- Microbiological Sciences Branch, Northeast Food and Feed Laboratory, United States Food & Drug Administration, Jamaica, NY, United States
Until recently, traditional serology and the Kauffmann White Scheme (KWS) have been the gold standard for Salmonella serotyping. Whole Genome Sequencing (WGS) has now emerged as an alternative in this field. Serotype information remains a cornerstone in food safety and public health activities to reduce the burden of salmonellosis. At the same time, recent advances in WGS have improved the ability to perform advanced pathogen characterization while improving trace back investigations to determine the source of foodborne illness during outbreaks. Serovar prediction based on WGS can be performed using in silico data analysis tools. Three such tools have been developed: (a). Salmonella in silico Typing Resource (SISTR), (b). SeqSero, and (c). in silico 7-gene MLST ST (Multilocus Sequence Typing Sub-Typing) which was generated using the SISTR platform. Public health officials around the world are diligently working to validate these tools for replacing traditional surveillance methods to provide a more powerful approach for molecular epidemiology in support of public health investigations. In this study, we report a retrospective analysis of our laboratory inventory of 1,041 Salmonella isolates collected between 1999 and 2017. These isolates are of public health significance since they all came from either food, feed or environmental swabs. They were all serotyped by both traditional serology and WGS using an in silico SeqSero tool for serovar prediction. Both predicted identical Salmonella serotypes in 899 isolates (86.4% of the 1,041 Salmonella isolates). SeqSero assignments differed from traditional serological testing in 80 isolates (7.7%) and no serotype prediction was ascertained from 62 isolates (5.9%). This retrospective study is an excellent example of using WGS and SeqSero as a data analysis tool to predict Salmonella serotypes that can provide numerous advantages including molecular and genetic details regarding the characteristics of the Salmonella isolates compared to traditional KWS serotyping. In conclusion, it is evident that using WGS and in silico tools for Salmonella serotyping might someday replace traditional serotyping.
Introduction
Salmonella was first visualized in 1880 by Karl Eberth. In 1884 George Theodor Gaffky successfully grew the pathogen in pure culture and in 1900 Joseph Leon Lignières proposed that the pathogen discovered by Daniel Salmon's group in 1885 be called Salmonella in his honor (Heymann et al., 2006). Salmonella has been known to cause 1.2 million foodborne illnesses annually in the United States with 23,000 hospitalizations and 450 deaths including many multistate outbreaks (CDC, 2018).
Although national surveillance data for Salmonella has been collected through laboratory-based surveillance systems based on serotype designation for over 50 years (Grimont and Weill, 2007), improvements in this surveillance system is desperately needed. It is crucial for public health and regulatory agencies to have more rapid, highly accurate, and discriminatory genomic and metagenomic serotyping methods to detect outbreaks and to link cases of illness to the originating source (Forbes et al., 2017).
Traditional Salmonella serotyping, according to the Kauffmann White Scheme (KWS) was developed in 1926 and has been used ever since as a proxy for genetics. It is maintained by the World Health Organization (WHO) Collaborating Centre for Reference and Research on Salmonella, located at the Pasteur Institute in Paris, France. The current (9th) edition issued in 2007 comprises antigenic variants that had been validated as of January 1, 2007. Currently there are 2,610 recognized Salmonella serovars (Dieckmann and Malorny, 2011).
The U.S. National Salmonella Surveillance System has been built upon serotyping in public health laboratories, a subtyping method performed through the agglutination of Salmonella cells with specific antisera that detect lipopolysaccharide O antigen and flagellar H antigens. Specific combinations of O and H antigenic types represent distinct serotypes or serovars (Samuel and Reeves, 2003).
Serovars are distinctive groups within a single species of microorganisms as bacteria and viruses which share common distinctive surface structures. In Salmonella, these surface structures are the O and H antigens. The O antigens are made of lipopolysaccharides which differ in their chemical constitution. The H antigens are slender threadlike structures which are parts of the flagella and are different in their protein content. Each O and H antigen has a unique code number and the scientists use their combinations to classify Salmonella bacteria that look similar under the microscope into many serotypes. Some serotypes are only found in one kind of animal or in a single place. Others can be found in many different animals and all over the world. Some can cause severe illness while others may only cause mild illness when they infect people. Public health scientists use serotyping to detect Salmonella outbreaks and to track them to their sources. All clinical strains of Salmonella are subtyped using molecular tools as well as being serotyped and when one molecular subtype increases above normal occurrence, an outbreak is suspected (CDC, 2015).
Molecular assays for serotyping of bacterial isolates are often developed on the Luminex platform to determine serotypes based on DNA markers within genes responsible for O and H antigen expression (Vanegas and Joys, 1995; Fitzgerald et al., 2007). The Luminex xMAP technology allows detection of nucleic acids by combining PCR with a multiplexed bead-based detection system. The antigen that is encoded by the PCR fragment is identified by hybridizing with antigen specific-DNA probes. Although it is fairly rapid and not prohibitively expensive when one is looking at specific or common serotypes, the one current limiting factor of this technology is that the molecular serotyping does not detect all serotype antigens and focuses primarily on the most common serotypes reported for human clinical specimens (McQuiston et al., 2004, 2011).
