- State Key Laboratory of Microbial Metabolism, Joint International Research Laboratory of Metabolic & Developmental Sciences, School of Life Sciences and Biotechnology, Shanghai Jiao Tong University, Shanghai, China
Soil is a unique environment in which the microbiota is frequently subjected to community coalescence. Additions of organic fertilizer and precipitation of dust induce coalescent events in soil. However, the fates of these communities after coalescence remain uncharted. Thus, to explore the effects of microbiota coalescence, we performed reciprocal inoculation and incubation experiments in microcosms using two distinct soils. The soils were, respectively, collected from a cropland and an industrial site, and the reciprocal inoculation was performed as models for the incursion of highly exotic microbiota into the soil. After incubation under either aerobic or anaerobic conditions for two months, the soils were assayed for their bacterial community structure and denitrification function. According to the 16S rRNA gene sequencing results, the inoculated soil showed a significant shift in bacterial community structure after incubation—particularly in the industrial soil. The structures of the bacterial communities changed following the coalescence but were predicted to have the same functional potential, e.g., nitrogen metabolism, as determined by the quantification of denitrifying genes and nitrogen gas production in the inoculated soil samples, which showed values equivalent those in the original recipient soil samples regardless of inoculum used. The functional prediction based on the known genomes of the taxa that shifted in the incubated sample communities indicates that the high functional overlap and redundancy across bacteria acted as a mechanism that preserved all the metabolic functions in the soil. These findings hint at the mechanisms underlying soil biodiversity maintenance and ecosystem function.
Introduction
Recent investigations have drawn attention to community coalescence, which is a newly coined term describing the phenomena of community-community encounters in which two or more entire communities (and their environments) interact and assemble into a coalescent community (Rillig et al., 2015, 2016a; Tikhonov, 2016; Rillig and Mansour, 2017). Because microbes are relatively small compared to their habitats, even small habitat volumes contain microbial communities with a great diversity of microbes. A mixing of exotic and resident microbiota can easily occur following additions of soil, litter, water flow and anthropogenic materials. Coalescence is likely an important factor for community assembly (Tikhonov, 2016) because the newly assembled community cannot easily be separated into different parts. Moreover, because the characteristics of the assembled community differ from those of the original communities, the significance of the effects of microbiota coalescence has recently been emphasized (Mansour et al., 2018) and may be fundamental for the generation of microbial communities (Rillig and Mansour, 2017).
Compared with other environments, soils harbor an unparalleled diversity of microorganisms (Fierer et al., 2012) and are uniquely suited for studying the coalescence of complex microbiota. Examples of the drivers of coalescence include the action of earthworms, the dynamics of soil aggregates, tillage, litter-fall, outplanting, flooding and the addition of materials containing existing microbial communities (such as stored biochar, manure or compost) (Rillig et al., 2016a). Human-associated pathways, including agricultural activities, can also accidentally transport exotic microbiota to soils (McNeill et al., 2011; Rillig et al., 2016a).
A number of investigations have already demonstrated that microbial coalescence is an important driving force in the assembly of soil microbial communities (Rillig et al., 2016b). An experiment performed with a mixture of soils from agricultural, forest and grassland sites to increase the diversity of soil microbes in the initial material and improve the ability of the soil to support the above-ground plants showed that the coalescence of soil communities promoted subsequent increases in plant biomass and an accompanying enhancement of microbial extracellular enzyme activities associated with nitrogen mineralization (Panke-Buisse et al., 2015; Rillig et al., 2016b). The reproducibility of the flowering phenotype across plant hosts suggests that microbiome mixtures can be selected that modify plant traits and coordinate changes in soil resource pools (Panke-Buisse et al., 2015; Rillig et al., 2016b). Another investigation using a culture-based method showed that adding sterilized leaves to soil led to a significantly different structure of the microbiota in leaf litter compared to leaf litter from which the endophyte community was not excluded (Osono, 2005). This outcome suggests that with the exception of nutrition, endophytes from the leaves contributed to the assembly of soil microbiota. In another experiment, root-colonizing microbiota in soil of Dactylis glomerata was observed to be similar to neighboring microbiota associated with Centaurea maculosa rather than with the typical root microbiota of D. glomerata, indicating the coalescence of root microbiota in the soil (Mummey et al., 2005). This finding was subsequently confirmed by Hausmann and Hawkes (2009). Thus, investigations into the coalescence of indigenous and invading communities are essential for understanding the mechanism by which soil microbiota is generated in situ.
Additionally, there has been growing interest in mixing foreign soil or other materials to improve soil-dressing technology in recent years (Swenson et al., 2000), especially in China (Zhou et al., 2003). However, little is known regarding the effects associated with the use of these technologies on soil ecosystems (Rillig et al., 2016a). In this study, we evaluated the ecological effects of the coalescence of two distinct bacterial communities from an agricultural soil and a severely contaminated industrial soil. This experiment may mimic the application of industrial waste to agricultural crop fields, where the introduction of polluted soil and their influences on the native soil microbiota and function is a serious concern. Thus, we set up microcosms and performed reciprocal inoculation using these two distinct soils. After two months of incubation, the bacterial compositions of the soil microcosms were examined, and the functional metagenomes were predicted based on 16S rRNA gene sequencing. The soil physicochemical parameters, nitrogen cycling-associated gene copy numbers, and nitrogen reduction function were also evaluated.
Materials and Methods
Soil Sampling
Two different soils were used in this investigation. The first soil sample (ACS) was collected from an industrial site in Baoshan (31°41′N, 121°46′E), Shanghai, where the soil has been severely contaminated by organic pollutants for many years, particularly aromatic compounds. The ACS soil samples were sampled using GeoProbe Systems (GeoProbe Inc., United States) at a depth of 150 cm. The parent material at the Baoshan site is primarily Yangtze River alluvial soil. The second soil sample (ONS) was obtained from cultivated cropland under optimized nitrogen fertilization and straw management practices in Quzhou (36°86′N, 115°02′E), Hebei Province. The ONS soil samples were collected from the top 20 cm using a sterile manual corer (10-cm diameter). The fields at the sampling site were planted on intensively managed agricultural soils typical of the North China Plain, where winter wheat–summer maize rotation is the dominant crop production system, and urea and ammonium (NH4+)-based fertilizers are the most commonly applied nitrogen fertilizers. The soil of the North China Plain is classified as a calcareous fluvo-aquic soil (Huang et al., 2013; Yang et al., 2017). The initial water-holding capacities (WHC) of the ONS and ACS soils were 38 and 49%, respectively. Samples of both soils were placed in sterile plastic self-sealing bags and kept on ice until being transported to the lab, as described previously (Xun et al., 2015). After sieving the soils, sterile water was added to maintain a constant moisture level (26 and 19% of field capacity for the ACS and ONS soils, respectively).
Reciprocal Inoculation of the Two Different Soils
In the mixture groups, 3 g each of the ONS and ACS soils were reciprocally inoculated and mixed with 30 g each of the ACS and ONS soils. The mixtures were subsequently individually incubated for 2 months under aerobic and anaerobic conditions (the details of the reciprocal inoculation experimental design are described in Table 1). For the control groups, 30 g of either ONS or ACS soil was incubated for 2 months under the same aerobic and anaerobic conditions (Table 1). Each soil sample was vigorously stirred with a sterile glass rod in a 100-mL vial, which was subsequently sealed for incubation. The bottles for the aerobic management treatment were sealed directly under a laminar flow bench (Shanghai, China), while the air in the anaerobic management treatment bottles was replaced with helium using a pump ventilation system (Shanghai, China). The vials were incubated at 25°C in darkness, and each treatment was performed in triplicate.
Determination of Basic Soil Characteristics
The soil pH was measured with a pH meter (Mettler-Toledo, Switzerland) at a soil: water ratio of 1:2.5. Dissolved organic carbon (DOC) was determined using an Elab-TOC analyzer (Suzhou Elab Analytical Instrument Co., Ltd., China), and dissolved nitrogen (DN) was determined using a Shimadzu TN (total nitrogen) unit (Shimadzu Corporation, Japan).
