- 1School of Environment and Civil Engineering, Jiangnan University, Wuxi, China
- 2State Key Laboratory of Lake Science and Environment, Nanjing Institute of Geography and Limnology, Chinese Academy of Sciences, Nanjing, China
- 3School of Biological Sciences, Nanjing Normal University, Nanjing, China
- 4University of Chinese Academy of Sciences, Beijing, China
Water-depth biodiversity gradient, one of the typical biogeographical patterns on Earth, is understudied for bacteria in freshwater ecosystems, and thus left the underlying mechanisms poorly understood especially for benthic bacteria. Here, we investigated the water-depth distribution of surface sediment bacterial phyla and their driving factors in Lake Lugu, a plateau lake in Southwest China. Our results revealed that the relative abundance of 11 dominant bacterial phyla showed various water-depth patterns, such as increasing, decreasing, hump-shaped, and U-shaped patterns. These patterns across phyla were consistent with their different niche positions of water depth, while the occupancy-abundance relationships were not dependent on phylum attributes. Consistently, phylum abundance was best explained by water depth; other physical and chemical factors, such as metal ion concentrations, SiO2, and pH, can also explain the variations in some bacterial phyla. Chemical variables were the main drivers of the dominant bacterial phyla. However, biotic variables also showed substantial importance for some phyla, such as Planctomycetes, Actinobacteria, and WS3. This work could provide new insights into the general water-depth patterns and underlying mechanisms of the relative abundance of bacterial phyla in freshwater ecosystems.
Introduction
Biogeographical patterns of biodiversity along gradients, such as those of latitude, elevation, and water depth, are among the most widely studied topics in ecology (Zintzen et al., 2017), and researchers have described the latitudinal and elevational patterns in biodiversity in a variety of ecosystems across the globe (Fuhrman et al., 2008; Wang et al., 2011; Shi et al., 2014). Similar to the more commonly studied latitudinal and elevational gradients, water-depth gradient show complex changes in various environmental attributes and thus impose strong environmental filtering on the aquatic microbial community (Bryant et al., 2012). The phylogenetic and physiological diversity of microbial phyla, especially those of bacteria, is considerably greater than that of animal and plant phyla (Prosser et al., 2007; Yeh et al., 2019). Furthermore, benthic bacteria play an important role in organic matter transformation and in the biogeochemical cycling of major elements such as nitrogen, phosphorus, sulfur, and iron (Martins et al., 2011; Cheng et al., 2014). Knowledge of benthic bacterial phyla distribution in aquatic ecosystems is of great significance and can provide novel perspectives regarding water-depth biodiversity patterns among microorganisms and their potential drivers and further contribute to the sustainable management of water resources. Nonetheless, as most studies focus on marine waters (Agogue et al., 2011; Bryant et al., 2012; Walsh et al., 2016), the general patterns and driving mechanisms of bacterial communities along water-depth gradients have been less studied for freshwater ecosystems.
In recent years, the niche position and niche breadth hypotheses have been used to investigate how niche characteristics account for species distribution and abundance (Brandle and Brandl, 2001). Among these two referenced niche parameters, niche position indicates the availability of habitat and resources (Teittinen et al., 2018). Some species have marginal niche positions at the very edges of the environmental ranges, while other species have nonmarginal niche positions that exist under average environmental conditions (Vilmi et al., 2019). Niche position, rather than niche breadth, is the chief predictive factor of species distributions, such as those of stream insects (Heino and de Mendoza, 2016). In addition, previous research has provided strong evidence that the occupancy and abundance relationships of species tend to be positively and often strongly linked with each other in a variety of ecosystems (Gaston et al., 2000; Foggo et al., 2007; Zuckerberg et al., 2009). Niche position could be a strong predictor of species variation in terms of occupancy and abundance. For instance, niche position is negatively associated with mean abundance in diatom and macroinvertebrate species, and the occupancy-abundance relationship clearly differs across taxonomic groups (Vilmi et al., 2019). At the community level, niche position and occupancy are related to the abundance distribution, but whether such a relationship exists at phylum level remains unexplored.
Traditional niche-based theory asserts that the relative abundances of species are determined by abiotic and biotic factors, such as environmental conditions, habitat heterogeneity, and species interactions (Dumbrell et al., 2010; Liao et al., 2016). Most studies have shown that local environmental conditions, such as physical and chemical attributes, can affect microbial community composition and species diversity in lake ecosystems and the biogeochemical processes they mediate (Staley et al., 2015; Qin et al., 2016). The bacterial community can be shaped by a variety of environmental factors, such as water depth, nitrogen, phosphorus, pH, and pollution (Brown et al., 2009; Haller et al., 2011; Song et al., 2012; Liu et al., 2015b; Zhang et al., 2019). In addition, biotic interactions could also affect microbial community structure. However, rarely considered are the important ecological processes associated with biotic variables, such as the community composition and diversity of other organisms that feed on bacteria (Langenheder et al., 2017). Ecosystems are constructed around interaction webs, such as predation, mutualism, competition, and host-parasite interactions, that connect every species to many others at an array of spatial scales (Estes et al., 2011; Amin et al., 2012). For example, viral abundance is typically strongly correlated with bacterial abundance in marine systems (Clasen et al., 2008; Hanson et al., 2017). In the soil, fungi and bacteria have an effect on the formation of each otherʼs community structure (Singh et al., 2009). Moreover, biotic attributes such as the composition of other taxonomic groups are generally better predictors of species diversity in streams than other environmental variables (Johnson and Hering, 2010).
Here, we explored the water-depth patterns and underlying drivers of the relative abundance of bacterial phyla in the surface sediments of Lake Lugu, a typical deep plateau lake system located in Southwest China. This lake has a water depth of 93.5 m and an area of 50.5 km2. We had the four following objectives. First, we examined the water-depth patterns in terms of the relative abundance of benthic bacterial phyla. Second, we expected that niche positions at the phylum and species levels are related and also the relationship between the occupancy and abundance of each phylum. Finally, we explored the main factors driving the above water-depth patterns in bacterial phyla and further quantified the relative importance of physical, chemical, and biological drivers.
Materials and Methods
Study Region
The studied Lake Lugu, with an elevation of 2,685 m, is located in the Yunnan Province, Southwest China (27°41′–27°45′N, 100°45′–100°50′E). It is one of the deepest freshwater plateau lakes in the region. This lake has spatial characteristics that include high connectivity and a small area, allowing species to be freely distributed at different locations with no apparent barriers to dispersal. Macrophytes were partly absent from the lake, allowing for the collection of surface sediments in shallow waters. In August 2010, we collected 37 surface sediment (~0–1 cm) samples along the water-depth gradient from 0 to 93.5 m. At each site, three sediment cores with a 6-cm diameter were retrieved for surface sediments. The sample collection process was described in detail in a previous study (Wang et al., 2012b).
Bacterial Community Analysis
Bacterial analyses were performed according to the previous literature (Wang et al., 2012a). Briefly, genomic DNA was extracted from surface sediment samples using the phenol chloroform method (Zhou et al., 1996). Bacterial tag-encoded FLX amplicon pyrosequencing was conducted as described previously (Dowd et al., 2008). Bacterial 16S rRNA genes were amplified using the 27F primer (5′ GAG TTT GAT CNT GGC TCA G 3′) with the 454 Life Sciences A sequencing adapter, and the modified 519R primer (5′ GTN TTA CNG CGG CKG CTG 3′) with a 8-bp barcode sequence and the 454 Life Sciences B sequencing adapter. The PCR amplification of a single 35 cycle was performed in a ABI9700 thermocycler (ABI, Foster City, USA) using the program 95°C for 2 min; 25 cycles of 95°C for 30 s, 55°C for 30 s, 72°C for 30 s; 72°C for 5 min; finally kept at 10°C. Triplicate positive PCR products were pooled and purified with AxyPrep DNA Gel Extraction Kit (Axygen, USA). Amplicon sequencing was performed based upon the manufacturer protocols (Roche Applied Science, Indianapolis, IN) for Titanium sequencing on FLX-titanium platform (Dowd et al., 2008). The purified amplicons were pooled at equal molality, and then sequenced using a Roche 454 FLX pyrosequencer (Roche, Switzerland).
