2009; Fraser et al., 2010; Cubillos et al., 2012). Additionally, budding yeast can be easily manipulated at the molecular level and represents a great model for genetic improvement and for understanding the consequences of mutations within coding and regulatory regions (Salinas et al., 2016). For example, early QTL mapping on sporulation efficiency between two North-American isolates has validated the role of non-coding regions on natural variation in yeast by showing the effects of a single nucleotide deletion upstream of RME1 (Gerke et al., 2009). In this context, we have previously demonstrated how widespread Allele Specific Expression (ASE) is across four S. cerevisiae isolates representative of different lineages of the species. (Salinas et al., 2016). Interestingly, estimates of the aspartic acid and glutamic acid consumption in the wine fermentation must of two yeast strains from different geographic origins have demonstrated that polymorphisms in both portions (coding and regulatory) of the ASN1 gene, are partly responsible for nitrogen assimilation differences between genetic backgrounds (Salinas et al., 2016). Moreover, this study provided a catalog of cis-variants between strains that directly influence allelic expression and which can be used as tools for the dissection of other phenotypes.
In this study, we utilize the existing standing genetic variation in yeast within non-coding regions to identify natural allelic variants for genes part of the alcoholic fermentation pathways that could impact glycerol production under synthetic wine must (SWM) conditions. For this, we searched our ASE database for genes involved in fermentation, such as alcohol dehydrogenases and in glycerol biosynthesis. From this, we studied two candidate genes, ADH3 and GPD1, with differently expressed alleles between strains. Through reciprocal hemizygosity, allele swapping, along with transcriptional and co-translational profiling across strains, we demonstrate that ADH3 and GPD1 allelic variants modulate glycerol yield and could be used as natural sources for genetic improvement and gene expression fine tuning.
Materials and Methods
Yeast Strains and Culture Media
The haploid strains Y12 (referred to as Sake, ‘SA’, Mat alpha ho::HygMX, ura3::KanMX), YPS128 (referred to as North American, ‘NA’, Mat alpha ho::HygMX, ura3::KanMX), DBVPG6044 (referred to as West African, ‘WA’, Mat alpha ho::HygMX, ura3::KanMX) and DBVPG6765 (referred to as Wine/European, ‘WE’, Mat a, ho::HygMX, ura3::KanMX) together with F1 hybrids (WE x SA, WE x NA, and WE x WA crosses) utilized in this study have been previously described (Cubillos et al., 2009, 2011). Before every experiment, strains were recovered from frozen glycerol stocks in rich yeast peptone dextrose (YPD) agar media and grown overnight at 28°C.
Fermentation in Synthetic Wine Must (SWM) MS300 and HPLC Analysis
Fermentations were carried out in at least three biological replicates depending on the experiment. Fermentations were conducted using SWM supplemented with 300 mgN/L) (MS300, hereafter referred to as SWM) and 270 g/L of total sugar (glucose and fructose and prepared as previously reported (Rossignol et al., 2003) (Jara et al., 2014). For each experiment, the strains were initially grown with constant agitation in 10 mL of SWM for 16 h at 25°C. Following this, 12 mL of fresh SWM were inoculated to a final concentration of 1x106 cells/mL of yeast (in 15 mL conical tubes) and incubated at 25°C with no agitation. Fermentations were weighed every day to calculate the CO2 output. The fermentations were maintained until the daily CO2 lost represented less than 10% of the accumulated CO2 lost. At the end of the fermentation, the fermented SWMs were centrifuged at 9000 ×g for 10 min and the supernatant was collected. From this, the concentration of extracellular metabolites was determined using HPLC. Specifically, 20 μL of filtered must were injected in a Shimadzu Prominence HPLC (Shimadzu, United States) with a Bio-Rad HPX –87H column (Nissen et al., 1997). In this way, the concentrations of glucose, fructose, trehalose, acetic acid, succinic acid, malic acid, ethanol, and glycerol was estimated (results found in Supplementary Tables S2, S4). Ethanol yield was estimated converting %v/v to g/L utilizing the ethanol density and then dividing by total sugar consumption. Similarly, glycerol yield was estimated by dividing the observed glycerol levels (g/L) by the total amount of sugar consumed.
Reciprocal Hemizygosity Assay
Reciprocal hemizygotes of the ADH3 and GPD1 candidate genes were generated as previously described (Cubillos et al., 2013; Jara et al., 2014; Salinas et al., 2016). Briefly, the URA3 gene previously deleted in the haploid parental strains (Cubillos et al., 2009) was used as a selectable marker for the deletion of each target gene. The haploid versions of the parental strains also contained opposite antibiotic markers in the HO locus (Hygromycin B for “Mat a” strains and Nourseothricin for “alpha” strains), which allowed us to cross the haploid mutant parental strains and construct all possible combinations of single deletions. Thus, mutated parental strains were crossed to generate the reciprocal hemizygote strains, selecting the diploid hybrids in antibiotic plates (300 ug/mL of Hygromycin B and 100 ug/mL of Nourseothricin). Finally, diploids were confirmed by MAT locus PCR (Huxley et al., 1990). Primers are listed in Supplementary Table S1.
Luciferase Expression Assay (Cloning and Phenotyping)
The GPD1 genetic constructs carrying the destabilized version of the firefly luciferase reporter gene under the control of the different regulatory allelic variants were assembled using yeast recombinational cloning as previously described (Salinas et al., 2016). Briefly, 700 bp upstream of the ATG start codon (regulatory region) and the firefly luciferase gene (Rienzo et al., 2012) were amplified by PCR using Phusion Flash High-Fidelity PCR Master Mix (Thermo scientific, United States). In addition, the Hygromycin HphMx antibiotic resistance gene was amplified by PCR and was included in the genetic constructs. Overall, the overlap between PCR products was 50 bp and were co-transformed with the linear plasmid pRS426 in the yeast lab strain BY4741 (MATa, his3Δ1, leu2Δ0, LYS2, met15Δ0, ura3Δ0). The circular plasmids generated in yeast were transferred to an E. coli DH5α strain and confirmed by colony PCR using standard conditions. At least three positives colonies containing the regulatory region, the luciferase gene, and the HphMx cassette were selected for plasmid isolation and sequencing. The sequence identity of the regulatory regions was confirmed using the SGRP2 BLAST database service (Bergstrom et al., 2014). Finally, the parental strains were transformed with the complete genetic constructs, which were amplified by PCR using a Phusion Flash High-Fidelity PCR Master Mix (Thermo scientific, United States). For the latter, 70 bp primers were utilized, which guided direct homologous recombination at the target locus, allowing for the integration of the genetic constructions in the genome. The positive yeast colonies were analyzed by colony PCR with standard conditions.
The use of a destabilized firefly luciferase (Rienzo et al., 2012) allowed quantifying expression of the targeted genes in real-time and, to avoid the effects of copy number and genetic context on gene expression, the genetic constructs were integrated into the original GPD1 locus, maintaining the genetic context. Additionally, reciprocal hemizygotes were generated with these constructions as previously described. Strains carrying the firefly luciferase constructs were analyzed for luciferase expression using a Cytation3 microplate reader (Biotek, United States). Briefly, the strains were pre-grown in YNB (Yeast nitrogen base, supplemented with 2% glucose) and SWM overnight. The cultures were then diluted 1/100 to inoculate a 96 well plate with 200 uL of fresh culture media containing 0.1 mM of luciferin. The in vivo OD600nm and the luminescence intensity of the cell cultures were monitored every 10 min. All the experiments were performed using, at least, three biological replicates.
Allele Swapping
Promoter allele swaps were carried out as previously described (Salinas et al., 2016). Briefly, genetic constructs carrying the regulatory regions of GPD1 and ADH3 (700 bp upstream of the ATG start codon) plus the HphMx cassette in the reverse direction (HphMxRv-PGPD1) were assembled using yeast recombinational cloning. See details above for full descriptions. Initially, we used the WE strain as a receiver of the promoters coming from the NA, SA, and WA strains. For this, the regulatory region of the target gene was deleted in the WE strain using URA3 gene as a selectable marker. Then, the construct containing the promoter of interest was amplified by PCR and used for transformation and direct recombination with the regulatory region. The final strains were confirmed by standard colony PCR and sequencing. Furthermore, we used the NA, SA and WA strains as receivers of the promoter coming from the WE strain following the same procedure.
Additionally, we used the strains carrying the promoter swaps as recipients of the luciferase reporter gene for direct quantification of gene expression in living cells. Again, see above for the full description of the methods. The construct containing the destabilized version of the luciferase gene plus the URA3 selectable marker (Luc-URA3) was amplified by PCR and used for GPD1 transformation of the strains carrying the promoter swaps. The final strains were confirmed by colony PCR using standard conditions.
Gpd1p Tagging With mCherry
The fusion of the Gpd1 protein with mCherry was carried out using one step PCR and recombination with the 3′end of the GPD1 ORF, which corresponds to the C-terminal of the Gpd1 protein. This allowed us to remove the stop codon of the GPD1 gene and fuse its ORF with the mCherry coding sequence (DeLuna et al., 2010). In this way, we generated a construct containing the mCherry sequence plus the hygromycin cassette (mCherry-HphMx). This allowed us to directly tag the GPD1 ORF and perform selection by hygromycin in each yeast strain. The mCherry-HphMx construct was assembled in a pRS426 plasmid using the above described yeast recombinational cloning method (Oldenburg et al., 1997). The yeast strains carrying the mCherry-HphMx cassette were confirmed using standard yeast colony PCR.
