- 1Key Laboratory of Dairy Science, Ministry of Education, College of Food Science, Northeast Agricultural University, Harbin, China
- 2Department of Ultrasound, Maternal and Child Health Hospital of Dapeng New District, Shenzhen, China
Modulating gut microbiota to promote host health is well recognized. Therefore, people consume dietary products containing traditional probiotics in wishing to improve their health, and they need more research-based advices on how to select products with suitable probiotic species. Probiotic designers are sometimes confused about how to design precision products for different consumers by taking advantages of different probiotic species’ strengths. Additionally, large-scale analyses on traditional probiotic complementarity potentials and their roles in gut microbiome related to common diseases are not well understood. Here, we comprehensively analyzed 444 genomes of major traditional probiotic (sub) species (MTPS, n = 15) by combining one newly sequenced genome with all of the public NCBI-available MTPS-related genomes. The public human fecal metagenomic data (n = 1,815) of eight cohorts were used to evaluate the roles of MTPS, compared to other main gut bacteria, in disease association by examining the species enrichment direction in disease group or the control group. Our work provided a comprehensive genetic landscape and complementarity relations for MTPS and shed light on personalized probiotic supplements for consumers with different health status and the necessity that researchers and manufactures could explore novel probiotics as well as traditional ones.
Introduction
Traditional probiotics bacteria are mainly lactic acid bacteria (LAB) (e.g., Lactobacillus, Lactococcus, and Bifidobacteria). They are a group of Gram-positive bacteria that produce lactic acid as a main end product and have been generally considered as safe for human consumption. It also has been shown that probiotics administrated closer to the first dose of antibiotics can reduce the risk of Clostridium difficile infection in hospitalized adults by more than 50% (Shen et al., 2017). Another traditional probiotic is Bacillus bacteria, mostly Bacillus subtilis, which was reported to have abolished colonization of Staphylococcus aureus, a dangerous pathogen in a rural Thai population by inhibiting S. aureus quorum sensing (Piewngam et al., 2018). Specific strains associated with health benefits had been used as probiotics, establishing a huge consumption market. However, reports of clinical infection or adverse effects, and the spread of antibiotic resistance genes, have raised concerns about safety. A case of an elderly diabetic patient with a complicated liver abscess and bacteremia from the consumption of Lactobacillus paracasei probiotic has been reported and confirmed by strain identification (Pararajasingam and Uwagwu, 2017). Probiotic therapy should be carefully selected in hemodialysis patients since probiotic supplements failed to reduce uremic toxins and inflammations (Borges et al., 2018). Compared to spontaneous post-antibiotic recovery, probiotics induced a significant delay and persistently incomplete indigenous stool/mucosal microbial reconstitution and host transcriptome recovery toward homeostatic configuration (Suez et al., 2018).
Gratefully, public research data and approaches provide a good foundation for further inspection of the host-microbiome relationships. The total amount of sequenced bacterial genomes has continued to expand markedly over the past few years (Land et al., 2015). Intestinal microbes have been explored for several decades, and the investigations of the role of human gut microbiota have drawn much attention about diseases, such as obesity, diabetes, liver diseases, and even cancer and neurodegenerative diseases. There is a trend that the human gut microorganisms are considered a resource of novel therapies (Cani, 2018). What is more, metagenome-wide association studies (MWAS) is a research method to survey the correlations between the human microbiota and many non-communicable diseases (Wang and Jia, 2016). Such many public bacterial genomes data, case-control cohort metagenomes data and advanced research methods make it possible to systematically compare the functional potential and safety factors of MTPS, as well as the detection of their abundance and evaluation of their impacts on the gut microbiomes.
In this article, 444 strains (of species labeled in the Chinese domestic market probiotics products and one children OTC drug) and 1,815 public human gut metagenomic data from eight cohorts were recruited, in the aims to describe the species diversity, potential risk gene components, strain complementarity and to assess the impacts of MTPS on gut microbiomes, shedding light on personalized probiotics supplement for consumers and urgent need that researchers and manufactures could explore more novel probiotics as well as traditional ones.
Materials and Methods
Genomes Recruitment
We collected most of the species names from labels of commercially available yogurt products and one children OTC drug (Omitting product names to avoid commercial benefits conflict). All associated genomes were downloaded from NCBI (updated to March 19th, 2018) by searching species keywords in the URL1. Items with “single cell,” “Partial,” “derived from environmental source,” “metagenome,” “too small,” “low contig N,” “frameshifted,” “missing tRNA genes,” or “abnormal gene to sequence ratio” were eliminated in this work in order to avoid low-quality genomes.
The Lactobacillus acidophilus (Lb. acidophilus) KLDS1.0901 was isolated in our laboratory. A small fragment library of 400 bp insert size and a mate-pair large fragment library of 6 Kbp insert size were constructed, respectively, and sequenced on the Illumina Genome Analyzer II, HiSeq/MiSeq platform. Approximately 150× clean reads were produced by filtering low quality (>40% ambiguous “N” bases or >40% bases of quality <20 in one or both of the paired-end reads), low complexity and duplicated reads, then were assembled into scaffolds by SOAPdenovo2 (version r240) (Luo et al., 2012). This Whole Genome Shotgun project of Lb. acidophilus KLDS1.0901 has been archived at DDBJ/ENA/GenBank under the accession MNPO00000000. The version involved in this study is version MNPO01000000.
Genome quality was further assessed. Firstly, more rigorous five high-quality draft genome criteria from the Human Microbiome Project (HMP) (Human Microbiome Jumpstart Reference Strains et al., 2010) was adopted to ensure the assembly quality. The criteria were (i) 98% of the total assembly must be present in contigs (>500 bp), (ii) the scaffold N50 must be more than 20 kb, (iii) the contig N50 must be more than 20 kb, (iv) the average contig length must be more than 10 kb, and (v) more than 90% of the “core genes” (Callister et al., 2008) must be present in the assembly. Secondly, the “complete” and “contamination” of genomes were checked using CheckM (v1.0.12) (Parks et al., 2015). Only genomes with “complete” >95% and “contamination” <5% were recruited. Thirdly, average nucleotide identity (ANI) (Richter and Rossello-Mora, 2009) was also employed to remove the far-distance genomes which may be mis-distributed to the species. The pairwise ANI should be >93% for each pair of genomes within the same species. For ANI-based whole genome comparison among genomes, identity alignments were done with BLAST (version 2.2.26) (Mount, 2007). ANI was counted for every genome pair using the method as previously described (Zhang et al., 2014) basing on the BLAST results. Another same alignment was done by exchanging the query genome and the reference genome. The pairwise ANI value was the average value of the two alignments. The average amino acid identity (AAI) calculation was same as ANI, except replacing nucleotide sequence with amino acid sequence.
Pan-Genome Analyses
A pan-genome for a (sub) species cluster consists of core genes shared by all the recruited genomes, plus dispensable genes consisting of accessory gene partially shared in members and strain-specific genes unique to single members (Tettelin et al., 2005). To identify orthologous genes for the strains in each species, CD-HIT (v4.6.6-2016-0711) (Li and Godzik, 2006) was applied to cluster genes as described previously (Kim et al., 2017), except that the thresholds of 95% protein sequence similarity and 90% alignment length for shorter sequence.
Phylogenetic Analyses
A group of 40 universally conserved single-copy gene coding proteins in prokaryotic microbes was applied for the construction of a phylogenetic tree. The marker genes were deduced by specI (v1.0) (Mende et al., 2013) and aligned using prank (v.170427) (Loytynoja, 2014). Alignments were truncated by trimal (v1.4.1) (Capella-Gutierrez et al., 2009) and concatenated into one amino acid sequence by in-house scripts. Maximum-likelihood phylogenetic trees for all the genomes were constructed in RAxML (version 8.2.11) (Stamatakis, 2014), and were visualized on iTOL (Letunic and Bork, 2016) online.
Functional Assignments and KEGG Module Complementarity in silico Calculation
Genes were identified using Genemark (version 2.6r) (Besemer and Borodovsky, 2005). The translated amino acid sequences of coding genes were aligned with RAPSearch (v2.23) (Ye et al., 2011) (default options, except for -s f -e 1e-2 -v 100 -u 2) against Kyoto Encyclopedia of Genes and Genomes (KEGG version 76) (Kanehisa and Goto, 2000) (query match length >=50%) or with BLASTp (default options, except for -e 1e-2 -F T -b 100 -K 1 -a 1 -m 8) against databases of Antibiotic Resistance Genes Database (ARDB) (Liu and Pop, 2009) (both query and subject match length >=40%, with identity more than the ARDB-recommended thresholds), Virulence Factor Database (VFDB) (Chen et al., 2016) (query match length >=50%, with identity >=60%), Carbohydrate-Active enZYmes Database (CAZy) (Lombard et al., 2014) (query match length >=50%, with identity >=60%).
