- 1Tumor Etiology and Screening Department of Cancer Institute and General Surgery, The First Hospital of China Medical University, Shenyang, China
- 2Key Laboratory of Cancer Etiology and Prevention in Liaoning Education Department, The First Hospital of China Medical University, Shenyang, China
- 3Key Laboratory of GI Cancer Etiology and Prevention in Liaoning Province, The First Hospital of China Medical University, Shenyang, China
The link between microbiota and gastric cancer (GC) has attracted widespread attention. However, the phylogenetic profiles of niche-specific microbiota in the tumor microenvironment is still unclear. Here, mucosa-associated microorganisms from 62 pairs of matched GC tissues and adjacent non-cancerous tissues were characterized by 16S rRNA gene sequencing. Functional profiles of the microbiota were predicted using PICRUSt, and a co-occurrence network was constructed to analyze interactions among gastric microbiota. Results demonstrated that mucosa-associated microbiota from cancerous and non-cancerous tissues established micro-ecological systems that differed in composition, structure, interaction networks, and functions. Microbial richness and diversity were increased in cancerous tissues, with the co-occurrence network exhibiting greater complexity compared with that in non-cancerous tissue. The bacterial taxa enriched in the cancer samples were predominantly represented by oral bacteria (such as Peptostreptococcus, Streptococcus, and Fusobacterium), while lactic acid-producing bacteria (such as Lactococcus lactis and Lactobacillus brevis) were more abundant in adjacent non-tumor tissues. Colonization by Helicobacter pylori, which is a GC risk factor, also impacted the structure of the microbiota. Enhanced bacterial purine metabolism, carbohydrate metabolism and denitrification functions were predicted in the cancer associated microbial communities, which was consistent with the increased energy metabolism and concentration of nitrogen-containing compounds in the tumor microenvironment. Furthermore, the microbial co-occurrence networks in cancerous and non-cancerous tissues of GC patients were described for the first time. And differential taxa and functions between the two groups were identified. Changes in the abundance of certain bacterial taxa, especially oral microbiota, may play a role in the maintenance of the local microenvironment, which is associated with the development or progression of GC.
Introduction
Gastric cancer (GC) is one of the most common malignant cancers and the third leading cause of cancer-associated death worldwide (Ferlay et al., 2015; Torre et al., 2015). The incidence of GC varies by region and race, with a high rate in East Asia. Both host factors (such as genetic susceptibility) and environmental factors (such as microbial infections) play crucial roles in gastric tumorigenesis (Compare et al., 2010). It is widely accepted that chronic Helicobacter pylori infection, which leads to enhanced inflammation and disorders of epithelial structure and function, is closely related to precancerous lesions such as atrophic gastritis. Nevertheless, only 1–3% of H. pylori-infected patients develop GC, and GC could not be completely prevented by the eradication of H. pylori (Wroblewski et al., 2010). Additionally, during the progression of GC, H. pylori colonization gradually decreases and even disappears (El-Omar et al., 1997). Therefore, biological factors other than H. pylori colonization may play an important role in the development of cancer and the maintenance of the local lesion microenvironment.
In recent years, the development of culture-independent technologies for characterizing microbiota composition has shed light on the profile of gastric microbiota. Studies have demonstrated the significant role played by non-H. pylori microbiota in gastric carcinogenesis in mice (Lofgren et al., 2011; Lertpiriyapong et al., 2014). In human studies, chronic H. pylori infection or the use of drugs such as omeprazole resulted in an elevated intragastric pH level by reducing the secretion of gastric acid, which allowed the proliferation and colonization of other bacteria (Mowat et al., 2000; Plottel and Blaser, 2011). As a result, the microbial balance of the gastric mucosa ecological niche was disrupted, and increased nitrosating species raised nitrite and N-nitrosamine levels in the stomach (Leach et al., 1987). Together, these findings highlighted the potential role of microbiota other than H. pylori in the development of GC.
Thus far, our understanding of the complex gastric flora in human is still limited. A few studies have revealed differences in the composition and function of the gastric microbiota between GC patients and control groups. However, there is no consensus on specific microbial taxa that play important roles in the pathogenesis of GC. In addition, microbial changes in the tumor microenvironment remain unclear. Unlike most previous studies that compared two groups of individuals, our research focused on the microbiota in the tumor microenvironment by comparing matched samples from GC patients. In this condition, the influence of the external environment and the genetic effects of the host would be controlled to some extent. We characterized the variations in the composition, interaction network and functions of gastric microbiota in cancerous and patient-matched non-cancerous tissues, aiming to explore the differential distribution profile of microbiota in the tumor microenvironment. Our findings will help to explore the role of mucosa-associated microbiota in carcinogenesis and in the maintenance of the local microenvironment in GC patients.
Materials and Methods
Study Population and Specimen Collection
A total of 124 gastric tissue samples, consisting of cancerous and paired non-cancerous tissues, were obtained from 62 GC patients who underwent subtotal gastrectomy at The First Hospital of China Medical University between 2012 and 2014. Patients who had received medical treatment (including probiotics, proton pump inhibitors, antibiotics, and H2 receptor antagonists) within 1 month, or those who had received chemotherapy or radiotherapy prior to the surgery were excluded. Gastric mucosa tissues, collected from the cancerous lesions and neighboring noncancerous sites (at least 5 cm away from the tumor site), were immediately frozen after surgical resections and stored at -80°C until further use. Epidemiologic information was obtained through questionnaire.
DNA Extraction, PCR Amplification, and 16S rRNA Gene Sequencing
Total DNA was extracted using methods as previously described (Chen et al., 2017). After treating the mucosal samples with lysozyme, proteinase K, and SDS, we purified the DNA through multiple steps with phenol-chloroform-isoamylalcohol, then precipitated the DNA with glycogen, sodium acetate, and cold isopropanol, followed by cleaning the DNA with 70% ethanol. Finally, the DNA was dissolved in TE buffer and stored at -20°C. The V4–V5 regions of the 16S rRNA gene were amplified by primers 515F, 5′-barcode-GTGCCAGCMGCCGCGGTAA-3′ and 907R, 5′-barcode CCGTCAATTCMTTTRAGTTT-3′ (Liu et al., 2018), using the PCR kit (TransGenAP221-02, Peking; containing the high fidelity enzyme). PCR was performed as follows: 95°C for 5 min, followed by 34 cycles of 94°C for 60 s, 57°C for 45 s, and 72°C for 60 s, with final extension at 72°C for 10 min. In order to avoid possible contamination, DNA extraction and PCR set up were performed in a laminar air flow bench, illuminated with a UV lamp before use. Two negative controls (containing DNA extraction reagents and PCR kit reagents) were amplified and sequenced to assess contamination. The concentration and length of the PCR amplicons were detected by 2% agarose gel electrophoresis. PCR products with bright main strip (approximately 400–450 bp) were chosen for further experiments. The amplicons in the target region were purified with Qiagen Gel Extraction Kit (Qiagen, Germany). Sequencing libraries were generated by using DNA PCR-Free Sample Preparation Kit (Illumina, San Diego, CA, United States) following manufacturers recommendations and index codes were added. The library quality was assessed on the Qubit@2.0 Fluorometer (Thermo Scientific) and Agilent Bioanalyzer 2100 system. The libraries were sequenced on the Illumina Hiseq 2500 platform and 250 bp paired-end reads were generated. The sequence data have been deposited in the NCBI Sequence Read Archive (SRA) database with the accession number PRJNA532731.
Processing of Sequencing Results and Taxonomical Annotation
The sequencing data were processed using the Quantitative Insights Into Microbial Ecology (QIIME, V1.9.1) pipeline as previously reported (Caporaso et al., 2010). Raw sequencing reads were assigned to each sample based on the unique barcode and identified as valid sequences. The low quality and short sequences were filtered out with the following criteria (Gill et al., 2006; Fadrosh et al., 2014): sequence reads with average Phred score ≤19, length less than 150 bp; paired reads having at least one with length less than 75% of their original length; reads with ambiguous bases; reads containing mononucleotide repeats more than 8 bp. Paired-end reads were assembled using FLASH (version 1.2.7) (Magoč and Salzberg, 2011). Chimeras were filtered out using UCHIME (v4.2.40) (Edgar et al., 2011). Sequence clustering analysis was performed using UPARSE pipeline (Edgar, 2013). Tags with at least 97% identity were clustered into the same operational classification unit (OTU; Supplementary Table S1). The Silva Database was used to annotate taxonomic information for OTU representative sequences by the ribosome database project (RDP) Classifier v.2.2.