All 16S rRNA pyrosequencing reads were analyzed using QIIME, version 1.9.0 (Caporaso et al., 2010b). We imported barcoded 16S rRNA gene sequences and removed the primers, demultiplexed reads, and then filtered them according to Phred quality scores. Quality criteria were a minimum sequence length of 200 bp, a maximum sequence length of 900 bp, and a minimum average quality score of 25. With such quality control, we allowed few ambiguous bases or mismatches in the primer sequence and no barcode errors, and a maximum homopolymer length of 6 bp.
Sequences were denoised with the Denoiser algorithm (Reeder and Knight, 2010), clustered into operational taxonomic units (OTUs) at 97% similarity level with the seed-based UCLUST algorithm (Edgar, 2010). We used the longest sequence in a cluster as the representative sequence for that OTU. Singletons, i.e., OTUs with only one read in the entire dataset, were removed and chimeras were excluded using ChimeraSlayer (Haas et al., 2011). After chimeras were removed via ChimeraSlayer, representative sequences from each OTU were aligned to the Greengenes v13.8 imputed core reference alignment (DeSantis et al., 2006) using PyNAST (Caporaso et al., 2010a). The taxonomic identity of each representative sequence was determined using the RDP Classifier (Wang et al., 2007) and chloroplast and archaeal sequences were removed. Before the subsequent analysis, bacterial community were rarefied at 1,139 sequences to avoid the variation in abundance or sampling intensity biased. The generated sequences can be found in figshare (https://doi.org/10.6084/m9.figshare.8052788).
Abiotic and Biotic Variables
We evaluated several environmental characteristics important to the biological community in the surface water of 0.5 m and bottom water near the sediment-water interface at each site (Supplementary Table S1). We analyzed the temperature, pH, conductivity, total nitrogen, total phosphorus, dissolved oxygen (DO), concentration (HCO3·water), and silica of the surface water, and measured the temperature, pH, conductivity, and DO of the bottom water. In addition, the surface sediment total phosphorus, loss-on-ignition (LOI), water content, grain size, porosity, and the metal ion concentrations were measured. The measured metal ions included Al, Ba, Be, Ca, Co, Cr, Cu, Fe, Li, K, Mg, Mn, Na, Ni, Pb, Sr., Ti, V, and Zn. The grain size was divided into five classes: <4, 4–16, 16–32, 32–64, and >64 μm. A detailed description of the methods for the measurement and calculation of the abiotic variables was described in a previous study (Wang et al., 2012b).
In terms of the biotic variables, diatoms and chironomids were classified and identified to the lowest possible taxonomic level (typically to the species level) as shown in previous studies (Wang et al., 2012b; Zhang et al., 2013). We used the following biotic factors as predictor variables: (1) the chlorophyll a concentration of the surface and bottom water, (2) the biomass of diatoms and chironomids, and (3) the richness of diatoms and chironomids. The concentration of chlorophyll a was the representative of the phytoplankton biomass. The biomass of diatoms and chironomids was indicated by the number of diatoms per gram of wet sediment and the count of head capsules per gram of wet sediment, respectively.
Statistical Analyses
First, we only included the highly abundant bacterial phyla by considering species occurrence percentages larger than 85%. We explored the relationships between water depth and the relative abundance of bacterial phyla with linear or quadratic models. The most appropriate model was selected based on the lowest value of Akaike’s information criterion (Yamaoka et al., 1978).
Second, we calculated the niche position of the water depth for both the species and phylum levels. For each species or phylum, we estimated the weighted water-depth position by averaging the product of their water-depth values and their abundances across all samples (Wang et al., 2013). We selected the linear or quadratic model based on the lower value of Akaike’s information criterion and explored the relationship of niche position between the phylum and species levels. In practice, the proportion of sites was considered as “occupancy,” and the mean abundance at occupied sites was considered as “abundance.” The relationship between the occupancy and abundance of bacterial phyla was examined by linear or quadric models, and the better model was selected based on the lower value of Akaike’s information criterion (Yamaoka et al., 1978).
Third, to identify the important factors affecting each phylum, we applied random forest model (Feld et al., 2016) and redundancy analysis (RDA). Statistical dependence between the explanatory variables was assessed using Pearson’s correlation coefficients, and variables with high correlation coefficients (Pearson r > 0.7) were excluded from the models. By performing principal component analysis, the 19 metal ion concentrations were reduced to the first two axes of the principal components analysis, which thus represented the environmental variables that reflected the geochemical factors. This process was performed to decrease the degrees of freedom so that they were lower than the number of sampled sites. The other measured variables were used as environmental variables without principal component analysis. For the random forest analysis, we used techniques such as cross-validation to prune the 2,000 trees to an optimal size (Prasad et al., 2006). The importance of a predictor variable was determined by its frequency of selection weighted by a measure of improvement of the model given each split and averaged across all trees (contributions were scaled to sum to 100). Furthermore, RDA was used to examine the potential explanatory variables of community composition at the phylum level. We used RDA with Hellinger-transformed abundance data of the bacterial community, as this transformation makes complex data with numerous zero values more suitable for the analysis with linear methods (Legendre and Gallagher, 2001).
Finally, we applied variation partitioning to quantify the relative importance of the main environmental drivers using linear model (Anderson and Cribble, 1998; Legendre, 2008). We categorized the explanatory variables into three groups: physical, chemical, and biological components. The physical component included water depth, conductivity of surface water, porosity, and grain size. The chemical component included surface water pH, total phosphorus, total nitrogen, SiO2 and HCO3, bottom water DO and pH, and surface sediment LOI, grain size, PC1 and PC2 of metals. For biological component, we considered the richness and biomass of diatoms and chironomids, and chlorophyll a of surface and bottom water. We generated three explanatory matrix models and estimated the proportions of variation in the relative abundance of the bacterial phyla explained by these three components. For each component, significant explanatory variables were selected by forward selection against the Hellinger-transformed abundance phylum data with 9,999 permutations.
We did not apply more statistical analyses because it is a challenge to differentiate the direct or indirect effects of underlying drivers on the relative abundance of bacterial phyla based on field observations. These above analyses were conducted with vegan V2.5-5 (Oksanen et al., 2013) and randomForestSRC V2.9.0 (Ishwaran and Kogalur 2014) in the R environment.
Results
In total, we selected 11 major bacterial phyla based on their relative abundance, including Proteobacteria, Nitrospirae, Chlorobi, Chloroflexi, and Bacteroidetes. Among these phyla, Proteobacteria was the most dominant, followed by Bacteroidetes, Chloroflexi, and Nitrospirae (Table 1). The relative abundance of most phyla, seven out of 11, exhibited significant (p < 0.05) and clear water-depth patterns (Figure 1), such as increasing, decreasing, hump-shaped, and U-shaped trends. For example, the relative abundance of Bacteroidetes decreased with water depth, while that of Nitrospirae increased toward deep water (Figure 1). In addition, Acidobacteria, Planctomycetes, and Gemmatimonadetes showed hump-shaped trends along the water-depth gradient (Figure 1). Interestingly, the phototrophic phyla Chlorobi and Chloroflexi showed U-shaped patterns.