The KEGG module integrity was defined as matched key/essential KO number divided by all key/essential KO number required by this module. To learn the KEGG module complementarity of every genome pair, all the KOs of every genome pair were merged to calculate the improvement of module integrity.
Gut Microbiome Profiling and Co-abundance Network Analyses
The clean reads (filtering out low-quality reads and host-derived DNA reads) from each cohort were, respectively, profiled (taxonomy at species level) by MetaPhlAn2 (v2.2.0) (Segata et al., 2012) (default options, except setting – ignore_viruses – ignore_eukaryotes –ignore_archaea). The clean reads from ACVD cohorts were also, mapped [SOAP2 (v2.22) (Li et al., 2009), with default parameters] to the gene set of each species or all genes of the target strain. The mapping ratio for each gut microbiome sample was defined as mapped reads divided by total reads of each sample.
Pearson correlation (p-value <0.05, correlation coefficient cutoff, >0.4 if positive, <-0.1 if negative) for species relative abundances (derived from MetaPhlAn2) in all samples (without consideration of health status) among the enriched species for each cohort were obtained, and co-abundance networks (all enriched species were showed only) were visualized with Cytoscape (v3.6.1) (Shannon et al., 2003) software.
Results
Diverse Openness Level of Pan-Genomes Could Be Related to Multiple Forces
The completeness, purity and quality of genomes can influence the pan-genome results (Kim et al., 2017), thus the quality control was necessary for all the recruited genomes. A total of 502 genomes belonging to 15 (sub)species were downloaded from NCBI as of March 19th, 2018, and 444 high-quality genomes (including our newly sequenced strain Lb. acidophilus KLDS1.0901) were retained after the removal of low quality, impure, and mis-allocated genomes recognized by HMP five genome draft standards, CheckM and intra-species ANI. In particular, 38 strains of Bacillus subtilis subsp. subtilis (Ba. subtilis), 19 strains of Bifidobacterium longum subsp. infantis (Bi. infantis), 35 strains of Bifidobacterium longum subsp. longum (Bi. longum), 22 strains of Bifidobacterium animalis subsp. lactis (Bi. lactis), 20 strains of Lb. acidophilus (containing KLDS1.0901), 15 strains of Lactobacillus delbrueckii subsp. bulgaricus (Lb. bulgaricus), 33 strains of Lactobacillus casei (Lb. casei), 31 strains of Lactobacillus fermentum (Lb. fermentum), 25 strains of Lactobacillus gasseri (Lb. gasseri), 16 strains of Lactobacillus helveticus (Lb. helveticus), 52 strains of Lactobacillus paracasei (Lb. paracasei), 9 strains of Lactobacillus plantarum subsp. plantarum (Lb. plantarum), 25 strains of Lactococcus lactis subsp. cremoris (Lc. cremoris), 61 strains of Lactococcus lactis subsp. lactis (Lc. lactis), and 43 strains of Streptococcus thermophilus (Sc. thermophilus) were retained to illuminate the pan-genomes, core genes and strain-specific genes to understand the characteristic of the pan-genomes of each species. Detailed genomic information for all the strains are summarized in Supplementary Table S1.
In order to quantitate the openness (genetic diversity) of pan-genome, pan-genome index (PI) was defined in this study at the first time, namely, average gene number divided by gene number of pan-genome. The openness of pan-genomes could be divided into three levels (Figure 1A) for these involved species. Level A, relatively conserved, PI<=1.5, including Lb. acidophilus (PI 1.24) and Bi. lactis (PI 1.29). Level B, half-open, 1.5<PI<=3, including Lb. plantarum (PI 1.7), Bi. infantis (PI 1.81), Ba. subtilis (PI 1.82), Lb. bulgaricus (PI 2.02), Lb. gasseri (PI 2.44), Lb. helveticus (PI 2.55), Lb. casei (PI 2.64), Lc. cremoris (PI 2.68), Bi. longum (PI 2.73), Sc. thermophilus (PI 2.76), Lb. fermentum (PI 2.81). Level C, relatively open, including Lb. paracasei (PI 3.1), and Lc. lactis (PI 4.19).
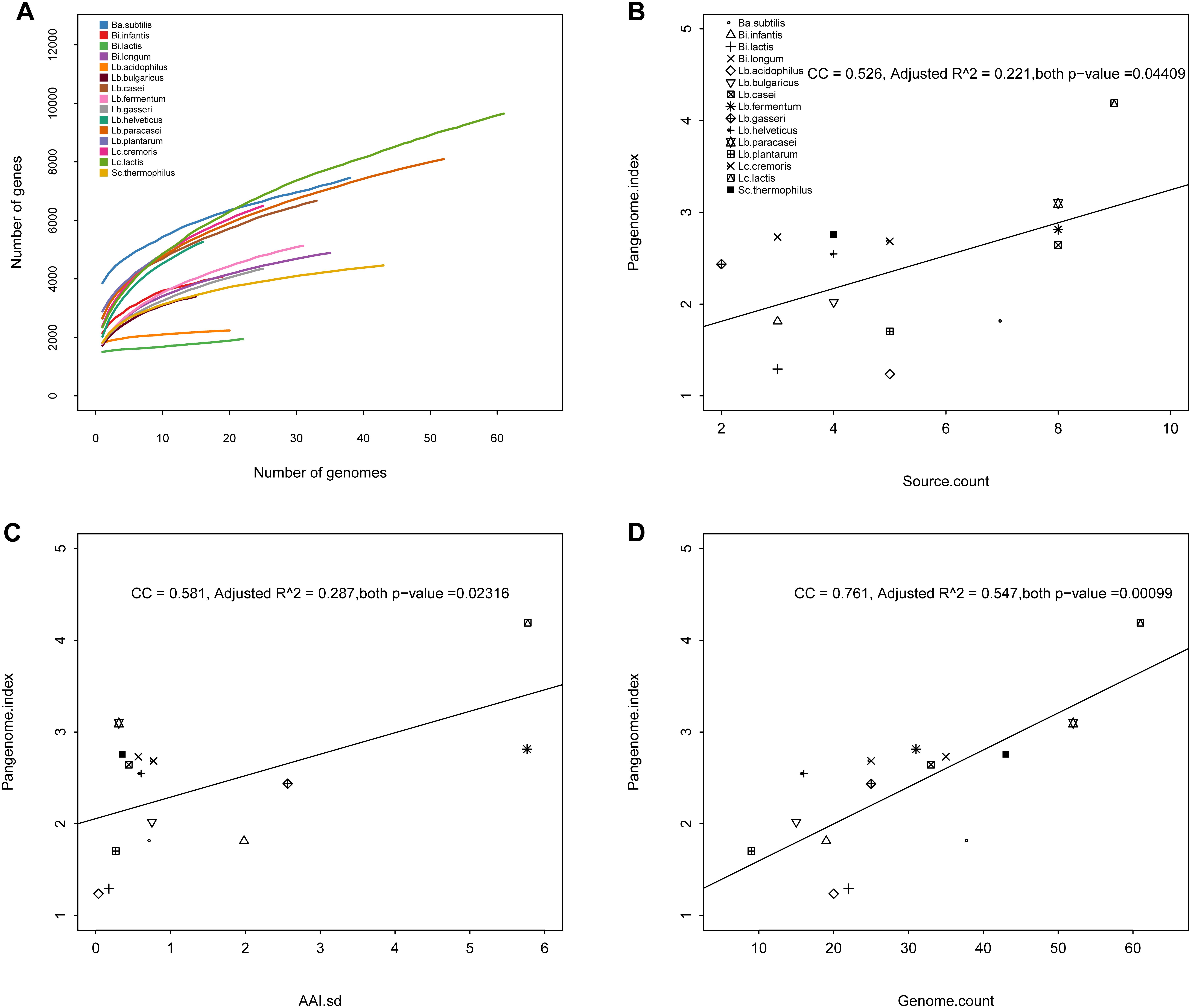
Figure 1. Pan-genome rarefaction and pan-genome index. (A) The pan-genome rarefaction curve of 15 species. (B) Correlation between pan-genome index and source count of each species, CC: correlation coefficient. (C) Correlation between pan-genome index and intra-species amino acid identity (AAI) SD. (D) Correlation between pan-genome index and genome count of each species.
