- 1Key Laboratory of Environmental and Applied Microbiology – Environmental Microbiology Key Laboratory of Sichuan Province, Chengdu Institute of Biology, Chinese Academy of Sciences, Chengdu, China
- 2University of Chinese Academy of Sciences, Beijing, China
- 3Chengdu Medical College, Chengdu, China
- 4Chengdu Zoo, Chengdu Institute of Wildlife, Chengdu, China
- 5Fujian Provincial Key Laboratory of Soil Environmental Health and Regulation, College of Resources and Environment, Fujian Agriculture and Forestry University, Fuzhou, China
Gastrointestinal microbiota may shape the adaptation of their hosts to different habitats and lifestyles, thereby driving their evolutionary diversification. It remains unknown if gastrointestinal microbiota diverge in congruence with the phylogenetic relationships of their hosts. To evaluate the phylosymbiotic relationships, here we analyzed the compositions of fecal microbiota of seven Cervinae species raised in the Chengdu Zoo. All sampled animals were kept in the same environmental condition and fed identical fodder for years. Results showed that Firmicutes and Bacteroidetes were dominant in their fecal microbiota. Even though some bacteria (e.g., Ruminococcaceae) were found to be common in the feces of all investigated species, some genera (e.g., Sharpea and Succinivibrio) were only observed in animals with particular digestive systems. As for the intraspecies variations of microbial communities, only a few operational taxonomic units (OTUs) were shared among replicates of the same host species although they accounted for most of the total abundance. Correlation was observed between the fecal microbiota divergence and host phylogeny, but they were not congruent completely. This may shed new light on the coevolution of host species and their microbiota.
Introduction
Gastrointestinal microbiota may play important roles in food digestion, energy metabolism, immunity regulation, and in shaping behavioral phenotypes of animals (Warnecke et al., 2007; Tremaroli and Bäckhed, 2012; Chevalier et al., 2015; De Palma et al., 2015). Correlations have been reported between the host phylogenies and divergence in their gut microbiota (Ochman et al., 2010; Brucker and Bordenstein, 2012a; Brooks et al., 2016; Groussin et al., 2017). It implies that the divergence of gut microbiota may link to the diversification of their hosts through evolution (Delsuc et al., 2014). The codivergent history of hosts’ genotypes and their microbiota is termed as “phylosymbiosis” (Brucker and Bordenstein, 2012a,b). This pattern has been observed in many species from sponges to primates (Ochman et al., 2010; Phillips et al., 2012; Easson and Thacker, 2014; Moeller et al., 2014; Sanders et al., 2014). The selective mechanisms of hosts for maintaining species-specific microbiota were revealed in a reciprocal transplantation study (Rawls et al., 2006). Moreover, Li et al. (2017) reported that the differences of gut microbiota were positively correlated with host divergence time of Glires, thus speculating that gut microbiota may reflect divergent time of diverse hosts. Additionally, the study of Kohl et al. (2018a) showed that the pattern of phylosymbiosis was stabilized across different gastrointestinal tract regions, as well as in feces.
Multiple factors, such as diet, geographic distribution, and physiological condition, can influence alimentary tract microbiota (Ley et al., 2005, 2006, 2008; Benson et al., 2010; Zhang et al., 2010). Although gut microbiota of conspecifics are often similar (Ochman et al., 2010), these factors also lead to discordances between gut microbiota divergence and hosts’ phylogenies. Diet, a dominant factor shaping the gut microbiota, often relates to environmental sources. Dietary change can alter gut microbiota vastly and instantly in humans (David et al., 2014). By investigating 32 ruminant livestock species, Henderson et al. (2015) demonstrated that the variations in rumen microbiota were related to host species’ identity than to diet. A similar pattern was observed in mice (Carmody et al., 2015). Most previous studies on the evolution of gut microbiota were carried out in mammalian species from different areas without strict control of diet or environment (Ochman et al., 2010; Li et al., 2017). Controlled studies were only performed in a few species, such as Peromyscus deer mice, Drosophila flies, mosquitoes, and Nasonia wasps (Brucker and Bordenstein, 2012a, 2013; Brooks et al., 2016; Kohl et al., 2018a). Considering shared effects of host phylogeny and their dietary strategies, the relationship between host genetic diversification and the gastrointestinal microbiota remains unclarified (Ley et al., 2008; Muegge et al., 2011).
Mammalian herbivores have the highest net diversification rate than carnivores and omnivores (Martin and Maron, 2012; Price et al., 2012). They exhibit the highest microbial diversity among mammalian species (Ley et al., 2008; Pope et al., 2010). Ruminants, with around 200 species, occupy many different habitats (Hofmann, 1989; Hackmann and Spain, 2010). Microbiota in herbivorous alimentary tract deliver myriad services to their hosts, such as breakdown of recalcitrant plant fiber, detoxification of plant secondary compounds, and production of essential amino acids and vitamins (Muegge et al., 2011; Karasov and Douglas, 2013; LeBlanc et al., 2013; Kohl et al., 2014; Kohl and Dearing, 2016). Microbiota have been investigated in some host species of ruminants; however, they are documented insufficiently in many rare species (Li et al., 2014; Henderson et al., 2015; Delgado et al., 2017; Guan et al., 2017).
To control the dietary impact on gastrointestinal microbiota, we surveyed seven Cervinae species fed with the same fodder for years in the Chengdu Zoo. Because previous evidence showed that fecal data can represent a combination of microbial communities distributed throughout the gastrointestinal tract, we used fecal samples as proxies of intestinal tract samples (Eckburg et al., 2005). Additionally, considering its non-invasive property, fecal sampling is beneficial for endangered species (Hu et al., 2017). Herein, we selected Cervini and Muntiacini subfamilies in the clade Cervinae. Within Cervini, there are five extant genera, namely Axis (hog deer), Cervus (red deer and sika), Rusa (sambar), Dama (fallow deer), and Elaphurus (Père David’s deer). Tufted deer (Elaphodus cephalophus), belonging to Elaphodus, was selected to represent Muntiacini. We examined compositions and diversity of fecal microbiota in these animals. Then we assessed the relationship between hosts’ mitochondrial genetic distances and microbial community dissimilarity matrices. This cross-species examination of the linkage between the microbiota and their hosts’ phylogenies will reveal a species-specific signature of symbiotic bacteria. More importantly, Père David’s deer, hog deer, and sambar are evaluated as threatened species in the International Union for Conservation of Nature (IUCN) Red List (Extinct in the Wild, Endangered, and Vulnerable, respectively); however, there is little information about their gut microbiota. Our findings will extend the knowledge about these rare mammalians’ gastrointestinal microbiota, which ultimately will serve in their conservation or management.
Materials and Methods
Ethics Statement
Compliance with the Ethics Committee of the Chengdu Institute of Biology, Chinese Academy of Sciences, and the methods used in this study were carried out in accordance with the approved guidelines.
Sample Collection
We collected samples on April 06, 2017. Axis (hog deer, Axis porcinus), Cervus (red deer, Cervus elaphus; sika, Cervus nippon), Rusa (sambar, Rusa unicolor), Dama (fallow deer, Dama dama), and Elaphurus (Père David’s deer, Elaphurus davidianus) in Cervini and Elaphodus (tufted deer, Elaphodus cephalophus) in Muntiacini (Muntiacini contains two genera, and only Elaphodus was investigated in this study) were raised in Chengdu Zoo, Sichuan Province of China. All animals lived in the same environment condition and consumed the same fodder for years (nutritional ingredients are shown in Supplementary Table S1). The adult animals were kept in enclosures. All of them were healthy and not injected with any antibiotics or other treatments in the past 6 months. We collected fresh samples immediately after excretion. For each species, three piles of fecal pellets were selected, and each pile was excreted by an individual. Fecal specimens were frozen at -20°C rapidly. Surface part of a fecal sample was scraped to escape contamination. Finally, all the samples were transferred and stored at -40°C.
Reconstructing Phylogeny of Host Species
The molecular phylogeny was reconstructed using complete mitochondrial DNA sequences. Corresponding sequences were downloaded from GenBank (Accession number: HQ832482.1, AB245427.2, NC_031835.1, JN632629.1, JN399997.1, MF435989, DQ873526.1). All sequences were aligned by Clustalw2 (Larkin et al., 2007). Reindeer (Rangifer tarandus) was used as the outgroup taxon (Accession number: AB245426.1). Pairwise distances of the complete mitochondrial sequences between host species were generated by Bayesian inference (Ronquist et al., 2012).