Analysis of the Soil Bacterial Community
DNA was extracted from 0.5 g of each soil sample with cetyltrimethylammonium ammonium bromide (CTAB), as described previously (Griffiths et al., 2000; Paulin et al., 2013). DNA quality was assessed based on the 260/280 nm absorbance ratio, which was measured using a BioDrop μLITE device (Biochrom, United Kingdom).
The DNA from 26 soil samples was sequenced, including 24 samples from the eight treatment groups (triplicates in each groups) and two samples from the original untreated soils (ONS and ACS) (Table 1 and Supplementary Table S1). The extracted DNA was used as a template to amplify the V3–V4 region of the 16S rRNA gene. The PCR operation, sequencing of the PCR amplicons and raw data analysis were performed in accordance with the protocol provided by Illumina for the MiSeq system (Part # 15044223 Rev. B; Illumina Inc., United States) as previously described with minor modifications (Qin et al., 2018). The preparation of the sequencing library, including DNA extraction and PCR amplification, was conducted as previously described (Qin et al., 2019). Specifically, the V3–V4 hypervariable region of the bacterial 16S rRNA gene was amplified from the genomic DNA using the universal primer set B341F/B785F (Li et al., 2018). The purified amplified products were sequenced using an Illumina MiSeq system (Qin et al., 2019).
Bioinformatics and Sequencing Data Analysis
Both the forward and reverse ends of the read were trimmed at the base of which a Q value being less than 20. If the pair of reads overlapped by a minimum of 50 bp, they were merged into a complete read. Reads smaller than 399 bp and those with more than one expected error were discarded from the analysis (Edgar, 2010). The quality-filtered reads were dereplicated into unique sequences, sorted in decreasing abundance, and singletons were discarded. Representative non-chimeric operational taxonomic unit (OTU) sequences were subsequently obtained using the default UPARSE settings (Edgar, 2013), and additional reference-based chimera detection was performed using UCHIME (Edgar et al., 2011). The OTU table was generated after mapping the quality controlled reads to the non-chimeric OTUs using Usearch (Edgar, 2010) global alignment algorithm at a 97% cutoff in conjunction with filtering to exclude non-bacterial data.
Analysis of the bacterial community structure was performed using QIIME (Caporaso et al., 2010). The number of high-quality reads was greater than 10,000 for all samples. Therefore, the sequences of each samples were randomly re-extracted with 10,000 reads each time with 1,000 permutations for rarefaction and normalization to equalize the differences in sequencing depth using QIIME (Caporaso et al., 2010). The purpose of these multiple rarefactions was to ensure that the data features were preserved verifiably; the multiple permutations can reduce errors caused by randomness. The alpha diversity of each sample was calculated by determining the observed OTUs, the Shannon index, the Simpson index and the phylogenetic diversity (PD) whole-tree index according to the normalized data. Representative sequences for each OTU were built into a phylogenetic tree using FastTree and subjected to the Ribosomal Database Project (RDP) classifier to determine the phylogeny using a bootstrap cutoff of 80% (RDP database version 2.10). The phylogenetic tree and the relative abundance table of the representative OTU sequences were subsequently subjected to principal coordinate analysis (PCoA) (Lozupone and Knight, 2005). The data were graphed using GraphPad Prism (version 4.0 for Windows, GraphPad Software, San Diego, CA, United States) and MATLAB 2014a (The MathWorks INC., MA, United States). The statistical significance of the community structural similarity between different treatments was assessed by multivariate analysis of variance (MANOVA) using MATLAB 2014a (The MathWorks Inc., Natick, MA, United States). The statistical significance of the differences in microbial communities among the samples from different treatments was assessed by MANOVA.
Prediction of Bacterial Community Function
The functional composition of the soil metagenome was predicted using Phylogenetic Investigation of Communities by Reconstruction of Unobserved States (PICRUSt) (Langille et al., 2013). The 16S rRNA gene data were referenced according to Greengenes 13.5, and the resulting data was used for predictive analysis by PICRUSt via the Kyoto Encyclopedia of Genes and Genomes (KEGG). The identified KEGG orthology (KO) pathways were subsequently sorted into functional categories based on the KEGG pathway subsystem hierarchy level 3 (according to KEGG module). The differences in the functional composition of the metagenomes in response to different soil management practices were compared using PICRUSt metagenome inferences, and the beta diversity analysis was conducted based on the Bray-Curtis distance. The differences in bacterial community biological function distributions were determined based on the predicted data categorized by function via the KOs. The statistical significance of the functional similarity between the samples from different treatments was assessed by MANOVA.
Quantitation of Nitrogen Cycling Functional Genes After Reciprocal Inoculation
Quantitative real-time PCR was performed with a LightCycler 96 instrument (Roche, Switzerland) using SYBR Green as a fluorescent dye to determine the relative abundances of functional genes involved in nitrogen cycling, including nitrate reductase (narG) (Bru et al., 2007), nitrite reductase (nirK and nirS) (Henry et al., 2004; Throbäck et al., 2004), nitrous oxide reductase (nosZ) (Henry et al., 2006) and ammonia monooxygenase (amoA) genes (Yang et al., 2017) (Supplementary Table S2). One-way ANOVA was performed to test the variations in gene copy numbers (log transformed) among the different treatments.
Determination of Soil Denitrification Function
Sodium nitrite (added concentration of nitrite-nitrogen (NO2--N): 50 mg/kg soil delivered weight) and glucose (added concentration of DOC: 1 g/kg soil delivered weight) were added to the experimental vials containing 20 g of soil (the remaining soil in each vial was collected for soil index and microbiota determinations). Sodium nitrite and glucose were dissolved in 1 mL of sterile water and added to each vial. The headspace gas in all the vials was replaced with helium using a pump ventilation system (Shanghai, China). The vials were subsequently placed in a robotized incubation system for incubation and gas monitoring (Molstad et al., 2007) for a duration of 5 days. The kinetics of nitric oxide (NO), nitrous oxide (N2O), and dinitrogen (N2) formation in the vials was monitored accordingly (Molstad et al., 2007). Student’s t-tests were performed to evaluate the differences in the N2-N concentration after 100 h of incubation between the uninoculated and inoculated samples.
Accession Numbers of the Sequence Data
The 16S rRNA gene sequences obtained in this study were submitted to the GenBank Sequence Read Archive (SRA) database of the National Center for Biotechnology Information (NCBI) under the accession numbers SRP153935 and SRP162142.
Results
Soil Properties Before and After Incubation
The ONS and ACS soils had significantly different properties in terms of the basic characteristic indices. Compared with the ACS soil, more DNA was extracted from the ONS soil and it had a higher nitrate concentration; however, the ACS soil had a much greater concentration of heavy metal and aromatic pollutants (Supplementary Table S3). After the reciprocal inoculation and incubation, a comparison of the soil parameters, including the pH and the carbon and nitrogen contents, revealed that inoculation with other soils did not significantly influence the recipient soils (Table 2 and Supplementary Table S4).
Shift in Bacterial Community Structure During Incubation
The bacterial community in each sample was analyzed via high-throughput sequencing of the V3–V4 region of the 16S rRNA gene. A total of 1,287,005 usable high-quality sequences (673,788 unique sequences) were selected for subsequent analyses after being checked for quality, and 4,566 OTUs were obtained (median = 31,585 sequences, ranging from 11,074 to 58,294 sequences). The ONS soil had a higher alpha diversity index than did the ACS soil. The bacterial richness showed no obvious increase with the exotic soil inoculation (Table 3 and Supplementary Table S5). The bacterial richness of the ONS soil inoculated with ACS soil (AintoO) was more stable than that of ACS soil inoculated with ONS soil (OintoA); the alpha diversity of the former treatment was similar to that of the ONS soil. However, the observed number of OTUs in the OintoA treatments was lower than the observed number in the ACS soil, and the number of OTUs observed in the AintoO treatments was lower than the number observed in the ONS soil (Table 3). The number of OTUs in the AintoO treatments was more stable than the number of OTUs in the OintoA treatments after incubation (Supplementary Table S5).