The species isolated from different hosts are enriched in specific gene sets, indicating host-specific adaptation (Sun et al., 2015b). That is to say, genetic diversity is related to the ecological niche, so we further surveyed relationship including this among MTPS. The original isolation sources were collected as much as possible for these strains. The association analysis results showed that PI was weakly positively associated [correlation coefficient (CC) 0.526, R square 0.221] with intra-species isolation source count (Figure 1B). PI was also weakly positively associated (CC 0.581, R square 0.287) with intra-species genome number (Figure 1D). PI was weakly positively associated (CC 0.761, R square 0.547) with intra-species AAI distance standard deviation (AAI SD, Figure 1C). In summary, the correlation was growing and being strengthened along with isolation source, genome number and AAI distance SD. Interestingly, the R square was improved to 0.585 (p-value 0.005) when combining all the three factors, implying that many factors would influence the PI and should be taken into consideration together. The reason for this might be that the bacteria could display genomic diversity in assorted niche sites for survival by insert, deletion, single nucleotide polymorphisms, structure variation, and lateral gene transfer, resulting in ever-increasing genetic diversity (Duranti et al., 2016).
Phylogenetic Tree Cluster on Species Level Even on Subspecies Level
To investigate the distance of 15 species, pairwise AAI of the core gene of each species were computed. Strains from the same microbial species share >95% AAI and ANI (Thompson et al., 2013). Around 95% ANI is the recommended threshold for species discrimination (Mende et al., 2013). Both Bi. infantis and Bi. longum belong to Bi. longum, and their AAI (98.4%) is greater than 95%, corroborating they were the same species at molecular level. Lb. paracasei and Lb. casei belong to different species, but their AAI (98.28%) is greater than 95%, showing that they should be the same species at molecular level. In some taxonomy annotation software, such as MetaPhlAn2, Lb. paracasei and Lb. casei are treated as same species with the name Lactobacillus_casei_paracasei. Lc. cremoris and Lc. lactis belong to Lc. lactis, but their AAI (93.92%) is less than 95%, showing that they should be different species at molecular level. At the 70% AAI cutoff, 15 species could be separated into 8 clusters (Figure 2A). The ANI matrix for 444 strains were summarized in Supplementary Table S2.
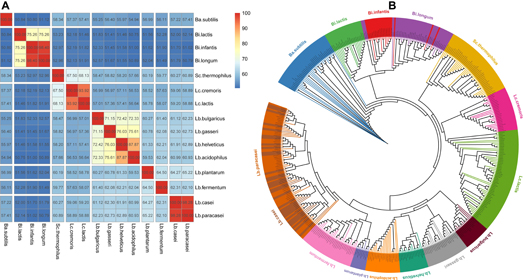
Figure 2. Amino acid identity matrix and phylogenetic tree. (A) AAI matrix at species level. The number in the cell indicated the AAI value of the two species. Clusters are separated by blank lines (B) Phylogenetic tree for 444 strains.
Further, the phylogenetic relationships among these strains of 15 species were also inspected based on 40 universally conserved single-copy proteins (Mende et al., 2013). We observed that the 15 species are also divided into 8 clusters (Figure 2B), which was in agreement with the clustering with AAI. As shown on the tree, Lb. paracasei and Lb. casei blended with each other, demonstrating they should be the same species again. Bi. infantis and Bi. longum (both isolated from human gut) also blended with each other, which implied that they are not fully differentiated due to their similar lifestyle adaptation (Yu et al., 2018).
Potential Risk Factors Differ Among Species and Are Elevated in Groups of Unfriendly Isolation Sources
To investigate the risk factors (Ronholm et al., 2016) of all 444 strains, VFDB and ARDB genes were considered and the annotation results were compared for every species pair to find species-enriched/specific features. In total, these strains contained 11 VFDB genes and 18 ARDB genes (Supplementary Table S3), suggesting they had moderate risk factors. Concretely speaking, Ba. subtilis harbored the most specific VFDB genes (VFG001373, VFG011430, VFG045350) and ARDB genes (ardb_1014, ardb_1206, ardb_1207, ardb_129, ardb_2757, ardb_295, ardb_3001, ardb_3001, ardb_365, ardb_676), while Bi. lactis harbored none. VFG000077 (ATP-dependent Clp protease proteolytic subunit), VFG000080 (ATP-dependent protease), VFG002182 (UDP-galactopyranose mutase) are prevalent for most of the species. VFG000079 (endopeptidase Clp ATP-binding chain C) was shared by Ba. subtilis, Lb. casei, and Lb. paracasei. VFG002165 (endocarditis specific antigen) and ardb_1371 (bacitracin) are shared by Lb. casei and Lb. paracasei. VFG013286 (UDP-glucose 4-epimerase) was shared by Bi. infantis and Bi. longum. ardb_2198 (bacitracin) was shared by Lb. bulgaricus, Lb. fermentum, and Lb. plantarum. ardb_2980 (bacitracin) was shared by Lc. lactis and Sc. thermophilus. VFG002187 (glycosyl transferase, group 2 family protein), ardb_1049 (fluoroquinolone) and ardb_751 (unknown function) are specific for Lc. cremoris. ardb_1050 (fluoroquinolone), ardb_2979 (bacitracin), and ardb_750 (unknown function) are specific for Lc. lactis. VFG005767 (3-ketoacyl-ACP-reductase CylG) was specific for Sc. thermophilus.
Additionally, risk factors between relatively friendly group (with isolation source of such as milk, cheese, yogurt, beer, human gut, food) and relatively unfriendly group (with isolation source of such as patient’s specimen, soil, blood, saliva, silage) were tested by unpaired Wilcoxon-rank sum test. As a result, the strains isolated from unfriendly environments harbored much more risk factors (Supplementary Figure S1, ∗p < 0.05, ∗∗p < 0.01), indicating that it is recommended to choose strains with a clear safe source when using them in food industry (Ronholm et al., 2016), such as yogurt starter.
Relatively Enhanced Functions Differ Among Species, Even Subspecies
The CAZy database is a extensively used resource associated with the enzymes that construct and breakdown complex carbohydrates and glycoconjugates (Cantarel et al., 2009). From the viewpoint of CAZy annotation results (Figure 3A), Ba. subtilis, Bi. infantis, Bi. longum, Lb. plantarum, Lc. cremoris, and Lc. lactis harbored leading capability of carbohydrate metabolism, while Lb. fermentum, Lb. helveticus, and Lb. bulgaricus harbored lagging capability relatively. There were also species-specific CAZy module, such as GT39, GH94, CBM10 and CBM23 for Bi. lactis, CBM61 and GH112 for Bi. infantis and Bi. longum (both are subspecies of Bi. longum), GH66 for Sc. thermophilus. To find all the enhanced CAZy modules for 15 species, all the modules were tested by unpaired Wilcoxon-rank sum test for every species pair, the detailed results were provided in Supplementary Table S3, which is helpful to compare carbohydrate metabolism characteristic of 15 species.
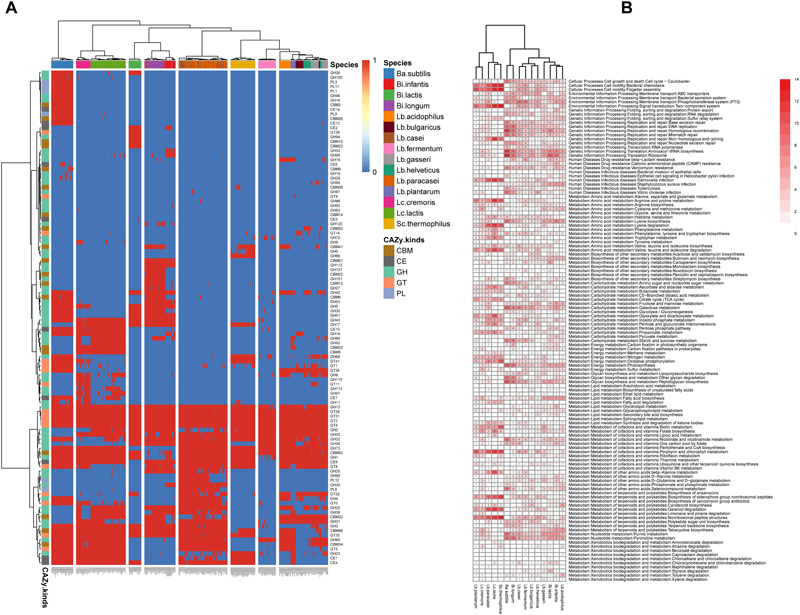
Figure 3. Different relatively enhanced function features among species. (A) Heat map illustrating the distribution of carbohydrate-active enzyme genes. (B) Different relatively enhanced function of KEGG pathway map. The number in the cell indicated how many other species are significantly weaker than this species in this function (q-value <0.05, FDR-controlled Wilcoxon rank-sum test).