The yeast strains were analyzed in microcultivation with a Cytation 3 microplate reader, which allowed for the dual measurement of OD600 and fluorescence of the cell cultures over time. Briefly, the yeast strains were grown overnight in a 96 well plate with 200 uL of YNB or SWM medium. 10 uL of these cultures were used to inoculate a new black 96 well plate containing 300 uL (30-fold dilution) of fresh media. The OD600 and the fluorescence were measured every 30 min using 587 nm of excitation and targeting emission wavelengths of 620 nm with a gain of 100 units.
GPD1 Sequence Analysis
GPD1 sequences were obtained from the SGRP2 database (Bergstrom et al., 2014) and regulatory regions together with ORF sequences were compared using Geneious 8.1.5. Transcription factor binding sites were predicted utilizing YeTFaSCo: Yeast Transcription Factor Specificity Compendium (de Boer and Hughes, 2012). The Codon Adaptation Index (CAI) for each allele variant was estimated using the CAIcal server (Puigbo et al., 2008) with default settings and utilizing the standard Saccharomyces cerevisiae genome codons usage from the Codon Usage Database (Nakamura et al., 2000).
Data Analysis
The significance of all comparisons was made through non-parametric test depending on whether two groups or multiples groups were compared. Fermentation metabolites results obtained from HPLC were compared across strains utilizing a non-parametric Kruskal-Wallis test and Dunn’s Multiple Test Comparison. Similarly, significance in metabolites levels between reciprocal hemizygotes were assessed utilizing a non-parametric Mann Whitney test. Gene expression and protein levels across the four parental strains were evaluated using a Friedman test and Dunn’s Multiple Test Comparison. Luciferase expression and glycerol yield was estimated utilizing a Spearman rank correlation test. Finally, gene expression and protein levels across hemizygotes were compared using a Wilcoxon signed rank test. All analyses were performed utilizing GraphPad Prism Software 5.2. In all cases p-values < 0.05 were considered as significant.
Results
Glycerol Production Differs Among S. cerevisiae Strains
Through the utilization of the ASE database, we aimed to identify natural allelic variants impacting glycerol production yields when grown in SWM. Thus, we initially characterized the fermentation profiles and ability to produce a series of metabolites in four strains grown in SWM. We estimated the concentrations of glucose, fructose, trehalose, acetic acid, succinic acid, malic acid, ethanol and glycerol after 21 days of micro-fermentations experiments.
Ethanol and glycerol significantly differed among some isolates (Supplementary Table S2 and Figure 1A, p-value < 0.05, and Kruskal-Wallis test). For example, the SA strain showed greater ethanol production than NA (Figure 1A). Conversely, the WA and the NA strain showed significant differences for glycerol production, the latter producing lower levels of glycerol (Figure 1A, p-value < 0.05, and Kruskal-Wallis test). Since we found relatively high amounts of residual sugars in our fermentations, we estimated yields to accurately measure the quantity of sugar transformed into ethanol and glycerol, respectively (Figures 1B,C). From this we observed that the WA strain (a non-domesticated strain) yielded significantly more glycerol than the SA strain (p-value < 0.05, Kruskal-Wallis test), in agreement with their glycerol and ethanol production levels, respectively. Thus, the WA strain produced the highest glycerol yields (Figure 1C), while the WE isolate, considered a domesticated strain, did not produce greater ethanol nor glycerol levels/yields compared to WA, demonstrating that other non-domesticated genetic backgrounds may represent potential sources of allelic variants that can be used to boost glycerol production in wine fermentation.
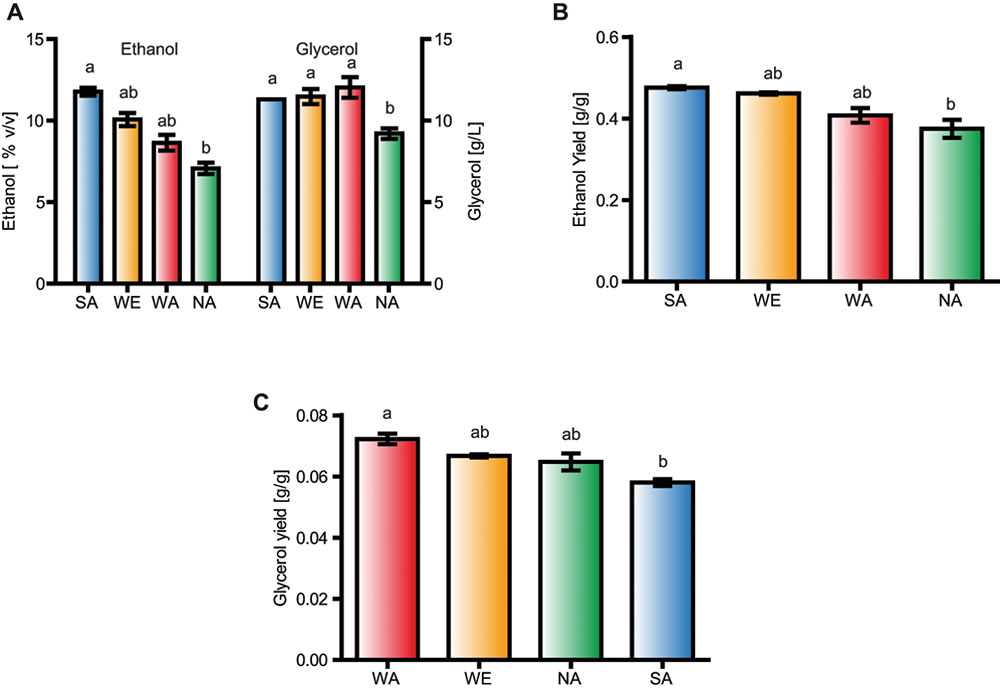
FIGURE 1. Glycerol production in S. cerevisiae strains. (A) Glycerol and ethanol levels; (B) Ethanol yield and (C) glycerol yield in Sake (SA), West African (WA), Wine/European (WE), and NA (North American strains) after fermentation in synthetic wine must. Different letters indicate significant differences between genotypes.
Reciprocal Hemizigosity Assay (RHA) Validates Glycerol Yield Differences Among ADH3 and GPD1 Allelic Variants
In order to identify allelic variants that could influence glycerol yield, we utilized the ASE database (Salinas et al., 2016) to find ethanol/glycerol biosynthesis alleles with divergent expression. Additionally, since our focus was the wine fermentation environment, we only selected genes in which the WE allele was differently expressed (Supplementary Table S3). Here, we found two genes, ADH3 and GPD1, which encode for an alcohol dehydrogenase III and a glycerol-3-phosphate dehydrogenase, respectively (Young and Pilgrim, 1985; Albertyn et al., 1994; de Smidt et al., 2008). Subsequently, to estimate the relative contribution of each genetic variant to ethanol and glycerol yields, we performed a functional analysis to compare the reciprocal hemizygotes derived from the three WE F1 hybrids. For both genes, we observed that hemizygotes carrying a WE variant produced substantially lower glycerol levels (except for ADH3 - NA × WEΔ, p-value < 0.05, Mann Whitney test) than hemizygotes carrying the WA and SA allelic variants (Figure 2A). For example, ADH3WA (ADH3 hemizygote carrying the WA variant) and ADH3WE hemizygotes produced 15.1 ± 0.4 g/L and 13.5 ± 0.6 g/L of glycerol, respectively. Similarly, this pattern was also observed for glycerol yields, where differences were maximized when sugar consumption was considered (Figure 2B). For example, the GPD1WA hemizygote yielded 66% more glycerol than the GPD1WE hemizygote. These results agree with the glycerol levels produced by the WA and WE parental strains, but not with SA strain, suggesting an antagonistic effect of the SA alleles. Interestingly, none of the hemizygotes with significantly greater glycerol yields had lower ethanol yields. Instead, WE hemizygotes produced increased yields of other metabolites including succinic acid and malic acid depending on the hybrid (Supplementary Table S4). This suggests quantitative differences in carbon molecules fluxes among strains. Overall, these results indicate that ADH3 and GPD1 allelic variants from the WA and SA strains could be used to maximize glycerol yields in wine fermentation; however, we found no evidence that selection of these alleles would affect ethanol production.