Distinct taxonomic compositions were observed in the initial soil samples used in this study. At the phylum level (Figure 1A), the ACS soil had greater abundances of Proteobacteria and Actinobacteria, while the ONS soil had greater abundances of Acidobacteria and Bacteroidetes. After incubation for two months, the ONS soil inoculated with ACS presented increased amounts of Proteobacteria but a reduction in Bacteroidetes. In contrast, the ACS soil inoculated with ONS presented increased amounts of Firmicutes and Bacteroides. At the genus level (Figure 1B), the ACS soil had high abundances of Pseudomonas, Arthrobacter, Nocardioides, Micromonospora, and Halomonas, whereas the ONS soil had high abundances of Gp6, Gp16, Gemmatimonas, Gp4, and Geminicoccus. Other than the high abundance of Gp6, Gp16, Geminicoccus, and Gemmatimonas, three genera, Gaiella, Nocardioides and Arthrobacter, which are common bacteria in agricultural soils (Topp et al., 2000; Aislabie et al., 2005; Eo et al., 2015), were enriched in the AintoO treatment soils. In contrast, the OintoA-treated soils exhibited increases in the abundances of Pseudomonas, Nocardioides, Prevotella, Arthrobacter, Gp6, Gp16, Gaiella, Geminicoccus, and Micromonospora. Moreover, the abundances of five genera, Gp6, Gp16, Gaiella, Geminicoccus, and Micromonospora, which have been reported as indigenous bacteria in aromatic and organic compound-contaminated sites (Mcalpine et al., 2008; Franzetti et al., 2011; Pérez-Leblic et al., 2012), increased in the soils in response to the OintoA treatment. Additionally, while Prevotella and Gaiella OTUs were rare in both ONS and ACS soil, both OTUs were abundant in the OintoA and AintoO treatments, respectively (Figure 1B).
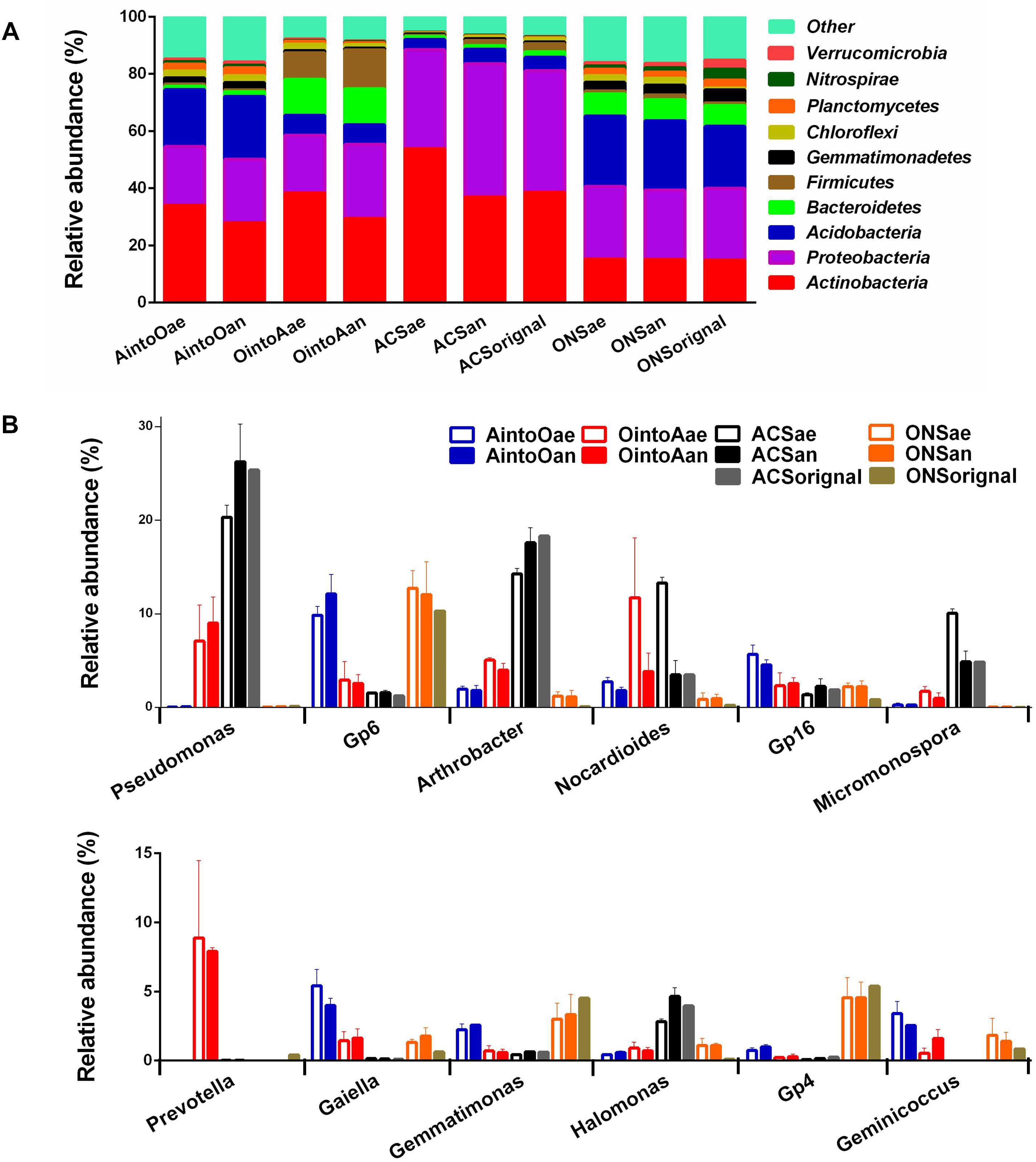
Figure 1. Community compositions of different treatments after incubation. (A) Relative abundances of predominant phyla in the samples of different treatments. (B) Relative abundance of predominant genera in the samples of different treatments.
The PCoA results obtained using the Bray-Curtis distance and both weighted and unweighted UniFrac distances revealed the same pattern (Figures 2A–C). Reciprocal inoculation resulted in a significant alteration in community structure between the uninoculated and inoculated samples after 2 months of incubation. The MANOVA test based on Bray-Curtis distance and both weighted and unweighted UniFrac distances showed that difference in community structure between the inoculated and uninoculated treatments was significant (P < 0.001).
