- 1Department of Zoology, University of Delhi, New Delhi, India
- 2PhiXGen Private Limited, Gurugram, India
- 3Department of Veterinary & Biomedical Sciences, South Dakota State University, Brookings, SD, United States
- 4National Centre for Microbial Resource, National Centre for Cell Science, Pune, India
- 5ICAR-Indian Institute of Soil Science, Bhopal, India
Genomic information for outlier strains of Pseudomonas aeruginosa is exiguous when compared with classical strains. We sequenced and constructed the complete genome of an environmental strain CR1 of P. aeruginosa and performed the comparative genomic analysis. It clustered with the outlier group, hence we scaled up the analyses to understand the differences in environmental and clinical outlier strains. We identified eight new regions of genomic plasticity and a plasmid pCR1 with a VirB/D4 complex followed by trimeric auto-transporter that can induce virulence phenotype in the genome of strain CR1. Virulence genotype analysis revealed that strain CR1 lacked hemolytic phospholipase C and D, three genes for LPS biosynthesis and had reduced antibiotic resistance genes when compared with clinical strains. Genes belonging to proteases, bacterial exporters and DNA stabilization were found to be under strong positive selection, thus facilitating pathogenicity and survival of the outliers. The outliers had the complete operon for the production of vibrioferrin, a siderophore present in plant growth promoting bacteria. The competence to acquire multidrug resistance and new virulence factors makes these strains a potential threat. However, we identified major regulatory hubs that can be used as drug targets against both the classical and outlier groups.
Introduction
Pseudomonas aeruginosa is one of the major opportunistic pathogens that has been isolated from a wide range of ecological niches including soil, water and clinical samples (Stover et al., 2000). This opportunistic pathogen is prototypical “multidrug-resistant” (MDR) with intrinsically advanced antibiotic resistance gene clusters (Lambert, 2002; Livermore, 2002). Some of the strains of P. aeruginosa have been associated with serious illnesses mainly imparting nosocomial infections (ventilator-associated pneumonia) and various sepsis syndromes (Planquette et al., 2013; Philippart et al., 2015). The pathogenicity of P. aeruginosa is attributed to the presence of prominent virulence factors that consist of proteases, pili, flagella, quorum sensing proteins, exotoxin A and type III secretion system (T3SS) (Lyczak et al., 2000). Amongst these, the T3SS- also called injectosome is responsible for the injection of toxins into the eukaryotic host cell and thus majorly determines the degree of pathogenicity (Cornelis, 2006). Therefore, strains lacking T3SS were thought to be less pathogenic due to the absence of injectosome machinery and its effector toxins (ExoS, ExoT, ExoU, and ExoY) that play a prominent role in acute as well as chronic infections (Hauser, 2009). These T3SS deficient strains are termed as outlier strains and they form a distinct phylogenetic clade represented by strain PA7 (Roy et al., 2010); and were shown to have diverged early from the classical strains of P. aeruginosa (Gomila et al., 2015). The outliers counteract T3SS deficiency by adopting novel virulence factors and attaining multidrug resistance, thus making them difficult to treat using antibiotics (Roy et al., 2010; Huber et al., 2016). An outlier clinical isolate of P. aeruginosa strain CLJ1 was reported to induce hemorrhagic pneumonia, mainly due to the presence of a two-partner secreted Exolysin (ExlA) with membrane lytic activity (Elsen et al., 2014). The two genes encoding this lytic toxin are present on a region of genomic plasticity (RGP) that is associated with outlier P. aeruginosa (Huber et al., 2016; Reboud et al., 2016). Although strains of P. aeruginosa are ubiquitous and there are more than 1500 assemblies present for classical strains, only a handful of draft genomes (n = 12) (Boukerb et al., 2015; Kos et al., 2015; Mai-Prochnow et al., 2015; van Belkum et al., 2015) are present in the genome database for outlier strains (either clinical isolates or with unknown origin), with a single complete genome of strain PA7 (Roy et al., 2010).
In this study, a new outlier strain CR1 of P. aeruginosa, an isolate from the rhizosphere of chili crop was genomically characterized (Supplementary Table S1). Its complete genome was sequenced and compared with other outliers of clinical and unknown origin. Virulence factors in association with acquired resistance play a significant role in inducing pathogenicity, therefore the genes associated with virulence and antibiotic resistance were analyzed in this environmental isolate. Here, we also report the presence of a plasmid pCR1 from strain CR1 which is not present in the genome of any of the outlier strains. The plasmid had VirB/D4 type IV secretion system in association with trimeric autotransporter adhesin protein having similarity with yadA like adhesin that can impart a pathogenic potential to CR1 (Christie, 2001; Linke et al., 2006; Basso et al., 2017). Like other outliers, CR1 lacks T3SS which majorly confers pathogenicity. The probability of these strains to be a pathogen was also assessed based on the number of pathogenic protein families present in the genome. Differences in the core and pan-genes of the two groups were determined. Core genome-wide genes under positive selection were determined for identifying the genes under strong positive selection that can provide advantageous variants to the outlier genomes. The ability of these strains to gain virulence factors and acquired antibiotic resistance require new drug targets to be identified. Therefore, protein–protein interaction on the core genome of both the groups was used to identify major regulators that can be targeted as drug targets.
Materials and Methods
Genome Sequencing and Assembly
The Pseudomonas strain CR1 used in the study is an isolate from the rhizosphere of chili crop grown with high inputs of chemical fertilizers and pesticides at Guntur, Andhra Pradesh, India (Supplementary Table S1). The DNA was extracted using Cetyl Trimethyl Ammonium Bromide (CTAB) extraction method (Wilson, 1987) and sequenced using Single Molecule Real Time sequencing (SMRT, Pacific Biosciences) at the Quebec Innovation Centre McGill University, Canada. The 16S rRNA sequence homology of the strain revealed its identity as Pseudomonas aeruginosa.
A total of 73,250 subreads of average length 10,488 bp were obtained from Pac Bio RS II with 20 kb library (Pacific Biosciences, Menlo Park, CA, United States). The reads were assembled into 2 contigs, using the HGAP2 algorithm (Chin et al., 2013) in SMRT Analysis 2.1 (Pacific Biosciences).
Selection and Annotation of Genomes for Comparative Analysis
For comparative genomic analysis, complete genomes of 50 P. aeruginosa strains (including the complete genome of PA7) were retrieved from the Pseudomonas Genome Database (Winsor et al., 2016) (Supplementary Table S2, data accessed on 14.07.2016). Further to increase the resolution, 15 draft genomes reported as the closest neighbor by RAST neighbor score were also included in the analysis. Preliminary analysis revealed that the strain CR1 was a member of the outlier PA7 clade. Therefore, we included all the draft genomes of outlier strains (n = 12) of P. aeruginosa available till 14.07.2016. The genomes were annotated by RAST server 2.0 (Aziz et al., 2008) and Glimmer-3 (Delcher et al., 2007) was used for predicting genes. The 12 draft genome of outliers and two complete genomes of strain CR1 and PA7 were taken for genomic comparison among outliers. The draft genomes were checked for completeness by analyzing the presence of 107 essential copy genes using the Comprehensive Microbial Resource as a database, where 107 Hidden Markov models (HMMs) of essential copy genes were analyzed in all phylogenetic outlier strains (Dupont et al., 2012).
Further, the 14 outlier strains were annotated for RNAs and tRNAs using RNAmmer (Lagesen et al., 2007) and ARAGORN, (Laslett and Canback, 2004), respectively. The CRISPR elements within the outlier strains were analyzed using CRISPR finder server (Grissa et al., 2007). The Phage genomic content was determined using PHAST (Zhou et al., 2011). The sequence type (ST) was also predicted for the strains clustered in outlier clade using MLST 1.8 tool (Larsen et al., 2012). The whole genome sequence of strain CR1 was deposited to PubMLST server (Jolley et al., 2018) for assigning new ST. The DNA-DNA hybridization values were also calculated to delineate at sub-species level using the Genome to Genome Distance Calculator (GGDC v 2.1) for the outliers (Auch et al., 2010a,b).
Phylogenomics Analysis
All Pseudomonas strains (n = 78) were subjected to phylogenetic/phylogenomic analysis using four different methods viz. Mummer based Average Nucleotide Identity (ANIm), PhyloPhlAn (Segata et al., 2013), phylogeny based on SNPs within the core genome using Harvest suite (Treangen et al., 2014) and pangenome consensus matrix using get_homologues (Contreras-Moreira and Vinuesa, 2013). The ANIm values were calculated for each pair of genomes using pyani1 module based on the Mummer algorithm at default parameters. Thereafter, a dual dendrogram based on ANIm values, was constructed in R (R Development Core Team, 2015). Further, to obtain a consensus tree topology, amino acid sequences predicted by Glimmer-3 were used for phylogenomic reconstruction using PhyloPhlAn. PhyloPhlAn locates 400 ubiquitous bacterial genes by sequence alignment in amino acid space, then builds a tree by concatenating the most discriminative positions in each gene into a single long sequence and applying FastTree (Price et al., 2009), and RaxML (Stamatakis, 2014) to construct phylogenetic tree based on maximum likelihood with 1000 bootstrap replicates. Unrooted maximum likelihood (100 bootstraps) tree of 78 P. aeruginosa genomes based on SNPs within the core genome was defined using Parsnp tool of the Harvest suite.
Pangenome matrix was also used to construct the dendrogram for closely related P. aeruginosa strains in order to obtain better resolution of phylogenetic clades. The consensus pangenome matrix was generated using the intersection of pan-genes predicted by COG and OMCL algorithms using get_homologues. This matrix was then used to construct a parsimony tree using PARS program from the PHYLIP suite to deduce the phylogeny implied in this matrix.
Determination of Regions of Genome Plasticity (RGP)
The strain CR1 was analyzed for regions of genomic plasticity (RGP) using the standard genome subtractive approach on pairwise genome comparisons with reference genomes of PAO1, UCBPP-PA14, and PA7. The criteria to characterize and discriminate RGPs from other features in a genome were based on the following: atypical composition, presence of tRNA- that serves as insertion sites, presence of insertion sequence (IS) elements and direct repeats (DR) flanking RGPs, presence of integrase and transposase (Langille et al., 2010; Che et al., 2014). These RGP were then plotted in genome map using CG view (Grant and Stothard, 2008) in the circular map of the chromosome 1 (Figure 1A).
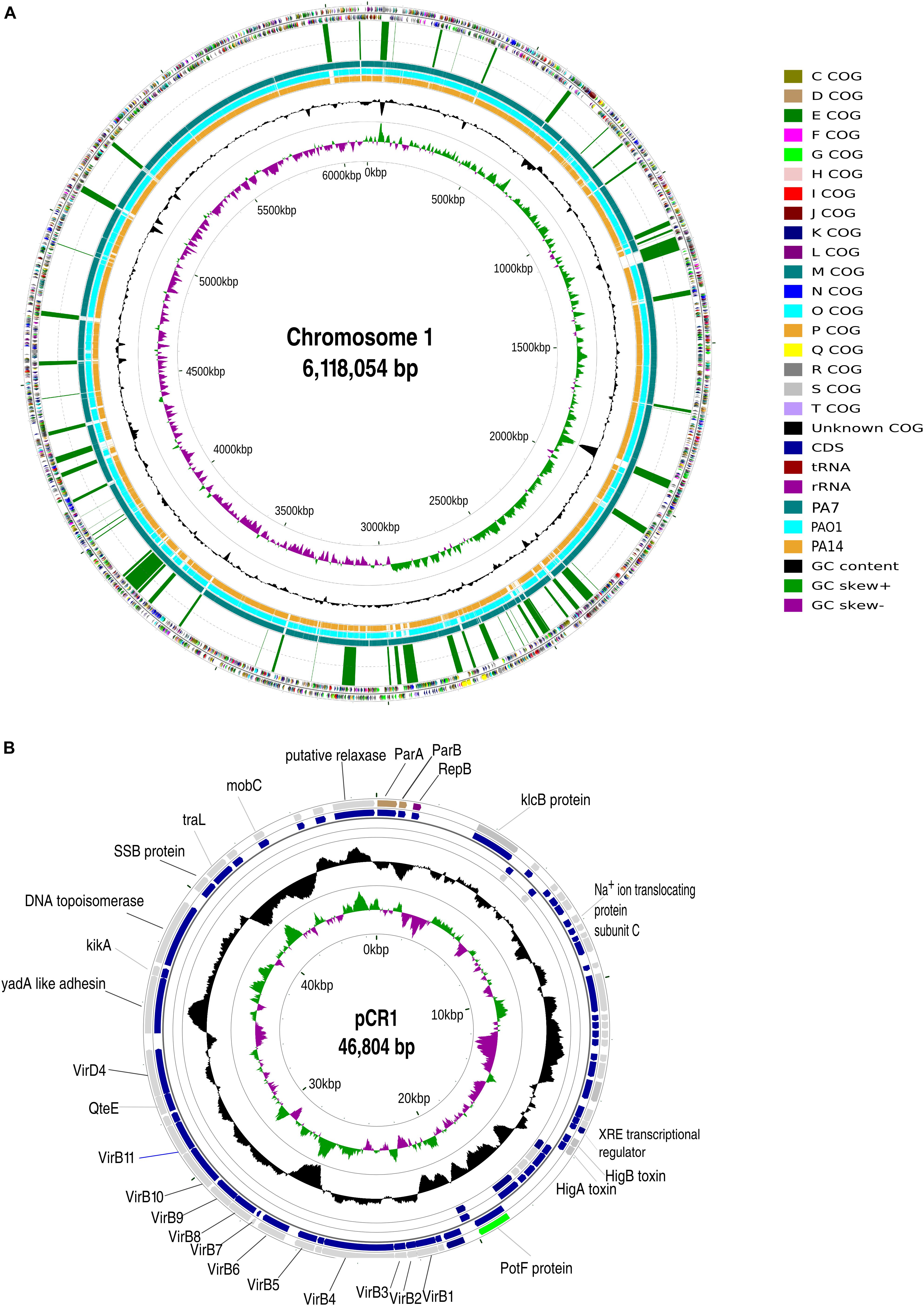
Figure 1. General genomic attributes of Pseudomonas aeruginosa CR1. (A) Circular map of chromosome 1 of CR1 strain. From outside to inside Ring 1: COG categories of ORFs in the positive strand. Ring 2: COG categories of ORFs in the negative strand. Ring 3: Regions of Genomic plasticity starting from zero bp mark in a clockwise direction. Ring 4: BLASTn alignment (expected threshold = 1e-220) between CR1 and previously sequenced outlier PA7 (teal); Ring 5: PAO1 (aqua); Ring 6: UCBPP-PA14 (orange). Rings 7 and 8: GC content and GC skew, respectively. (B) Circular map of plasmid pCR1. From outside to inside Rings 1, 2, 3, and 4 depicts COG categories of ORFs of the positive strand, genes on positive strand, genes on negative strand and COG categories of ORFs of negative strand, respectively. Rings 5 and 6 represents GC content and GC skew, respectively.