DNA Extraction, PCR Amplification, and Sequencing
Genomic DNA extraction of fecal samples was performed with MoBio DNeasy PowerSoil DNA isolation kit (Qiagen, Germany). DNA concentration was checked using NanoDrop 2000 Spectrophotometer (Thermo Scientific, United States). V4–V5 hypervariable region of bacterial 16S rRNA gene was amplified by pair primers (515F: 5-GTGYCAGCMGCCGCGGTA-3 and 909R: 5-CCCCGYCAATTCMTTTRAGT-3) (Tamaki et al., 2011). A unique barcode at the 5′ end of 515F primer was incorporated to distinguish each sample. For each sample, we conducted two PCR reactions. Each 25 μL PCR reaction system included 10 ng DNA template, 2.0 μM each primer, 1.5 mM MgCl2, 0.4 μM dNTPs, 0.25 U of Taq (TaKaRa, Dalian) and 1x reaction buffer. The PCR condition was composed of 3 min at 94°C, 30 cycles of 94°C for 30 s, 56°C for 30 s, 72°C for 30 s, and 10 min at 72°C. Blank controls were used in DNA extraction and PCR amplification, and no amplification band was observed. Finally, samples were sequenced using an Illumina MiSeq sequencer (MiSeq Reagent Kit V.2, 500 cycles) at Environmental Genomic Platform of Chengdu Institute of Biology. Because the negative controls did not show any PCR bands, we did not sequence blank controls although blank sequencing controls may be useful in determining possible contaminations. The raw sequences were deposited in NCBI Sequence Read Archive with accession number SRP142187.
Bioinformatics Analysis
QIIME Pipeline1 (Version 1.7.0) was used to analyze raw reads (Caporaso et al., 2010). Reads were merged using FLASH-1.2.8 software (Magoc and Salzberg, 2011). Low-quality sequences, chimeras, mitochondria, and chloroplasts were removed. Then operational taxonomic units (OTUs) were clustered at a cutoff of 97% sequence identity using CD-HIT (Li and Godzik, 2006; Edgar et al., 2011). After filtering out singleton sequences, the number of sequences per sample was normalized to 9,683, and the rarefaction curves were generated. Alpha diversity indices (observed OTUs, Chao 1, Shannon, Simpson, and equitability index) were calculated. One-way ANOVA was performed to test the difference among host species. To identify core microbiota of each host species, the number of shared OTUs among all replications were calculated. The shared OTUs/sequences were shown as the proportion of total OTUs/sequences per species. The bioinformatics tool Tax4Fun was used to examine whether the microbiota of these animals exhibited different predicted functions (Aßhauer et al., 2015). UpSet plots were generated using UpSetR in R (Conway et al., 2017).
Both OTUs and phylogeny-based approaches were used to explore the relationship between fecal microbiota and their hosts. For an OTU-based method, we visualized a Jaccard dissimilarity matrix of each host species dataset using unweighted pair group approach with arithmetic mean (UPGMA) dendrogram. Host mitochondria sequence data were analyzed using maximum likelihood (ML) implemented in RaxmlGUI 1.3 (Silvestro and Michalak, 2012) and Bayesian inference (BI) using MrBayes 3.12 (Ronquist and Huelsenbeck, 2003). The phylogenetic tree was constructed using ML and BI methods. For ML analysis, the bootstrap consensus tree inferred from 1,000 replicates was used to represent the evolutionary history of the taxa analyzed. Branches corresponding to partitions reproduced in less than 70% of bootstrap replicates were collapsed (Hillis and Bull, 1993). For BI analysis, two independent runs with four Markov Chain Monte Carlo simulations were performed for ten million iterations and sampled every 1,000th iteration. The first 25% of samples were discarded as burn-in. Convergence of the Markov Chain Monte Carlo simulations was assessed using Tracer v.1.42. The congruent results were shown in Supplementary Figure S4. To test the correlations between the fecal microbiota and their hosts’ phylogenies, we used Mantel test (with 9,999 iterations) to compare the distance matrix of host mitochondrial completed sequences and Jaccard dissimilarity matrix of fecal microbiota. We validated the comparison by calculating the Robinson–Foulds and Matching Cluster congruency scores as previously described (Brooks et al., 2016). Matching Cluster and Robinson–Foulds p-values were determined by the probability of 80,000 randomized bifurcating dendrogram topologies yielding equivalent or more congruent phylosymbiotic patterns than the microbiota dendrograms (Brooks et al., 2016). For a phylogeny-based approach, unweighted UniFrac matrix was produced through the QIIME pipeline and plotted in principal coordinates analysis (PCoA) (Lozupone and Knight, 2005). To further assess the effects of host phylogeny, unweighted UniFrac distance matrices of different hosts were compared using Analysis of Similarity (ANOSIM) (with 999 iterations). For the comparisons among microbial community structures, weighted UniFrac matrix was also calculated.
Results
Overall Microbial Community Compositions
Microbial community compositions of 21 fecal samples from six Cervini species (A. porcinus, C. elaphus, C. nippon, R. unicolor, D. dama, and E. davidianus) and one Muntiacini species (E. cephalophus) were obtained by MiSeq sequencing method. In total, 8,849 non-singleton OTUs (at 97% sequence identity) were identified in the datasets. The fitted OTU-level rarefaction curves of Good’s coverage index reached stable values for all samples (Supplementary Figure S1), showing that sequencing depth was enough to capture most of the microbial diversity.
Fecal microbiota were identified at different taxonomic levels. At phylum level, samples mainly contained Firmicutes (mean ± SD = 49.61% ± 5.04% of total sequences), Bacteroidetes (37.74% ± 7.43%), Spirochaetes (3.76% ± 3.03%), Proteobacteria (3.57% ± 8.06%), Tenericutes (2.68% ± 1.39%), and other phyla accounted for less than 1% of total sequences, e.g., Fibrobacteres 0.75% ± 2.13%, Actinobacteria, 0.68% ± 0.69%, Verrucomicrobia 0.25% ± 0.21%, and Planctomycetes 0.21% ± 0.22% (Figure 1A). Low variations among replications were observed in most deer species except for A. porcinus. At family level, Ruminococcaceae (28.50% ± 6.70%) was predominant in most samples (Figure 1B). The dominant taxa with the relative abundances greater than 5% included an unclassified family of Bacteroidales (9.20% ± 5.12%), Bacteroidaceae (8.43% ± 3.96%), and an unclassified family of Clostridiales (6.19% ± 1.78%). The relative abundance of Planococcaceae was much higher in Muntiacini (Elaphodus cephalophus) (5.33–10.38%) than that in Cervini species <0.14%). The taxonomic compositions for A. porcinus and Elaphodus cephalophus species at the family level may be doubtful because of the great variabilities among replicates. For instance, in certain replicates, the abundance of Succinivibrionaceae maximized at 39.05% in A. porcinus and 11.21% in Elaphodus cephalophus, whereas it ranged between 0 and 0.03% in other species. Most OTUs identified in our samples are related to strict or facultative anaerobic or facultative anaerobic microbes being representative members of gut microbiota. They are obviously different from the majority components of contamination (aerobic microbes) described by Salter et al. (2014). It may partially support the reliability of our sampling and sequencing procedures.
The Inter- and Intra-Species Core Microbiota in Cervinae
Core genera affiliated to phylum Actinobacteria, Bacteroidetes, Firmicutes, Proteobacteria, Tenericutes, and Verrucomicrobia were further investigated across all samples. In addition, TM7, Chloroflexi, and Elusimicrobia served as species-specific core phyla (presented in all samples per species) with low relative abundances (0.04% ± 0.01%, 0.33% ± 0.28% and 0.02% ± 0.02%) in C. nippon, Elaphurus davidianus, and D. dama, respectively. Twenty-eight core genera (11 of them can be annotated at genus level) were identified almost within Firmicutes (19 genera), Bacteroidetes (7 genera), and Tenericutes (1 genus). Most OTUs (74.42% ± 9.23%) in each sample were affiliated to these genera. Spearman’s rank correlation coefficient was calculated to demonstrate the co-occurrence patterns among 11 annotated core genera. Our results indicated that patterns among core taxa of microbiota were not consistent in different host species (Figure 2 and Supplementary Figure S2).