Microbial Diversity Analysis and Network Construction
QIIME (V1.9.1) was used to calculate diversity parameters. Alpha diversity analysis was performed to describe the richness and diversity of the microbiota in each sample. The Chao1 and ACE indices were used to estimate community richness, and the Shannon and phylogenetic diversity (PD) whole tree indices were applied to measure microbial diversity. Good’s coverage was used to evaluate the coverage quality of sequencing results. Beta diversity was measured by weighted UniFrac distance matrices and Bray–Curtis, and visualized via principal coordinate analysis (PCoA) and non-metric multidimensional scaling (NMDS) plots. Co-occurrence networks were structured by Spearman’s correlation analysis and visualized using the Cytoscape software (V.3.0.2., United States).
Functional Prediction of Mucosal-Associated Microbiota
Functions of mucosal-associated microbiota were predicted using PICRUSt (Langille et al., 2013). Accuracy of the predicted metagenomes was evaluated by the nearest sequenced taxon index (NSTI; Langille et al., 2013). The enrichment analysis of pathways was performed based on Kyoto Encyclopedia of Genes and Genomes (KEGG) database. Additionally, predicted functional genes were also categorized into clusters of orthologous groups (COG), and compared across cancer and non-cancer groups by STAMP (Parks et al., 2014) to identify gene functions that differentiated bacterial communities in the two-group comparison.
Statistical Analysis
Continuous variables that were not normally distributed were represented by inter-quartile range (IQR). Mann–Whitney U test was used to examine the correlation between alpha diversity parameters and epidemiological risk factors of GC. Tests were performed with SPSS 22.0 software (SPSS Inc., Chicago, IL, United States). Analysis of similarity (ANOSIM) and permutational multivariate analysis of variance (PERMANOVA) were performed to test the dissimilarity of beta diversity between groups by using ANOSIM and Adonis functions of vegan package in R (version 3.4.1). Linear discriminant analysis effect size (LEfSe) algorithm (Segata et al., 2011) was used to identify specific microbial taxa and functions that differed significantly between groups. Differences with linear discriminant analysis (LDA) scores >2.0 were considered significant. The analysis of differences in the abundance of the microbiota between two groups were also performed using the DESeq. 2 package in R (Weiss et al., 2017). The White’s non-parametric t-test was applied to determine statistical differences of COG between groups by STAMP. P-values were adjusted by Benjamini-Hochberg false discovery rate correction for multiple comparisons. P < 0.05 was considered statistically significant.
Results
Sequencing Results and Basic Characteristics of the Study Subjects
After PCR amplification, no bands were observed in the negative controls on the gel. The negative controls both had <130 reads, and the sequences could not be assembled. After sequencing and quality control, libraries of 16S rRNA gene V4–V5 region amplicon sequences from 61 cancerous and 62 adjacent non-cancerous tissue samples were used for further analysis, with an average of 67,958 effective tags per sample. The number of raw reads and effective tags for each sample are shown in Supplementary Table S2. At the 3% dissimilarity level, the number of OTUs were 152 (119-200) [median (IQR)] for the non-cancer group and 221 (177-350) for the cancer group. Good’s coverage was estimated to ensure quality assessment. All samples had a value >0.99, suggesting that the sequencing results were sufficient to represent the bacterial diversity of the bacteria in the gastric mucosa.
Detailed information regarding individuals included in the study is provided in Table 1. All cases were diagnosed as gastric adenocarcinoma. The median age of the patients was 60 years old. Samples with relative abundance of H. pylori more than 1% were identified as H. pylori sequencing positive, while others with relative abundance less than 1% were identified as H. pylori sequencing negative as previously proposed (Kim et al., 2015). Among the patients, H. pylori sequencing-positive cases accounted for 29%.
Characteristics of Mucosa-Associated Microbiota in Cancerous Tissues
Microbial Community Profile of Cancerous Tissues
Proteobacteria was the predominant phylum in the cancerous samples, followed by Firmicutes, Bacteroidetes, Actinobacteria, Acidobacteria, and Fusobacteria (Table 2). Overall, 90% of cancerous samples were dominated by Proteobacteria, with relative abundance >50% in each case. Four samples were dominated by Firmicutes or Bacteroidetes, while the remaining two samples had no obviously dominant phylum (Supplementary Figure S1).
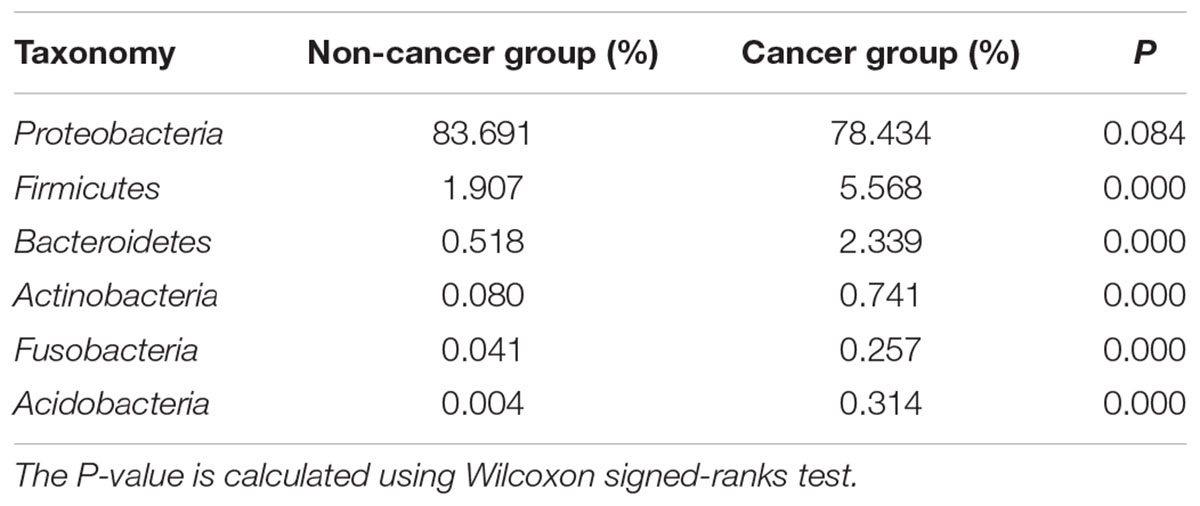
Table 2. The relative abundances of major bacterial phyla in cancerous and adjacent non-cancerous tissues.
Correlation Between Cancerous Tissue Microbiota and GC Risk Factors
We next analyzed the association between GC-related epidemiological risk factors (such as age, gender, smoking, alcohol consumption, family history, and H. pylori colonization status) and the microbiota. Results showed that in tumor tissues, the alpha diversity of the microbiota, estimated by Shannon index and PD whole tree, was significantly increased in GC patients aged over 60 years old compared with that of younger patients (P = 0.043, 0.022, respectively, Supplementary Table S3). No significant differences in the microbial richness or community structure were discovered in relation to the other risk factors.
Ecological Network of Gastric Microbiota in Cancerous Tissues
Co-occurrence network analysis was used to describe the interactions among the microbiota in the complex gastric microbial population. As shown in Figure 1A, the interactions across the mucosa-associated microbiota mainly occurred among taxa belonging to the phyla Firmicutes and Proteobacteria, the two predominant phyla in the taxonomic profiles. Collectively, co-occurrence interactions dominated in the networks. In addition, co-occurrence interactions between Helicobacter and Lachnoclostridium and between Helicobacter and Ezakiella were also observed in the cancer tissue network. No co-exclusion interaction was identified in strong correlations (r > 0.6 or <-0.6).