Orthologs Under Positive Selection
The orthologs were predicted using OrthoMCL v1.4 using –mode 1. The orthologous groups were then added to POTION v 1.1.3 pipeline (Supplementary File S1) (Hongo et al., 2015). The genes in the orthologous group were detected for recombination signals and removed using PhiPack (Bruen and Bruen, 2005). For all genes tested for positive selection, the false discovery rate (FDR) was controlled using the BY method (Benjamini and Yekutieli, 2001) and the significance level was set to 10%. The rates of synonymous and non-synonymous substitutions were estimated using site-model of the codeml program in the PAML v4.8 package (Yang, 2007). trimAl (Capella-Gutiérrez et al., 2009) was used for alignment trimming. A phylogenetic tree for each gene was built using the maximum-likelihood approach implemented by PhyML v3.0 (Guindon and Gascuel, 2003). The search for positive selection in codeml is done by comparing the log-likelihood values of codon evolution models that do not allow sites with positive selection (M1a, M7, and M8a) with the values obtained from the more general nested models that also allow for site classes with positive selection occurrence (M2 and M8, respectively). The p-values are calculated as 2Δl (twice the difference in the likelihood of the two nested models evaluated) based on the χ2 distribution with 2° of freedom for nested models M1a/M2 and M7/M8 and 1° of freedom for nested models M8a/M8. This being a multiple testing scenario, corrected q-values from the list of p-values obtained for all groups evaluated using the given nested model pair were determined (Hongo et al., 2015).
Core and Pan-Genome Analysis
To decipher the core genome content of the outlier strains, the get_homologues pipeline was used with OrthoMCL (OMCL) at 75% query coverage as well as sequence similarity (Contreras-Moreira and Vinuesa, 2013). The pan-genome was obtained using OrthoMCL methods and at default parameters (with t = 0). The core and pan-genomic contents were determined for all the Pseudomonas strains (n = 78), classical (n = 64), and outliers (n = 14) separately. The pangenome for 78 strains was used to find out the genes exclusively present in all the outliers (n = 14) that were absent in all the classical strains (n = 64). The core and pangenome for all groups were annotated against the COG database by rpsblast (e-value 0.001) using WebMGA server (Wu S. et al., 2011).
Functional Annotations of the Outlier Strains
To determine the functional variability across the 14 outlier genomes in the PA7 clade, the comparative genomic analysis was performed based on proteins and metabolic pathways. The ORFs were predicted using Glimmer-3 and annotated by KAAS (KEGG Automatic Annotation Server) (Moriya et al., 2007) using Bi-directional Best Hit (BBH) algorithm. Further, the metabolic pathways were reconstructed for the outlier genomes using MinPath (Minimal set of Pathways) (Ye and Doak, 2009) to obtain the minimal set of pathways. COG categories were also assigned to the proteome of all the outlier strains by rpsblast (e-value 0.001) against the COG database using WebMGA server. The matrix thus generated was plotted as a heatmap with dual dendrogram in R.
Pathogenic Potential, Resistance Profile and Virulence Factors of Outlier Strains
The genomic data of all the outlier strains were annotated for pathogenic protein families using PathogenFinder and the probability of being a pathogen was calculated using the whole genome data (Cosentino et al., 2013). Resfinder (Kleinheinz et al., 2014) was used to determine the acquired resistance genes in the outliers. Virulence factor database (VFDB) (Chen et al., 2005) was used for deciphering bacterial virulence factors.
Protein–Protein Interactions
For determining the major key regulatory proteins that are specific to particular clade and can act as potential drug targets in the classical and outlier strains, the interactome of the core genes was analyzed. The protein–protein interaction (PPI) model was constructed using STRING Database v10.5 (Szklarczyk et al., 2017). Pseudomonas aeruginosa was selected as the reference organism for PPI construction. The core genes of both the groups were used as input and queried against P. aeruginosa PPIs present in the STRING database. The PPI networks were visualized using Cytoscape Version 3.3.0 (Shannon et al., 2003). Network analyzer plugin was used for determining the statistical and functional significance of the PPI networks, calculated using different parameters such as the probability of degree distribution, average clustering coefficient and average neighborhood connectivity as described in previous studies (Albert and Barabasi, 2002; Gupta et al., 2016; Kumar et al., 2017).
Statistical Analyses of the Network
The degree of probability distribution, P(k), of a network defined by P(k) = nk/N, which is the ratio of the number of nodes having a k degree in the network (nk) to the size of the network (N), was used to capture the network structure, identification of hubs, and modular organization of the network. The constructed network obeyed the power law, P(k) ∼k-γ, indicating the scale-free nature of the network, where γ is an order parameter that identified the different topological structure of a scale-free network. The clustering coefficient C(k), which is defined by C (k) = 2E/[k (k - 1)] is the ratio of the number of edges E of the node having a k degree with neighbors to the total possible number of such edges, [k (k - 1)]/2 which is a measure of the topological structure of the network (Watts and Strogatz, 1998). The average clustering coefficient C(k) identifies overall organization of formation of clusters in the network. Similar to P(k), C(k) may depend on network size and characterizes various properties of the network: (i) for scale-free and random networks where C(k) is independent of k, C(k) ∼ constant, and (ii) for hierarchical networks where C(k) follows power-law scaling behavior, C(k) ∼kβ with β ∼ 1. The neighborhood connectivity of a node is the number of neighbors connected to it and characterizes the correlation pattern of connectivity of interacting nodes in the network. This connectivity correlation would be measured by defining a conditional probability P(k′n|kn) which is the probability of making a link from a node having degree kn to another node of degree k′n. Then, the average neighborhood connectivity of nodes with connectivity kn is given by Cn (kn) = Σk′n k′nP (k′n|kn) ∼kn-α (Girvan and Newman, 2002) following a power law scaling behavior with α < 1 for most of the real networks (Maslov and Sneppen, 2002). If Cn(kn) is an increasing function of kn (for negative values of α), then the topology of the network shows assortative mixing (Almaas, 2007) where nodes with a high number of edges per node (high-degree nodes) have an affinity to connect to other high-degree nodes in the network. However, with positive values for α is the signature of the network having a hierarchical structure, where low-degree nodes tend to connect high-degree hubs (Almaas, 2007) and few high-degree hubs present in the network try to control the low-degree nodes.
Results and Discussion
Genomic Features Among Outliers
The sequences obtained for the strain CR1 were assembled into two replicons of sizes 6,138,025 bp (Chr 1) and 61,310 bp (pCR1). In order to circularize both the replicons, the ends of the assembled contigs were mapped using BLASTn (Altschul et al., 1990). An overlapping region of 19,971 bp in case of the chromosome and 14,506 bp in the plasmid were detected and removed. The chromosome and plasmid were found to be 6,118,054 and 46,804 bp, respectively, making a total genome size of 6,164,858 bp (6.16 Mbp) (Figures 1A,B). CR1 and PA7 had the complete set of 107 essential marker genes and all the draft genomes were more than 96–98% complete based on essential marker gene approach.
The average genome size of P. aeruginosa outliers was 6,539,166 bp ± 233906 bp, with strain AZPAE14941 showing the maximum size (6,881,480 bp) and minimum in strain CR1 (6,164,858 bp) (Supplementary Figure S1). The present results show that the genome size of strain CR1 is smallest among the outlier strains, primarily due to the lack of type I restriction-modification system and mercury resistance cluster (RGP63 and RGP79). In contrast to PA7 (Roy et al., 2010) three RGPs (RGP4, RGP78, and RGP79) having characteristic phage signatures and a putative integrated plasmid (RGP75) were also absent in CR1. Out of the 18 RGP reported exclusive to PA7 (Roy et al., 2010), 13 were present in CR1. As the missing regions mostly coded for resistance to antibiotics and phage-related elements it can be reasonably ascertained that resistance is acquired by the organism under stress in a clinical setup that is missing in the environmental strains. A similar scenario was observed in the genome of an environmental isolate of classical clade- strain M18 (6,327,754 bp) that was closely related to clinical strain LESB58 (6,601,757 bp) but had a smaller genome and was more susceptible to several antimicrobial agents (Wu D. Q. et al., 2011). Based on this, it can be hypothesized that CR1 type genomes are the progenitors of PA7 type genomes and might have acquired multi drug resistance probably in a hospital environment or hospital waste disposal site. Our hypothesis gains support from the fact that an outlier strain CLJ3 that was resistant to antibiotics was isolated after antibiotic administration from the same patient from which CLJ1 was isolated (Elsen et al., 2014). This, strengthens the view that the environment shapes the genomic content of an organism (Kumar et al., 2017). Among all the outliers, only strain CR1 possessed a plasmid of size 46,804 bp with GC content of 59.2% and approximately 45% of ORFs in the plasmid coded for hypothetical proteins. The presence of plasmid within the strain CR1 suggested horizontal gene transfer (HGT) mediated acquisition as none of the outliers reported in the literature possessed any plasmids. Further, the percentage GC content of the plasmid (59.2%) is well below the average GC content (66.5%) of outliers’ genome and members of classical strains of P. aeruginosa (Mathee et al., 2008) suggesting its extra-chromosomal origin.
Outlier strains (n = 14) possessed a wide variety of CRISPR elements, with a maximum number of CRISPR arrays (n = 5) in case of CR1 and AZPAE14901 (Table 1). The presence of a large number of CRISPR elements suggested that these outliers perhaps were vulnerable to bacteriophage attacks that led to the incorporation of CRISPR elements. Eleven outlier strains showed phage protein signatures from Pseudomonas phage Pf1 (NC_001331), Pseudomonas phage YMC11/02/R656 (NC_028657) and 8 strains showed the presence of phage proteins from Pseudomonas phage F116 (NC_006552). The abundance of these phage proteins within the outliers suggested the common phage pool for the P. aeruginosa outlier strains. However, a prophage region showing similarity to proteins (Supplementary Table S3) of various phages that are known to infect bacteria associated with soil or environmental samples were found only in CR1 strain indicative of its environmental origin.
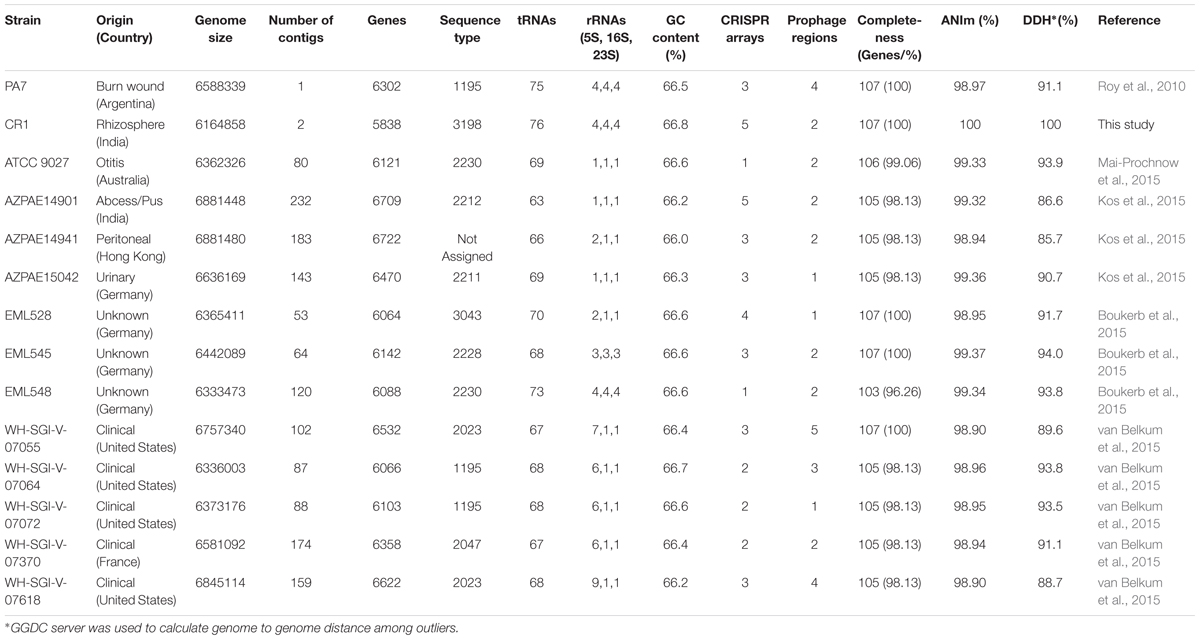
Table 1. General genomic attributes of 14 outlier strains of P. aeruginosa: ANIm values were calculated between CR1 and other outliers.
For differentiating these outliers based on their genetic background and deciphering the sequence type, a combination of DNA sequences of housekeeping alleles acs-5, aro-8, gua-3, mut-5, nuo-1, pps-11, and trp-3, and their whole genome data was used2. Amongst the outliers (n = 14), 11 were assigned known sequence type and it was found that strain CR1, AZPAE14941, and EML528 corresponds to an unknown sequence type (Table 1), highlighting a possibility of novel sequence type for these three outlier strains. Subsequently, strain CR1 and EML528 were assigned new sequence types, while no sequence type was assigned to AZPAE14941 due to its allele profile being incomplete (Table 1).
Conjugative Plasmid pCR1
The genes encoded by pCR1 had no significant sequence similarity with the genomic sequence of the outliers making the genes encoded by pCR1 specific to strain CR1. Although it had 45% hypothetical proteins, pCR1 had all proteins required for replication (B7D75_29005), plasmid maintenance (ParA: B7D75_28995, ParB: B7D75_29000 KikA protein: B7D75_29260) and conjugation (TraL: B7D75_29275, T4SS B7D75_29180-29235) (Figure 1B) that made up the plasmid backbone. Additionally, a HigB/A toxin/antitoxin (TA) (B7D75_29125/B7D75_29130) system that is shown to influence the virulence factors pyochelin, pyocyanin, and biofilm formation in P. aeruginosa (Wood and Wood, 2016) was also detected. pCR1 showed an overall identity (Iden) of 97% with a query coverage (QC) of 76% with a newly sequenced plasmid p14057 having KPC resistance (BLASTn; NCBI NR database). It also contained VirB/D4 type IV secretion system (T4SS) gene cluster (B7D75_29180-29240), showing similarity with the T4SS present on the chromosome of P. savastanoi (QC: 83% and Iden.: 100%; Accession: FN645745.1) and to T4SS on the plasmid of P. syringae B76 (QC: 65% and Iden: 68%; Accession: JQ418525.1). VirB/D4 type T4SS mediates the transfer of DNA-protein complexes and other proteins like virulence factors to the host cell by conjugation (Fronzes et al., 2009; Wallden et al., 2010). The putative relaxase (B7D75_28990) on pCR1 in association with the T4SS system might be responsible for rolling circle replication-mediated conjugation (Chandler et al., 2013) in strain CR1. Notably, this plasmid had no identity to the conjugative integrated pKLC102 region (RGP75) of the strain PA7. Additionally, a conjugal transfer protein (B7D75_29255) having yadA like anchor protein domain (LPXTG motif) similar to the putative adhesin on the plasmid of strain PA3448 isolated from blood of human (QC: 100%, Iden.: 74%; Accession No: OGX61696.1) was found in pCR1. This protein belongs to the class of trimeric autotransporters adhesins (TAAs) and is characterized as a virulence factor by BLASTp based on similarity with VFDB protein dataset, increasing the capability of adhesion to both biotic and abiotic surfaces and auto-aggregation leading to biofilm formation (Linke et al., 2006). The genes for antibiotic resistance often reported from clinical P. aeruginosa plasmids were absent.