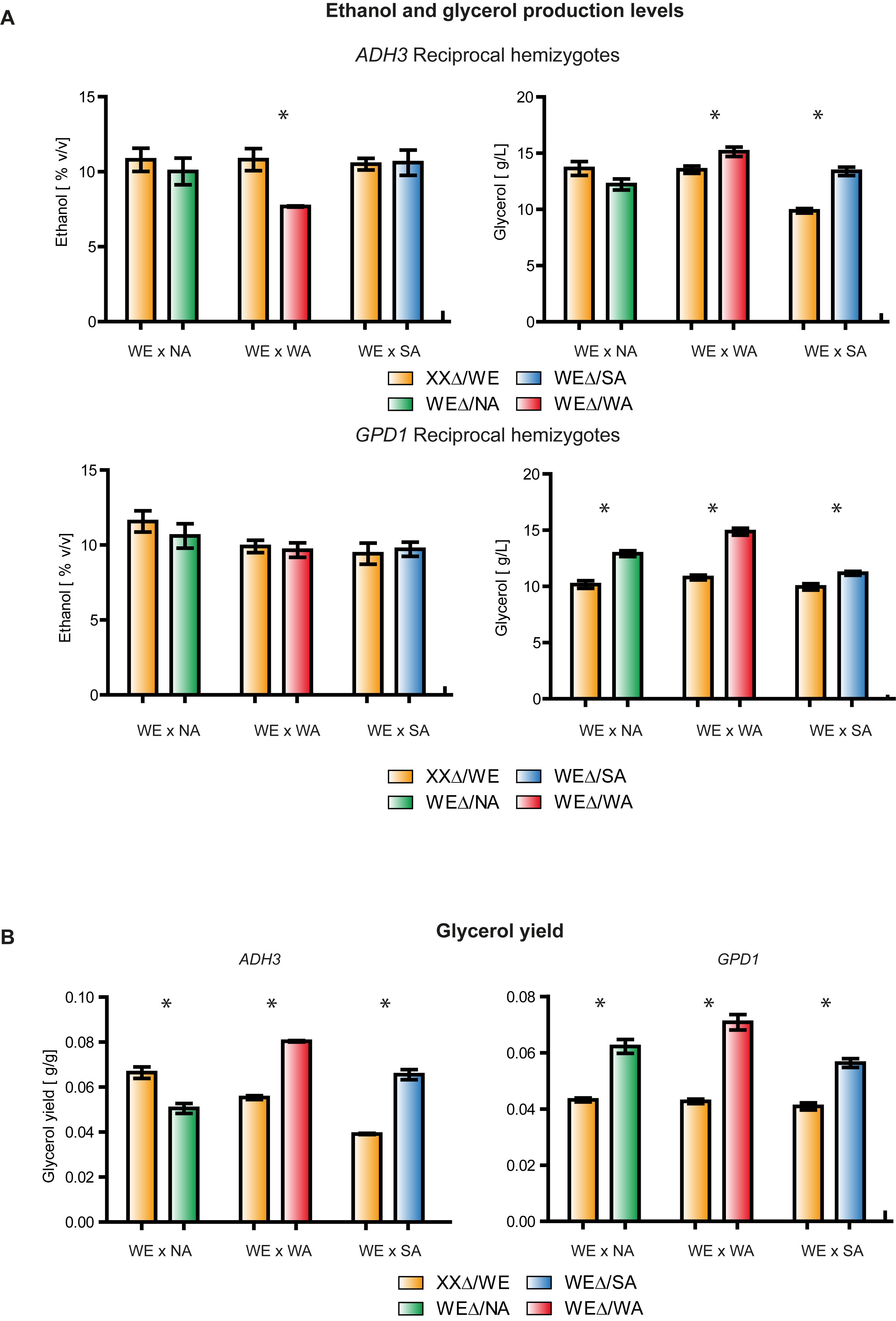
FIGURE 2. Reciprocal hemizygosity assay for GPD1 and ADH3. (A) Ethanol (%v/v) and Glycerol (g/L) levels in WE × NA, WE × SA, and WE × WA reciprocal hemizygotes. The Glycerol yield for the same strains is shown in (B). XX denotes either the NA, WA, or SA genotype depending on the bar. ∗p-value < 0.05.
Transcriptional Profiling Demonstrates That GPD1 Expression Levels Are G x E Dependent
We obtained expression profiles for each allelic variant to determine how allelic differences in regulatory regions affected expression levels, timing, and were condition dependent. For this, we focused on GPD1 since this gene was involved in the greatest glycerol yield differences among hemizygotes. We generated transcriptional fusions in all strains by inserting a destabilized luciferase reporter gene immediately downstream of the regulatory region and replacing the original GPD1 locus (Salinas et al., 2016). Firstly, the luciferase expression levels were obtained for all parental strains under micro-cultivation conditions in YNB and in SWM to evaluate the strength of the promoters in these two scenarios. From the transcriptional expression profiling, assay we found differences in expression between strains and environments, clearly indicating a G × E interaction (Figures 3A,B and Supplementary Figure S1). For example, the luminescence of PGPD1WE-Luc (the WE GPD1 promoter controlling luciferase gene expression) was lower when the strain was cultivated in laboratory media than when cultivated in SWM (p-value < 0.05, Friedman test). Overall, the expression of PGPD1WE-Luc under SWM was highest among all strains (Figure 3A, p-value < 0.05, Friedman test). Interestingly, when cultivated in SWM each strain had a unique GPD1 expression profile with expression levels clearly increasing among strains, WE > SA = WA > NA (p-value < 0.05, Friedman test). Conversely, the results differed when the strains were cultivated in YNB media. Here, PGPD1SA-Luc exhibited the strongest luminescence (Figure 3B, p-value < 0.05, Friedman test). It is worth noting that the luciferase expression levels were ∼10 times higher when strains were cultivated in SWM than when cultivated in YNB. These results demonstrate that the expression of GPD1 is highly induced under fermentative conditions and its strength is dependent on the promoter allelic variant, yet the role of cis- and/or trans regulation is uncertain. Interestingly, the parental strain expression profiles did not correlate with the glycerol yields previously estimated (Figure 1 and Supplementary Table S2, p-value = 0.2 Spearman rank correlation).
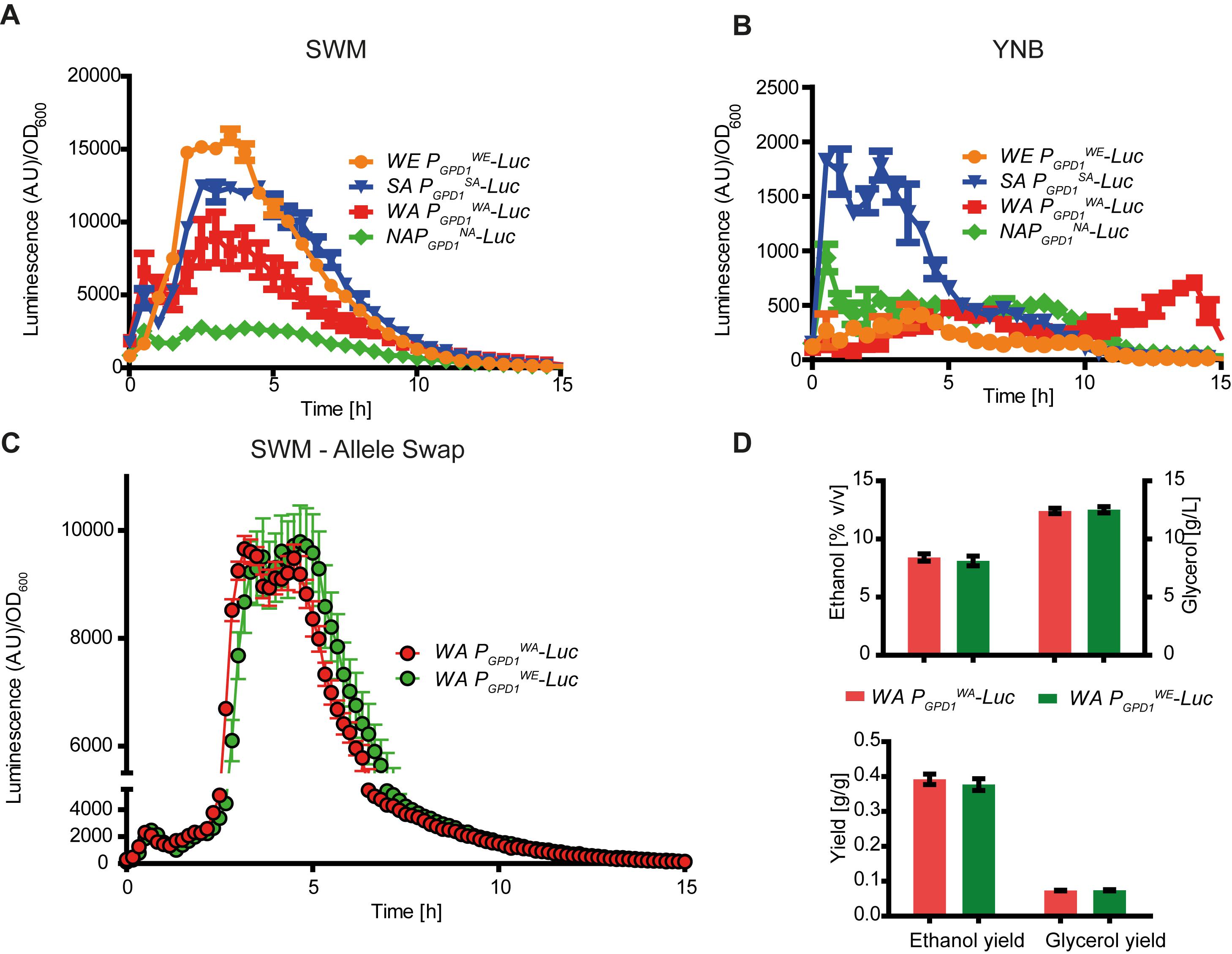
FIGURE 3. GPD1 gene expression profile. Luciferase reporter gene expression assay for GPD1 promoters in their native genetic backgrounds grown in (A) synthetic wine must and (B) yeast nitrogen base (YNB). (C) Allele swap in the WA strain with a luciferase reporter gene controlled by the wild type promoter (red) and the WE promoter (green). (D) Metabolite levels in the allele swap assay.