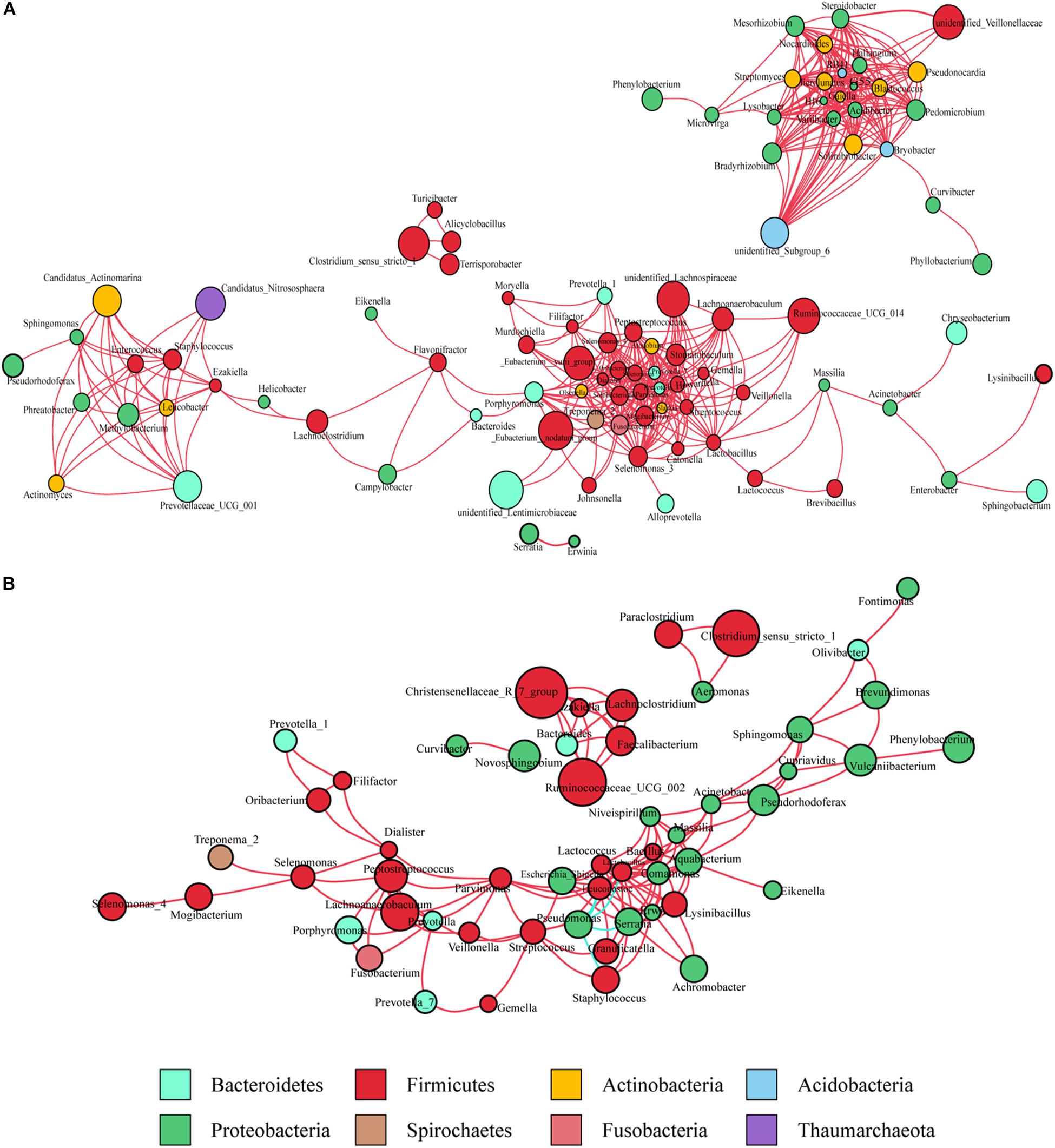
Figure 1. Co-occurrence network analysis of gastric bacterial genera with correlation coefficient >0.6 or < –0.6 (A) in cancer tissues and (B) non-cancer tissues. The nodes represent different genera, whose colors indicates different phyla. The size of node shows relative abundance of the genus. Positive and negative correlations are drawn in red and blue, respectively.
Characteristics of Mucosa-Associated Microbiota in Non-cancerous Tissues
Microbial Community Profile of Non-cancerous Tissues
The gastric microbiota in non-cancerous tissues was also dominated by Proteobacteria and consisted mainly of the six main phyla observed in the cancer group. However, the ranking of phyla differed slightly (Table 2), with a decreased abundance of Firmicutes, Bacteroidetes, Actinobacteria, Acidobacteria, Fusobacteria (all P < 0.01), but an increased abundance of Proteobacteria (P = 0.084) when compared with the cancer group.
Correlation Between Non-cancerous Tissue Microbiota and GC Risk Factors
Unlike the cancer group, H. pylori colonization significantly impacted the composition of the microbiota in the non-cancerous samples. The H. pylori sequencing-positive group showed greater bacterial diversity (Shannon index) than the sequencing-negative group (P = 0.022, Supplementary Table S4). The differences in microbial structure were assessed by Bray–Curtis and weighted UniFrac distance matrices. PERMANOVA showed significant differences between H. pylori sequencing positive and negative group (Bray–Curtis, P = 0.001; weighted UniFrac distance matrices, P = 0.004). No significant association was found between microbial richness or community structure and the other risk factors.
LEfSe analysis (Segata et al., 2011) was conducted to further explore the taxa correlated with H. pylori colonization status in non-tumor tissues (Supplementary Figure S2). In the H. pylori sequencing-positive group, the enrichment of 15 genera was observed other than Helicobacter. Among them, Serratia, Lactobacillus, and Streptococcus were abundant in all non-cancerous tissues, ranking in the top 20 abundant genera. In comparison, Pseudomonas aeruginosa and its higher level taxa from genus to phylum were significantly more abundant in the H. pylori negative group. In summary, H. pylori colonization status, which is a well-known epidemiological risk factor for GC, was closely associated with the composition and structure of the gastric microbiota.
Ecological Network of Gastric Microbiota in Non-cancerous Tissues
As observed in the cancerous tissue samples, the interactions within the microbiota of the non-cancerous samples also occurred mainly in the two taxonomically dominant phyla, Firmicutes and Proteobacteria; however, fewer phyla were involved. The network diagram revealed denser and more complicated co-occurrence interactions across the microbiota in the cancerous tissues compared with the non-cancerous tissues, especially with regard to oral bacteria (such as Streptococcus, Peptostreptococcus, Fusobacterium, Dialister, and Prevotella) (Figures 1A,B). However, several co-exclusion interactions were observed in the non-cancerous tissue network, with Pseudomonas as the interaction node. These interactions occurred among Pseudomonas and Serratia, Lactobacillus, Lactococcus, Staphylococcus, as well as Leuconostoc.
Differential Microbial Taxa of the GC Tissues Compared With the Non-cancerous Tissues
Bacterial Taxonomic Richness and Diversity
The cancerous tissues had a significantly higher number of OTUs than the non-cancer tissues (219 versus 148 OTUs; P < 0.05). In terms of alpha diversity (Figures 2A–D), compared with non-cancerous tissues, cancer samples had significantly increased community richness, which was estimated by Chao1 and ACE index (both P < 0.001), and diversity, which was estimated by Shannon index and PD whole tree (both P < 0.001).