Next generation sequencing technology provides lots of information about species, serovar, virulence, pathogenicity, antimicrobial resistance, and subtype of bacteria in just one sequencing test. WGS can generate high-quality sequence data in public health laboratories with better identification of clinical strains relating them to outbreak strains with better determination of virulence and antimicrobial-resistance genes. WGS has already dramatically changed the field of genomics and could enable researchers to study gene expression by sequencing RNA and examining host-pathogen interactions (Oakeson et al., 2017).
WGS can also be used to identify the path of disease transmission within a population and provide information on the probable source. It is essential for mutation detection and in understanding of genetics of Salmonella and other microorganisms. It can also evaluate the evolution of strains during an outbreak and detect contextual data on the genetic interrelatedness (Gilchrist et al., 2015). A major application for WGS is to identify outbreak clusters and efficiently infer phylogenies from the sequencing reads (Ahrenfeldt et al., 2017).
WGS is used now as an alternative technique for obtaining fast and reliable serotype information (Gymoese et al., 2017). In this study we evaluate the potential use of WGS to serve as the sole method for the routine serotyping of Salmonella isolates. This approach offers a rapid identification of Salmonella serotypes as well as, identifying an array of single nucleotide polymorphisms (SNP) within the genome. These SNPs can be used to investigate the epidemiology of an outbreak to link human cases of illness to the point source of contamination and differentiate between outbreak-related and unrelated sporadic clinical cases (Lienau et al., 2011). WGS also has the potential to provide researchers and clinicians with additional information regarding antibiotic resistance markers and virulence factors to better understand serotypes and to quickly identify, and investigate outbreaks while providing trace-back and trace-forward information (Inns et al., 2015; Taylor et al., 2015).
WGS is rapidly replacing current molecular subtyping methods for surveillance and for foodborne outbreak purposes (Bakker et al., 2011; Leekitcharoenphon et al., 2014). It enables high-resolution molecular subtyping and provides valuable additional data regarding further characterization of emerging clones based on genetic differences and evolutionary studies (Bale et al., 2016; Bekal et al., 2016). This information is critical during any outbreak response for gathering clonal information in outbreak investigations. Several studies have shown that WGS-based typing has an enhanced discriminatory power compared to current molecular typing methods used for Salmonella (Koser et al., 2012; Deng et al., 2015). Public health microbiology is currently being transformed by next generation sequencing, which opens the door to more rapid serotype determination using WGS data. SeqSero (www.denglab.info/SeqSero) is a novel web-based tool for determining Salmonella serotypes using whole genome sequencing data (Zhang et al., 2015).
Materials and Methods
Study Design
A total of 1,041 Salmonella isolates from foods, feed and environmental samples were derived from our laboratory inventory between 1999 and 2017. All isolates were previously serotyped using traditional serology as well as having their genome sequenced. We used the online tool, SeqSero to evaluate whether traditional serology could be replaced with WGS and such online tool.
Metadata was collected for each Salmonella isolate which included a WGS accession ID number, an FDA tracking number, a date that our laboratory recorded the result in our internal database, and the date when the traditional serology was uploaded within our internal database. The metadata also included the results summary, traditional serotyping results, the WGS run date, flow cell bar code number, base calls and the predicted Salmonella serotype for every isolate.
Genome Sequencing
WGS analysis starts by extraction of genomic deoxyribonucleic acid (DNA) with a fully automated Qiagen QIAcube™ from 1 mL of bacterial culture grown overnight in Brain Heart Infusion (BHI) broth at 35°C as per the FDA GenomeTrakr protocol. DNA is quantitated, fragmented and tagged with adapter sequences added to the ends (Nextera XT Library Prep Kit, Illumina, Inc.). The library is amplified by PCR followed by cleanup and size selection using AMPure XP™ (Beckman Coulter, Inc.) beads to remove very short library fragments from the sample. Library normalization is performed using the Nextera kit to ensure equal library representation in the pooled sample. The pooled amplicon library (PAL) is performed by combining equal volumes of normalized library, quantitated, and diluted in hybridization buffer forming a diluted amplicon library (DAL). This DAL is heat denatured and loaded onto the MiSeq reagent cartridge for sequencing1.
Serovar Prediction From WGS Using in silico SeqSero Tool
SeqSero, was used to determine the serotype of the 1,041 Salmonella isolates. Paired reads of WGS raw data (fastq files) were uploaded to the online SeqSero tool version 1.0. The data input and output to and from SeqSero database predicted the Salmonella serotype of the requested isolate within a few minutes.
Interpretation of Results
In order to calculate the number of days it took to complete the traditional serotyping, we subtracted the date on which our laboratory recorded the result in the FDA tracking database from the date of traditional serology results uploaded in the FDA tracking database for each isolate. We then reported the mean number of days it took to achieve traditional serology.
The results from the traditional Salmonella serotyping and from the WGS serotyping using SeqSero tool were analyzed for their differences and similarities, as well as their antigenic nomenclature.