KEGG is another well-known database resource for understanding advanced functions and tools of the biological system from molecular-level information (Kanehisa and Goto, 2000). From the insight of KEGG annotation results (Figure 3B, KEGG pathway map; Supplementary Figure S2, KEGG pathway module), we could find the differences of 15 species in metabolism capability other than carbohydrate metabolism, such as Cellular processes, Environmental information processing, Genetic information processing, Amino acid metabolism, Lipid metabolism, Vitamins metabolism and energy metabolism. There were two main clusters, cluster 1 contained Lb. plantarum, Lc. cremoris, Lb. paracasei, Lc. lactis, and Sc. thermophilus, cluster 2 contained Ba. subtilis, Bi. longum, Lb. casei, Lb. fermentum, Lb. bulgaricus, Lb. helveticus, Lb. gasseri, Bi. lactis, Bi. infantis, and Lb. acidophilus. Cluster 1 was dominated by Sc. thermophilus, Lc. lactis, and Lb. paracasei, and was superior in functions associated with Bacterial chemotaxis, Flagellar assembly, Two-component system, Arginine and proline metabolism, Lysine degradation, Tryptophan metabolism, Valine, leucine, and isoleucine degradation, Oxidative phosphorylation, Biotin metabolism, Porphyrin and chlorophyll metabolism, Beta-Alanine metabolism, Iosynthesis of siderophore group non-ribosomal peptides, Geraniol degradation, Non-ribosomal peptide structures. Cluster 2 was dominated by Ba. subtilis and Bi. longum, and was superior in functions associated with Cell cycle-caulobacter, Phosphotransferase system, Homologous recombination, Aminoacyl-tRNA biosynthesis, Ribosome, Vancomycin resistance, Lysine biosynthesis, Peptidoglycan biosynthesis, Other glycan degradation, Nicotinate and nicotinamide metabolism, Purine metabolism and pyrimidine metabolism.
At the subspecies level, there were still a few differences (between Lc. cremoris and Lc. lactis, or between Bi. infantis and Bi. longum). Lc. cremoris was superior in CAZy functions associated with GH5, GT41, GT1, GT8, and in KEGG functions associated with Cysteine and methionine metabolism, Sulfur metabolism, Fatty acid biosynthesis, Nicotinate and nicotinamide metabolism, Tetracycline biosynthesis and Purine metabolism, while Lc. lactis was superior in CAZy functions associated with GH85, GH2, and in KEGG functions associated with Non-homologous end-joining, Arginine and proline metabolism, Lysine degradation, Valine, leucine and isoleucine degradation, Ascorbate and aldarate metabolism, Glyoxylate and dicarboxylate metabolism. Bi. infantis was superior in CAZy functions associated with GH33, GH95, GH29, and in KEGG functions associated with Sulfur relay system, S. aureus infection, Cysteine and methionine metabolism, Butirosin and neomycin biosynthesis, Fructose and mannose metabolism, Inositol phosphate metabolism, Fatty acid biosynthesis, Glycerolipid metabolism, Tetracycline biosynthesis, while Bi. longum was superior in CAZy functions associated with CBM41, GH121, CBM22, GH101, CBM13, GH27, CBM6, GH30, CBM32, GH31, and in KEGG functions associated with DNA replication, Homologous recombination, Mismatch repair, Nucleotide excision repair, Vibrio cholerae infection, Amino sugar and nucleotide sugar metabolism, Starch and sucrose metabolism, Peptidoglycan biosynthesis, Other glycan degradation.
The function components difference served as a foundation for species complementation and implied that species (even subspecies) diversity and characters should be taken into account for co-cultivation (Stewart, 2012).
Farther Distance for Genomes, Higher Complementarity for Function
The complementarity of KEGG module was in silico computed for each strain. As a result, 444 surveyed strains form eight distinct clusters (Figure 4A), which was in line with the previous cluster results derived from CAZy and phylogenetic tree, revealing that there were significant differences not only in genomic features but also in functions between these strains at cluster level (mostly at species level).
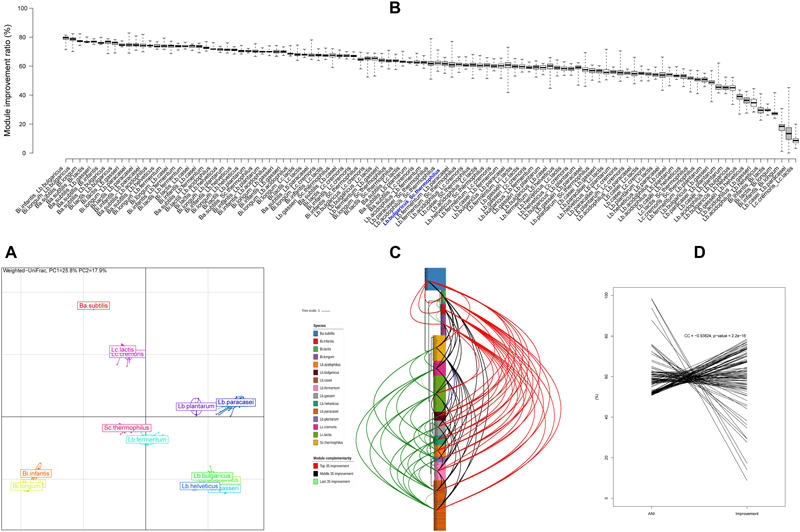
Figure 4. KEGG module feature and complementarity. (A) Principal components analysis based on the KEGG module integrity profile for all strains. Isolates on the first and second principal components (PC1 and PC2) are plotted by nodes. Lines link strains of the same species, and colored circles pack the strains near the barycenter for each species. (B) Module complementarity at strain level. (C) Module complementarity at species level showed on the phylogenetic tree with three groups; red lines, top 1/3 combination; black lines, medium combination; green lines, last 1/3 combinations; blue lines, the classical combination of Lb. bulgaricus and Sc. thermophilus. (D) Correlation between ANI and module complementarity at species level.
In addition, the complementarity improvement ratios of KEGG module were counted for each pair of strains belonging to different species. Consequently, 90,543 combinations (Supplementary Table S4) were obtained, and were displayed by the average improvement ratio at species level sequentially from high to low (Figure 4B, 105 boxes) and also displayed on the phylogenetic tree at species level (Figure 4C, 105 connection lines) and also plotted against ANI values of every species pair (Figure 4D, 105 connection lines). The complementarity improvement ratio varied over a broad range (from 0 to 82.95%), illustrating that strain heterogeneities were prevalent. The farther distance between branches, the higher complementarity for species. The smaller ANI values, the higher complementarity for species. Lb. bulgaricus and Sc. thermophilus is a classical traditional combination for yogurt starter, whereas their complementarity only laid the middle position (No. 50) of all these complementarities.
Altogether, there were huge potentials for developing more excellent multi-strain probiotic products, and it seems like that the strain with farther genomic distance will improve the function complementarity at higher levels.
Notably Different Roles in the Human Cohort Gut Microbiomes
To investigate the relative abundances and roles of MTPS in human gut microbiomes, public fecal metagenomics sequencing clean reads (n = 1,815) from obesity (Liu et al., 2017) (n = 200, 105 control and 95 case, without weight-loss intervention), type 2 diabetes (Qin et al., 2012) (T2D, n = 368, 185 control and 183 case), atherosclerotic cardio-vascular disease (Jie et al., 2017) (ACVD, n = 385, 171 control and 214 case), Crohn’s disease (He et al., 2017) (CD, n = 102, 53 control and 49 case), colorectal adenoma-carcinoma (Feng et al., 2015) (CRC, n = 147, 57 control and 90 case), liver cirrhosis (Qin et al., 2014) (LC, n = 237, 114 control and 123 case), ankylosing spondylitis (Wen et al., 2017) (AS, n = 207, 114 control and 93 case), and rheumatoid arthritis (Zhang et al., 2015) (RA, n = 169, 74 control and 95 case) human cohorts were downloaded to form species profiles by MetaPhlAn2. Detailed species enrichment direction, species abundance rank and network-linkages rank in each cohort gut microbiomes are in Supplementary Table S5.