In order to evaluate the role of the cis-regulatory region and whether the PGPD1WE could sufficiently increase expression levels and thus modify glycerol yield in other genetic backgrounds, we performed an allele swap. Immediately upstream of the luciferase reporter, we replaced the native GPD1WA regulatory region with PGPD1WE in the WA strain (700 bp upstream of the ORF). Microcultivation in SWM revealed that the WA strain carrying the PGPD1WE variant had greater expression than WA strains with the native promoter (p-value < 0.001, Wilcoxon signed rank test). Expression levels reached a maximum discrepancy of 18% around 5 h of cultivation (Figure 3C) and significant differences were found throughout the cultivation period (p-value < 0.001, Wilcoxon signed rank test). This demonstrates the role of cis- regions and the potential to increase GPD1 expression in foreign genetic backgrounds. We next evaluated the impact of a promoter swap on glycerol yield. PGPD1WE was introduced in the WA strain controlling the expression of GPD1WA ORF. Fermentation was carried out for 21 days in SWM, and glycerol together with ethanol yields were estimated. No significant differences were found as raw metabolite levels and yields were similar between strains (Figure 3D), suggesting that the promoter itself is not sufficient to increase glycerol yield or that we did not have the experimental power to detect minor glycerol yield differences due to experimental noise.
Subsequently, to evaluate the influence of all promoters on gene expression and to avoid polymorphic trans-effects that could modulate mRNA levels, we proceeded to examine expression levels in F1 reciprocal hemizygotes. Interestingly, we observed greater expression in the strains with the WE promoter controlling the reporter gene (p-value < 0.001, Wilcoxon signed rank test, Figure 4 and Supplementary Figure S2). Specifically, expression was higher in the PGPD1WE-Luc x WA-GPD1 (WE x WA F1 hybrid with the WE GPD1 promoter controlling luciferase expression) and PGPD1WE-Luc x SA-GPD1 hemizygotes (WE x SA F1 hybrid with the WE GPD1 promoter controlling luciferase expression gene) compared to the PGPD1WA-Luc x WE-GPD1 and PGPD1SA-Luc x WE-GPD1 hemizygotes (Figure 4). No significant differences in expression were found for the WE x NA hemizygotes.
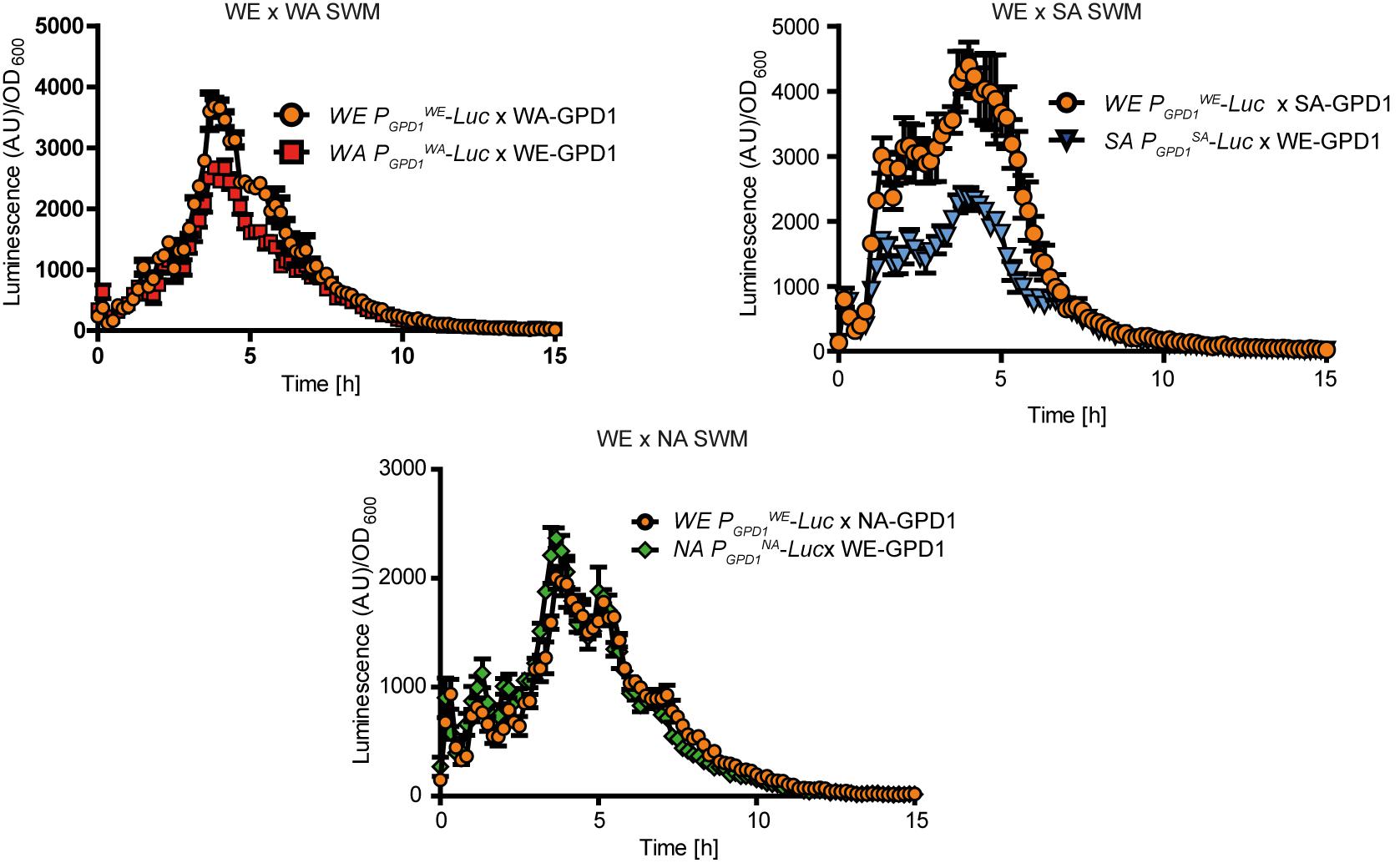
FIGURE 4. Luciferase reporter assay in GPD1 reciprocal hemizygotes. Luminescence levels in GPD1 WE × WA, WE × SA, and WE × NA hemizygotes in micro cultivation in SWM.
To identify putative transcription factor binding sites that could modulate GPD1 gene expression, we analyzed the cis-regulatory region (up to 200 bp upstream of the ATG start site). This was done via sequence alignment and by predicting binding sites utilizing the YeTFaSCo database. (de Boer and Hughes, 2012). Three SNPs are found between the four strains, and two of these were exclusively present in the WE strain. The closest polymorphism to the ATG start site corresponded to a deletion of two nucleotides in the West African and North American strains (del-32CC). Nevertheless, this deletion does not yield polymorphic binding sites for this region, and the same transcription factors would bind in all strains. The other two polymorphisms upstream of del-32CC encode a thymine instead of a cytosine (T-180C) around nucleotide -180 (from the ATG site) and a cytosine instead of a guanine (C-202G) around -202 in the WE background. The last polymorphism could potentially influence allele specific binding and could alter Crz1 binding, which is a transcription factor associated with the response to ethanol stress (Araki et al., 2009).
Overall, these results suggest the presence of powerful cis-factors that increase GPD1WE levels independently of the genetic background. This being said, the lower glycerol yields in WE could be explained by other regulatory mechanisms (negative post-transcriptional regulation, post-translational modifications) or reduced Gpd1WEp activity.
Gpd1-mCherry Fusions Suggest Negative Post-transcriptional Regulation on Gpd1pWE
Since a negative correlation was found between GPD1 expression levels and glycerol yield in the WE strain, we sought to quantify Gpd1 protein levels as a means to detect putative post-transcriptional regulation. For this, we generated a Gpd1p-mCherry fusion in all parental strains by genetically linking mCherry to the Gpd1p C-terminal. Protein levels were estimated in micro-cultivation in SWM. We found significant differences between all strains (p-value < 0.05, Friedman test). During the first hours of incubation, the mCherry fluorescence was strong in the SA and WA strains, whereas the signals in the WE and NA strains were low (Figure 5A and Supplementary Figure S3). Interestingly, the mCherry fluorescence of the SA and WA strains was two times higher than the WE strain. This indicates that the WE strain produces significantly less Gpd1p, which contrasts with the increased expression of GPD1 in this strain. Overall, this suggests that post-transcriptional modifications affect protein levels and influence glycerol yields in all strains.