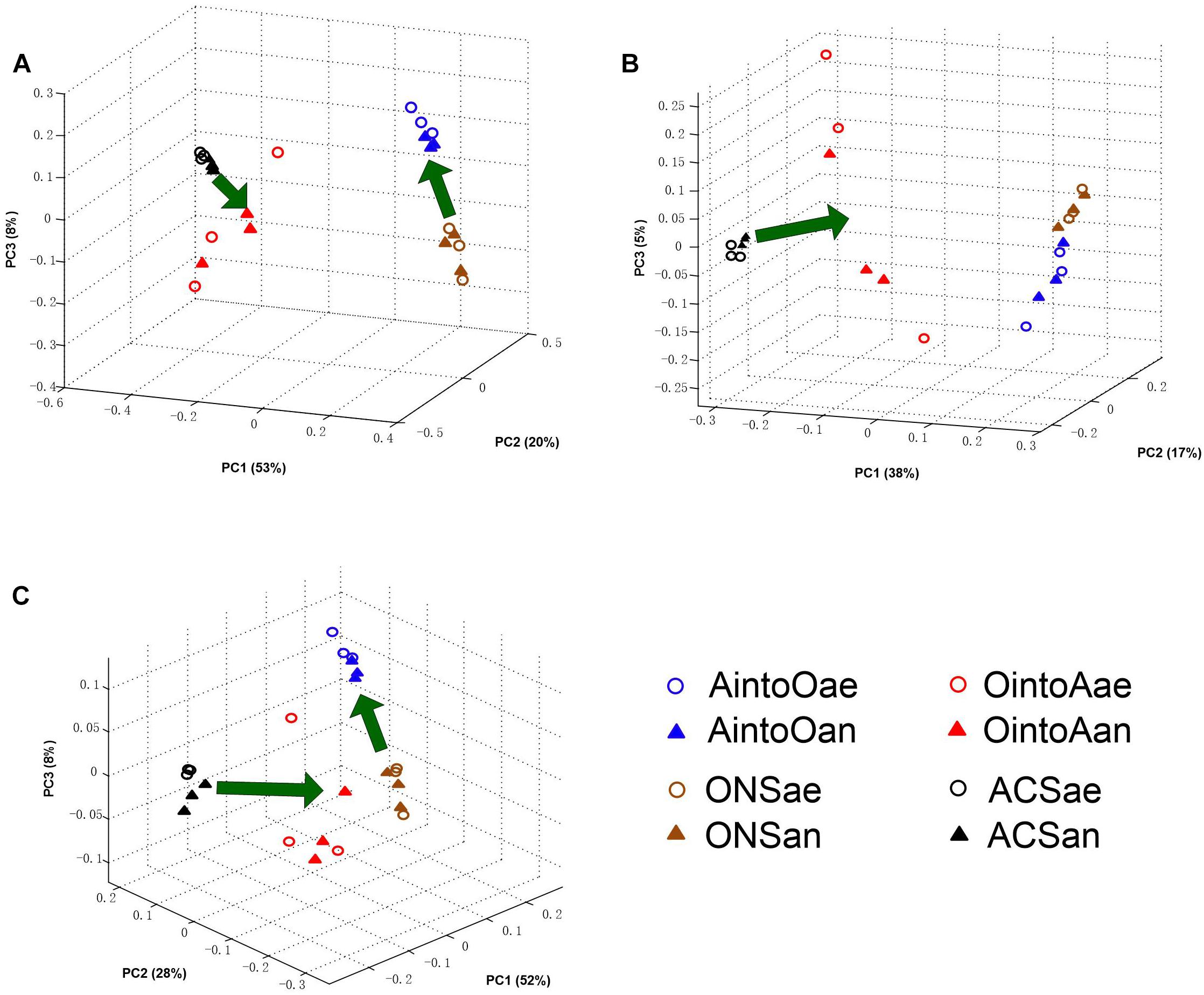
Figure 2. Community coalescence of the soil bacterial communities after reciprocal inoculation. (A) Bray-Curtis PCoA of the soil bacterial community structure based on the OTU data. (B) Unweighted UniFrac PCoA of the soil bacterial community structure based on the OTU data. The percentage of the variation explained by the plotted PCs is shown in parentheses. (C) Weighted UniFrac PCoA of the soil bacterial community structure based on the OTU data. The percentage of the variation explained by the plotted PCs is shown in parentheses. The percentage of the variation explained by the plotted principal coordinates (PCs) is shown in parentheses.
Response of Bacterial Functional Genes to the Reciprocal Inoculations
To evaluate the bacterial functions in the soil samples before and after incubation, we predicted the functional gene content based on the 16S rRNA gene sequencing data using PICRUSt. The PCoA plots based on the PICRUSt data showed that the functional composition of the AintoO samples was most similar to that of the ONS soil samples and that the functional composition of the OintoA samples was most similar to that of the ACS soil samples (Figure 3A). The principal functions of the samples involved components such as transporters, ABC transporters, DNA repair and recombination proteins, two-component system proteins and processes such as purine metabolism (Figure 3B). The ONS samples had an increased abundance of DNA repair and recombination proteins; ribosomes; peptidases; bacterial motility proteins; and proteins involved in purine metabolism, oxidative phosphorylation and pyrimidine metabolism. In contrast, the ACS samples had an increased abundance of transporters, ATP-binding cassette (ABC) transporters and transcription factors (Figure 3B). However, the relative abundances of principal functions did not shift obviously in the inoculated samples. These results demonstrate that although the inoculation slightly altered the abundances of bacterial functional genes, the bacterial functional metagenomes remained stable.
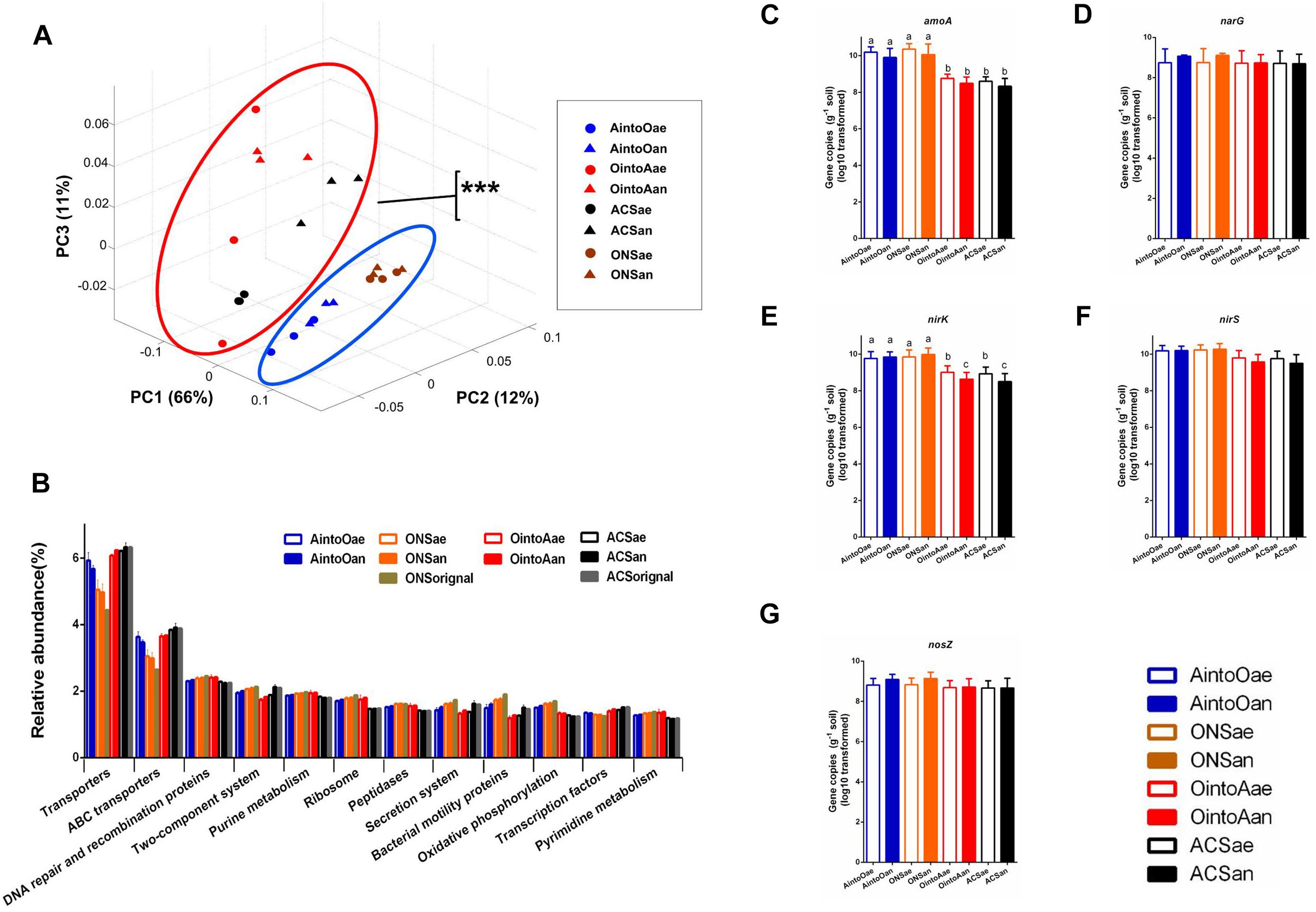
Figure 3. Predicted bacterial community biological function in soil treatments based on PICRUSt, according to both 16S rRNA gene sequencing data and the copy number of genes involved in nitrogen cycling within a gram of soil after long-term incubation. (A) PCoA of soil bacterial biological functions based on the Bray-Curtis distance according to KEGG module predictions in conjunction with the 16S rRNA gene sequencing data. The percentage of the variation explained by the plotted PCs is shown in parentheses. MANOVA analysis indicated significant difference between the circled clusters (∗∗∗P < 0.001). (B) Relative abundance of principal functions by PICRUSt predictions via a KEGG subsystem analysis of samples. All of the data are shown as the means ± standard deviations. The columns and error bars represent the means and standard deviations, respectively. (C–G) Copy number of genes involved in nitrogen cycling in the samples. (C) amoA gene, (D) narG gene, (E) nirK gene, (F) nirS gene, and (G) nosZ gene. One-way ANOVA was used to analyze variations among all the treatments. Different small letters indicate significant differences at the P < 0.05 level, and no significant differences were observed among the soil incubation samples for the narG, nirS, and nosZ genes.