CD is an inflammatory bowel disease, and specific pathogenic bacteria that potentially cause CD have been anchored, such as adherent-invasive Escherichia coli (He et al., 2017). In our data, there were 35 CD-enriched species and 130 CD-depleted species (Figure 5A), indicating CD might be mainly induced by the deficiency of beneficial strains. E. coli, Clostridium ramosum, and Leptotrichia buccalis might be the main contributors in CD-enriched group, while Faecalibacterium prausnitzii, Ruminococcus sp. 5_1_39BFAA, Dorea formicigenerans, Coprococcus catus, Ruminococcus obeum might be the main contributors in CD-depleted groups. It is interesting to find that B. longum was also enriched in the CD-depleted group with 13th network-rank and 21st abundance (Supplementary Table S5).
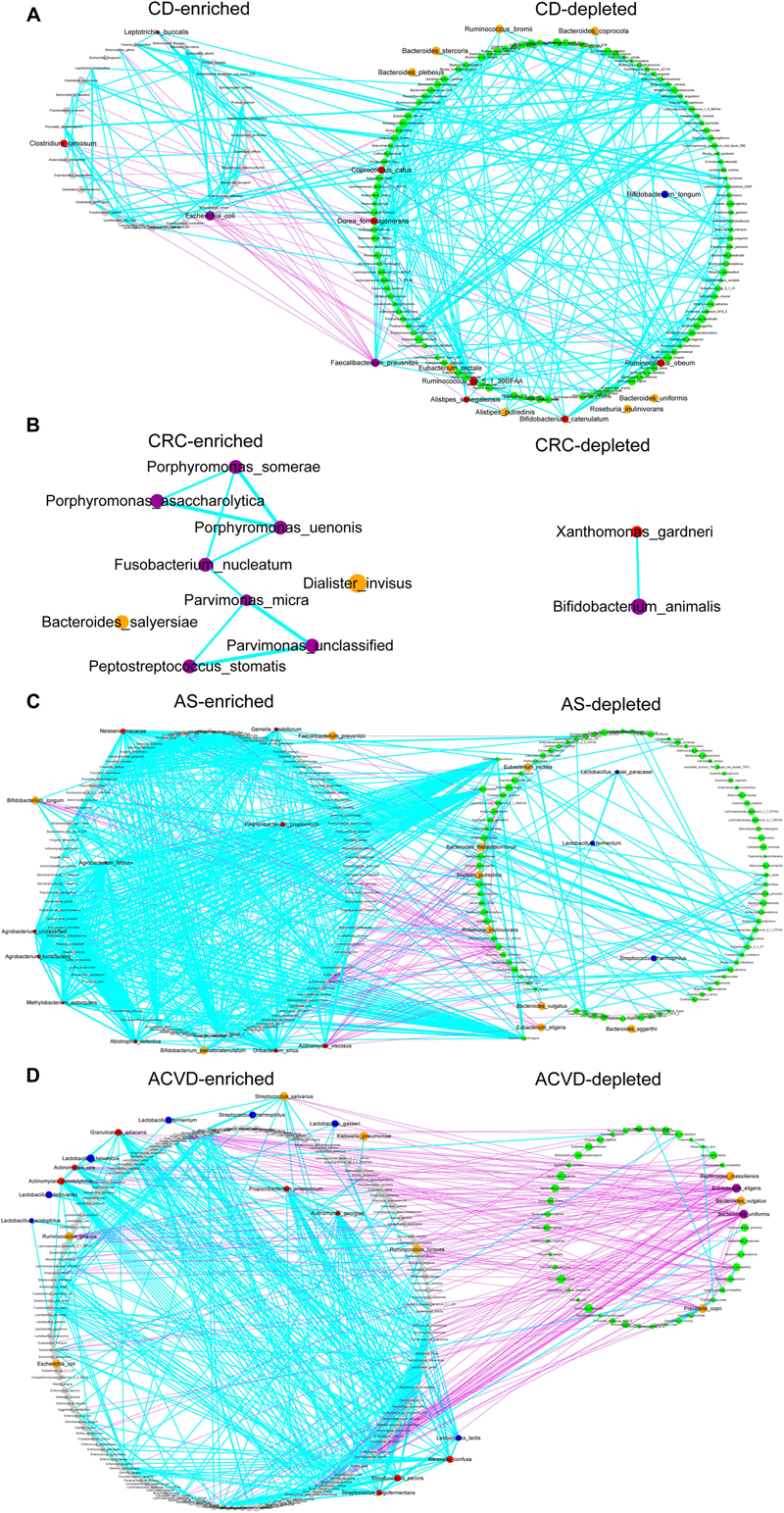
Figure 5. Traditional probiotics roles in the cohort gut microbiome. (A) The cohort of CD. (B) The cohort of CRC. (C) The cohort of AS. (D) The cohort of ACVD. Co-abundance network of species significantly enriched in case or control subjects. Left, network in individuals with disease (case-enriched); right, network in controls (case-depleted). Species with significantly different relative abundances between the groups are displayed (q-value <0.05, FDR-controlled Wilcoxon rank-sum test). Gray circles, case-enriched; green circles, control-enriched; purple circles, species with top 10 edges and top 10 abundance; red circles, species with top 10 edges; orange circles, species with top 10 abundance; blue circles, MTPS. The size of each circle indicates the mean relative abundance of species (1/10, 1/100, …, 1/10∗∗6, <1/10∗∗6). Please see Supplementary Table S5 for more detailed information on enriched or depleted species. Cyan edges means positive correlations; pink edges means negative correlations. The edge width declines along the absolute value of the Pearson cc: thick edges, |cc| >=0.7; medium, 0.4 <=|cc| <0.7; thin, 0.1 < |cc| < 0.4.
CRC is one of the top three most frequently diagnosed cancers worldwide and a chief cause of cancer mortality. The gut microbiota is believed to be directly involved in colorectal carcinogenesis (Feng et al., 2015). There were 9 CRC-enriched species and 2 CRC-depleted species (Figure 5B). Porphyromonas uenonis, Porphyromonas somerae, Fusobacterium nucleatum, Parvimonas micra, Porphyromonas asaccharolytica, Peptostreptococcus stomatis might be the main contributors in CRC-enriched group, while B. animalis was one of the two CRC-depleted group members. Altogether, CRC might be mainly induced by the excessive growth of harmful strains.
AS is a chronic, systemic, inflammatory autoimmune disease featured by the inflammation of the axial skeleton, the peripheral joints, and the attachments of ligaments and enthuses (Wen et al., 2017). There were 108 AS-enriched species and 96 AS-depleted species (Figure 5C), indicating AS might be a complicated disease with both excessive growth of harmful strains and deficiency of beneficial strains. Lb. fermentum, Lb. casei paracasei, and St. thermophilus of MTPS were the AS-depleted group members, while B. longum, which showed benefits in CD disease, was enriched in AS-enriched group with potentially adverse effects.
ACVD is a cardiometabolic disease associated with high morbidity and mortality (Jie et al., 2017). There were 169 ACVD-enriched species and 46 ACVD-depleted species (Figure 5D), indicating ACVD might be a complicated disease with both mainly excessive growth of harmful strains and remarkable deficiency of beneficial strains. Unexpectedly, Lb. fermentum, Lb. acidophilus, Lb. delbrueckii, Lb. gasseri, Lb. helveticus, Lc. lactis, and St. thermophilus of MTPS were the ACVD-enriched group members. On the other hand, Bacteroides uniformis, Eubacterium eligens, Prevotella copri, Bacteroides vulgatus, and Bacteroides massiliensis were in ACVD-depleted group with top network-linkages and top abundances, suggesting that they might be the main contributors and the novel potential candidate probiotics for ACVD prevention and remission.
LC is an advanced-stage liver disease originating from acute or chronic liver injury, comprising alcohol abuse, obesity, and hepatitis virus infection (Qin et al., 2014). There were 246 LC-enriched species and 113 LC-depleted species (Supplementary Figure S3a), indicating LC might be a complicated disease with both mainly excessive growth of harmful strains and remarkable deficiency of beneficial strains. Veillonella unclassified, Veillonella parvula, Haemophilus parainfluenzae, Haemophilus influenzae and so on were the main contributors in the LC-enriched group. Unexpectedly, Lb. fermentum, Lb. delbrueckii, Lb. gasseri, and St. thermophilus (all four were ACVD-enriched) of MTPS were the LC-enriched group members, showing a very similar phenomenon between LC and ACVD. On the other hand, Bilophila unclassified, Alistipes senegalensis, Alistipes putredinis, Coprococcus catus were in LC-depleted group with top network-linkages, suggesting that they might be the main contributors and the potential candidate novel probiotics for LC prevention and remission.