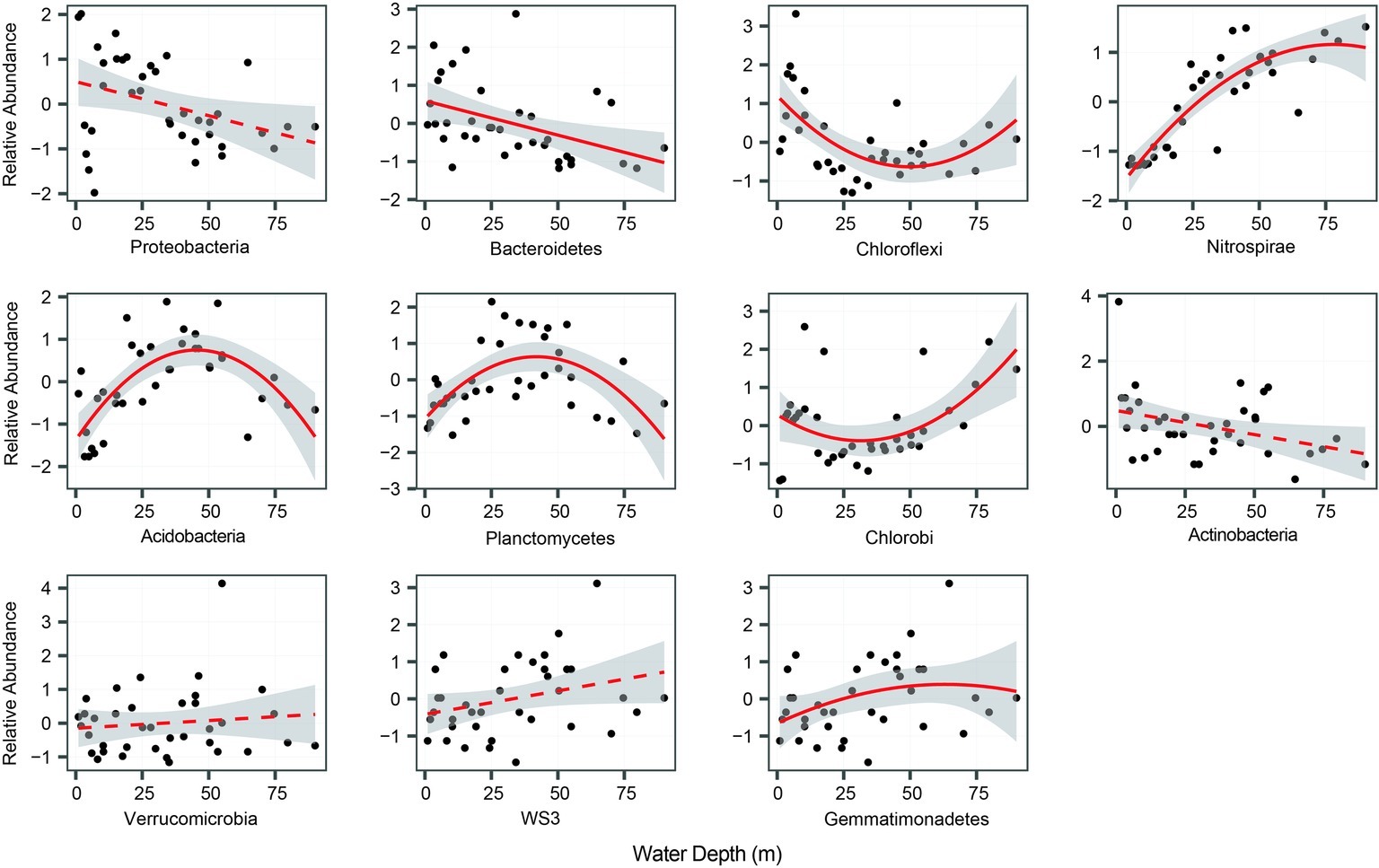
Figure 1. The relative abundance of bacterial phyla along the water-depth gradient. The relationships between water depth and relative abundance were modeled with linear and quadratic models. The better model was selected based on the lower value of Akaike’s information criterion. For better visualization, species relative abundance was scaled as mean = 0 and SD = 1.
At the phylum level, the niche position of water depth showed great variations, ranging from 26 to 48 m (Figure 2A). The phylum-level niche positions had significant (p < 0.05) positive correlations with those at the species level (Figure 2A). For the whole bacterial community, there was a significant relationship between occupancy and abundance (Figure 2B). This is consistent with the finding at the phylum level, which also showed rather strong and positive occupancy-abundance relationships (Figure 2B). However, there were no clear differences among phyla in terms of the relationship of occupancy and mean abundance.
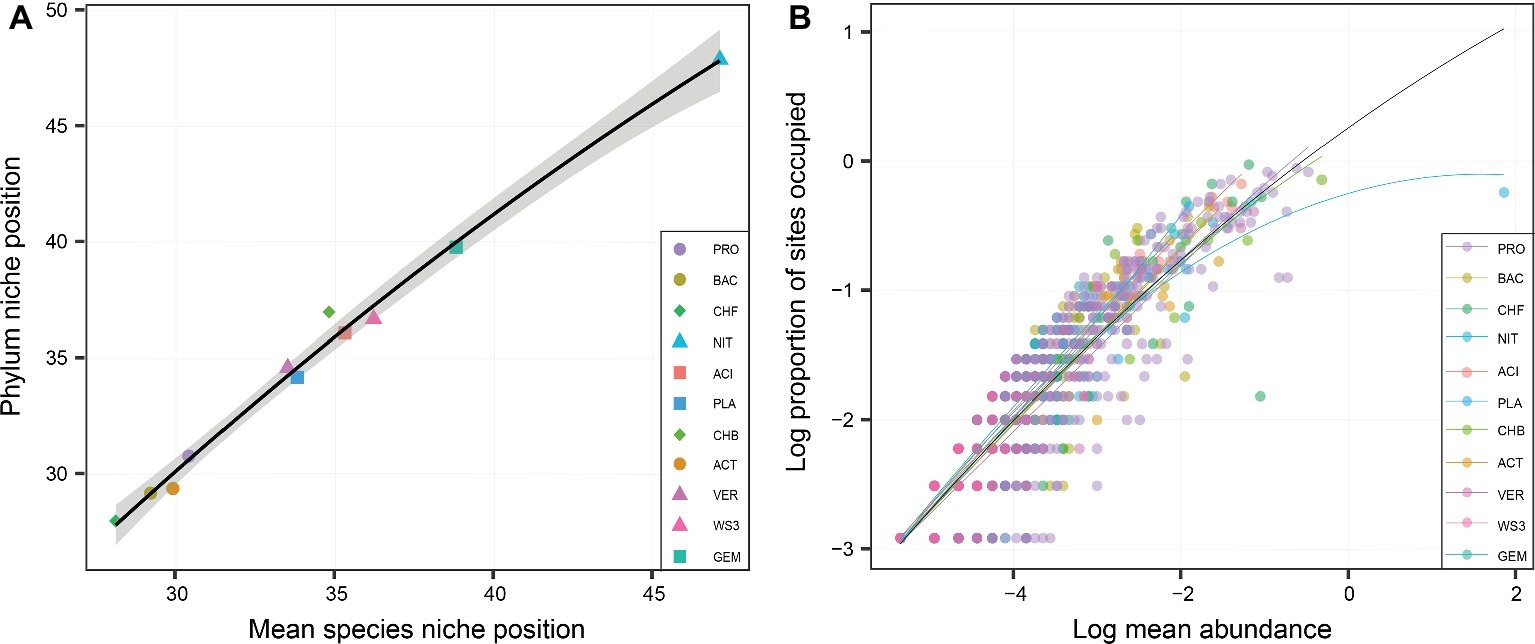
Figure 2. The relationship of niche position between the phylum and species levels (A), and the relationships between the occupancy and abundance of each phylum (B). The shapes in (A) represent various distributional patterns: ■, hump-shaped pattern; ●, decreasing pattern; ▲, increasing pattern; ♦, U-shaped pattern. (B) Relationships between occupancy and mean local abundance for bacterial phyla. The points in (B) represent the different species. Linear or quadratic models were selected according to a lower value of Akaike’s information criterion.
The random forest analyses showed that water depth was the strongest variable correlating with the relative abundance of the most bacterial phyla. Other environmental variables, such as metal ion concentrations, SiO2, pH, total phosphorus, DO, and conductivity also have important effects on bacterial phyla (Figure 3A). Interestingly, biological factors such as chironomid richness and chlorophyll a concentration were also important for some bacterial phyla (Figure 3A). Such findings were confirmed by RDA, which shows that water depth was the strongest factor (p < 0.001) related to the distribution of bacterial phyla, while biological attributes, such as diatoms biomass, also showed significant (p = 0.038) correlations (Figure 3B).