Results
Description of Entire Cohort
We performed whole genome sequencing on 1,041 Salmonella isolates from our laboratory stock inventory and used the SeqSero database for predicting the serotype. SeqSero could not assign 62 isolates and the other 979 isolates contained 160 different Salmonella serovars, of which, S. Weltevreden was the most predicted serotype being assigned to 113 isolates of the 979 isolates (11.54%). Second in frequency was S. Typhimurium in 62 isolates (6.33%). S. Virchow came next being predicted in 50 isolates (5.11%). S. Senftenberg or S. Dessau which share the same general formula: 1,3,19:g,s,t:- was predicted in 45 isolates (4.6%). S. Tananarive and S. Brunei which share the same general formula: 8:y:1,5 were predicted in 32 isolates (3.27%). S. Infantis was found in 31 isolates (3.17%). S. Kentucky and S. Newport came next in frequency, each being predicted in 30 isolates (3.06%). S. Bardo was reported by SeqSero to share the same general formula as S. Newport, however, Bardo is very rare. S. Mbandka came next in frequency and was predicted in 29 isolates (2.96%). S. Paratyphi B or S. potential monophasic variant of Paratyphi B was predicted in 27 isolates (2.76%). Supplementary Table 1 shows all 160 serovars and their abundance.
In comparing the predicted serotypes based on WGS and SeqSero tool against the traditional Salmonella serology (KWS), both SeqSero (WGS) and traditional serotyping predicted identical Salmonella serotypes in 899 isolates (86.4% of the 1,041 Salmonella isolates). No serotype prediction was ascertained from 62 isolates (5.9% of the 1,041 Salmonella isolates) where SeqSero's result was “N/A (The predicted antigenic profile does not exist in the White-Kauffmann-LE Minor Scheme)” as shown in Supplementary Table 2. They will decrease overtime as more whole genomes are added to the Salmonella national database (NCBI). SeqSero assignments differed from traditional serological testing in 80 isolates (7.7% of the 1,041 Salmonella isolates) as shown in Table 1.
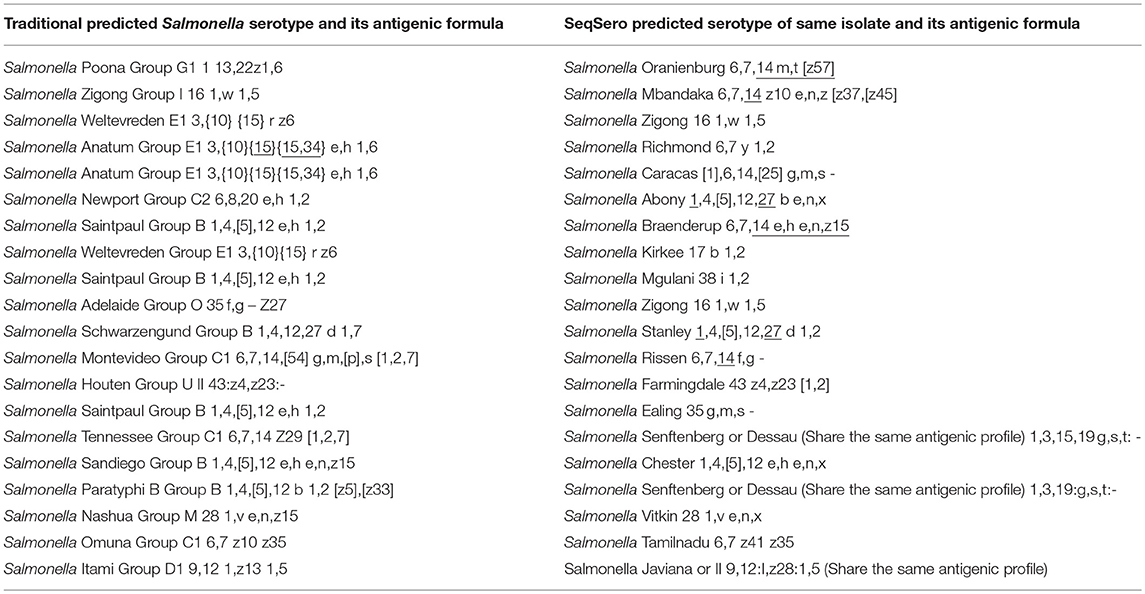
Table 1. Examples of Salmonella strains with different outcomes between traditional serotyping and SeqSero prediction.
SeqSero Could Predict 36 Isolates When Traditional Serotyping Could Not
Among the 899 isolates, 36 isolates were only predicted as monophasic, diphasic or non-motile by traditional serology without any further speciation, while SeqSero analysis provided the strain name of these same isolates. For example an isolate assigned as Salmonella Saintpaul by SeqSero, was only serotyped as Salmonella Monophasic Group B by traditional Salmonella serology. Another example was an isolate serotyped as Salmonella Monophasic Group C1 by traditional serology but assigned as Salmonella Virchow by SeqSero, as shown in Table 2.