Using quantitative real-time PCR, we also analyzed the abundances of genes involved in nitrogen cycling in soil. The quantification results for the amoA, narG, nirK, nirS, and nosZ genes after 2 months of incubation showed that sample inoculation with a different community did not affect the copy numbers of these genes for either soil recipient (Figures 3C–G and Supplementary Table S6). However, the gene copy numbers for amoA and nirK genes were significantly different between the two soil types; the ONS soil samples had more copies of the amoA and nirK genes than did the ACS soil samples.
Response of Denitrification to the Reciprocal Inoculations
The dynamics of the denitrification metabolism of the soil samples were measured by monitoring emitted gases using the robotized incubation system. The dynamics analysis of the emitted gaseous nitrogen showed that the denitrification activity in the reciprocally inoculated soil samples was unaltered compared with the uninoculated soils (Figure 4 and Supplementary Figure S1). Student’s t-tests indicated that there were no significant differences in the N2-N concentration after 100 h of monitoring between samples of AintoOae and ONSae (P = 0.9193), AintoOan and ONSan (P = 0.3413), OintoAae and ACSae (P = 0.7459), or OintoAan and ACSan (P = 0.0607). The results also showed that gas production trends were similar between the AintoO and ONS soil samples and the OintoA and ACS soils. Thus, the denitrification metabolism remained stable following the reciprocal inoculations.
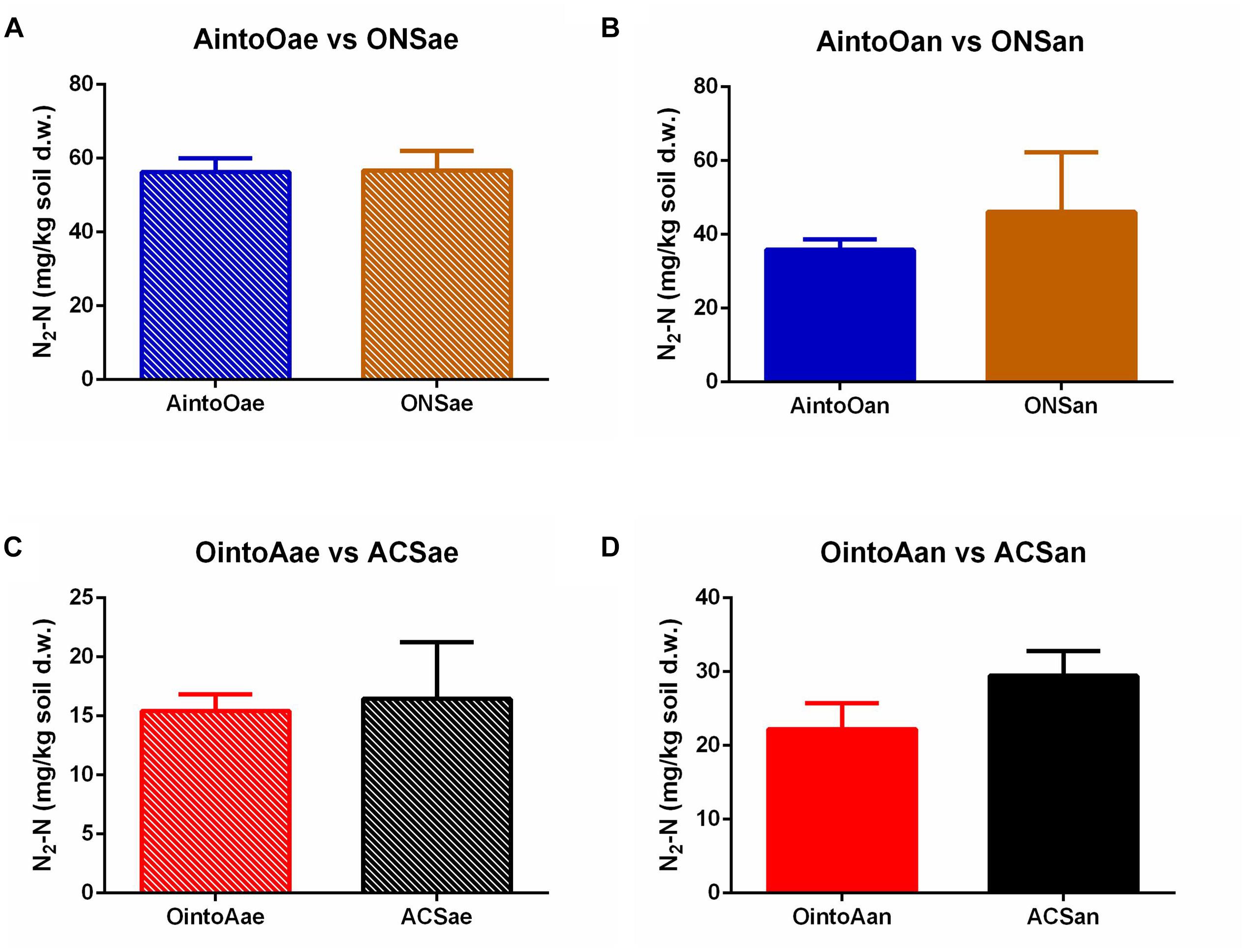
Figure 4. Dinitrogen content in different treatments after 100 h of kinetics monitoring. (A) AintoOae and ONSae. (B) AintoOan and ONSan. (C) OintoAae and ACSae. (D) OintoAan and ACSan. The bars indicate the means, and the error bars indicate the SD. No significant differences in the N2-N levels were observed between the inoculated and uninoculated soils.
Discussion
Microbial community coalescence can result from the intermixing of diverse communities into a new environment or from one microbial community being mixed with another. Regarding the mixing ratios, coalescence processes can be differentiated by equal vs. unequal community mixing (Rillig et al., 2015). In our study, one microbiota was added to another at an unequal ratio to simulate the application of exotic soil into host soil. Two distinct recipient soils initially harbored different microbiota, and—as expected—the soil bacterial community structures shifted significantly after being inoculated with exotic bacteria. The MANOVA analysis showed that the introduced communities significantly influenced the host bacterial communities. Previous studies have shown that the biological invasion of exotic microbes resulting from soil management, i.e., fertilization and irrigation, influences the biodiversity and ecological function of soil recipients (Zhao et al., 2005; Watts et al., 2010; Zhang et al., 2012; Xiong et al., 2017). Recent studies have also demonstrated that species immigrating into a habitat may play an important role in shaping the habitat’s microbial community structure (Nemergut et al., 2013). This result is consistent with our findings: that inoculating communities instead of species can also reshape community structures, which is similar to organisms invading soils. In our study, some bacteria were enriched during the coalescence process. Specifically, some OTUs that were rare in both the ONS and ACS soils became abundant in the coalescent communities.