To identify inter- and intra-species core bacterial taxa, the shared and the host species-specific core OTUs were examined (Table 1 and Figure 3). The shared OTUs among replicates of each species were relatively low (3.15–22.39% of total OTUs), especially for Elaphodus cephalophus. Nevertheless, for the majority of species, these shared OTUs contributed a large fraction of the total sequences (55.24%–77.00%), with the exceptions of A. porcinus (39.26% ± 13.14%) and Elaphodus cephalophus (27.16% ± 1.96%). Among 8,849 OTUs, 136 core OTUs of Muntiacini occurred in Cervini and 24 of them served as core OTUs across all species. Most (66.67%) of these common core OTUs of each species were from Ruminococcaceae family. Species-specific core OTUs were also assessed. For example, OTUs associated with Succinivibrio showed high abundances in A. porcinus (11.58%), whereas they were scarcely detected in other species (except for one sample of Elaphodus cephalophus). Sharpea had high prevalence and little variation in all individuals of D. dama (0.88%) and low abundance in a small number of individuals of other species (∼0.03%). The taxonomic assignments of core OTUs were summarized in Supplementary Table S2. Despite the low percentages of common core OTUs, the 274 KEGG pathways were discovered through predicted functional analysis of microbiota and 259 of them were present among all sampled animals (Supplementary Figure S3 and Supplementary Table S3).
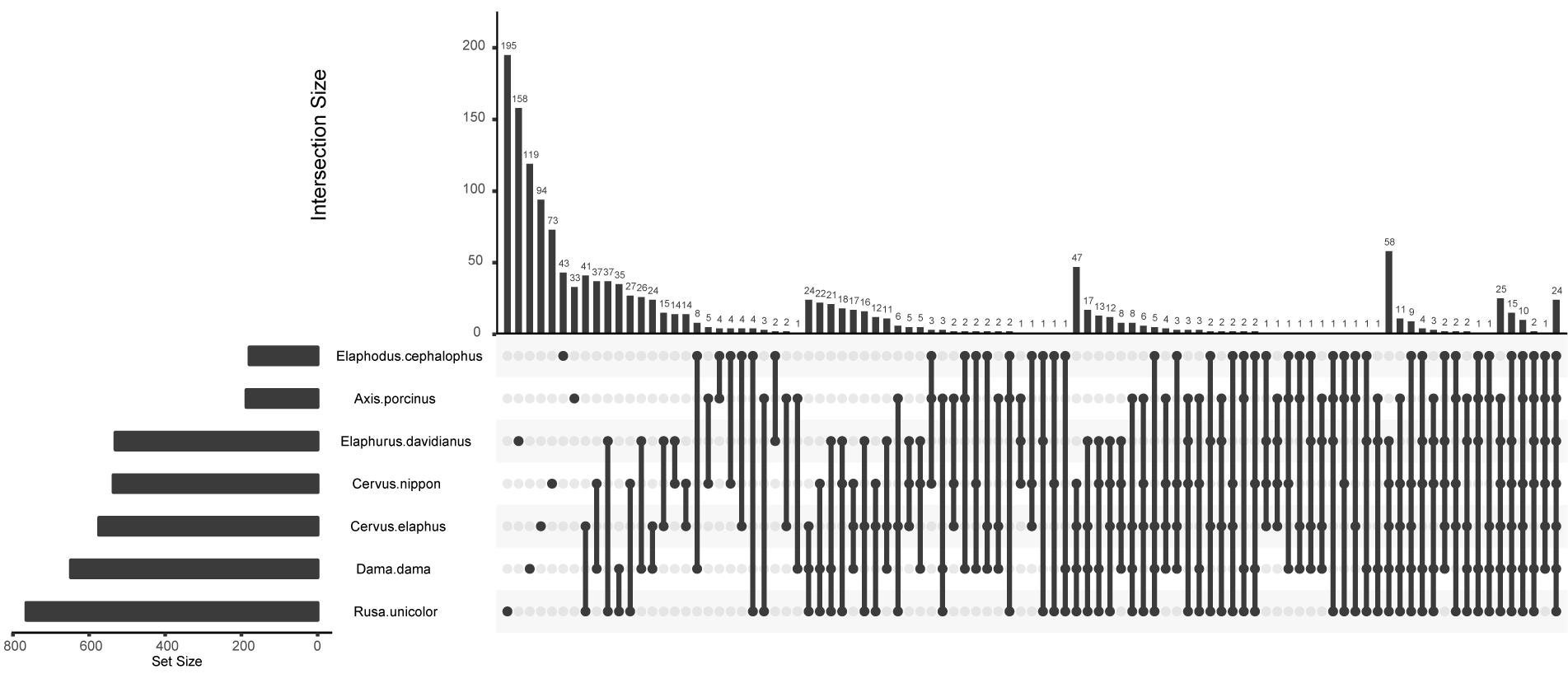
FIGURE 3. An Upset plot of host species-specific core OTUs. Set metadata of each host species was plotted to the left of the set size bar (charts). Dark circles indicated samples with containing accessions and connecting bar indicated multiple overlapping samples.
Comparisons of Gut Microbiota Among Cervinae Species
The variations of gut microbiota between and within each Cervinae species were reflected in the alpha diversity (Table 1). R. unicolor had the highest Chao 1 richness values (3,141 ± 204), whereas A. porcinus owned the highest variability in the replications (2,202 ± 681). In contrast, Elaphodus cephalophus possessed the lowest mean richness value (1,793 ± 801), whereas D. dama held the lowest variable richness value among the replications (2,675 ± 51). However, no significant differences were observed in terms of Shannon and Simpson indices.
A remarkable overall correlation between fecal microbiota and their hosts’ phylogenies was observed based on Mantel test (r = 0.8025, P < 0.05). The significance of this pattern was validated using Robinson–Foulds and Matching Cluster analysis (nRF = 0.5, P = 0.0535; nMC = 0.4, P = 0.0461). Nevertheless, the hierarchical dendrogram of microbiota and their hosts’ phylogenies were not completely congruent (Figure 4). ANOSIM method identified significant differences of unweighted UniFrac distance matrices at tribe (r = 0.68, P < 0.05), genus (r = 0.60, P = 0.001), and species level (r = 0.52, P = 0.001). In addition, Axis (r = 0.80, P < 0.05) and Elaphodus (r = 0.40, P < 0.05) showed significant differences when compared with Cervus. The global differences in microbial community compositions were clearly visualized by PCoA of unweighted UniFrac distance matrix (Figure 5A). Higher community dispersions were detected in individuals of Elaphodus cephalophus and A. porcinus, and samples from these two species deviated from other five species. Weighted UniFrac distance matrix (including bacterial taxa abundance) revealed a similar pattern but with less variability among individuals, especially for Cervini species (expect A. porcinus) (Figure 5B).
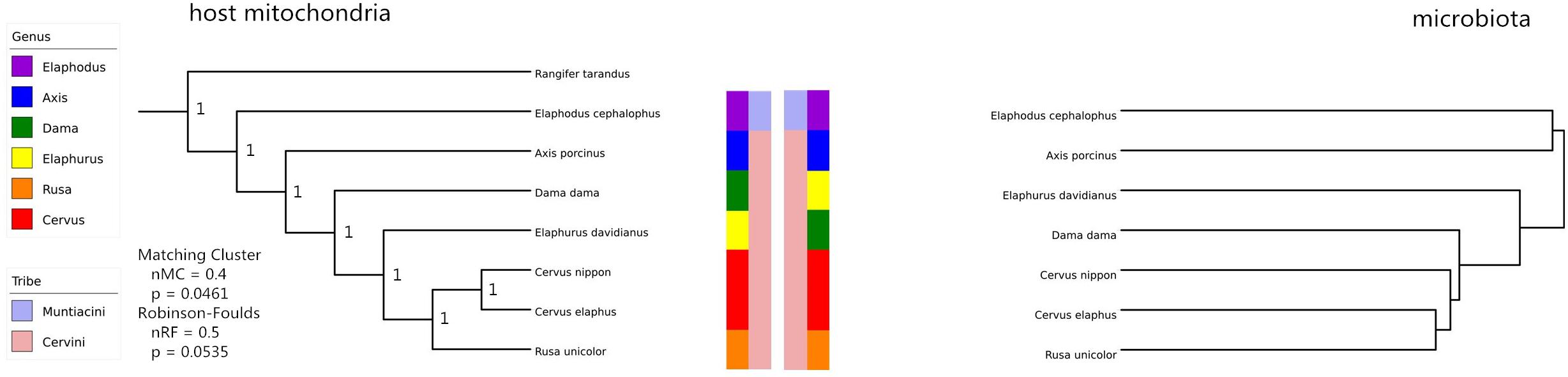
FIGURE 4. Mitochondrial completed sequences gene-based Cervinae phylogeny (left) and host microbiota based on OUT (right). Normalized Robinson–Foulds (RF) and normalized Matching Cluster (MC) metrics were determined as Brooks et al. (2016) described. Normalized metrics (nRF and nMC) scale from 0.0 (complete congruence) to 1.0 (complete incongruence).