Consensus Phylogenomic Clustering of Outliers
To demarcate the outlier strains of P. aeruginosa, the phylogenetic and phylogenomic analyses were performed by using 400 conserved marker genes, ANIm, core genome alignment, and pan-genome gene presence/absence matrix. All the analyses revealed three clades with representative genomes of PAO1, UCBPP-PA14, and PA7. It corresponded with the phylogeny obtained by Freschi et al., 2015. CR1 was the member of the PA7 clade with members that are completely devoid of type III secretion system. Strains in the outlier clade shared nearly 94% identity with the classical strains whereas, within the clade, the percentage identity increased up to 98–99%, suggesting the higher similarity in outliers’ genomic repertoire. A similar trend of clustering was observed in the phylogeny based on 400 ubiquitous conserved bacterial genes, pan-genome matrix using maximum likelihood method (Guindon and Gascuel, 2003) (Figure 2 and Supplementary Figures S2, S3) and unrooted tree based on core genome alignment (Supplementary Figure S4). This confirmed the high level of genomic relatedness among the classical strains and outliers yet reflecting the two groups to be different. The results from our analyses suggest that the whole genome-based phylogeny methods should be used for delineating the closely related strains which are found to be 100% similar on the basis of 16S rRNA gene sequence.
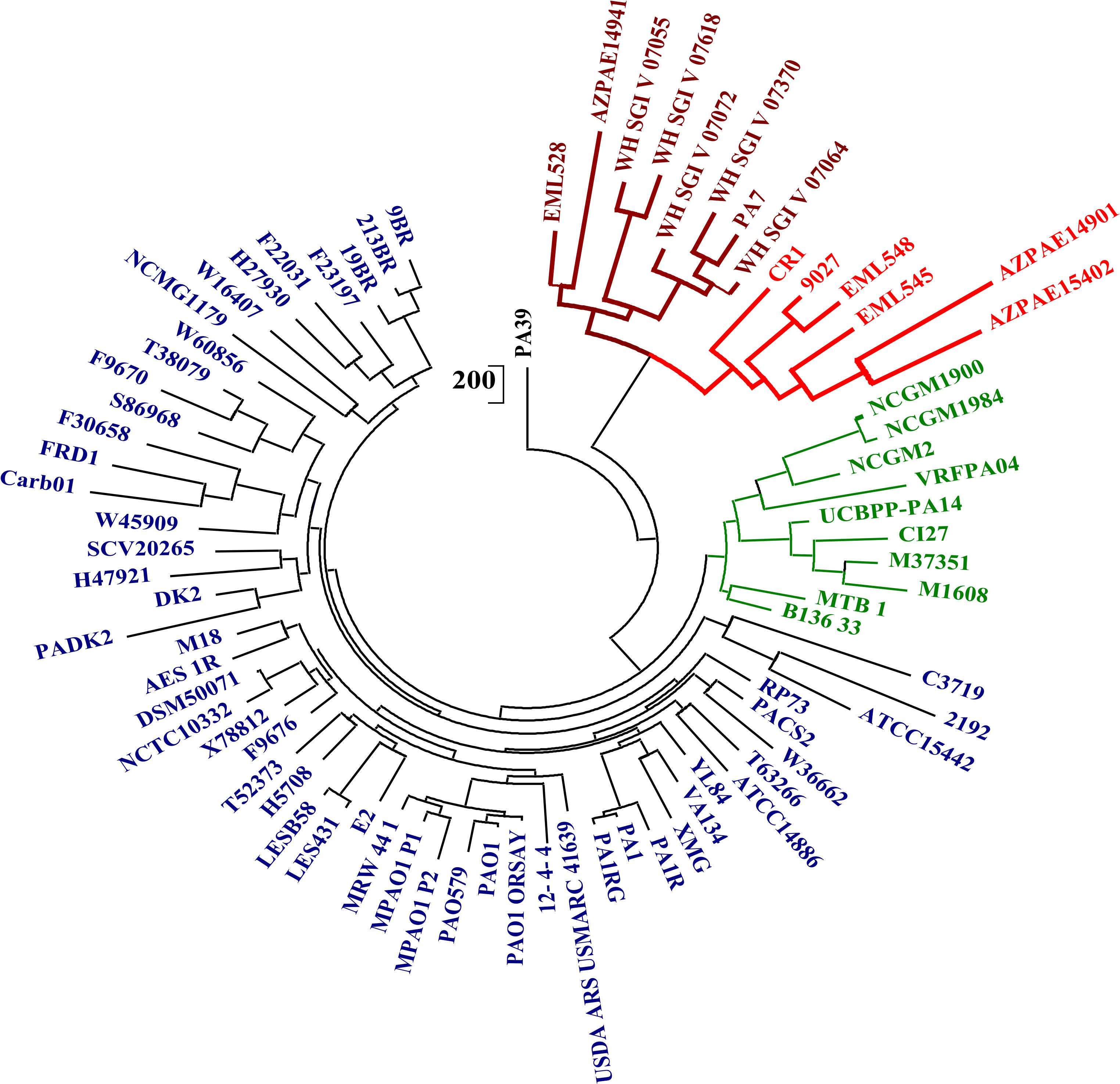
Figure 2. Phylogenetic analysis of P. aeruginosa strains using pan-genome matrix. The consensus pan-genome matrix was generated using the intersection of pan genes predicted by COG and OMCL algorithms to construct a parsimony tree using PARS from the PHYLIP. Three major clusters represented by PAO1 (Blue), UCBPP-PA14 (Green), and PA7 (Maroon) were observed. The outlier clade represented by PA7 was split into 2 clades one with a representative genome of PA7 (Maroon) and second by strain CR1 (red).
The dendrograms based on ANI, tree based on core-genome alignment and pangenome matrix provided better resolution of phylogenetic distance among the clades as these strains differed on the basis of the pan-genomic content and SNPs in the core genome alignment and in case of 400 conserved genes, the resolution was less due to high sequence identity among the 400 marker genes (98–99%). Based on this the outlier clade was distinctly represented by two sub-clades one with the representative complete genome of CR1 with draft genomes of strains ATCC9027, EML548, EML545, AZPAE14901, and AZPAE15042 and the second clade had PA7 as the representative with the genomes of EML528, AZPAE14941, WH-SGI-V-07055, WH-SGI-V-07618, WH-SGI-V-07072, WH-SGI-V-07370, and WH-SGI-V-07064. Based on the genetic divergence, we propose two sub-clades within the outliers.
Regions of Genomic Plasticity (RGP) in Strain CR1
Previous studies have shown the presence of various regions of genomic plasticity (RGP) within P. aeruginosa strains (Mathee et al., 2008; Roy et al., 2010; Jani et al., 2016). As the genome of strain CR1 is nearly 99% similar to PA7, there were large regions of DNA that were 100% similar (Supplementary Figure S5). At the time of analysis 87 RGPs (numbered 1 to 97) had been reported in P. aeruginosa strains (Klockgether et al., 2011; Boukerb et al., 2016). Out of the 87 RGPs, 32 were missing in strain CR1 and it harbored eight new RGPs which were added to already known 87 RGPs (numbered RGP98-RGP105; Supplementary Table S4). Although the sequence similarity of strain CR1 and PA7 based on ANIm was 98.97%, strain CR1 harbored 11 prominent regions (eight new RGPs and differences in three already known RGPs) having features not reported earlier. Two previously known regions (RGP5 and RGP72) had particular ORFs in strain CR1 which were absent in strain PAO1, UCBPP-PA14, and PA7. The third region, RGP58 was found to be missing in PA7 but was present in CR1. The entire list of RGPs has been compiled (Supplementary Table S4) and the strain-specific features of CR1 have been listed (Table 2 and Supplementary Figure S6). Replacements islands were identified in RGP9, RGP31, RGP60, and RGP68 that had the strain-specific genes for flagellin glycosylation, O-antigen gene cluster, pilA gene, and pyoverdine synthesis gene cluster, respectively. RGP63 and RGP64 had homologous ORF disrupted by insertion in strain CR1. The ORFs included in the RGPs were identified and numbered as per the NCBI locus tag for strain CR1 (B7D75) and PA7 (PSPA7). The flanking loci (conserved) of each RGP called anchors in strains PAO1 and UCBPP-PA14 were also identified as listed in Supplementary Table S4.
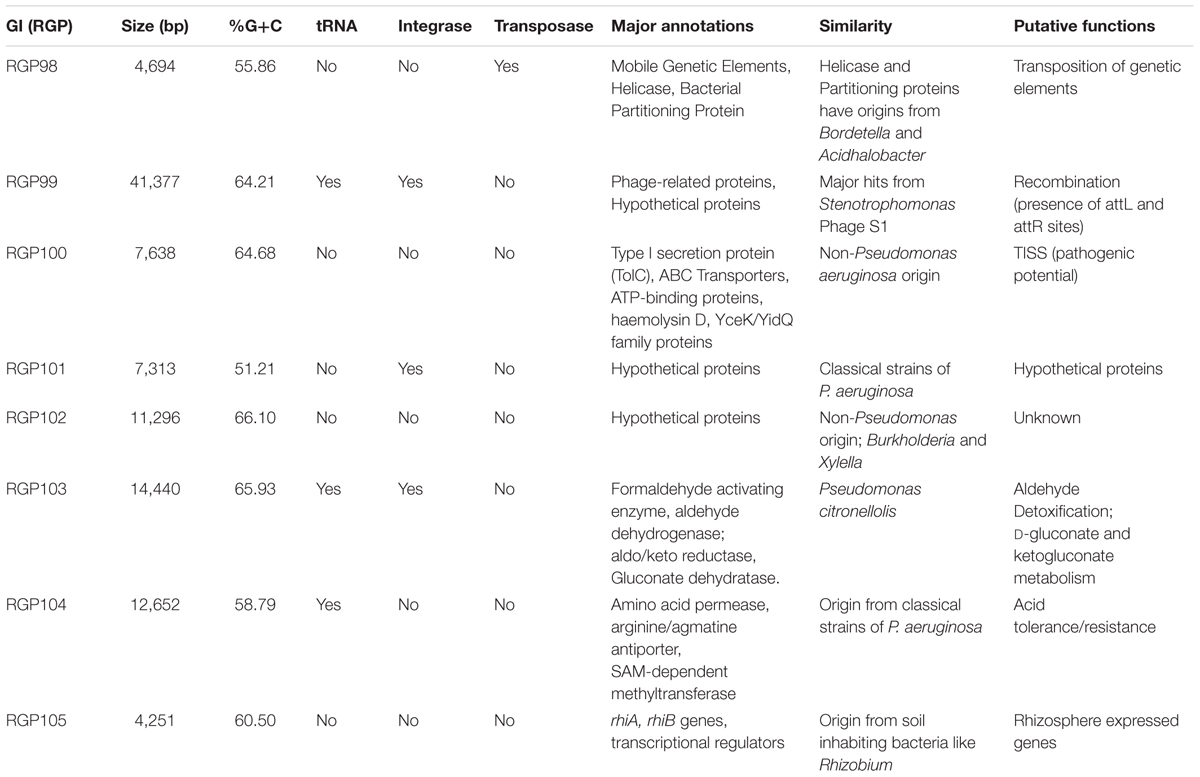
Table 2. Details to RGPs found in strain CR1: number of ORFs, GC content, tRNA, integrase and transposase genes, major annotation, similarity/origin, and putative functions.
These regions of genomic plasticity as observed in strain CR1 might provide various strain specific advantages to the organism. For instance, RGP72 in strain CR1 harbored genes for galactonate metabolism from the non-Pseudomonas origin. Genes involved in D-gluconate, ketogluconate metabolism and choline degradation that were absent in PA7 were present in RGP103. The ORFs in this region had 87–93% similarity with P. citronellis, indicative of HGT. Furthermore, the RGP104 region had an arginine/agmatine antiporter that has a major role in maintaining the internal pH of the cell and also found in enteric pathogenic bacteria (Fang et al., 2010). All these regions are important in maintaining the osmotic balance of cell, protect it against reactive oxygen species (ROS) and provide continuous energy to the cell by supplying intermediates for the Entner-Doudoroff (ED) pathway and prevent starvation (Buch et al., 2008; Ghasempur et al., 2014). RGP100 contained genes for type I secretion system (T1SS) and RGP58 coded for type VI secretion system proteins that can impart a pathogenic potential to strain CR1 (Hood et al., 2010; Thomas et al., 2014). BLASTn analysis revealed that ORFs in this region had a non-Pseudomonas origin. ORFs in replacement island RGP31 had genes for O-antigen biosynthesis protein that is known to be the most variable component of the LPS (Steimle et al., 2016). It showed only 66% identity (QC: 55%; e-value: 1e-105) to its homolog present in PA7 and was more closely related to strain NCMG1179 (QC: 99%; identity: 98%). Regions like RGP99, RGP98, and RGP101 carrying transposases, partitioning proteins, and phage proteins could help in recombination and efficient transposition of the genetic elements across the genus. RGP105 had genes coding for rhizosphere expressed (rhi) gene operon which has been linked with plant-microbe interactions in Rhizobium leguminosarum (Giordano, 2015), thus supporting the environmental origin in CR1 strain. Rhizosphere expressed genes were absent in all the clinical genomes.
Genome-Scale Positive Selection Detection
A total of 5457 orthologous groups were analyzed for gene undergoing positive selection, after filtering 128 genes that were identified to exhibit significant evidence for homologous recombination (FDR < 10%). Among these, 20 genes were found to be subjected to strong selection pressure (Table 3) with ω > 1 (ω equal to the ratio of dN to dS for amino acid sites under positive selection). Genes coding for proteases, bacterial transporters, DNA replication-stabilization, and hypothetical proteins with unknown function in the P. aeruginosa outlier genomes were under positive selection (Table 3). A carbohydrate binding protein with metzincin protease and transglutaminase cysteine protease may have a role in biofilm formation and dispersal (Passmore et al., 2015; Rybtke et al., 2015). Epoxide hydrolase that has been shown to decrease mucociliary transport and restrict bacterial clearance from the lung (Hvorecny et al., 2018) was observed as undergoing positive Darwinian selection. In addition to these, Metallo-β-lactamase that can degrade β-lactam antibiotics (Palzkill, 2013) and peptidase M48 with dual nature of chaperone and metalloprotease that are believed to mediate tissue penetration and infection (Maredia et al., 2012) were also positively selected. Further, oprD porin was also found to be under positive selection, and it has been shown to carry mutations in classical strains of P. aeruginosa and has been regarded as a mosaic gene (Lynch et al., 1987; Pirnay et al., 2002) that plays a role in antibiotic resistance against carbapenems due to its reduced uptake (Li et al., 2012). Various transporters like lysine exporter, sulfate transporter, amino acid permease, and transporter for branched-chain amino acids were also positively selected (Table 3). They provide a continuous supply of amino acids to the cell and prevent starvation (Tralau et al., 2007). Although these transporters are important for maintaining adequate nutrient levels, the branched-chain amino acids (BCAA) are an important link between the metabolic state of the cell and virulence in Staphylococcus aureus by acting as co-repressors of global transcriptional regulators (Kaiser et al., 2015). These proteases and transporters have a dual role in survival and pathogenicity of these outliers and can play a key role in shaping the pathogenic potential of these strains in the course of evolution.