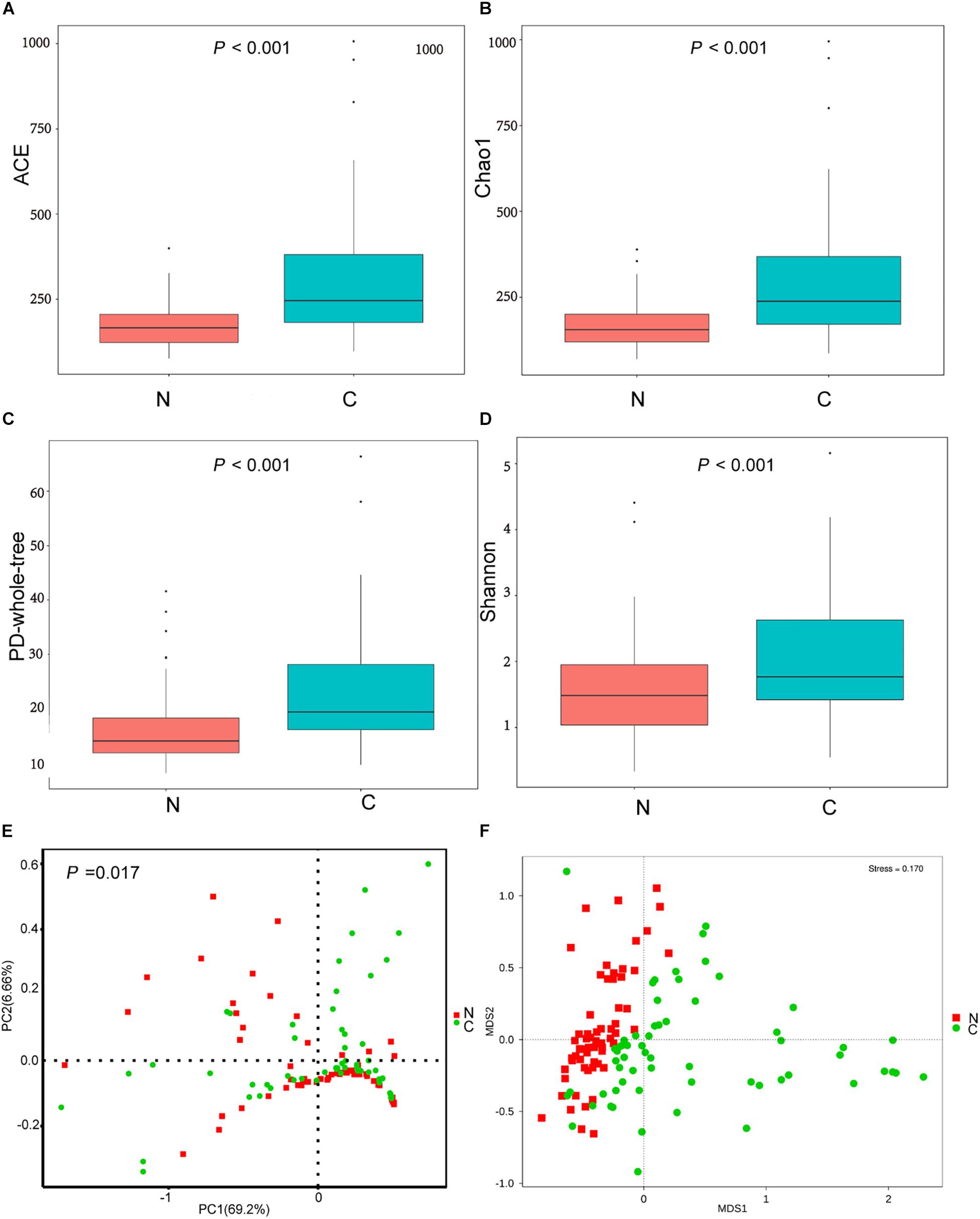
Figure 2. (A–D) The alpha diversity of the microbial communities in cancer and non-cancer groups. The global microbial structure differs between the two groups in (E) PCoA plot and (F) NMDS plot. C, cancer group; N, non-cancer group.
Bacterial Community Structure
To analyze differences in microbial community structure between groups, we assessed the beta diversity (Figures 2E,F). The overall differences were visualized using PCoA and NMDS plots. The diversity described in the PCoA plots by the top two principal coordinates was 75.86% based on weighted UniFrac phylogenetic distance matrices. The non-cancerous and cancerous samples were clustered separately, with a significant difference confirmed by ANOSIM (P = 0.017, Figure 2E). The results of NMDS analysis based on OTU level also divided samples into two separate clusters (Figure 2F), suggesting significant differences in the overall community structure of mucosal microorganisms between the cancer and non-cancer groups.
Specific Microbial Taxa Associated With GC in the Cancer Microenvironment
We sought to identify the differential microbiota between the two sample groups, using two different methods. First, the LEfSe analysis (Segata et al., 2011) was conducted to identify the specific taxa responsible for the statistically significant differences (Figure 3). Overall, 49 taxa were identified as being differentially abundant between the cancer and non-cancer samples at the phylum, class, order, family, genus, and species levels (LDA = 3). 33 of them were enriched in the cancer group, including the genera Streptococcus, Peptostreptococcus, Prevotella, Prevotella_7, Acinetobacter, Bacillus, Selenomonas, Lachnoanaerobaculum, and Sphingomonas and the species Acinetobacter baumannii, P. aeruginosa, Prevotella oris, and Prevotella denticola, most of which were oral microbiota. 16 taxa were enriched in the non-cancer group, including the genera Serratia, Helicobacter, Niveispirillum, and Lactococcus, and the species H. pylori, Serratia marcescens, Lactococcus lactis, and Lactobacillus brevis.
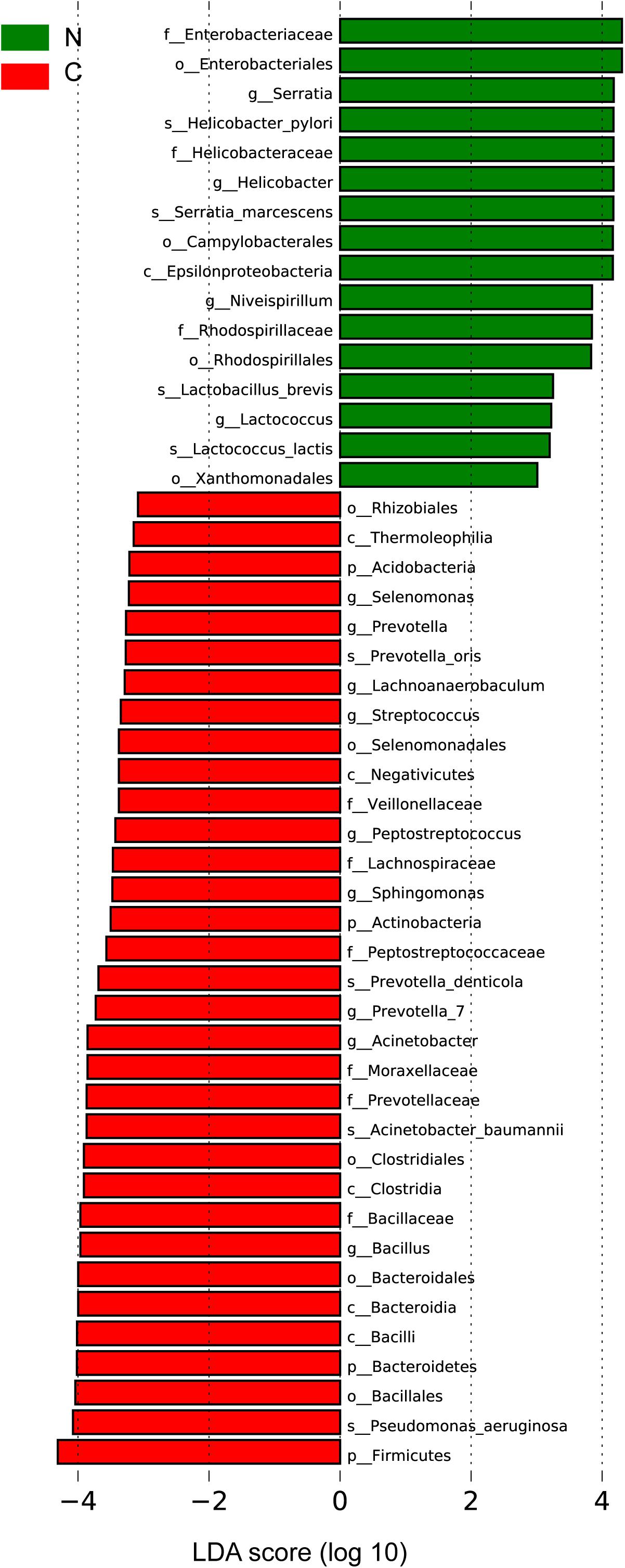
Figure 3. Differential bacteria between the two groups by LEfSe analysis (LDA scores >3.0). Green indicates taxa enriched in non-cancerous tissues and red indicates taxa enriched in cancerous tissues. C, cancer group; N, non-cancer group.