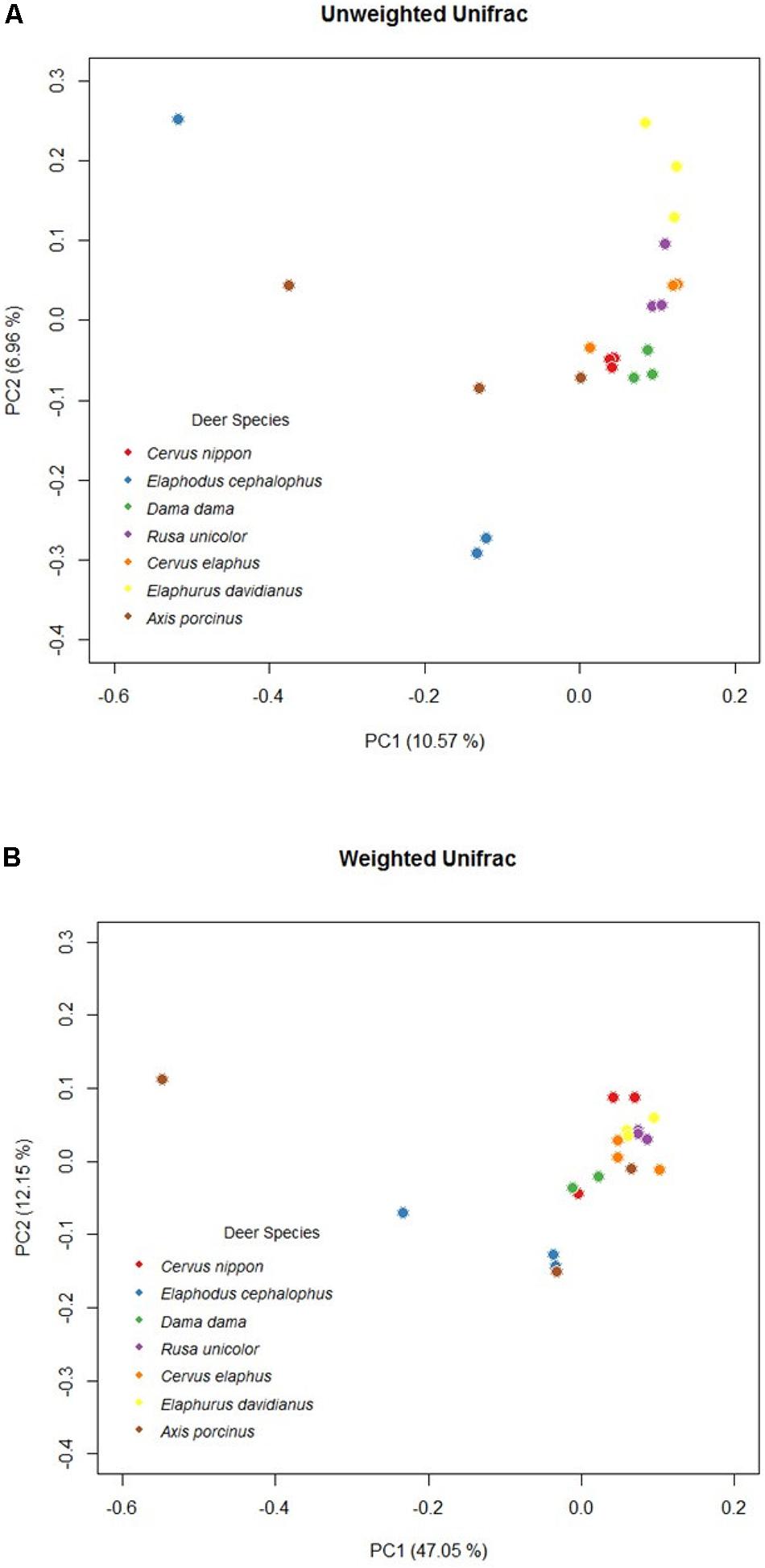
FIGURE 5. Principal coordinates plots based on OTU-level unweighted(A) weighted (B) UniFrac distance among Cervinae.
Discussion
Cervidae is the second most diverse subclade among terricolous artiodactyls. They natively inhabit from tropics to arctic regions and adapt themselves to various environments (Mattioli, 2011). We did the first study to investigate bacterial communities in closely related Cervinae species reared in the same environmental conditions. Our sample collection strategy provided a framework for characterizing host-bacterial generality and specificity in Cervinae species.
Our data revealed that the dominant phyla in these seven Cervidae species were composed of Firmicutes and Bacteroidetes, which play important roles in food fermentation (Ley et al., 2006, 2008; Durso et al., 2010; Li et al., 2014; Delgado et al., 2017; Hu et al., 2017). Low-abundant bacteria were affiliated to Spirochaetes, Fibrobacteres, Verrucomicrobia, Proteobacteria, Tenericutes, and Actinobacteria. These microbial components were also observed in other ruminants (Kong et al., 2010; Pope et al., 2012; Jami et al., 2013; Kittelmann et al., 2013; Pitta et al., 2014a,b). It seems that only a small number of bacterial phyla can adapt to the gut environment (Ley et al., 2006, 2008). At family level, as described in a previous study about moose (Ishaq and Wright, 2012), Peptococcaceae, Clostridiaceae, Succinivibrionaceae, and Lachnospiraceae were observed in the seven Cervinae species. These bacterial families are found in healthy rats rather than in their counterparts with irritable bowel syndrome (Nelson et al., 2011). This may indicate the health condition of these cervids in this experiment.
Interestingly, high levels of the variability were observed for some taxa (Figure 1). At phylum level, Bacteroidetes and Proteobacteria were evaluated as highly variable taxa. It was in agreement with the previous studies for cows, yaks, and humans (Arumugam et al., 2011; Jami and Mizrahi, 2012; Guo et al., 2015; Tang et al., 2017). Jami and Mizrahi (2012) revealed that the abundance of Bacteroidetes accounted for 26–70% of all reads, whereas the abundances of Proteobacteria ranged from 0.5% to 20% of all reads in some samples although all cows were fed in the same farm with same diet. Guo et al. (2015) also observed a significant fluctuation of the relative abundance of Proteobacteria among individual yaks though all yaks were male and lived in the same farm under similar nutritional conditions. At family level, Succinivibrionaceae and S24-7 were dominantly variable taxa. Tang et al. (2017) also observed the same pattern in cows that these taxa contribute to cow-to-cow variation under the same feeding regimen at the same farm. This may indicate that different enterotypes exist in cervids.
Important genera were identified owning cellulolytic (e.g., Fibrobacter, Ruminococcus, Butyrivibrio), lipolytic (e.g., Anaerovibrio), proteolytic (e.g., Clostridium, Bacteroides), and amylolytic (e.g., Prevotella, Bifidobacterium) functions (Weimer, 1993; Warnecke et al., 2007; Brulc et al., 2009; Pitta et al., 2010; Dai et al., 2014; Nie et al., 2018). Ruminococcus contained almost all of the core OTUs shared among host species. Ruminococcus is well known for its fiber-degrading capability (Leatherwood, 1965). It is common and dominant in the gastrointestinal tracts of herbivores (Henderson et al., 2015). Apart from deer, Ruminococcus was observed in many other species, e.g., alpaca, cattle, goat, horse, sheep, pika, and rhinoceros (Dowd et al., 2008; Kim et al., 2011; Robert, 2012; Li et al., 2017; Neumann et al., 2017; O’Donnell et al., 2017; Kohl et al., 2018b). It may indicate the housekeeping functions of this genus. We also assessed the signature core taxa for each species from these 21 samples. Sharpea azabuensis owns rapid heterofermentative growths, and it plays an essential role in lactate production and utilization (Kamke et al., 2016). Despite some anomalies, S. azabuensis had higher relative abundance (0.88%) in D. dama than those in other host species (∼0.03%). A previous study showed that a smaller rumen size with a higher turnover rate may tend to select microorganisms that are capable of fast, heterofermentative growth on sugars (Bain et al., 2014; Goopy et al., 2014; Kittelmann et al., 2014). Thus, we speculated that D. dama might rely more on lactate heterofermentative metabolism than other examined species. A previous report revealed that the populations of Succinivibrio sp., Eubacterium sp., and Robinsoniella sp. correlate with digestion efficiency because of their potential metabolic capability (e.g., formate production, propionate synthesis, and syntrophic interactions with methanogens) (Hernandez-Sanabria et al., 2012). Our datasets showed that A. porcinus harbored high abundance of Succinivibrio (11.58%) and Eubacterium (0.028%) than other host species, which suggested that A. porcinus might have higher digestion efficiency.