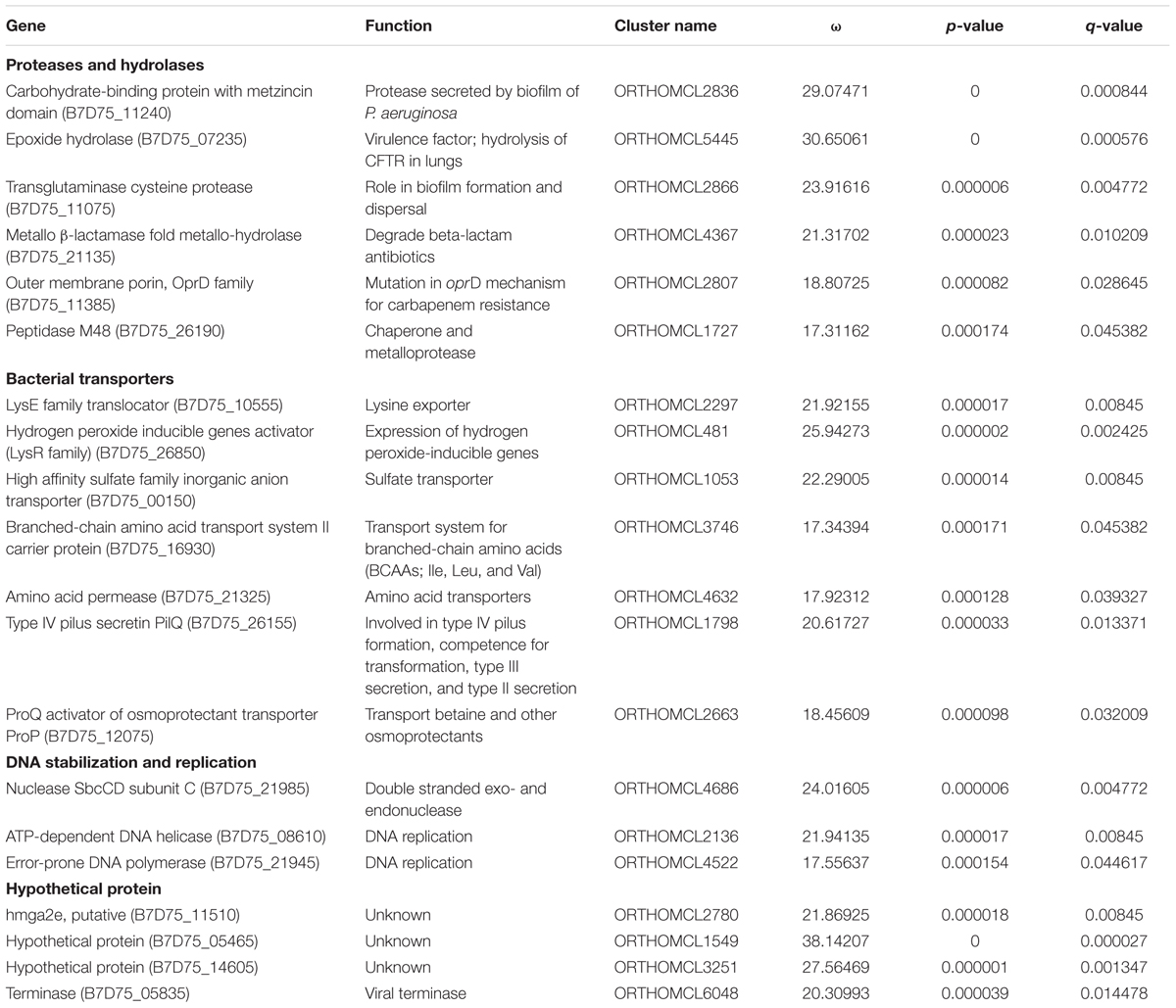
Table 3. The list of genes under positive natural selection [ω represents the value of dN/dS, p-value: probability value; q-value: false discovery rate (FDR < 10%)].
Core-Pan-Genome Dynamics and Functional Analysis
To examine the core-pan dynamics, we made three groups: outliers (n = 14), classical strains (n = 64), and all strains (n = 78). The groups were analyzed for their core genome content using OrthoMCL with 75% query coverage and sequence similarity and pangenome at default parameters in get_homologues and plotted with Tettelin fit (Figure 3) (Tettelin et al., 2005). The analysis revealed a core genome size of 4,708 genes and 10,429 non-redundant pangenes within 14 outlier genomes. This was further followed by the analysis for core and pan-genome of classical strains (n = 64). The core and pan-genome size in case of 64 classical strains were 3199 and 16705 genes, respectively, and as soon as 14 outliers were added, the core genome shrank to 2885 genes while the pangenome increased to 19736 genes, clearly signifying the expansion of pangenome. The frequency of core and accessory genes between outliers and classical strains were compared using the Chi-square test of independence, and were found to be significant [χ2(1) = 51.63, p < 0.001]. Further, the outlier genomes were more similar than the classical strains as reflected by the high prevalence of core genes in them (44.60%) vis-a-vis classical strains (19.15%). The core genome size in outliers was estimated using Tettelin fit under the assumption of 64 genomes (g = 64) for outlier group. This suggested that the core genome in case of hypothetical 64 outlier genomes (using the equation in Figure 3B) would be ∼4700 genes. This supports the observation that all the 14 genomes are highly identical to each other and the majority of the genes are conserved as core genes and adding more genomes will not decrease the core genome size drastically. On the contrary, there was a decline (9.82%) in the core genes and increase (15.35%) in the pangenes of the classical strains when all the genomes (n = 78) were subjected to analysis supporting the fact that these two groups are significantly different.
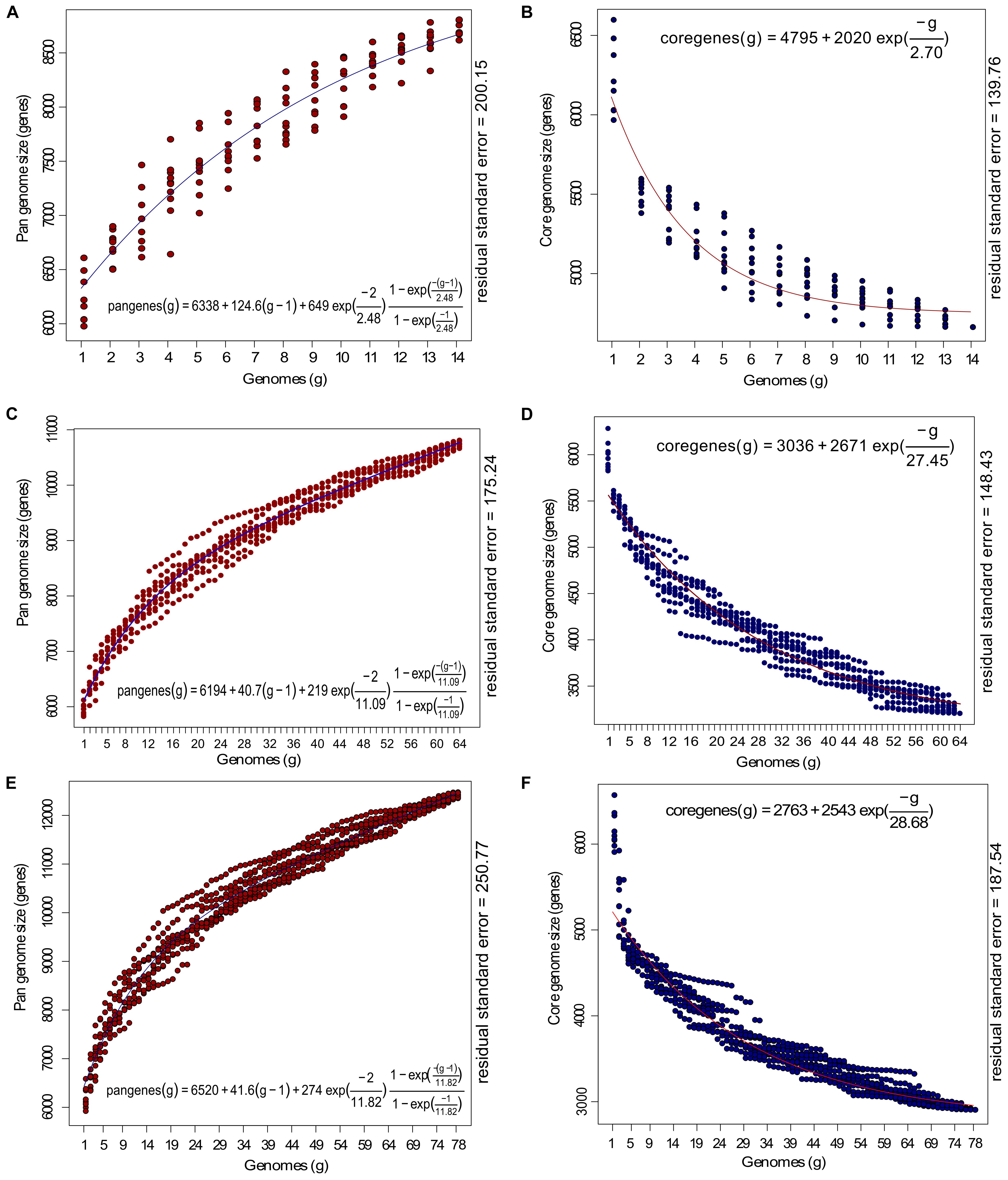
Figure 3. Estimation of core and pan-genome of P. aeruginosa strains with Tettelin fit (blue: pangenome; red: core-genome). (A,B) Represents the estimate of the pan and core genome of 14 outlier strains; (C,D) represents the estimate of core and pan-genome of 64 P. aeruginosa strains included in the current study and (E,F) represents estimate of the core and pan-genome curves for 78 genomes. The x-axis represents the number of genomes (g) while the y-axis represents the core genome and pan-genome size (number of genes). The equation for estimating pan and core-genome size according to Tettelin fit are given with the respected graphs.
The core and accessory genes in both the groups were annotated for COG (Clusters of Orthologous Groups of proteins) functional categories. The distribution of COG functional categories was remarkably different between core genes and accessory genes. In comparison to the core genome in 14 outliers, the accessory genes encoding proteins involved in replication, recombination and repair, cell cycle control, cell division, chromosome partitioning and intracellular trafficking (p-value < 0.001 and FDR < 0.03) were more in number as compared to the core genes (Figure 4A). Replication, recombination and repair, and cell motility categories had more genes in accessory and core categories, respectively, in both the outlier and classical groups. This can be attributed to the fact that these outlier and classical strains are from diverse niches and therefore possessed a large number of accessory genes for maintaining replication and cell division in various environments, at the same time, maintained a core set of genes required for motility. We also found three genes of the replication gene category to be under positive selection which can be a major cause of generation of advantageous variants for the population and make these organisms ubiquitous. However, the COG categories responsible for functions like amino acid metabolism, translation, ribosomal structure and biogenesis, energy production and conversion (p-value < 0.001 FDR < 0.03), post-translational modification, protein turnover, signal transduction mechanisms, nucleotide transport and metabolism, and cell wall biogenesis were significantly (p-value < 0.05 FDR < 0.05) more enriched in the core genes of the outliers while a higher number of accessory genes were found in almost all the COG categories of the classical strains (Figure 4B). The high abundance of gene categories in the core of the outlier genome (n = 14) reflected that they are metabolically similar amongst themselves but distinct from the classical strains.
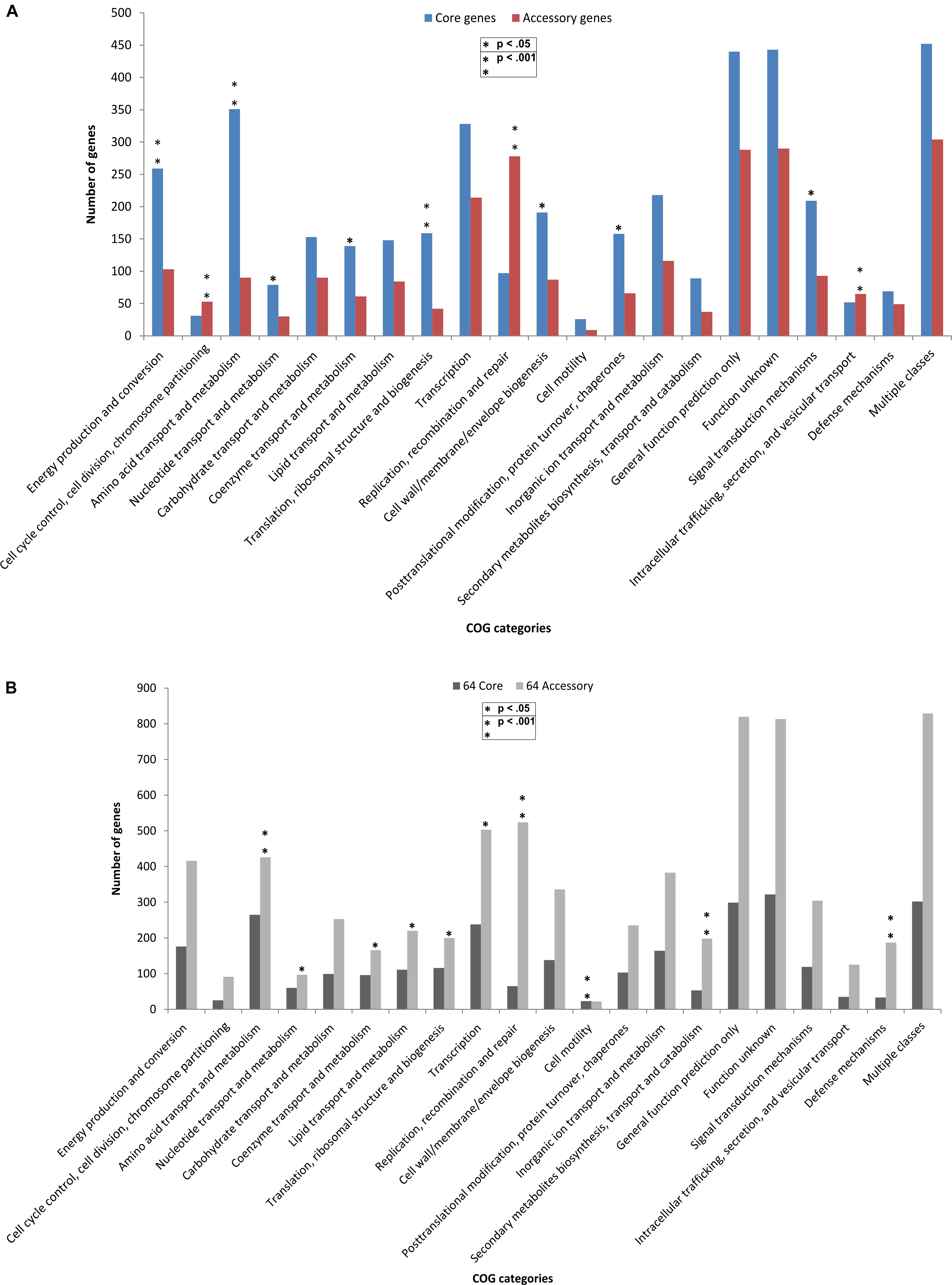
Figure 4. Comparative functional analysis based on COG categories between core and accessory genes in (A) outlier strains (n = 14) and (B) classical strains (n = 64). COG-based binning of core genes and accessory genes. The abscissa denotes different COG functional categories. The ordinate denotes the number of genes in each COG category. Four COG functional categories (RNA processing and modification, Chromatin structure and dynamics, Extracellular structures, and Cytoskeleton) including only one or without homologs in the COG collection are not displayed. Significant enrichment of gene occurrence in the individual category is marked by asterisks (∗p < 0.05, ∗∗p < 0.001; FDR < 10% Chi-square test).
