- 1College of Pharmacy, Sahmyook University, Seoul, South Korea
- 2College of Pharmacy, Chungbuk National University, Cheongju, South Korea
- 3College of Animal Biotechnology and Resource, Sahmyook University, Seoul, South Korea
Dysbiosis of the gut microbiota is a contributing factor for obesity-related metabolic diseases such as hyperglycemia and hyperlipidemia. Pharmacotherapy for metabolic diseases involves the modulation of gut microbiota, which is suggested to be a potential therapeutic target. In this study, the modulation of gut microbiota by statins (cholesterol-lowering drugs: atorvastatin and rosuvastatin) was investigated in an aged mouse model of high-fat diet-induced obesity, and the association between gut microbiota and immune responses was described. Atorvastatin and rosuvastatin significantly increased the abundance of the genera Bacteroides, Butyricimonas, and Mucispirillum. Moreover, the abundance of these genera was correlated with the inflammatory response, including levels of IL-1β and TGFβ1 in the ileum. In addition, oral fecal microbiota transplantation with fecal material collected from rosuvastatin-treated mouse groups improved hyperglycemia. From these results, the effect of statins on metabolic improvements could be explained by altered gut microbiota. Our findings suggest that the modulation of gut microbiota by statins has an important role in the therapeutic actions of these drugs.
Introduction
Obesity-related metabolic diseases including hyperlipidemia, hypertension, and type 2 diabetes (T2D) are prevalent, global health burdens (Wang et al., 2008). Lifestyle, including a lack of physical exercise and a Westernized diet, is a main contributing factor for the prevalence of metabolic diseases (Lee S.E. et al., 2018). Metabolic diseases are associated with an increased mortality risk in the elderly population; in particular, hyperlipidemia is an important risk factor for cardiovascular disease (Isomaa et al., 2001; Felix-Redondo et al., 2013).
Recently, American College of Cardiology/American Heart Association (ACC/AHA) recommended statins as a first-line treatment of hyperlipidemia, and statins are currently the most widely used treatment for hyperlipidemia (Safeer and Lacivita, 2000; Stone et al., 2014). Statins work by inhibiting 3-hydroxy-3-methylglutaryl-coenzyme A (HMG-CoA) reductase, an important enzyme involved in the cholesterol synthesis pathway (Stancu and Sima, 2001). Moreover, statins induce the expression of low-density lipoprotein (LDL) receptors and promote the removal of LDL cholesterol from the blood (Young and Fong, 2012). Furthermore, statins have been reported to have anti-inflammatory and immunomodulatory effects (Lee et al., 2016).
Gut microbiota is an important environmental factor for the regulation of energy homeostasis and immune system (Wu and Wu, 2012; Sanz et al., 2015). Modulation of gut microbiota improves the outcome of various diseases, including metabolic syndrome and inflammatory diseases, and influences pharmacotherapy (Wilson and Nicholson, 2009; Karkman et al., 2017). Recently, various interventions such as dietary supplement (Lee and Ko, 2016; Talsness et al., 2017), prebiotics or probiotics (Delzenne et al., 2011; Markowiak and Slizewska, 2017), and drugs (Shin et al., 2013; Lee and Ko, 2014) were reported to alter the composition of gut microbiota, which has an important role in the alleviation of metabolic diseases. In recent studies, statin therapy was found to affect the composition of gut microbiota (Khan et al., 2018; Liu et al., 2018). Nolan et al. (2017) showed that rosuvastatin influenced gut microbiota and significantly increased the abundance of the family Lachnospiraceae, and the genera Rikenella and Coprococcus in HFD-fed C57BL/6 mice.
The objectives of this study were (1) to identify the effects of statins on gut microbiota and metabolic improvements and (2) to investigate the correlation between significant changes in microbiota induced by statins and inflammatory regulation.
Materials and Methods
Animals and Experimental Protocol
Male 4-week-old C57BL/6N mice were purchased from Samtako Co., Ltd (Osan, South Korea) and acclimated to laboratory conditions for 1 week, in which the animals were housed with free access to water and food in a temperature–humidity-controlled animal facility under a 12-h light–dark cycle at 22 ± 2°C and 55 ± 5% humidity. Mice were fed either a 45% kcal high-fat diet (HFD) (FeedLab, Inc., Guri, South Korea) to induce metabolic disorders such as obesity, hyperglycemia, and hyperlipidemia, or a regular diet (RD) (Purina Korea, Inc., Seoul, South Korea) for 39 weeks. Atorvastatin (HFD-Ator: 10 mg/kg of body weight, n = 6) or rosuvastatin (HFD-Rosu: 3 mg/kg of body weight, n = 6) was administered every day during the HFD for the last 16 weeks. A RD-fed mice group (n = 6) and a HFD-fed mice group (n = 6) were included as normal and disease controls, respectively. All experimental procedures were performed in accordance with ethical guidelines issued by the Institutional Animal Care and Use Committee (IACUC) of Sahmyook University for the care and use of laboratory animals (SYUIACUC 2015001).
Metabolic Measurements
Body weight and serum glucose levels in mice were monitored every 2nd week. Mice were fasted overnight (12 h), blood samples were taken from a tail cut, and serum glucose levels were measured using the Accu-Chek Performa system (Roche, Switzerland). Intraperitoneal glucose tolerance testing (IPGTT) was performed 16 weeks after statin (Atorvastatin; Ator, Rosuvastatin; Rosu) administration. After overnight fasting (12 h), mice were intraperitoneally injected with glucose solution [2 g/kg of body weight, in phosphate-buffered saline (PBS)] and blood samples were obtained from the tail vein 0, 30, 60, 90, and 120 min after glucose injection. At the end of the experimental period, mice were sacrificed under ether anesthesia and blood was collected via cardiac puncture. Blood sample were centrifuged at 10,000 rpm for 5 min to isolate serum. Serum was prepared to determine levels of total cholesterol, LDL, apolipoprotein A-1 (ApoA-1), and apolipoprotein B (ApoB) using a biochemical analyzer (AU480, Beckman Coulter, United States).
Immunomodulatory Biomarkers
Ileum tissue from each group were immediately frozen in liquid nitrogen and stored at -70°C to determine levels of gene transcripts. The expression of interleukin-1β (IL-1β; forward primer: 5′-CAGGATGAGGACATGACACC-3′, reverse primer: 5′-CTCTGCAGACTCAAACTCCAC-3′), interleukin-6 (IL-6; forward primer: 5′-GTACTCCAGAAGACCAGAGC-3′, reverse primer: 5′-TGC TGG TGA CAA CCA CGG CC-3′), transforming growth factor β 1 (TGFβ1; forward primer: 5′-GCGGACTACTATGCTAAAGAGG-3′, reverse primer: 5′- GTAGAGTTCCACATGTTGCTCC-3′), interleukin-4 (IL-4; forward primer: 5′- GAGCCATATCCACGGATGCGACAA-3′, reverse primer: 5′- CATGGTGGCTCAGTACTACGAGTA-3′), and interleukin-10 (IL-10; forward primer: 5′-TGGCCACACTTGAGAGCTGC-3′, reverse primer: 5′-TTCAGGGATGAAGCGGCTGG-3′) was investigated in ileum tissue. Total RNA was extracted using a RiboExTM (GeneAll, Korea). RNA was then quantified by reading the absorbance at 260 nm. cDNA was synthesized using a HyperScriptTM RT premix (GeneAll, Korea). To quantify the level of mRNA expression, SYBR® Green PCR Master Mix (Applied Biosystems, United States) and a StepOnePlusTM real-time PCR system (Applied Biosystems, United States) were used. GAPDH was used as an internal control.
Gut Microbiota Analysis
Total DNA was extracted using the PowerSoil DNA Isolation Kit (MO BIO Laboratories, Inc., United States) from cecum samples, which included fecal materials. Partial sequences of 16S rRNA genes were amplified based on the 16S rRNA amplification protocol from the Earth Microbiome Project (Gilbert et al., 2010). 16S rRNA genes were amplified using the 515F/806R primer set, which includes an adapter sequence for amplification of the V4 region (515F forward primer: 5′-TCG TCG GCA GCG TCA GAT GTG TAT AAG AGA CAG GTG CCA GCM GCC GCG GTA A-3′; 806R reverse primer: 5′-GTC TCG TGG GCT CGG AGA TGT GTA TAA GAG ACA GGG ACT ACH VGG GTW TCT AAT-3′). To attach the dual indices and adapter to amplified polymerase chain reaction (PCR) products, an index PCR was additionally performed using an AmpONETM α-Pfu DNA polymerase (GeneAll, Korea) and Nextera® XT Index Kit v2 (Illumina, United States). PCR products after amplification and attachment were purified using the ExpinTM PCR SV (GeneAll, Korea). Partial bacterial 16S rRNA genes were amplified using the MiSeq Reagent Kit V3 (600 cycles) and MiSeq platform (Illumina, United States) at KoBioLabs, Inc.