Then, we used the DESeq. 2 package to calculate and compare the top 20 abundant genera with a median relative abundance >0.1% between the two groups. In addition to the eight genera (Peptostreptococcus, Streptococcus, Acinetobacter, Bacillus, Bacteroides, Sphingomonas, Prevotella_1, and Prevotella_7) described above as being enriched in the cancer group, Fusobacterium was also shown to be significantly more abundant in cancerous tissues. On the other hand, Helicobacter and Lactobacillus showed significant increase in the adjacent non-cancerous tissues (Supplementary Table S5).
To explore the interactions among these differential bacteria, the network within each group was constructed (Figures 4A,B). We found that co-occurrence and co-excluding interactions were significantly different between the two groups. Oral microbiota including Prevotella, Prevotella_7, Peptostreptococcus, Streptococcus, and Fusobacterium had higher weighted node connectivity (WNC) scores in cancerous tissues (Figure 4A), while Serratia, Lactococcus, and L. brevis, which were identified above as being enriched in non-cancer group, had higher WNC scores in non-cancer tissues (Figure 4B). Higher WNC scores indicated centralities and their important roles in the interaction network.
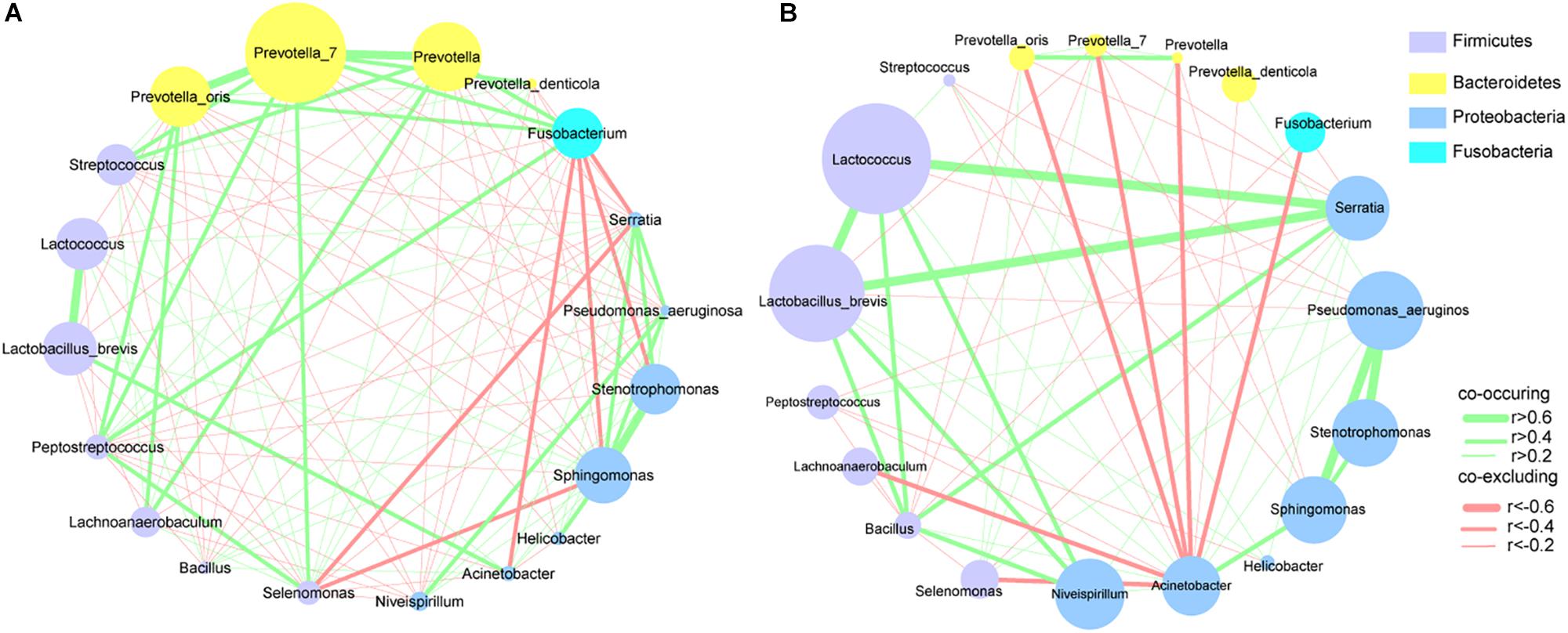
Figure 4. Correlation networks of differential bacteria (A) in cancer and (B) non-cancer tissues. A subset of significant correlations with strengths of at least 0.2 were selected for visualization. The size of the nodes correspond to weighted node connectivity (WNC) scores.
Functional Prediction of Mucosal-Associated Microbiota
Based on 16S rRNA gene sequencing data, PICRUSt was performed to predict the functional profiling of microbial communities using the KEGG databases (Langille et al., 2013). The NSTI scores (0.02–0.13) (Langille et al., 2013) demonstrated a reasonable prediction accuracy (Supplementary Table S6). The LEfSe algorithm was used to detect differences in the functional pathways of the microbiota between the cancer and non-cancer groups. Several metabolic pathways were enriched in the cancerous samples, including those involved in nucleotide metabolism (pyrimidine and purine metabolism), energy metabolism (methane metabolism), carbohydrate metabolism (e.g., glycolysis and gluconeogenesis), etc. (Supplementary Figure S3), while other predicted pathways were significantly increased in the non-cancerous samples, such as bacterial motility (motility proteins and chemotaxis), membrane transport (e.g., secretion system and phosphotransferase system), lipopolysaccharide biosynthesis and signal transduction (two component system), etc. (Supplementary Figure S3).
Given that the accumulation of nitrogen-containing compounds such as nitrate and nitrite in the stomach can increase the risk of GC and promote the malignant transformation of cells in the stomach (Correa, 1992; Alarcon et al., 2017), we focused on the microbial functions relevant to the metabolism of nitrogen-containing compounds in the cancerous and non-cancerous tissues. Compared with the non-cancer group, metabolic enzymes related to denitrification, including nitrate reductase (COG1116) and nitrous oxide reductase (COG4263), were enriched in the gastric microbiota of the cancer group (Supplementary Table S7).
Discussion
Microbial communities have been universally considered as an important biological factor in the occurrence and development of GC. Recent studies have shown the changes in the microbial populations of GC patients compared with control groups (Eun et al., 2014; Coker et al., 2018; Ferreira et al., 2018; Hsieh et al., 2018). However, microbial profiles in the tumor microenvironment were still unclear. Our findings confirmed that gastric mucosa-associated microbiota from cancerous and adjacent non-cancerous tissues established distinct micro-ecological systems. We observed significant microbial community disturbances in the cancer lesions, where the richness and diversity of the microbial communities increased significantly, the interaction network exhibited greater complexity, and the relative abundance of H. pylori decreased compared with non-cancerous tissues. The bacterial taxa enriched in the cancer group mostly consisted of oral bacteria (such as Peptostreptococcus, Streptococcus, and Fusobacterium), while lactic acid-producing bacteria (such as L. lactis, and L. brevis) were more abundant in the adjacent non-tumor tissues. These results suggest that the occurrence and development of GC disturbs the structure of the endogenous bacterial community, and that H. pylori might play a limited role in the development and/or progression of malignant tumors.
Characteristics of Mucosal-Associated Microbiota in GC and Non-cancerous Tissues
Changes in localized niches in GC patients result from long-term interactions among microorganisms, the host, and the environment. Shifts in gastric acidity and nutrient availability, as well as the innate immune response all contribute to the disruption of the microbial ecological balance in GC patients, leading to the colonization and overgrowth of non-H. pylori bacteria (Brawner et al., 2014). Gastric microbiota in cancerous and adjacent non-cancerous tissues were both dominated by Proteobacteria at the phylum level. The significant differences between the two groups mainly occurred at the genus and species level. Oral bacteria such as Fusobacterium, Streptococcus, Peptostreptococcus, and Prevotella were enriched in cancerous tissues. On the other hand, Serratia and lactic acid producing bacteria such as Lactococcus and Lactobacillus were more abundant in non-cancer group. Importantly, we identified some differential taxa that have not been reported in gastric microbiota studies before, such as P. denticola, P. aeruginosa, and Serratia. Although Streptococcus, Peptostreptococcus, Fusobacterium, and Lactobacillus have been discussed in recent studies of GC (Castano-Rodriguez et al., 2017; Hsieh et al., 2018), our research is the first to identify their abundance differences in the tumor microenvironment.