Although 6276 more pangenes were present in classical strains, comparison of the pangenes in 64 classical and 14 outlier strains revealed 136 genes that were exclusively present in the outliers. Most of these genes were hypothetical. COG annotation revealed 78 genes which belonged mainly to transcription, energy production and conversion, amino acid transport and metabolism and secondary metabolites biosynthesis, transport and catabolism. These in our opinion contributed to the outlier specific genes and hence their presence could be an important parameter to characterize the outliers’ clade. All the outliers had the D-Araf biosynthetic pathway, but it was also present in Exl-A positive strain CF_PA39 (Huber et al., 2016). From the 136 genes we found the complete cluster for enzymes involved in L-Ectoine degradation viz. doeA (B7D75_20030; ectoine hydrolase; EC 3.5.4.44), doeB (B7D75_20035; N2-acetyl-L-2,4-diaminobutanoate deacetylase; EC 3.5.1.125), and doeC (B7D75_09470; aspartate-semialdehyde dehydrogenase; EC 1.2.1.M5). Many environmental isolates use ectoine as a growth substrate (Schwibbert et al., 2011) that also serves as key osmoprotectant (Yu et al., 2017). In addition to this, we found a region specific to all the outliers with genes L-Proline/Glycine betaine transporter (ProP) (B7D75_13890), nitrilase (B7D75_13895), cytochrome c (B7D75_13900) and cytochrome d1 nitrite reductase (B7D75_13905) that help bacteria to cope with osmotic stress in environment (Chen and Beattie, 2008), catalyzes the hydrolysis of nitriles to carboxylic acid and ammonia without formation of free amides, and have roles in hormone synthesis, nutrient assimilation and detoxification of exogenous and endogenous nitriles (Howden and Preston, 2009; Raczynska et al., 2011). All these characteristics were specific to outliers and were absent in the classical strains of P. aeruginosa. In addition to being the group-specific genes for the outliers, the presence of these genes also pointed out that the outliers as a group are very distinct in their functional attributes and possess many genes which confer an advantage in surviving under environmental stress.
For deciphering the functional disparities in outliers, the genomes (n = 14) were annotated using KEGG orthology and subjected to pathway reconstruction by MinPath. The functional analysis revealed the differential abundance of genes encoding Type IV secretion system, nucleotide sugars metabolism, ABC transporters and pathways linked to energy production and metabolism based on the minimal set of pathways (Figure 5A). This observational difference suggests different metabolic preferences of P. aeruginosa outlier strains. Further, T3SS was completely missing- suggesting the genotypic validation for outliers as they were reported not to harbor T3SS (Roy et al., 2010). Interestingly, the analysis based on minimal pathways clearly indicated that CR1 was in the clade of PA7; thus suggesting that common complete metabolic pathways exist in both the strains. However, the clustering in case of COG categories generated a topology similar to the phylogenetic analysis where the two genomes were present in different sub-clades (Figure 5B).
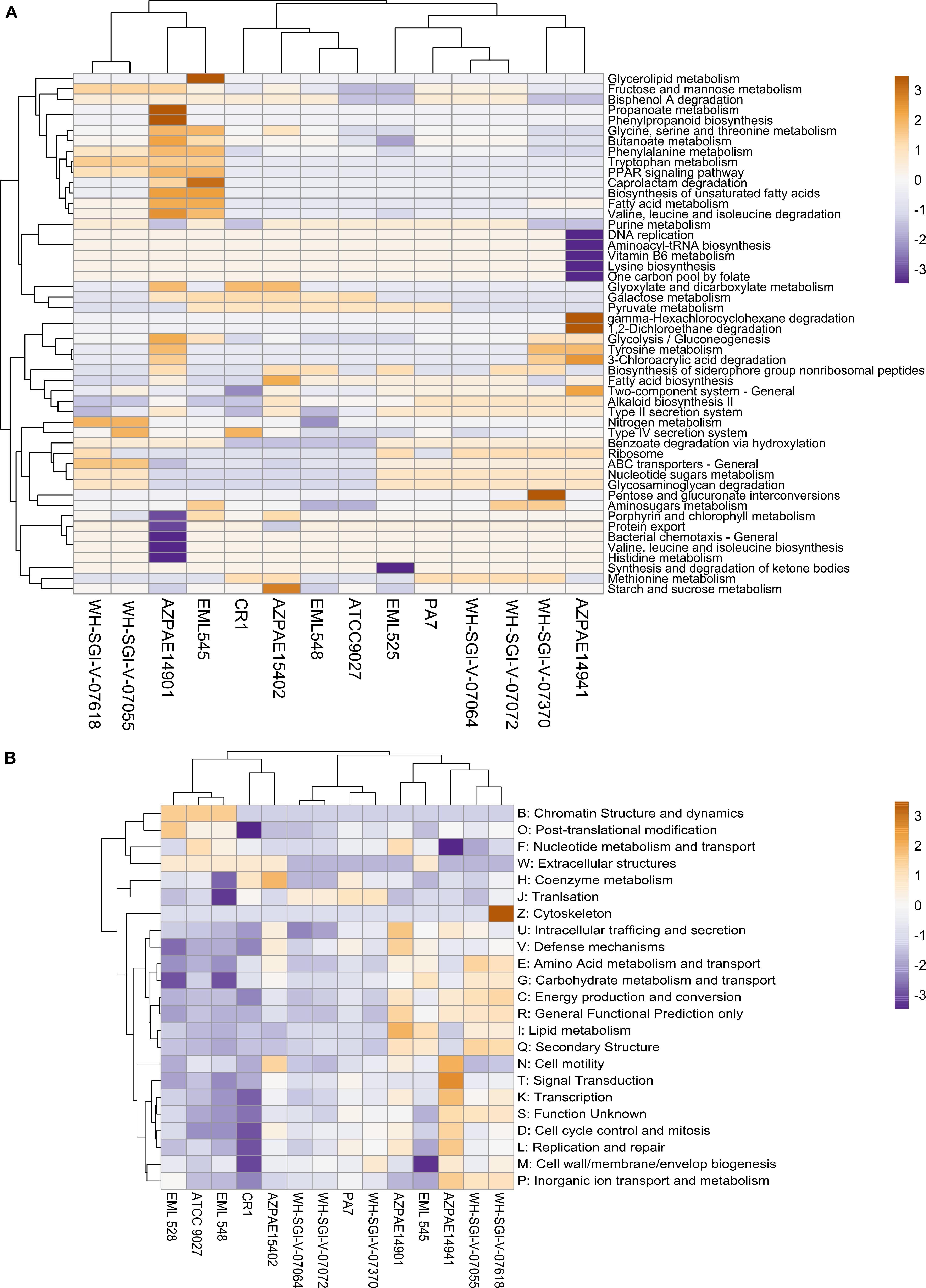
Figure 5. Functional analysis of Pseudomonas outlier strains. (A) The amino acid sequences of all the 14 genomes were assigned KO number by KAAS server. The protein families were then mapped for a minimal set of pathways using a parsimony approach. The heat map was constructed based on most abundant top 50 subsystems using Pearson correlation. (B) The ORF’s were mapped against the COG database using rpsblast. For both the heatmaps rows are centered; unit variance scaling is applied to rows. Both rows and columns are clustered using correlation distance and average linkage.
Virulence Genotype
All the outlier strains (n = 14) were found to be highly identical (98.5–99.5%). CR1 strain possessed all the genes required for flagella formation, type IV pili biosynthesis, type IV pili twitching motility, alginate biosynthesis and regulation but lacked a toxA gene similar to PA7. It also harbored the exlA (B7D75_20920) and exlB (B7D75_20915) required for the formation of exolysin A. Apart from the similarity in the genetic repertoire of most of the flagellar assembly genes, strain CR1 possessed flaB gene (B7D75_19585) instead of flaC gene (PSPA7_4279). These two genes have higher similarity at their N-terminus and comparatively lesser similarity at the C-terminus with an overall query coverage and percentage similarity of 85 and 63%, respectively. Three more outlier strains viz., EML545, WH-SGI-V-07055, and WH-SGI-V-07618 had the same genotype and rest of the outliers had flaC gene as in strain PA7 indicating this locus to be distinct in comparison to other genes required for flagella biosynthesis. Recently flaC locus from Campylobacterales was reported to have a high intrinsic property to activate the innate immune response (Faber et al., 2016). Genes coding for LPS-O antigen in CR1 lacks three genes UDP-N-acetylglucosamine 2-epimerase (EC 5.1.3.14; PSPA7_1971), UDP-glucose 4-epimerase (EC 5.1.3.2; PSPA7_1984), and Undecaprenyl-phosphate N-acetylglucosaminyl 1-phosphate transferase (EC 2.7.8-; PSPA7_1985) that can lead to strain-specific lipopolysaccharide in the cell wall of CR1 strain.
Phenazines are key virulence factors that are characteristic of P. aeruginosa. Outliers (n = 14) had fused phzA1 with phzB1 and this can be used as a marker gene to distinguish an outlier strain from the classical strains. Our analysis to identify outlier specific genes from the core-genes which were present in all the 14 outliers and absent from 64 classical strains also confirmed that all the 14 outliers had the fused phzA1 and phzB1 (B7D75_03695). Strain CR1 and PA7 were found to harbor complete operons phzB1-phzG1 (B7D75_03695-03670); phzA2-phzG2 (B7D75_15445-15415), phzM (B7D75_03705), and phzS gene (B7D75_03660) but lacked phzH. The fused gene does not alter phenazine production, and strain CR1 produced the characteristic blue color pigment in the culture broth which is indicative of the production of pyocyanin. Further, all the outliers barring EML528 had the complete operon pvsA-pvsE (B7D75_14020- B7D75_14040) and vibrioferrin receptor pvuA (B7D75_14015) that are required for the production of vibrioferrin, a siderophore; which was also reported in agriculturally important Azotobacter vinelandii and was previously reported only in marine bacteria (Baars et al., 2016). This may in part explain why the CR1 strain has been known to have the ability to promote the growth of plants (data not presented here). The entire cluster of vibrioferrin was absent in classical strains like PAO1 and UCBPP-PA14. Recently vibrioferrin has been reported in P. fragi (Stanborough et al., 2017) but so far vibrioferrin production has not been reported in P. aeruginosa. This indicates that all the outliers possess extra siderophore producing genes that are absent in classical strains. This suggests the acquisition of this gene cluster by horizontal gene transfer from other strains of Pseudomonas spp. and other bacteria in soil. The genes for pyoverdine were also present in all strains. PA7 is known to produce type II pyoverdine and has the characteristic 966-bp coding region that contained an esterase/lipase protein domain (PSPA7_2860) in strain CR1 (B7D75_13235) (Smith et al., 2005). However, the ferripyoverdine receptor (fpvA) was of the type IIb in all strains. Interestingly strain CR1 lacked both hemolytic phospholipase C (plcH) and phospholipase D (pldA). Rest all factors for quorum sensing systems, regulation by GacS/GacA two-component systems and hydrogen cyanide production showed complete synteny to all the complete genomes of P. aeruginosa.
The probability of being a pathogen depicting the pathogenic potential of the outliers was in the range of 62–68% among the 14 strains, lowest being for ATCC9027 and EML548 and highest for PA7, WH-SGI-V-07072, and WH-SGI-V-07370. The pathogenicity potential of strain CR1 (66.0%) was significantly higher [χ2(1) = 10.24, p < 0.005]. The number of pathogenic families annotated in the genome was highest in strain PA7 with input proteome coverage 15.72% and 757 pathogenic families and lowest in case of strain ATCC9027 (Table 4). The principal component analysis that is used to visualize genetic distance and relatedness were performed using four parameters (probability of being a pathogen, input proteome coverage, matched pathogenic family and matched non-pathogenic families) among the 14 genomes. The PCA bi-plot (Figure 6) clearly showed that strain PA7, WH-SGI-V-07370, WH-SGI-V-07064, and WH-SGI-V-07072 formed a distinct compact cluster, separate from other strains indicating them to be more pathogenic than other outlier strains. Interestingly even among the outliers, CR1 clustered in the group of less virulent strains represented by strain ATCC9027 possibly due to its non-clinical origin and less pathogenic proteins. Although pathogenicity is context dependent and strains of PA7 clade have been shown to be avirulent in mammalian infection models but were pathogenic in invertebrate and plant infection models (Hilker et al., 2015). Insights into the pathogenic protein families clearly indicated that these strains were less pathogenic when compared to the strains of classical clade having a higher probability of being a pathogen in the range of 70.8–76.4% (Supplementary Table S5) but have a significant number of pathogenic families in their genomes.
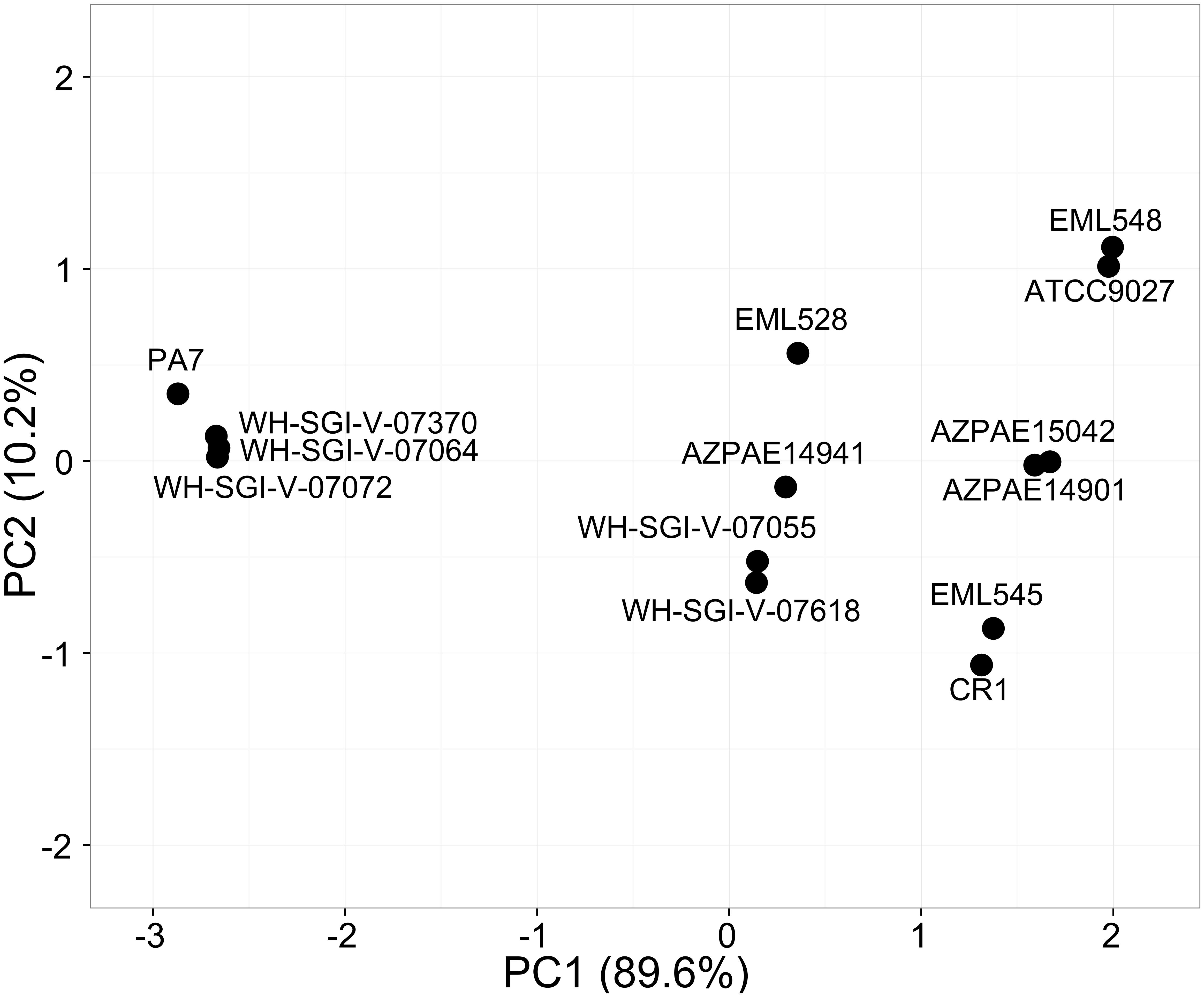
Figure 6. PCA analysis on pathogenic potential of P. aeruginosa outlier strains. The PCA- analysis was performed on the four parameters namely number of pathogenic and non-pathogenic families annotated, probability of being a pathogen and input proteome percentage among the 14 strains. PA7 along with WH-SGI-V-07370, WH-SGI-V-07064, and WH-SGI-V-07072 clustered in a different quadrant from the other strains showing high pathogenicity.