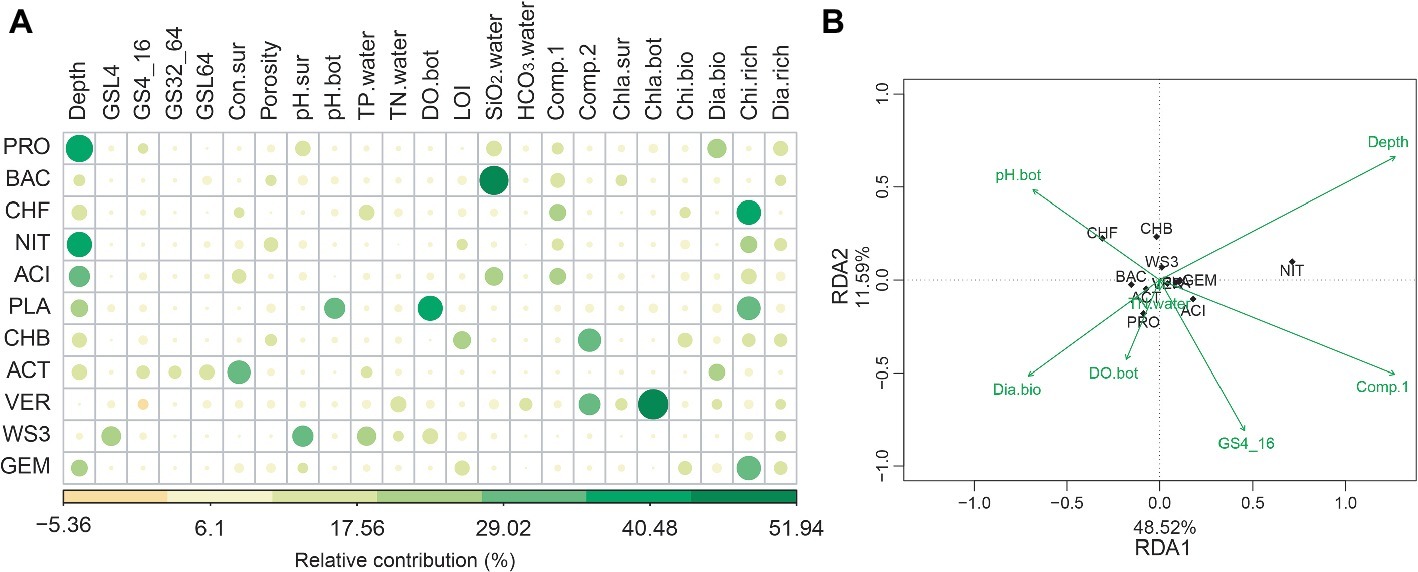
Figure 3. The abiotic and biotic factors related to the relative abundance of bacterial phyla. These factors were identified with random forest (A) and redundancy analysis (RDA, B). For abiotic factors, we considered water depth (depth), total phosphorus (TP.water), total nitrogen (TN.water), conductivity (Con.sur) and concentrations of (HCO3·water) and SiO2 (SiO2·water), pH (pH.bot and pH.sur), dissolved oxygen (DO.bot), sediment porosity, loss-on-ignition (LOI), the first two axes of metal ion principal component analysis (Comp.1 and Comp.2) and grain size. Grain size: <4 μm (GSL4), 4–16 μm (GS4_16), 32–64 μm (GS32_64), and > 64 μm (GSL64). Biotic factors included the biomass (Dia.bio and Chi.bio) and richness (Dia.rich and Chi,rich) of diatoms and chironomids, and chlorophyll a (Chla.bot and Chla.sur). For RDA, the abiotic variables were automatically selected based on Monte Carlo permutation tests (999 permutations).
In the variation partitioning analyses with the three variable components, the pure effect of chemical component was the higher for bacterial phyla rather than the pure effects of physical and biological components. The relative abundance of most phyla, such as Planctomycetes (29.9%), WS3 (22.1%), and Verrucomicrobia (21.0%), was well explained by the pure effect of chemical component. This is especially true for Verrucomicrobia and Gemmatimonadetes, which were only explained by the pure effect of chemical component (Figure 4). Physical and biological variables had effects on seven and six bacterial phyla, respectively. For Nitrospirae, physical variables were especially important, which explained 16.3% of its variation. The pure effect of biotic variables accounted for 8.1% variations of the relative abundance of Planctomycetes. For Proteobacteria, only 0.1% was explained by biological variables. Four bacterial phyla can be explained by the combination of pure effects of biological, chemical, and physical variables.
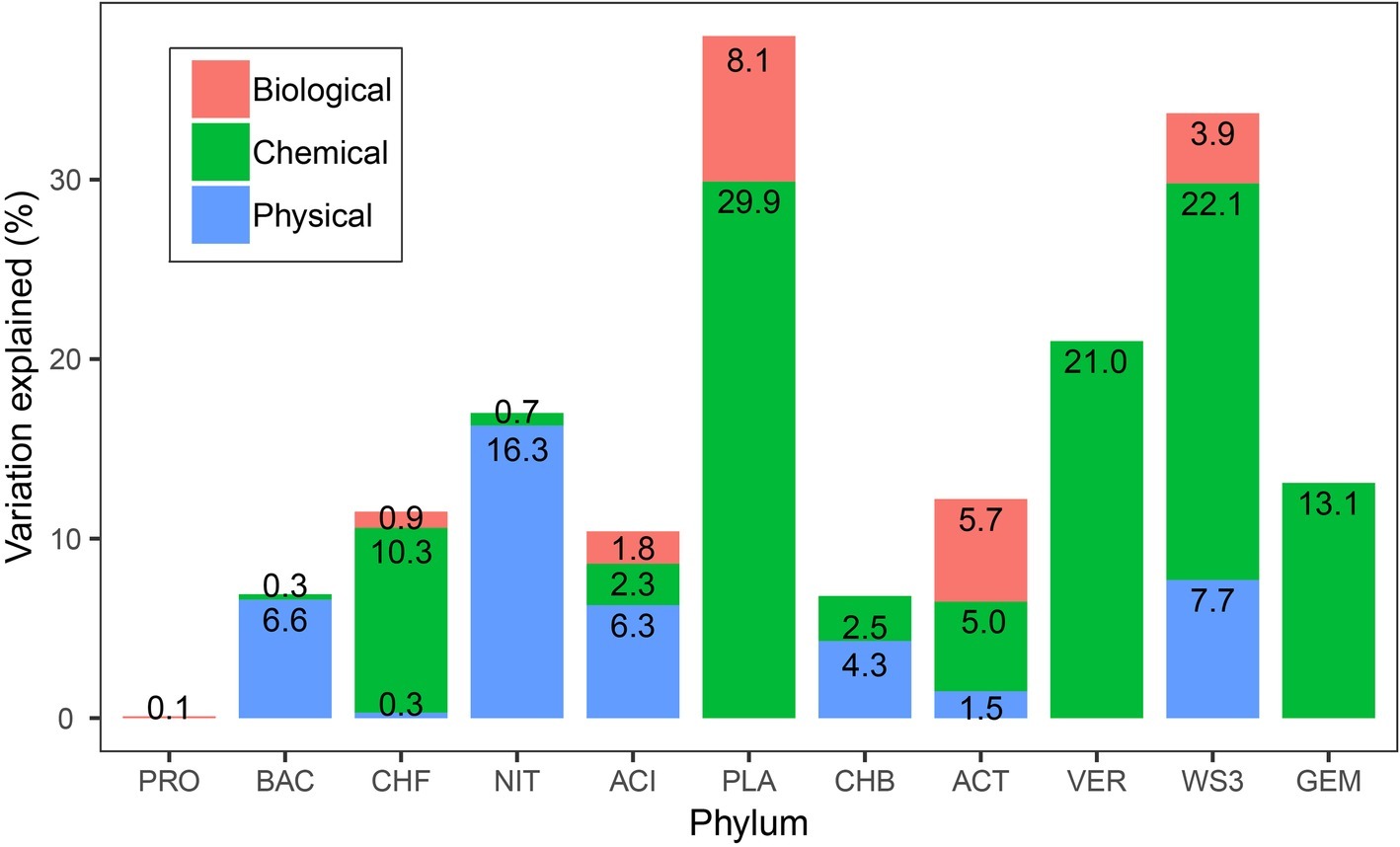
Figure 4. The proportion of the variance in bacterial phylum relative abundance explained by physical, chemical, and biological variables. For simplicity, the pure effects of the three components in predicting the relative abundance are shown, but not the joint effects or unexplained variances. An alternative version of this figure showing the unique and shared variance of each group can be found in Supplementary Figure S1.
Discussion
A long-standing task in ecology has been to explain biological distribution patterns and the drivers underlying these patterns. In recent years, the water-depth patterns of microbial community distribution have been extensively investigated in aquatic systems. However, most studies have focused on marine environments (Smith and Brown, 2002; Wang et al., 2013), while few studies have been conducted in freshwater ecosystems, such as lake sediments (Haglund et al., 2003; De Wever et al., 2005; Yu et al., 2014). The main aim of our study was to explore the distributional patterns of bacterial abundance at the phylum level and to analyze the abiotic and biotic variables explaining these patterns. Our results highlight four main findings. First, the bacterial phyla exhibited various patterns along the water-depth gradient, some of which are rarely reported, such as the hump-shaped and U-shaped patterns. Second, the occurrence of these patterns across phyla might be explained by the variation in their niche positions, while the occupancy-abundance relationships were not dependent on phylum attributes. Third, among all measured exploratory variables, water depth was the most important predictor of the relative abundance of bacterial phyla. Finally, although the environmental variables were shown to have pivotal roles in terms of the relative abundance of bacterial phyla, biotic variables could also substantially explain the abundance of some phyla, such as Planctomycetes, Actinobacteria, and WS3.