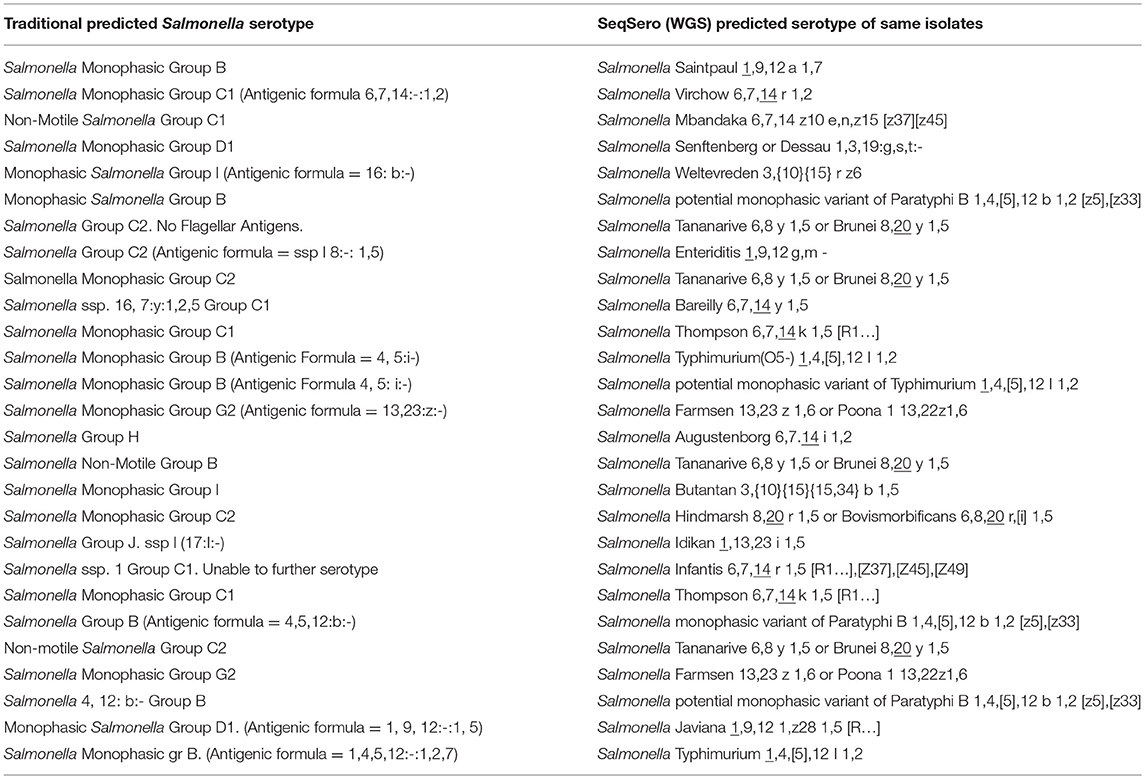
Table 2. Examples of isolates in which traditional serology predicted monophasic or non-motile Salmonella while WGS was able to predict Salmonella serotypes as nominated in KWS.
SeqSero Provided More Molecular Details Regarding Salmonella Typhimurium
Traditional serology predicted it as Salmonella Typhimurium in 62 isolates, while SeqSero was able to further detect the absence of the O5 epitope in 20 of these isolates.
SeqSero Assigned Two Possible Serotypes Sharing the Same Antigenic Profile
SeqSero analysis of 105 isolates provided two possible serotypes sharing the same antigenic profile (or formula) but differed in minor O antigenic factors. Traditional serotyping predicted only one serotype from each of these isolates which was in agreement with one of the two SeqSero calls. For example, traditional serology predicted some isolates as Salmonella Brunei and these same isolates were assigned by SeqSero as Salmonella Tananarive or Brunei since both share the same general formula of “8:y:1,5.” Other isolates which were predicted as Salmonella Senftenberg by traditional serotyping, were assigned as Salmonella Senftenberg or Dessau by SeqSero because both share the same general formula “1,3,19:g,s,t:-” as shown in Table 3.
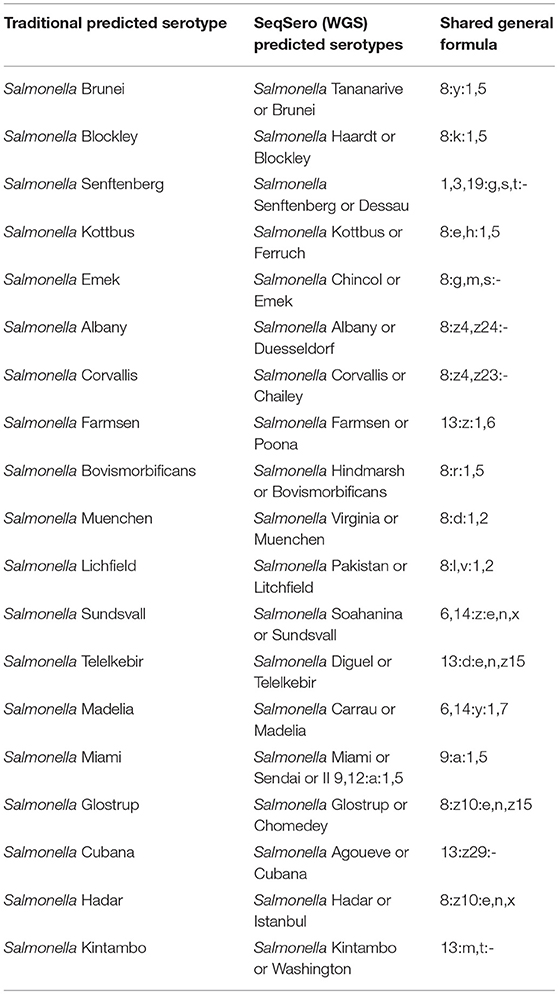
Table 3. Examples of Salmonella strains sharing the same general formula as assigned by SeqSero vs. traditional serology, which predicted only one serotype.