Antibiotic Resistance of Outlier Strains
Resfinder-v2.1 predicted the presence of acquired resistance genes for β-lactam resistance, aminoglycoside resistance, phenicol resistance, and sulphonamide resistance. Interestingly, all the strains (n = 14) including strain CR1 possessed complete genetic repertoire (blaOXA-50 (B7D75_28665), blaPAO (B7D75_04145) genes with all the associated regulators and activators) required for β-lactam resistance providing evidence for pan -β-lactam resistance development in P. aeruginosa (Moya et al., 2012). Further, all outliers (n = 14) harbored aph(3’)-IIb (B7D75_04095) that confers resistance to several important aminoglycoside antibiotics, including kanamycin A and B, neomycin B and C, butirosin and seldomycin F5 (Zeng and Jin, 2003). The genes reported imparting chloramphenicol resistance were searched in the genome of CR1 strain. CR1 had catA gene (B7D75_19145) but had frameshift in the catB7 gene (B7D75_21755), and cmx was exclusive to PA7 as a gene in RGP75 (Roy et al., 2010). catB7 is an effector of chloramphenicol resistance (White et al., 1999). Only WH-SGI-V-07072 and WH-SGI-V-07370 along with PA7 showed the presence of strA, strB, sul1 genes for aminoglycoside and sulphonamide resistance. Further, aadB (2″-aminoglycoside nucleotidyltransferase) was identified only in the genomes of WH-SGI-V-07072 and WH-SGI-V-07370 and was not present in any other genome including the complete genomes of CR1 and PA7.
PA7 has been exclusively known to possess amikacin resistance due to the presence of AAC4 gene and its product is an AAC (6′)-II constituted in the RGP75, but our analysis revealed that the gene is not exclusive to PA7 and these are also present in strain WH-SGI-V-07055. The genes exclusive to PA7 were aph(6)-Ic aph(3′)-IIa in addition to cmx gene. Interestingly, strains CR1, ATCC9027, AZPAE14901, AZPAE15042, EML545, and EML548 lack the arnBCAD operon (present in PA7, PAO1 and UCBPP-PA14 and remaining outliers) required for resistance to polymyxin B and cationic antimicrobial peptides.
Point mutations in gyrA (Thr83Ile) and parC (Ser87Leu) known to be associated with Fluoroquinolone (FQ) resistance were checked by aligning both these genes among the 14 strains. Strains WH-SGI-V-07064, WH-SGI-V-07072 and the known multi-drug resistant strain PA7 had these mutations. Strain CR1 along with the remaining 10 strains showed susceptible genotype for Fluoroquinolones.
All the genomes had intact mexABOprM (B7D75_02135-02140-02145), mexCDOprJ (B7D75_23705-23700-23695), mexEFOprN (B7D75_12715-12710-12705) and its regulator mexT (B7D75_12720). CR1 had the mexXYOprA with oprA (B7D75_14890) gene linked to mexXY (B7D75_14880-14885) as in the genome of PA7. The multidrug efflux system was ubiquitously present in all the genomes. The analysis revealed that majority of outlier strains including CR1 possessed a reduced number of acquired resistant genes and is susceptible to a certain antibiotic(s), however, strains PA7, WH-SGI-V-07072, and WH-SGI-V-07370 were most pathogenic and resistant to majority of the antibiotics.
Protein–Protein Interactions
The core amino acid sequences of the classical (n = 3199) and outlier (n = 4708) genomes were used for constructing complex PPI network using STRING Database v10.5 (Szklarczyk et al., 2017). The outlier strains showed five major hubs namely gltB (glutamate synthase), PA1400 (pyruvate carboxylase), polA (DNA polymerase I), gacS (histidine kinase) and guaA (GMP synthase) whereas the classical strains had gltB, dnaK (chaperone protein), guaB (inosine 5′-monophosphate dehydrogenase), hemE (uroporphyrin decarboxylase) and rpoA (DNA-directed RNA polymerase) as the major regulatory hubs. gltB emerged to be the major regulator having the highest degree (d) in outliers (d = 239) as well as the classical strains (d = 127); whereas, rest of the regulatory hubs were unique for both the groups (Figure 7 and Table 5). The difference in the degree of association in both the groups can be attributed to higher overall nucleotide relatedness among outlier strains than between the members of the outlier and classical clades. Outliers had more genes in the core genome and therefore gltB had a higher degree of association in outlier PPI network. The gene gltB that codes for glutamate synthase [NADPH] large chain (EC 1.4.1.13), engaged in L-glutamate biosynthesis via GLT pathway (Pahel et al., 1978), which is itself part of amino acid biosynthesis has a role in the formation of enzymes involved in nitrogen assimilation (Janssen et al., 1981) and enhancing the yield of exotoxin A (Somerville et al., 1999). Further, two studies have shown that the gltB gene in concert with glutamine synthase (glnA) is a potential target for drug development (Mowbray et al., 2014; Murima et al., 2014). Interestingly, all the five hubs in case of outliers were connected to each other in sequential order according to the degree of interactions. However, in the classical strains, there was no interaction between gltB and the second prominent regulator dnaK which itself interacted with the next three hubs (Figure 7). Therefore, gltB can be regarded as the master of the proteome network with dnaK also playing an important role as it is the second most prominent regulatory hub (d = 67). Thus, targeting both these genes gltB and dnaK) can have a better impact against their pathogenesis (Chiappori et al., 2015). Therefore, in classical strains, a combination of drugs targeting both these regulatory hubs can prove to be more effective against multi-drug resistance. Similarly, the remaining major regulatory (Figure 7) protein hubs of the outliers can also be targeted as drug targets. Although these are considered as strains of the same species, the regulatory hub differs with gltB to be the major regulator.
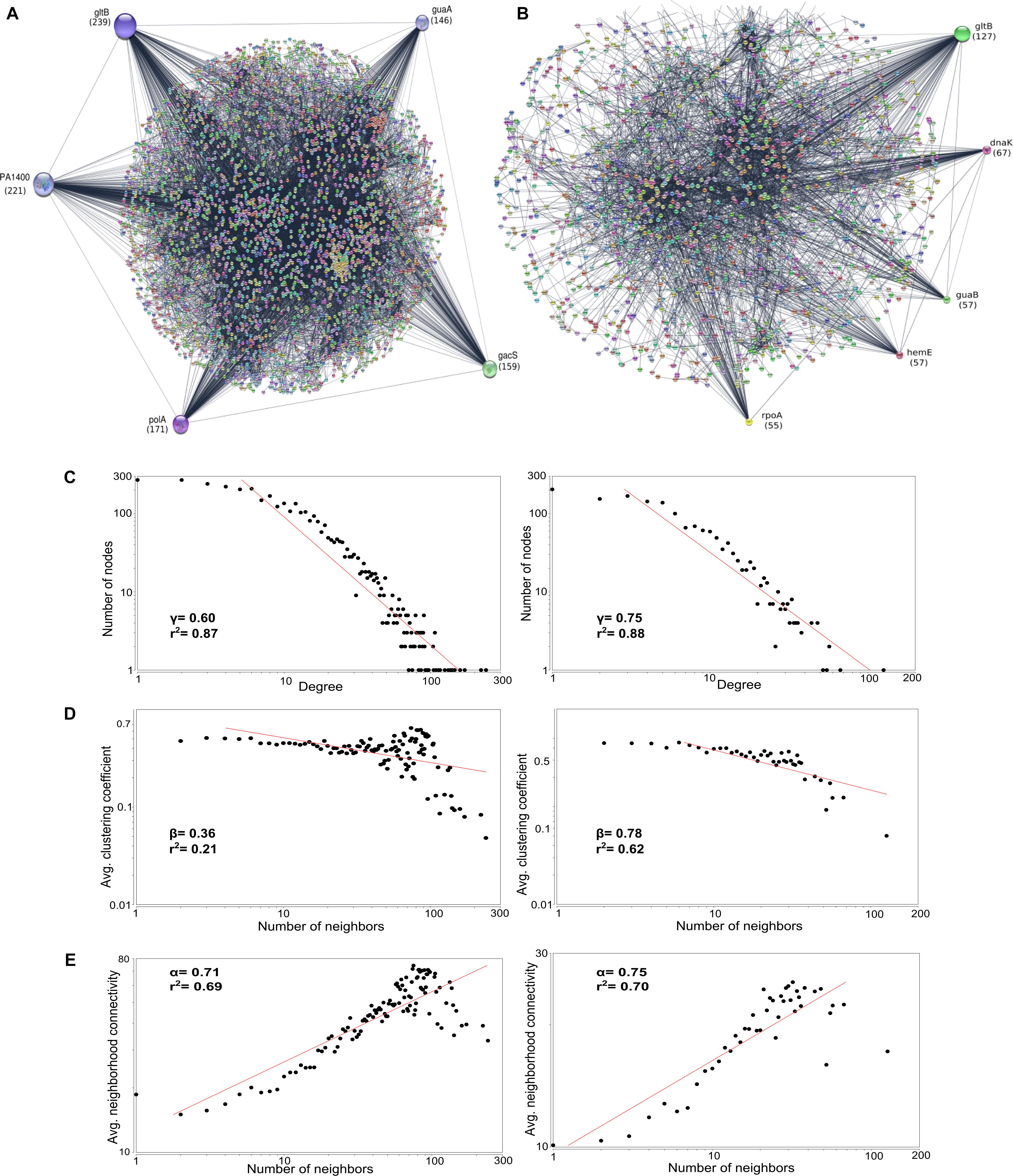
Figure 7. Protein–Protein Interaction network of (A) Core genes of outlier strains (n = 14) and (B) Core genes of classical strains (n = 64). The circles represent protein hubs and line represent edges. The radius of major regulatory hubs decreases as the number of interactions decreases. The topological properties of these networks depicting the correlation coefficient values (r2). (C) Node degree distribution, (D) average clustering coefficient, (E) average neighborhood connectivity. All these properties follow the power distribution and show the nature of the scale-free network and hierarchical organization.
The network followed a power scaling behavior as the degree exponent γ were 0.60 and 0.75, and the value was less than 2 (Barabasi and Oltvai, 2004) signifying the emergence of hierarchical modules and/or communities (Ravasz et al., 2002). Few highly connected hubs were connected to many low degree hubs indicating the regulatory power of the hubs over these nodes. Further, the average clustering coefficient and average neighborhood connectivity were calculated and it also followed power scaling law with β values of 0.36 and 0.78 and α values of 0.71 and 0.75 in outlier and classical strains, respectively, confirming that the network was hierarchical and the hub proteins in each network are indicative of the key molecules for habitat adaptation in each genome of the respective groups.
Conclusion
Represented by very few genomic sequences, clinical strains of P. aeruginosa outlier group have shown to harbor novel virulence factors. We report here a non-clinical outlier strain CR1 characterized by a reduced genome size lacking few virulent factors found in clinical strains, the presence of a new type I secretion system from the non-Pseudomonas origin in RGP100 and a plasmid bearing trimeric autotransporter linked with VirB/D4 type IV secretion system. CR1 strain has genes responsible for siderophore production that is important in plants ability to acquire iron. We observed that outliers can be distinguished into 2 sub-clades one represented by CR1 having increased antibiotic susceptibility and other by strain PA7 having multidrug-resistant phenotypes. The genomes of the outliers were significantly more conserved than that of the classical clinical strains due to the high prevalence of core genes thus strengthening the fact that the two groups are significantly different. Outlier strains had genes normally found in soil-dwelling bacteria like ectoine degradation, plant-induced nitrilase in their pangenome that were absent in the classical strains. Although the outliers are considered as the strain of same species, they show major ambiguities in virulence factors, antibiotic resistance pattern, and protein regulatory hubs when compared with classical strains.
Data Availability
The complete genome sequence of chromosome and plasmid of CR1 were deposited into the GenBank database with Accession number CP020560.1 and CP020561.2. The genome of CR1 was also submitted to PubMLST database with organism id 6661 for assigning new sequence type.
Author Contributions
US, MS, and RL planned the study. DR provided the strain. US and PH did the experimental work for genomic DNA isolation and quantification. US, PH, RK, and AB did the computational analysis. All authors were involved in the writing and improving of the manuscript.
Funding
This work was supported by grants from the All India Network Project on Soil Biodiversity-Biofertilizers by Indian Council of Agricultural Research (ICAR) and Department of Biotechnology (DBT), New Delhi.
Conflict of Interest Statement
The authors US, RK and RL were employed by the company PhiXGen Private Limited.
The remaining authors declare that the research was conducted in the absence of any commercial or financial relationships that could be construed as a potential conflict of interest.
Acknowledgments
US, PH, RK, and AB gratefully acknowledge the University Grants Commission (UGC), Council for Scientific and Industrial Research (CSIR), DBT and UGC-DS Kothari Scheme for providing research fellowship.
Supplementary Material
The Supplementary Material for this article can be found online at: https://www.frontiersin.org/articles/10.3389/fmicb.2019.00053/full#supplementary-material
FIGURE S1 | 3-D scatterplot of genome size (x-axis), number of coding sequences (y-axis) and DDH values (z-axis) among 14 outlier strains.
FIGURE S2 | Heatmap with dual dendrogram based on the average nucleotide identity. The strains were compared using ANIm (mummer based) at default parameters. The matrix was then was plotted as a dual dendrogram in R (R Development Core Team, 2015).
FIGURE S3 | Phylogeny based on 400 conserved marker genes using the maximum likelihood method. Azotobacter vinelandii CA6 was taken as outgroup.
FIGURE S4 | Phylogenetic tree of 78 P. aeruginosa genomes based on SNPs within the core genome was defined using Parsnp tool of the Harvest suite.
FIGURE S5 | Circos representation of the whole genome alignment in strain CR1 and PA7 with a window size of 10 kb. Colors of arcs depict the orientation of the blocks (green: positive strand; red: negative strand).
FIGURE S6 | Representation of new RGPs (RGP98-105) of CR1 against the type strain PA7. (A) RGP98, (B) RGP99, (C) RGP100, (D) RGP101, (E) RGP102, (F) RGP103, (G) RGP104, (H) RGP105.
TABLE S1 | Soil properties of the isolation source of CR1 (rhizosphere soil of chili plantation).
TABLE S2 | Details of all the 78 strains of P. aeruginosa.
TABLE S3 | Annotation of environmental phage exclusive to CR1 as predicted by PHAST server.
TABLE S4 | Complete list of regions of genomic plasticity in P. aeruginosa.
TABLE S5 | Pathogenic potential of classical strains predicted by PathogenFinder.
FILE S1 | Configuration file used in POTION pipeline.