Our results indicated that bacterial richness was more stable in the agricultural soil than in the industrial soil (Supplementary Table S5). This result is probably due to the lower nutrient content and consequent stronger competition in the deep soil from the industrial site compared to the agricultural soil. It is well known that only species adapted to a specific habitat can survive; they would otherwise become extinct (Bazzaz, 1991; Woodward and Diament, 1991; Kolar and Lodge, 2001). Studies have also revealed the importance of phenotypic, physiological and biochemical traits (such as size, growth rate and resource utilization, respectively) on the performance of invasive species (Matz and Kjelleberg, 2005; Dawson et al., 2012; Mächler and Altermatt, 2012). Exotic bacteria must overcome the stability of the soil bacterial community to survive in a new habitat. In this study, we observed that the enriched exotic bacteria often dominated in soils whose environmental characteristics were the same as those of the recipient soils, including members of the genera Gaiella (Eo et al., 2015), Nocardioides (Topp et al., 2000), Arthrobacter (Aislabie et al., 2005), Gp6 (Pérez-Leblic et al., 2012), Gp16 (Pérez-Leblic et al., 2012), Geminicoccus (Franzetti et al., 2011), and Micromonospora (Mcalpine et al., 2008).
In this study, we also evaluated nitrite reduction to investigate the effect of coalescence when two distinct soils were reciprocally mixed in different ratios. Nitrite reduction, which eliminates the harmful compound nitrite from soil, is crucial to the soil nitrogen cycle (Malone and Stevens, 1998). Most bacterial genera that were predominant in our study samples, such as Bradyrhizobium (Fernandez et al., 2008), Azoarcus (Lee and Wong, 2014), Thiobacillus (Yang et al., 2018), and Nitrosomonas (Schmidt et al., 2004) are typical nitrite-reducing bacteria. The sum of the relative abundances of these bacteria remained stable during the incubation period (Supplementary Table S7). Some inoculated microorganisms may have a similar metabolic capacity for nitrite removal, although the abundances of different individual species varied after inoculation with exotic bacteria and incubation for the evaluated period of time. A robust correlation between community composition and ecological function has not been observed in various studies. The most direct reason for this lack of correlation has been attributed to the existence of functional redundancy (Rosenfeld, 2002). When a bacterial community structure is altered because of a disturbance, functional redundancy is very important for maintaining the function of the community (Baho et al., 2012). Functional redundancy refers to an overlap in the ecological functions of various species (Wohl et al., 2004; Comte and Giorgio, 2010). Many studies have attributed the stability (resistance and resilience) of soil ecosystems to the functional redundancy caused by intrinsic soil biodiversity, i.e., bacterial species decay is not always accompanied by bacterial functional decay (Chaer et al., 2009; Berga et al., 2012). Stable biological functions in conjunction with dynamic bacterial community structure have been reported in both studies of bioreactors (Xia et al., 2014; Wu et al., 2016; Qin et al., 2019) and of the human microbiome (Human Microbiome Project Consortium [HMPC], 2012; Lozupone et al., 2012; Bletz et al., 2016). For example, fecal and oral communities analyzed according to the 16S rRNA gene showed divergent community structure, whereas the results of shotgun metagenomics sequencing of the same samples showed obviously similar functional profiles (Human Microbiome Project Consortium [HMPC], 2012; Lozupone et al., 2012). In our study, we observed a reduction in the abundances of specific bacteria due to coalescence after mixing the host community with an exotic community, especially in the industrial soil. The abundances of some phylogenetically different bacteria increased to replace the indigenous bacteria. However, the incursion of exotic microbiota barely influenced the soil metabolism. Interestingly, these enriched bacteria were predicted to be functionally redundant with the replaced bacteria.
Conclusion
In this study, the soil bacterial community structure shifted significantly in response to unequal mixing of two soils. The introduction of exotic microbiota impacted the soil bacterial community structure. Compared with the community structure observed in the industrial soil, the bacterial richness in the agricultural soil was less influenced by inoculation with the other soil, which may be due to a discrepancy in the nutritional conditions. Some bacteria taxa that were rare in both original soils became abundant after mixing and incubation, indicating that the new soil communities had coalesced. The soil microbiota is considered to be a self-organizing system that—due to functional redundancy—maintains the relative stability of biological function and adapts to disturbances caused by the incursion of exotic microbial communities.
Author Contributions
XZ and XiaoW contributed conception and designed the study. XiaoW, JL, MJ, and QW performed the experiments and generated the data. XiaoW analyzed the data with assistance from XinW, YM, and WS. XiaoW wrote the first draft of the manuscript. XiaoW and XZ led the writing of the manuscript, with input from LZ.
Funding
This work was supported by the National Key Research and Development Program of China (2017YFD0200102), and the National Natural Science Foundation of China (NSFC 31670105, 31861133018, and Key Project 41230856).
Conflict of Interest Statement
The authors declare that the research was conducted in the absence of any commercial or financial relationships that could be construed as a potential conflict of interest.
Acknowledgments
The authors would like to express their gratitude to Prof. Xiaotang Ju for his help in collecting the ONS soil sample and Dr. Changzheng Cui for his help for collecting the ACS soil sample.
Supplementary Material
The Supplementary Material for this article can be found online at: https://www.frontiersin.org/articles/10.3389/fmicb.2019.01125/full#supplementary-material
References
Aislabie, J., Bej, A. K., Ryburn, J., Lloyd, N., and Wilkins, A. (2005). Characterization of Arthrobacter nicotinovorans HIM, an atrazine-degrading bacterium, from agricultural soil New Zealand. FEMS Microbiol. Ecol. 52, 279–286. doi: 10.1016/j.femsec.2004.11.012
Baho, D. L., Peter, H., and Tranvik, L. J. (2012). Resistance and resilience of microbial communities - temporal and spatial insurance against perturbations. Environ. Microbiol. 14, 2283–2292. doi: 10.1111/j.1462-2920.2012.02754.x
Berga, M., Székely, A. J., and Langenheder, S. (2012). Effects of disturbance intensity and frequency on bacterial community composition and function. PLoS One 7:e36959. doi: 10.1371/journal.pone.0036959