Traditional serotyping determined some isolates to be Salmonella Newport and although SeqSero assigned them as the same designation, SeqSero also noted that Salmonella Bardo shares an identical antigenic profile however Bardo is an exceedingly rare serotype across the globe (Gupta et al., 2016).
Discussion
Limitations of Traditional Serotyping
The cost of performing traditional serotyping can be exorbitant. The price of 3 ml antisera vial varies from $87 to $267, depending on the type of poly group. For instance, in 2017 NFFL spent $4,000 to purchase poly A to poly G antisera for preliminary serotyping that is required for identification and confirmation of Salmonella isolates. Then, these isolates were shipped to a central laboratory where serovar (phenotype) identification was performed. The weekly shipping cost was $350, this rate remained the same regardless of the number of isolates in the shipping container. Overall, the budget required for traditional Salmonella serotyping including shipping was ~$20,000 with increasing costs each year.
The phenotypic determination of isolates in the central laboratory is labor-intensive and time-consuming with a turnaround time between 1 and 3 weeks depending on the isolate being processed. Some isolates require several passes through semi-solid media to enhance motility and flagellar antigenic expression (CDC, 2015) and some do not express serotype antigens due to a single nucleotide change in the genome (Li et al., 2017) thus, limiting the utility of traditional serotyping. Traditional serotyping requires a large number of tubes and slides to complete the whole process, which requires large laboratory space and may compromise quality control, as maintenance of all the necessary reagents can be difficult when managing a full array of antisera.
Using SeqSero Tool to Predict Salmonella Serotypes
WGS has been recently used in the field of Salmonella subtyping and may ultimately prove to be more reliable and efficient. SeqSero is a web-based tool, which can predict most Salmonella serotypes using high-throughput genome sequencing data based on the databases of Salmonella serotype determinants. SeqSero extracts the relevant genomic regions, specifically the rfb gene cluster for somatic antigen determination and the fliC and fljB alleles for the H1 and H2 antigens, from the genome assemblies and aligns these regions to curated databases. The performance of SeqSero was evaluated by testing (i) raw reads from genomes of 308 Salmonella isolates of known serotype; (ii) raw reads from genomes of 3,306 Salmonella isolates sequenced and made publicly available by GenomeTrakr, a U.S. national monitoring network operated by the Food and Drug Administration; and (iii) 354 other publicly available draft or complete Salmonella genomes. Salmonella serotype determination from raw sequencing reads of fecal metagenomes from mice which were orally infected with this pathogen were also demonstrated (Zhang et al., 2015).
Limitations of Whole Genome Sequencing
Further considerations must also be given to the costs associated with sequencing, the technical and informational capacities of national and partner laboratories, turnaround times associated with the batching of isolates, and data sharing models to improve the flow of information between various partners (Alkan et al., 2011).
SeqSero gave some incorrect results due to incorrect calling of various antigenic determinants, especially in regards to closely related serovars, such as those that differ on the basis of flagellar antigens of the g-complex. SeqSero analysis of some isolates provided two possible serotypes sharing the same antigenic profile (or formula) but differed on minor O antigenic factors. SeqSero could not predict some isolates. These unpredicted serovars are either rare Salmonella isolates or possibly there were some gaps within the SeqSero database.
Advantages of Using SeqSero Tool For Serovar Prediction
Although in silico serotyping may have some limitations, our study shows that whole genome sequencing used with the SeqSero tool can have many advantages that far out way the limitations. It proved to be a very fast and efficient way to accurately predict Salmonella serotypes. By using the SeqSero database with the raw whole genome sequencing, we were able to accurately predict serotypes for Salmonella isolates with as much accuracy (or better) as that provided by the traditional KWS protocols. We found that successful results were obtained for 899 (86.4%) isolates having the same antigenic formula and serotype call. This percentage can only be expected to increase over time with the addition of new strains to the national database and improvements made to the SeqSero algorithm. This study shows the overall suitability of replacing traditional phenotypic methods with genomic serotyping using the SeqSero tool.
While both SISTR and MLST also provide us with increased phylogenetic classification which can be used to answer additional epidemiological questions; SeqSero provides the opportunity to analyze results directly from raw reads (Yachison et al., 2017).
In addition, SeqSero provided a wealth of genomic details that can be very important in determining Salmonella phylogenetic associations or understanding nuances of pathogenic differences. At the same time, future investigational studies on the isolates that did not yield matching results between SeqSero and traditional serotyping will be performed.
One example of SeqSero providing useful pathogenic information was discovered in 20 Salmonella Typhimurium isolates that were found to be absent of the O5 epitope. This epitope is absent due to a frameshift deletion detected in the oafA gene mutation which can have a significant role in complement-mediated killing of bacteria (Fox et al., 2005).