T2D is a complicated disorder affected by both genetic and environmental components, and has become a major public health problem worldwide (Qin et al., 2012). There were 23 T2D-enriched species and 21 T2D-depleted species (Supplementary Figure S3b), indicating that T2D might not be as complicated as AS, ACVD, and LC. Clostridiales bacterium 1_7_47FAA, Eggerthella lenta, Lactobacillus mucosae, C. ramosum, Clostridium citroniae and so on might be the main contributors in the T2D-enriched group. Unexpectedly, Lb. helveticus of MTPS was the non-primary member in the T2D-enriched group. On the other hand, Faecalibacterium prausnitzii, Actinobacillus unclassified, Roseburia inulinivorans, and Eubacterium rectale were in the T2D-depleted group with top network-linkages, suggesting that they might be the main contributor and the potential candidate for novel probiotics for T2D prevention and remission. Although H. parainfluenzae, Haemophilus sputorum, and Haemophilus paraphrohaemolyticus were in T2D-depleted group with top network-linkages, even some in top abundance, their roles should be seriously recognized since H. parainfluenzae was enriched in LC.
Obesity has become a global epidemic and is a major risk factor for type 2 diabetes, cardiovascular diseases and certain cancers. Evidence has accumulated that the gut microbiota is an important environmental factor contributing to obesity by altering host energy harvest and storage (Cox et al., 2015). There were 60 Obesity-enriched species and 50 Obesity-depleted species (Supplementary Figure S3c), indicating Obesity might be not so complicated as to AS, ACVD and LC. Streptococcus mitis, Streptococcus mitis/oralis/pneumoniae, Streptococcus pneumoniae, Ruminococcus gnavus, Abiotrophia defectiva, Granulicatella elegans, Streptococcus oligofermentans, Gemella sanguinis, and Streptococcus cristatus might be the main contributors in Obesity-enriched group. On the other hand, Alistipes shahii, A. putredinis, and Odoribacter splanchnicus were in Obesity-depleted group with top network-linkages, suggesting that they might be the main contributor and the potential candidate novel probiotics for Obesity prevention and remission. It is interesting to see that MTPS showed no significant effects in both groups.
RA is a common autoimmune disorder that causes progressive disability and systemic complications. There were 4 RA-enriched species and 11 RA-depleted species (Supplementary Figure S3d), indicating RA might be not so complicated as to AS, ACVD and LC. Atopobium parvulum, Actinomyces oris, Actinomyces viscosus, and Lactobacillus salivarius might be the main contributors in RA-enriched group. On the other hand, Megamonas hypermegale, Megamonas unclassified, Megamonas funiformis, Megamonas rupellensis, Xanthomonas gardneri, Pseudomonas protegens, and Pseudomonas extremaustralis were in RA-depleted group with top network-linkages, suggesting that they might be the main contributor and the potential candidate novel probiotics for RA prevention and remission. It is interesting to see that MTPS showed no significant effects in both groups, as same as Obesity.
After manual inspection, we found that the enriched species enrichment directions in eight cohorts in this study were basically in agreement with the reports of literatures from which the public data derived. Except for B. animalis in CRC, MTPS were not the main contributors in disease association, even make no influences (in Obesity and RA cohorts), with respect to relative abundance, co-abundance networks and enriched MTPS proportion in the total enriched species in each cohort.
To get a stronger proof for the reliabilities of species enrichment directions and the relative abundance of MTPS based on the species annotation by MetaPhlAn2, the host-free clean reads of ACVD cohorts as a representative were directly mapped onto each gene set of the 15 species, respectively. Difference of reads mapping ratio between control and case were tested by unpaired Wilcoxon-rank sum test. As shown in Supplementary Figure S4a, at species level, Bi. infantis and Bi. longum were high-abundance, while other species were very low-abundance in human gut microbiomes. The enrichment direction for above-mentioned ACVD-enriched species among MTPS, such as Lb. fermentum, Lb. acidophilus, Lb. bulgaricus, Lb. gasseri, Lb. helveticus, Lc. Lactis, and St. thermophilus, were in line with that by MetaPhlAn2.
To further investigate whether each strain of high-abundance species show significances of reads mapping ratio between control and case, all strains belonging to Bi. infantis and Bi. longum were, respectively, mapped by ACVD reads. As a result, there were no significant differences (adjusted p-value > 0.05, unpaired Wilcoxon-rank sum test) between control group and case group at strain level (Supplementary Figure 4b).
Discussion
Designing multi-strain probiotics products combining pre-existing strains with ever-increasing newly isolated ones is challenging, since it is costly to conduct physiological and biochemical experiments by combining them together and personalized probiotics (Zmora et al., 2018) consumption would make a future trend. As many as possible, this study collected the public genome sequences of the bacterial names deduced from the labels of probiotics products and one children OTC drug on the Chinese supermarket and conducted comparative genomic analyses. We recruited genomes with high-quality standard (Chain et al., 2009), which are suited to mine all associated phylogenetic and functional information, and enable analyses for genome regions of interest or whole genomes (Sun et al., 2015a). Firstly, we defined PI and investigate the impact factors on the openness of pan-genome, showing that there were notably differences in the openness level of pan-genome in different species, which might be affected by multi factors encompassing the strain isolation source, the genome characteristics and the number of genomes within species. Thus, when selecting strains, the strains with open pan-genome or subspecies should be looked into more carefully than other relatively conservative species. Secondly, we compared the safety scores with respect to virulence factor, antibiotic resistance genes between groups with friendly isolation sources (e.g., milk, dairy or specimen from healthy people) or unfriendly sources, showing that the strains isolated from the friendly ecological niche harbored significant lower virulence factors and antibiotic resistance genes, while it must be admitted that the reported isolation source of any involved species might not necessarily be where it evolved (Sun et al., 2015a). Thirdly, we highlighted the species/strain enriched/specific advantage of metabolic capacity and quantified the combination complementarity based on KEGG module function, demonstrating that farther genetic distance resulted in a greater possibility to achieve stronger combinations. However, co-cultivation experiments are still needed in the future (Marmann et al., 2014).
With respect to the species (MetaPhlAn2) enrichment analyses and co-abundance association analyses, some of MTPS showed benefits to digestive tract-associated diseases, such as CD (Bi. longum) and CRC (B. animalis), and solid organ-associated diseases, such as LC (Ba. subtilis) and AS (Lb. casei_paracasei, Lb. fermentum, Sc. thermophilus). Whereas some of MTPS showed potentially adverse effects to cardiometabolic diseases, such T2D (Lb. helveticus) and ACVD (Lb. acidophilus, Lactobacillus_delbrueckii, Lb. fermentum, Lb. gasseri, Lb. helveticus, Lactococcus_lactis, Sc. thermophilus), and solid organ associated diseases, such as LC (Lactobacillus_delbrueckii, Lb. fermentum, Lb. gasseri, Lb. helveticus, Sc. thermophilus) and AS (Bifidobacterium_longum). Additionally, with respect to the reads mapping results, most of MTPS were relatively more abundant in ACVD group than control group, which was basically in line with the result by MetaPhlAn2. However, there were too few mapped reads for most of the MTPS, therefore it remains to be seen whether this trend will apply to other experiments (and whether these species will indeed be prevalent in the human gut). And even if this phenomenon will be corroborated, the impacts on human health might be very limited due to their rather low proportion and their limited role, compared to other key players among gut microbiota. It is worth noting that both the differences in the gut microbiota abundance but also the differences in its metabolism and functions need to be taken into consideration in evaluating their contributions to the physiopathology of related diseases (Villanueva-Millan et al., 2015). In any case, it seems that the patients of T2D, LC, AS or ACVD might pay more attention to the selection of live probiotic products with best-suited strains.
On the other hand, the metagenomics-based microbiome abundance comparison between case and control subjects might lead to the false claim that a bacterium is causally correlated with the protection or the promotion of a disease (Cani, 2018). In fact, environmental factors such as dietary habits, drug treatments, intestinal motility, and stool frequency and consistency are all factors that influence the composition of the microbiota and should be considered (Cani, 2018). Insights into the impacts of spectrum and power of MTPS on the host still need to be strengthened in the future.
All in all, although the causal role of MTPS in gut microbiome of eight cohorts, especially T2D, ACVD, LC, and AS, still needs further investigation, our analyses exhibit a global correlation between gut microbiome (including MTPS) and the protection or the promotion of common diseases. This study provides conceptual advances that lays the foundation for future applications in personalized probiotics, which must consider both the species/strain heterogeneity and individual heterogeneity.