Our results highlight the possible pathogenic role of oral microbiota in GC. The changed acidity environment of GC may provide increased opportunities for oral bacteria to colonize the gastrointestinal tract. Previous studies have shown that oral bacteria were associated with colorectal cancer (CRC; Nakatsu et al., 2015) and pancreatic cancer (Michaud, 2013), which attracted widespread attention. Patients with certain oral pathogens had a higher risk of developing pancreatic cancer (Ertz-Archambault et al., 2017). There has been no studies directly analyzing changes in the oral microbiota of GC patients. A more in-depth investigation is needed to characterize its role as a driver or passenger in carcinogenicity. Whether oral microbes could be used as a non-invasive diagnostic marker for GC requires further studies.
It is noteworthy that Fusobacterium was more abundant in GC specimens than non-cancerous tissues. Fusobacterium is a genus of anaerobic bacteria closely related to CRC. Increasing evidence has shown its roles in carcinogenesis, diagnosis, progression and prognosis of CRC (Zhang et al., 2018). A recent report revealed that Fusobacterium species were over-represented in GC patients, and that it could be used as a diagnostic marker for GC. It supported our findings, despite being based on a relatively small cohort (11 GC patients vs. 16 controls) (Hsieh et al., 2018).
Previous reports had shown the significant increase in the abundance of Lactobacillus species in GC compared with the control population (Aviles-Jimenez et al., 2014; Eun et al., 2014; Wang et al., 2016; Castano-Rodriguez et al., 2017). However, changes in lactic acid producing bacteria in the tumor microenvironment were previously unknown. We observed an obvious enrichment of lactic acid producing bacteria (such as L. lactis and L. brevis) in non-cancerous tissues compared with cancerous tissues. Lactococcus and Lactobacillus species are generally thought of as probiotics and considered beneficial to the host. Reports show that lactic acid production has immunomodulative, anti-cancer and anti-inflammatory activities, and is conducive to the eradication therapy of H. pylori (Kim et al., 2014; Han et al., 2015; Kanayama et al., 2018). Another noteworthy taxon enriched in the non-cancer tissues here was S. marcescens, which has not been reported in cancer-related microbiota research before. Prodigiosin, a secondary metabolite of S. marcescens, could induce GC cell apoptosis and inhibit human oral squamous carcinoma cell growth in vitro (Diaz-Ruiz et al., 2001; Cheng et al., 2017).
Correlation Between Microbial Community Characteristics and GC Risk Factors
Results of association analyses between epidemiological risk factors and gastric microbiota revealed an increased Shannon’s diversity in the older patients (≥60 years old). This may be due to the overall decline in immunity associated with age, and the elevated pH caused by local mucosal atrophy, both of which are conducive to bacterial growth (Sheh and Fox, 2013). We also showed that sex, smoking, drinking, and family history of upper gastrointestinal cancer were not significantly associated with microbial community characteristics. In addition, H. pylori colonization changed the microbial community structure, with a significant increase in alpha diversity, which was observed in H. pylori sequencing-positive group compared with negative group. This finding is supported by a previous report showing that the abundance of H. pylori can remarkably affect the diversity of the gastric microbiota (Wang et al., 2016). How H. pylori impacts on the diversity and structure of the gastric microbiota is not yet understood. Nevertheless, it is plausible that changes in the gastric niche induced by H. pylori may influence the colonization and growth of other microbes.
Ecological Networks of Microbial Taxa in Cancer and Adjacent Mucosal Tissues
The microbiota inhabiting the mucosal surface affects the development of cancer by altering the metabolome and regulating cell proliferation and tumor growth (Johnson et al., 2015). A niche-specific microbial network could affect the disease-associated microenvironment. A study has shown stronger interactions among differential OTUs in the microbiota of GC patients compared with those in patients with precancerous lesions (superficial gastritis, atrophic gastritis, intestinal metaplasia) (Coker et al., 2018). We extended previous work by delineating the interaction networks of microbiota in cancerous and adjacent non-cancerous tissues. Our results revealed that the distribution of microbial interactions differed between cancerous and adjacent non-cancerous mucosae. In the strong correlation network (r > 0.6 or r < -0.6), compared with the non-cancer group, more microbial taxa were involved in the cancer group. And they formed a denser and more complex association network, in which only co-occurrence interactions were observed. This may be due to the decrease in abundance of H. pylori, the increased abundance of other microbes in cancerous tissues, and shifts of the local pathogenic microenvironment. The enrichment of a larger number of microbial taxa, particularly oral microbes, in the cancerous samples contributed to the formation of a disease-specific interaction network. Further, the markedly increased symbiotic interactions in the cancer group might also contribute to the maintenance of the tumor microenvironment and even affect further disease development. Interestingly, strong co-occurrence interactions formed by Streptococcus, Peptostreptococcus, Fusobacterium, Dialister, and Prevotella, showed the centralities of these taxa in the whole cancer group network. They also played significant roles in the network constructed of the differentially abundant bacteria. These results suggest that these oral microbiota may have major impact on the structure of the microbiota in GC patients, which deserves further investigations. On the other hand, several co-exclusion interactions presented in the whole non-cancer group network, occurred separately between Serratia, Lactobacillus, Lactococcus with Pseudomonas (as the interaction node). Moreover, Serratia, Lactobacillus, and Lactococcus exhibited their centralities in the network constructed of the differentially abundant bacteria. These findings show their potential protective effects.
Functional Analysis of Mucosal-Associated Microbiota in GC and Non-cancer Tissues
Our work showed differences in the predicted microbiota functions in cancerous and adjacent non-cancerous tissues. Purines are rich in the cancer microenvironment, with the capability of regulating immune cell responses and the release of cytokines (Di Virgilio, 2012). In this study, the purine metabolism pathways were enriched in the cancer group, indicating the metabolism of released purines in tumor microenvironment by GC microbiota (Coker et al., 2018). In addition, the microbiota in cancerous tissues had an increase in denitrification functions compared with non-cancerous tissues. The abundant nitrate reductase (COG1116) is associated with bacterial-mediated N-nitrosylation (Hillman, 2004), while the N-nitroso compound is a causative factor in carcinogenesis. Additionally, several pathways that facilitated host cell recognition were decreased in the microbiota of cancerous tissues, such as bacterial movement (bacterial motor proteins and chemotaxis) and bacterial signal transduction (membrane transport, etc.). To develop a deeper understanding of gastric carcinogenesis, further studies are needed to examine the significance of microbial functional variations in the GC microenvironment.
Advantages and Limitations
In this study, gastric mucosa samples were obtained from GC patients undergoing surgical treatment, thus avoiding possible oral microbial contamination that may occur during upper digestive endoscopy sampling. Our work provided insights into the composition, function and interaction network of the mucosa-associated bacterial community in the tumor microenvironment, and its links with GC risk factors. We identified specific genera and species that may be involved in gastric carcinogenesis and the maintenance of the tumor microenvironment. However, this study had several limitations. Firstly, PICRUSt, which was used for microbial functional assessment, is a predictive method by nature. Although it has been widely applied in studies of disease-associated microorganisms, this approach may not fully reflect the biological functions of the microorganisms. Furthermore, this study did not include gastric tissues from individuals without GC for comparison. However, to a certain extent, this reduced the impact of inter-subject dissimilarity. In addition, our DNA extraction protocol did not include a bead-beating step, which was an extra cell lysis process to destroy the hard-to-break cell membranes of certain species. A previous study had compared DNA extraction methods with and without a bead-beating step. The result demonstrated that the extraction method without a bead-beating step inevitably missed some taxa with hard-to-break cell membranes, however, these taxa were exceedingly rare and would not have a detrimental impact on the final results (Yu et al., 2017). The research revealed the correlation between gastric microbiota and GC, but could not determine the causal relationship. This will require follow-up animal models and cell culture experiments.