Routine and real-time implementation of WGS has the potential to transform public health microbiology (Joensen et al., 2014). Efforts have been made to enable a variety of pathogen subtyping and characterization analyses through the use of WGS data, such as multi-locus sequence typing (Inouye et al., 2012; Larsen et al., 2012), antimicrobial resistance identification, and virulence characterization (Zankari et al., 2012). Beyond WGS of pure cultures, recent application of metagenome sequencing in diagnosis and outbreak investigation of infectious diseases has demonstrated the potential for culture-independent detection of pathogens from complex clinical samples (Loman et al., 2013). For Salmonella serotyping, databases were built for O and H antigen determination (Jiang et al., 1991). The significant use of WGS technology has clearly been demonstrated in epidemiological studies and outbreak detection for Salmonella and other enteric pathogens revealing outbreak associations missed by standard Pulsed-Field Gel Electrophoresis (Den Bakker et al., 2014; Scaltriti et al., 2015).
Our laboratory routinely uses WGS for predicting Salmonella serotypes for every isolate from current investigations (food/feed/environmental) and from our archived isolates from previous years. Given our results, serotyping based on WGS prediction using SeqSero can be widely used as reliable method for Salmonella serotyping due to its numerous advantages of accurate and more granular details describing the molecular characteristics of Salmonella isolates. Furthermore, major budgetary savings as well as expeditious result times compared to the traditional serotyping using the KWS or molecular serotyping methods are other significant advantages for SeqSero serotyping.
Conclusion
It is now routine for our laboratory to perform whole genome sequencing and traditional serotyping for every real time Salmonella isolate from animal feed, human foods, and environmental swabs. Using WGS and the in silico SeqSero tool as the sole method for Salmonella serotyping can have numerous advantages as it allows for very quick and accurate serotype predictions, detailed genetic information (antimicrobial resistance and virulence), significant budgetary savings, and reduced labor requirements. As more whole genomes are added daily to the NCBI Salmonella national database, the ability of SeqSero and online databases to make accurate assignments of Salmonella serotypes will continue to improve and expand.
Author Contributions
GI designed study, collected data, performed statistical analysis, analyzed data, and main contributor to manuscript writing. PM assisted with study design and critically revised intellectual content of the manuscript.
Conflict of interest statement
The authors declare that the research was conducted in the absence of any commercial or financial relationships that could be construed as a potential conflict of interest.
Acknowledgments
The authors would like to gratefully thank John Leazer, Kent Hermann and Yelena Karaseva for supporting research in NFFL. Special thanks to John Leazer, Ivica Arsov, and Christopher Welch for critical reading of the manuscript. Also, we would like to acknowledge the hard work of our NFFL Microbiology team, WGS team and ARL Traditional Serology team. We also recognize the intelligent and dedicated work of the SeqSero team.
Supplementary Material
The Supplementary Material for this article can be found online at: https://www.frontiersin.org/articles/10.3389/fmicb.2018.02993/full#supplementary-material
Footnotes
1. ^Methods/Pulsenet/CDC, W. G. S. W. P, and Available online at: www.cdc.gov/pulsenet/pathogens/wgs.html. February 11.
References
Ahrenfeldt, J., Skaarup, C., Hasman, H., Pedersen, A. G., Aarestrup, F. M., and Lund, O. (2017). Bacterial whole genome-based phylogeny: construction of a new benchmarking dataset and assessment of some existing methods. BMC Genomics 18:19. doi: 10.1186/s12864-016-3407-6
Alkan, C., Sajjadian, S., and Eichler, E. E. (2011). Limitations of next-generation genome sequence assembly. Nat. Methods 8, 61–65. doi: 10.1038/nmeth.1527
Bakker, H. C., Switt, A. I., Cummings, C. A., Hoelzer, K., Degoricija, L., Rodriguez-Rivera, L. D., et al. (2011). A whole-genome single nucleotide polymorphism-based approach to trace and identify outbreaks linked to a common Salmonella enterica subsp. enterica serovar Montevideo pulsed-field gel electrophoresis type. Appl Environ Microbiol. 77, 8648–8655. doi: 10.1128/AEM.06538-11
Bale, J., Meunier, D., Weill, F. X., Depinna, E., Peters, T., and Nair, S. (2016). Characterization of new Salmonella serovars by whole-genome sequencing and traditional typing techniques. J. Med. Microbiol. 65, 1074–1078. doi: 10.1099/jmm.0.000325
Bekal, S., Berry, C., Reimer, A. R., Van Domselaar, G., Beaudry, G., Fournier, E., et al. (2016). Usefulness of high-quality core genome single-nucleotide variant analysis for subtyping the highly clonal and the most prevalent Salmonella enterica Serovar Heidelberg clone in the context of outbreak investigations. J. Clin. Microbiol. 54, 289–295. doi: 10.1128/JCM.02200-15
CDC (2015). Serotypes and the Importance of Serotyping Salmonella. Available online at: https://www.cdc.gov/salmonella/reportspubs/salmonella-atlas/serotyping-importance.html (accessed July 16, 2018).
CDC (2018). Salmonella Homepage|CDC. Available online at: https://www.cdc.gov/salmonella/ (accessed July 18, 2018).