Prior to analysis of 16S rRNA sequences, BCL files were converted into raw FASTQ files including read1, index, and read2 sequences using CASAVA-1.8.2 (Illumina). After preprocessing (quality filtering and trimming steps using FASTX-Toolkit) (Gordon and Hannon, 2010), sequences were assigned to operational taxonomic units (OTUs, 97% identity), and representative sequences were selected using QIIME 1.7.0 software (Caporaso et al., 2010). Next, taxonomic composition, alpha diversity (rarefaction curve and Shannon index of bacterial diversity) and beta diversity (PCoA of UniFrac distances) were analyzed. LDA effect size (LEfSe) was used to estimate taxonomic abundance and characterize differences between groups (Segata et al., 2011). Hierarchical clustering of the top ten most abundant bacterial genera by groups was performed using Spearman’s rank correlation, and a heat map was generated using MultiExperiment Viewer (MEV) software (v4.8.1). Next-generation sequencing (NGS) data are available at1.
The relative abundances of four bacterial genera were confirmed using SYBR® Green PCR Master Mix (Applied Biosystems, United States) and a StepOnePlusTM real-time PCR system (Applied Biosystems, United States). Genus-specific primer sets for Eubacteria (UniF340; forward primer: 5′-ACTCCTACGGGAGGCAGCAGT-3′, UniR514; reverse primer: 5′-ATTACCGCGGCTGCTCCG-3′), Akkermansia (AM1; forward primer: 5′-CAGCACGTGAAGGTGGGGAC-3′, AM2; reverse primer: 5′-CCTTGCGGTTGGCTTCAGAT-3′), Bacteroides (AllBac296f; forward primer: 5′-GAGAGGAA GGTCCCCCAC-3′, AllBac412r; reverse primer: 5′-CGCTAC TTGGCTGGTTCAG-3′), Butyricimonas (Buty1f; forward primer: 5′-GGTGAGTAACACGTGTGCAAC-3′, Buty1r; reverse primer: 5′-TACCCCGCCAACTACCTAATG-3′), and Mucispirillum (MucisF; forward primer: 5′-CGTTTGCAAGAA TGAAACTCAAA-3′, MucisR; reverse primer: 5′-CACAGCATTATCTCTAACGCCTT-3′) were used for amplification (Layton et al., 2006; Collado et al., 2007; Cahenzli et al., 2013).
Fecal Microbiota Transplantation (FMT)
Fecal material (0.1 g) from rosuvastatin-treated mice (HFD-Rosu group) was pooled in 1 mL of PBS. Mice were allowed to drink water containing penicillin G procaine (2,000 IU/L) and streptomycin (2.5 mg/L) for 5 days prior to FMT. After centrifugation at 2,000 g for 2 min, 500 μL of supernatant was administered to antibiotic-treated mice fed a HFD for 48 weeks (HFD-fRosu, n = 6) via oral gavage. Metabolic profiles, inflammatory cytokines and bacterial abundances were analyzed and compared between the RD (n = 5) and HFD (n = 4) groups 4 weeks after FMT.
Statistical Analysis
Data for each group are presented as the mean ± standard error of mean (SEM). Relative abundance was analyzed using LEfSe based on the Kruskal–Wallis and Wilcoxon tests, and significance was defined at P < 0.05 (alpha value = 0.05). The logarithmic LDA score threshold was set at 3.0. To quantify in vivo mRNA levels relative to an internal control (GAPDH), the 2–Δ Δ Ct relative quantification method (ΔΔCt = (Ct.Target − Ct.β–actin)Group1 − (Ct.Target − Ct.β–actin)Group2) was used. Statistical significance was assessed by one-way analysis of variance (ANOVA), followed by Duncan’s post hoc test with agricolae package in RStudio. All statistical analyses were performed using RStudio. A P value < 0.05 was considered to indicate statistical significance.
Results
Effects of Statins on Metabolic Improvements
As expected, both atorvastatin and rosuvastatin significantly reduced total cholesterol levels (Figure 1). Moreover, statins significantly reduced serum glucose levels and tended to improve glucose tolerance (Figure 1). In addition, statins decreased levels of ApoA-1 but not ApoB. There was no significant change in body weight and levels of LDL in response to statins compared to the corresponding values in the HFD group.
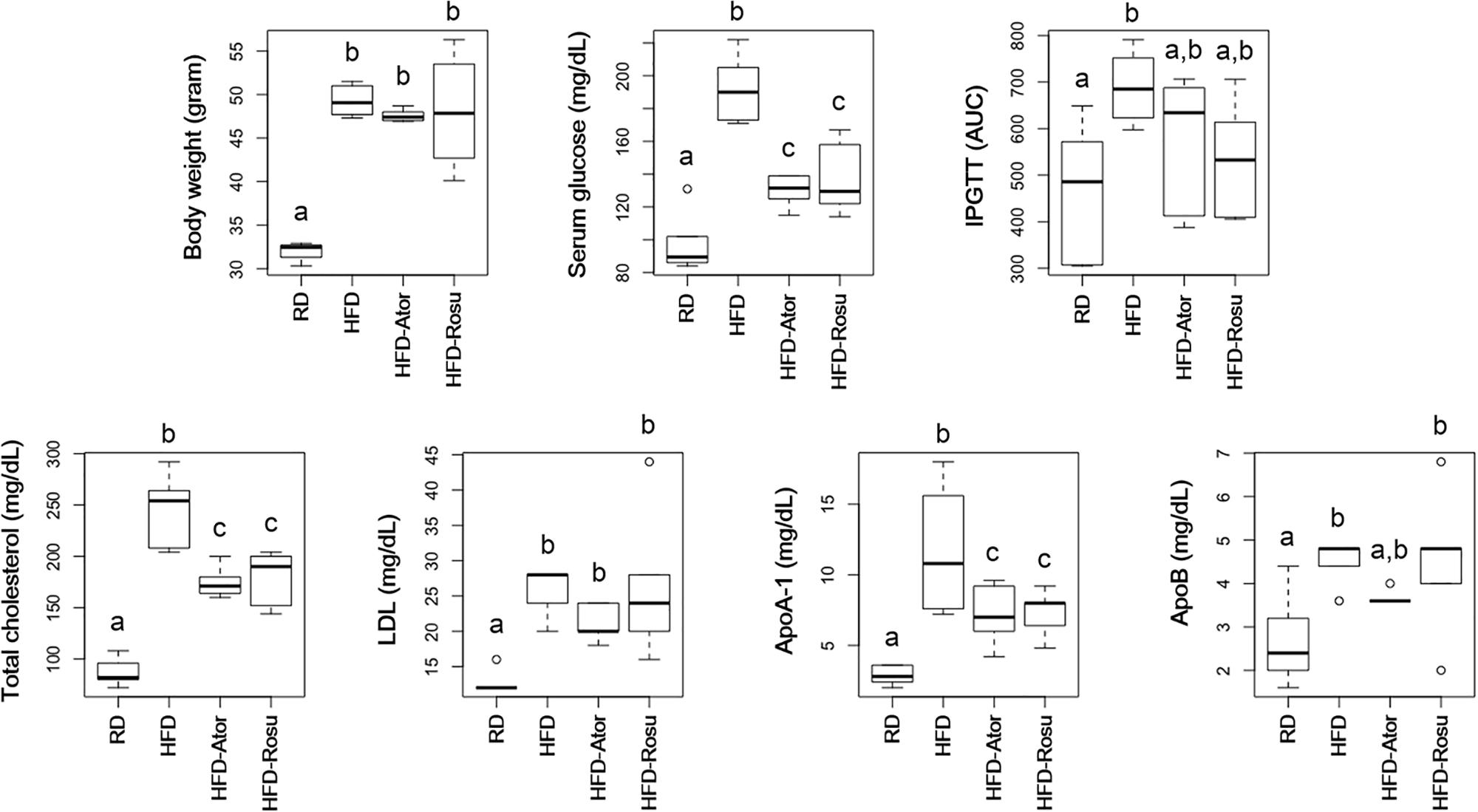
Figure 1. Effect of statins on body weight, serum glucose, intraperitoneal glucose tolerance test (IPGTT), total cholesterol, LDL, ApoA-1, and ApoB. Five-week-old C57BL/6N mice were fed a HFD for 39 weeks to induce metabolic disorders, and then statins were administered daily for 16 weeks. RD: regular diet (n = 6); HFD: high-fat-diet (n = 6); HFD-Ator: atorvastatin administration during HFD feeding (n = 6); HFD-Rosu: rosuvastatin administration during HFD feeding (n = 6). Different superscript letters indicate significant differences (P < 0.05) according to Duncan’s post hoc test following ANOVA.