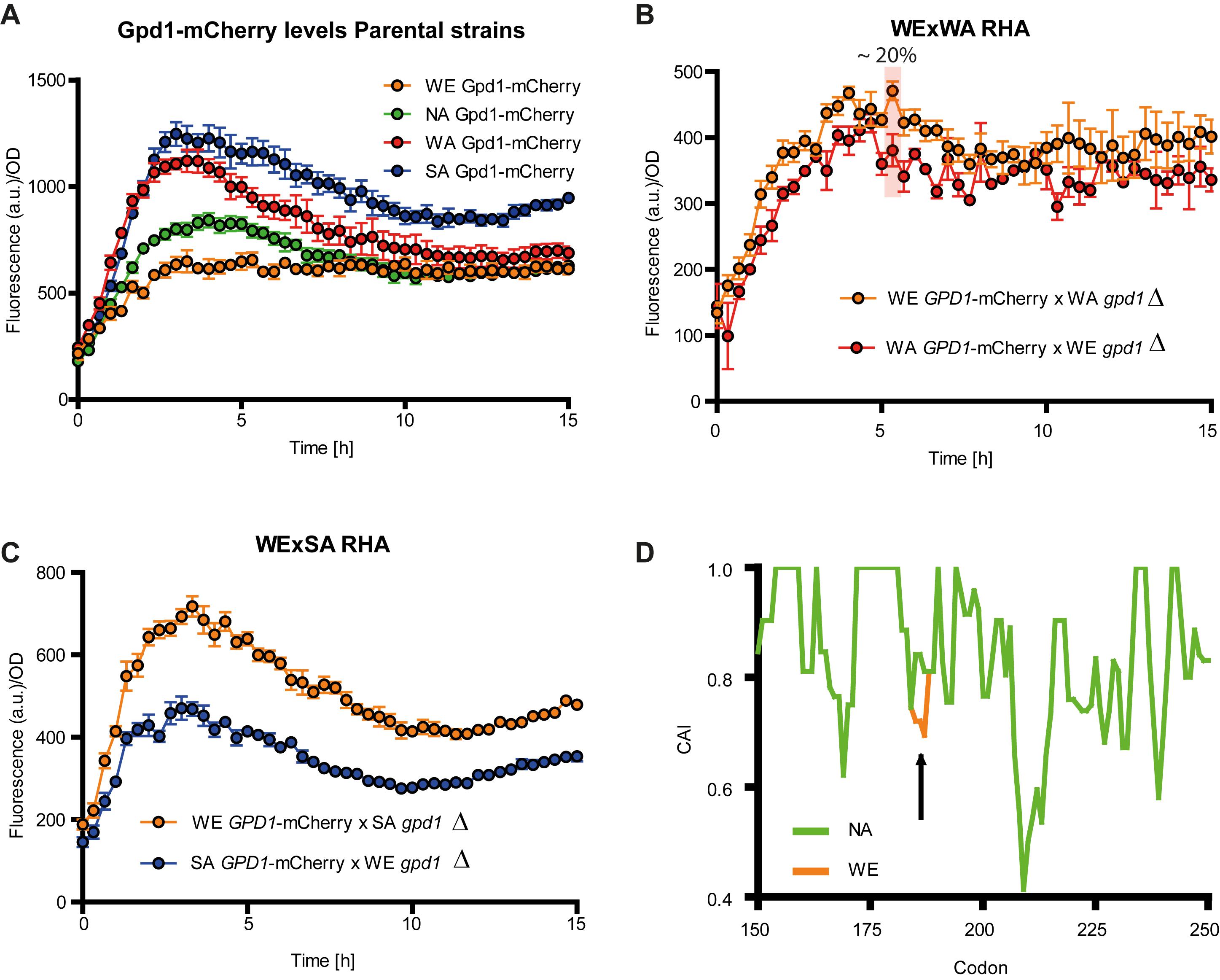
FIGURE 5. Gpd1p levels in S. cerevisiae strains. Gpd1-mCherry levels in (A) parental strains, (B) WE × WA reciprocal hemizygotes, and (C) WE × SA reciprocal hemizygotes in micro cultivation in SWM. (D) Codon Adaptation Index (CAI) in NA, WA or SA strains (green) respect to the WE strain for GPD1 between codons 150 and 250. The arrow indicates the codon for which a CAI difference was found between GPD1NA, GPDWA and GPD1SA versus GPD1WE.
Subsequently, in order to evaluate the role of a dominant or recessive trans-factor we quantified protein fluorescence in the WE × WA and WE × SA hemizygotes (Supplementary Figure S3). We detected differences in the fluorescence among genotypes; the fluorescence of hemizygotes carrying Gpd1pWA was up to 20% (at 5 h’ time point) lower than that of hemizygotes with Gpd1pWE. Overall, the fluorescence of Gpd1pWE hemizygote was high throughout the incubation period (Figure 5B, p-value < 0.001, Wilcoxon signed rank test). Likewise, after only 3 h of incubation the fluorescence of the WE × SA reciprocal hemizygote carrying Gpd1pWE was 35% greater than that of the hemizygote carrying Gpd1pWE, and this trend was significant throughout the entire incubation period (Figure 5C, p-value < 0.001, Wilcoxon signed rank test). These results demonstrate the stronger expression induction profile of pGPD1WE and suggests that recessive trans-effects, such as post-transcriptional modifications, could negatively impact Gpd1pWE expression levels, which would explain the glycerol yield differences among the GPD1 allelic variants. We compared the coding GPD1 sequence between strains and identified six synonymous polymorphisms. Thus, we rule out the possibility of a polymorphism that could be targeted by alternative post-translational modifications (i.e., phosphorylation or acetylation). This being said, one of the synonymous polymorphisms in the GPD1WE allele reduces the CAI respect to the other variants (GPD1WA, GPD1NA and GPD1SA); specifically, the WE allele carries the ACC codon, whereas the WA, SA and NA alleles carry an ACT codon, yet both code for threonine (T186) (Figure 5D).
Discussion
Here, we explored the natural genetic variation of S. cerevisiae to determine how ASE can modulate glycerol production. Differences in metabolites production, such as glycerol and ethanol, were found among strains representative of the main genetic clusters recognized for this species. Interestingly, a non-wine strain had greater glycerol yields (Figure 1B) yet similar ethanol yields when grown in SWM. This result agrees with previous results by our group for fermentation in high nitrogen concentrations (Salinas et al., 2012). Despite this, here the wine strain yielded more glycerol than that found in the previous study, suggesting that glycerol production is influenced by nitrogen concentration. Indeed, it has been shown that the ratio of carbon to nitrogen in wine musts can significantly alter fermentation performance (Varela et al., 2004), and it is well known that strains differ in their ability to assimilate nitrogen (Crepin et al., 2012; Cubillos et al., 2017; Brice et al., 2018). During fermentation, strains can retain glycerol when under osmotic stress by decreasing the glycerol dissimilation and therefore total extracellular glycerol yields, thus impacting the final product of the fermentation (Nevoigt and Stahl, 1997; Hohmann, 2002). Yet, our results were obtained under static and small volume fermentations, and therefore we believe some differences can be expected when scaling up to larger industrial volumes.
Phenotypic differences between yeast strains can originate from polymorphic coding or non-coding regions (Thompson and Cubillos, 2017). In the analyzed strains, we found only synonymous polymorphisms in the coding portion of GPD1. Therefore, it is likely that polymorphisms in the regulatory region are the cause of the observed genotype – phenotype variation, however, we cannot rule out that translation speed could impact protein levels. Indeed, previous reports have demonstrated that expression variants can directly impact phenotypic differences between yeast isolates (Gerke et al., 2009; Salinas et al., 2016; Cubillos et al., 2017). In an earlier study, we have demonstrated that the four strains chosen for this study have different ASE levels (Salinas et al., 2016; Cubillos et al., 2017), and this can directly impact oenological phenotypes such as nitrogen assimilation or fermentation capacity (Salinas et al., 2016). The results presented herein of glycerol yields are consistent with this. The non-wine alleles of ADH3 and GPD1 in reciprocal hemizygotes (both exhibit ASE in at least a single cross involving the wine strain, Supplementary Table S3) produced higher glycerol levels, consumed lower amounts of sugar, and exhibited greater glycerol yields. In the case of the ADH3SA and GPD1SA alleles, we observed an antagonistic effect relative to the glycerol yields reported in parental strains (Figures 1, 2). Antagonistic alleles and QTLs, refer as those alleles with a different effect from their parental origin, have been extensively described in yeast for different phenotypes and crosses (Liti and Louis, 2012) and together with other unlinked variants can expand the phenotypic landscape (Cubillos et al., 2011). These results together suggest that strains differ in their metabolic fluxes, and cis- and trans-regulation significantly impacts glycerol yields. Moreover, our results demonstrate that non-wine alleles can be potential targets of genetic improvement aimed at increasing glycerol yields. Indeed, several studies have targeted GPD1 over-expression in wine strains to favor glycerol production. For example, introduction of a high copy number vector containing the coding portion of GPD1 controlled by the ADH1 promoter into the commercial wine strains K1M, VL1 and BC increases glycerol production by three-fold, while ethanol production is reduced (Cambon et al., 2006). The effective modulation of glycerol and ethanol production was affected by an increase in the production of undesirable secondary metabolites exceeding thresholds allowed for wine. Specifically, the production of acetate, acetaldehyde, and acetoin due to the redox imbalance generated by the overproduction of glycerol confers unacceptable aromas and flavors to wine. Alternatively, the overexpression of GPD1 complemented by the overexpression of BDH1 increases acetoin reduction to produce 2,3-butanediol, a compound that has neutral sensory properties (Ehsani et al., 2009). Yet, similar approaches should be targeted for natural variants. Here, we have identified differences in glycerol yields between GPD1 variants and evaluated their effect in different genetic backgrounds.