Bletz, M. C., Goedbloed, D. J., Sanchez, E., Reinhardt, T., Tebbe, C. C., Bhuju, S., et al. (2016). Amphibian gut microbiota shifts differentially in community structure but converges on habitat-specific predicted functions. Nat. Commun. 7:13699. doi: 10.1038/ncomms13699
Bru, D., Sarr, A., and Philippot, L. (2007). Relative abundances of proteobacterial membrane-bound and periplasmic nitrate reductases in selected environments. Appl. Environ. Microbiol. 73, 5971–5974. doi: 10.1128/AEM.00643-07
Caporaso, J. G., Kuczynski, J., Stombaugh, J., Bittinger, K., Bushman, F. D., Costello, E. K., et al. (2010). QIIME allows analysis of high-throughput community sequencing data. Nat. Methods 7, 335–336. doi: 10.1038/nmeth.f.303
Chaer, G., Fernandes, M., Myrold, D., and Bottomley, P. (2009). Comparative resistance and resilience of soil microbial communities and enzyme activities in adjacent native forest and agricultural soils. Microb. Ecol. 58, 414–424. doi: 10.1007/s00248-009-9508-x
Comte, J., and Giorgio, P. A. D. (2010). Linking the patterns of change in composition and function in bacterioplankton successions along environmental gradients. Ecology 91, 1466–1476. doi: 10.1890/09-0848.1
Dawson, W., Rohr, R. P., Van, K. M., and Fischer, M. (2012). Alien plant species with a wider global distribution are better able to capitalize on increased resource availability. New Phytol. 194, 859–867. doi: 10.1111/j.1469-8137.2012.04104.x
Edgar, R. C. (2010). Search and clustering orders of magnitude faster than BLAST. Bioinformatics 26, 2460–2461. doi: 10.1093/bioinformatics/btq461
Edgar, R. C. (2013). UPARSE: highly accurate OTU sequences from microbial amplicon reads. Nat. Methods 10, 996–998. doi: 10.1038/nmeth.2604
Edgar, R. C., Haas, B. J., Clemente, J. C., Quince, C., and Knight, R. (2011). UCHIME improves sensitivity and speed of chimera detection. Bioinformatics 27, 2194–2200. doi: 10.1093/bioinformatics/btr381
Eo, J., Park, K. C., and Kim, M. H. (2015). Plant-specific effects of sunn hemp (Crotalaria juncea) and sudex (Sorghum bicolor × Sorghum bicolor var. sudanense) on the abundance and composition of soil microbial community. Agric. Ecosyst. Environ. 213, 86–93. doi: 10.1016/j.agee.2015.07.025
Fernandez, L. A., Perotti, E. B., Sagardoy, M. A., and Gomez, M. A. (2008). Denitrification activity of Bradyrhizobium sp isolated from Argentine soybean cultivated soils. World J. Microbiol. Biotechnol. 24, 2577–2585. doi: 10.1007/s11274-008-9828-x
Fierer, N., Leff, J. W., Adams, B. J., Nielsen, U. N., Bates, S. T., Lauber, C. L., et al. (2012). Cross-biome metagenomic analyses of soil microbial communities and their functional attributes. Proc. Natl. Acad. Sci. U.S.A. 109, 21390–21395. doi: 10.1073/pnas.1215210110
Franzetti, A., Gandolfi, I., Bertolini, V., Raimondi, C., Piscitello, M., Papacchini, M., et al. (2011). Phylogenetic characterization of bioemulsifier-producing bacteria. Int. Biodeterior. Biodegradation 65, 1095–1099. doi: 10.1016/j.ibiod.2011.01.014
Griffiths, R. I., Whiteley, A. S., O’Donnell, A. G., and Bailey, M. J. (2000). Rapid method for coextraction of DNA and RNA from natural environments for analysis of ribosomal DNA- and rRNA-based microbial community composition. Appl. Environ. Microbiol. 66, 5488–5491. doi: 10.1128/Aem.66.12.5488-5491.2000
Hausmann, N. T., and Hawkes, C. V. (2009). Plant neighborhood control of arbuscular mycorrhizal community composition. New Phytol. 183, 1188–1200. doi: 10.1111/j.1469-8137.2009.02882.x
Henry, S., Baudoin, E., López-Gutiérrez, J. C., Martin-Laurent, F., Brauman, A., and Philippot, L. (2004). Quantification of denitrifying bacteria in soils by nirK gene targeted real-time PCR. J. Microbiol. Methods 59, 327–335. doi: 10.1016/j.mimet.2004.07.002
Henry, S., Bru, D., Stres, B., Hallet, S., and Philippot, L. (2006). Quantitative detection of the nosZ gene, encoding nitrous oxide reductase, and comparison of the abundances of 16S rRNA, narG, nirK, and nosZ genes in soils. Appl. Environ. Microbiol. 72, 5181–5189. doi: 10.1128/AEM.00231-06
Huang, T., Gao, B., Christie, P., and Ju, X. (2013). Net global warming potential and greenhouse gas intensity in a double-cropping cereal rotation as affected by nitrogen and straw management. Biogeosciences 10, 7897–7911. doi: 10.5194/bg-10-7897-2013
Human Microbiome Project Consortium [HMPC] (2012). Structure, function and diversity of the healthy human microbiome. Nature 486, 207–214. doi: 10.1038/nature11234
Kolar, C. S., and Lodge, D. M. (2001). Progress in invasion biology: predicting invaders. Trends Ecol. Evol. 16, 199–204. doi: 10.1016/S0169-5347(01)02101-2
Langille, M. G., Zaneveld, J., Caporaso, J. G., McDonald, D., Knights, D., Reyes, J. A., et al. (2013). Predictive functional profiling of microbial communities using 16S rRNA marker gene sequences. Nat. Biotechnol. 31, 814–821. doi: 10.1038/nbt.2676
Lee, D. J., and Wong, B. T. (2014). Denitrifying sulfide removal and nitrososulfide complex: azoarcus sp. NSC3 and Pseudomonas sp. CRS1 mix. Bioresour. Technol. 166, 616–619. doi: 10.1016/j.biortech.2014.05.099
Li, C., Wang, W. M., Liu, T., Zhang, Q., Wang, G. X., Li, F. D., et al. (2018). Effect of early weaning on the intestinal microbiota and expression of genes related to barrier function in lambs. Front. Microbiol. 9:1431. doi: 10.3389/fmicb.2018.01431
Lozupone, C., and Knight, R. (2005). UniFrac: a new phylogenetic method for comparing microbial communities. Appl. Environ. Microbiol. 71, 8228–8235. doi: 10.1128/aem.71.12.8228-8235.2005
Lozupone, C. A., Stombaugh, J. I., Gordon, J. I., Jansson, J. K., and Knight, R. (2012). Diversity, stability and resilience of the human gut microbiota. Nature 489, 220–230. doi: 10.1038/nature11550
Mächler, E., and Altermatt, F. (2012). Interaction of species traits and environmental disturbance predicts invasion success of aquatic microorganisms. PLoS One 7:e45400. doi: 10.1371/journal.pone.0045400
Malone, J. P., and Stevens, R. J. (1998). Removal of nitrite impurity from nitrate labeled with nitrogen-15. Soil Sci. Soc. Am. J. 62, 651–653. doi: 10.2136/sssaj1998.03615995006200030015x
Mansour, I., Heppell, C. M., Ryo, M., and Rillig, M. C. (2018). Application of the microbial community coalescence concept to riverine networks. Biol. Rev. 93, 1832–1845. doi: 10.1111/brv.12422
Matz, C., and Kjelleberg, S. (2005). Off the hook–how bacteria survive protozoan grazing. Trends Microbiol. 13, 302–307. doi: 10.1016/j.tim.2005.05.009
Mcalpine, J. B., Banskota, A. H., Charan, R. D., Schlingmann, G., Zazopoulos, E., Piraee, M., et al. (2008). Biosynthesis of diazepinomicin/ECO-4601, a micromonospora secondary metabolite with a novel ring system. J. Nat. Prod. 71, 1585–1590. doi: 10.1021/np800376n
McNeill, M., Phillips, C., Young, S., Shah, F., Aalders, L., Bell, N., et al. (2011). Transportation of nonindigenous species via soil on international aircraft passengers’ footwear. Biol. Invasions 13, 2799–2815.