Effects of Statins on the Gut Microbiota
A total of 334,356 sequences were generated from 24 samples. An average of 13,932 ± 6,137 sequences were recovered per sample and used for comparative analyses. Figures 2A,B show the differences in microbial diversity between the RD, HFD, HFD-Ator, and HFD-Rosu groups. The alpha diversities of gut microbiota analyzed using Chao 1 richness and Shannon index did not reveal any significant difference between the groups (Figure 2A). The Principal coordinates analysis (PCoA) of UniFrac distances showed a separation between the RD, HFD, HFD-Ator, and HFD-Rosu groups, which was clearly classified in the unweighted PCoA (Figure 2B).
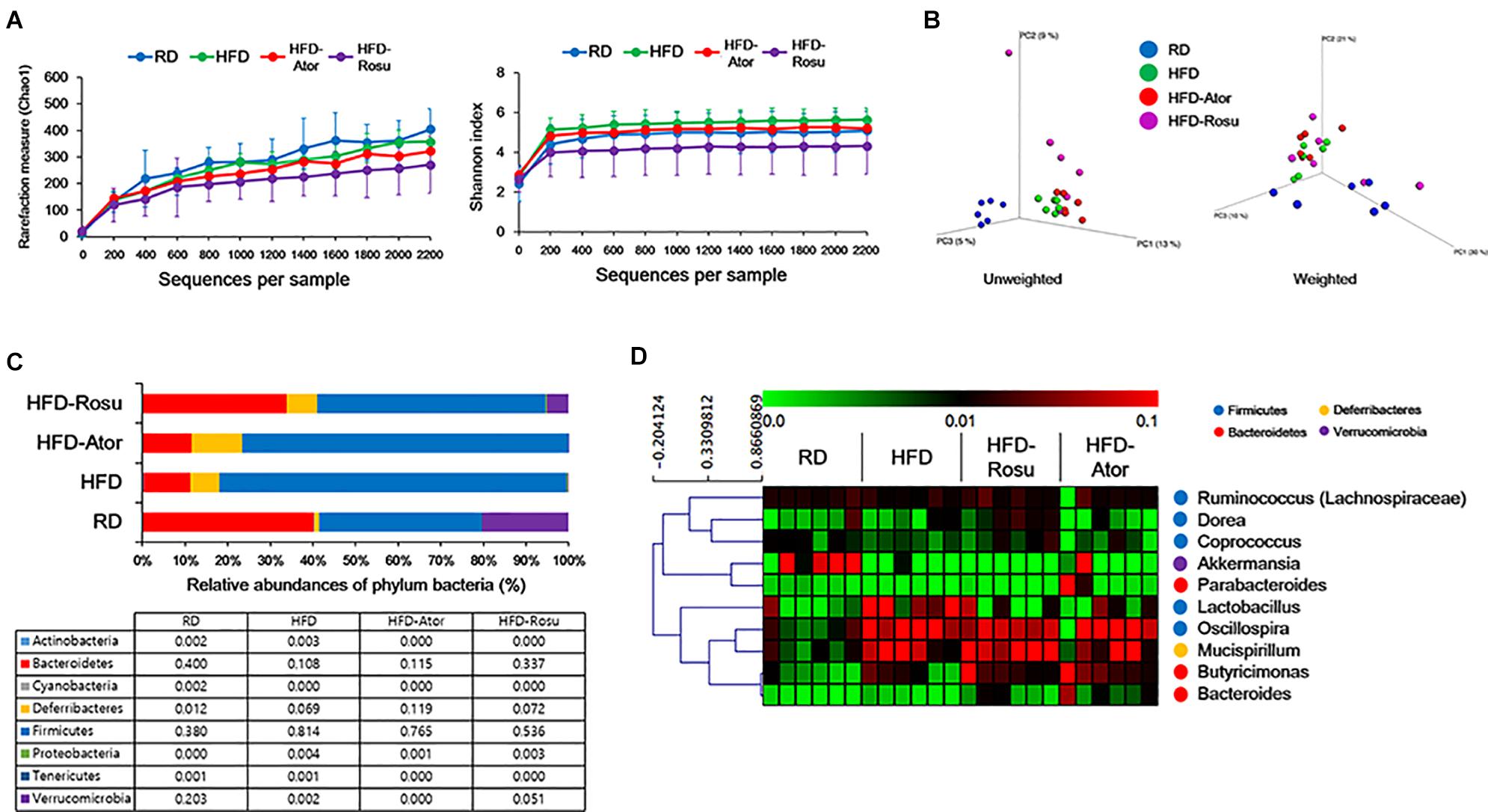
Figure 2. Microbial diversity according to diet and statin therapy. (A) Rarefaction curve and Shannon index of bacterial diversity. (B) PCoA of UniFrac distances. (C) Bacterial classification at the phylum level. Table value indicates relative abundance of phylum level. (D) Heatmap of average linkage hierarchical clustering of the top 10 most abundant bacterial genera using Spearman’s rank correlation. A heat map was generated using MultiExperiment Viewer (MEV) software (v4.8.1). Rows represent bacterial genera, and columns represent samples.
Effects of Statins and Diet on the Composition of the Gut Microbiota
The phyla Bacteroidetes and Firmicutes from the RD group consisted of 40.0 ± 19.6 [average of relative abundance (%) ± standard deviation] and 38.0 ± 16.0% of gut microbiota, respectively, and the Firmicutes/Bacteroidetes (F/B) ratio was 1.3 ± 1.1%. The abundance of Bacteroidetes (10.8 ± 9.3%) decreased in the HFD group and that of Firmicutes (81.4 ± 13.4%) increased compared to the corresponding abundances in the RD group. The F/B ratio was 24.5 ± 28.6%. In contrast, the abundance of Bacteroidetes and Firmicutes from the HFD-Ator group was 11.5 ± 9.9% and 76.5 ± 10.6%, respectively; the F/B ratio was 10.6 ± 6.4%; and the abundance of Bacteroidetes and Firmicutes in the HFD-Rosu group was 33.7 ± 29.1% and 53.6 ± 24.9%, respectively. The F/B ratio was 2.8 ± 1.9%. These results indicate that rosuvastatin effectively restores the altered gut microbiota by HFD compared to atorvastatin. In addition, the abundance of Deferribacteres in the HFD-Ator group was 11.9 ± 4.4%, which was greater than that in the RD (1.2 ± 0.9%) and HFD (6.9 ± 4.4%) groups (Figure 2C). Relative abundances of Bacteroidetes, Firmicutes, and Deferribacteres in the HFD-Ator and HFD-Rosu group were not significantly different compared to the HFD group.
Among the top 10 most abundant bacterial genera found in the four groups, Bacteroides and Butyricimonas of the phylum Bacteroidetes, Oscillospira of the phylum Firmicutes, and Mucispirillum of the phylum Deferribacteres were closely clustered (Figure 2D). Their abundance was enriched in the HFD-Ator and HFD-Rosu groups compared to that in the RD and HFD groups, accounting for 24.3 ± 5.6% and 27.8 ± 14.3% of the total identified bacterial OTUs, respectively (Figure 2D). In the LEfSe analysis, the abundances of Anaerotruncus, Bacteroides, Butyricimonas, Dorea, Mucispirillum, and Turicibacter were significantly greater in the HFD-Ator group than in the RD and HFD groups, and the abundances of Bacteroides, Butyricimonas, Clostridium, and Mucispirillum were significantly greater in the HFD-Rosu group. The abundances of Bacteroides, Butyricimonas, and Mucispirillum overlapped between the HFD-Ator and the HFD-Rosu groups (Figures 3A,B). Moreover, in comparison between the HFD-Ator and the HFD-Rosu groups, the abundances of Turicibacter, Dorea, and Ruminococcus were significantly greater in the HFD-Ator group than in the HFD-Rosu group, and the abundance of Clostridium was significantly greater in the HFD-Rosu group than in the HFD-Ator group (Figure 3C).