The correlations among core bacterial genera were not completely congruent among different species. For instance, genera Prevotella and Clostridium showed markedly positive correlations between D. dama and A. porcinus but negative correlations between R. unicolor and C. nippon. It contrasted with the results of Li et al. (2017), which showed completely consistent co-occurrence patterns of core genera among eight Glires species. Because the digestive tracts of different host species differ in niche specificity and nutrient availability, taxa with positive or negative core bacteria correlation patterns may indicate that the co-occurrence of core genera is only achieved in some specific environments (Faust et al., 2012).
Although all hosts were raised in identical living conditions with the same fodder, shared OTUs among replicates were surprisingly low (3.15–22.39%), particularly in A. porcinus and Elaphodus cephalophus (6.56% and 3.15%, respectively). Besides, in terms of alpha-diversity indices, D. dama species harbored relatively constant bacterial diversity between the replicates, whereas A. porcinus and Elaphodus cephalophus had large intraspecies variations. A previous study elucidated that the hosts can select specific taxa (Smith et al., 2015), and the variations of microbiota existed among individuals of species or genotype (Smith et al., 2015). These observed intra- and inter-species variations on microbiota may serve as indicators of the ecological processes, which shape host-associated microbial community. Because host-associated microbial communities are shaped by both deterministic and stochastic processes, we can speculate that stochastic processes may play more important roles in shaping microbiota in A. porcinus and Elaphodus cephalophus species rather than that in D. dama. In addition, despite the low counts of shared OTUs among replications of each host-species, the shared OTUs accounted for most retrieved sequences (except A. porcinus and Elaphodus cephalophus). This was consistent with a previous study on surgeonfishes (Miyake et al., 2015). Despite the variations of community and structure, functional stability was observed. An increasing number of studies revealed that microbial taxa can be de-coupled with their function (Burke et al., 2011; Purahong et al., 2014; Louca et al., 2016; Bletz et al., 2017). Multiple microbial taxa selected by or adapted to a host may be functionally redundant in a host-associated community (Lozupone et al., 2012; Bletz et al., 2017). It must be noted that the functional prediction through a bioinformatics approach in this study was based on current database and the assumptions of functional equivalence of 16S gene matches. However, the results in our study may serve as a preliminary indication. Future analyses concerning metagenomic and metatranscriptomic approaches will be helpful to elucidate the interactions of host–microbiota, as well as microbial structure and function.
Although the distance matrices of hosts’ phylogenies were not strictly consistent with those of microbial compositions, a remarkable association between them was observed in this study. It is in line with the study of surgeonfishes (Miyake et al., 2015). Ley et al. (2008) demonstrated complete congruence between gut microbiota and host phylogeny at order level, whereas Roeselers et al. (2011) and Li et al. (2017) observed similar patterns at species and even subspecies level. These studies suggest that host phylogeny may be a driver shaping gut microbiota. However, because evidence showed that gastrointestinal microbiota can be influenced by multiple factors, such as diet, geographic distribution, and physiology, the intention to predict the divergent histories of hosts seems less convincing, especially among affinis species merely relying on dissimilarities among gut microbiota. In addition, there were substantial variations between replicates when the community compositions were considered rather than community structure. Because unweighted UniFrac analysis is sensitive to rare taxa and weighted UniFrac analysis is sensitive to abundant taxa, it is possible that the differences in bacterial communities among host species are mainly induced by rare taxa (Koren et al., 2013).
Conclusion
This study provides the first investigation of fecal microbiota of Cervinae animals (including some threatened species) at different phylogenetic levels fed with same fodder. Results showed that Cervinae animals shared common fecal microbiota (e.g., Ruminococcaceae), but some bacterial genera (e.g., Sharpea and Succinivibrio) were associated only with particular digestion types. Because common and host-specific gastrointestinal microbiota were selected and maintained in Cervinae, the distance matrices of gastrointestinal microbiota and their hosts’ phylogenies were not completely congruent. To clarify the accurate relationship between gastrointestinal microbiota and their hosts’ phylogenies, a large sample size of both reared and wild populations with more information (e.g., gender, age, and body size) is needed.
Author Contributions
JL and XiL designed the research. JL, SZ, XuL, QL, and JJ contributed to experimental work. JL performed the data analysis and wrote the manuscript. JL and XiL revised the manuscript.
Funding
This work was supported by Sichuan Province Science and Technology Project (2017SZ0004), the 13th Five-year Informatization Plan of Chinese Academy of Sciences (XXH13503-03-106), Open Fund of Key Laboratory of Environmental and Applied Microbiology CAS (KLCAS-2017-3, KLCAS-2016-03), and China Biodiversity Observation Networks (Sino BON).
Conflict of Interest Statement
The authors declare that the research was conducted in the absence of any commercial or financial relationships that could be construed as a potential conflict of interest.
Supplementary Material
The Supplementary Material for this article can be found online at: https://www.frontiersin.org/articles/10.3389/fmicb.2018.01823/full#supplementary-material
FIGURE S1 | OTU-level rarefaction curves of Goods coverage across all samples.
FIGURE S2 | Co-occurrence patterns of core genera across the all host species (A) and within one host species (B–H), as determined by Spearman’s rank correlation analysis.
FIGURE S3 | UpsetR plot of functional prediction.
FIGURE S4 | Host cladogram.
TABLE S1 | Component of fodder.
TABLE S2 | Intraspecies core microbiota in Cervinae.
TABLE S3 | Pathways of functional prediction.
Footnotes
References
Arumugam, M., Raes, J., Pelletier, E., Le Paslier, D., Yamada, T., Mende, D. R., et al. (2011). Enterotypes of the human gut microbiome. Nature 473, 174. doi: 10.1038/nature09944
Aßhauer, K. P., Wemheuer, B., Daniel, R., and Meinicke, P. (2015). Tax4Fun: predicting functional profiles from metagenomic 16S rRNA data. Bioinformatics 31, 2882–2884. doi: 10.1093/bioinformatics/btv287
Bain, W. E., Bezuidenhout, L., Jopson, N. B., Pinares-Patiño, C. S., and McEwan, J. C. (2014). Rumen differences between sheep identified as being low or high emitters of greenhouse gas. Proc. Assoc. Adv. Anim. Breed. Genet. 20, 376–378.