Footnotes
References
Albert, R., and Barabasi, A. L. (2002). Statistical mechanics of complex networks. Rev. Mod. Phys. 74, 47–97. doi: 10.1103/RevModPhys.74.47
Almaas, E. (2007). Biological impacts and context of network theory. J. Exp. Biol. 210, 1548–1558. doi: 10.1242/jeb.003731
Altschul, S. F., Gish, W., Miller, W., Myers, E. W., and Lipman, D. J. (1990). Basic local alignment search tool. J. Mol. Biol. 215, 403–410. doi: 10.1016/S0022-2836(05)80360-2
Auch, A. F., Klenk, H. P., and Göker, M. (2010a). Standard operating procedure for calculating genome-to-genome distances based on high-scoring segment pairs. Stand. Genomic Sci. 2, 142–148. doi: 10.4056/sigs.541628
Auch, A. F., Jan, M., Klenk, H. P., and Göker, M. (2010b). Digital DNA-DNA hybridization for microbial species delineation by means of genome-to-genome sequence comparison. Stand. Genomic Sci. 2, 117–134. doi: 10.4056/sigs.531120
Aziz, R. K., Bartels, D., Best, A. A., DeJongh, M., Disz, T., Edwards, R. A., et al. (2008). The RAST server, rapid annotations using subsystems technology. BMC Genomics 9:75. doi: 10.1186/1471-2164-9-75
Baars, O., Zhang, X., Morel, F. M., and Seyedsayamdost, M. R. (2016). The siderophore metabolome of Azotobacter vinelandii. Appl. Environ. Microbiol. 82, 27–39. doi: 10.1128/AEM.03160-15
Barabasi, A. L., and Oltvai, Z. N. (2004). Network biology: understanding the cell’s functional organization. Nat. Rev. Genet. 5, 101–113. doi: 10.1038/nrg1272
Basso, P., Ragno, M., Elsen, S., Reboud, E., Golovkine, G., Bouillot, S., et al. (2017). Pseudomonas aeruginosa pore-forming exolysin and type IV pili cooperate to induce host cell lysis. mBio 8:e02250-16. doi: 10.1128/mBio.02250-16
Benjamini, Y., and Yekutieli, D. (2001). The control of the false discovery rate in multiple testing under dependency. Ann. Stat. 29, 1165–1188. doi: 10.1186/1471-2105-9-114
Boukerb, A. M., Décor, A., Ribun, S., Tabaroni, R., Rousset, A., Commin, L., et al. (2016). Genomic rearrangements and functional diversification of lecA and lecB lectin-coding regions impacting the efficacy of glycomimetics directed against Pseudomonas aeruginosa. Front. Microbiol. 7:811. doi: 10.3389/fmicb.2016.00811
Boukerb, A. M., Marti, R., and Cournoyer, B. (2015). Genome sequences of three strains of the Pseudomonas aeruginosa PA7 clade. Genome Announc. 3:e01366-15. doi: 10.1128/genomeA.01366-15
Bruen, T., and Bruen, T. (2005). PhiPack: PHI test and other Tests of Recombination. Montreal: McGill University.
Buch, A., Archana, G., and Kumar, G. N. (2008). Metabolic channeling of glucose towards gluconate in phosphate-solubilizing Pseudomonas aeruginosa P4 under phosphorus deficiency. Res. Microbiol. 159, 635–642. doi: 10.1016/j.resmic.2008.09.012
Capella-Gutiérrez, S., Silla-Martínez, J. M., and Gabaldón, T. (2009). trimAl: a tool for automated alignment trimming in large-scale phylogenetic analyses. Bioinformatics 25, 1972–1973. doi: 10.1093/bioinformatics/btp348
Chandler, M., De La Cruz, F., Dyda, F., Hickman, A. B., Moncalian, G., and Ton-Hoang, B. (2013). Breaking and joining single-stranded DNA: the HUH endonuclease superfamily. Nat. Rev. Microbiol. 11, 525–538. doi: 10.1038/nrmicro3067
Che, D., Hasan, M. S., and Chen, B. (2014). Identifying pathogenicity islands in bacterial pathogenomics using computational approaches. Pathogens 3, 36–56. doi: 10.3390/pathogens3010036
Chen, C., and Beattie, G. A. (2008). Pseudomonas syringae BetT is a low-affinity choline transporter that is responsible for superior osmoprotection by choline over glycine betaine. J. Bacteriol. 190, 2717–2725. doi: 10.1128/JB.01585-07
Chen, L., Yang, J., Yu, J., Yao, Z., Sun, L., Shen, Y., et al. (2005). VFDB: a reference database for bacterial virulence factors. Nucleic Acids Res. 33, D325–D328. doi: 10.1093/nar/gki008
Chiappori, F., Fumian, M., Milanesi, L., and Merelli, I. (2015). DnaK as antibiotic target: hot spot residues analysis for differential inhibition of the bacterial protein in comparison with the human HSP70. PLoS One 10:e0124563. doi: 10.1371/journal.pone.0124563
Chin, C. S., Alexander, D. H., Marks, P., Klammer, A. A., Drake, J., Heiner, C., et al. (2013). Nonhybrid, finished microbial genome assemblies from long-read SMRT sequencing data. Nat. Methods 10, 563–569. doi: 10.1038/nmeth.2474
Christie, P. J. (2001). Type IV secretion: intercellular transfer of macromolecules by systems ancestrally related to conjugation machines. Mol. Microbiol. 40, 294–305. doi: 10.1046/j.1365-2958.2001.02302.x
Contreras-Moreira, B., and Vinuesa, P. (2013). GET_HOMOLOGUES: a versatile software package for scalable and robust microbial pangenome analysis. Appl. Environ. Microbiol. 79, 7696–7701. doi: 10.1128/AEM.02411-13
Cornelis, G. R. (2006). The type III secretion injectisome. Nat. Rev. Microbiol. 4, 811–825. doi: 10.1038/nrmicro1526
Cosentino, S., Larsen, M. V., Aarestrup, F. M., and Lund, O. (2013). PathogenFinder-distinguishing friend from foe using bacterial whole genome sequence data. PLoS One 8:e77302. doi: 10.1371/journal.pone.0077302
Delcher, A. L., Bratke, K. A., Powers, E. C., and Salzberg, S. L. (2007). Identifying bacterial genes and endosymbiont DNA with Glimmer. Bioinformatics 23, 673–679. doi: 10.1093/bioinformatics/btm009
Dupont, C. L., Rusch, D. B., Yooseph, S., Lombardo, M. J., Richter, R. A., Valas, R., et al. (2012). Genomic insights to SAR86, an abundant and uncultivated marine bacterial lineage. ISME J. 6, 1186–1199. doi: 10.1038/ismej.2011.189
Elsen, S., Huber, P., Bouillot, S., Couté, Y., Fournier, P., Dubois, Y., et al. (2014). A type III secretion negative clinical strain of Pseudomonas aeruginosa employs a two-partner secreted exolysin to induce hemorrhagic pneumonia. Cell Host Microbe 15, 164–176. doi: 10.1016/j.chom.2014.01.003
Faber, E., Gripp, E., Maurischat, S., Kaspers, B., Tedin, K., Menz, S., et al. (2016). Novel immunomodulatory flagellin-like protein FlaC in Campylobacter jejuni and other Campylobacterales. mSphere 1:e00028-15. doi: 10.1128/mSphere.00028-15
Fang, Y., Shane, T., Wu, F., Williams, C., and Miller, C. (2010). The structure and transport mechanism of AdiC-an arginine/agmatine antiporter. Biophys. J. 98:418a. doi: 10.1016/j.bpj.2009.12.2259
Freschi, L., Jeukens, J., Kukavica-Ibrulj, I., Boyle, B., Dupont, M. J., Laroche, J., et al. (2015). Clinical utilization of genomics data produced by the international Pseudomonas aeruginosa consortium. Front. Microbiol. 6:1036. doi: 10.3389/fmicb.2015.01036
Fronzes, R., Christie, P. J., and Waksman, G. (2009). The structural biology of type IV secretion systems. Nat. Rev. Microbiol. 7, 703–714. doi: 10.1038/nrmicro2218
Ghasempur, S., Eswaramoorthy, S., Hillerich, B. S., Seidel, R. D., Swaminathan, S., Almo, S. C., et al. (2014). Discovery of a novel l-lyxonate degradation pathway in Pseudomonas aeruginosa PAO1. Biochemistry 53, 3357–3366. doi: 10.1021/bi5004298
Giordano, W. (2015). “Rhizobial extracellular signaling molecules and their functions in symbiotic interactions with legumes,” in Quorum Sensing vs Quorum Quenching: A Battle with No End in Sight, ed. V. Kalia (New Delhi: Springer).
Girvan, M., and Newman, M. E. (2002). Community structure in social and biological networks. Proc. Natl. Acad. Sci. U.S.A. 99, 7821–7826. doi: 10.1073/pnas.122653799
Gomila, M., Pena, A., Mulet, M., Lalucat, J., and García-Valdés, E. (2015). Phylogenomics and systematics in Pseudomonas. Front. Microbiol. 6:214. doi: 10.3389/fmicb.2015.00214
Grant, J. R., and Stothard, P. (2008). The CGView Server: a comparative genomics tool for circular genome. Nucleic Acids Res. 36, W181–W184. doi: 10.1093/nar/gkn179
Grissa, I., Vergnaud, G., and Pourcel, C. (2007). CRISPRFinder, a web tool to identify clustered regularly interspaced short palindromic repeats. Nucleic Acids Res. 35, 52–57. doi: 10.1093/nar/gkm360
Guindon, S., and Gascuel, O. (2003). A simple, fast, and accurate algorithm to estimate large phylogenies by maximum likelihood. Syst. Biol. 52, 696–704. doi: 10.1080/10635150390235520
Gupta, V., Haider, S., Sood, U., Gilbert, J. A., Ramjee, M., Forbes, K., et al. (2016). Comparative genomic analysis of novel Acinetobacter symbionts: a combined systems biology and genomics approach. Sci. Rep. 6:29043. doi: 10.1038/srep29043
Hauser, A. R. (2009). The type III secretion system of Pseudomonas aeruginosa: infection by injection. Nat. Rev. Microbiol. 7, 654–665. doi: 10.1038/nrmicro2199
Hilker, R., Munder, A., Klockgether, J., Losada, P. M., Chouvarine, P., Cramer, N., et al. (2015). Interclonal gradient of virulence in the Pseudomonas aeruginosa pangenome from disease and environment. Environ. Microbiol. 17, 29–46. doi: 10.1111/1462-2920.12606
Hongo, J. A., Castro, G. M., Cintra, L. C., Zerlotini, A., and Lobo, F. P. (2015). POTION: an end-to-end pipeline for positive Darwinian selection detection in genome-scale data through phylogenetic comparison of protein-coding genes. BMC Genomics 16:567. doi: 10.1186/s12864-015-1765-0
Hood, R. D., Singh, P., Hsu, F., Güvener, T., Carl, M. A., Trinidad, R. R., et al. (2010). A type VI secretion system of Pseudomonas aeruginosa targets a toxin to bacteria. Cell Host Microbe 7, 25–37. doi: 10.1016/j.chom.2009.12.007
Howden, A. J., and Preston, G. M. (2009). Nitrilase enzymes and their role in plant-microbe interactions. Microb. Biotechnol. 2, 441–451. doi: 10.1111/j.1751-7915.2009.00111.x
Huber, P., Basso, P., Reboud, E., and Attrée, I. (2016). Pseudomonas aeruginosa renews its virulence factors. Environ. Microbiol. Rep. 8, 564–571. doi: 10.1111/1758-2229.12443
Hvorecny, K. L., Dolben, E., Moreau-Marquis, S., Hampton, T. H., Shabaneh, T. B., Flitter, B. A., et al. (2018). An epoxide hydrolase secreted by Pseudomonas aeruginosa decreases mucociliary transport and hinders bacterial clearance from the lung. Am. J. Physiol. Lung Cell. Mol. Physiol. 314, L150–L156. doi: 10.1152/ajplung.00383.2017
Jani, M., Mathee, K., and Azad, R. K. (2016). Identification of novel genomic islands in Liverpool epidemic strain of Pseudomonas aeruginosa using segmentation and clustering. Front. Microbiol. 7:1210. doi: 10.3389/fmicb.2016.01210
Janssen, D. B., Herst, P. M., Joosten, H. M., and van der Drift, C. (1981). Nitrogen control in Pseudomonas aeruginosa: a role for glutamine in the regulation of the synthesis of NADP-dependent glutamate dehydrogenase, urease and histidase. Arch. Microbiol. 128, 398–402. doi: 10.1007/BF00405920
Jolley, K. A., Bray, J. E., and Maiden, M. C. J. (2018). Open-access bacterial population genomics: BIGSdb software, the PubMLST.org website and their applications. Wellcome Open Res. 3:124. doi: 10.12688/wellcomeopenres.14826.1
Kaiser, J. C., Omer, S., Sheldon, J. R., Welch, I., and Heinrichs, D. E. (2015). Role of BrnQ1 and BrnQ2 in branched-chain amino acid transport and virulence in Staphylococcus aureus. Infect. Immun. 83, 1019–1029. doi: 10.1128/IAI.02542-14
Kleinheinz, K. A., Joensen, K. G., and Larsen, M. V. (2014). Applying the ResFinder and VirulenceFinder web-services for easy identification of acquired antibiotic resistance and E. coli virulence genes in bacteriophage and prophage nucleotide sequences. Bacteriophage 4:e27943. doi: 10.4161/bact.27943
Klockgether, J., Cramer, N., Wiehlmann, L., Davenport, C. F., and Tummler, B. (2011). Pseudomonas aeruginosa genomic structure and diversity. Front. Microbiol. 2:150. doi: 10.3389/fmicb.2011.00150
Kos, V. N., Déraspe, M., McLaughlin, R. E., Whiteaker, J. D., Roy, P. H., Alm, R. A., et al. (2015). The resistome of Pseudomonas aeruginosa in relationship to phenotypic susceptibility. Antimicrob. Agents Chemother. 59, 427–436. doi: 10.1128/AAC.03954-14
Kumar, R., Verma, H., Haider, S., Bajaj, A., Sood, U., Ponnusamy, K., et al. (2017). Comparative genomic analysis reveals habitat-specific genes and regulatory hubs within the genus Novosphingobium. mSystems 2:e00020-17. doi: 10.1128/mSystems.00020-17
Lagesen, K., Hallin, P., Rødland, E. A., Stærfeldt, H. H., Rognes, T., and Ussery, D. W. (2007). RNAmmer, consistent and rapid annotation of ribosomal RNA genes. Nucleic Acids Res. 35, 3100–3108. doi: 10.1093/nar/gkm160
Lambert, P. A. (2002). Mechanisms of antibiotic resistance in Pseudomonas aeruginosa. J. R. Soc. Med. 95, 22–26.