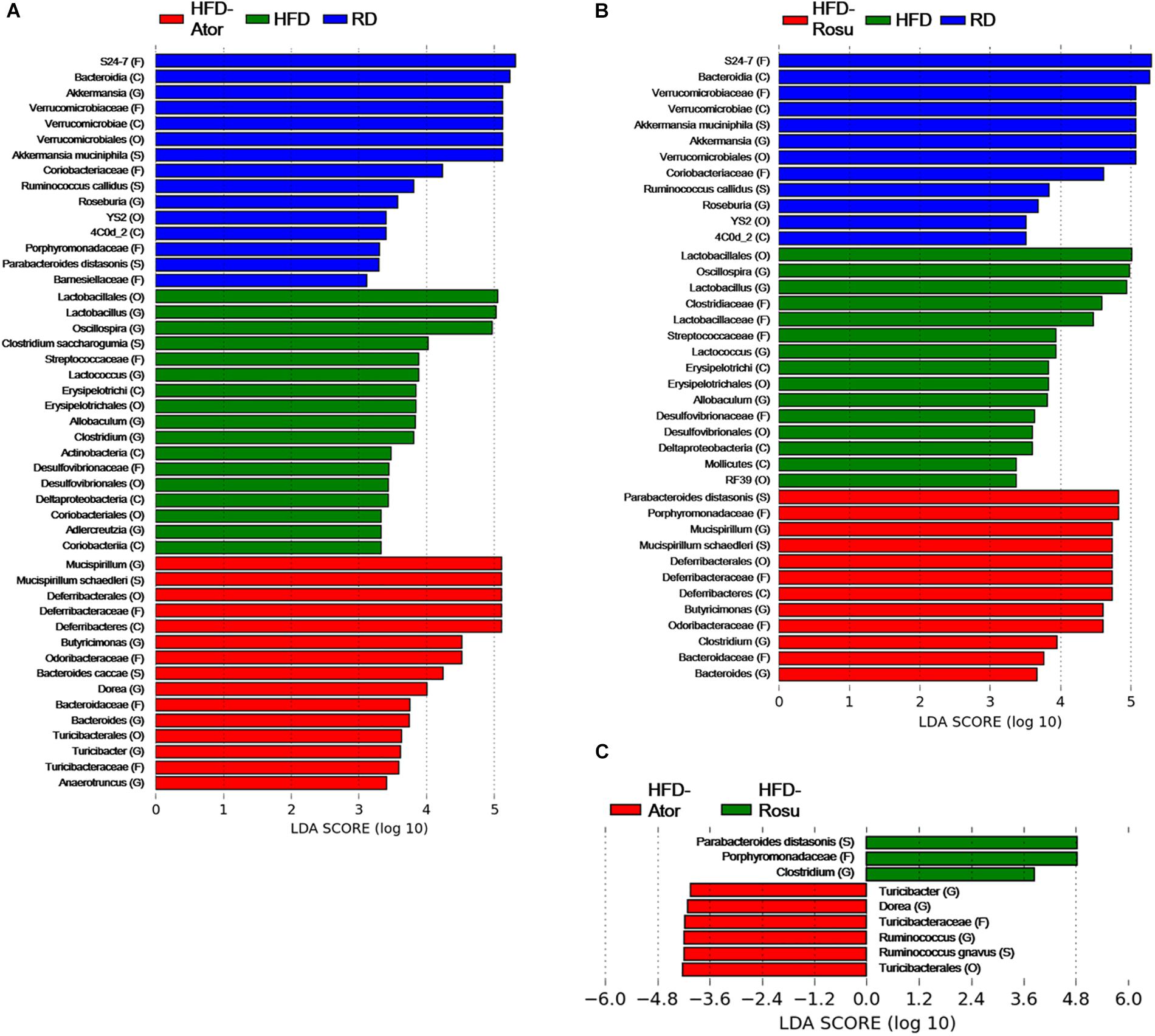
Figure 3. Significant differences in bacterial abundance according to diet and statin therapy. (A) Comparison between RD, HFD, and HFD-Ator groups. (B) Comparison between RD, HFD, and HFD-Rosu groups. (C) Comparison between HFD-Ator and HFD-Rosu groups. Significant differences were identified by LEfSe analysis at P < 0.05 by the Kruskal–Wallis test (among classes) and Wilcoxon test (between subclasses). The threshold logarithmic LDA score was 3.0. Three and four bacterial families were identified by atorvastatin and rosuvastatin, respectively. Among them, Odoribacteraceae, Deferribacteraceae, and Bacteroidaceae overlapped. At the genus level, Bacteroides, Butyricimonas, and Mucispirillum were increased by both atorvastatin and rosuvastatin.
Among them, Odoribacteraceae, Deferribacteraceae, and Bacteroidaceae overlapped. At the genus level, Bacteroides, Butyricimonas, and Mucispirillum were increased by both atorvastatin and rosuvastatin.
Effects of Statins on Inflammatory Cytokines
TGFβ1 expression increased significantly in the HFD-Ator and HFD-Rosu groups compared to that in the HFD group, whereas IL-1β expression decreased with statin treatments. There was no significant change in the expression of IL-4, IL-6, and IL-10 by statins compared to that in the HFD group (Figure 4A). In addition, the expression of TGFβ1 and IL-1β was correlated with increased abundance of the Clostridium, Dorea, Mucispirillum, Butyricimonas, Bacteroides, Anaerotruncus, and Turicibacter by statins. Among them, the positive correlation between TGFβ1 and the abundance of Dorea and the negative correlation between IL-1β and the abundance of Dorea and Mucispirillum were significant in the HFD-Ator group (Figure 4B).
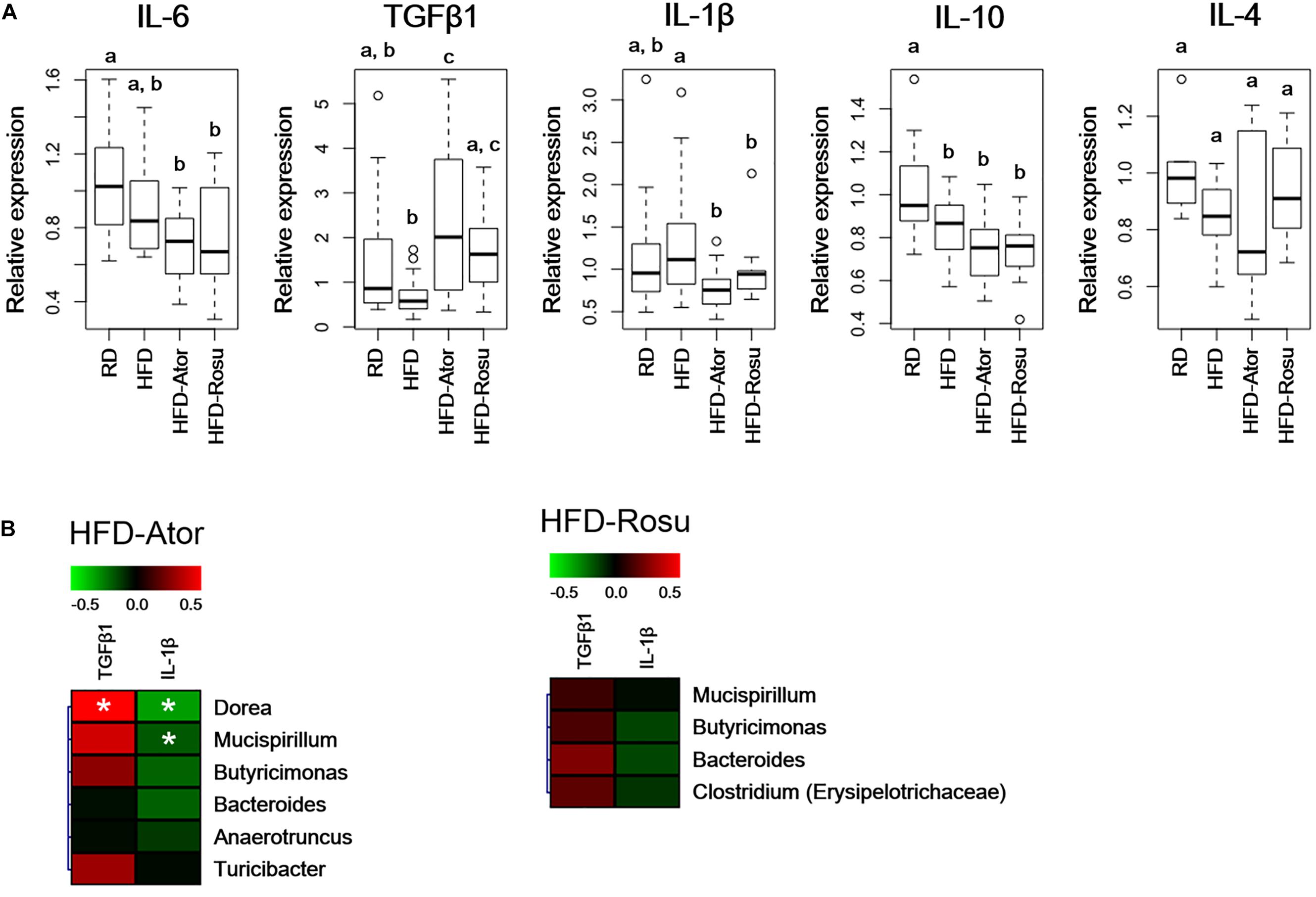
Figure 4. Relative expression levels of inflammatory cytokines in the ileum. Relative mRNA levels of cytokines were analyzed by qPCR. (A) Relative expression levels of IL-6, TGFβ1, IL-1β, IL-10, and IL-4. Different superscript letters indicate significant differences (P < 0.05) according to Duncan’s post hoc test following ANOVA. (B) Correlations between immunological biomarkers and the abundance of bacterial genera in the HFD-Ator (n = 6) and HFD-Rosu group (n = 6). Statistical significance (Spearman’s correlation coefficient; P < 0.05).