Benson, A. K., Kelly, S. A., Legge, R., Ma, F., Low, S. J., Kim, J., et al. (2010). Individuality in gut microbiota composition is a complex polygenic trait shaped by multiple environmental and host genetic factors. Proc. Natl. Acad. Sci. U.S.A. 107, 18933–18938. doi: 10.1073/pnas.1007028107
Bletz, M. C., Perl, R. G. B., Bobowski, B. T. C., Japke, L. M., Tebbe, C. C., Dohrmann, A. B., et al. (2017). Amphibian skin microbiota exhibits temporal variation in community structure but stability of predicted Bd-inhibitory function. ISME J. 11, 1521–1534. doi: 10.1038/ismej.2017.41
Brooks, A. W., Kohl, K. D., Brucker, R. M., van Opstal, E. J., and Bordenstein, S. R. (2016). Phylosymbiosis: relationships and functional effects of microbial communities across host evolutionary history. PLoS Biol. 14:e2000225. doi: 10.1371/journal.pbio.2000225
Brucker, R. M., and Bordenstein, S. R. (2012a). The roles of host evolutionary relationships (genus: Nasonia) and development in structuring microbial communities. Evolution 66, 349–362. doi: 10.1111/j.1558-5646.2011.01454.x
Brucker, R. M., and Bordenstein, S. R. (2012b). Speciation by symbiosis. Trends Ecol. Evol. 27, 443–451. doi: 10.1016/j.tree.2012.03.011
Brucker, R. M., and Bordenstein, S. R. (2013). The hologenomic basis of speciation: gut bacteria cause hybrid lethality in the genus Nasonia. Science 341, 667–669. doi: 10.1126/science.1240659
Brulc, J. M., Antonopoulos, D. A., Miller, M. E., Wilson, M. K., Yannarell, A. C., Dinsdale, E. A., et al. (2009). Gene-centric metagenomics of the fiber-adherent bovine rumen microbiome reveals forage specific glycoside hydrolases. Proc. Natl. Acad. Sci. U.S.A. 106, 1948–1953. doi: 10.1073/pnas.0806191105
Burke, C., Steinberg, P., Rusch, D., Kjelleberg, S., and Thomas, T. (2011). Bacterial community assembly based on functional genes rather than species. Proc. Natl. Acad. Sci. U.S.A. 108, 14288–14293. doi: 10.1073/pnas.1101591108
Caporaso, J. G., Kuczynski, J., Stombaugh, J., Bittinger, K., Bushman, F. D., Costello, E. K., et al. (2010). QIIME allows analysis of high-throughput community sequencing data. Nat. Methods 7, 335–336. doi: 10.1038/nmeth.f.303
Carmody, R. N., Gerber, G. K., Luevano, J. M. Jr., Gatti, D. M., Somes, L., Svenson, K. L., et al. (2015). Diet dominates host genotype in shaping the murine gut microbiota. Cell Host Microbe 17, 72–84. doi: 10.1016/j.chom.2014.11.010
Chevalier, C., Stojanović, O., Colin, D. J., Suarez-Zamorano, N., Tarallo, V., Veyrat-Durebex, C., et al. (2015). Gut microbiota orchestrates energy homeostasis during cold. Cell 163, 1360–1374. doi: 10.1016/j.cell.2015.11.004
Conway, J. R., Lex, A., and Gehlenborg, N. (2017). UpSetR: an R package for the visualization of intersecting sets and their properties. Bioinformatics 33, 2938–2940. doi: 10.1093/bioinformatics/btx364
Dai, X., Tian, Y., Li, J., Su, X., Wang, X., Zhao, S., et al. (2014). Metatranscriptomic analyses of plant cell wall polysaccharide degradation by microorganisms in cow rumen. Appl. Environ. Microbiol. 81, 1375–1386. doi: 10.1128/AEM.03682-14
David, L. A., Maurice, C. F., Carmody, R. N., Gootenberg, D. B., Button, J. E., Wolfe, B. E., et al. (2014). Diet rapidly and reproducibly alters the human gut microbiome. Nature 505, 559–563. doi: 10.1038/nature12820
De Palma, G., Blennerhassett, P., Lu, J., Deng, Y., Park, A. J., Green, W., et al. (2015). Microbiota and host determinants of behavioural phenotype in maternally separated mice. Nat. Commun. 6:7735. doi: 10.1038/ncomms8735
Delgado, M. L., Singh, P., Funk, J. A., Moore, J. A., Cannell, E. M., Kanesfsky, J., et al. (2017). Intestinal microbial community dynamics of White-Tailed Deer (Odocoileus virginianus) in an Agroecosystem. Microb. Ecol. 74, 496–506. doi: 10.1007/s00248-017-0961-7
Delsuc, F., Metcalf, J. L., Wegener Parfrey, L., Song, S. J., González, A., and Knight, R. (2014). Convergence of gut microbiomes in myrmecophagous mammals. Mol. Ecol. 23, 1301–1317. doi: 10.1111/mec.12501
Dowd, S., Callaway, T., Wolcott, R., Sun, Y., McKeehan, T., Hagevoort, R., et al. (2008). Evaluation of the bacterial diversity in the feces of cattle using 16S rDNA bacterial tag-encoded FLX amplicon pyrosequencing (bTEFAP). BMC Microbiol. 8:125. doi: 10.1186/1471-2180-8-125
Durso, L. M., Harhay, G. P., Smith, T. P. L., Bono, J. L., DeSantis, T. Z., Harhay, D. M., et al. (2010). Animal-to-animal variation in fecal microbial diversity among beef cattle. Appl. Environ. Microbiol. 76, 4858–4862. doi: 10.1128/AEM.00207-10
Easson, C. G., and Thacker, R. W. (2014). Phylogenetic signal in the community structure of host-specific microbiomes of tropical marine sponges. Front. Microbiol. 5:532. doi: 10.3389/fmicb.2014.00532
Eckburg, P. B., Bik, E. M., Bernstein, C. N., Purdom, E., Dethlefsen, L., Sargent, M., et al. (2005). Diversity of the human intestinal microbial flora. Science 308, 1635–1638. doi: 10.1126/science.1110591
Edgar, R. C., Haas, B. J., Clemente, J. C., Quince, C., and Knight, R. (2011). UCHIME improves sensitivity and speed of chimera detection. Bioinformatics 27, 2194–2200. doi: 10.1093/bioinformatics/btr381
Faust, K., Sathirapongsasuti, J. F., Izard, J., Segata, N., Gevers, D., Raes, J., et al. (2012). Microbial co-occurrence relationships in the human microbiome. PLoS Comput. Biol. 8:e1002606. doi: 10.1371/journal.pcbi.1002606
Goopy, J. P., Donaldson, A., Hegarty, R., Vercoe, P. E., Haynes, F., Barnett, M., et al. (2014). Low-methane yield sheep have smaller rumens and shorter rumen retention time. Br. J. Nutr. 111, 578–585. doi: 10.1017/S0007114513002936
Groussin, M., Mazel, F., Sanders, J. G., Smillie, C. S., Lavergne, S., Thuiller, W., et al. (2017). Unraveling the processes shaping mammalian gut microbiomes over evolutionary time. Nat. Commun. 8:14319. doi: 10.1038/ncomms14319
Guan, Y., Yang, H., Han, S., Feng, L., Wang, T., and Ge, J. (2017). Comparison of the gut microbiota composition between wild and captive sika deer (Cervus nippon hortulorum) from feces by high-throughput sequencing. AMB Express 7:212. doi: 10.1186/s13568-017-0517-8
Guo, W., Li, Y., Wang, L., Wang, J., Xu, Q., Yan, T., et al. (2015). Evaluation of composition and individual variability of rumen microbiota in yaks by 16S rRNA high-throughput sequencing technology. Anaerobe 34, 74–79. doi: 10.1016/j.anaerobe.2015.04.010
Hackmann, T. J., and Spain, J. N. (2010). Invited review: ruminant ecology and evolution: perspectives useful to ruminant livestock research and production. J. Dairy Sci. 93, 1320–1334. doi: 10.3168/jds.2009-2071
Henderson, G., Cox, F., Ganesh, S., Jonker, A., Young, W., Janssen, P. H., et al. (2015). Rumen microbial community composition varies with diet and host, but a core microbiome is found across a wide geographical range. Sci. Rep. 5, 1–13. doi: 10.1038/srep14567