Author Contributions
GH and GL designed the study. GL, BL, CY, YW, XB, WL, and FL performed the experiments and analyzed the data. GL wrote the manuscript.
Funding
This research was supported by the National Key R&D Program of China (Grant No. 2017YFD0400303).
Conflict of Interest Statement
The authors declare that the research was conducted in the absence of any commercial or financial relationships that could be construed as a potential conflict of interest.
Acknowledgments
We are indebted to many additional faculty and staff of KLDS who contributed to this work. We thank Smith Etareri Evivie for the support to the manuscript revision.
Supplementary Material
The Supplementary Material for this article can be found online at: https://www.frontiersin.org/articles/10.3389/fmicb.2019.00712/full#supplementary-material
FIGURE S1 | Comparison of risk factors between relatively friendly group and relatively unfriendly group. Unpaired wilcoxon-rank sum test, ∗p < 0.05, ∗∗p < 0.01.
FIGURE S2 | Different relatively enhanced function of KEGG pathway module. The number in the cell indicated how many other species are significantly weaker than this species in this function (q-value < 0.05, FDR-controlled Wilcoxon rank-sum test).
FIGURE S3 | Traditional probiotics roles in another four cohort gut microbiome. (a) The cohort of LC. (b) The cohort of T2D. (c) The cohort of Obesity. (d) The cohort of RA. The notes for the networks are same as that in Figure 5.
FIGURE S4 | Difference of reads mapping ratio between control and case in ACVD at species or strain level. (a) Species level for 15 species. (b) Strain level within Bi.infantis and Bi.longum. Unpaired wilcoxon-rank sum test, ∗p < 0.05, ∗∗p < 0.01, ∗∗∗p < 0.001, ∗∗∗∗p < 0.0001.
TABLE S1 | Detailed genomic information for all the 444 strains.
TABLE S2 | The ANI matrix for all the 444 strains.
TABLE S3 | Caption for Sheet “risk.factors”: Species-enriched VFDB and ARDB gene counts and annotations. Caption for Sheet “CAZy”: Species-enriched CAZy gene counts and annotations.
TABLE S4 | The complementarity improvement ratios of KEGG module for each pair strains belonging to different species.
TABLE S5 | Detailed species enrichment direction, species abundance rank and network-linkages rank in each cohort gut microbiomes.
Footnotes
References
Besemer, J., and Borodovsky, M. (2005). GeneMark: web software for gene finding in prokaryotes, eukaryotes and viruses. Nucleic Acids Res. 33, W451–W454. doi: 10.1093/nar/gki487
Borges, N. A., Carmo, F. L., Stockler-Pinto, M. B., de Brito, J. S., Dolenga, C. J., Ferreira, D. C., et al. (2018). Probiotic supplementation in chronic kidney disease: a double-blind, randomized, placebo-controlled trial. J. Ren. Nutr. 28, 28–36. doi: 10.1053/j.jrn.2017.06.010
Callister, S. J., McCue, L. A., Turse, J. E., Monroe, M. E., Auberry, K. J., Smith, R. D., et al. (2008). Comparative bacterial proteomics: analysis of the core genome concept. PLoS One 3:e1542. doi: 10.1371/journal.pone.0001542
Cani, P. D. (2018). Human gut microbiome: hopes, threats and promises. Gut 67, 1716–1725. doi: 10.1136/gutjnl-2018-316723
Cantarel, B. L., Coutinho, P. M., Rancurel, C., Bernard, T., Lombard, V., and Henrissat, B. (2009). The Carbohydrate-Active EnZymes database (CAZy): an expert resource for glycogenomics. Nucleic Acids Res. 37, D233–D238. doi: 10.1093/nar/gkn663
Capella-Gutierrez, S., Silla-Martinez, J. M., and Gabaldon, T. (2009). trimAl: a tool for automated alignment trimming in large-scale phylogenetic analyses. Bioinformatics 25, 1972–1973. doi: 10.1093/bioinformatics/btp348
Chain, P. S., Grafham, D. V., Fulton, R. S., Fitzgerald, M. G., Hostetler, J., Muzny, D., et al. (2009). Genomics. Genome project standards in a new era of sequencing. Science 326, 236–237. doi: 10.1126/science.1180614
Chen, L., Zheng, D., Liu, B., Yang, J., and Jin, Q. (2016). VFDB 2016: hierarchical and refined dataset for big data analysis–10 years on. Nucleic Acids Res. 44, D694–D697. doi: 10.1093/nar/gkv1239
Cox, A. J., West, N. P., and Cripps, A. W. (2015). Obesity, inflammation, and the gut microbiota. Lancet Diabetes Endocrinol. 3, 207–215.
Duranti, S., Milani, C., Lugli, G. A., Mancabelli, L., Turroni, F., Ferrario, C., et al. (2016). Evaluation of genetic diversity among strains of the human gut commensal Bifidobacterium adolescentis. Sci. Rep. 6:23971. doi: 10.1038/srep23971
Feng, Q., Liang, S., Jia, H., Stadlmayr, A., Tang, L., Lan, Z., et al. (2015). Gut microbiome development along the colorectal adenoma-carcinoma sequence. Nat. Commun. 6:6528. doi: 10.1038/ncomms7528
He, Q., Gao, Y., Jie, Z., Yu, X., Laursen, J. M., Xiao, L., et al. (2017). Two distinct metacommunities characterize the gut microbiota in Crohn’s disease patients. Gigascience 6, 1–11. doi: 10.1093/gigascience/gix050
Human Microbiome Jumpstart Reference Strains, C., Nelson, K. E., Weinstock, G. M., Highlander, S. K., Worley, K. C., Creasy, H. H., et al. (2010). A catalog of reference genomes from the human microbiome. Science 328, 994–999. doi: 10.1126/science.1183605
Jie, Z., Xia, H., Zhong, S. L., Feng, Q., Li, S., Liang, S., et al. (2017). The gut microbiome in atherosclerotic cardiovascular disease. Nat. Commun. 8:845. doi: 10.1038/s41467-017-00900-1
Kanehisa, M., and Goto, S. (2000). KEGG: kyoto encyclopedia of genes and genomes. Nucleic Acids Res. 28, 27–30. doi: 10.1093/nar/28.1.27
Kim, Y., Koh, I., Young Lim, M., Chung, W. H., and Rho, M. (2017). Pan-genome analysis of Bacillus for microbiome profiling. Sci. Rep. 7:10984. doi: 10.1038/s41598-017-11385-9
Land, M., Hauser, L., Jun, S. R., Nookaew, I., Leuze, M. R., Ahn, T. H., et al. (2015). Insights from 20 years of bacterial genome sequencing. Funct. Integr. Genomics 15, 141–161. doi: 10.1007/s10142-015-0433-4
Letunic, I., and Bork, P. (2016). Interactive tree of life (iTOL) v3: an online tool for the display and annotation of phylogenetic and other trees. Nucleic Acids Res. 44, W242–W245. doi: 10.1093/nar/gkw290
Li, R., Yu, C., Li, Y., Lam, T. W., Yiu, S. M., Kristiansen, K., et al. (2009). SOAP2: an improved ultrafast tool for short read alignment. Bioinformatics 25, 1966–1967. doi: 10.1093/bioinformatics/btp336
Li, W., and Godzik, A. (2006). Cd-hit: a fast program for clustering and comparing large sets of protein or nucleotide sequences. Bioinformatics 22, 1658–1659. doi: 10.1093/bioinformatics/btl158
Liu, B., and Pop, M. (2009). ARDB–antibiotic resistance genes database. Nucleic Acids Res. 37, D443–D447. doi: 10.1093/nar/gkn656
Liu, R., Hong, J., Xu, X., Feng, Q., Zhang, D., Gu, Y., et al. (2017). Gut microbiome and serum metabolome alterations in obesity and after weight-loss intervention. Nat. Med. 23, 859–868. doi: 10.1038/nm.4358
Lombard, V., Golaconda Ramulu, H., Drula, E., Coutinho, P. M., and Henrissat, B. (2014). The carbohydrate-active enzymes database (CAZy) in 2013. Nucleic Acids Res. 42, D490–D495. doi: 10.1093/nar/gkt1178
Loytynoja, A. (2014). Phylogeny-aware alignment with PRANK. Methods Mol. Biol. 1079, 155–170. doi: 10.1007/978-1-62703-646-7_10