Effects of Fecal Material Transplantation on Metabolic Improvements
Both atorvastatin and rosuvastatin significantly improved the metabolic disorders, and microbial diversity of the rosuvastatin-treated mice group was more different compared to the HFD group than the atorvastatin-treated mice group based on beta diversity. Therefore, fecal material transplantation (FMT) was performed using fecal material from rosuvastatin-treated mice, which significantly improved serum glucose levels and glucose tolerance with the HFD (Figure 5A). However, body weight and total cholesterol and LDL levels were not improved by FMT. The abundances of Bacteroides, Butyricimonas, and Mucispirillum were 2.2 ± 4.0%, 0.04 ± 0.02%, and 0.06 ± 0.03% in the HFD-fRosu group, respectively. Among them, the abundance of Bacteroides was significantly decreased and that of Butyricimonas was significantly increased after FMT compared to that in the HFD group (Figure 5B). TGFβ1 expression increased significantly, whereas IL-1β expression decreased significantly, in the HFD-fRosu group compared to that in the HFD group (Figure 5C).
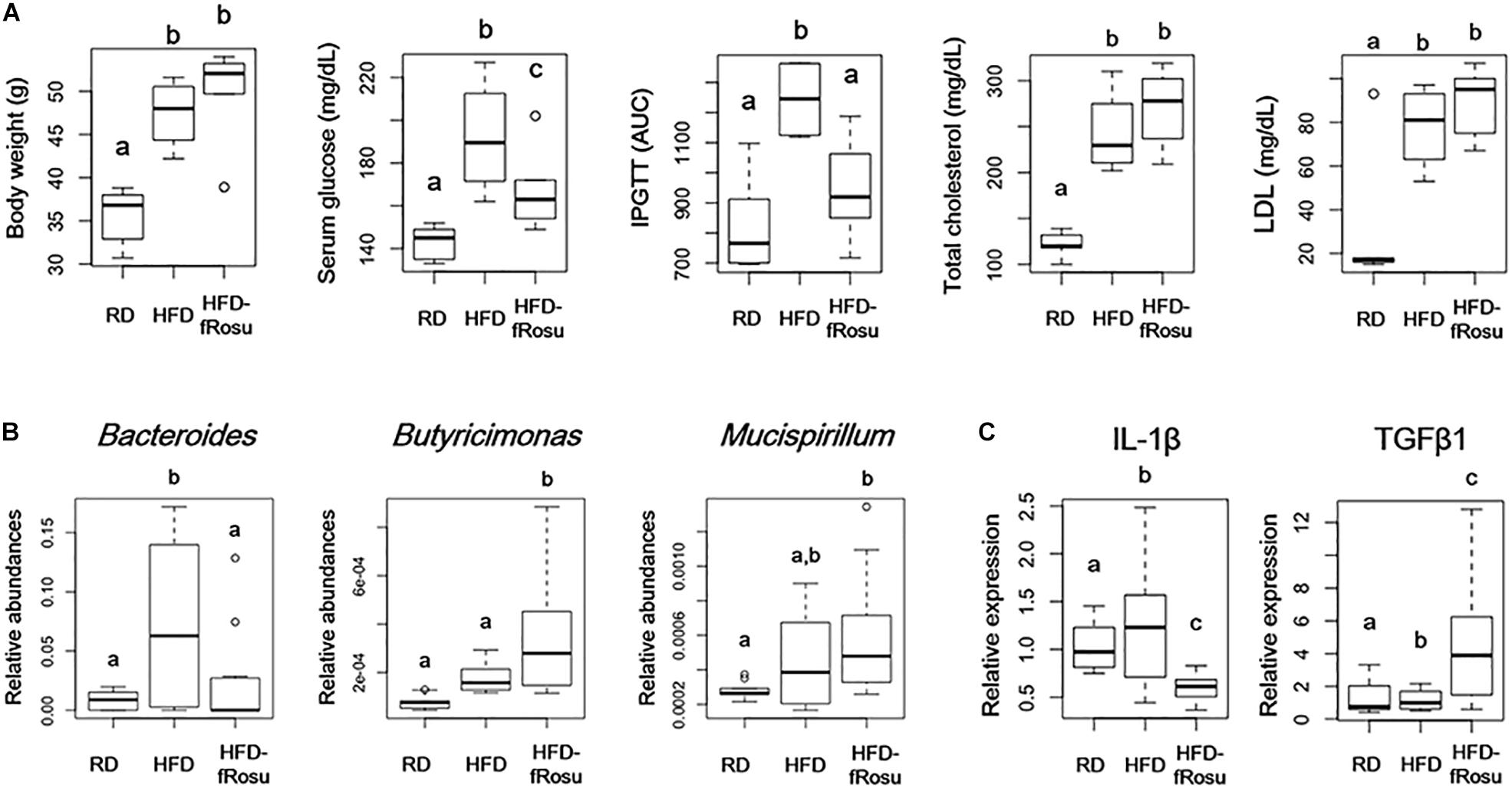
Figure 5. Effect of fecal microbiota transplantation (FMT) on metabolic parameters, gut microbiota, and inflammatory cytokines. Six-week-old mice were fed a HFD for 43 weeks and then inoculated orally with pooled fecal material from the HFD-Rosu group for 4 weeks (HFD-fRosu, n = 6). All parameters were compared to those in the RD (n = 5) and HFD (n = 4) groups. (A) The effect of FMT on body weight, serum glucose, IPGTT, total cholesterol, and LDL. (B) Abundance of Bacteroides, Butyricimonas, and Mucispirillum. Relative abundance was analyzed using qPCR. (C) Relative expression levels of IL-1β and TGFβ1 in the ileum. Relative mRNA levels were analyzed using qPCR. Different superscript letters indicate significant differences (P < 0.05) according to Duncan’s post hoc test following ANOVA.
Discussion
Modulation of gut microbiota has an impact on metabolic improvements in obesity and T2D (Clarke et al., 2012; Mardinoglu et al., 2016). Recent studies have revealed that certain pharmacotherapies can improve metabolic diseases through specific compositional changes in gut microbiota (Shin et al., 2013; Lee and Ko, 2014). Statins are cholesterol-lowering drugs, which also affect glucose tolerance in animal models. The characteristics of gut microbiota in response to statins have not yet been fully investigated in metabolic diseases (Caparros-Martin et al., 2017; Khan et al., 2018). In this study, changes in gut microbiota in response to atorvastatin and rosuvastatin were observed in mice with HFD-induced obesity, and the abundances of Bacteroides, Butyricimonas, and Mucispirillum were all remarkable. Moreover, FMT with fecal materials from the HFD-Rosu group has an impact on hyperglycemia improvement, which may be related to the abundance of Butyricimonas.
Therapy with atorvastatin and rosuvastatin both induced significant changes in the gut microbiota during the HFD. In a meta-analysis comparing the two statins, similar pharmacokinetics, efficacy, and side effects were observed, which may be associated with the similar changes in gut microbiota induced by atorvastatin and rosuvastatin (Abbas et al., 2012). Nevertheless, differences in gut bacterial composition were observed with atorvastatin and rosuvastatin, which may be caused by differences in chemical structure and the effects on hyperlipidemia and glucose metabolism. Atorvastatin is lipophilic and rosuvastatin is hydrophilic due to the side chain of the methane sulfonamide group, and across the dose range used, rosuvastatin was significantly more effective in decreasing cholesterol than atorvastatin. However, it is unclear how those differences influence gut microbiota.