Previous QTL mapping efforts have identified GPD1 variants affecting glycerol and ethanol production, however the effect of these polymorphisms is unclear (Hubmann et al., 2013b). More difficult than generating genetically modified strains, however, is identifying and quantifying the polymorphisms within GPD1 that underlie phenotypic differences. In this context, regulatory regions are known to finely influence phenotypes (Wray, 2007; Gerke et al., 2009; Salinas et al., 2016), and here we suggest that differences upstream the ATG start site are partly responsible for expression differences between strains. From the luciferase reporter assay we show increased expression in strains containing the wine allele. This result is in contrast with the lower glycerol yields found for reciprocal hemizygotes containing the wine alleles (Figure 2). Interestingly, greater expression of GPD1WE was found in parental strains and reciprocal hemizygotes, suggesting a robust cis effect (Figures 3, 4). Indeed, our results agree with other reports in model organisms demonstrating that cis-variants explain a large proportion of expression differences between alleles (Brem et al., 2002; Yvert et al., 2003; Kliebenstein, 2009; McManus et al., 2010; Goncalves et al., 2012; Cubillos et al., 2014; Thompson and Cubillos, 2017), however, trans-eQTLs impact the expression of a greater number of genes (Brem et al., 2002; Yvert et al., 2003). Remarkably, we observed that GPD1 was only expressed in strains grown in fermentation conditions and not in laboratory settings. The wine strain responded positively to fermentation and activation of the GPD1 promoter was high; the mRNA levels of this strain exceeded those of other strains (Figure 3).
The comparison of allele expression allowed us to identify at least three different GPD1 regulatory variants (Figure 3). The existence of these unique variants indicates that fine-tuning gene expression utilizing natural variants is possible. While significant differences in expression among variants were evident from the gene expression profiles (Figure 3), these expression patterns did not fully reflect the relative glycerol yield differences when the alleles were introduced into other strains (Figure 3D). One possibility for this discrepancy is that our experimental approach was insufficient to identify mild phenotypic differences due to cis regulation; thus, more sensitive experiments should be conducted in the future. Also, cis-regulatory variants can be found up to10 kb from the targeted gene and therefore by only considering 700 bp upstream the ATG start site we might be missing variants with a stronger effect upon glycerol production (Zheng et al., 2010), yet variants with stronger effects upon gene expression and phenotypes are mostly found nearby regulated genes. A more likely hypothesis is the existence of a trans-factor, which would agree with the patterns observed in the allele swap experiments and the lack of a positive correlation between GPD1 expression levels, Gpd1p and glycerol yields. From the Gpd1p-mCherry fusions we found low protein levels only in the parental WE strain and not in the reciprocal hemizygotes (Figure 3). This suggests that a cis-active module strongly induces GPD1 mRNA expression in the WE strain however a recessive post-transcriptional trans-acting factor could be downregulating Gpd1p decreasing glycerol yields. One could argue that technical settings could be responsible for differences between the pGPD1-Luc expression patterns (estimated under 200 μL in microcultivation conditions) and glycerol yields (estimated in fermentations utilizing 12 mL), however, it has been previously demonstrated that biomass and cells physiological states under microcultivation conditions correlate with larger volumes cultures (Warringer and Blomberg, 2003) and many of these findings are relevant under wine fermentation conditions (Gutierrez et al., 2013; Ibstedt et al., 2015; Brice et al., 2018; Peltier et al., 2018) and many other environments (DeLuna et al., 2010 #1056). In this context, we found a positive correlation between our Gpd1p-mCherry fusions and glycerol yields, suggesting that both set-ups would be comparable. Indeed, our findings are in line with several studies that demonstrate that mRNA levels do not necessarily correlate with protein levels due to post-transcriptional regulation that directly impacts the phenotypic outcome. As such, low protein levels can result from accelerated mRNA degradation (Liu et al., 2016). Indeed, post-transcriptional regulation of glucose production has been demonstrated in yeast, where gluconeogenic mRNA targets, such as FBP1 and PCK1, are degraded (Yin et al., 2000). Apparent GPD1 epistatic interactions have also been observed in other similar studies (Hubmann et al., 2013b). Overall, the identification of the mechanisms regulating GPD1 ASE is a challenge. This being said, our luciferase kinetics approach did allow us to determine expressions pattern through time. It has recently been reported that time-resolved experiments are significantly more informative than genetic perturbations for inferring metabolic adaptation (Goncalves et al., 2017); thus, we were able to profile how GPD1 regulatory regions respond to environmental perturbations through time in different genetic backgrounds.
Based on our gene expression and protein fusions assays conducted in SWM, we show that natural GPD1 variants produce different glycerol levels and yields. Depending on the strain, this variation in glycerol production is controlled by cis and/or trans regulators, thought the trans-factors involved remain to be identified. These trans-factors likely module mRNA degradation decreasing overall Gpd1p levels. Identification of these factors requires further approaches, such as QTL mapping or Genome wide association studies involving a large number of wine strains. Indeed, our previous findings demonstrate that RIM15 is responsible for differences in glycerol production in a WE x SA recombinant population (Salinas et al., 2012; Kessi-Perez et al., 2016). Future studies of epistatic interactions could help to determine whether differences among strains are due to trans-factors. Nevertheless, the set of GPD1 regulatory variants characterized here can be used in different strains to modulate GPD1 expression (in fermentation conditions) and glycerol production. It remains to be explored if these observations can be applied in the wine industry under larger fermentations and real industrial settings.
Author Contributions
FS, VG, and FC designed the experiments. ST, MC, FS, VA, VD, CB, and VR performed the experiments. ST, FS, VG, CM, LL, and FC discussed the results and experiments. FS and FC wrote the paper. CM, LL, and FC provided reagents.
Funding
This work was supported by grants from Comisión Nacional de Investigación Científica y Tecnológica CONICYT FONDECYT (Grant 11140097) to FC, CONICYT FONDECYT (Grant 1150522) to CM, CONICYT FONDECYT (11130148) to VG, CONICYT FONDECYT (11170158) to FS. This research is funded by MIISSB Iniciativa Científica Milenio-MINECON to FC and LL. LL is an International Research Scholar of the Howard Hughes Medical Institute. Experiments in Cyation3 were funded by FONDEQUIP (EQM130158). VD was supported by CONICYT/Ph.D. scholarship (6313018).
Conflict of Interest Statement
The authors declare that the research was conducted in the absence of any commercial or financial relationships that could be construed as a potential conflict of interest. The reviewer PM and handling Editor declared their shared affiliation.
Supplementary Material
The Supplementary Material for this article can be found online at: https://www.frontiersin.org/articles/10.3389/fmicb.2018.01460/full#supplementary-material
FIGURE S1 | GPD1 gene expression profiles in (A) parental SWM, (B) parental YNB, and (C) allele swapped strains in SWM. Raw luminescence and OD values are provided.
FIGURE S2 |GPD1 gene expression profiles in reciprocal hemizygotes. Raw luminescence and OD values are provided.
FIGURE S3 | Gpd1p protein levels in parental and reciprocal hemizygote strains. Raw fluorescence and OD values are provided.
TABLE S1 | Primers used in this study.
TABLE S2 | Physico-chemical characteristics of must after fermentations.
TABLE S3 | Genes involved in ethanol and glycerol production in yeast and evaluated for ASE.
TABLE S4 | Reciprocal hemizygotes’ physico-chemical characteristics of must after fermentations.