Proteobacteria was the most dominant phyla in the sediments of this lake, followed by Bacteroidetes, Chloroflexi, and Nitrospirae. This is consistent with previous studies that Proteobacteria and Bacteroidetes are the most abundant phyla in sediments (Diao et al., 2017). These phyla have frequently been shown to dominate freshwater bacterial communities (Dai et al., 2016). At present, investigations of the spatial pattern of bacterial relative abundance in freshwater lake sediments indicate that bacterial abundance shows clear spatial variations (Bouzat et al., 2013; Liu et al., 2015a). However, the distributional patterns of bacterial abundance along water-depth gradients, especially among bacterial phyla, have been less explored. Bacterial abundance generally decreases with water depth in freshwater systems (Liu et al., 2016). Our results reveal various water-depth patterns in terms of the relative abundance of bacterial phyla, among which some patterns have been rarely reported so far. For instance, there were significantly (p < 0.05) decreasing patterns for Bacteroidetes, increasing patterns for Nitrospirae, and hump-shaped patterns for Acidobacteria, Planctomycetes, and Gemmatimonadetes. The decreasing and increasing water-depth patterns of the relative abundance of bacterial phyla are consistent with the patterns found in earlier studies along other environmental gradients, such as elevations. For instance, the relative abundance of Actinobacteria, Bacteroidetes, and Deltaproteobacteria increases with elevation in stream biofilms, while that of Alphaproteobacteria decreases with elevation (Wang et al., 2012a). However, the U-shaped patterns of Chlorobi and Chloroflexi were unexpected because such patterns are extremely rarely observed in nature, and these two phyla have phototrophic capability (Gupta, 2010). The two phyla are commonly present in the surface waters and bottom waters of deep lakes and oceans (Newton et al., 2011). The phylum Chlorobi is resistant to very low light conditions (Freed et al., 2019) and primarily exists in the anoxic aquatic environment of stratified lakes (Gupta, 2010). Chloroflexi, however, contains both photosynthetic and nonphotosynthetic members (Bryant and Frigaard, 2006), but metabolic insights suggest that a primarily heterotrophic lifestyle boosted by light-driven energy generation via aerobic anoxygenic phototrophy occurs in some groups (Mehrshad et al., 2018). It should be noted that alternative explanations may be needed for such U-shaped patterns of Chlorobi and Chloroflexi.
These water-depth patterns may be explained by the niche positions of the bacterial phyla. Consistent with the various water-depth patterns of relative abundance, the niche position also obviously differed among phyla. For instance, the phyla with decreasing water-depth patterns had a lower niche position, while those with increasing and hump-shaped patterns had a higher niche position. In other words, niche position may have a certain impact on bacterial phylum distributional patterns. This phenomenon has also been reported for other taxonomic groups, such as the distribution of stream insects, the predictability of which depends on niche position (Heino and de Mendoza, 2016). Furthermore, niche position could be an important predictor of the regional occupancy and local abundance of diatoms and insects, such as those in streams (Rocha et al., 2018). However, the occupancy-abundance relationship showed a relatively similar trend for all phyla. This result may have occurred because of the taxonomic relatedness among bacteria, as taxonomic relatedness has only minor influence on occupancy and abundance, as observed among the diatoms and macroinvertebrates across a set of lakes (Heino and Tolonen, 2018). Hence, the occupancy-abundance relationship could not explain the variation in water-depth patterns across phyla.
Physicochemical variables were important in explaining the relative abundance of bacterial phyla along the water-depth gradient. Among them, water depth was the most important environmental factor affecting the relative abundance of bacterial phyla in this lake ecosystem, which is consistent with previous studies showing that water depth has important effects on bacterial community composition (Yokokawa et al., 2010; Zhang et al., 2015). However, water depth could also be associated with many other physiochemical variables, including temperature, pressure, productivity, and nutrient availability (Smith and Brown, 2002; Bryant et al., 2012). It seems that water depth is only a proxy for multiple physiochemical variables, and we cannot completely exclude the influence of other environmental and biological factors on the vertical distribution of bacterial phyla.
In addition, some other environmental factors, such as metal ion concentrations, SiO2, pH, total phosphorus, and conductivity, were also related to some bacterial phyla abundance in our study. This is in line with the finding that species are filtered by environmental factors and reproduce more under more suitable conditions (Schei et al., 2012; Alofs and Jackson, 2015). For instance, sediment bacterial abundance in freshwater plateau lakes could be influenced by pH and conductivity (Xiong et al., 2012; Liu et al., 2016), and ion concentrations are an important factor controlling bacterial community composition in lake system (Liu et al., 2014). Environmental variables, such as pH and silicate silicon, are important for the composition of the bacterial community in lake sediments, such as those in an Arctic lake area (Wang et al., 2016).
However, we also found that biotic variables were important in accounting for the relative abundance of bacterial phyla. For example, biotic variables explained 8.1, 5.7, 3.9, and 1.8% of the variation in Planctomycetes, Actinobacteria, WS3, and Acidobacteria, respectively. In particular, the relative abundance of Proteobacteria was only explained by biological variables of 0.1%, indicating that additional abiotic and biotic interactions are involved. Biotic variables, such as chlorophyll a, and chironomid richness, were identified to be important for the abundance of some bacterial phyla. This is in line with previous findings that biotic interactions can affect species distributions and alter biodiversity patterns (Alofs and Jackson, 2015; Sarker et al., 2018). Bacterial abundances within the lakes are positively correlated with chlorophyll a (Lee and Bong, 2008; Liu et al., 2016). In aquatic ecosystems, phytoplankton excrete dissolved organic matter, such as extracellular organic carbon, which may be an important source of bacterial growth. Phytoplankton and bacteria also compete for common inorganic phosphorus resources (Aota and Nakajima, 2001). Bacteria and benthic diatoms have a mutually beneficial effect in terms of promoting microalgae growth and maintaining the bacterial community (Jauffrais et al., 2017). For example, in marine environments, the bacterial abundance and community structure largely depend on the growth and physiological status of diatoms (Grossart et al., 2005), and bacteria can also control algal populations by inhibiting the growth of diatoms or by the active lysis of algal cells (Paul and Pohnert, 2011). Moreover, experimental evidence has shown that bacterial communities and chironomid larvae have a symbiotic relationship (Kuncham et al., 2017), chironomids can influence bacterial densities by grazing, and bacterial densities can in turn be related to variation in larval chironomids density (Schmid and Schmid-Araya, 2010).
Conclusion
In summary, we found that the relative abundance of bacterial phyla showed various water-depth patterns, such as hump-shaped and U-shaped trends. The niche position of each phylum was significantly different, which is consistent with their abundance distribution patterns. The occupancy-abundance relationship did not show differences among phyla, and niche position could be one of the predictors of the distribution of bacterial phyla. We further found that water depth was the predominant driver of the relative abundance of bacterial phyla. The relative abundance was influenced mainly by chemical variables, while the pure effect of biological variables was also important for some phyla, such as Planctomycetes, Actinobacteria, and WS3. We provided new evidence regarding the important role of biological variables in explaining the variation in bacterial communities from the perspective of the relative abundance of bacterial phyla.
Data Availability
The datasets generated for this study can be found in figshare, https://doi.org/10.6084/m9.figshare.8052788.
Author Contributions
JW conceived the idea and performed the bioinformatic analyses. JW, XY, and QW provided physiochemical and biological data. KW led the statistical analyses with the contributions from WZ and JW and wrote the first draft of the manuscript. KW, JW, and WZ finished the manuscript with the contributions from JS, LZ, and XC. All the authors contributed substantially to the study.
Funding
The work was financially supported by the Program of Global Change and Mitigation (2017YFA0605200), CAS Strategic Pilot Science and Technology (XDB31000000), CAS Key Research Program of Frontier Sciences (QYZDB-SSW-DQC043), NSFC Science Fund for Creative Research Groups (41621002), and NSFC (41571058, 41871048, 91851117).