Alterations in gut microbiota in recent studies were not consistent with the findings of the present study (Nolan et al., 2017; Khan et al., 2018), which may be due to two major differences in the experimental method used. The first is due to differences in the target region of the 16S rRNA gene. In NGS analysis, the target region of the 16S rRNA gene impacts the microbiota results (Rintala et al., 2017). Results of this study are based on PCR amplification and sequencing targeting V4 region, and a different short variable region was targeted in different previous studies. Consequently, that affected the universality of the NGS result, which is why amplification of multiple variable regions is recommended to increase the universality and resolution (Fuks et al., 2018). Second, although both studies were performed using C57BL/6 mice, the gender and age of the mice were different. The effect of medication on the gut microbiota is influenced by gender (Lee and Ko, 2014). Liu et al. (2018) reported that variation in gut microbiota was associated with the efficacy of rosuvastatin therapy in a clinical study, with differences in gut microbiota observed by age and gender (Liu et al., 2018). In the present study, statins were administered for 16 weeks in 29-week-old aged male mice; therefore, our microbiota results may reflect the characteristics of gut microbiota in response to statin therapy. A decrease in the F/B ratio was observed with the metabolic improvement induced by statins treatment. Bacteroidetes and Firmicutes are the major bacterial phyla, which represent most bacteria in the gut, and their ratio is related with obesity-related metabolic disorders (Ley et al., 2006; Chakraborti, 2015; Louis et al., 2016). Moreover, in a recent study, statin therapy with pravastatin and atorvastatin resulted in gut dysbiosis in HFD-fed female C57BL/6 mice, which was associated with adverse effects induced by statins intolerance, including myopathy and T2D (Caparros-Martin et al., 2017). The results of recent studies suggest that statins influence changes in gut microbiota and that various factors affect the composition of gut microbiota, including age, gender, and diet. Nevertheless, recent studies reported that statin therapy increased butyric acid-producing bacteria, including those belonging to the families Lachnospiraceae, Bacteroidaceae, and Prevotellaceae. The genera Bacteroides and Butyricimonas, which were increased in this study, are butyric acid-producing bacteria, and short-chain fatty acids (SCFAs) may play key roles in metabolic improvements, including hyperlipidemia and hyperglycemia.
SCFAs are produced from dietary fiber by intestinal bacteria, and this has beneficial effects on host energy metabolism (Den Besten et al., 2013). Among the SCFAs, acetic acid, propionic acid, and butyric acid are most abundant in the intestine, representing over 90% of the SCFAs present (Den Besten et al., 2013). A high abundance of Bacteroides spp. is characteristic of non-obese individuals and is caused by the releases of propionic acid and acetic acid from Bacteroides spp. (Chakraborti, 2015). Another SCFA, butyric acid, improves the outcome of insulin resistance and dyslipidemia (Gao et al., 2009). Several bacterial strains including Clostridium butyricum and Butyricicoccus pullicaecorum are representative butyric acid-producing anaerobic bacteria, which are involved in T2D and inflammatory bowel disease, respectively (Geirnaert et al., 2014; Jia et al., 2017). Additionally, the majority of members of the Prevotellaceae, Clostridiaceae, Ruminococcaceae, Lactobacillaceae, and Lachnospiraceae families are putative butyric acid producers and have anti-inflammatory properties (Esquivel-Elizondo et al., 2017). In the present study, Butyricimonas, a butyric acid-producing bacterium, may have played a key role in metabolic improvements induced by statins, especially in glucose metabolism. Moreover, FMT using fecal materials from the HFD-Rosu group resulted in improved glucose tolerance, and the high abundance of Butyricimonas was solely maintained. However, butyric acid was not investigated in this study, and the mechanisms through which Butyricimonas induce metabolic improvements are unknown. This limitation should be addressed in future studies.
Apart from butyric acid-producing bacteria, Mucispirillum, which inhabits the intestinal mucus layer, may have an important role in the metabolic improvements induced by statins. To date, few studies have discussed how Mucispirillum schaedleri are associated with intestinal inflammation (Loy et al., 2017). However, the role of Mucispirillum in lipid metabolism and inflammation is poorly understood. In the present study, Mucispirillum was more abundant in the HFD group than in the RD group, and was significantly increased by statins with a HFD. Moreover, the abundance of those bacteria was negatively correlated with pro-inflammatory cytokine and positively correlated with anti-inflammatory cytokines in the statin-treated HFD group. Conversely, based on genome analysis, M. schaedleri is not predicted to be a primary degrader of glycan from mucin. Instead, M. schaedleri is likely to utilize SCFAs for energy metabolism (Loy et al., 2017); those abundant in this study may be accompanied by SCFA-producing bacteria in response to statins.
Regulation of IL-1β and TGFβ expression may play a key role in the metabolic improvements associated with statin therapy, due to changes in gut microbiota. A previous study reported that statin therapy regulates inflammatory cytokines such as IL-1β, IL-6, and TNF-α (Lee et al., 2016). In the present study, IL-1β and TGFβ expression increased in the ileum, indicating that the regulation of IL-1β and TGFβ is associated with the effect of statins on the alleviation of hyperglycemia, hyperlipidemia, and inflammation. In the intestinal immune system, the pro-inflammatory cytokine IL-1β plays an important role in insulin resistance and hyperlipidemia (Rotter et al., 2003; Fentoglu et al., 2011). Especially, upregulation of IL-1β is a general feature underlying obesity-related insulin resistance, which increases intestinal epithelial tight junction permeability and inflammation (Al-Sadi and Ma, 2007). Intestinal permeability has been observed in dysbiosis by HFD, and changes in gut microbiota in response to statin therapy with a HFD played an important role in the downregulation of IL-1β expression and the improvement of downstream glucose control (Winer et al., 2016). Secretion of TGFβ, an anti-inflammatory mediator, occurs under normal physiological conditions in the intestine; statin therapy increased TGFβ expression (Porreca et al., 2002). TGFβ is a pleiotropic cytokine involved in various immune signaling pathways, including intestinal anti-inflammation (Letterio and Roberts, 1998). Above all, TGFβ signaling suppresses T-cell proliferation and activation through Treg differentiation, which is essential for intestinal homeostasis (Bauche and Marie, 2017). In intestinal immunity, TGFβ suppresses inflammatory responses through the canonical pathway in a mechanism mediated by the intracellular signaling proteins SMAD2/3 (Massague, 2012). Interestingly, TGFβ production is induced by gut microbiota, and Clostridium strains including Clostridium butyricum (or derived butyric acid) and Bacteroides fragilis were reported to induce TGFβ production and Treg differentiation (Round and Mazmanian, 2010; Kashiwagi et al., 2015; Hong et al., 2017; Goncalves et al., 2018). Therefore, the anti-inflammatory effects of statin therapy may be associated with the regulation of TGFβ expression in the intestine via the modulation of gut microbiota.
In this study, the effect of gut microbiota on metabolic improvements, especially anti-hyperglycemic, was shown to be induced by FMT. After FMT, the relative abundance of Butyricimonas increased significantly, compared to that in the HFD group, which may play a key role in the anti-hyperglycemic effect. In a previous study, the increased abundance of Butyricimonas and Bacteroides with metformin therapy was associated with metabolic improvements, including body weight, glucose tolerance, and lipid metabolism, and the administration of fecal material from metformin-treated mice had an effect on body weight change only, which was associated with the abundance of Bacteroides (Lee H. et al., 2018). Additionally, a recent study reported that Bacteroides prevented obesity and insulin resistance (Yang et al., 2017). Along with previous studies, the present study indicates that Butyricimonas may have a more important role in hyperglycemia than in obesity. Moreover, the regulation of IL-1β and TGFβ1 by FMT was consistent with the effects of statin therapy during HFD. FMT is a therapeutic option for inflammatory bowel disease induced by Clostridium difficile infection, and recent studies have reported metabolic improvements, including insulin sensitivity, with FMT (Kootte et al., 2017). However, the mechanism underlying the effect of FMT on metabolism is unclear, and neither criteria for donor selection nor optimal processes were established. In this study, the anti-hyperglycemic effect persisted for 1 month after FMT in antibiotic-treated mouse model, which provides information for successful FMT therapy in metabolic diseases treatment.
Conclusion
Statin therapy with atorvastatin and rosuvastatin significantly altered the gut microbiota during HFD in an aged obese mice model. The abundance of the genera Bacteroides, Butyricimonas, and Mucispirillum was significantly increased by statins, and this was related to hyperglycemia and hyperlipidemia. In particular, Butyricimonas may be related to the anti-hyperglycemic effect of statins. Furthermore, the downregulation of IL-1β and the upregulation of TGFβ1 by statins were significantly correlated with the abundance of those bacteria. These results suggest that the alterations in gut microbiota by statins may be one of therapeutic targets for the treatment of hyperglycemia.
Data Availability
The datasets generated for this study can be found in FigShare https://figshare.com/articles/NGS_data/9104477.
Ethics Statement
This study was approved by the Institutional Animal Care and Use Committee (IACUC) of Sahmyook University for the care and use of laboratory animals (SYUIACUC 2015001).
Author Contributions
HL, HK, YS, and KK contributed to the study conception and design. HL, JK, and JA performed by the experiments. HL and JA performed the data analysis and interpretation of the data. HL and JA contributed to the manuscript drafting. All authors have approved the final version of this manuscript.
Funding
This research was supported by the Korea Institute of Planning and Evaluation for Technology in Food, Agriculture, Forestry, and Fisheries, IPET (No. 314044-3).