Den Bakker, H. C., Allard, M. W., Bopp, D., Brown, E. W., Fontana, J., Iqbal, Z., et al. (2014). Rapid whole-genome sequencing for surveillance of Salmonella enterica serovar enteritidis. Emerging Infect. Dis. 20, 1306–1314. doi: 10.3201/eid2008.131399
Deng, X., Shariat, N., Driebe, E. M., Roe, C. C., Tolar, B., Trees, E., et al. (2015). Comparative analysis of subtyping methods against a whole-genome-sequencing standard for Salmonella enterica serotype enteritidis. J. Clin. Microbiol. 53, 212–218. doi: 10.1128/JCM.02332-14
Dieckmann, R., and Malorny, B. (2011). Rapid screening of epidemiologically important Salmonella enterica subsp. enterica serovars by whole-cell matrix-assisted laser desorption ionization-time of flight mass spectrometry. Appl. Environ. Microbiol. 77, 4136–4146. doi: 10.1128/AEM.02418-10
Fitzgerald, C., Collins, M., Van Duyne, S., Mikoleit, M., Brown, T., and Fields, P. (2007). Multiplex, bead-based suspension array for molecular determination of common Salmonella serogroups. J. Clin. Microbiol. 45, 3323–3334. doi: 10.1128/JCM.00025-07
Forbes, J. D., Knox, N. C., Ronholm, J., Pagotto, F., and Reimer, A. (2017). Metagenomics: the next culture-independent game changer. Front. Microbiol. 8:1069. doi: 10.3389/fmicb.2017.01069
Fox, K. L., Yildirim, H. H., Deadman, M. E., Schweda, E. K., Moxon, E. R., and Hood, D. W. (2005). Novel lipopolysaccharide biosynthetic genes containing tetranucleotide repeats in Haemophilus influenzae, identification of a gene for adding O-acetyl groups. Mol. Microbiol. 58, 207–216. doi: 10.1111/j.1365-2958.2005.04814.x
Gilchrist, C. A., Turner, S. D., Riley, M. F., Petri, W. A. Jr, and Hewlett, E. L. (2015). Whole-genome sequencing in outbreak analysis. Clin. Microbiol. Rev. 28, 541–563. doi: 10.1128/C.M.R.00075-13
Grimont, P., and Weill, F. (2007). Antigenic Formulae of the Salmonella Serovars. 9th Edn. Paris: WHO Collaborating Centre for Reference and Research on Salmonella.
Gupta, S. K., Mcmillan, E. A., Jackson, C. R., Desai, P. T., Porwollik, S., Mcclelland, M., et al. (2016). Draft genome sequence of Salmonella enterica subsp. enterica Serovar Bardo Strain CRJJGF_00099 (Phylum Gammaproteobacteria). Genome Announc. 4:e00964-16. doi: 10.1128/genomeA.00964-16
Gymoese, P., Sorensen, G., Litrup, E., Olsen, J. E., Nielsen, E. M., and Torpdahl, M. (2017). Investigation of outbreaks of Salmonella enterica serovar typhimurium and its monophasic variants using whole-genome sequencing, Denmark. Emerging Infect. Dis. 23, 1631–1639. doi: 10.3201/eid2310.161248
Heymann, D. A. B. A., Edward, I., and Heymann, D. L. (2006). Salmonella (Deadly Diseases & Epidemics). Philadelphia, PA: Chelsea House Publishers.
Inns, T., Lane, C., Peters, T., Dallman, T., Chatt, C., Mcfarland, N., et al. (2015). A multi-country Salmonella enteritidis phage type 14b outbreak associated with eggs from a German producer: ‘near real-time' application of whole genome sequencing and food chain investigations, United Kingdom, May to September 2014. Euro Surveill. 20:21098. doi: 10.2807/1560-7917.ES2015.20.16.21098
Inouye, M., Conway, T. C., Zobel, J., and Holt, K. E. (2012). Short read sequence typing (SRST): multi-locus sequence types from short reads. BMC Genomics 13:338. doi: 10.1186/1471-2164-13-338
Jiang, X. M., Neal, B., Santiago, F., Lee, S. J., Romana, L. K., and Reeves, P. R. (1991). Structure and sequence of the rfb (O antigen) gene cluster of Salmonella serovar typhimurium (strain LT2). Mol. Microbiol. 5, 695–713. doi: 10.1111/j.1365-2958.1991.tb00741.x
Joensen, K. G., Scheutz, F., Lund, O., Hasman, H., Kaas, R. S., Nielsen, E. M., et al. (2014). Real-time whole-genome sequencing for routine typing, surveillance, and outbreak detection of verotoxigenic Escherichia coli. J. Clin. Microbiol. 52, 1501–1510. doi: 10.1128/JCM.03617-13
Koser, C. U., Ellington, M. J., Cartwright, E. J., Gillespie, S. H., Brown, N. M., Farrington, M., et al. (2012). Routine use of microbial whole genome sequencing in diagnostic and public health microbiology. PLoS Pathog. 8:e1002824. doi: 10.1371/journal.ppat.1002824
Larsen, M. V., Cosentino, S., Rasmussen, S., Friis, C., Hasman, H., Marvig, R. L., et al. (2012). Multilocus sequence typing of total-genome-sequenced bacteria. J. Clin. Microbiol. 50, 1355–1361. doi: 10.1128/JCM.06094-11
Leekitcharoenphon, P., Nielsen, E. M., Kaas, R. S., Lund, O., and Aarestrup, F. M. (2014). Evaluation of whole genome sequencing for outbreak detection of Salmonella enterica. PLoS ONE 9:e87991. doi: 10.1371/journal.pone.0087991