Conflict of Interest Statement
The authors declare that the research was conducted in the absence of any commercial or financial relationships that could be construed as a potential conflict of interest.
Acknowledgments
We are grateful to X. Chen, M. Yao, and Z. Hu for field sampling.
Supplementary Material
The Supplementary Material for this article can be found online at: https://www.frontiersin.org/articles/10.3389/fmicb.2019.01521/full#supplementary-material
References
Agogue, H., Lamy, D., Neal, P. R., Sogin, M. L., and Herndl, G. J. (2011). Water mass-specificity of bacterial communities in the North Atlantic revealed by massively parallel sequencing. Mol. Ecol. 20, 258–274. doi: 10.1111/j.1365-294x.2010.04932.x
Alofs, K. M., and Jackson, D. A. (2015). The abiotic and biotic factors limiting establishment of predatory fishes at their expanding northern range boundaries in Ontario, Canada. Glob. Chang. Biol. 21, 2227–2237. doi: 10.1111/gcb.12853
Amin, S. A., Parker, M. S., and Armbrust, E. V. (2012). Interactions between diatoms and bacteria. Microbiol. Mol. Biol. Rev. 76, 667–684. doi: 10.1128/mmbr.00007-12
Anderson, M., and Cribble, N. (1998). Partitioning the variation among spatial, temporal and environmental components in a multivariate data set. Aust. J. Ecol. 23, 158–167.
Aota, Y., and Nakajima, H. (2001). Mutualistic relationships between phytoplankton and bacteria caused by carbon excretion from phytoplankton. Ecol. Res. 16, 289–299. doi: 10.1046/j.1440-1703.2001.00396.x
Bouzat, J. L., Hoostal, M. J., and Looft, T. (2013). Spatial patterns of bacterial community composition within Lake Erie sediments. J. Great Lakes Res. 39, 344–351. doi: 10.1016/j.jglr.2013.03.003
Brandle, M., and Brandl, R. (2001). Distribution, abundance and niche breadth of birds: scale matters. Glob. Ecol. Biogeogr. 10, 173–177. doi: 10.1046/j.1466-822x.2001.00213.x
Brown, M. V., Philip, G. K., Bunge, J. A., Smith, M. C., Bissett, A., Lauro, F. M., et al. (2009). Microbial community structure in the North Pacific ocean. ISME J. 3, 1374–1386. doi: 10.1038/ismej.2009.86
Bryant, D. A., and Frigaard, N. U. (2006). Prokaryotic photosynthesis and phototrophy illuminated. Trends Microbiol. 14, 488–496. doi: 10.1016/j.tim.2006.09.001
Bryant, J. A., Stewart, F. J., Eppley, J. M., and Delong, E. F. (2012). Microbial community phylogenetic and trait diversity declines with depth in a marine oxygen minimum zone. Ecology 93, 1659–1673. doi: 10.1890/11-1204.1
Caporaso, J. G., Bittinger, K., Bushman, F. D., DeSantis, T. Z., Andersen, G. L., and Knight, R. (2010a). PyNAST: a flexible tool for aligning sequences to a template alignment. Bioinformatics 26, 266–267. doi: 10.1093/bioinformatics/btp636
Caporaso, J. G., Kuczynski, J., Stombaugh, J., Bittinger, K., Bushman, F. D., Costello, E. K., et al. (2010b). QIIME allows analysis of high-throughput community sequencing data. Nat. Methods 7, 335–336. doi: 10.1038/nmeth.f.303
Cheng, W., Zhang, J. X., Wang, Z., Wang, M., and Xie, S. G. (2014). Bacterial communities in sediments of a drinking water reservoir. Ann. Microbiol. 64, 875–878. doi: 10.1007/s13213-013-0712-z
Clasen, J. L., Brigden, S. M., Payet, J. P., and Suttle, C. A. (2008). Evidence that viral abundance across oceans and lakes is driven by different biological factors. Freshw. Biol. 53, 1090–1100. doi: 10.1111/j.1365-2427.2008.01992.x
Dai, Y., Yang, Y., Wu, Z., Feng, Q., Xie, S., and Liu, Y. (2016). Spatiotemporal variation of planktonic and sediment bacterial assemblages in two plateau freshwater lakes at different trophic status. Appl. Microbiol. Biotechnol. 100, 4161–4175. doi: 10.1007/s00253-015-7253-2
De Wever, A., Muylaert, K., Van Der Gucht, K., Pirlot, S., Cocquyt, C., Descy, J. P., et al. (2005). Bacterial community composition in Lake Tanganyika: vertical and horizontal heterogeneity. Appl. Environ. Microbiol. 71, 5029–5037. doi: 10.1128/AEM.71.9.5029-5037.2005
DeSantis, T. Z., Hugenholtz, P., Larsen, N., Rojas, M., Brodie, E. L., Keller, K., et al. (2006). Greengenes, a chimera-checked 16S rRNA gene database and workbench compatible with ARB. Appl. Environ. Microbiol. 72, 5069–5072. doi: 10.1128/aem.03006-05
Diao, M., Sinnige, R., Kalbitz, K., Huisman, J., and Muyzer, G. (2017). Succession of bacterial communities in a seasonally stratified lake with an anoxic and sulfidic hypolimnion. Front. Microbiol. 8:15. doi: 10.3389/fmicb.2017.02511
Dowd, S. E., Sun, Y., Wolcott, R. D., Domingo, A., and Carroll, J. A. (2008). Bacterial tag–encoded FLX amplicon pyrosequencing (bTEFAP) for microbiome studies: bacterial diversity in the ileum of newly weaned salmonella-infected pigs. Foodborne Pathog. Dis. 5, 459–472. doi: 10.1089/fpd.2008.0107
Dumbrell, A. J., Nelson, M., Helgason, T., Dytham, C., and Fitter, A. H. (2010). Relative roles of niche and neutral processes in structuring a soil microbial community. ISME J. 4, 337–345. doi: 10.1038/ismej.2009.122
Edgar, R. C. (2010). Search and clustering orders of magnitude faster than BLAST. Bioinformatics 26, 2460–2461. doi: 10.1093/bioinformatics/btq461
Estes, J. A., Terborgh, J., Brashares, J. S., Power, M. E., Berger, J., Bond, W. J., et al. (2011). Trophic downgrading of planet earth. Science 333, 301–306. doi: 10.1126/science.1205106
Feld, C. K., Segurado, P., and Gutierrez-Canovas, C. (2016). Analysing the impact of multiple stressors in aquatic biomonitoring data: a ‘cookbook’ with applications in R. Sci. Total Environ. 573, 1320–1339. doi: 10.1016/j.scitotenv.2016.06.243
Foggo, A., Bilton, D. T., and Rundle, S. D. (2007). Do developmental mode and dispersal shape abundance-occupancy relationships in marine macroinvertebrates? J. Anim. Ecol. 76, 695–702. doi: 10.1111/j.1365-2656.2007.01245.x
Freed, S., Robertson, S., Meyer, T., and Kyndt, J. (2019). Draft whole-genome sequence of the green sulfur photosynthetic bacterium Chlorobaculum sp. strain 24CR, isolated from the carmel river. Microbiol. Resour. Announc. 8, 1–2. doi: 10.1128/MRA.00116-19
Fuhrman, J. A., Steele, J. A., Hewson, I., Schwalbach, M. S., Brown, M. V., Green, J. L., et al. (2008). A latitudinal diversity gradient in planktonic marine bacteria. Proc. Natl. Acad. Sci. USA 105, 7774–7778. doi: 10.1073/pnas.0803070105
Gaston, K. J., Blackburn, T. M., Greenwood, J. J. D., Gregory, R. D., Quinn, R. M., and Lawton, J. H. (2000). Abundance-occupancy relationships. J. Appl. Ecol. 37, 39–59. doi: 10.1046/j.1365-2664.2000.00485.x
Grossart, H. P., Levold, F., Allgaier, M., Simon, M., and Brinkhoff, T. (2005). Marine diatom species harbour distinct bacterial communities. Environ. Microbiol. 7, 860–873. doi: 10.1111/j.1462-2920.2005.00759.x
Gupta, R. S. (2010). Molecular signatures for the main phyla of photosynthetic bacteria and their subgroups. Photosynth. Res. 104, 357–372. doi: 10.1007/s11120-010-9553-9
Haas, B. J., Gevers, D., Earl, A. M., Feldgarden, M., Ward, D. V., Giannoukos, G., et al. (2011). Chimeric 16S rRNA sequence formation and detection in Sanger and 454-pyrosequenced PCR amplicons. Genome Res. 21, 494–504. doi: 10.1101/gr.112730.110
Haglund, A. L., Lantz, P., Tornblom, E., and Tranvik, L. (2003). Depth distribution of active bacteria and bacterial activity in lake sediment. FEMS Microbiol. Ecol. 46, 31–38. doi: 10.1016/s0168-6496(03)00190-9
Haller, L., Tonolla, M., Zopfi, J., Peduzzi, R., Wildi, W., and Pote, J. (2011). Composition of bacterial and archaeal communities in freshwater sediments with different contamination levels (Lake Geneva, Switzerland). Water Res. 45, 1213–1228. doi: 10.1016/j.watres.2010.11.018
Hanson, A., Berges, J., and Young, E. (2017). Virus morphological diversity and relationship to bacteria and chlorophyll across a freshwater trophic gradient in the Lake Michigan watershed. Hydrobiologia 794, 93–108. doi: 10.1007/s10750-016-3084-0
Heino, J., and De Mendoza, G. (2016). Predictability of stream insect distributions is dependent on niche position, but not on biological traits or taxonomic relatedness of species. Ecography 39, 1216–1226. doi: 10.1111/ecog.02034
Heino, J., and Tolonen, K. T. (2018). Ecological niche features override biological traits and taxonomic relatedness as predictors of occupancy and abundance in lake littoral macroinvertebrates. Ecography 41, 2092–2103. doi: 10.1111/ecog.03968
Ishwaran, H., and Kogalur, U. (2014). RandomForestSRC: Random forests for survival, regression and classification (RF-SRC). R package version 1.