Conclusion
Compared with non-cancerous tissues, mucosa-associated microbiota in cancer tissues showed significant differences in distribution profile. The alterations in microbial community composition, function and ecological network in GC tissues may be involved in carcinogenesis and the maintenance of local microenvironment of GC. In future studies, we would focus on verification using a larger number of samples and multicentric populations, and extend our work into cell culture systems and animal models to examine the pathogenic roles of microorganisms in GC. These investigations into the mucosa-associated microbiota of GC patients may contribute to the development of new strategies for prevention, diagnosis, early intervention, and treatment of GC.
Ethics Statement
This study was approved by the Human Ethics Review Committee of The First Hospital of China Medical University (Shenyang, China [2012]115), and written informed consent was obtained from all patients.
Author Contributions
YY contributed conceptualization, funding acquisition, project administration, writing–review, and editing. X-HC contributed data curation, investigation, formal analysis, and writing the original draft. AW contributed methodology and software. A-NC partly contributed to the validation. Y-HG contributed resources and supervision.
Funding
This work was supported by the National Key R&D Program of China, grant no. 2017YFC0907400 to YY, and grant no. 2017YFC0908300 to Y-HG.
Conflict of Interest Statement
The authors declare that the research was conducted in the absence of any commercial or financial relationships that could be construed as a potential conflict of interest.
Supplementary Material
The Supplementary Material for this article can be found online at: https://www.frontiersin.org/articles/10.3389/fmicb.2019.01261/full#supplementary-material
FIGURE S1 | Bacterial profiling plot of relative abundances of operational taxonomic units (OTUs) at the phylum level.
FIGURE S2 | LEfSe analysis in non-cancer tissues with and without H. pylori colonization. Green indicates taxa enriched in H. pylori positive group and red indicates taxa enriched in H. pylori negative group.
FIGURE S3 | Differences in functional compositions between the cancer and non-cancer groups by LEfSe analysis (LDA scores >2.0). Green indicates functions enriched in cancer group and red indicates functions enriched in non-cancer group. C, cancer group; N, non-cancer group.
TABLE S1 | OUT abundance table.
TABLE S2 | Sequencing data.
TABLE S3 | Association between alpha diversity indices of microbiota in cancerous tissues and GC risk factors.
TABLE S4 | Association between alpha diversity indices of microbiota in non-cancerous tissues and GC risk factors.
TABLE S5 | Significantly differential bacteria identified among the top 20 abundant genera of the cancer and non-cancer groups.
TABLE S6 | Nearest sequence taxon index (NSTI) scores for all samples.
TABLE S7 | Comparison for microbial functions associated with nitrogen-containing compound metabolism between cancer and non-cancer groups.
References
Alarcon, T., Llorca, L., and Perez-Perez, G. (2017). Impact of the microbiota and gastric disease development by Helicobacter pylori. Curr. Top. Microbiol. Immunol. 400, 253–275. doi: 10.1007/978-3-319-50520-6_11
Aviles-Jimenez, F., Vazquez-Jimenez, F., Medrano-Guzman, R., Mantilla, A., and Torres, J. (2014). Stomach microbiota composition varies between patients with non-atrophic gastritis and patients with intestinal type of gastric cancer. Sci. Rep. 4:4202. doi: 10.1038/srep04202
Brawner, K. M., Morrow, C. D., and Smith, P. D. (2014). Gastric microbiome and gastric cancer. Cancer J. 20, 211–216. doi: 10.1097/PPO.0000000000000043
Caporaso, J. G., Kuczynski, J., Stombaugh, J., Bittinger, K., Bushman, F. D., Costello, E. K., et al. (2010). QIIME allows analysis of high-throughput community sequencing data. Nat. Methods 7, 335–336. doi: 10.1038/nmeth.f.303
Castano-Rodriguez, N., Goh, K. L., Fock, K. M., Mitchell, H. M., and Kaakoush, N. O. (2017). Dysbiosis of the microbiome in gastric carcinogenesis. Sci. Rep. 7:15957. doi: 10.1038/s41598-017-16289-16282
Chen, C., Song, X., Wei, W., Zhong, H., Dai, J., Lan, Z., et al. (2017). The microbiota continuum along the female reproductive tract and its relation to uterine-related diseases. Nat. Commun. 8:875.
Cheng, M. F., Lin, C. S., Chen, Y. H., Sung, P. J., Lin, S. R., Tong, Y. W., et al. (2017). Inhibitory growth of oral squamous cell carcinoma cancer via bacterial prodigiosin. Mar. Drugs 15:E224. doi: 10.3390/md15070224
Coker, O. O., Dai, Z., Nie, Y., Zhao, G., Cao, L., Nakatsu, G., et al. (2018). Mucosal microbiome dysbiosis in gastric carcinogenesis. Gut 67, 1024–1032. doi: 10.1136/gutjnl-2017-314281
Compare, D., Rocco, A., and Nardone, G. (2010). Risk factors in gastric cancer. Eur. Rev. Med. Pharmacol. Sci. 14, 302–308.
Correa, P. (1992). Human gastric carcinogenesis: a multistep and multifactorial process–first American cancer society award lecture on cancer epidemiology and prevention. Cancer Res. 52, 6735–6740.
Di Virgilio, F. (2012). Purines, purinergic receptors, and cancer. Cancer Res. 72, 5441–5447. doi: 10.1158/0008-5472.can-12-1600
Diaz-Ruiz, C., Montaner, B., and Perez-Tomas, R. (2001). Prodigiosin induces cell death and morphological changes indicative of apoptosis in gastric cancer cell line HGT-1. Histol. Histopathol. 16, 415–421. doi: 10.14670/HH-16.415
Edgar, R. (2013). UPARSE: highly accurate OTU sequences from microbial amplicon reads. Nat. Methods 10, 996–998. doi: 10.1038/nmeth.2604
Edgar, R. C., Haas, B. J., Clemente, J. C., Quince, C., and Knight, R. (2011). UCHIME improves sensitivity and speed of chimera detection. Bioinformatics 27, 2194–2200. doi: 10.1093/bioinformatics/btr381
El-Omar, E. M., Oien, K., El-Nujumi, A., Gillen, D., Wirz, A., Dahill, S., et al. (1997). Helicobacter pylori infection and chronic gastric acid hyposecretion. Gastroenterology 113, 15–24. doi: 10.1016/s0016-5085(97)70075-1
Ertz-Archambault, N., Keim, P., and Von Hoff, D. (2017). Microbiome and pancreatic cancer: a comprehensive topic review of literature. World J. Gastroenterol. 23, 1899–1908. doi: 10.3748/wjg.v23.i10.1899
Eun, C. S., Kim, B. K., Han, D. S., Kim, S. Y., Kim, K. M., Choi, B. Y., et al. (2014). Differences in gastric mucosal microbiota profiling in patients with chronic gastritis, intestinal metaplasia, and gastric cancer using pyrosequencing methods. Helicobacter 19, 407–416. doi: 10.1111/hel.12145
Fadrosh, D. W., Ma, B., Gajer, P., Sengamalay, N., Ott, S., Brotman, R. M., et al. (2014). An improved dual-indexing approach for multiplexed 16S rRNA gene sequencing on the Illumina MiSeq platform. Microbiome 2: 6. doi: 10.1186/2049-2618-2-6
Ferlay, J., Soerjomataram, I., Dikshit, R., Eser, S., Mathers, C., Rebelo, M., et al. (2015). Cancer incidence and mortality worldwide: sources, methods and major patterns in GLOBOCAN 2012. Int. J. Cancer 136, E359–E386. doi: 10.1002/ijc.29210
Ferreira, R. M., Pereira-Marques, J., Pinto-Ribeiro, I., Costa, J. L., Carneiro, F., Machado, J. C., et al. (2018). Gastric microbial community profiling reveals a dysbiotic cancer-associated microbiota. Gut 67, 226–236. doi: 10.1136/gutjnl-2017-314205
Gill, S. R., Pop, M., Deboy, R. T., Eckburg, P. B., Turnbaugh, P. J., Samuel, B. S., et al. (2006). Metagenomic analysis of the human distal gut microbiome. Science 312, 1355–1359. doi: 10.1126/science.1124234
Han, K. J., Lee, N. K., Park, H., and Paik, H. D. (2015). Anticancer and anti-inflammatory activity of probiotic Lactococcus lactis NK34. J. Microbiol. Biotechnol. 25, 1697–1701. doi: 10.4014/jmb.1503.03033
Hillman, B. (2004). Role of Gut Bacteria in Human Toxicology and Pharmacology. Boca Raton, FL: CRC Press.