Molstad, L., Dörsch, P., and Bakken, L. R. (2007). Robotized incubation system for monitoring gases (O2, NO, N2O N2) in denitrifying cultures. J. Microbiol. Methods 71, 202–211. doi: 10.1016/j.mimet.2007.08.011
Mummey, D. L., Rillig, M. C., and Holben, W. E. (2005). Neighboring plant influences on arbuscular mycorrhizal fungal community composition as assessed by T-RFLP analysis. Plant Soil 271, 83–90. doi: 10.1007/s11104-004-2066-6
Nemergut, D. R., Schmidt, S. K., Fukami, T., O’Neill, S. P., Bilinski, T. M., Stanish, L. F., et al. (2013). Patterns and processes of microbial community assembly. Microbiol. Mol. Biol. Rev. 77, 342–356. doi: 10.1128/MMBR.00051-12
Osono, T. (2005). Colonization and succession of fungi during decomposition of Swida controversa leaf litter. Mycologia 97, 589–597. doi: 10.3852/mycologia.97.3.589
Panke-Buisse, K., Poole, A. C., Goodrich, J. K., Ley, R. E., and Kao-Kniffin, J. (2015). Selection on soil microbiomes reveals reproducible impacts on plant function. ISME J. 9, 980–989. doi: 10.1038/ismej.2014.196
Paulin, M. M., Nicolaisen, M. H., Jacobsen, C. S., Gimsing, A. L., Sorensen, J., and Baelum, J. (2013). Improving Griffith’s protocol for co-extraction of microbial DNA and RNA in adsorptive soils. Soil Biol. Biochem. 63, 37–49. doi: 10.1016/j.soilbio.2013.02.007
Pérez-Leblic, M. I., Turmero, A., Hernández, M., Hernández, A. J., Pastor, J., Ball, A. S., et al. (2012). Influence of xenobiotic contaminants on landfill soil microbial activity and diversity. J. Environ. Manag. 95, S285–S290. doi: 10.1016/j.jenvman.2010.07.017
Qin, X., Wu, X., Li, L., Li, C., Zhang, Z., and Zhang, X. (2018). The advanced anaerobic expanded granular sludge bed (AnaEG) possessed temporally and spatially stable treatment performance and microbial community in treating starch processing wastewater. Front. Microbiol. 9:589. doi: 10.3389/fmicb.2018.00589
Qin, X. C., Ji, M. M., Wu, X. G., Li, C. J., Gao, Y. S., Li, J., et al. (2019). Response of treatment performance and microbial community structure to the temporary suspension of an industrial anaerobic bioreactor. Sci. Total Environ. 646, 229–237. doi: 10.1016/j.scitotenv.2018.07.309
Rillig, M. C., Antonovics, J., Caruso, T., Lehmann, A., Powell, J. R., Veresoglou, S. D., et al. (2015). Interchange of entire communities: microbial community coalescence. Trends Ecol. Evol. 30, 470–476. doi: 10.1016/j.tree.2015.06.004
Rillig, M. C., Lehmann, A., Aguilar-Trigueros, C. A., Antonovics, J., Caruso, T., Hempel, S., et al. (2016a). Soil microbes and community coalescence. Pedobiologia 59, 37–40. doi: 10.1016/j.pedobi.2016.01.001
Rillig, M. C., Tsang, A.,and Roy, J. (2016b). Microbial community coalescence for microbiome engineering. Front. Microbiol. 7:1967. doi: 10.3389/Fmicb.2016.01967
Rillig, M. C., and Mansour, I. (2017). Microbial ecology: community coalescence stirs things up. Curr. Biol. 27, R1280–R1282. doi: 10.1016/j.cub.2017.10.027
Rosenfeld, J. S. (2002). Functional redundancy in ecology and conservation. Oikos 98, 156–162. doi: 10.1034/j.1600-0706.2002.980116.x
Schmidt, I., van Spanning, R. J., and Jetten, M. S. (2004). Denitrification and ammonia oxidation by Nitrosomonas europaea wild-type, and NirK- and NorB-deficient mutants. Microbiology 150, 4107–4114. doi: 10.1099/mic.0.27382-0
Swenson, W., Wilson, D. S., and Elias, R. (2000). Artificial ecosystem selection. Proc. Natl. Acad. Sci. U.S.A. 97, 9110–9114. doi: 10.1073/pnas.150237597
Throbäck, I. N., Enwall, K., Jarvis,Å., and Hallin, S. (2004). Reassessing PCR primers targeting nirS, nirK and nosZ genes for community surveys of denitrifying bacteria with DGGE. FEMS Microbiol. Ecol. 49, 401–417. doi: 10.1016/j.femsec.2004.04.011
Tikhonov, M. (2016). Community-level cohesion without cooperation. eLife 5:e15747. doi: 10.7554/eLife.15747
Topp, E., Mulbry, W. M., Zhu, H., Nour, S. M., and Cuppels, D. (2000). Characterization of S-triazine herbicide metabolism by a Nocardioides sp. Isolated from agricultural soils. Appl. Environ. Microbiol. 66, 3134–3141. doi: 10.1128/AEM.66.8.3134-3141.2000
Watts, D. B., Torbert, H. A., Feng, Y. C., and Prior, S. A. (2010). Soil microbial community dynamics as influenced by composted dairy manure, soil properties, and landscape position. Soil Sci. 175, 474–486. doi: 10.1097/SS.0b013e3181f7964f
Wohl, D. L., Arora, S., and Gladstone, J. R. (2004). Functional redundancy supports biodiversity and ecosystem function in a closed and constant environment. Ecology 85, 1534–1540. doi: 10.1890/03-3050
Woodward, F. I., and Diament, A. D. (1991). Functional approaches to predicting the ecological effects of global change. Funct. Ecol. 5, 202–212. doi: 10.2307/2389258
Wu, L., Yang, Y., Si, C., Zhao, M., Zhu, Z., Yang, S., et al. (2016). Long-term successional dynamics of microbial association networks in anaerobic digestion processes. Water Res. 104, 1–10. doi: 10.1016/j.watres.2016.07.072
Xia, Y., Wang, X., Wen, X., Ding, K., Zhou, J., Yang, Y., et al. (2014). Overall functional gene diversity of microbial communities in three full-scale activated sludge bioreactors. Appl. Microbiol. Biotechnol. 98, 7233–7242. doi: 10.1007/s00253-014-5791-7
Xiong, W., Guo, S., Jousset, A., Zhao, Q., Wu, H., Li, R., et al. (2017). Bio-fertilizer application induces soil suppressiveness against Fusarium wilt disease by reshaping the soil microbiome. Soil Biol. Biochem. 114, 238–247. doi: 10.1016/j.soilbio.2017.07.016
Xun, W., Huang, T., Zhao, J., Ran, W., Wang, B., Shen, Q., et al. (2015). Environmental conditions rather than microbial inoculum composition determine the bacterial composition, microbial biomass and enzymatic activity of reconstructed soil microbial communities. Soil Biol. Biochem. 90, 10–18. doi: 10.1016/j.soilbio.2015.07.018
Yang, L., Zhang, X., and Ju, X. (2017). Linkage between N2O emission and functional gene abundance in an intensively managed calcareous fluvo-aquic soil. Sci. Rep. 7:43283. doi: 10.1038/srep43283
Yang, Y., Gerrity, S., Collins, G., Chen, T. H., Li, R. H., Xie, S. H., et al. (2018). Enrichment and characterization of autotrophic thiobacillus denitrifiers from anaerobic sludge for nitrate removal. Process Biochem. 68, 165–170. doi: 10.1016/j.procbio.2018.02.017
Zhang, X., Nesme, J., Simonet, P., and Frostegård, Å. (2012). Fate of invading bacteria in soil and survival of transformants after simulated uptake of transgenes, as evaluated by a model system based on lindane degradation. Res. Microbiol. 163, 200–210. doi: 10.1016/j.resmic.2012.01.007
Zhao, Y., Zhou, Z., Wang, L., and Zhao, L. (2005). Dynamics of microbial community structure and cellulolytic activity in agricultural soil amended with two biofertilizers. Eur. J. Soil Biol. 41, 21–29. doi: 10.1016/j.ejsobi.2005.03.002
Keywords: soil bacterial community, reciprocal inoculation, biological function, community coalescence, functional redundancy
Citation: Wu X, Li J, Ji M, Wu Q, Wu X, Ma Y, Sui W, Zhao L and Zhang X (2019) Non-synchronous Structural and Functional Dynamics During the Coalescence of Two Distinct Soil Bacterial Communities. Front. Microbiol. 10:1125. doi: 10.3389/fmicb.2019.01125
Received: 08 November 2018; Accepted: 03 May 2019;
Published: 29 May 2019.
Edited by:
Aymé Spor, INRA UMR1347 Agroécologie, FranceReviewed by:
Stavros D. Veresoglou, Freie Universität Berlin, GermanySana Romdhane, Institut National de la Recherche Agronomique (INRA), France
Copyright © 2019 Wu, Li, Ji, Wu, Wu, Ma, Sui, Zhao and Zhang. This is an open-access article distributed under the terms of the Creative Commons Attribution License (CC BY). The use, distribution or reproduction in other forums is permitted, provided the original author(s) and the copyright owner(s) are credited and that the original publication in this journal is cited, in accordance with accepted academic practice. No use, distribution or reproduction is permitted which does not comply with these terms.
*Correspondence: Xiaojun Zhang, eGp6aGFuZzY4QHNqdHUuZWR1LmNu