Conflict of Interest Statement
The authors declare that the research was conducted in the absence of any commercial or financial relationships that could be construed as a potential conflict of interest.
Footnotes
References
Abbas, A., Milles, J., and Ramachandran, S. (2012). Rosuvastatin and atorvastatin: comparative effects on glucose metabolism in non-diabetic patients with dyslipidaemia. Clin. Med. Insights Endocrinol. Diabetes 5, 13–30. doi: 10.4137/CMED.S7591
Al-Sadi, R. M., and Ma, T. Y. (2007). IL-1beta causes an increase in intestinal epithelial tight junction permeability. J. Immunol. 178, 4641–4649. doi: 10.4049/jimmunol.178.7.4641
Bauche, D., and Marie, J. C. (2017). Transforming growth factor beta: a master regulator of the gut microbiota and immune cell interactions. Clin. Transl. Immunol. 6:e136. doi: 10.1038/cti.2017.9
Cahenzli, J., Koller, Y., Wyss, M., Geuking, M. B., and Mccoy, K. D. (2013). Intestinal microbial diversity during early-life colonization shapes long-term IgE levels. Cell Host Microbe 14, 559–570. doi: 10.1016/j.chom.2013.10.004
Caparros-Martin, J. A., Lareu, R. R., Ramsay, J. P., Peplies, J., Reen, F. J., Headlam, H. A., et al. (2017). Statin therapy causes gut dysbiosis in mice through a PXR-dependent mechanism. Microbiome 5:95. doi: 10.1186/s40168-017-0312-4
Caporaso, J. G., Kuczynski, J., Stombaugh, J., Bittinger, K., Bushman, F. D., Costello, E. K., et al. (2010). QIIME allows analysis of high-throughput community sequencing data. Nat. Methods 7, 335–336.
Chakraborti, C. K. (2015). New-found link between microbiota and obesity. World J. Gastrointest. Pathophysiol. 6, 110–119. doi: 10.4291/wjgp.v6.i4.110
Clarke, S. F., Murphy, E. F., Nilaweera, K., Ross, P. R., Shanahan, F., O’toole, P. W., et al. (2012). The gut microbiota and its relationship to diet and obesity: new insights. Gut Microbes 3, 186–202. doi: 10.4161/gmic.20168
Collado, M. C., Derrien, M., Isolauri, E., De Vos, W. M., and Salminen, S. (2007). Intestinal integrity and Akkermansia muciniphila, a mucin-degrading member of the intestinal microbiota present in infants, adults, and the elderly. Appl. Environ. Microbiol. 73, 7767–7770. doi: 10.1128/aem.01477-07
Delzenne, N. M., Neyrinck, A. M., Backhed, F., and Cani, P. D. (2011). Targeting gut microbiota in obesity: effects of prebiotics and probiotics. Nat. Rev. Endocrinol. 7, 639–646. doi: 10.1038/nrendo.2011.126
Den Besten, G., Van Eunen, K., Groen, A. K., Venema, K., Reijngoud, D. J., and Bakker, B. M. (2013). The role of short-chain fatty acids in the interplay between diet, gut microbiota, and host energy metabolism. J. Lipid Res. 54, 2325–2340. doi: 10.1194/jlr.R036012
Esquivel-Elizondo, S., Ilhan, Z. E., Garcia-Pena, E. I., and Krajmalnik-Brown, R. (2017). Insights into butyrate production in a controlled fermentation system via gene predictions. mSystems 2:e00051-17. doi: 10.1128/mSystems.00051-17
Felix-Redondo, F. J., Grau, M., and Fernandez-Berges, D. (2013). Cholesterol and cardiovascular disease in the elderly. Facts and gaps. Aging Dis. 4, 154–169.
Fentoglu, O., Koroglu, B. K., Hicyilmaz, H., Sert, T., Ozdem, M., Sutcu, R., et al. (2011). Pro-inflammatory cytokine levels in association between periodontal disease and hyperlipidaemia. J. Clin. Periodontol. 38, 8–16. doi: 10.1111/j.1600-051X.2010.01644.x
Fuks, G., Elgart, M., Amir, A., Zeisel, A., Turnbaugh, P. J., Soen, Y., et al. (2018). Combining 16S rRNA gene variable regions enables high-resolution microbial community profiling. Microbiome 6:17. doi: 10.1186/s40168-017-0396-x
Gao, Z., Yin, J., Zhang, J., Ward, R. E., Martin, R. J., Lefevre, M., et al. (2009). Butyrate improves insulin sensitivity and increases energy expenditure in mice. Diabetes 58, 1509–1517. doi: 10.2337/db08-1637
Geirnaert, A., Steyaert, A., Eeckhaut, V., Debruyne, B., Arends, J. B., Van Immerseel, F., et al. (2014). Butyricicoccus pullicaecorum, a butyrate producer with probiotic potential, is intrinsically tolerant to stomach and small intestine conditions. Anaerobe 30, 70–74. doi: 10.1016/j.anaerobe.2014.08.010
Gilbert, J. A., Meyer, F., Antonopoulos, D., Balaji, P., Brown, C. T., Brown, C. T., et al. (2010). Meeting report: the terabase metagenomics workshop and the vision of an earth microbiome project. Stand. Genomic. Sci. 3, 243–248. doi: 10.4056/sigs.1433550
Goncalves, P., Araujo, J. R., and Di Santo, J. P. (2018). A cross-talk between microbiota-derived short-chain fatty acids and the host mucosal immune system regulates intestinal homeostasis and inflammatory bowel disease. Inflamm. Bowel Dis. 24, 558–572. doi: 10.1093/ibd/izx029
Gordon, A., and Hannon, G. (2010). Fastx-Toolkit. FASTQ/A Short-Reads Pre-Processing Tools. Available at: http://hannonlab.cshl.edu/fastx_toolkit/ (accessed June 25, 2016).
Hong, S. W., Kim, K. S., and Surh, C. D. (2017). Beyond hygiene: commensal microbiota and allergic diseases. Immune Netw. 17, 48–59. doi: 10.4110/in.2017.17.1.48
Isomaa, B., Almgren, P., Tuomi, T., Forsen, B., Lahti, K., Nissen, M., et al. (2001). Cardiovascular morbidity and mortality associated with the metabolic syndrome. Diabetes Care 24, 683–689. doi: 10.2337/diacare.24.4.683
Jia, L., Li, D., Feng, N., Shamoon, M., Sun, Z., Ding, L., et al. (2017). Anti-diabetic effects of Clostridium butyricum CGMCC0313.1 through promoting the growth of gut butyrate-producing bacteria in Type 2 diabetic mice. Sci. Rep. 7:7046. doi: 10.1038/s41598-017-07335-0
Karkman, A., Lehtimaki, J., and Ruokolainen, L. (2017). The ecology of human microbiota: dynamics and diversity in health and disease. Ann. N. Y. Acad. Sci. 1399, 78–92. doi: 10.1111/nyas.13326
Kashiwagi, I., Morita, R., Schichita, T., Komai, K., Saeki, K., Matsumoto, M., et al. (2015). Smad2 and Smad3 inversely regulate TGF-beta autoinduction in Clostridium butyricum-activated dendritic cells. Immunity 43, 65–79. doi: 10.1016/j.immuni.2015.06.010
Khan, T. J., Ahmed, Y. M., Zamzami, M. A., Mohamed, S. A., Khan, I., Baothman, O. A. S., et al. (2018). Effect of atorvastatin on the gut microbiota of high fat diet-induced hypercholesterolemic rats. Sci. Rep. 8:662. doi: 10.1038/s41598-017-19013-2
Kootte, R. S., Levin, E., Salojarvi, J., Smits, L. P., Hartstra, A. V., Udayappan, S. D., et al. (2017). Improvement of insulin sensitivity after lean donor feces in metabolic syndrome is driven by baseline intestinal microbiota composition. Cell Metab. 2661:e616. doi: 10.1016/j.cmet.2017.09.008
Layton, A., Mckay, L., Williams, D., Garrett, V., Gentry, R., and Sayler, G. (2006). Development of Bacteroides 16S rRNA gene TaqMan-based real-time PCR assays for estimation of total, human, and bovine fecal pollution in water. Appl. Environ. Microbiol. 72, 4214–4224. doi: 10.1128/aem.01036-05
Lee, H., and Ko, G. (2014). Effect of metformin on metabolic improvement and gut microbiota. Appl. Environ. Microbiol. 80, 5935–5943. doi: 10.1128/AEM.01357-14
Lee, H., and Ko, G. (2016). Antiviral effect of vitamin A on norovirus infection via modulation of the gut microbiome. Sci. Rep. 6:25835. doi: 10.1038/srep25835
Lee, H., Lee, Y., Kim, J., An, J., Lee, S., Kong, H., et al. (2018). Modulation of the gut microbiota by metformin improves metabolic profiles in aged obese mice. Gut Microbes 9, 155–165. doi: 10.1080/19490976.2017.1405209
Lee, S. E., Han, K., Kang, Y. M., Kim, S. O., Cho, Y. K., Ko, K. S., et al. (2018). Trends in the prevalence of metabolic syndrome and its components in South Korea: findings from the Korean national health insurance service database (2009-2013). PLoS One 13:e0194490. doi: 10.1371/journal.pone.0194490
Lee, S., Lee, Y., Kim, J., An, J., Kim, K., Lee, H., et al. (2016). Atorvastatin and rosuvastatin improve physiological parameters and alleviate immune dysfunction in metabolic disorders. Biochem. Biophys. Res. Commun. 478, 1242–1247. doi: 10.1016/j.bbrc.2016.08.101
Letterio, J. J., and Roberts, A. B. (1998). Regulation of immune responses by TGF-beta. Annu. Rev. Immunol. 16, 137–161.