Hsieh, Y. Y., Tung, S. Y., Pan, H. Y., Yen, C. W., Xu, H. W., Lin, Y. J., et al. (2018). Increased abundance of Clostridium and Fusobacterium in gastric microbiota of patients with gastric cancer in Taiwan. Sci. Rep. 8:158. doi: 10.1038/s41598-017-18596-18590
Johnson, C. H., Dejea, C. M., Edler, D., Hoang, L. T., Santidrian, A. F., Felding, B. H., et al. (2015). Metabolism links bacterial biofilms and colon carcinogenesis. Cell Metab. 21, 891–897. doi: 10.1016/j.cmet.2015.04.011
Kanayama, M., Kato, Y., Tsuji, T., Konoeda, Y., Hashimoto, A., Kanauchi, O., et al. (2018). Enhancement of immunomodulative effect of lactic acid bacteria on plasmacytoid dendritic cells with sucrose palmitate. Sci. Rep. 8:3147. doi: 10.1038/s41598-018-21527-21522
Kim, J., Kim, N., Jo, H. J., Park, J. H., Nam, R. H., Seok, Y. J., et al. (2015). An appropriate cutoff value for determining the colonization of Helicobacter pylori by the pyrosequencing method: comparison with conventional methods. Helicobacter 20, 370–380. doi: 10.1111/hel.12214
Kim, J. E., Kim, M. S., Yoon, Y. S., Chung, M. J., and Yum, D. Y. (2014). Use of selected lactic acid bacteria in the eradication of Helicobacter pylori infection. J. Microbiol. 52, 955–962. doi: 10.1007/s12275-014-4355-y
Langille, M. G., Zaneveld, J., Caporaso, J. G., McDonald, D., Knights, D., Reyes, J. A., et al. (2013). Predictive functional profiling of microbial communities using 16S rRNA marker gene sequences. Nat. Biotechnol. 31, 814–821. doi: 10.1038/nbt.2676
Leach, S. A., Thompson, M., and Hill, M. (1987). Bacterially catalysed N-nitrosation reactions and their relative importance in the human stomach. Carcinogenesis 8, 1907–1912. doi: 10.1093/carcin/8.12.1907
Lertpiriyapong, K., Whary, M. T., Muthupalani, S., Lofgren, J. L., Gamazon, E. R., Feng, Y., et al. (2014). Gastric colonisation with a restricted commensal microbiota replicates the promotion of neoplastic lesions by diverse intestinal microbiota in the Helicobacter pylori INS-GAS mouse model of gastric carcinogenesis. Gut 63, 54–63. doi: 10.1136/gutjnl-2013-305178
Liu, Z., Li, J., Liu, H., Tang, Y., Zhan, Q., Lai, W., et al. (2018). The intestinal microbiota associated with cardiac valve calcification differs from that of coronary artery disease. Atherosclerosis 284, 121–128. doi: 10.1016/j.atherosclerosis.2018.11.038
Lofgren, J. L., Whary, M. T., Ge, Z., Muthupalani, S., Taylor, N. S., Mobley, M., et al. (2011). Lack of commensal flora in Helicobacter pylori-infected INS-GAS mice reduces gastritis and delays intraepithelial neoplasia. Gastroenterology 140, 210–220. doi: 10.1053/j.gastro.2010.09.048
Magoč, T., and Salzberg, S. (2011). FLASH: fast length adjustment of short reads to improve genome assemblies. Bioinformatics 27, 2957–2963. doi: 10.1093/bioinformatics/btr507
Michaud, D. S. (2013). Role of bacterial infections in pancreatic cancer. Carcinogenesis 34, 2193–2197. doi: 10.1093/carcin/bgt249
Mowat, C., Williams, C., Gillen, D., Hossack, M., Gilmour, D., Carswell, A., et al. (2000). Omeprazole, Helicobacter pylori status, and alterations in the intragastric milieu facilitating bacterial N-nitrosation. Gastroenterology 119, 339–347. doi: 10.1053/gast.2000.9367
Nakatsu, G., Li, X., Zhou, H., Sheng, J., Wong, S. H., Wu, W. K., et al. (2015). Gut mucosal microbiome across stages of colorectal carcinogenesis. Nat. Commun. 6:8727. doi: 10.1038/ncomms9727
Parks, D. H., Tyson, G. W., Hugenholtz, P., and Beiko, R. G. (2014). STAMP: statistical analysis of taxonomic and functional profiles. Bioinformatics 30, 3123–3124. doi: 10.1093/bioinformatics/btu494
Plottel, C. S., and Blaser, M. J. (2011). Microbiome and malignancy. Cell Host. Microbe 10, 324–335. doi: 10.1016/j.chom.2011.10.003
Segata, N., Izard, J., Waldron, L., Gevers, D., Miropolsky, L., Garrett, W., et al. (2011). Metagenomic biomarker discovery and explanation. Genome Biol. 12:R60. doi: 10.1186/gb-2011-12-6-r60
Sheh, A., and Fox, J. G. (2013). The role of the gastrointestinal microbiome in Helicobacter pylori pathogenesis. Gut Microbes 4, 505–531. doi: 10.4161/gmic.26205
Torre, L. A., Bray, F., Siegel, R. L., Ferlay, J., Lortet-Tieulent, J., and Jemal, A. (2015). Global cancer statistics, 2012. CA Cancer J. Clin. 65, 87–108. doi: 10.3322/caac.21262
Wang, L., Zhou, J., Xin, Y., Geng, C., Tian, Z., Yu, X., et al. (2016). Bacterial overgrowth and diversification of microbiota in gastric cancer. Eur. J. Gastroenterol. Hepatol. 28, 261–266. doi: 10.1097/meg.0000000000000542
Weiss, S., Xu, Z. Z., Peddada, S., Amir, A., Bittinger, K., Gonzalez, A., et al. (2017). Normalization and microbial differential abundance strategies depend upon data characteristics. Microbiome 5:27. doi: 10.1186/s40168-017-0237-y
Wroblewski, L. E., Peek, R. M. Jr., and Wilson, K. T. (2010). Helicobacter pylori and gastric cancer: factors that modulate disease risk. Clin. Microbiol. Rev. 23, 713–739. doi: 10.1128/cmr.00011-10
Yu, G., Torres, J., Hu, N., Medrano-Guzman, R., Herrera-Goepfert, R., Humphrys, M. S., et al. (2017). Molecular characterization of the human stomach microbiota in gastric cancer patients. Front. Cell. Infect. Microbiol. 7:302. doi: 10.3389/fcimb.2017.00302
Keywords: gastric cancer, microbiota, 16S rDNA, cancer microenvironment, risk
Citation: Chen X-H, Wang A, Chu A-N, Gong Y-H and Yuan Y (2019) Mucosa-Associated Microbiota in Gastric Cancer Tissues Compared With Non-cancer Tissues. Front. Microbiol. 10:1261. doi: 10.3389/fmicb.2019.01261
Received: 20 December 2018; Accepted: 21 May 2019;
Published: 05 June 2019.
Edited by:
Gary Moran, Trinity College Dublin, IrelandReviewed by:
Robert J. Moore, RMIT University, AustraliaSuleyman Yildirim, Istanbul Medipol University, Turkey
Copyright © 2019 Chen, Wang, Chu, Gong and Yuan. This is an open-access article distributed under the terms of the Creative Commons Attribution License (CC BY). The use, distribution or reproduction in other forums is permitted, provided the original author(s) and the copyright owner(s) are credited and that the original publication in this journal is cited, in accordance with accepted academic practice. No use, distribution or reproduction is permitted which does not comply with these terms.
*Correspondence: Yuan Yuan, eXVhbnl1YW5AY211LmVkdS5jbg==