Jauffrais, T., Agogue, H., Gemin, M.-P., Beaugeard, L., and Martin-Jezequel, V. (2017). Effect of bacteria on growth and biochemical composition of two benthic diatoms Halamphora coffeaeformis and Entomoneis paludosa. J. Exp. Mar. Biol. Ecol. 495, 65–74. doi: 10.1016/j.jembe.2017.06.004
Johnson, R. K., and Hering, D. (2010). Spatial congruency of benthic diatom, invertebrate, macrophyte, and fish assemblages in European streams. Ecol. Appl. 20, 978–992. doi: 10.1890/08-1153.1
Kuncham, R., Sivaprakasam, T., Puneeth Kumar, R., Sreenath, P., Nayak, R., Thayumanavan, T., et al. (2017). Bacterial fauna associating with chironomid larvae from lakes of Bengaluru city, India - A 16s rRNA gene based identification. Genom. Data 12, 44–48. doi: 10.1016/j.gdata.2017.03.001
Langenheder, S., Wang, J. J., Karjalainen, S. M., Laamanen, T. M., Tolonen, K. T., Vilmi, A., et al. (2017). Bacterial metacommunity organization in a highly connected aquatic system. FEMS Microbiol. Ecol. 93, 1–9. doi: 10.1093/femsec/fiw225
Lee, C. W., and Bong, C. W. (2008). Bacterial abundance and production, and their relation to primary production in tropical coastal waters of Peninsular Malaysia. Mar. Freshw. Res. 59, 10–21. doi: 10.1071/MF07099
Legendre, P. (2008). Studying beta diversity: ecological variation partitioning by multiple regression and canonical analysis. J. Plant Ecol. 1, 3–8. doi: 10.1093/jpe/rtm001
Legendre, P., and Gallagher, E. D. (2001). Ecologically meaningful transformations for ordination of species data. Oecologia 129, 271–280. doi: 10.1007/s004420100716
Liao, J. Q., Cao, X. F., Zhao, L., Wang, J., Gao, Z., Wang, M. C., et al. (2016). The importance of neutral and niche processes for bacterial community assembly differs between habitat generalists and specialists. FEMS Microbiol. Ecol. 92, 1–10. doi: 10.1093/femsec/fiw174
Liu, K., Liu, Y., Jiao, N., Zhu, L., Wang, J., Hu, A., et al. (2016). Vertical variation of bacterial community in Nam Co, a large stratified lake in central Tibetan Plateau. Anton. Leeuw. Int. J. Gen. Mol. Microbiol. 109, 1323–1335. doi: 10.1007/s10482-016-0731-4
Liu, Y. Q., Priscu, J. C., Yao, T. D., Vick-Majors, T. J., Michaud, A. B., Jiao, N. Z., et al. (2014). A comparison of pelagic, littoral, and riverine bacterial assemblages in Lake Bangongco, Tibetan Plateau. FEMS Microbiol. Ecol. 89, 211–221. doi: 10.1111/1574-6941.12278
Liu, S., Ren, H. X., Shen, L. D., Lou, L. P., Tan, G. M., Zheng, P., et al. (2015b). pH levels drive bacterial community structure in sediments of the Qiantang river as determined by 454 pyrosequencing. Front. Microbiol. 6:7. doi: 10.3389/fmicb.2015.00285
Liu, L.-X., Xu, M., Qiu, S., and Shen, R.-C. (2015a). Spatial patterns of benthic bacterial communities in a large lake. Int. Rev. Hydrobiol. 100, 97–105. doi: 10.1002/iroh.201401734
Martins, G., Terada, A., Ribeiro, D. C., Corral, A. M., Brito, A. G., Smets, B. F., et al. (2011). Structure and activity of lacustrine sediment bacteria involved in nutrient and iron cycles. FEMS Microbiol. Ecol. 77, 666–679. doi: 10.1111/j.1574-6941.2011.01145.x
Mehrshad, M., Salcher, M. M., Okazaki, Y., Nakano, S., Simek, K., Andrei, A. S., et al. (2018). Hidden in plain sight-highly abundant and diverse planktonic freshwater Chloroflexi. Microbiome 6:13. doi: 10.1186/s40168-018-0563-8
Newton, R. J., Jones, S. E., Eiler, A., McMahon, K. D., and Bertilsson, S. (2011). A guide to the natural history of freshwater lake bacteria. Microbiol. Mol. Biol. Rev. 75, 14–49.
Oksanen, J., Blanchet, F. G., Kindt, R., Legendre, P., Minchin, P. R., O’Hara, R., et al. (2013). Package ‘vegan’. Community ecology package, version 2.