References
Albertyn, J., Hohmann, S., Thevelein, J. M., and Prior, B. A. (1994). GPD1, which encodes glycerol-3-phosphate dehydrogenase, is essential for growth under osmotic stress in Saccharomyces cerevisiae, and its expression is regulated by the high-osmolarity glycerol response pathway. Mol. Cell Biol. 14, 4135–4144. doi: 10.1128/MCB.14.6.4135
Araki, Y., Wu, H., Kitagaki, H., Akao, T., Takagi, H., and Shimoi, H. (2009). Ethanol stress stimulates the Ca2 + -mediated calcineurin/Crz1 pathway in Saccharomyces cerevisiae. J. Biosci. Bioeng. 107, 1–6. doi: 10.1016/j.jbiosc.2008.09.005
Bergstrom, A., Simpson, J. T., Salinas, F., Barre, B., Parts, L., Zia, A., et al. (2014). A high-definition view of functional genetic variation from natural yeast genomes. Mol. Biol. Evol. 31, 872–888. doi: 10.1093/molbev/msu037
Brem, R. B., Yvert, G., Clinton, R., and Kruglyak, L. (2002). Genetic dissection of transcriptional regulation in budding yeast. Science 296, 752–755. doi: 10.1126/science.1069516
Brice, C., Cubillos, F. A., Dequin, S., Camarasa, C., and Martinez, C. (2018). Adaptability of the Saccharomyces cerevisiae yeasts to wine fermentation conditions relies on their strong ability to consume nitrogen. PLoS One 13:e0192383. doi: 10.1371/journal.pone.0192383
Brice, C., Sanchez, I., Bigey, F., Legras, J. L., and Blondin, B. (2014). A genetic approach of wine yeast fermentation capacity in nitrogen-starvation reveals the key role of nitrogen signaling. BMC Genomics 15:495. doi: 10.1186/1471-2164-15-495
Cambon, B., Monteil, V., Remize, F., Camarasa, C., and Dequin, S. (2006). Effects of GPD1 overexpression in Saccharomyces cerevisiae commercial wine yeast strains lacking ALD6 genes. Appl. Environ. Microbiol. 72, 4688–4694. doi: 10.1128/AEM.02975-05
Crepin, L., Nidelet, T., Sanchez, I., Dequin, S., and Camarasa, C. (2012). Sequential use of nitrogen compounds by Saccharomyces cerevisiae during wine fermentation: a model based on kinetic and regulation characteristics of nitrogen permeases. Appl. Environ. Microbiol. 78, 8102–8111. doi: 10.1128/AEM.02294-12
Cubillos, F. A. (2016). Exploiting budding yeast natural variation for industrial processes. Curr. Genet. 62, 745–751. doi: 10.1007/s00294-016-0602-6
Cubillos, F. A., Billi, E., Zorgo, E., Parts, L., Fargier, P., Omholt, S., et al. (2011). Assessing the complex architecture of polygenic traits in diverged yeast populations. Mol. Ecol. 20, 1401–1413. doi: 10.1111/j.1365-294X.2011.05005.x
Cubillos, F. A., Brice, C., Molinet, J., Tisne, S., Abarca, V., Tapia, S. M., et al. (2017). Identification of nitrogen consumption genetic variants in yeast through QTL mapping and bulk segregant RNA-Seq analyses. G3 7, 1693–1705. doi: 10.1534/g3.117.042127
Cubillos, F. A., Coustham, V., and Loudet, O. (2012). Lessons from eQTL mapping studies: non-coding regions and their role behind natural phenotypic variation in plants. Curr. Opin. Plant Biol. 15, 192–198. doi: 10.1016/j.pbi.2012.01.005
Cubillos, F. A., Louis, E. J., and Liti, G. (2009). Generation of a large set of genetically tractable haploid and diploid Saccharomyces strains. FEMS Yeast Res. 9, 1217–1225. doi: 10.1111/j.1567-1364.2009.00583.x
Cubillos, F. A., Parts, L., Salinas, F., Bergstrom, A., Scovacricchi, E., Zia, A., et al. (2013). High-resolution mapping of complex traits with a four-parent advanced intercross yeast population. Genetics 195, 1141–1155. doi: 10.1534/genetics.113.155515
Cubillos, F. A., Stegle, O., Grondin, C., Canut, M., Tisne, S., Gy, I., et al. (2014). Extensive cis-regulatory variation robust to environmental perturbation in Arabidopsis. Plant Cell 26, 4298–4310. doi: 10.1105/tpc.114.130310
de Boer, C. G., and Hughes, T. R. (2012). YeTFaSCo: a database of evaluated yeast transcription factor sequence specificities. Nucleic Acids Res. 40, D169–D179. doi: 10.1093/nar/gkr993
de Smidt, O., Du Preez, J. C., and Albertyn, J. (2008). The alcohol dehydrogenases of Saccharomyces cerevisiae: a comprehensive review. FEMS Yeast Res. 8, 967–978. doi: 10.1111/j.1567-1364.2008.00387.x
DeLuna, A., Springer, M., Kirschner, M. W., and Kishony, R. (2010). Need-based up-regulation of protein levels in response to deletion of their duplicate genes. PLoS Biol. 8:e1000347. doi: 10.1371/journal.pbio.1000347
Ehrenreich, I. M., Gerke, J. P., and Kruglyak, L. (2009). Genetic dissection of complex traits in yeast: insights from studies of gene expression and other phenotypes in the BYxRM cross. Cold Spring Harb. Symp. Quant. Biol. 74, 145–153. doi: 10.1101/sqb.2009.74.013
Ehsani, M., Fernandez, M. R., Biosca, J. A., Julien, A., and Dequin, S. (2009). Engineering of 2, 3-butanediol dehydrogenase to reduce acetoin formation by glycerol-overproducing, low-alcohol Saccharomyces cerevisiae. Appl. Environ. Microbiol. 75, 3196–3205. doi: 10.1128/AEM.02157-08
Fay, J. C., Mccullough, H. L., Sniegowski, P. D., and Eisen, M. B. (2004). Population genetic variation in gene expression is associated with phenotypic variation in Saccharomyces cerevisiae. Genome Biol. 5:R26. doi: 10.1186/gb-2004-5-4-r26
Fraser, H. B., Moses, A. M., and Schadt, E. E. (2010). Evidence for widespread adaptive evolution of gene expression in budding yeast. Proc. Natl. Acad. Sci. U.S.A. 107, 2977–2982. doi: 10.1073/pnas.0912245107
Garcia-Rios, E., Morard, M., Parts, L., Liti, G., and Guillamon, J. M. (2017). The genetic architecture of low-temperature adaptation in the wine yeast Saccharomyces cerevisiae. BMC Genomics 18:159. doi: 10.1186/s12864-017-3572-2
Gawel, R., Francis, L., and Waters, E. J. (2007). Statistical correlations between the in-mouth textural characteristics and the chemical composition of Shiraz wines. J. Agric. Food Chem. 55, 2683–2687. doi: 10.1021/jf0633950
Gerke, J., Lorenz, K., and Cohen, B. (2009). Genetic interactions between transcription factors cause natural variation in yeast. Science 323, 498–501. doi: 10.1126/science.1166426
Goncalves, A., Leigh-Brown, S., Thybert, D., Stefflova, K., Turro, E., Flicek, P., et al. (2012). Extensive compensatory cis-trans regulation in the evolution of mouse gene expression. Genome Res. 22, 2376–2384. doi: 10.1101/gr.142281.112
Goncalves, E., Raguz Nakic, Z., Zampieri, M., Wagih, O., Ochoa, D., Sauer, U., et al. (2017). Systematic analysis of transcriptional and post-transcriptional regulation of metabolism in yeast. PLoS Comput. Biol. 13:e1005297. doi: 10.1371/journal.pcbi.1005297
Gutierrez, A., Beltran, G., Warringer, J., and Guillamon, J. M. (2013). Genetic basis of variations in nitrogen source utilization in four wine commercial yeast strains. PLoS One 8:e67166. doi: 10.1371/journal.pone.0067166
Hohmann, S. (2002). Osmotic stress signaling and osmoadaptation in yeasts. Microbiol. Mol. Biol. Rev. 66, 300–372. doi: 10.1128/MMBR.66.2.300-372.2002
Hubmann, G., Foulquie-Moreno, M. R., Nevoigt, E., Duitama, J., Meurens, N., Pais, T. M., et al. (2013a). Quantitative trait analysis of yeast biodiversity yields novel gene tools for metabolic engineering. Metab. Eng. 17, 68–81. doi: 10.1016/j.ymben.2013.02.006
Hubmann, G., Mathe, L., Foulquie-Moreno, M. R., Duitama, J., Nevoigt, E., and Thevelein, J. M. (2013b). Identification of multiple interacting alleles conferring low glycerol and high ethanol yield in Saccharomyces cerevisiae ethanolic fermentation. Biotechnol. Biofuels 6:87. doi: 10.1186/1754-6834-6-87
Huxley, C., Green, E. D., and Dunham, I. (1990). Rapid assessment of S. cerevisiae mating type by PCR. Trends Genet. 6:236. doi: 10.1016/0168-9525(90)90190-H
Ibstedt, S., Stenberg, S., Bages, S., Gjuvsland, A. B., Salinas, F., Kourtchenko, O., et al. (2015). Concerted evolution of life stage performances signals recent selection on yeast nitrogen use. Mol. Biol. Evol. 32, 153–161. doi: 10.1093/molbev/msu285
Jara, M., Cubillos, F. A., Garcia, V., Salinas, F., Aguilera, O., Liti, G., et al. (2014). Mapping genetic variants underlying differences in the central nitrogen metabolism in fermenter yeasts. PLoS One 9:e86533. doi: 10.1371/journal.pone.0086533
Katou, T., Namise, M., Kitagaki, H., Akao, T., and Shimoi, H. (2009). QTL mapping of sake brewing characteristics of yeast. J. Biosci. Bioeng. 107, 383–393. doi: 10.1016/j.jbiosc.2008.12.014
Kessi-Perez, E. I., Araos, S., Garcia, V., Salinas, F., Abarca, V., Larrondo, L. F., et al. (2016). RIM15 antagonistic pleiotropy is responsible for differences in fermentation and stress response kinetics in budding yeast. FEMS Yeast Res. 16:fow021. doi: 10.1093/femsyr/fow021
Kliebenstein, D. (2009). Quantitative genomics: analyzing intraspecific variation using global gene expression polymorphisms or eQTLs. Annu. Rev. Plant Biol. 60, 93–114. doi: 10.1146/annurev.arplant.043008.092114
Kvitek, D. J., Will, J. L., and Gasch, A. P. (2008). Variations in stress sensitivity and genomic expression in diverse S. cerevisiae isolates. PLoS Genet. 4:e1000223. doi: 10.1371/journal.pgen.1000223
Liti, G., and Louis, E. J. (2012). Advances in quantitative trait analysis in yeast. PLoS Genet. 8:e1002912. doi: 10.1371/journal.pgen.1002912
Liu, Y., Beyer, A., and Aebersold, R. (2016). On the dependency of cellular protein levels on mRNA abundance. Cell 165, 535–550. doi: 10.1016/j.cell.2016.03.014
Marchal, A., Marullo, P., Moine, V., and Dubourdieu, D. (2011). Influence of yeast macromolecules on sweetness in dry wines: role of the Saccharomyces cerevisiae protein Hsp12. J. Agric. Food Chem. 59, 2004–2010. doi: 10.1021/jf103710x
Marullo, P., Aigle, M., Bely, M., Masneuf-Pomarede, I., Durrens, P., Dubourdieu, D., et al. (2007). Single QTL mapping and nucleotide-level resolution of a physiologic trait in wine Saccharomyces cerevisiae strains. FEMS Yeast Res. 7, 941–952. doi: 10.1111/j.1567-1364.2007.00252.x
McManus, C. J., Coolon, J. D., Duff, M. O., Eipper-Mains, J., Graveley, B. R., and Wittkopp, P. J. (2010). Regulatory divergence in Drosophila revealed by mRNA-seq. Genome Res. 20, 816–825. doi: 10.1101/gr.102491.109
Nakamura, Y., Gojobori, T., and Ikemura, T. (2000). Codon usage tabulated from international DNA sequence databases: status for the year 2000. Nucleic Acids Res. 28:292. doi: 10.1093/nar/28.1.292
Nevoigt, E., and Stahl, U. (1997). Osmoregulation and glycerol metabolism in the yeast Saccharomyces cerevisiae. FEMS Microbiol. Rev. 21, 231–241. doi: 10.1111/j.1574-6976.1997.tb00352.x
Nissen, T. L., Schulze, U., Nielsen, J., and Villadsen, J. (1997). Flux distributions in anaerobic, glucose-limited continuous cultures of Saccharomyces cerevisiae. Microbiology 143(Pt 1), 203–218.