Hernandez-Sanabria, E., Goonewardene, L. A., Wang, Z., Durunna, O. N., and Moore, S. S. (2012). Impact of feed efficiency and diet on adaptive variations in the bacterial community in the rumen fluid of cattle. Appl. Environ. Microbiol. 78, 1203–1214. doi: 10.1128/AEM.05114-11
Hillis, D. M., and Bull, J. J. (1993). An empirical test of bootstrapping as a method for assessing confidence in phylogenetic analysis. Syst. Biol. 42, 182–192. doi: 10.1093/sysbio/42.2.182
Hofmann, R. R. (1989). Evolutionary steps of ecophysiological adaptation and diversifcation of ruminants: a comparative view of their digestive system. Oecologia 78, 443–457. doi: 10.1007/BF00378733
Hu, X., Liu, G., Shafer, A. B. A., Wei, Y., Zhou, J., Lin, S., et al. (2017). Comparative analysis of the gut microbial communities in forest and alpine musk deer using high-throughput sequencing. Front. Microbiol. 8:572. doi: 10.3389/fmicb.2017.00572
Ishaq, S. L., and Wright, A. D. G. (2012). Insight into the bacterial gut microbiome of the North American moose (Alces alces). BMC Microbiol. 12:212. doi: 10.1186/1471-2180-12-212
Jami, E., Israel, A., Kotser, A., and Mizrahi, I. (2013). Exploring the bovine rumen bacterial community from birth to adulthood. ISME J. 7, 1069–1079. doi: 10.1038/ismej.2013.2
Jami, E., and Mizrahi, I. (2012). Composition and similarity of bovine rumen microbiota across individual animals. PLoS ONE 7:e33306. doi: 10.1371/journal.pone.0033306
Kamke, J., Kittelmann, S., Soni, P., Li, Y., Tavendale, M., Ganesh, S., et al. (2016). Rumen metagenome and metatranscriptome analyses of low methane yield sheep reveals a Sharpea-enriched microbiome characterised by lactic acid formation and utilisation. Microbiome 4, 1–16. doi: 10.1186/s40168-016-0201-2
Karasov, W. H., and Douglas, A. E. (2013). Comparative digestive physiology. Compr. Physiol. 3, 741–783. doi: 10.1002/cphy.c110054
Kim, M., Morrison, M., and Yu, Z. (2011). Phylogenetic diversity of bacterial communities in bovine rumen as affected by diets and microenvironments. Folia Microbiol. 56, 453–458. doi: 10.1007/s12223-011-0066-5
Kittelmann, S., Pinares-Patiño, C. S., Seedorf, H., Kirk, M. R., Ganesh, S., McEwan, J. C., et al. (2014). Two different bacterial community types are linked with the low-methane emission trait in sheep. PLoS One 9:e103171. doi: 10.1371/journal.pone.0103171
Kittelmann, S., Seedorf, H., Walters, W. A., Clemente, J. C., Knight, R., Gordon, J. I., et al. (2013). Simultaneous amplicon sequencing to explore co-occurrence patterns of bacterial, archaeal and eukaryotic microorganisms in rumen microbial communities. PLoS One 8:e47879. doi: 10.1371/journal.pone.0047879
Kohl, K. D., and Dearing, M. D. (2016). The woodrat gut microbiota as an experimental system for understanding microbial metabolism of dietary toxins. Front. Microbiol. 7:1165. doi: 10.3389/fmicb.2016.01165
Kohl, K. D., Dearing, M. D., and Bordenstein, S. R. (2018a). Microbial communities exhibit host species distinguishability and phylosymbiosis along the length of the gastrointestinal tract. Mol. Ecol. 27, 1874–1883. doi: 10.1111/mec.14460
Kohl, K. D., Varner, J., Wilkening, J. L., and Dearing, M. D. (2018b). Gut microbial communities of American pikas (O chotona princeps): evidence for phylosymbiosis and adaptations to novel diets. J. Anim. Ecol. 87, 323–330. doi: 10.1111/1365-2656.12692
Kohl, K. D., Weiss, R. B., Cox, J., Dale, C., and Dearing, M. D. (2014). Gut microbes of mammalian herbivores facilitate intake of plant toxins. Ecol. Lett. 17, 1238–1246. doi: 10.1111/ele.12329
Kong, Y., Teather, R., and Forster, R. (2010). Composition, spatial distribution, and diversity of the bacterial communities in the rumen of cows fed different forages. FEMS Microbiol. Ecol. 74, 612–622. doi: 10.1111/j.1574-6941.2010.00977.x
Koren, O., Goodrich, J. K., Cullender, T. C., Spor, A. A., Laitinen, K., Backhed, H. K., et al. (2013). During Pregnancy. Cell 150, 470–480. doi: 10.1016/j.cell.2012.07.008
Larkin, M. A., Blackshields, G., Brown, N. P., Chenna, R., Mcgettigan, P. A., McWilliam, H., et al. (2007). Clustal W and Clustal X version 2.0. Bioinformatics 23, 2947–2948. doi: 10.1093/bioinformatics/btm404
Leatherwood, J. M. (1965). Cellulase from Ruminococcus albus and mixed rumen microorganisms. Appl. Environ. Microbiol. 13, 771–775.
LeBlanc, J. G., Milani, C., de Giori, G. S., Sesma, F., Van Sinderen, D., and Ventura, M. (2013). Bacteria as vitamin suppliers to their host: a gut microbiota perspective. Curr. Opin. Biotechnol. 24, 160–168. doi: 10.1016/j.copbio.2012.08.005
Ley, R. E., Bäckhed, F., Turnbaugh, P., Lozupone, C. A., Knight, R. D., and Gordon, J. I. (2005). Obesity alters gut microbial ecology. Proc. Natl. Acad. Sci. U.S.A. 102, 11070–11075. doi: 10.1073/pnas.0504978102
Ley, R. E., Hamady, M., Lozupone, C., Turnbaugh, P. J., Ramey, R. R., Bircher, J. S., et al. (2008). Evolution of mammals and their gut microbes. Science 320, 1647–1651. doi: 10.1126/science.1155725
Ley, R. E., Peterson, D. A., and Gordon, J. I. (2006). Ecological and evolutionary forces shaping microbial diversity in the human intestine. Cell 124, 837–848. doi: 10.1016/j.cell.2006.02.017
Li, H., Qu, J., Li, T., Yao, M., Li, J., and Li, X. (2017). Gut microbiota may predict host divergence time during Glires evolution. FEMS Microbiol. Ecol. 93, 1–9. doi: 10.1093/femsec/fix009
Li, W., and Godzik, A. (2006). Cd-hit: a fast program for clustering and comparing large sets of protein or nucleotide sequences. Bioinformatics 22, 1658–1659. doi: 10.1093/bioinformatics/btl158
Li, Z., Zhang, Z., Xu, C., Zhao, J., Liu, H., Fan, Z., et al. (2014). Bacteria and methanogens differ along the gastrointestinal tract of Chinese roe deer (Capreolus pygargus). PLoS One 9:e114513. doi: 10.1371/journal.pone.0114513
Louca, S., Jacques, S. M., Pires, A. P., Leal, J. S., Srivastava, D. S., Parfrey, L. W., et al. (2016). High taxonomic variability despite stable functional structure across microbial communities. Nat. Ecol. Evol. 1:15. doi: 10.1038/s41559-016-0015
Lozupone, C., and Knight, R. (2005). UNIFRAC: a new phylogenetic method for comparing microbial communities. Appl. Environ. Microbiol. 71, 8228–8235. doi: 10.1128/AEM.71.12.8228-8235.2005
Lozupone, C. A., Stombaugh, J. I., Gordon, J. I., Jansson, J. K., and Knight, R. (2012). Diversity, stability and resilience of the human gut microbiota. Nature 489, 220–230. doi: 10.1038/nature11550
Magoc, T., and Salzberg, S. L. (2011). FLASH: fast length adjustment of short reads to improve genome assemblies. Bioinformatics 27, 2957–2963. doi: 10.1093/bioinformatics/btr507
Martin, T. E., and Maron, J. L. (2012). Climate impacts on bird and plant communities from altered animal-plant interactions. Nat. Climate Change 2, 195–200. doi: 10.1038/nclimate1348
Mattioli, S. (2011). “Family Cervidae (Deer),” in Handbook of the mammals of the world, Vol. 2, eds D. E. Wilson and R. A. Mittermeier (Barcelona: Lynx Edicion), 886.