Ley, R. E., Turnbaugh, P. J., Klein, S., and Gordon, J. I. (2006). Microbial ecology: human gut microbes associated with obesity. Nature 444, 1022–1023.
Liu, Y., Song, X., Zhou, H., Zhou, X., Xia, Y., Dong, X., et al. (2018). Gut microbiome associates with lipid-lowering effect of rosuvastatin in vivo. Front. Microbiol. 9:530. doi: 10.3389/fmicb.2018.00530
Louis, S., Tappu, R. M., Damms-Machado, A., Huson, D. H., and Bischoff, S. C. (2016). Characterization of the gut microbial community of obese patients following a weight-loss intervention using whole metagenome shotgun sequencing. PLoS One 11:e0149564. doi: 10.1371/journal.pone.0149564
Loy, A., Pfann, C., Steinberger, M., Hanson, B., Herp, S., Brugiroux, S., et al. (2017). Lifestyle and horizontal gene transfer-mediated evolution of Mucispirillum schaedleri, a core member of the murine gut microbiota. mSystems 2:e00171-16. doi: 10.1128/mSystems.00171-16
Mardinoglu, A., Boren, J., and Smith, U. (2016). Confounding effects of metformin on the human gut microbiome in type 2 diabetes. Cell Metab. 23, 10–12. doi: 10.1016/j.cmet.2015.12.012
Markowiak, P., and Slizewska, K. (2017). Effects of probiotics, prebiotics, and synbiotics on human health. Nutrients 9:E1021. doi: 10.3390/nu9091021
Massague, J. (2012). TGFbeta signalling in context. Nat. Rev. Mol. Cell Biol. 13, 616–630. doi: 10.1038/nrm3434
Nolan, J. A., Skuse, P., Govindarajan, K., Patterson, E., Konstantinidou, N., Casey, P. G., et al. (2017). The influence of rosuvastatin on the gastrointestinal microbiota and host gene expression profiles. Am. J. Physiol. Gastrointest. Liver Physiol. 312, G488–G497. doi: 10.1152/ajpgi.00149.2016
Porreca, E., Di Febbo, C., Baccante, G., Di Nisio, M., and Cuccurullo, F. (2002). Increased transforming growth factor-beta(1) circulating levels and production in human monocytes after 3-hydroxy-3-methyl-glutaryl-coenzyme a reductase inhibition with pravastatin. J. Am. Coll. Cardiol. 39, 1752–1757. doi: 10.1016/s0735-1097(02)01857-0
Rintala, A., Pietila, S., Munukka, E., Eerola, E., Pursiheimo, J. P., Laiho, A., et al. (2017). Gut microbiota analysis results are highly dependent on the 16S rRNA gene target region, whereas the impact of DNA extraction is minor. J. Biomol. Tech. 28, 19–30. doi: 10.7171/jbt.17-2801-003
Rotter, V., Nagaev, I., and Smith, U. (2003). Interleukin-6 (IL-6) induces insulin resistance in 3T3-L1 adipocytes and is, like IL-8 and tumor necrosis factor-alpha, overexpressed in human fat cells from insulin-resistant subjects. J. Biol. Chem. 278, 45777–45784. doi: 10.1074/jbc.m301977200
Round, J. L., and Mazmanian, S. K. (2010). Inducible Foxp3+ regulatory T-cell development by a commensal bacterium of the intestinal microbiota. Proc. Natl. Acad. Sci. U.S.A. 107, 12204–12209. doi: 10.1073/pnas.0909122107
Safeer, R. S., and Lacivita, C. L. (2000). Choosing drug therapy for patients with hyperlipidemia. Am. Fam. Physician 61, 3371–3382.
Sanz, Y., Olivares, M., Moya-Perez, A., and Agostoni, C. (2015). Understanding the role of gut microbiome in metabolic disease risk. Pediatr. Res. 77, 236–244. doi: 10.1038/pr.2014.170
Segata, N., Izard, J., Waldron, L., Gevers, D., Miropolsky, L., Garrett, W. S., et al. (2011). Metagenomic biomarker discovery and explanation. Genome Biol. 12:R60. doi: 10.1186/gb-2011-12-6-r60
Shin, N. R., Lee, J. C., Lee, H. Y., Kim, M. S., Whon, T. W., Lee, M. S., et al. (2013). An increase in the Akkermansia spp. population induced by metformin treatment improves glucose homeostasis in diet-induced obese mice. Gut 63, 727–735. doi: 10.1136/gutjnl-2012-303839
Stancu, C., and Sima, A. (2001). Statins: mechanism of action and effects. J. Cell. Mol. Med. 5, 378–387. doi: 10.1111/j.1582-4934.2001.tb00172.x
Stone, N. J., Robinson, J. G., Lichtenstein, A. H., Bairey Merz, C. N., Blum, C. B., Eckel, R. H., et al. (2014). 2013 ACC/AHA guideline on the treatment of blood cholesterol to reduce atherosclerotic cardiovascular risk in adults: a report of the American college of cardiology/American heart association task force on practice guidelines. Circulation 129, S1–S45.
Talsness, C. E., Penders, J., Jansen, E., Damoiseaux, J., Thijs, C., and Mommers, M. (2017). Influence of vitamin D on key bacterial taxa in infant microbiota in the Koala birth cohort study. PLoS One 12:e0188011. doi: 10.1371/journal.pone.0188011
Wang, Y., Beydoun, M. A., Liang, L., Caballero, B., and Kumanyika, S. K. (2008). Will all Americans become overweight or obese? estimating the progression and cost of the US obesity epidemic. Obesity 16, 2323–2330.
Wilson, I. D., and Nicholson, J. K. (2009). The role of gut microbiota in drug response. Curr. Pharm. Des. 15, 1519–1523.
Winer, D. A., Luck, H., Tsai, S., and Winer, S. (2016). The intestinal immune system in obesity and insulin resistance. Cell Metab. 23, 413–426. doi: 10.1016/j.cmet.2016.01.003
Wu, H. J., and Wu, E. (2012). The role of gut microbiota in immune homeostasis and autoimmunity. Gut Microbes 3, 4–14.
Yang, J. Y., Lee, Y. S., Kim, Y., Lee, S. H., Ryu, S., Fukuda, S., et al. (2017). Gut commensal Bacteroides acidifaciens prevents obesity and improves insulin sensitivity in mice. Mucosal Immunol. 10, 104–116. doi: 10.1038/mi.2016.42
Keywords: atorvastatin, rosuvastatin, gut microbiota, IL-1β, TGFβ1, short-chain fatty acid
Citation: Kim J, Lee H, An J, Song Y, Lee C-K, Kim K and Kong H (2019) Alterations in Gut Microbiota by Statin Therapy and Possible Intermediate Effects on Hyperglycemia and Hyperlipidemia. Front. Microbiol. 10:1947. doi: 10.3389/fmicb.2019.01947
Received: 16 March 2019; Accepted: 08 August 2019;
Published: 04 September 2019.
Edited by:
Yuheng Luo, Sichuan Agricultural University, ChinaReviewed by:
Monica Di Paola, University of Florence, ItalyNatasa Golic, University of Belgrade, Serbia
Copyright © 2019 Kim, Lee, An, Song, Lee, Kim and Kong. This is an open-access article distributed under the terms of the Creative Commons Attribution License (CC BY). The use, distribution or reproduction in other forums is permitted, provided the original author(s) and the copyright owner(s) are credited and that the original publication in this journal is cited, in accordance with accepted academic practice. No use, distribution or reproduction is permitted which does not comply with these terms.
*Correspondence: Hyunseok Kong, aHNrb25nMDgxM0BnbWFpbC5jb20=
†These authors have contributed equally to this work