Oldenburg, K. R., Vo, K. T., Michaelis, S., and Paddon, C. (1997). Recombination-mediated PCR-directed plasmid construction in vivo in yeast. Nucleic Acids Res. 25, 451–452. doi: 10.1093/nar/25.2.451
Pais, T. M., Foulquie-Moreno, M. R., Hubmann, G., Duitama, J., Swinnen, S., Goovaerts, A., et al. (2013). Comparative polygenic analysis of maximal ethanol accumulation capacity and tolerance to high ethanol levels of cell proliferation in yeast. PLoS Genet. 9:e1003548. doi: 10.1371/journal.pgen.1003548
Peltier, E., Bernard, M., Trujillo, M., Prodhomme, D., Barbe, J. C., Gibon, Y., et al. (2018). Wine yeast phenomics: a standardized fermentation method for assessing quantitative traits of Saccharomyces cerevisiae strains in enological conditions. PLoS One 13:e0190094. doi: 10.1371/journal.pone.0190094
Peter, J., De Chiara, M., Friedrich, A., Yue, J. X., Pflieger, D., Bergstrom, A., et al. (2018). Genome evolution across 1,011 Saccharomyces cerevisiae isolates. Nature 556, 339–344. doi: 10.1038/s41586-018-0030-5
Puigbo, P., Bravo, I. G., and Garcia-Vallve, S. (2008). CAIcal: a combined set of tools to assess codon usage adaptation. Biol. Direct 3:38. doi: 10.1186/1745-6150-3-38
Rienzo, A., Pascual-Ahuir, A., and Proft, M. (2012). The use of a real-time luciferase assay to quantify gene expression dynamics in the living yeast cell. Yeast 29, 219–231. doi: 10.1002/yea.2905
Rossignol, T., Dulau, L., Julien, A., and Blondin, B. (2003). Genome-wide monitoring of wine yeast gene expression during alcoholic fermentation. Yeast 20, 1369–1385. doi: 10.1002/yea.1046
Salinas, F., Cubillos, F. A., Soto, D., Garcia, V., Bergstrom, A., Warringer, J., et al. (2012). The genetic basis of natural variation in oenological traits in Saccharomyces cerevisiae. PloS One 7:e49640. doi: 10.1371/journal.pone.0049640
Salinas, F., De Boer, C. G., Abarca, V., Garcia, V., Cuevas, M., Araos, S., et al. (2016). Natural variation in non-coding regions underlying phenotypic diversity in budding yeast. Sci. Rep. 6:21849. doi: 10.1038/srep21849
Steensels, J., Snoek, T., Meersman, E., Picca Nicolino, M., Voordeckers, K., and Verstrepen, K. J. (2014). Improving industrial yeast strains: exploiting natural and artificial diversity. FEMS Microbiol. Rev. 38, 947–995. doi: 10.1111/1574-6976.12073
Swinnen, S., Schaerlaekens, K., Pais, T., Claesen, J., Hubmann, G., Yang, Y., et al. (2012). Identification of novel causative genes determining the complex trait of high ethanol tolerance in yeast using pooled-segregant whole-genome sequence analysis. Genome Res. 22, 975–984. doi: 10.1101/gr.131698.111
Thompson, D. A., and Cubillos, F. A. (2017). Natural gene expression variation studies in yeast. Yeast 34, 3–17. doi: 10.1002/yea.3210
Tilloy, V., Ortiz-Julien, A., and Dequin, S. (2014). Reduction of ethanol yield and improvement of glycerol formation by adaptive evolution of the wine yeast Saccharomyces cerevisiae under hyperosmotic conditions. Appl. Environ. Microbiol. 80, 2623–2632. doi: 10.1128/AEM.03710-13
Varela, C., Pizarro, F., and Agosin, E. (2004). Biomass content governs fermentation rate in nitrogen-deficient wine musts. Appl. Environ. Microbiol. 70, 3392–3400. doi: 10.1128/AEM.70.6.3392-3400.2004
Warringer, J., and Blomberg, A. (2003). Automated screening in environmental arrays allows analysis of quantitative phenotypic profiles in Saccharomyces cerevisiae. Yeast 20, 53–67. doi: 10.1002/yea.931
Wray, G. A. (2007). The evolutionary significance of cis-regulatory mutations. Nat. Rev. Genet. 8, 206–216. doi: 10.1038/nrg2063
Yin, Z., Hatton, L., and Brown, A. J. (2000). Differential post-transcriptional regulation of yeast mRNAs in response to high and low glucose concentrations. Mol. Microbiol. 35, 553–565. doi: 10.1046/j.1365-2958.2000.01723.x
Young, E. T., and Pilgrim, D. (1985). Isolation and DNA sequence of ADH3, a nuclear gene encoding the mitochondrial isozyme of alcohol dehydrogenase in Saccharomyces cerevisiae. Mol. Cell Biol. 5, 3024–3034. doi: 10.1128/MCB.5.11.3024
Yvert, G., Brem, R. B., Whittle, J., Akey, J. M., Foss, E., Smith, E. N., et al. (2003). Trans-acting regulatory variation in Saccharomyces cerevisiae and the role of transcription factors. Nat. Genet. 35, 57–64. doi: 10.1038/ng1222
Zhao, X., Procopio, S., and Becker, T. (2015). Flavor impacts of glycerol in the processing of yeast fermented beverages: a review. J. Food Sci. Technol. 52, 7588–7598. doi: 10.1007/s13197-015-1977-y
Zheng, W., Zhao, H., Mancera, E., Steinmetz, L. M., and Snyder, M. (2010). Genetic analysis of variation in transcription factor binding in yeast. Nature 464, 1187–1191. doi: 10.1038/nature08934
Keywords: wine, yeast, glycerol, alleles, Saccharomyces
Citation: Tapia SM, Cuevas M, Abarca V, Delgado V, Rojas V, García V, Brice C, Martínez C, Salinas F, Larrondo LF and Cubillos FA (2018) GPD1 and ADH3 Natural Variants Underlie Glycerol Yield Differences in Wine Fermentation. Front. Microbiol. 9:1460. doi: 10.3389/fmicb.2018.01460
Received: 19 March 2018; Accepted: 12 June 2018;
Published: 03 July 2018.
Edited by:
Aline Lonvaud, Université de Bordeaux, FranceReviewed by:
Philippe Marullo, BIOLAFFORT and Université de Bordeaux, FranceJohan M. Thevelein, KU Leuven, Belgium
Copyright © 2018 Tapia, Cuevas, Abarca, Delgado, Rojas, García, Brice, Martínez, Salinas, Larrondo and Cubillos. This is an open-access article distributed under the terms of the Creative Commons Attribution License (CC BY). The use, distribution or reproduction in other forums is permitted, provided the original author(s) and the copyright owner(s) are credited and that the original publication in this journal is cited, in accordance with accepted academic practice. No use, distribution or reproduction is permitted which does not comply with these terms.
*Correspondence: Francisco A. Cubillos, francisco.cubillos.r@usach.cl