Paul, C., and Pohnert, G. (2011). Interactions of the algicidal bacterium Kordia algicida with diatoms: regulated protease excretion for specific algal lysis. PLoS One 6:8. doi: 10.1371/journal.pone.0021032
Prasad, A. M., Iverson, L. R., and Liaw, A. (2006). Newer classification and regression tree techniques: bagging and random forests for ecological prediction. Ecosystems 9, 181–199. doi: 10.1007/s10021-005-0054-1
Prosser, J. I., Bohannan, B. J. M., Curtis, T. P., Ellis, R. J., Firestone, M. K., Freckleton, R. P., et al. (2007). Essay - the role of ecological theory in microbial ecology. Nat. Rev. Microbiol. 5, 384–392. doi: 10.1038/nrmicro1643
Qin, Y., Hou, J., Deng, M., Liu, Q., Wu, C., Ji, Y., et al. (2016). Bacterial abundance and diversity in pond water supplied with different feeds. Sci. Rep. 6, 1–13. doi: 10.1038/srep35232
Reeder, J., and Knight, R. (2010). Rapidly denoising pyrosequencing amplicon reads by exploiting rank-abundance distributions. Nat. Methods 7, 668–669. doi: 10.1038/nmeth0910-668b
Rocha, M. P., Bini, L. M., Siqueira, T., Hjort, J., Gronroos, M., Lindholm, M., et al. (2018). Predicting occupancy and abundance by niche position, niche breadth and body size in stream organisms. Oecologia 186, 205–216. doi: 10.1007/s00442-017-3988-z
Sarker, S., Al-Noman, M., Basak, S. C., and Islam, M. M. (2018). Do biotic interactions explain zooplankton diversity differences in the Meghna river estuary ecosystems of Bangladesh? Estuar. Coast. Shelf Sci. 212, 146–152. doi: 10.1016/j.ecss.2018.07.012
Schei, F. H., Blom, H. H., Gjerde, I., Grytnes, J. A., Heegaard, E., and Saetersdal, M. (2012). Fine-scale distribution and abundance of epiphytic lichens: environmental filtering or local dispersal dynamics? J. Veg. Sci. 23, 459–470. doi: 10.1111/j.1654-1103.2011.01368.x
Schmid, P. E., and Schmid-Araya, J. M. (2010). Scale-dependent relations between bacteria, organic matter and invertebrates in a headwater stream. Fundam. Appl. Limnol. 176, 365–375. doi: 10.1127/1863-9135/2010/0176-0365
Shi, L.-L., Mortimer, P. E., Slik, J. W. F., Zou, X.-M., Xu, J., Feng, W.-T., et al. (2014). Variation in forest soil fungal diversity along a latitudinal gradient. Fungal Divers. 64, 305–315. doi: 10.1007/s13225-013-0270-5
Singh, B. K., Dawson, L. A., Macdonald, C. A., and Buckland, S. M. (2009). Impact of biotic and abiotic interaction on soil microbial communities and functions: a field study. Appl. Soil Ecol. 41, 239–248. doi: 10.1016/j.apsoil.2008.10.003
Smith, K. F., and Brown, J. H. (2002). Patterns of diversity, depth range and body size among pelagic fishes along a gradient of depth. Glob. Ecol. Biogeogr. 11, 313–322. doi: 10.1046/j.1466-822x.2002.00286.x
Song, H., Li, Z., Du, B., Wang, G., and Ding, Y. (2012). Bacterial communities in sediments of the shallow Lake Dongping in China. J. Appl. Microbiol. 112, 79–89. doi: 10.1111/j.1365-2672.2011.05187.x
Staley, C., Gould, T. J., Wang, P., Phillips, J., Cotner, J. B., and Sadowsky, M. J. (2015). Species sorting and seasonal dynamics primarily shape bacterial communities in the Upper Mississippi River. Sci. Total Environ. 505, 435–445. doi: 10.1016/j.scitotenv.2014.10.012
Teittinen, A., Weckstrom, J., and Soininen, J. (2018). Cell size and acid tolerance constrain pond diatom distributions in the subarctic. Freshw. Biol. 63, 1569–1578. doi: 10.1111/fwb.13186
Vilmi, A., Tolonen, K. T., Karjalainen, S. M., and Heino, J. (2019). Niche position drives interspecific variation in occupancy and abundance in a highly-connected lake system. Ecol. Indic. 99, 159–166. doi: 10.1016/j.ecolind.2018.12.029
Walsh, E. A., Kirkpatrick, J. B., Rutherford, S. D., Smith, D. C., Sogin, M., and D’hondt, S. (2016). Bacterial diversity and community composition from seasurface to subseafloor. ISME J. 10, 979–989. doi: 10.1038/ismej.2015.175
Wang, Q., Garrity, G. M., Tiedje, J. M., and Cole, J. R. (2007). Naive Bayesian classifier for rapid assignment of rRNA sequences into the new bacterial taxonomy. Appl. Environ. Microbiol. 73, 5261–5267. doi: 10.1128/aem.00062-07
Wang, J., Shen, J., Wu, Y., Tu, C., Soininen, J., Stegen, J. C., et al. (2013). Phylogenetic beta diversity in bacterial assemblages across ecosystems: deterministic versus stochastic processes. ISME J. 7, 1310–1321. doi: 10.1038/ismej.2013.30
Wang, J., Soininen, J., He, J., and Shen, J. (2012a). Phylogenetic clustering increases with elevation for microbes. Environ. Microbiol. Rep. 4, 217–226. doi: 10.1111/j.1758-2229.2011.00324.x
Wang, J., Soininen, J., Zhang, Y., Wang, B., Yang, X., and Shen, J. (2011). Contrasting patterns in elevational diversity between microorganisms and macroorganisms. J. Biogeogr. 38, 595–603. doi: 10.1111/j.1365-2699.2010.02423.x
Wang, Q., Yang, X., Hamilton, P. B., and Zhang, E. (2012b). Linking spatial distributions of sediment diatom assemblages with hydrological depth profiles in a plateau deep-water lake system of subtropical China. Fottea 12, 59–73. doi: 10.5507/fot.2012.005
Wang, N. F., Zhang, T., Yang, X., Wang, S., Yu, Y., Dong, L. L., et al. (2016). Diversity and composition of bacterial community in soils and lake sediments from an arctic lake area. Front. Microbiol. 7:9. doi: 10.3389/fmicb.2016.01170
Xiong, J. B., Liu, Y. Q., Lin, X. G., Zhang, H. Y., Zeng, J., Hou, J. Z., et al. (2012). Geographic distance and pH drive bacterial distribution in alkaline lake sediments across Tibetan Plateau. Environ. Microbiol. 14, 2457–2466. doi: 10.1111/j.1462-2920.2012.02799.x
Yamaoka, K., Nakagawa, T., and Uno, T. (1978). Application of Akaike’s information criterion (AIC) in the evaluation of linear pharmacokinetic equations. J. Pharmacokinet. Biopharm. 6, 165–175. doi: 10.1007/BF01117450
Yeh, C.-F., Soininen, J., Teittinen, A., and Wang, J. (2019). Elevational patterns and hierarchical determinants of biodiversity across microbial taxonomic scales. Mol. Ecol. 28, 86–99. doi: 10.1111/mec.14935
Yokokawa, T., De Corte, D., Sintes, E., and Herndl, G. J. (2010). Spatial patterns of bacterial abundance, activity and community composition in relation to water masses in the eastern Mediterranean Sea. Aquat. Microb. Ecol. 59, 185–195. doi: 10.3354/ame01393
Yu, Z., Yang, J., Amalfitano, S., Yu, X. Q., and Liu, L. M. (2014). Effects of water stratification and mixing on microbial community structure in a subtropical deep reservoir. Sci. Rep. 4, 1–7. doi: 10.1038/srep05821
Zhang, E. L., Cao, Y. M., Langdon, P., Wang, Q., Shen, J., and Yang, X. D. (2013). Within-lake variability of subfossil chironomid assemblage in a large, deep subtropical lake (Lugu lake, Southwest China). J. Limnol. 72, 117–126. doi: 10.4081/jlimol.2013.e10
Zhang, J., Yang, Y., Zhao, L., Li, Y., Xie, S., and Liu, Y. (2015). Distribution of sediment bacterial and archaeal communities in plateau freshwater lakes. Appl. Microbiol. Biotechnol. 99, 3291–3302. doi: 10.1007/s00253-014-6262-x
Zhang, L., Zhao, T. T., Shen, T. T., and Gao, G. (2019). Seasonal and spatial variation in the sediment bacterial community and diversity of Lake Bosten, China. J. Basic Microbiol. 59, 224–233. doi: 10.1002/jobm.201800452
Zhou, J. Z., Bruns, M. A., and Tiedje, J. M. (1996). DNA recovery from soils of diverse composition. Appl. Environ. Microbiol. 62, 316–322.
Zintzen, V., Anderson, M. J., Roberts, C. D., Harvey, E. S., and Stewart, A. L. (2017). Effects of latitude and depth on the beta diversity of New Zealand fish communities. Sci. Rep. 7, 1–10. doi: 10.1038/s41598-017-08427-7
Keywords: bacteria, phyla, relative abundance, water depth, biotic effects, abiotic effects
Citation: Wu K, Zhao W, Wang Q, Yang X, Zhu L, Shen J, Cheng X and Wang J (2019) The Relative Abundance of Benthic Bacterial Phyla Along a Water-Depth Gradient in a Plateau Lake: Physical, Chemical, and Biotic Drivers. Front. Microbiol. 10:1521. doi: 10.3389/fmicb.2019.01521
Edited by:
Haihan Zhang, Xi’an University of Architecture and Technology, ChinaReviewed by:
Jie Wang, China Agricultural University, ChinaMan Kit Cheung, The Chinese University of Hong Kong, China
Copyright © 2019 Wu, Zhao, Wang, Yang, Zhu, Shen, Cheng and Wang. This is an open-access article distributed under the terms of the Creative Commons Attribution License (CC BY). The use, distribution or reproduction in other forums is permitted, provided the original author(s) and the copyright owner(s) are credited and that the original publication in this journal is cited, in accordance with accepted academic practice. No use, distribution or reproduction is permitted which does not comply with these terms.
*Correspondence: Xiaoying Cheng, chengxiaoysytu@163.com; Jianjun Wang, jjwang@niglas.ac.cn
†These authors have contributed equally to this work