Luo, R., Liu, B., Xie, Y., Li, Z., Huang, W., Yuan, J., et al. (2012). SOAPdenovo2: an empirically improved memory-efficient short-read de novo assembler. Gigascience 1:18. doi: 10.1186/2047-217X-1-18
Marmann, A., Aly, A. H., Lin, W., Wang, B., and Proksch, P. (2014). Co-cultivation–a powerful emerging tool for enhancing the chemical diversity of microorganisms. Mar. Drugs 12, 1043–1065. doi: 10.3390/md12021043
Mende, D. R., Sunagawa, S., Zeller, G., and Bork, P. (2013). Accurate and universal delineation of prokaryotic species. Nat. Methods 10, 881–884. doi: 10.1038/nmeth.2575
Mount, D. W. (2007). Using the basic local alignment search tool (BLAST). CSH Protoc. 2007:dbto17. doi: 10.1101/pdb.top17
Pararajasingam, A., and Uwagwu, J. (2017). Lactobacillus: the not so friendly bacteria. BMJ Case Rep. 2017:bcr-2016-218423. doi: 10.1136/bcr-2016-218423
Parks, D. H., Imelfort, M., Skennerton, C. T., Hugenholtz, P., and Tyson, G. W. (2015). CheckM: assessing the quality of microbial genomes recovered from isolates, single cells, and metagenomes. Genome Res. 25, 1043–1055. doi: 10.1101/gr.186072.114
Piewngam, P., Zheng, Y., Nguyen, T. H., Dickey, S. W., Joo, H. S., Villaruz, A. E., et al. (2018). Pathogen elimination by probiotic Bacillus via signalling interference. Nature 562, 532–537. doi: 10.1038/s41586-018-0616-y
Qin, J., Li, Y., Cai, Z., Li, S., Zhu, J., Zhang, F., et al. (2012). A metagenome-wide association study of gut microbiota in type 2 diabetes. Nature 490, 55–60. doi: 10.1038/nature11450
Qin, N., Yang, F., Li, A., Prifti, E., Chen, Y., Shao, L., et al. (2014). Alterations of the human gut microbiome in liver cirrhosis. Nature 513, 59–64. doi: 10.1038/nature13568
Richter, M., and Rossello-Mora, R. (2009). Shifting the genomic gold standard for the prokaryotic species definition. Proc. Natl. Acad. Sci. U.S.A. 106, 19126–19131. doi: 10.1073/pnas.0906412106
Ronholm, J., Nasheri, N., Petronella, N., and Pagotto, F. (2016). Navigating microbiological food safety in the era of whole-genome sequencing. Clin. Microbiol. Rev. 29, 837–857. doi: 10.1128/CMR.00056-16
Segata, N., Waldron, L., Ballarini, A., Narasimhan, V., Jousson, O., and Huttenhower, C. (2012). Metagenomic microbial community profiling using unique clade-specific marker genes. Nat. Methods 9, 811–814. doi: 10.1038/nmeth.2066
Shannon, P., Markiel, A., Ozier, O., Baliga, N. S., Wang, J. T., Ramage, D., et al. (2003). Cytoscape: a software environment for integrated models of biomolecular interaction networks. Genome Res. 13, 2498–2504. doi: 10.1101/gr.1239303
Shen, N. T., Maw, A., Tmanova, L. L., Pino, A., Ancy, K., Crawford, C. V., et al. (2017). Timely use of probiotics in hospitalized adults prevents clostridium difficile infection: a systematic review with meta-regression analysis. Gastroenterology 152:1889-1900.e9. doi: 10.1053/j.gastro.2017.02.003
Stamatakis, A. (2014). RAxML version 8: a tool for phylogenetic analysis and post-analysis of large phylogenies. Bioinformatics 30, 1312–1313. doi: 10.1093/bioinformatics/btu033
Stewart, E. J. (2012). Growing unculturable bacteria. J. Bacteriol. 194, 4151–4160. doi: 10.1128/JB.00345-12
Suez, J., Zmora, N., Zilberman-Schapira, G., Mor, U., Dori-Bachash, M., Bashiardes, S., et al. (2018). Post-antibiotic gut mucosal microbiome reconstitution is impaired by probiotics and improved by autologous FMT. Cell 174:1406-1423.e16. doi: 10.1016/j.cell.2018.08.047
Sun, Z., Harris, H. M., McCann, A., Guo, C., Argimon, S., Zhang, W., et al. (2015a). Expanding the biotechnology potential of lactobacilli through comparative genomics of 213 strains and associated genera. Nat. Commun. 6:8322. doi: 10.1038/ncomms9322
Sun, Z., Zhang, W., Guo, C., Yang, X., Liu, W., Wu, Y., et al. (2015b). Comparative genomic analysis of 45 type strains of the genus Bifidobacterium: a snapshot of its genetic diversity and evolution. PLoS One 10:e0117912. doi: 10.1371/journal.pone.0117912
Tettelin, H., Masignani, V., Cieslewicz, M. J., Donati, C., Medini, D., Ward, N. L., et al. (2005). Genome analysis of multiple pathogenic isolates of Streptococcus agalactiae: implications for the microbial “pan-genome”. Proc. Natl. Acad. Sci. U.S.A. 102, 13950–13955. doi: 10.1073/pnas.0506758102
Thompson, C. C., Chimetto, L., Edwards, R. A., Swings, J., Stackebrandt, E., and Thompson, F. L. (2013). Microbial genomic taxonomy. BMC Genomics 14:913. doi: 10.1186/1471-2164-14-913
Villanueva-Millan, M. J., Perez-Matute, P., and Oteo, J. A. (2015). Gut microbiota: a key player in health and disease. A review focused on obesity. J. Physiol. Biochem. 71, 509–525. doi: 10.1007/s13105-015-0390-3
Wang, J., and Jia, H. (2016). Metagenome-wide association studies: fine-mining the microbiome. Nat. Rev. Microbiol. 14, 508–522. doi: 10.1038/nrmicro.2016.83
Wen, C., Zheng, Z., Shao, T., Liu, L., Xie, Z., Le Chatelier, E., et al. (2017). Quantitative metagenomics reveals unique gut microbiome biomarkers in ankylosing spondylitis. Genome Biol. 18:142. doi: 10.1186/s13059-017-1271-6
Ye, Y., Choi, J. H., and Tang, H. (2011). RAPSearch: a fast protein similarity search tool for short reads. BMC Bioinformatics 12:159. doi: 10.1186/1471-2105-12-159
Yu, J., Zhao, J., Song, Y., Zhang, J., Yu, Z., Zhang, H., et al. (2018). Comparative genomics of the herbivore gut symbiont lactobacillus reuteri reveals genetic diversity and lifestyle adaptation. Front. Microbiol. 9:1151. doi: 10.3389/fmicb.2018.01151
Zhang, W., Du, P., Zheng, H., Yu, W., Wan, L., and Chen, C. (2014). Whole-genome sequence comparison as a method for improving bacterial species definition. J. Gen. Appl. Microbiol. 60, 75–78. doi: 10.2323/jgam.60.75
Zhang, X., Zhang, D., Jia, H., Feng, Q., Wang, D., Liang, D., et al. (2015). The oral and gut microbiomes are perturbed in rheumatoid arthritis and partly normalized after treatment. Nat. Med. 21, 895–905. doi: 10.1038/nm.3914
Keywords: major traditional probiotics species, comparative genomic analyses, complementarity potentials, roles in gut microbiome, cohorts
Citation: Luo G, Li B, Yang C, Wang Y, Bian X, Li W, Liu F and Huo G (2019) Major Traditional Probiotics: Comparative Genomic Analyses and Roles in Gut Microbiome of Eight Cohorts. Front. Microbiol. 10:712. doi: 10.3389/fmicb.2019.00712
Received: 21 November 2018; Accepted: 21 March 2019;
Published: 09 April 2019.
Edited by:
Giuseppe Spano, University of Foggia, ItalyReviewed by:
Manoj Kumar, ICMR-National Institute for Research in Environmental Health, IndiaBolin Zhang, Beijing Forestry University, China
Copyright © 2019 Luo, Li, Yang, Wang, Bian, Li, Liu and Huo. This is an open-access article distributed under the terms of the Creative Commons Attribution License (CC BY). The use, distribution or reproduction in other forums is permitted, provided the original author(s) and the copyright owner(s) are credited and that the original publication in this journal is cited, in accordance with accepted academic practice. No use, distribution or reproduction is permitted which does not comply with these terms.
*Correspondence: Guicheng Huo, Z3VpY2hlbmdodW9AMTI2LmNvbQ==
†These authors have contributed equally to this work