Li, P., Liu, Q., Luo, H., Liang, K., Yi, J., Luo, Y., et al. (2017). O-serotype conversion in Salmonella typhimurium induces protective immune responses against invasive non-typhoidal Salmonella infections. Front. Immunol. 8:1647. doi: 10.3389/fimmu.2017.01647
Lienau, E. K., Strain, E., Wang, C., Zheng, J., Ottesen, A. R., Keys, C. E., et al. (2011). Identification of a salmonellosis outbreak by means of molecular sequencing. N. Engl. J. Med. 364, 981–982. doi: 10.1056/NEJMc1100443
Loman, N. J., Constantinidou, C., Christner, M., Rohde, H., Chan, J. Z., Quick, J., et al. (2013). A culture-independent sequence-based metagenomics approach to the investigation of an outbreak of Shiga-toxigenic Escherichia coli O104:H4. JAMA 309, 1502–1510. doi: 10.1001/jama.2013.3231
McQuiston, J. R., Parrenas, R., Ortiz-Rivera, M., Gheesling, L., Brenner, F., and Fields, P. I. (2004). Sequencing and comparative analysis of flagellin genes fliC, fljB, and flpA from Salmonella. J. Clin. Microbiol. 42, 1923–1932. doi: 10.1128/JCM.42.5.1923-1932.2004
McQuiston, J. R., Waters, R. J., Dinsmore, B. A., Mikoleit, M. L., and Fields, P. I. (2011). Molecular determination of H antigens of Salmonella by use of a microsphere-based liquid array. J. Clin. Microbiol. 49, 565–573. doi: 10.1128/JCM.01323-10
Oakeson, K. F., Wagner, J. M., Mendenhall, M., Rohrwasser, A., and Atkinson-Dunn, R. (2017). Bioinformatic analyses of whole-genome sequence data in a public health laboratory. Emerging Infect. Dis. 23, 1441–1445. doi: 10.3201/eid2309.170416
Samuel, G., and Reeves, P. (2003). Biosynthesis of O-antigens: genes and pathways involved in nucleotide sugar precursor synthesis and O-antigen assembly. Carbohydr. Res. 338, 2503–2519. doi: 10.1016/j.carres.2003.07.009
Scaltriti, E., Sassera, D., Comandatore, F., Morganti, M., Mandalari, C., Gaiarsa, S., et al. (2015). Differential single nucleotide polymorphism-based analysis of an outbreak caused by Salmonella enterica serovar Manhattan reveals epidemiological details missed by standard pulsed-field gel electrophoresis. J. Clin. Microbiol. 53, 1227–1238. doi: 10.1128/JCM.02930-14
Taylor, A. J., Lappi, V., Wolfgang, W. J., Lapierre, P., Palumbo, M. J., Medus, C., et al. (2015). Characterization of foodborne outbreaks of Salmonella enterica serovar enteritidis with whole-genome sequencing single nucleotide polymorphism-based analysis for surveillance and outbreak detection. J. Clin. Microbiol. 53, 3334–3340. doi: 10.1128/JCM.01280-15
Vanegas, R. A., and Joys, T. M. (1995). Molecular analyses of the phase-2 antigen complex 1,2,. of Salmonella spp. J. Bacteriol. 177, 3863–3864.
Yachison, C. A., Yoshida, C., Robertson, J., Nash, J. H. E., Kruczkiewicz, P., Taboada, E. N., et al. (2017). The validation and implications of using whole genome sequencing as a replacement for traditional serotyping for a National Salmonella Reference Laboratory. Front. Microbiol. 8:1044. doi: 10.3389/fmicb.2017.01044
Zankari, E., Hasman, H., Cosentino, S., Vestergaard, M., Rasmussen, S., Lund, O., et al. (2012). Identification of acquired antimicrobial resistance genes. J. Antimicrob. Chemother. 67, 2640–2644. doi: 10.1093/jac/dks261
Keywords: Salmonella, traditional serology, Kauffmann White scheme, whole genome sequencing, SeqSero
Citation: Ibrahim GM and Morin PM (2018) Salmonella Serotyping Using Whole Genome Sequencing. Front. Microbiol. 9:2993. doi: 10.3389/fmicb.2018.02993
Received: 14 May 2018; Accepted: 20 November 2018;
Published: 13 December 2018.
Edited by:
George Grant, University of Aberdeen, United KingdomReviewed by:
Andrea Isabel Moreno Switt, Universidad Andrés Bello, ChileJonathan Gray Frye, US National Poultry Research Centre (USDA-ARS), United States
Hassan Bruno Hartman, Public Health England, United Kingdom
Copyright © 2018 Ibrahim and Morin. This is an open-access article distributed under the terms of the Creative Commons Attribution License (CC BY). The use, distribution or reproduction in other forums is permitted, provided the original author(s) and the copyright owner(s) are credited and that the original publication in this journal is cited, in accordance with accepted academic practice. No use, distribution or reproduction is permitted which does not comply with these terms.
*Correspondence: George M. Ibrahim, Z2VvcmdlLmlicmFoaW1AZmRhLmhocy5nb3Y=