Langille, M. G., Hsiao, W. W., and Brinkman, F. S. (2010). Detecting genomic islands using bioinformatics approaches. Nat. Rev. Microbiol. 8, 373–382. doi: 10.1038/nrmicro2350
Larsen, M. V., Cosentino, S., Rasmussen, S., Friis, C., Hasman, H., Marvig, R. L., et al. (2012). Multilocus sequence typing of total genome sequenced bacteria. J. Clin. Microbiol. 50, 1355–1361. doi: 10.1128/JCM.06094-11
Laslett, D., and Canback, B. (2004). ARAGORN, a program to detect tRNA genes and tmRNA genes in nucleotide sequences. Nucleic Acids Res. 32, 11–16. doi: 10.1093/nar/gkh152
Li, H., Luo, Y. F., Williams, B. J., Blackwell, T. S., and Xie, C. M. (2012). Structure and function of OprD protein in Pseudomonas aeruginosa: from antibiotic resistance to novel therapies. Int. J. Med. Microbiol. 302, 63–68. doi: 10.1016/j.ijmm.2011
Linke, D., Riess, T., Autenrieth, I. B., Lupas, A., and Kempf, V. A. (2006). Trimeric autotransporter adhesins: variable structure, common function. Trends Microbiol. 14, 264–270. doi: 10.1016/j.tim.2006.04.005
Livermore, D. M. (2002). Multiple mechanisms of antimicrobial resistance in Pseudomonas aeruginosa: our worst nightmare? Clin. Infect. Dis. 34, 634–640. doi: 10.1086/338782
Lyczak, J. B., Cannon, C. L., and Pier, G. B. (2000). Establishment of Pseudomonas aeruginosa infection, lessons from a versatile opportunist. Microbes Infect. 2, 1051–1060. doi: 10.1016/S1286-4579(00)01259-4
Lynch, M. J., Drusano, G. L., and Mobley, H. L. (1987). Emergence of resistance to imipenem in Pseudomonas aeruginosa. Antimicrob. Agents Chemother. 31, 1892–1896. doi: 10.1128/AAC.31.12.1892
Mai-Prochnow, A., Bradbury, M., and Murphy, A. B. (2015). Draft genome sequence of Pseudomonas aeruginosa ATCC 9027 (DSM 1128), an important rhamnolipid surfactant producer and sterility testing strain. Genome Announc. 3:e01259-15. doi: 10.1128/genomeA.01259-15
Maredia, R., Devineni, N., Lentz, P., Dallo, S. F., Yu, J., Guentzel, N., et al. (2012). Vesiculation from Pseudomonas aeruginosa under SOS. Sci. World J. 2012:402919. doi: 10.1100/2012/402919
Maslov, S., and Sneppen, K. (2002). Specificity and stability in topology of protein networks. Science 296, 910–913. doi: 10.1126/science.1065103
Mathee, K., Narasimhan, G., Valdes, C., Qiu, X., Matewish, J. M., Koehrsen, M., et al. (2008). Dynamics of Pseudomonas aeruginosa genome evolution. Proc. Natl. Acad. Sci. U.S.A. 105, 3100–3105. doi: 10.1073/pnas.0711982105
Moriya, Y., Itoh, M., Okuda, S., Yoshizawa, A. C., and Kanehisa, M. (2007). KAAS: an automatic genome annotation and pathway reconstruction server. Nucleic Acids Res. 35, 182–185. doi: 10.1093/nar/gkm321
Mowbray, S. L., Kathiravan, M. K., Pandey, A. A., and Odell, L. R. (2014). Inhibition of glutamine synthetase: a potential drug target in Mycobacterium tuberculosis. Molecules 19, 13161–13176. doi: 10.3390/molecules190913161
Moya, B., Beceiro, A., Cabot, G., Juan, C., Zamorano, L., Alberti, S., et al. (2012). Pan-β-lactam resistance development in Pseudomonas aeruginosa clinical strains: molecular mechanisms, PBPs profiles and binding affinities. Antimicrob. Agents Chemother. 56, 4771–4778.
Murima, P., McKinney, J. D., and Pethe, K. (2014). Targeting bacterial central metabolism for drug development. Chem. Biol. 21, 1423–1432. doi: 10.1016/j.chembiol.2014.08.020
Pahel, G., Zelenetz, A. D., and Tyler, B. M. (1978). gltB gene and regulation of nitrogen metabolism by glutamine synthetase in Escherichia coli. J. Bacteriol. 133, 139–148.
Palzkill, T. (2013). Metallo-β-lactamase structure and function. Ann. N. Y. Acad. Sci. 1277, 91–104. doi: 10.1111/j.1749-6632.2012.06796.x
Passmore, I. J., Nishikawa, K., Lilley, K. S., Bowden, S. D., Chung, J. C., and Welch, M. (2015). Mep72, a metzincin protease that is preferentially secreted by biofilms of Pseudomonas aeruginosa. J. Bacteriol. 197, 762–773. doi: 10.1128/JB.02404-14
Philippart, F., Gaudry, S., Quinquis, L., Lau, N., Ouanes, I., Touati, S., et al. (2015). Randomized intubation with polyurethane or conical cuffs to prevent pneumonia in ventilated patients. Am. J. Respir. Crit. Care. Med. 191, 637–645. doi: 10.1164/rccm.201408-1398OC
Pirnay, J. P., Vos, D. D., Mossialos, D., Vanderkelen, A., Cornelis, P., and Zizi, M. (2002). Analysis of the Pseudomonas aeruginosa oprD gene from clinical and environmental isolates. Environ. Microbiol. 4, 872–882.
Planquette, B., Timsit, J. F., Misset, B. Y., Schwebel, C., Azoulay, E., Adrie, C., et al. (2013). Pseudomonas aeruginosa ventilator-associated pneumonia. predictive factors of treatment failure. Am. J. Respir. Crit. Care Med. 188, 69–76. doi: 10.1164/rccm.201210-1897OC
Price, M. N., Dehal, P. S., and Arkin, A. P. (2009). FastTree: computing large minimum evolution trees with profiles instead of a distance matrix. Mol. Biol. Evol. 26, 1641–1650. doi: 10.1093/molbev/msp077
R Development Core Team (2015). R: A Language and Environment for Statistical Computing. Vienna: R Foundation for Statistical Computing.
Raczynska, J. E., Vorgias, C. E., Antranikian, G., and Rypniewski, W. (2011). Crystallographic analysis of a thermoactive nitrilase. J. Struct. Biol. 173, 294–302. doi: 10.1016/j.jsb.2010.11.017
Ravasz, E., Somera, A. L., Mongru, D. A., Oltvai, Z. N., and Barabási, A. L. (2002). Hierarchical organization of modularity in metabolic networks. Science 297, 1551–1555. doi: 10.1126/science.1073374
Reboud, E., Elsen, S., Bouillot, S., Golovkine, G., Basso, P., Jeannot, K., et al. (2016). Phenotype and toxicity of the recently discovered exlA-positive Pseudomonas aeruginosa strains collected worldwide. Environ. Microbiol. 18, 3425–3439. doi: 10.1111/1462-2920.13262
Roy, P. H., Tetu, S. G., Larouche, A., Elbourne, L., Tremblay, S., Ren, Q., et al. (2010). Complete genome sequence of the multiresistant taxonomic outlier Pseudomonas aeruginosa PA7. PLoS One 5:e8842. doi: 10.1371/journal.pone.0008842
Rybtke, M., Hultqvist, L. D., Givskov, M., and Tolker-Nielsen, T. (2015). Pseudomonas aeruginosa biofilm infections: community structure, antimicrobial tolerance and immune response. J. Mol. Biol. 427, 3628–3645. doi: 10.1016/j.jmb.2015.08.016
Schwibbert, K., Marin-Saguino, A., Bagyan, I., Heidrich, G., Lentzen, G., Seitz, H., et al. (2011). A blueprint of ectoine metabolism from the genome of the industrial producer Halomonas elongata DSM 2581T. Environ. Microbiol. 13, 1973–1994. doi: 10.1111/j.1462-2920.2010.02336.x
Segata, N., Börnigen, D., Morgan, X. C., and Huttenhower, C. (2013). PhyloPhlAn is a new method for improved phylogenetic and taxonomic placement of microbes. Nat. Commun. 4:2304. doi: 10.1038/ncomms3304
Shannon, P., Markiel, A., Ozier, O., Baliga, N. S., Wang, J. T., Ramage, D., et al. (2003). Cytoscape: a software environment for integrated models of biomolecular interaction networks. Genome Res. 13, 2498–2504. doi: 10.1101/gr.1239303
Smith, E. E., Sims, E. H., Spencer, D. H., Kaul, R., and Olson, M. V. (2005). Evidence for diversifying selection at the pyoverdine locus of Pseudomonas aeruginosa. J. Bacteriol. 187, 2138–2147. doi: 10.1128/JB.187.6.2138-2147.2005
Somerville, G., Mikoryak, C. A., and Reitzer, L. (1999). Physiological characterization of Pseudomonas aeruginosa during exotoxin A synthesis: glutamate, iron limitation, and aconitase activity. J. Bacteriol. 181, 1072–1078.
Stamatakis, A. (2014). RAxML version 8: a tool for phylogenetic analysis and post-analysis of large phylogenies. Bioinformatics 30, 1312–1313. doi: 10.1093/bioinformatics/btu033
Stanborough, T., Fegan, N., Powell, S. M., Tamplin, M., and Chandry, P. S. (2017). Vibrioferrin production by the food spoilage bacterium Pseudomonas fragi. FEMS Microbiol. Lett. 365:fnx279. doi: 10.1093/femsle/fnx279
Steimle, A., Autenrieth, I. B., and Frick, J. S. (2016). Structure and function: lipid A modifications in commensals and pathogens. Int. J. Med. Microbiol. 306, 290–301. doi: 10.1016/j.ijmm.2016.03.001
Stover, C. K., Pham, X. Q., Erwin, A. L., Mizoguchi, S. D., Warrener, P., Hickey, M. J., et al. (2000). Complete genome sequence of Pseudomonas aeruginosa PAO1, an opportunistic pathogen. Nature 406, 959–964. doi: 10.1038/35023079
Szklarczyk, D., Morris, J. H., Cook, H., Kuhn, M., Wyder, S., Simonovic, M., et al. (2017). The STRING database in 2017: quality-controlled protein–protein association networks, made broadly accessible. Nucleic Acids Res. 45,D362–D368. doi: 10.1093/nar/gkw937
Tettelin, H., Masignani, V., Cieslewicz, M. J., Donati, C., Medini, D., Ward, N. L., et al. (2005). Genome analysis of multiple pathogenic isolates of Streptococcus agalactiae: implications for the microbial “pan-genome”. Proc. Natl. Acad. Sci. U.S.A. 102, 13950–13955. doi: 10.1073/pnas.0506758102
Thomas, S., Holland, I. B., and Schmitt, L. (2014). The type 1 secretion pathway—the hemolysin system and beyond. Biochem. Biophys. Acta 1843, 1629–1641. doi: 10.1016/j.bbamcr.2013.09.017
Tralau, T., Vuilleumier, S., Thibault, C., Campbell, B. J., Hart, C. A., and Kertesz, M. A. (2007). Transcriptomic analysis of the sulfate starvation response of Pseudomonas aeruginosa. J. Bacteriol. 189, 6743–6750. doi: 10.1128/JB.00889-07
Treangen, T. J., Ondov, B. D., Koren, S., and Phillippy, A. M. (2014). The Harvest suite for rapid core-genome alignment and visualization of thousands of intraspecific microbial genomes. Genome Biol. 15:524. doi: 10.1186/s13059-014-0524-x
van Belkum, A., Soriaga, L. B., LaFave, M. C., Akella, S., Veyrieras, J. B., Barbu, E. M., et al. (2015). Phylogenetic distribution of CRISPR-Cas systems in antibiotic-resistant Pseudomonas aeruginosa. mBio 6:e01796-15. doi: 10.1128/mBio.01796-15
Wallden, K., Rivera-Calzada, A., and Waksman, G. (2010). Microreview: type IV secretion systems: versatility and diversity in function. Cell. Microbiol. 12, 1203–1212. doi: 10.1111/j.1462-5822.2010.01499.x
Watts, D. J., and Strogatz, S. H. (1998). Collective dynamics of small-world networks. Nature 393, 440–442.
White, P. A., Stokes, H. W., Bunny, K. L., and Hall, R. M. (1999). Characterisation of a chloramphenicol acetyltransferase determinant found in the chromosome of Pseudomonas aeruginosa. FEMS Microbiol. Lett. 175, 27–35.
Winsor, G. L., Griffiths, E. J., Lo, R., Dhillon, B. K., Shay, J. A., and Brinkman, F. S. (2016). Enhanced annotations and features for comparing thousands of Pseudomonas genomes in the Pseudomonas genome database. Nucleic Acids Res. 44, 646–653. doi: 10.1093/nar/gkv1227
Wood, T. L., and Wood, T. K. (2016). The HigB/HigA toxin/antitoxin system of Pseudomonas aeruginosa influences the virulence factors pyochelin, pyocyanin, and biofilm formation. Microbiologyopen 5, 499–511. doi: 10.1002/mbo3.346
Wu, D. Q., Ye, J., Ou, H. Y., Wei, X., Huang, X., He, Y. W., et al. (2011). Genomic analysis and temperature-dependent transcriptome profiles of the rhizosphere originating strain Pseudomonas aeruginosa M18. BMC Genomics 12:438. doi: 10.1186/1471-2164-12-438
Wu, S., Zhu, Z., Fu, L., Niu, B., and Li, W. (2011). WebMGA: a customizable web server for fast metagenomic sequence analysis. BMC Genomics 12:444. doi: 10.1186/1471-2164-12-444
Yang, Z. (2007). PAML 4: phylogenetic analysis by maximum likelihood. Mol. Biol. Evol. 24, 1586–1591. doi: 10.1093/molbev/msm088
Ye, Y., and Doak, T. G. (2009). Minpath: a parsimony approach to biological pathway reconstruction/inference for genomes and metagenomes. PLoS Comput. Biol. 5:e1000465. doi: 10.1371/journal.pcbi.1000465
Yu, Q., Cai, H., Zhang, Y., He, Y., Chen, L., Merritt, J., et al. (2017). Negative regulation of ectoine uptake and catabolism in Sinorhizobium meliloti: characterization of the EhuR Gene. J. Bacteriol. 199:e00119-16. doi: 10.1128/JB.00119-16
Zeng, L., and Jin, S. (2003). aph (3′)-IIb, a gene encoding an aminoglycoside-modifying enzyme, is under the positive control of surrogate regulator HpaA. Antimicrob. Agents Chemother. 47, 3867–3876.
Keywords: Pseudomonas aeruginosa, outliers, environmental genomics, drug targets, positive selection
Citation: Sood U, Hira P, Kumar R, Bajaj A, Rao DLN, Lal R and Shakarad M (2019) Comparative Genomic Analyses Reveal Core-Genome-Wide Genes Under Positive Selection and Major Regulatory Hubs in Outlier Strains of Pseudomonas aeruginosa. Front. Microbiol. 10:53. doi: 10.3389/fmicb.2019.00053
Received: 21 November 2018; Accepted: 14 January 2019;
Published: 06 February 2019.
Edited by:
Vasco Ariston De Carvalho Azevedo, Universidade Federal de Minas Gerais, BrazilReviewed by:
Vasvi Chaudhry, University of Tübingen, GermanyMarcus Vinicius Canário Viana, Universidade Federal do Pará, Brazil
Copyright © 2019 Sood, Hira, Kumar, Bajaj, Rao, Lal and Shakarad. This is an open-access article distributed under the terms of the Creative Commons Attribution License (CC BY). The use, distribution or reproduction in other forums is permitted, provided the original author(s) and the copyright owner(s) are credited and that the original publication in this journal is cited, in accordance with accepted academic practice. No use, distribution or reproduction is permitted which does not comply with these terms.
*Correspondence: Rup Lal, cnVwbGFsQGdtYWlsLmNvbQ== Mallikarjun Shakarad, YmVlbGFiLm1zQGdtYWlsLmNvbQ==