Miyake, S., Ngugi, D. K., and Stingl, U. (2015). Diet strongly influences the gut microbiota of surgeonfishes. Mol. Ecol. 24, 656–672. doi: 10.1111/mec.13050
Moeller, A. H., Li, Y., Ngole, E. M., Ahuka-Mundeke, S., Lonsdorf, E. V., Pusey, A. E., et al. (2014). Rapid changes in the gut microbiome during human evolution. Proc. Natl. Acad. Sci. U.S.A. 111, 16431–16435. doi: 10.1073/pnas.1419136111
Muegge, B. D., Kuczynski, J., Knights, D., Clemente, J. C., González, A., Fontana, L., et al. (2011). Diet drives convergence in gut microbiome functions across mammalian phylogeny and within humans. Science 332, 970–974. doi: 10.1126/science.1198719
Nelson, T. A., Holmes, S., Alekseyenko, A. V., Shenoy, M., Desantis, T., Wu, C. H., et al. (2011). PhyloChip microarray analysis reveals altered gastrointestinal microbial communities in a rat model of colonic hypersensitivity. Neurogastroenterol. Motil. 23:169. doi: 10.1111/j.1365-2982.2010.01637.x
Neumann, A. P., McCormick, C. A., and Suen, G. (2017). Fibrobacter communities in the gastrointestinal tracts of diverse hindgut-fermenting herbivores are distinct from those of the rumen. Environ. Microbiol. 19, 3768–3783 doi: 10.1111/1462-2920.13878
Nie, Y., Luo, F., and Lin, Q. (2018). Dietary nutrition and gut microflora: a promising target for treating diseases. Trends Food Sci. Technol. 75, 72–80. doi: 10.1016/j.tifs.2018.03.002
Ochman, H., Worobey, M., Kuo, C. H., Ndjango, J. B. N., Peeters, M., Hahn, B. H., et al. (2010). Evolutionary relationships of wild hominids recapitulated by gut microbial communities. PLoS Biol. 8:e1000546. doi: 10.1371/journal.pbio.1000546
O’Donnell, M. M., Harris, H., Ross, R. P., and O’Toole, P. W. (2017). Core fecal microbiota of domesticated herbivorous ruminant, hindgut fermenters, and monogastric animals. Microbiologyopen 6:e00509. doi: 10.1002/mbo3.509
Phillips, C. D., Phelan, G., Dowd, S. E., McDonough, M. M., Ferguson, A. W., Delton Hanson, J., et al. (2012). Microbiome analysis among bats describes influences of host phylogeny, life history, physiology and geography. Mol. Ecol. 21, 2617–2627. doi: 10.1111/j.1365-294X.2012.05568.x
Pitta, D. W., Kumar, S., Veiccharelli, B., Parmar, N., Reddy, B., and Joshi, C. G. (2014a). Bacterial diversity associated with feeding dry forage at different dietary concentrations in the rumen contents of Mehshana buffalo (Bubalus bubalis) using 16S pyrotags. Anaerobe 25, 31–41. doi: 10.1016/j.anaerobe.2013.11.008
Pitta, D. W., Kumar, S., Vecchiarelli, B., Shirley, D. J., Bittinger, K., Baker, L. D., et al. (2014b). Temporal dynamics in the ruminal microbiome of dairy cows during the transition period. Jb. Anim. Sci. 92, 4014–4022. doi: 10.2527/jas.2014-7621
Pitta, D. W., Pinchak, W. E., Dowd, S. E., Osterstock, J., Gontcharova, V., Youn, E., et al. (2010). Rumen bacterial diversity dynamics associated with changing from bermudagrass hay to grazed winter wheat diets. Microb. Ecol. 59, 511–522. doi: 10.1007/s00248-009-9609-6
Pope, P. B., Denman, S. E., Jones, M., Tringe, S. G., Barry, K., Malfatti, S. A., et al. (2010). Adaptation to herbivory by the Tammar wallaby includes bacterial and glycoside hydrolase profiles different from other herbivores. Proc. Natl. Acad. Sci. U.S.A. 107, 14793–14798. doi: 10.1073/pnas.1005297107
Pope, P. B., Mackenzie, A. K., Gregor, I., Smith, W., Sundset, M. A., McHardy, A. C., et al. (2012). Metagenomics of the Svalbard reindeer rumen microbiome reveals abundance of polysaccharide utilization loci. PLoS One 7:e38571. doi: 10.1371/journal.pone.0038571
Price, S. A., Hopkins, S. S. B., Smith, K. K., and Roth, V. L. (2012). Tempo of trophic evolution and its impact on mammalian diversification. Proc Natl Acad Sci U.S.A. 109, 7008–7012. doi: 10.1073/pnas.1117133109
Purahong, W., Schloter, M., Pecyna, M. J., Kapturska, D., Däumlich, V., Mital, S., et al. (2014). Uncoupling of microbial community structure and function in decomposing litter across beech forest ecosystems in Central Europe. Sci. Rep. 4:7014. doi: 10.1038/srep07014
Rawls, J. F. J., Mahowald, M. A. M., Ley, R. E. R., and Gordon, J. I. J. (2006). Reciprocal gut microbiota transplants from zebrafish and mice to germ-free recipients reveal host habitat selection. Cell 127, 423–433. doi: 10.1016/j.cell.2006.08.043
Robert, W. (2012). The bacterial community composition of the bovine rumen detected using pyrosequencing of 16S rRNA genes. Metagenomics 1, 235571.
Roeselers, G., Mittge, E. K., Stephens, W. Z., Parichy, D. M., Cavanaugh, C. M., Guillemin, K., et al. (2011). Evidence for a core gut microbiota in the zebrafish. ISME J. 5, 1595. doi: 10.1038/ismej.2011.38
Ronquist, F., and Huelsenbeck, J. P. (2003). MrBayes 3: bayesian phylogenetic inference under mixed models. Bioinformatics 19, 1572–1574. doi: 10.1093/bioinformatics/btg180
Ronquist, F., Teslenko, M., Van Der Mark, P., Ayres, D. L., Darling, A., Höhna, S., et al. (2012). MrBayes 3.2: efficient Bayesian phylogenetic inference and model choice across a large model space. Syst. Biol. 61, 539–542. doi: 10.1093/sysbio/sys029
Salter, S. J., Cox, M. J., Turek, E. M., Calus, S. T., Cookson, W. O., Moffatt, M. F., et al. (2014). Reagent and laboratory contamination can critically impact sequence-based microbiome analyses. BMC Biol. 12:87. doi: 10.1186/s12915-014-0087-z
Sanders, J. G., Powell, S., Kronauer, D. J., Vasconcelos, H. L., Frederickson, M. E., and Pierce, N. E. (2014). Stability and phylogenetic correlation in gut microbiota: lessons from ants and apes. Mol. Ecol. 23, 1268–1283. doi: 10.1111/mec.12611
Silvestro, D., and Michalak, I. (2012). raxmlGUI: a graphical front-end for RAxML. Organ. Div. Evol. 12, 335–337. doi: 10.1007/s13127-011-0056-0
Smith, C. C. R., Snowberg, L. K., Gregory Caporaso, J., Knight, R., and Bolnick, D. I. (2015). Dietary input of microbes and host genetic variation shape among-population differences in stickleback gut microbiota. ISME J. 9, 2515–2526. doi: 10.1038/ismej.2015.64
Tamaki, H., Wright, C. L., Li, X., Lin, Q., Hwang, C., Wang, S., et al. (2011). Analysis of 16S rRNA amplicon sequencing options on the Roche/454 next-generation titanium sequencing platform. PLoS One 6:e25263. doi: 10.1371/journal.pone.0025263
Tang, M. T., Han, H., Yu, Z., Tsuruta, T., and Nishino, N. (2017). Variability, stability, and resilience of fecal microbiota in dairy cows fed whole crop corn silage. Appl. Microbiol. Biotechnol. 101, 6355–6364. doi: 10.1007/s00253-017-8348-8
Tremaroli, V., and Bäckhed, F. (2012). Functional interactions between the gut microbiota and host metabolism. Nature 489:242. doi: 10.1038/nature11552
Warnecke, F., Luginbu, P., Ivanova, N., Ghassemian, M., Richardson, T. H., Stege, J. T., et al. (2007). Metagenomic and functional analysis of hindgut microbiota of a wood-feeding higher termite. Nature 450, 560–565. doi: 10.1038/nature06269
Weimer, P. J. (1993). Effects of dilution rate and pH on the ruminal cellulolytic bacterium Fibrobacter succinogenes S85 in cellulose-fed continuous culture. Arch. Microbiol. 160, 288–294. doi: 10.1007/BF00292079
Keywords: Cervinae, fecal microbiota, species specificity, host divergence, phylosymbiosis
Citation: Li J, Zhan S, Liu X, Lin Q, Jiang J and Li X (2018) Divergence of Fecal Microbiota and Their Associations With Host Phylogeny in Cervinae. Front. Microbiol. 9:1823. doi: 10.3389/fmicb.2018.01823
Received: 23 April 2018; Accepted: 23 July 2018;
Published: 30 August 2018.
Edited by:
Malka Halpern, University of Haifa, IsraelReviewed by:
Kevin R. Theis, Wayne State University School of Medicine, United StatesGil Sharon, California Institute of Technology, United States
Seth Bordenstein, Vanderbilt University, United States
Copyright © 2018 Li, Zhan, Liu, Lin, Jiang and Li. This is an open-access article distributed under the terms of the Creative Commons Attribution License (CC BY). The use, distribution or reproduction in other forums is permitted, provided the original author(s) and the copyright owner(s) are credited and that the original publication in this journal is cited, in accordance with accepted academic practice. No use, distribution or reproduction is permitted which does not comply with these terms.
*Correspondence: Xiangzhen Li, bGl4ekBjaWIuYWMuY24=